
An official website of the United States government
The .gov means it’s official. Federal government websites often end in .gov or .mil. Before sharing sensitive information, make sure you’re on a federal government site.
The site is secure. The https:// ensures that you are connecting to the official website and that any information you provide is encrypted and transmitted securely.
- Publications
- Account settings
Preview improvements coming to the PMC website in October 2024. Learn More or Try it out now .
- Advanced Search
- Journal List
- Biomed Res Int

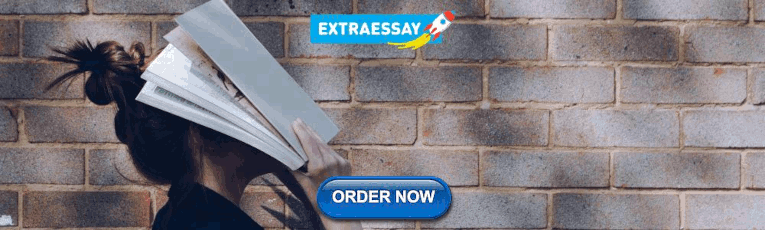
Privacy Protection and Secondary Use of Health Data: Strategies and Methods
Dingyi xiang.
1 Internet Rule of Law Institute, East China University of Political Science and Law, Shanghai, China
2 Humanities and Law School, Northeast Forest University, Harbin, Heilongjiang, China
3 Beidahuang Information Company, Harbin, Heilongjiang, China
Health big data has already been the most important big data for its serious privacy disclosure concerns and huge potential value of secondary use. Measurements must be taken to balance and compromise both the two serious challenges. One holistic solution or strategy is regarded as the preferred direction, by which the risk of reidentification from records should be kept as low as possible and data be shared with the principle of minimum necessary. In this article, we present a comprehensive review about privacy protection of health data from four aspects: health data, related regulations, three strategies for data sharing, and three types of methods with progressive levels. Finally, we summarize this review and identify future research directions.
1. Introduction
The rapid development and application of multiple health information technologies enabled medical organizations to store, share, and analyze a large amount of personal medical/health and biomedical data, of which the majority are electronic health records (EHR) and genomic data. Meanwhile, the emerging technologies, such as smart phones and wearable devices, also enabled third-party firms to provide many kinds of complementary mHealth services and collect huge tons of consumer health data. Health big data has already been the most important big data for its serious privacy disclosure concerns and huge potential value of secondary use.
Health big data stimulated the development of personalized medicine or precision medicine. Empowered by health informatics and analytic techniques, secondary use of health data can support clinical decision making; extract knowledge about diseases, genetics, and medicine; improve patients' healthcare experiences; reduce healthcare costs; and support public health policies [ 1 – 3 ]. On the other side of the coin, health data contains much personal privacy and confidential information. For the guidance of protecting health-related privacy, the Health Insurance Portability and Accountability Act (HIPAA) of the US specifies 18 categories of protected health information (PHI) [ 4 ]. The heavy concerns about privacy disclosure much hinder secondary use of health big data. Much efforts tried to balance between privacy management and health data secondary use from both the legislation side [ 5 ] and the technology side [ 6 , 7 ]. But for much more circumstances, a perfect balance is difficult to achieve; instead, a certain tradeoff or compromise must always be made. Recently, COVID-19 may perfectly illustrate the conundrum between protecting health information and ensuring its availability to meet the challenges posed by a significant global pandemic. In this ongoing battle, China and South Korea have mandated public use of contact tracing technologies, with few privacy controls; other countries are also adopting contact tracing technologies [ 7 ].
The direct and also important strategy to balance both issues is reusing health data under the premise of protecting privacy. The most primary idea is to share deidentified health data by removing 18 specified PHI. Based on deidentified health data, machine learning and data mining can be used for knowledge extraction or learning health system building for the purpose of analyzing and improving care, whereby treatment is tailored to the clinical or genetic features of the patient [ 8 ]. However, transforming data or anonymizing individuals may minimize the utility of the transferred data and lead to inaccurate knowledge [ 9 ]. This tradeoff between privacy and utility, also accuracy, is the center issue of sensitive data secondary usage [ 10 ]. Deidentification refers to a collection of techniques devised for removing or transforming identifiable information into nonidentifiable information and also introducing random noise into the dataset. By deidentification, privacy protection will be leveraged, but the outcome of analysis may be not exact, rather an approximation. To reconcile this conflict, the privacy loss parameter, also called privacy budget, was proposed to tune the tradeoff between privacy and accuracy: by changing the value of this parameter, more or less privacy resulting in less or more accuracy, respectively [ 11 ]. Furthermore, deidentified data may become reidentifiable through data triangulation from other datasets, which means that the privacy harms of big health data arise not merely in the collection of data but in their eventual use [ 12 ]. Just deidentification is far from needed. Instead, a holistic solution is the right direction, by which the risk of reidentification from records should be kept as low as possible and data be shared with the principle of minimum necessary [ 13 ]. For the minimum necessary, user-controlled access [ 6 , 14 ] and secure network architecture [ 15 ] can be a practical implementation. For effective reusing health data while reducing the risk of reidentification, attempts in three aspects can be applicable references, that is, risk-mitigation methods, privacy-preserving data mining, and distributed data mining without sharing out data.
The remainder of this paper is organized as follows. Section 2 describes the scope of health data and its corresponding category. Section 3 summarizes regulations about privacy protection of health data in several countries. Section 4 concisely reviews two strategies for privacy protection and secondary use of health data. Section 5 reviews three aspects of tasks and methods for privacy preservation and data mining the primary tasks of data mining. Section 6 concludes this study.
2. Health Data and Its Category
Generally speaking, any data associated with users' health conditions can be viewed as health data. The most important health data is clinical data, especially electronic medical records (EMR), produced by different level hospitals. With the development of health information technology and the popularization of wearable health device, vast amounts of health-relevant data, such as monitored physiological data and diet or exercise data, are collected from individuals and entities elsewhere, both passively and actively. According to the review article by Deven McGraw and Kenneth D. Mandl, health-relevant data can be classified into four categories [ 7 ]. In this research, we focus on the first two categories of data, which are directly related to users' health and privacy.
Category 1. Health data generated by healthcare system. This type of data is clinical data and is recorded by clinical professionals or medical equipment when a patient gets healthcare service in a hospital or clinic. Clinical data includes EMR, prescriptions, laboratory data, pathology images, radiography, and payor claims data. Patients' historical condition and current condition are recorded for treatment requirement. For making better health service for patients, it is important to track patients' lifelong clinical data and make clinical data sharing among different healthcare providers. Personal health record (PHR) was proposed to integrate patients' cross-institutions and lifelong clinical data [ 16 ]. This type of health data is generated and collected routinely in the process of healthcare, with the explicit aim that those data be used for the purpose of analyzing and improving care. For the purpose of clinical treatment, and also because of consumers' firm trust on healthcare experts and institutions, clinical data contains a high degree of health-related privacy. Therefore, the majority of health privacy laws mainly cover the privacy protection of clinical data [ 7 ]. Under the constraints of health privacy laws, tons of clinical data have been restricted only for internal use in medical institutions. Meanwhile, the clinical data is also extremely valuable for secondary usage since the data is created by professional experts and is direct description of consumers' health conditions. The tradeoff between utility and privacy of this type of health data has been one of the most important issues in the age of medical big data.
Category 2. Health data generated by consumer health and wellness industry. This type of health data is an important complementation to clinical data. With the widespread application of new-generation information technology, such as IoT, mHealth, smart phone, and wearable device, consumers' health attitude has greatly changed from passive treatment to active health. Consumers' health data can be generated through wearable fitness tracking devices, medical wearables such as insulin pumps and pacemakers, medical or health monitoring apps, and online health service. These health data can include breath, heart rate, blood pressure, blood glucose, walking, weight, diet preference, position, and online health consultation. These products or services and health data play important role in consumers' daily heath management, especially for chronic disease patients. This area has gained more and more focus from industry and academia. Consumer health informatics is the representative direction [ 17 ]. This type of nontraditional health-relevant data, often equally revealing of health status, is in widespread commercial use and, in the hands of commercial companies, yet often less accessible by providers, patients, and public health for improving individual and population health [ 18 ]. These big health data are scattered across institutions and intentionally isolated to protect patient privacy. For this type of health data, integration and linking at individual level are an extra challenge except for the utility-privacy tradeoff.
Table 1 summarizes the two categories of health data and their comparative features.
Summarization of clinical data and consumer health data.
3. Regulations about Privacy Protection of Health Data
Personal information and health-relevant data are necessary to record in order to provide regular health service. Meanwhile, personal information and health-relevant data are closely associated with user privacy and confidential information. Therefore, several important privacy protection-related regulations or acts are published to guide health data protection and reuse. Modern data protection law is built on “fair information practice principles” (FIPPS) [ 19 ].
The most referenced regulation is Health Insurance Portability and Accountability Act (HIPAA) [ 4 ]. HIPAA was created primarily to modernize the flow of healthcare information, stipulate how personally identifiable information maintained by the healthcare and healthcare insurance industries should be protected from fraud and theft, and address limitations on healthcare insurance coverage. The HIPAA Safe Harbor (SH) rule specifies 18 categories of explicitly or potentially identifying attributes, called protected health information (PHI), that must be removed before the health data is released to a third party. HIPAA also covers electronic PHI, ePHI. This includes medical scans and electronic health records. A full list of PHI elements is provided in Table 2 . PHI elements in Table 2 only cover identity information and do not include any sensitive attribute. That is, HIPAA does not provide guidelines on how to protect sensitive attribute data; instead, the basic idea of the HIPAA SH rule is to protect privacy by preventing identity disclosure. However, other sensitive attributes may still uniquely combine into a quasi-identifier (QI), which can allow data recipients to reidentify individuals to whom the data refer. Therefore, a strict implementation of the SH rule, however, may be inadequate for protecting privacy or preserving data quality. Recognizing this limitation, HIPAA also provides alternative guidelines that enable a statistical assessment of privacy disclosure risk to determine if the data are appropriate for release [ 20 ].
Protected health information defined by HIPAA.
The Health Information Technology for Economic and Clinical Health (HITECH) Act [ 21 ] was enacted as part of the American Recovery and Reinvestment Act of 2009 to promote the adoption and meaningful use of health information technology. Subtitle D of the HITECH Act addresses the privacy and security concerns associated with the electronic transmission of health information, in part, through several provisions that strengthen the civil and criminal enforcement of the HIPAA rules. It is complimentary with HIPAA and strengthens HIPAA's privacy regulations. HITECH has also widened the scope of HIPAA through the Omnibus Rule. This extends the privacy and security reach of HIPAA/HITECH to business associates. According to HIPAA and HITECH Act, much of data beyond category 1 in Table 1 is outside of the scope of comprehensive health privacy laws in the U.S.
The Consumer Data Right (CDR) [ 22 ] is coregulated by the Office of the Australian Information Commissioner (OIAC) and Australian Competition and Consumer Commission (ACCC). “My Health Record System” is run to track citizen medical conditions, test results, and so on. The OIAC sets out controls on how health information in a My Health Record can be collected, used, and disclosed, which corresponds to PHR integration. The Personal Information Protection and Electronic Documents Act (PIPEDA) [ 23 ] of Canada applies to all personal health data. PIPEDA is stringent and although has many commonalities with HIPAA; it goes beyond HIPAA requirements in several areas. One such area is in the protection of data generated by mobile health apps which is not strictly covered by HIPAA. PIPEDA runs to protected consumer health data. Under PIPEDA, organizations can seek implied or explicit consent, which is based on the sensitivity of the personal information collected and the reasonable data processing consent expectations of the data subject. The General Data Protection Regulation (GDPR) is a wide-ranging data protection regulation in EU, which covering health data as well as all other personal data, even they contain sensitive attributes. GDPR also has data consent and breach notification expectations and contains several key provisions, including notification, right to access, right to be forgotten, and portability. Under GDPR, organizations are required to gain explicit consent from data subjects, and individuals have the right to restriction of processing and not to be subject to automated decision-making.
China has no specific regulations for health data privacy protection. Several restriction rules to prohibit privacy disclosure scatter in China Civil Code (CCC), Medical Practitioners Act of the PRC (MPAPRC), and Regulations on Medical Records Management in Medical Institutions (RMRMMMI), which make privacy disclosure restrictions to individuals, medical practitioners, and medical institutions, respectively. CCC specifies 9 categories of personal information to be protected, including name, birthday, ID number, biometric information, living address, phone number, email address, health condition information, and position tracking information. RMRMMMI only approves reuse of health data just for medical care, teaching, and academic research. Recently, the Personal Information Protection Law of the PRC (PIPILRC) [ 24 ] is released and will come into force on November 1, 2021. This is the first complete and comprehensive regulation on personal information protection. In this regulation, the definition of sensitive personal information and automatic decision making both involve health data, so, this regulation is applicable to privacy protection of health data. According to this regulation, secondary use of deidentified or anonymized health data for automatic decision making is permitted, and data processing consent from consumers is also required. This regulation, so far as can be foreseen, will greatly stimulate the exploitation and exploration of health big data.
According to the comparison of these data privacy relevant regulations, shown in Table 3 , PIPEDA and GDPR and the newly released PIPILRC can cover both clinical data and consumer health data, and others pay the majority of attention to clinical data. Health data need to be reused for multiple important purposes. In fact, health data processing and reusing are never absolutely prohibited in the regulations mentioned above, as long as privacy protection is achieved as the important prerequisite. In this respect, HIPAA sets Safe Harbor rules to make sure PHI be removed before the health data is released to a third party. Furthermore, PIPEDA and GDPR require consumers' consent for data processing. Regulations from China also encourage health data to be reused in certain restricted areas. As the newcomer, PIPILRC presents a more complete and comprehensive guidance to protect and process health data.
Regulations and corresponding data category.
4. Strategies and Framework
The exploitation of health data can provide tremendous benefits for clinical research, but methods to protect patient privacy while using these data have many challenges. Some of these challenges arise from a misunderstanding that the problem should be solved by a foolproof solution. There exists a paradox: well deidentified and scrubbed data may lose much meaningful information results in low quality, maintaining much PHI may have high risk of privacy breach. Therefore, a holistic solution, or to say a unified strategy, is needed. Three strategies are summarized in this section. The first is for clinical data and provides a practical user access rating system, and the second is majority for genomic data and designs a network architecture to address both security access and potential risk of privacy disclosure and reidentification. From a more practical starting point, the third tries to share a model without exposing any data. The tree strategies present solutions from different perspectives, therefore can be complementary to each other.
4.1. Strategies for Clinical Data
As for clinical data, Murphy et al. proposed an effective strategy to build a clinical data sharing platform while protecting patient privacy [ 6 ]. The proposed approach to resolving the balance between privacy management and data secondary use is to match the level of data deidentification with the trustworthiness of the data recipients, in which the more identified the data, the more “trustworthy” the recipients are required to be, and vice versa. The level of trust for a data recipient becomes a critical factor in determining what data may be seen by that person. This type of hierarchical access rating is similar to the film rating, which can accommodate the requirement and appetites of different types of audiences. Murphy et al.'s strategy sets up five patient privacy levels with three aspects of requirements: availability of the data, trust in the researcher and the research, and the security of the technical platforms. Corresponding to the privacy levels are five user role levels.
The lowest level of user is “obfuscated data user.” For this user, data are obfuscated as it is served to a client machine with possibly low technical security. Obfuscation methods try to add a random number to the aggregated counts instead of providing accurate result [ 25 , 26 ]. The second level of user is “aggregated data user,” to whom exact numbers from aggregate query results are permissible. The third is “LDS data user,” who is granted to access HIPAA-defined LDS (limited dataset) and structured patient data in which PHI must be removed. The fourth is “Notes-enabled LDS data user,” who is additionally allowed to view PHI scrubbed text notes (such as discharge summary). The final level of user is “PHI-viewable data user,” who has access to all patient data.
These access level categories are summarized in Table 4 .
Health data access level categories.
With the guidance of health data access level categories, Murphy et al. implemented five cases in clinical research. In a realistic project, multiple use role or different access privileges must be needed to reconcile different data access requirements. Murphy et al. also provided three exemplar projects and their possible privacy level user distributions. This proposed strategy gave a complete reference for data sensitive project and also implemented a holistic approach to patient privacy solutions in Informatics for Integrating Biology and the Bedside (i2b2) research framework [ 27 ]. The i2b2 framework is the most widespread open-source framework for exploring clinical research data-warehouses and was jointly developed by the Harvard Medical School and Massachusetts Institute of Technology to enable clinical researchers to use existing deidentified clinical data and only IRB-approved genomic data for research aims. Yet, i2b2 does not provide any specific protection mechanism for genomic data.
4.2. Strategies for Genomic Data
As for genomic data, two potential privacy threats are loss of patients' health data confidentiality due to illegitimate data access and patients' reidentification and resulting sensitive attribute disclosure from legitimate data access. On the basis of the i2b2 framework, Raisaro et al. [ 15 ] proposed to apply homomorphic encryption [ 28 ] to the first threat and differential privacy [ 29 ] to the second threat. Furthermore, Raisaro et al. designed a system model, consisting of two physically separated networks, from the perspective of architecture. The network architecture is shown in Figure 1 . This network architecture is aimed at isolating data that is used for clinical/medical care and that is used for research activities by a few trusted and authorized individuals.

Network architecture of privacy protection for health data including genomic data.
The clinical network is used for hospital's clinical daily activities, containing clinical and genomic data of patients. This network is very controlled and protected by a firewall that blocks all incoming network traffic. Authorized users are permitted to log in.
The research network hosts i2b2 service used by researchers in their research activities. The i2b2 service is composed of an i2b2 server and a proxy server, in which a homomorphic encryption method and a differential privacy method are implemented and deployed. The i2b2 server can receive deidentified clinical data and encrypted genomic data from the clinical network and perform security data query and computation. The proxy server is devoted to support the decryption phase and the storage of partial decryption keys for homomorphic encryption. Through the research network, researchers can get authorized data via query execution module by the sequential five steps: query generation, query processing, result perturbation, result partial decryption, and result decryption at the final user-client side.
This network architecture and its privacy-preserving solution have been successfully deployed and tested in Lausanne University Hospital and used for exploring genomic cohorts in a real operational scenario. This application is also a practicable demonstration for similar scenario. It is not a unique instance but has its counterpart. Azencott reviewed how breaches in patient privacy can occur, and recent developments in computational data protection also proposed a similar secure framework for genomic data sharing around three aspects, which includes algorithmic solutions to deidentification, database security, and user trustworthy access [ 3 ].
4.3. Strategies for Sharing Not Data but Models
Since the new paradigm of the machine learning method, namely, federated learning (FL), was first introduced in 2016 [ 30 ], has achieved a rapid development, and become a hot research topic in the field of artificial intelligence, its core idea is to train machine learning models on separate datasets that are distributed across different devices or parties, which can preserve the local data privacy to a certain extent. This development mainly benefits from the following three facts [ 31 ]: (1) the wide successful applications of machine learning technologies, (2) the explosive growth of big data, and (3) the legal regulations for data privacy protection worldwide.
The idea of federated learning is to only share the model parameters instead of the original data. By this way, many of these initiatives are based on federated models in which the actual data never leave the institution of origin, allowing researchers to share models without necessarily sharing patient data. Federated learning has inspired another important strategy to develop smart healthcare based on sensitive and private medical records which exist in isolated medical centers and hospitals. As shown in Figure 2 , federated learning offers a framework to jointly train a global model using datasets stored in separate clients.

Architecture for a federated learning system.
Model building of this kind has been used in real-world applications where user privacy is crucial, e.g., for hospital data or text predictions on mobile devices, and it has been stated that model updates are considered to contain less information than the original data, and through the aggregation of updates from multiple data points, original data is considered impossible to recover. Federated learning emphasizes the data privacy protection of the data owner during the model training process. Effective measures to protect data privacy can better cope with the increasingly stringent data privacy and data security regulatory environment in the future [ 32 ].
5. Tasks and Methods
Under the strategies of health data protection, specific tasks and methods about privacy and data processing can be employed and deployed. The tasks and methods can be viewed at three progressive levels. Methods in the first level are aimed at mitigating the risk of privacy disclosure, from four aspects. Methods in the second level target on data mining or knowledge extraction from deidentified or anonymized health data. No need to share health data, methods in the third level try to build a learning model or extract knowledge in a distributed manner, then share the model or knowledge.
5.1. Risk-Mitigation Methods
There are two widely recognized types of privacy disclosure [ 33 ]: identity disclosure (or reidentification) and attribute disclosure. The former occurs when illegitimate data users try to match a record in a dataset to an individual, and the latter occurs when illegitimate data users try to predict the sensitive value(s) of an individual record. According to Malin et al. [ 34 ], methods of mitigating the risk of two types of privacy disclosure can be divided into four classes: suppression, generalization, randomization, and synthetization. This perspective of method categories expects to well summarize the recent research on risk-mitigation methods.
5.1.1. Suppression Methods
Suppression methods are aimed at scrubbing (remove or mask) 18 PHI defined in HIPAA, which is the most important deidentification method. Before PHI scrubbing, the major task is to identify the PHI from health data. For structural data, PHI identification can be done easily according to data schema. For narrative data or free text, such as discharge summary or progress note, natural language processing (NLP) is the preferred technology for PHI identification. Specifically, named entity recognition (NER) is the mainstream technology used in clinical data for deidentification and medical knowledge extraction. The 18 PHI are regarded as predefined entity types, and machine learning is employed to annotate type tags for each word in a sentence, then those tags are merged, and finally, the position and type of PHI can be identified. Conditional random fields (CRFs) are the classic sequential tagging model for NER and are often applied for deidentification [ 35 ]. Meystre et al. made a systematic review of deidentification methods [ 36 ], and Uzuner et al. [ 37 ] and Deleger et al. [ 38 ] both conducted some evaluations on a certain human-annotated dataset. The identified PHI values are then simply removed from or replaced with a constant value in the released text documents, which may be inadequate for protecting privacy or preserving data quality. Li and Qin proposed a new systematic approach to integrate methods developed in both data privacy and health informatics fields. The key novel elements of the proposed approach include a recursive partitioning method to cluster medical text records and a value enumeration method to anonymize potentially identifying information in the text data, which essentially masks the original values, to improve privacy protection and data utility [ 20 ].
For genomic data, homomorphic encryption [ 28 ] is applied to encrypting genomic data, and then, encrypted data can be shared for secondary use. Raisaro et al. employed homomorphic encryption to build a data warehouse for genomic data [ 15 ]. Kamm et al. [ 39 ] also proposed a framework for generating aggregated statistics on genomic data by using secure multiparty computation based on homomorphic secret sharing. Several other works [ 28 , 40 , 41 ] proposed using homomorphic encryption to protect genomic information in order to allow researchers to perform some statistics directly on the encrypted data and decrypt only the final result.
5.1.2. Generalization Methods
These methods transform data into more abstract representations. The much easier implementation is abbreviation. For instance, the age of a patient may be generalized from 1-year to 5-year age groups. Based on this type of generation, sensitive attributes can be generalized subgroup and be anonymized to some extent, which is the back idea of k -anonymity and its variations. k -anonymity seeks to prevent reidentification by stripping enough information from the released data that any individual record becomes indistinguishable from at least ( k − 1) other records [ 42 ]. The idea of k -anonymity is based on modifying the values of the QI attributes to make it difficult for an attacker to unravel the identity of persons in a particular dataset while the released data remain as useful as possible. This modification is a sort of generalization, by which stored values can be replaced with semantically consistent but less precise alternatives [ 43 ]. For example, let us consider a dataset in which age is a quasi-identifier. While the three records {age = 30, gender = male}, {age = 35, gender = male}, and {age = 31, gender = female} are all distinct, releasing them as {age = 3∗, gender = male}, {age = 3∗, gender = male}, and {age = 3∗, gender = female} ensures they all belong to the same age category and the anonymity is 3-anonymity. Based on k -anonymity, l -diversity [ 44 , 45 ] were proposed to address further disclosure issues of sensitive attributes.
5.1.3. Randomization Methods
Randomization can be used for attribute-level data. In this case, original sensitive values are replaced with similar but different values, with a certain probability. For example, a patient's name may be masked by a randomly selected made-up name. This basic approach may result in worse data quality. Li and Qin proposed to obtain value via a clustering method [ 20 ].
Randomization can further be used for aggregation operation. Obfuscation is a sort of such randomization. Numerous repetitions of a query by a single user must be detected and interrupted because they will converge on the true patient count making proper user identification absolutely necessary for the methods to function properly [ 6 ]. Aiming to deidentify aggregated data, obfuscation methods include the addition of a random number to the patient counts that has a distribution defined by a Gaussian function.18. Obfuscation is applied to aggregate patient counts that are reported as a result of ad hoc queries on the client machine [ 26 ]. Another protection model for preventing reidentification is differential privacy [ 10 , 46 ]. In this model, reidentification is prevented by the addition of noise to the data. The model is based on the fact that auxiliary information will always make it easier to identify an individual in a dataset, even if anonymized. Instead, differential privacy seeks to guarantee that the information that is released when querying a dataset is nearly the same whether a specific person is included or not [ 46 ]. Unlike other methods, differential privacy provides formal statistical privacy guarantees.
5.1.4. Synthetization Methods
Synthetization is compelling for two main reasons: preserving confidentiality and valid inferences for various estimates [ 47 ]. In this case, the original data are never shared. Instead, general aggregate statistics about the data are computed, and new synthetic records are generated from the statistics to create fake, but realistic-like, data. Exploiting clinical data for building an intelligent system is one of the scenarios. Developing clinical natural language processing systems often requires access to many clinical documents, which are not widely available to the public due to privacy and security concerns. To address this challenge, Li et al. proposed to develop methods to generate synthetic clinical notes and evaluate their utility in real clinical natural language processing tasks. Thanks to the development of deep learning, recent advances in text generation have made it possible to generate synthetic clinical notes that could be useful for training NER models for information extraction from natural clinical notes, thus lowering the privacy concern and increasing data availability [ 48 ].
5.2. Privacy-Preserving Data Mining
Data mining is also synonymously called knowledge discovery from data (KDD), which highlights the goal of the mining process. To obtain useful knowledge from data, the mining process can be divided into four iterative steps: data preprocessing, data transformation, data mining, and pattern evaluation and presentation. Based on the stage division in the process of KDD, Xu et al. developed a user-role-based methodology and identified four different types of users in a typical data mining scenario: data provider, data collector, data miner, and decision maker. By differentiating the four different user roles, privacy-preserving data mining (PPDM) can be explored in a principled way, by which all users care about the security of sensitive information but each user role views the security issue from its own perspective [ 49 ]. In this research, PPDM is explored from the view of a data miner role, that is, from the data mining stage of KDD.
Privacy-preserving data mining is aimed at mining or extracting information, via a certain machine learning-based model, from privacy-preserving data in which the values of individual records have been perturbed or masked [ 50 ]. The key challenge is that the privacy-preserving data look very different from the original records and the distribution of data values is also very different from the original distribution. Researches for this issue have started very early. Agrawal and Srikant proposed a reconstruction procedure to estimate the distribution of original data values and then built a decision-tree classifier [ 50 ]. Recent studies on PPDM include privacy-preserving association rule mining, privacy-preserving classification, and privacy-preserving cluster.
Association rule mining is aimed at finding interesting associations and correlation relationships among large sets of data items. For PPDM, some of the rules may be considered to be sensitive. For hiding these rules, the original data need to be modified to generate a sanitized dataset from which sensitive rules cannot be mined, while those nonsensitive ones can still be discovered [ 51 ]. Classification is a task of data analysis that learns models to automatically classify data into defined categories. Privacy-preserving classification evolves decision tree, Bayesian model, support vector machine, and neural classification. The strategies of adapting the classification method to a privacy-preserving scenario can simply be described as two aspects. The first is learning the classification model based on data transformation, since the transformed data is difficult to be recovered [ 52 , 53 ]. The second is learning the classification model based on secure multiparty computation (SMC) [ 54 ], where multiparties collaborate to develop a classification model from vertically partitioned or horizontally partitioned data, but no one wants to disclose its data to others [ 55 , 56 ]. Cluster analysis is the process of grouping a set of records into multiple groups or clusters so that objects within a cluster have high similarity but are very dissimilar to objects in other clusters. This process runs in an unsupervised manner. Similar to classification, current researches on privacy-preserving clustering can be roughly categorized into two types, based on data transformation [ 57 , 58 ] and based on secure multiparty computation [ 59 , 60 ].
5.3. Federated Privacy-Preserving Data Mining
For the distributed or isolated data, distributed data mining is the research topic. Distributed data mining can be further categorized into data mining over horizontally partitioned data and data mining over vertically partitioned data. Research on distributed data mining attracts much attention. To overcome the difficulty of data integration and promote efficient information exchange without sharing sensitive raw data, Que et al. developed a Distributed Privacy-Preserving Support Vector Machine (DPP-SVM). The DPP-SVM enables privacy-preserving collaborative learning, in which a trusted server integrates “privacy-insensitive” intermediary results [ 61 ]. In medical domain, much raw data can hardly leave the institution of origin. Instead of bringing data to a central repository for computation, Wu et al. proposed a new algorithm, Grid Binary LOgistic REgression (GLORE), to fit a LR model in a distributed fashion using information from locally hosted databases containing different observations that share the same attributes [ 62 ].
It is worth to note that learning (classification or clustering) on secure multiparty computation is an important distributed learning strategy, by which privacy disclosure concern can be much reduced since data need not to be shared out. This research topic probably inspired federated machine learning [ 30 , 32 ]. Today's AI still faces two major challenges. One is that data exists in the form of isolated islands. The other is the strengthening of data privacy and security. The two challenge is much severer in the healthcare domain. Federated machine learning is aimed at building a learning model from decentralized data [ 30 ]. Federated learning can be classified into horizontally federated learning, vertically federated learning, and federated transfer learning based on how data is distributed among various parties in the feature and sample ID space [ 32 ]. Horizontal federated learning, or sample-based federated learning, is introduced in the scenarios that datasets share the same feature space but different in samples. At the end of the learning, the universal model and the entire model parameters are exposed to all participants. Vertical federated learning or feature-based federated learning is applicable to the cases that two datasets share the same sample ID space but differ in feature space. At the end of learning, each party only holds the model parameters associated with its own features; therefore, at inference time, the two parties also need to collaborate to generate output. Federated transfer learning (FTL) applies to the scenarios that the two datasets differ not only in samples but also in feature space. FTL is an important extension to the existing federated learning systems and is more similar to vertical federated learning. The challenge of protecting data privacy while maintaining the data utility through machine learning still remains. For a comprehensive introduction of federated privacy-preserving data mining, please refer to the survey based on the proposed 5 W-scenario-based taxonomy [ 31 ].
5.4. Summary: Privacy vs. Accuracy
Privacy protection is the indispensable prerequisite of secondary usage of health data. As discussed above, risk-mitigation methods are aimed at anonymizing private or sensitive information so as to reduce the risk of reidentification. Methods about privacy-preserving data mining target to process the privacy-scrubbed data and extract knowledge and even build AI systems. If absolute privacy safe is pursued, the scrubbed data is definitely useless, since the data quality is severely corrupted. With the poor-quality data, accuracy and effectiveness of data utilization are extremely affected. Therefore, in a practical scenario, a certain tradeoff or compromise between privacy and accuracy must always be made. The tradeoff can be tuned to provide more or less privacy resulting in less or more accuracy, respectively, according to the requirements of privacy level and utility level. Federated privacy-preserving data mining sheds light on the new direction to compromise, even to balance, the privacy and accuracy. No need to share data out, federated privacy-preserving data mining first processes the original health data within institutions, and the conduct federated mining or learning. This type of method is expected to reconcile privacy and accuracy with more elegant style and more acceptable way.
6. Conclusions
Clinical data, genomic data, and consumer health data are the majority of health big data. Protection and reuse always gain much focused research topics. In this review article, the type and scope of health data are firstly discussed, followed by the related regulations for privacy protection. Then, strategies for user-controlled access and secure network architecture are presented. Sharing trained model without original data leaving out is a new important strategy and gains more and more focus. According to different data reuse scenarios, tasks and methods at three different levels are summarized. The strategies and methods can be combined to form a holistic solution.
With the rapid develop health information technology and artificial intelligence, the capability of privacy protection will impede the urgent demand of reusing health data. Some potential research directions may include (1) applying modern machine learning to deidentification and anonymization for multimodal health data while ensuring its data quality; (2) learning model construction and knowledge extraction based on anonymized data to leverage secondary use of health data; (3) federated learning on isolated heath data can both protect privacy perfectly and improve the efficiency of data transferring and processing, being deserved more attention; (4) research on alleviating reidentification risk, such as linkage or inference, from a trained model.
Acknowledgments
This study was funded by the China Postdoctoral Science Foundation Grant (2020M671059) and the Fundamental Research Funds for the Central Universities (2572020BN02).
Conflicts of Interest
The authors declare that they have no conflicts of interest.
- Search Menu
- Accident and Trauma
- Anaesthesia
- Cardiothoracic Surgery
- Cardiovascular Disease
- Child and Adolescent Psychiatry
- Critical Care/Intensive Care/Emergency Medicine
- Dermatology
- Endocrinology
- Environment and Disease
- Gastroenterology
- General Practice
- Geriatric Medicine
- Haematology
- Health Policy
- Health Economics
- Infectious Diseases
- Liver Disease
- Neonate Medicine
- Neurophysiology
- Neurosurgery
- Obstetrics and Gynaecology
- Occupational Medicine
- Ophthalmology
- Otolaryngology
- Paediatric Medicine & Surgery
- Palliative Medicine
- Perioperative Medicine
- Public Health Medicine
- Renal Medicine
- Respiratory Medicine
- Rheumatology
- Sports Medicine
- Transplantation
- Tropical Medicines
- Advance articles
- Editor's Choice
- Author Guidelines
- Submission Site
- Open Access
- About British Medical Bulletin
- Editorial Board
- Advertising and Corporate Services
- Journals Career Network
- Self-Archiving Policy
- Dispatch Dates
- Journals on Oxford Academic
- Books on Oxford Academic

Article Contents
Introduction, the gdpr principles, lawful bases for processing, data subjects' rights, the relationship between gdpr lawful basis and other consents required in research.
- < Previous
The impact of the General Data Protection Regulation on health research
- Article contents
- Figures & tables
- Supplementary Data
Victoria Chico, The impact of the General Data Protection Regulation on health research, British Medical Bulletin , Volume 128, Issue 1, December 2018, Pages 109–118, https://doi.org/10.1093/bmb/ldy038
- Permissions Icon Permissions
On the May 25, 2018 the General Data Protection Regulation (hereafter the GDPR or the Regulation) came into force, replacing the Data Protection Directive 95/46/EC (upon which the Data Protection Act 1998 is based), and imposing new responsibilities on organizations which process the data of European Union citizens.
This piece examines the impact of the Regulation on health research.
The Regulation seeks to harmonize data privacy laws across Europe, to protect and empower all EU citizen’s data privacy and to reshape the way that organizations approach data privacy (See the GDPR portal at: https://www.eugdpr.org/ (accessed 8 May 2018). As a Regulation the GDPR is directly applicable in all member states as opposed to a directive which requires national implementing measures (In the UK the Data Protection Act 1998 was the implementing legislation for the Data Protection Directive 95/46/EC.).
The Regulation is sector wide, but its impact on organizations us sector specific. In some sectors, the Regulation inhibits the processing of personal data, whilst in others it enables that processing. The Regulation takes the position that the ‘processing of data should be designed to serve mankind’ (Recital 4). Whilst it does not spell out what exactly is meant by this, it indicates that a proportionate approach will be taken to the protection of personal data, where that data can be processed for common goods such as healthcare. Thus, the protection of personal data is not absolute, but considered in relation to its function in society and balance with other fundamental rights in accordance with the principle of proportionality (Recital 4). Differing interpretations of proportionality can detract from the harmonization objective of the Regulation.
Reflecting the commitment to proportionality, scientific research holds a privileged position in the Regulation. Throughout the Regulation provision is made for organizations that process personal data for scientific research purposes to avoid restrictive measures which might impede the increase of knowledge. However, the application of the Regulation differs across health research sectors and across jurisdictions. Transparency and engagement across the health research sector is required to promote alignment.
Research which focuses on the particular problems which arise in the context of the regulation’s application to health research would be welcome. Particularly in the context of the operation of the Regulation alongside the duty of confidentiality and the variation in approaches across Member States.
The General Data Protection Regulation (hereafter the GDPR or the Regulation) is an extensive piece of legislation which spans sectors. Any consideration of its impact needs to be sector specific to have relevance to particular areas of practice. Even within a particular sector, drilling down into specific areas gives a greater granularity to the consideration of the impact of the Regulation in that particular area. With this in mind, this article focuses on the impact of the Regulation on ‘health research’. It does not discuss the implications for delivering healthcare, as the impact and application in the context of clinical care, are different and require different attentions. The article begins by considering the principles which underpin the Regulation. Within this discussion, the focus is on the restriction of these principles in the context of scientific research. Following this, the piece addresses how the processing of personal data for scientific research (The Regulation interprets scientific research broadly as shown in Recital 159: For the purposes of this Regulation, the processing of personal data for scientific research purposes should be interpreted in a broad manner including for example technological development and demonstration, fundamental research, applied research and privately funded research. Scientific research purposes should also include studies conducted in the public interest in the area of public health.) might be legitimized in the regulation (The definition of personal data is currently an issue of some controversy. It is defined in Article 4 (1) of the Regulation as follows: ‘personal data’ means any information relating to an identified or identifiable natural person (‘data subject’); an identifiable natural person is one who can be identified, directly or indirectly, in particular by reference to an identifier such as a name, an identification number, location data, an online identifier or to one or more factors specific to the physical, physiological, genetic, mental, economic, cultural or social identity of that natural person. However, whether it is possible to attribute personal data to an identifiable person directly or indirectly is not clear. This is an issue which the Information Commissioner’s Office (ICO) is currently considering. The ICO has further guidance on what constitutes ‘personal data’ on its website: https://ico.org.uk/for-organisations/guide-to-the-general-data-protection-regulation-gdpr/key-definitions/what-is-personal-data/ (accessed 3 October 2018). The historical practice of relying on consent for the processing of personal data for research is considered in the context of the alternative bases that the Regulation provides for the lawful processing of personal data for scientific research (The Regulation provides six grounds which make the processing of personal data lawful. See Article 6). In this discussion of lawful bases, it is necessary to consider the relationship between the Regulation and the duty of confidence, as any processing of confidential information also needs to have a lawful basis at common law to negate a common law action for breach of confidence (The piece does not have the space to consider the National Data Opt Out Programme, which relates to the healthcare professional’s duty of confidence, in depth). Finally, the piece considers how the Regulation safeguards data subjects’ rights and freedoms, where it enables the processing of personal data for scientific research.
lawfully, fairly and in a transparent manner
collected for specified, explicit and legitimate purposes and not further processed in a manner that is incompatible with those purposes (purpose limitation)
adequate, relevant and limited to what is necessary in relation to the purposes for which they are processed (data minimization)
accurate and, where necessary, kept up to date (accuracy)
kept in a form which permits identification of data subjects for no longer than is necessary (storage limitation)
in a manner that ensures appropriate security (integrity and confidentiality)
in a way which demonstrates compliance (accountability)
These principles promote a culture of data protection, which seek to encourage organizations which process personal data to ensure that data protection measures are baked into all aspects of planning and operations (see Article 25). A crucial aspect of this is that organizations are open and honest about how they process personal data and that they seek to minimize the personal data that they process by removing identifiable information wherever possible.
further processing for archiving purposes in the public interest, scientific or historical research purposes or statistical purposes shall, in accordance with Article 89(1), ‘not be considered to be incompatible’ with the initial purposes (Article 5 1. (b) (my emphasis)).
This provision concerns the secondary processing of personal data for research. Ordinarily, the further processing of that data for another purpose would have to be demonstrated to be compatible with the original purpose for that processing to be lawful (Article 5 1. (b)). The Regulation establishes a presumption that further processing of personal data for scientific research purposes will be compatible with the purpose for which they were originally collected (Article 5 1. (b) and Recital 50). The presumption of compatibility for secondary processing of data for scientific research purposes is a significant relaxation of the restrictions on repurposing personal data for scientific research purposes. When further processing personal data for research, the principle of data minimization still applies and requires the data controller (Controller is defined in Article 4 (7): ‘controller’ means the natural or legal person, public authority, agency or other body which, alone or jointly with others, determines the purposes and means of the processing of personal data. This is as opposed to a data processor which is defined in Article 4 (8): ‘processor’ means a natural or legal person, public authority, agency or other body which processes personal data on behalf of the controller. As the controller determines the means of processing most of the data protection obligations in the GDPR fall on the controller. In the context of research projects involving NHS data, the concepts of processor, controller and joint controller can be complex. For more detail on when an organization will be a controller or a processor in the health research context see https://www.hra.nhs.uk/planning-and-improving-research/policies-standards-legislation/data-protection-and-information-governance/gdpr-guidance/what-law-says/data-controllers-and-personal-data-health-and-care-research-context/ (accessed 3 October 2018).) to assess the feasibility to fulfil those purposes by processing data which do not permit or no longer permit identification (Recital 156).
personal data may be stored for longer periods insofar as the personal data will be processed solely for archiving purposes in the public interest, scientific or historical research purposes or statistical purposes in accordance with Article 89(1) subject to implementation of the appropriate technical and organizational measures required by this Regulation in order to safeguard the rights and freedoms of the data subject (Article 5 1. (e)).
Thus although in a general sense the Regulation seeks to restrict organizations from processing data collected for a particular purpose, for other purposes where that processing is incompatible with the initial purpose, this compatibility limitation is not applied in the context of secondary processing for scientific research purposes. In addition to this relaxation of the processing of personal data for scientific research as a secondary purpose, the regulation also provides significant scope for the processing of personal data for the ‘primary purpose’ of scientific research. This is achieved by providing grounds (usually referred to as lawful bases) for processing personal data which specifically embrace the processing of personal data for scientific research purposes.
the data subject has given consent
processing is necessary for the performance of a contract
processing is necessary for compliance with a legal obligation
processing is necessary in order to protect the vital interests of the data subject or of another natural person
processing is necessary for the performance of a task carried out in the public interest or in the exercise of official authority vested in the controller
processing is necessary for the purposes of the legitimate interests pursued by the controller or by a third party, except where such interests are overridden by the interests or fundamental rights and freedoms of the data subject
Consent is one among six lawful bases and does not enjoy status superior to the other lawful bases. This may seem counter intuitive to research organizations and researchers working in the context of health and social care, where the ethical importance of consent, and the autonomy it underpins, have become deeply rooted aspects of good practice. However, in recent years the ability of consent to protect people’s interests in the health and social care context has been questioned (See, for example, Foster C, Choosing Life, Choosing Death: The Tyranny of Autonomy in Medical Ethics and Law. Oxford: Hart Publishing, 2009). In particular the achievement of informed consent has been doubted, on the basis that even best practice often falls short of the theoretical ideal (See, for example, Grady C, Enduring and Emerging Changes of Informed Consent. N Engl J Med 2015;372:855–62).
consent should not provide a valid legal ground for the processing of personal data in a specific case where there is a clear imbalance between the data subject and the controller, in particular where the controller is a public authority and it is therefore unlikely that consent was freely given (see Recital 43 for the full text)
In line with this move away from consent as the lawful basis for processing data in the Regulation (This should not lead the reader to assume that consent is not required at all. The duty of confidence applies to confidential patient information and consent may still be required to negate a breach of confidence. This is considered in detail below), the national policy position is that the lawful basis for processing data for health and social care research should not be consent (See guidance provided by the following organizations: Health Research Authority (HRA) https://www.hra.nhs.uk/hra-guidance-general-data-protection-regulation/ Information Governance Alliance file:///C:/Users/lw1vlc/AppData/Local/Temp/igagdprconsent-1.pdf Information Commissioners Office (ICO) https://ico.org.uk/for-organisations/guide-to-the-general-data-protection-regulation-gdpr/consent/what-is-valid-consent/ and Medical Research Council (MRC) https://mrc.ukri.org/research/facilities-and-resources-for-researchers/regulatory-support-centre/gdpr-resources/ (all accessed 16 May 2018). Thus, organizations who process personal health data for research purposes should not assume that they need to get consent to the processing to comply with the GDPR. Instead the national policy position is that research organizations that process personal data will be doing so on the basis of either a task carried out in the public interest or in the exercise of official authority vested in them (Article 61. (e)), or for the purposes of legitimate interests (Article 61. (f). This does not mean that organizations do not need to tell people what they will do with their data. The GDPR requires that people are provided with information about the processing of their personal data. However, this is an independent requirement to provide information it is not part of a consent process, see Articles 12, 13 and 14.)
The NHS and universities are public authorities under The Data Protection Act 2018 (See clause 7 of the Data Protection Act 2018 which provides that a public authority is as defined by the Freedom of Information Act 2000). In their official authority, they carry out research as part of the official authority vested in them. The Information Commissioners Office states that ‘in the exercise of official authority’ covers public functions and powers that are set out in law; or specific tasks in the public interest that are set out in law. This does not mean that the organization needs a specific statutory power to process personal data, but its underlying task, function or power must have a clear basis in law. The NHS has research vested in it via the Health and Social Care Act 2012 which imposes a legal duty on NHS England to promote research and the use of research evidence in the NHS (Health and Social Care Act 2012 s 6 1E (a) and (b)). Further the NHS Constitution is committed to innovation and to the promotion, conduct and use of research to improve the current and future health and care of the population ( https://www.gov.uk/government/publications/the-nhs-constitution-for-england/the-nhs-constitution-for-england (accessed 4 October 2018). The Secretary of State must have regard to the NHS Constitution by virtue of the Health and Social Care Act 2012s 3 1B (1)). Universities have research officially vested in them as demonstrated universally by university charters which generally provide that they shall be a teaching and research bodies. Thus, national policy (Note 25 above) recommends that university and NHS controllers rely on the ground that processing is necessary for the performance of a task in the public interest or in the exercise of an official authority vested in them (Article 61. (e)) as their basis for processing personal data for research purposes (Although where there is a lawful basis for the processing of personal data, the controller could also seek to rely on Article 61. (c) that the processing is necessary for compliance with a legal obligation, but this may have a narrower remit that task in the public interest or official authority vested in the controller.).
Commercial research organizations are not public authorities. National policy (Note 25 above) recommends that they rely on the ground that processing is necessary for the purposes of their legitimate interests (Article 61. (f)). Where legitimate interests are relied on, the controller must conduct a balancing exercise to ensure that their legitimate interests are not overridden by the data subject’s fundamental rights and freedoms. The data subject’s rights could be overriding where they would not reasonably expect that personal data would be collected and processed for the particular purpose. Thus, where the organization performing the research is an NHS or university institution or a commercial entity, it is possible to process personal data for research purposes without consent. However, the importance of the word necessary in these lawful bases should not be underestimated. The Regulation makes clear that necessity is essential such that personal data should be processed:
Only if the purpose of the processing could not be reasonably fulfilled by other means (Recital 39).
This reflects the overriding principle of data minimization that underpins the Regulation (see discussion above).
In addition to having a lawful basis, organizations processing personal data for scientific research also need to determine whether the type of data they are processing is a special category of personal data’ (see Article 9).
Processing of personal data revealing racial or ethnic origin, political opinions, religious or philosophical beliefs, or trade union membership, and the processing of genetic data, biometric data for the purpose of uniquely identifying a natural person, data concerning health or data concerning a natural person’s sex life or sexual orientation shall be prohibited (Article 9 1).
The Regulation lays down 10 exceptions to this prohibition. Two exceptions to the prohibitions are particularly relevant in the context of scientific research. First, the prohibition does not apply where the subject of the data has given explicit consent (Article 9) to the processing of the data. Second, it does not apply where the processing is necessary for scientific research purposes (Article 9 where this condition is relied on safeguards must be in place—in particular the principle of data minimization must be applied).
any freely given, specific, informed and unambiguous indication of the data subject’s wishes by which he or she, by a statement or by a clear affirmative action, signifies agreement to the processing of personal data relating to him or her.
Consent must give individuals real choice. It needs to be clear, concise, specific and granular. It requires an affirmative action which means that any form of opt-out is not consent for the purposes of the Regulation (For further detail on his see ICO guidance at: https://ico.org.uk/for-organisations/guide-to-the-general-data-protection-regulation-gdpr/lawful-basis-for-processing/consent/ (accessed 14 May 2018). Further a number of conditions need to be met before a consent can be valid under the Regulation (see Article 7). Consent needs to be demonstrable (This relates to the underpinning principle of accountability enshrined in Article 5 of the Regulation. See discussion above) distinguishable from other matters in a written declaration, intelligible and capable of being withdrawn as easily as giving consent. As processing of patient data for research will concern a special category of personal data, if consent is the condition relied on for setting aside the prohibition on processing, that consent will need to be ‘explicit consent’.
Whilst the concept of ‘explicit consent’ is not further defined in the Regulation, the ICO states that:
Explicit consent requires a very clear and specific statement of consent ( https://ico.org.uk/for-organisations/guide-to-the-general-data-protection-regulation-gdpr/lawful-basis-for-processing/consent/ (accessed 14 May 2018).
It is often not possible to fully identify the purpose of personal data processing for scientific research purposes at the time of data collection. Therefore, data subjects should be allowed to give their consent to certain areas of scientific research when in keeping with recognized ethical standards for scientific research. Data subjects should have the opportunity to give their consent only to certain areas of research or parts of research projects to the extent allowed by the intended purpose (Recital 33).
Thus, whilst the advice for research organizations is to move away from consent under the Regulation, the recognition of the need to preserve broad consent in the scientific research context might provide reassurance for research organizations that wish to rely on consent as the condition for setting aside the prohibition. However, reliance on consent gives data subjects significantly more scope to control the processing of their data than if they relied on the research condition discussed above. In the context of data driven approaches to research, this continuing control and ability to withdraw data may affect the quality of the data set and the research which is conducted upon it. Furthermore, reliance on consent gives rise to a number of linked rights, which could hinder the potential of scientific research ‘designed to serve mankind’ (Recital 4).
The Regulation provides protection for a number of rights in chapter III. These are as follows: a right of access (Article 15), a right to rectification (Article 16), a right to erasure (Article 17), a right to restriction of processing (Article 18), a right to data portability (Article 20) and a right to object (Article 21). However, the Regulation allows Member States to derogate from the rights to access, rectification, restriction and objection, where these rights are likely to render impossible or seriously impair the achievement of the purpose of the processing, where the data are processed for scientific research purposes (Article 89 (2)). These derogations must be provided for in Union or Member State law. The Data Protection Act 2018 implements these derogations in the context of processing for scientific research (Schedule 2 part 6). The Regulation also provides direct exemptions to two rights where processing is for the purposes of scientific research (This means they do not need further Union or Member State law for implementation). First, processing is exempt from the right to object where data are processed for the purposes of scientific research and those rights are likely to render impossible or seriously impair the objective of the processing. Second, the right to erasure does not apply where the data are processed for scientific research purposes where that processing is necessary for a task carried out for reasons of public interest.
This approach to data subjects’ rights in the context of processing for scientific research seeks to strike a proportionate balance between the pursuit of knowledge which is ‘designed to serve mankind’ (Recital 4) and the protection of individual interests. The ability to derogate from the protection of these rights is not similarly available where the organization is relying on consent as the basis for processing personal data. Thus, where scientific research is aimed at ultimately improving human health, the concept of proportionality permits some limitation of the rights in the Regulation. Any limitation must be justified. Where safeguards accompany a limitation of rights this can support the justification of that limitation. Indeed, the reliance on the condition that processing is necessary for scientific research does not necessarily provide less protection for data subjects than relying on consent. As argued above, achieving an informed consent can be difficult in the context of future uses of personal data and the research condition is accompanied by safeguards which seek to protect data subjects in ways which they may not be similarly protected if consent is the condition for processing.
Any processing of data on the condition that it is necessary for the purposes of scientific research (Article 9 2. (j)) is subject to appropriate safeguards for the rights and freedoms of the data subject (Article 89 (1)). The Regulation requires that organizations have the technical and organization measures in place, in particular to respect the principle of data minimization (Article 89 (1)). The Data Protection Act 2018 affords further protection by providing that the research condition (Article 9 2 . (j)) will only be met if the processing is in the public interest (Schedule 1. Part 1. 4. Public interest is a difficult concept to define and no attempt is made to do this in the Data Protection Act 2018. For academic consideration of what amounts to the public interest in the context of health research see: Taylor MJ, Health research, data protection, and the Public Interest in Notification. Medical Law Review 2011;19:267–303). Furthermore, the Data Protection Act 2018 provides that processing on the basis of the research purposes condition does not satisfy the requirements of the Regulation for the processing to be subject to appropriate safeguards for the rights and freedoms of the data subject, if it is likely to cause substantial damage or substantial distress to a data subject. Or if the processing is carried out for the purposes of measures or decisions with respect to a particular data subject, unless the purposes for which the processing is necessary include the purposes of approved medical research (Clause 19 Data Protection Act 2018 ‘approved medical research’ means medical research carried out by a person who has approval to carry out that research from a research ethics committee recognized or established by the Health Research Authority or similar approvals processes in Scotland, Wales and Northern Ireland. This exception to individual decision making was an amendment to the Bill which arose following concern that without this exception, this safeguard would prevent the processing of data in interventional research).
This reliance on bases other than consent for the processing of personal data for scientific research may feel counter intuitive to researchers schooled in the ethical importance of informed consent in human subject research. Nevertheless, the fact that consent may not be the lawful basis for data processing under the GDPR does not affect the need to comply with other separate legal obligations to gain consent in the process of conducting research.
Where a person consents to participate in research they are consenting to a number of things other than the processing of their personal data. In clinical trials the Clinical trials on Medicinal Products for Human Use Regulations requires informed consent to be given by the individual or their legal representative (The Medicines for Human Use (Clinical Trials) Regulations 2004, Article 29 1). This is consent to the risks, implications, inconveniences, objectives and benefits of the clinical trial (Article 29 2. 9a (i)). The need to obtain this consent is unchanged by the data processing requirements in the GDPR, and the fact that this consent must still be sought to run the trial risks does not indicate that the lawful basis for data processing under the GDPR in the trial should also be consent. Similarly, in the context of medical treatment of capacitated adults, consent must always be sought to the medical intervention. First, this consent negates a battery. It also enables the patient to decide whether or not to accept the risks associated with the intervention, any failure to provide information about material risks may lead to an action in negligence. Neither the consent to the physical intervention, nor the consent to the running of the risks, will provide a lawful basis for associated processing of personal data under the GDPR. Where the personal data which is processed for research is patient data collected in the course of delivering healthcare, this personal data will be subject to the duty of confidentiality as well as the GDPR. This creates an added level of complexity for those seeking to process patient information for scientific research.
Explicit consent
Statutory basis (In the healthcare research context, the most relevant statutory basis is s 251 of the NHS Act 2006 and the Control of Patient Information (COPI) Regulations.)
Overriding public interest (This ground has been reserved for serious physical threats to the public see W v Egdell [1989] EWCA Civ 13 and is not generally relied on the negate a breach of confidence in the context of the use of personal data in healthcare research.)
In the context of healthcare research, the most common lawful bases for setting aside the duty of confidence are explicit consent or a statutory basis provided by section 251 of the National Health Service Act 2006 which allows the duty of confidentiality to be set aside for defined purposes, including medical research, where it is not possible to use anonymised information or seek consent. The legal bases for setting aside the duty of confidentiality are less extensive and developed than the legal bases for processing personal data under the GDPR. This divergence creates a situation where the legal regimes are developing in different directions, whilst the GDPR proving a regime which enables the processing of personal data for health research, our common law duty is more restrictive and prevents the wide scope provided in the Regulation where the information is also subject to a duty of confidence. However, this concern might be somewhat tempered by the fact that achieving a consent which is sufficient to set aside the duty of confidentiality is easier to achieve that one which satisfies the requirements of the GDPR.
The Regulation requires that a valid consent is specific and granular (See discussion above. This is subject to Recital 33. However, the potential for adopting a particularly broad approach to consent for scientific research purposes on the basis of Recital 33 is diminished by the Article 29 Data Protection Working Party Guidelines on consent under Regulation 2016/679, April 2018, which provide that ‘Considering the strict conditions stated by Article 9 GDPR regarding the processing of special categories of data, WP29 notes that when special categories of data are processed on the basis of explicit consent, applying the flexible approach of Recital 33 will be subject to a stricter interpretation and requires a high degree of scrutiny’). Whilst the common law is likely to recognize broad consent as an acceptable basis for setting aside the duty of confidence (See my previous work on this issue Chico V and Taylor MJ, Using and disclosing confidential patient information and the English common law: what are the information requirements of a valid consent? Medical Law Review 2018; 26:51–72. In the context of choices about medical treatment, the common law does place emphasis on the need to provide specific information about material risks and alternatives. See ‘Montgomery v Lanarkshire Health Board’ [2015] UKSC 11).
The concerns raised above regarding the fact that reliance on consent might devalue some scientific research, on the basis that the existence of data subjects’ rights could interfere with the quality of data available, do not apply in the same way to consent to set aside the duty of confidence. Here there are no clear rights to further control information that one has consented to the use of. The strong position on withdrawal in the GDPR might not have the same application in the context of the duty of confidence. This also means that a consent which is sufficient to set aside the duty of confidence, which, in the research context, will often be obtained in the context of consent to a research intervention, is unlikely to be sufficient to also meet the stringent requirements for consent under the GDPR. Given this research organizations may rely on consent to set aside the duty of confidence but rely on a different legal basis under the GDPR (namely the public interest basis and the research condition discussed above).
This piece highlights the proportionate approach to data protection that the GDPR provides in the context of data processing for the purposes of scientific research. The Regulation’s position on the need to process personal data ‘designed to serve mankind’ (Recital 4), reflects a regulatory position that enables, rather than inhibits, the processing of personal data for scientific research which fulfils that potential. However, in the context of using personal health information for research that is designed to promote human health, the duty of confidentiality currently provides more limited scope for lawful use of patient data which could hinder the enabling effect that the GDPR seeks to achieve in the context of scientific research.
- confidentiality
- european union
- statutes and laws
- transparency
Email alerts
Citing articles via.
- Recommend to your Library
Affiliations
- Online ISSN 1471-8391
- Print ISSN 0007-1420
- Copyright © 2024 Oxford University Press
- About Oxford Academic
- Publish journals with us
- University press partners
- What we publish
- New features
- Open access
- Institutional account management
- Rights and permissions
- Get help with access
- Accessibility
- Advertising
- Media enquiries
- Oxford University Press
- Oxford Languages
- University of Oxford
Oxford University Press is a department of the University of Oxford. It furthers the University's objective of excellence in research, scholarship, and education by publishing worldwide
- Copyright © 2024 Oxford University Press
- Cookie settings
- Cookie policy
- Privacy policy
- Legal notice
This Feature Is Available To Subscribers Only
Sign In or Create an Account
This PDF is available to Subscribers Only
For full access to this pdf, sign in to an existing account, or purchase an annual subscription.
Cybersecurity, Data Privacy and Blockchain: A Review
- Review Article
- Open access
- Published: 12 January 2022
- Volume 3 , article number 127 , ( 2022 )
Cite this article
You have full access to this open access article
- Vinden Wylde 1 ,
- Nisha Rawindaran 1 ,
- John Lawrence 1 ,
- Rushil Balasubramanian 1 ,
- Edmond Prakash ORCID: orcid.org/0000-0001-9129-0186 1 ,
- Ambikesh Jayal 2 ,
- Imtiaz Khan 1 ,
- Chaminda Hewage 1 &
- Jon Platts 1
19k Accesses
41 Citations
Explore all metrics
In this paper, we identify and review key challenges to bridge the knowledge-gap between SME’s, companies, organisations, businesses, government institutions and the general public in adopting, promoting and utilising Blockchain technology. The challenges indicated are Cybersecurity and Data privacy in this instance. Additional challenges are set out supported by literature, in researching data security management systems and legal frameworks to ascertaining the types and varieties of valid encryption, data acquisition, policy and outcomes under ISO 27001 and the General Data Protection Regulations. Blockchain, a revolutionary method of storage and immutability, provides a robust storage strategy, and when coupled with a Smart Contract, gives users the ability to form partnerships, share information and consent via a legally-based system of carrying out business transactions in a secure digital domain. Globally, ethical and legal challenges significantly differ; consent and trust in the public and private sectors in deploying such defensive data management strategies, is directly related to the accountability and transparency systems in place to deliver certainty and justice. Therefore, investment and research in these areas is crucial to establishing a dialogue between nations to include health, finance and market strategies that should encompass all levels of society. A framework is proposed with elements to include Big Data, Machine Learning and Visualisation methods and techniques. Through the literature we identify a system necessary in carrying out experiments to detect, capture, process and store data. This includes isolating packet data to inform levels of Cybersecurity and privacy-related activities, and ensuring transparency demonstrated in a secure, smart and effective manner.
Similar content being viewed by others
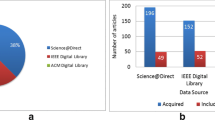
Blockchain smart contracts: Applications, challenges, and future trends
Shafaq Naheed Khan, Faiza Loukil, … Anoud Bani-Hani
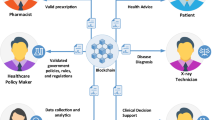
Blockchain for healthcare data management: opportunities, challenges, and future recommendations
Ibrar Yaqoob, Khaled Salah, … Yousof Al-Hammadi
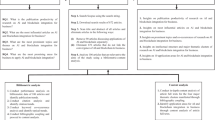
Artificial Intelligence and Blockchain Integration in Business: Trends from a Bibliometric-Content Analysis
Satish Kumar, Weng Marc Lim, … Jaspreet Kaur
Avoid common mistakes on your manuscript.
Introduction
With the recent emphasis on societies in increasing their dependency on cloud technologies, coupled with the human need to communicate and share data via digital networks, Internet of Things (IoT) devices to include smart phones, industrial and domestic appliances, continue to be a necessary function in conducting business. Social exchanges and transactional types of data for example, drive the financial markets thus facilitating in the swift development of emerging technologies at an ever faster rate to keep up with supply and demand trends. In a domestic setting, the sharing of digital media (videos, music, pictures, documents (data)) through messaging services to enhance subject areas such as information technology, sport, social sciences, education and health for example, IoT devices enable the efficient and effective transfer of data world-wide instantly via the Internet of Everything (IoE) via the cloud. In an industrial context, Smart Sensors, Application Programming Interfaces (API) and IoT networks facilitate remote working across digital boundaries globally.
These potentially devastating instances of data sharing and/or criminality, influence the confidentiality and protections set out by governments, businesses and organisations, culminating in legal and ethical disputes with significant financial ramifications due to Denial of Service (DDoS) attacks for example, that would damage and disrupt entire business data architectures, infrastructures networks and services on a large scale. Consequently, with society relying more and more on the exchange and processing of Personal Identifiable Information (PII) via IoT, trust in renowned institutions and government organisations to include broadcast and digital media outlets becomes a main issue. As a user chooses to share social network, personal and confidential information whilst shopping on-line for example, they should be aware of the nature and intent of cyber-criminality and have faith in the criminal justice system of a given territory.
On the other hand, for businesses, organisations, government bodies and academic institutions to be able to freely validate and authenticate their data in the service of societies globally, Artificial Intelligence (AI), Big Data (BD), Blockchain (BC) Combined Technologies and methodologies, contribute significantly in mitigating cyber-crime, whilst providing legal bodies the power to hold companies, organisation and institutions to account. One such method is the Smart Contract (SC) for example, and when utilised in the drafting and consenting of a legal document or digital certificate, provides an evidence-based transparent method in enhancing the legal credibility and value of a financial transaction. As a function of BC, the SC is validated, implemented then shared across a Pier-to-Pier (P2P) network as a Distributed Ledger Technology (DLT) for all parties to see which provides transparency and accountability.
- Cybersecurity
When utilising elements of cybersecurity, these technical requirements facilitate in the effective management of IoT hardware and software operations, physical interfaces and internal policy development. Additionally, the management system ISO 27001 supports network communication protocols, data access control and cryptography (i.e., password encryption), that contribute in ensuring a robust and secure communication method inclusive of cybersecurity staff training; all whilst minimising network communication attacks in the presence of malicious third-parties [ 1 ].
However, to harness and derive value from the volume, variety and veracity of data available, concepts such as BD, AI and Machine Learning (ML) utilise prescribed algorithms and analysis techniques across vast quantities of public, private and sensitive data through digital networks, that exponentially increases the risk of data breaches, viruses and malicious attacks. In other words, in successfully utilising these technologies in the legal acquisition and processing of data from the public and private sectors, also to include practical user measures, potentially reveals challenges and vulnerabilities that can further expose a user or group to cyber-criminality.
Data Privacy
Additionally, the ISO 27001 framework functions in conjunction with the General Data Protection Regulation (GDPR) Regulation (EU) 2016/679, and Data Protection Act 2018 c. 12 (DPA), in facilitating personal data controls and measures in the UK and European Unions (EU) digital boundaries. In processing medical data for example, a mandatory Data Protection Impact Assessment (DPIA) is undertaken in identifying and establishing the risks alongside eight core principles to include; lawful and ethical methods of data acquisition, data storage security and duration, fair use, and for data to be kept within specified locations and regions [ 2 ].
In utilising these legal frameworks and management systems, tracking tools such as ‘cookies’ for example may utilise the aforementioned AI, ML and algorithmic analysis unlawfully, and as a result, a user may not be aware of the tracking nature and capabilities contained within the software for analysis and marketing purposes. Additionally, without user consent, the awareness and continual levels of maintenance required of said cookies, that are a necessary function in surfing the web, could expose business networks to anti-forensic methods, legal jurisdiction matters, system hardware and Service Level Agreement (SLA) breaches, which compound over time and further aggravate technical, legal and ethical challenges in operating IoT devices in a compliant, safe and secure business environment.
Furthermore, when utilising in a healthcare service context, a SC policy with cryptography as a cybersecurity control method, gives transparency, protected agency and responsibility to the public, financial markets, business professionals and legal representatives, in conducting valid and transparent actions or investigations on behalf of the directorate or client. When this method is applied retrospectively, it also gives accountability in upholding vigilance and resilience when managing cyberspace, an operators duty of care and consideration of confidential data breaches, its sharing, and ramifications of exposing vast amounts of confidential National Health Service (NHS) patient data for example [ 3 ].
Blockchain Security
BC based functions, methods and systems utilise concepts like Cryptocurrency (i.e., Bitcoin and Etherium) as an alternative to fiat currencies, representative consensus protocols, anonymous signatures, off-chain storage and non-interactive zero-knowledge proofs. These concepts provide validity, anonymity, and transparency when coupled with inner corporate or organisational audit, policy deployment, healthcare provider and security service function of carrying out legal and domestic activities. This system is trustless by design and offers promise for equitable and transparent transactions.
As per all the above, this review and study proposes an intelligent framework to aide in the identification and detection of compromised network packet data. The use of BC and SC are to be utilised as an information carrier (data) and for evaluation, validation and testing with pre-prescribed control protocols. Then, to conduct a literature review in ascertaining current methodologies, techniques and protocols in aiding the development of said framework. To minimise human intervention, an intelligent automated approach is utilised in the capturing of network data at pre-determined intervals. Ultimately, the data events are tested against a framework with analysis of findings to demonstrate comprehensive framework feasibility (see Fig. 1 ).
Cybersecurity refers to: “a measure for protecting computer systems, networks, and information from disruption or unauthorized access, use, disclosure, modification or destruction” [ 4 ]. Therefore, in trying to understand cybersecurity and its applications towards IoT and smart devices, brings additional questions that need analysis through various notions of cyberspace. One solution is unifying all the terminologies above to bring together the importance of understanding where network intrusion comes from, how it is detected, and how prevention of cyber threats occur. When looking at prevention, AI and ML uses could also potentially contribute to the rise in using this technology to secure and protect data [ 5 ].
Cybersecurity IoT and ML
As Information Technology (IT) facilities expanded, overall digital technology saw growth in more devices being introduced and connected to the internet, so that access to data is freely available to allow for more activities to be undertaken. These activities allow for outcomes to be predicted [ 6 ]. Therefore, in response, various ML mathematical algorithms allow for classification usage such as Support Vector Machines (SVM), Decision Trees and Neural Networks. These algorithms all compound and highlight how data is treated and managed to produce an outcome, and predictability that is required to contribute to economic growth as societies move forward. ML capabilities go far beyond the expectations of conquering human hobbies, but lends further into everyday chores and events in daily lives.
Other real-life examples of ML usage rest in many industries focusing on identifying fake news, implementation of spam filters, identifying fraudulent or criminal activities online, and improving marketing campaigns. These large quantities of data are often private and sensitive, whilst travelling through Cyberspace transferring data along the way. Disadvantageously, this existence of cyberspace creates a wider security attack surface for potential malicious activities to occur. This demonstrates that human factors and the large influence it has on the security of IoT [ 7 ] is highly impactful.
Humans’ perceptions of security and privacy concerning these devices are also a subject to be discussed, for example, the concept of ‘Cookies’ as a tracking tool for online web surfing, and its safety measures, which are often shoehorned as a debate in itself, and the awareness of how it should be used has been seen through glazed eyes [ 8 ]. However, recent reports suggest that many contributory questions arise from understanding IoT and the safety net around it, and how humans cope and live alongside IoT. Anti-forensic methods, jurisdiction and Service Level Agreements (SLA) for example, all further aggravate technical, privacy, security, and legal challenges. In addition, the presence of GDPR and IoT, coupled with the human factors involved, present immense challenges in keeping these devices safe and secure.
Cybersecurity and SMEs
UK Small to Medium Enterprises (SME’s) have always seen challenges in understanding cybersecurity due to the increase in threats that have risen in recent years. The European Commission’s employment criterion for an SME minimum cyber-criterion is that for any business that employs less than 250 people [ 9 ]. The challenges faced are both operational and commercial in SMEs using Intrusion Detection mechanisms coupled together with AI and ML techniques in the protection of their data.
SMEs intrusion, detection, and prevention methods has become a priority in the realisation of keeping their data secure and safe with the integration of real-world objects and IoT, with understanding how ML techniques and AI can help secure zero-day attacks. Rawindaran et al. [ 1 ] took particular interest in the SME market and showcased an experimental scenario in which the intrusion, detection and prevention models were compared, and the views of the SME examined. The study looked at the various approaches in identifying ways to detect and protect any intrusions coming into the network and what operating devices would help in this process. The paper also explored the understanding in trying to protect the data and how government policies and procedures such as GDPR in the UK/EU, could assist towards this process [ 10 ].
Cybersecurity and SME Attacks
Rawindaran et al. [ 11 ] further examined the impact of how threat levels of attacks such as Ransomware, Phishing, Malware, and Social-engineering amongst others, were compared between an Open-Source device, such as SNORT and pfSense, and Commercial Network Intrusion Detection (NIDs) such as Cisco. There were three different NIDs and their features were compared. It was concluded that whilst SNORT and pfSense were free to use from the Open-Source market, it required a certain level of expertise to implement and embed the rules into a business solution. It was also noted that Cisco, due to their engineering expertise and their position as market leaders in the industry, were able to embed these free rules and use it to their advantage.
What emerged from this study was how businesses and organisations with the help of government policies and processes, needed to work together to combat these hackers, malicious actors, and their bots, and manage and stay ahead of the game [ 4 ]. The paper also discussed various ML approaches such as signature based models and anomaly based rules used by these devices to combat these attacks [ 12 ].
Additionally, signature based models could only detect attacks that were known, whereas anomaly-based systems were able to detect unknown attacks [ 13 ]. Anomaly-based NIDs made it possible to detect attacks whose signatures were not included in rule files. Unfortunately, due to the maturity of Anomaly NIDs, the costs were still very high to run and required computing power that were unrealistic in the SME environment. Anomaly based NIDs whilst still in its infancy, require a deeper analysis and future study.
Rawindaran’s study provided perspectives on better comparisons and relative conclusions and how it was important to explore further both the empirical as well as in scenario analysis for different dimensions, the nature and context of cyber security in the current world of internet and cyber connections. Rawindaran also explored how ML techniques have become vital in the growth and dependencies of these SMEs in the UK in their operations and commercial environment. This study took on an initial look at success stories from big technology companies such as Amazon, Google, and Facebook, in their use of ML techniques for their cybersecurity [ 14 ]. The methodology adopted in this study focused on structured survey questions on a selected sample number of respondents and directed its questions to the SMEs management, technical and non-technical professionals.
Cybersecurity and ML to Mitigate Attacks
Rawindaran et al., found that awareness of ML and its uses is still on a learning curve and has yet to be defined. The study brought to surface the three main categories of ML that being Supervised Learning, Unsupervised Learning and Reinforcement Learning and the algorithms that sit behind them [ 15 ]. Examples of Supervised Learning included real life predictive text in tweets in Twitter and product reviews in Amazon and eBay, calculating temperature, insurance premiums, pricing, and number of workers to the revenue of a business.
Examples of Unsupervised Learning include examples include identifying fake news, implementation of spam filter, identifying fraudulent or criminal activity online, and marketing campaigns. Reinforcement Learning shows example of playing a video game that provides a reward system when the algorithm takes an action. Each learning method used algorithms that helped with calculations and predictions and a dataset that helped in the development and structures of its uses. It also deducted and quantified examples and showed strength in the SMEs perception and awareness towards ML and its uses.
The methods of ML and its algorithms lead into the focus of this study in which SMEs were given the opportunity to make themselves aware of these algorithms that exist within their own cybersecurity software package. Further the analysis of this study showed the existence of these algorithms such as Neural Networks, Support Vector Machines, Deep Networks and Bayesian, however most of these were cleverly embedded within the software used [ 16 ].
The initial idea of using an Intrusion, Detection and Prevention System (IDPS) method, from either a commercial or Open-Source device to protect the data of the SME, comes with the knowledge of ML and AI. As hackers become increasingly clever and the uses of bots take over, their ‘attacking’ methods, as protectors of the systems, society has had to lean on ML and AI technology to help. An IDPS system is able to help through the use of ML, to learn about malicious patterns compared to valid patterns on the internet. These various approaches are needed to protect and shield data. ML through anomaly detection, proved to be more effective in its zero-day detection than that of signature based in its effectiveness towards cybersecurity and adoption within the UK SMEs. There is a significant gap that needs to be fulfilled by perhaps more variations in the devices used for SMEs such as opensource and voluntary participants from knowledge of the community to keep future proofing these devices.
Cybersecurity and Adversarial ML
With the increased use of ML in Intrusion Detection Systems (IDS) and IDPS systems within cyber security packages of SME communities, there suddenly lies the introduction of a new type of attack called Adversarial Machine Learning (AML) [ 1 ]. In a paper by Anthi et al. [ 17 ] states that with the introduction of ML IDSs, comes the creation of additional attack vectors specifically trying to break the ML algorithms and causing a bypass to these IDS and IDPS systems. This causes the learning models of ML algorithms subject to cyber-attacks, often referred to as AML.
These AMLs are thought to be detrimental as they can cause further delayed attack detection which could result in infrastructure damages, financial loss, and even loss of life. As [ 17 ] suggests, the emergence of Industrial Control Systems (ICS) plays a critical part on national infrastructure such as manufacturing, power/smart grids, water treatment plants, gas and oil refineries, and health-care. With ICS becoming more integrated and connected to the internet, the degree of remote access and monitoring functionalities increases thus becoming a vulnerable point target for cyber war. Additionally, with ICS more prone to targeted attacks, new IDS systems have been used to cater for the niche market of ICS, thus introducing vulnerabilities in particular to the training model of ML.
With the introduction of these new IDSs, has also introduced new attack vectors into the mix. The definition of AML provided by Anthi states that: “The act of deploying attacks towards machine learning-based systems is known as Adversarial Machine Learning (AML) and its aim is to exploit the weaknesses of the pre-trained model which has ’blind spots’ between data points it has seen during training”.
This is challenging as ML usage in IDS is becoming a tool used in daily attack detection. The study showed how AML is used to target supervised models by generating adversarial samples and exploring and penetrating classification behaviours. This was utilised by the use of authentic power system datasets to train and test supervised machine learning classifiers through its vulnerabilities. The two popular methods that were used in AML testing were automatically generated perturbed samples that were the Fast Gradient Sign Method (FGSM) and the Jacobian based Saliency Map Attack (JSMA).
Both methods showed how AML was used in penetration of systems through ML training models leading onto cyber-attacks. In another study by Catak et al. [ 18 ], further explored the security problems associated with AML, this time through the networks of 6G applications in communicative technology, that focused on deep learning methods and training. With the rapid development and growth of deep learning and its algorithms in the future technology pipeline of 6G was to further understand the security concerns around it.
Cataks’ paper [ 18 ] produced faulty results through manipulation of deep learning models for 6G applications to understand AML attacks using Millimetre Wave (mmWave) beam prediction in this case. AML mitigation and preventative methods were also used to try and stop these attacks from occurring for 6G security in mmWave beam prediction application with fast gradient sign method attack. In conclusion to Cataks’ paper found that several iterations of introducing faulty results gave a more secure outcome of the performance and security of the device. ML deep learning methods and algorithms were able to use these faulty results in altering the adversarial training approach. This increased the RF beam-forming prediction performance and created a more accurate predictor in identifying these attacks against the ML applications use.
Cybersecurity: Summary
As with any new technology that stems to improve the cyber highways in lessening the effects of cyber-attacks, it is always coupled by the counterattack measure within this space. Being aware of these adversaries and future research will help reduce, or at least control the level of attacks being present in any cyberspace and landscape moving forward. The recognition of funding gaps that could be fulfilled by the government to support SMEs in the form of grants, subsidies, and similar financial assistance, through various public sector policies is also an important route to consider. Awareness and training for all SME management and their staff is important to understand the basic and perhaps advanced appreciation of cybersecurity through the eyes of ML and AI.
Whilst technology giants might lead the path in its implementation of ML and cybersecurity through its many variations of intrusion, detection, and prevention methods, it is these firms that will set precedence and bring awareness down to a SME level and the importance of ML in keeping our cyber world safe. Understanding whilst ML is increasing in usage through IDS and IDPS systems to reduce the cyber attack footprint, means that the rise in AML also is something to be concerned about.
An example in GDPR Recital 4 and in the proceeding Directive 1995/46/EC Recital 2, a main objective “the processing of personal data should be designed to serve mankind”. For this purpose, the Data Controller ensures legal compliance and legal justification of data processing out of necessity (not only processing convenience) and proportionality. For the acquisition of high-risk health data for example, GDPR mandates that a DPIA is carried out to mitigate risk and assess risk level to include if the data should be processed or not [ 19 ]. With data protection law, the UK and EU demonstrate cooperation, ethics, transparency with robust control methods in mitigating data privacy breaches. However, this also brings attention to the range of legal frameworks and the general movement of people globally. This should inform governments and business in data protection strategies.
Data Privacy: Legal Frameworks [UK-EU]
Between the UK and EU, the Data Protection Act 2018 (DPA) and General Data Protection Regulations 2016 (GDPR) function together in overseeing how businesses, organisations and governments, utilise personal data. Eight key objectives guide anyone responsible for the handing and processing of personal data, and strictly imposes that data has to be lawful [acquisition], fair, accurate and up-to-date, not kept longer than needed, kept safe and secure, and not to be transferred outside the European Economic Area (EEA). By design, GDPR encompasses human rights with additional data collecting and processing principles (e.g. purpose, data-types and processing duration) [ 20 ].
Data Privacy: SARS-Cov-2: Covid-19
In supporting the effort in mitigating disease transmission from the coronavirus pandemic (Covid-19), the cloud, cell-networks and IoT devices such as smart-phones, sensors and domestic appliances, continue to play a vital role in a wide range of global Tracing-Testing-Tracking programs. Many different approaches are adopted by global communities in minimising person-to-person transmission [ 21 , 22 ]. This demonstrates that in response to the pandemic, coupled with the urgency in developing and deploying digital solutions, data privacy implications become ever more challenging with increasing data privacy risks. As a result, the handling of personal data [acquisition] research has developed and expanded [ 23 ].
However, in mitigating data privacy risks under adverse social and environmental conditions, it is not simply a matter of deploying digital solutions. The challenges presented in terms of service delivery (consistency, proportionality and transparency), also potentially increases the risk of data privacy breaches. Therefore, in terms of scalability via the cloud, partnerships between populations, businesses and governments could harmonise policy development and implementation with digital solutions.
Data Privacy: Consent—Contact Tracing Apps
In a Republic of Ireland survey conducted with over 8000 participants, it was found that 54% would accept using a contact tracing app. Similarly, in the UK from a survey of 2000 participants found that 55% would accept using a government-controlled app, with higher uptake specifically for the NHS contact tracing app [ 21 ]. This information demonstrates a lack of app uptake in the remaining 45% of the British population that could undermine a governments ability in effectively handling data collection and the processing of critical medical information.
In contrast, other countries infer citizen consent when data collection is initiated for the public good. Meaning that private parties’ access to data is also endorsed by governments. Amnesty International (2020) also brings attention to many instances of questionable data privacy practices throughout numerous countries [ 21 ]. The examples potentially show the scale of data protection perceptions and attitudes and how they are interpreted, thus justifying a more focused and intensive approach to data privacy collaborative research. By analysing a variety of legal and regulatory frameworks, solutions and practices in a pandemic or crisis situation, we can learn how to effectively apply powerful and scalable outcomes. For example, robust and transparent data is necessary for the urgently needed Covid-19 vaccine distribution efforts for each nation [ 24 ].
Transparency: NHS Test-Trace App
In response to the pandemic, the UK Government and NHS X (Digital) contact tracing app, aided by the private sector, brought into question their overall GDPR utility and compliance. Sub-contractors and companies that represent NHS X are also considered as processors of data, which bring additional GDPR compliance pressures. In this instance, the NHS X app code and DPIA was voluntarily submitted to the Information Commissioners Office (ICO) without the data store. This potentially highlights a lack of transparency with GDPR compliance, health surveillance capabilities and data storage capacities. The Joint Committee on Human Rights (JCHR) for example, were concerned at the rapid development and deployment of the contact tracing app in March 2020 [ 19 ].
Data Storage and Identification
Clear definitions and solutions are needed for data and storage methods. Currently, obtaining an integrated and comprehensive view of (1) internal organisational personal data storage, (2) full organisational content comprehension of regulation, and (3) an auditable trail of necessary data processing activities [ 20 ]. Although GDPR compliance has significantly enhanced personal data protection (e.g. PII, PII sharing via add and marketing, collecting and sharing location data, child PII sharing, law enforcement, and data aggregation), more research is needed in facilitating a users right to erasure, to update and delete data and to completely satisfy the GDPR promise [ 25 ].
Accountability and Traceability: BC & SC
To aide government transparency and societal trust, part of a solution is robust data privacy and accountability policies. Antal et al., discusses how BC can be effective in traceability, transparency, vaccine ID, assurances of it’s delivery, storage to include self-reporting of side effects. The authors implement a BC strategy using the inherent integrity and immutability of BC with ’in case of beneficiary registration for vaccination’ provision, thus eliminating identity impersonations and identity theft [ 26 ].
An example from Honduras demonstrates how a Toronto-based technology launched ’Civitas’, with user and government linked ID on a BC-based network. The BC contains the necessary data for determining when an individual can buy medicine, go food shopping, and also data to inform government agencies in resource and deployment strategies [ 27 ]. The GDPR for example, would conflict with this contact tracing methodology. More specifically, the right for a user to be forgotten (Article 17: Right to Erasure) due to BC immutability, and processing speed that would also inhibit BC network uptake and scalability.
However, BC in this case could operate within the confines of management and governance of BD repositories and warehouses whilst leveraging SC to enhance accountability, transparency and consistency in the appropriate forum.
Trust: Vaccine Hesitancy in UK Households
Whilst a global effort was underway in mass vaccination programs, the UK strategy highlighted disparities from a lack of public engagement between public health bodies and ethnic minorities from historic mistrust and a lack of understanding in technology [ 24 , 28 ]. Additional hesitancy included acute and chronic health effects from the vaccine.
A UK survey from 2020 for example, illustrated how Black, Asian, Minorities and Ethnic (BAME) communities had high vaccine hesitancy rates, when compared to white ethnic populations [ 28 ]. In Robertson 2021, the authors state that “Herd immunity may be achievable through vaccination in the UK but a focus on specific ethnic minority and socioeconomic groups is needed to ensure an equitable vaccination program” [ 29 ]. Including a more targeted approach to mental illness and disability [ 30 ].
Data Privacy—Summary
In a global setting, is it possible to ethically and accurately collect data [also without consent] whilst also providing legibility for effective data collection, resource allocation and deployment strategies? A small part of the solution is in gaining a populations’ trust in technologies such as NHS app uptake, and for future research in global deployment strategies. This means a wide-ranging and continual assessment of legal frameworks and outcomes between companies, organisations and institutions for long-term data privacy planning. Strategies also include ensuring groups and individuals have faith in their data integrity in the cloud.
As necessary components of GDPR, the collecting, processing and deleting data remain a challenge. The enable user to fully engage with confidence, education and engagement with minorities, and with mental illnesses is an effective way to provide group assurances. As with different countries, data protection concepts and public engagement practices vary significantly. For anticipating any future disaster or pandemic scenario, it is clear that accountability through public engagement should help restore national and international trust. Also research needs to be undertaken to design and promote a flexible and global strategy to encompass technical solutions, operational resource strategy, and policy development. This would enhance data protection objectives, build population trust in government monitored apps and ultimately provide a successful and robust global protection strategy.
Blockchain for Security
Blockchain—integrity of data.
BC is one of the most commonly discussed DLT for ensuring the integrity of data storage and exchange in trust-less and distributed environments. It is a P2P decentralized distributed ledger [ 31 ] that can enable trusted data exchanges among untrusted participants in a network. BC systems such as Ethereum and Hyperledger fabric, have become popular BC frameworks for many BC-based software applications. Core features of BC such as immutability and decentralization are recognized by many sectors such as healthcare and finance to improve their operations. Although BC is a relatively new technology—just over a decade old—it seems to be revolutionary and there is a substantial number of research articles and white papers to justify this remark.
Blockchain—Cybersecurity
It is important to answer how emerging technologies such as BC can offer solutions to mitigate emerging cybersecurity threats and there is great research interest to study how BC can provide foundations for robust internet security infrastructures [ 32 ]. Many of the articles propose frameworks, prototypes and experimental beta BC-based solutions to problems in complex computing systems. Most of these experimental solutions are developed on Ethereum and Hyperledger fabric. In the case of Hyperledger fabric for example, this is due to its ease of software development, extensive customisability and interactivity.
Although Bitcoin is a most popular BC network, it has many cons such as its latency and great resource requirement. Some of practical solutions among them use innovative techniques to resolve critical cybersecurity issues. However, they imply infeasible changes to the existing system infrastructures that are difficult to readily test for efficiency and effectiveness when compared with conventional cybersecurity frameworks [ 33 ].
Blockchain—IoT
In our increasingly interconnected IoT world, there is a great need to improve cybersecurity. As explained in [ 34 , 35 ], cyber-attacks that exploit vulnerabilities in IoT devices raise serious concern and demand for appropriate mitigation strategies to tackle these threats. Ensuring integrity of data management and malware detection/prevention is an exciting topic of research [ 36 ].
It should be noted here that BC cannot eliminate cyber risks, but it can significantly minimize cyber threats with its core features. While most IT systems are built with cybersecurity frameworks that use advanced cryptographic techniques, they rely on centralized third-party intermediaries such as certificate authorities to ensure the integrity of their data management. Malicious parties can exploit weaknesses in such relationships to disrupt/penetrate these systems with cyber threats such as DDoS attack, malware, ransomware, etc.
Blockchain—Protocols
BC can resolve these issues due to its decentralization; it eliminates single points of failures and the need for third-party intermediaries in IT systems and ensures the integrity of data storage and exchange with encryption and hash functions [ 37 ] so that data owners can completely audit their data in the systems.
A BC network with many mutually trustless nodes is more secure than a network with few nodes that rely on trusted/semi-trusted centralized third-party intermediaries because, in a BC network, every node has a complete copy of the unique record of all transactions in the network that is maintained with the network consensus protocol. The robustness of a BC network i.e. its safety and security, depends on its decentralization, and this depends on its governance and consensus protocols. A good comparative study of DLT consensus protocols is provided by Shahaab et al. [ 38 ].
Blockchain—Summary
What are some future research directions and challenges for BC and Cybersecurity?
Consensus Protocols: Generally, public BC networks have high latency due to their consensus protocols. This makes them a non-starter for applications in real-time environment. Research on consensus protocols should be holistic and consider both, hardware and software, for such environments [ 39 ].
Cryptocurrencies: more research on cryptoassets is needed to tackle challenges to legal enforcement and forensics - both domestic and international—that enable cybercriminal activity such as terrorism financing.
IoT: As explained in [ 40 ], consortium BC networks can be used to improve the overall internet connectivity and access. Future research on IoT-BC integration should demonstrate feasible implementations that can be evaluated and compared with existing IoT solutions. They should also quantitatively study fault tolerance, latency, efficiency, etc. of BC-based IoT networks.
Data Analytics: BC can ensure the integrity of data and with AI/BD analytics it can be used to reduce risks and fraudulent activities in B2B networks. Hyperledger fabric is a DLT project that can be used for this relatively unexplored research areas.
Cybersecurity, Data Privacy and Blockchain
As stated in [ 41 ], BC-based digital services offer transparency, accountability and trust, however not one size fits all, as there are paradoxes between cybersecurity, GDPR compliance and the operation of BC. Haque et al., demonstrate in a systematic literature review regarding GDPR-BC compliance and highlights six major categories that are:
Data modification and deletion (Articles 16–18)
Default protection by design (Article 25)
Controllers/processors responsibilities (Articles 24, 26 and 28)
Consent management (Article 7)
Lawfulness and principles (Articles 5, 6 and 12)
Territorial scope (Article 3)
Haque et al. [ 41 ] states that use-cases of BC should be retrospectively applied in a way that can be made compliant to GDPR. The literature review also highlighted additional GDPR-BC research domains that include areas such as smart cities, information governance, healthcare, financial data and personal identity.
GDPR vs Blockchain
Vast amounts of PII are being collected, screened, and utilsed illegally due to cyber-espionage, phishing, spamming identity theft, and malpractice. BC on the other hand, due to the immutability in design and utility in tracking, storing and distributing DLT data, can clash with GDPR, especially with the “Right to be forgotten: Article 17”, including various rights to erasure [ 42 ]. Al-Zaben et al., proposes a framework that is on a separate off-chain mechanism that stores PII and non-PII in a different location. It is best to design and regulate network participation in fulfilling GDPR requirements, although not a perfect fit, this example shows how by design, a compliant use-case can be augmented in fulfilling parts of GDPR.
Ransomware Defense vs Blockchain
In [ 43 ], their paper describes that for malicious software to use configuration commands or information, malware has to be able to connect to the original owner. Therefore, a fairly new principle of domain generation is proposed, in that actively deployed ransomware is utilised to track user coordinates based on transactional data in a bitcoin BC. The gives a malware author the ability to dynamically change and update locations of servers in realtime.
Supply Chain Attack vs Blockchain
Recent and alarming increases in supply chain cyber attacks, has given various implementation strategies of BC in security of IoT data, that generally produces positive outcomes due to the transparency and traceability elements inherent in the technology by design. This paper highlights and discusses challenges to include many BC based systems in various industries, and focuses on the pharmaceutical supply chain. In conclusion, [ 44 ] states that the application of BCT can enhance supply chain security via authenticity and confidentiality principles.
Data Storage vs Blockchain
Due to the full-replication data storage mechanism in existing BC technologies, this produces scalability problems due to copying at each node, thus increases overall storage per-block [ 45 ]. Additionally, this mechanism can limit throughput in a permissioned BC. A novel storage system is proposed to enhance scalability by integrating erasure coding that can reduce data acquisition per block and enlarge overall storage capacity.
Of the many challenges that face legal, operational and performance criteria with utilising BC, it is clear to see that as we gather more and more personal data, endure more cyber attacks, and encounter storage disadvantages, many proposed frameworks seek to provide solutions that are only a part of compounding and escalating situation. The transactional speed and scalability of technologies such as BC, can hinder data protection rights, focused cyber-attacks, and the ability to update and track users, however there are advantages in creating separate mechanisms that when produced as a whole, that can indeed support data verification, transparency and accountability in many industries.
Results: Brief Overview of Intelligent Framework
Key Data Management Architecture Components: Fig. 1 shows the block diagram of the proposed framework. Key components of the framework are explained and synthesised in the following paragraphs.
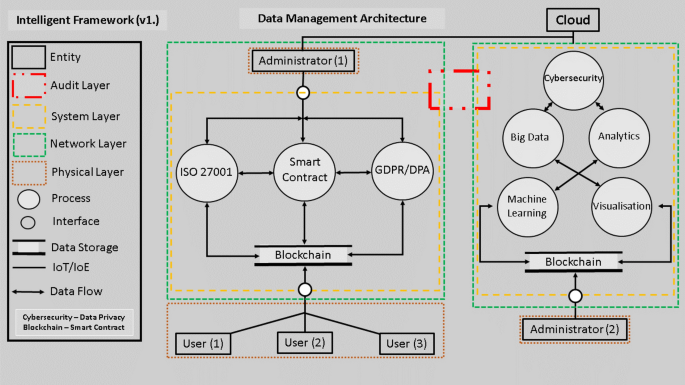
Data flow audit mechanism
Blockchain: Data Storage and Immutability
To provide system accountability, transparency and traceability from network system traffic point of view, an article by Kumar et al., 2020 demonstrates how DLT systems are applied in e-commerce to include health medicines, security devices, food products to ensure BC technological and e-commerce sustainability. Also, [ 46 ] presents a study that explores the potential of DLT in the publication industry and present a technological review. The studies demonstrate how research is being explored and influencing DLTs globally alongside their synergies of application across academic, private and public sectors.
Standardisation of IoT Interface Portal
For purposes of legal acquisition and processing of data with consent, users can connect from IoT smart devices and appliances, such as; smart phones, sensors, tablets and user desktops. User applications and interfaces also provide a level of protection by design in most cases, however the applications can also compound and conflict with each other to produce security vulnerabilities (e.g. Cookies). Networks include; Cellular, Local and Personal Area Networks (PAN/LAN), Low Power Wide Area Networks (LPWAN) and Campus Area Network (CAN) carrier methods operate and maintain IoT system stability. Some IoT devices are capable of ensuring seamless connectivity in data access. However, at the point of access, a user interfaces with a given IoT device could be one of multiple architectures that present challenges in correctly identifying and processing data in a legal, reliable and consistent fashion. Therefore an overarching framework to ensure a standardised system whilst mitigating risk (security Vulnerabilities) is catered for in utilising network protocols with a prescribed profile limited to key information such as, Personal Identification Number (PIN), Account Number and password encryption.
Administrator 1: Public LAN/WLAN/CAN
A main purpose here is the execution of network communication protocols for the processing and or keeping (storage) of PII and data access control to include cryptography. At the level of an SME, the types of regulatory compliance’s necessary to operate as a business include a retrospective and current auditable trail to demonstrate good practices. A selection of operational scenarios are to be emulated (e.g. from case law) in the preparation of codifying, selecting and the setting of chosen principles, standards and legal frameworks. Other objectives to explore include, Confidentiality, Integrity, Availability and Data Minimisation. As shown in [ 47 ], stakeholders are required to initialise and validate a product block, this activates the wallet, to include pseudo-identity generation with a public and private key pair. The keys are utilised for signature and verification processes. Here, administrator 1 oversees and combines the execution of network communication policies to govern a user or a given set of protocols.
Administrator 2: Private LAN Network
The function of the administrator here is to utilise criteria to facilitate accountability, transparency and traceability from network system traffic. Data entry points provide group integrity as each user, or entry, is available for all to see. More fundamentally, this data will help inform, develop, calibrate and test the setting of audit and assessment parameters. The information is then combined, contrasted and compared to the Administrator 1 data collection. Resulting information then updates the Valid Data Acquisition IDPS System and Cyber-Detection Methods (e.g. Packet Sniffing) of Network Packet Data communication protocols with data effective access control. In this case, Administrator 2 provides an array of users insights into the performance of ISO 27001 and DPA/GDPR policies to identify optimum operational cost in various prescribed operating scenarios. Through analysis with tools such as BD Analytics and ML for example, nuanced data, pattern identification and aggregation provides a basis for speculation as to an ideal operating system from within a business.
Smart Contract: Agreement or Terms of Contract
Unfortunately, maintaining these systems incur at significant cost, on the other hand, these systems also cut out the “middle-man” and save resources to empower individuals and business owners. For example, individual and group scenarios are negotiated and interpreted between users in partnerships. In emulating this function, key objectives are identified and embedded from legal frameworks to produce an automatic transaction protocol with consensus in the implementation of a codex (e.g. OPCODES). Therefore, a codex of legal precedent and statutory instrumental data protection, data operation and dissemination laws will be emulated to start. The codex is the library and framework that enables partners to equitably participate in a sustainable and trust-less operational environment. In utilising ISO 27001 for example, a collection of policies are negotiated and agreed upon prior to formally undertaking a contract between parties. Therefore, GDPR and ISO 27001 are transcribed, layered and mapped with verification mechanisms derived from case-law and by design into a SC agreement. This dynamic process forms the centre of any given exchange or process of data acquisition and data dissemination.
To enable an effective cybersecurity strategy for SME’s and alike, government and private sector finance initiatives are key. This includes awareness and training for management, with oversight and additional support for staff to incorporate ML and AI into the workplace more effectively. Intrusion, detection and prevention policy from SME to government level can then flourish in promoting and sustaining the full benefits and protections of cybersecurity from cyber-criminality. However, for global data security coverage, the concept in itself is interpreted differently as the legal, ethical and consensual implementation challenges remain formidable as a result. Acquiring personal data from regional divisions to aide authorities in resource strategy at this scale, requires trust in institutions and technologies to be fully beneficial to all.
Accountability and transparency efforts also require the continual assessment of legal frameworks, systems and outcomes, with generous investment from public and private sectors. Public awareness, perception and confidence levels in the justice system through transparency and education, with focus to include mental illness and minority group engagement policies, can benefit societies substantially. The earlier proposed framework from research, demonstrates a robust and complex strategy, however looking to the future, BC network latency present real-time challenges to assist SME technology adoption. Increasing digitalisation and decentralisation leads to diverse communications, hence creating a wider array of participants to collaborate and share. However, these digital systems are not mature in terms of security and inevitably create attack space for attackers.
In this review paper, we highlighted several security problems that arise in digital systems, computation data and associated trust mechanisms. These challenges have resulted in evolution of technical solutions. Current solutions are so diverse that range from preliminary in small organisations to the state-of-the-art in mega-organisations. The cyber landscape is likely to change even further that necessitates robust solutions. This paper also brings in research from different collaborators with the potential to identify the challenges and move in the direction of designing novel solutions. This we believe as a result, will enhance and lead to secure cyber systems which achieve data security comprehensiveness.
Rawindaran N, Jayal A, Prakash E. Artificial intelligence and machine learning within the context of cyber security used in the UK SME Sector. In: AMI 2021— the 5th advances in management and innovation conference 2021. Cardiff Metropolitan University. 2021.
Wylde V, Prakash E, Hewage C, Jon. Platts. Covid-19 Crisis: Is our Personal Data Likely to be Breached? In AMI 2021 - The 5th Advances in Management and Innovation Conference 2021. Cardiff Metropolitan University, 2021.
Balasubramanian R, Prakash E, Khan I, Platts J. Blockchain technology for healthcare. In: AMI 2021—the 5th advances in management and innovation conference 2021. Cardiff Metropolitan University; 2021.
Gallaher MP, Link AN, Rowe B. Cyber security: economic strategies and public policy alternatives. Chentanham: Edward Elgar Publishing; 2008.
Google Scholar
Zarpelão BB, Miani RS, Kawakani CT, de Alvarenga SC. A survey of intrusion detection in Internet of Things. J Netw Comp Appl. 2017;84:25–37.
Article Google Scholar
Are Your Operational Decisions Data-Driven? 2021. https://www.potentiaco.com/what-is-machine-learning-definition-typesapplications-and-examples/ . Accessed 11 Jul 2021.
Biju SM, Mathew A. Internet of Things (IoT): securing the next frontier in connectivity. ISSN. 2020.
Cahn A, Alfeld S, Barford P, Muthukrishnan S. An empirical study of web cookies. In: Proceedings of the 25th international conference on world wide web; 2016. pp. 891–901.
Cressy R, Olofsson C. European SME Financing: An Overview. Small Business Economics, 1997. pp 87–96.
General Data Protection Regulations (GDPR). https://ico.org.uk/for-organisations/guide-to-dataprotection/guide-to-the-general-data-protectionregulation-gdpr/ . Accessed 16-10-2020.
Roesch M, et al. SNORT: lightweight intrusion detection for networks. Lisa. 1999;99:229–38.
Dunham K, Melnick J. Malicious bots: an inside look into the cyber-criminal underground of the internet. Boca Raton: Auerbach Publications; 2008.
Book Google Scholar
Kabiri P, Ghorbani AA. Research on intrusion detection and response: a survey. Int J Netw Secur. 2005;1(2):84–102.
Fraley JB, Cannady J. The promise of machine learning in cybersecurity. In: SoutheastCon 2017, IEEE; 2017. pp. 1–6.
Buczak AL, Guven E. A survey of data mining and machine learning methods for cyber security intrusion detection. IEEE Commun Surv Tutor. 2015;18(2):1153–76.
Machine learning algorithm cheat sheet for azure machine learning designer. 2021. https://docs.microsoft.com/en-us/azure/machine-learning/algorithm-cheat-sheet . Accessed 3- Mar 2021.
Anthi E, Williams L, Rhode M, Burnap P, Wedgbury A. Adversarial attacks on machine learning cybersecurity defences in industrial control systems. J Inf Secur Appl. 2021;58:102717.
Catak E, Catak FO, Moldsvor A. Adversarial machine learning security problems for 6G: mmWave beam prediction use-case. arXiv:2103.07268 .2021.
Guinchard A. Our digital footprint under Covid-19: should we fear the UK digital contact tracing app? Int Rev Law Comput Technol. 2021;35(1):84–97.
Tran J, Ngoc C. GDPR handbook for record of processing activities. Case: the color club A/S. 2020.
Raman R, Achuthan K, Vinuesa R, Nedungadi P. COVIDTAS COVID-19 tracing app scale-an evaluation framework. Sustainability. 2021;13(5):2912.
Juneidi JS. Covid-19 tracing contacts apps: technical and privacy issues. Int J Adv Soft Comput Appl. 2020;12:3.
Majeed A. Towards privacy paradigm shift due to the pandemic: a brief perspective. Inventions. 2021;6(2):24.
Black M, Lee A, Ford J. Vaccination against COVID-19 and inequalities-avoiding making a bad situation worse. Public health in practice. England: Elsevier; 2021.
Zaeem RN, Barber SK. The effect of the GDPR on privacy policies: recent progress and future promise. ACM Trans Mgmt Inf Syst. 2020;12(1):1–20.
Antal CD, Cioara T, Antal M, Anghel I. Blockchain platform for COVID-19 vaccine supply management. 2021. arXiv:2101.00983 .
How Blockchain is helping in the fight against Covid-19. 2021. https://www.lexology.com/library/detail.aspx?g=8b5ef0f0-05b3-4909-b5d5-da7bd57f0381 . Accessed 24 Apr 2021.
Razai MS, Osama T, McKechnie D, Majeed A. Covid-19 vaccine hesitancy among ethnic minority groups. 2021.
Robertson E, Reeve KS, Niedzwiedz CL, Moore J, Blake M, Green M, Katikireddi SV, Benzeval MJ. Predictors of COVID-19 vaccine hesitancy in the UK Household Longitudinal Study. Brain Behavior Immunity. 2021.
MacKenna B, Curtis HJ, Morton CE, Inglesby P, Walker AJ, Morley J, Mehrkar A, Bacon S, Hickman G, Bates C, et al. Trends, regional variation, and clinical characteristics of COVID-19 vaccine recipients: a retrospective cohort study in 23.4 million patients using OpenSAFELY. 2021.
Zheng Z, Xie S, Dai H, Chen X, Wang H. An overview of blockchain technology: architecture, consensus, and future trends. In: 2017 IEEE international congress on big data (BigData Congress); 2017. pp. 557–64.
Salman T, Zolanvari M, Erbad A, Jain R, Samaka M. Security services using blockchains: a state of the art survey. IEEE Commun Surv Tutor. 2019;21(1):858–80.
Zhang R, Xue R, Liu L. Security and privacy on blockchain. ACM Comput Surv. 2019;52:3.
Pinno OJA, Gregio ARA, De Bona LCE. ControlChain: blockchain as a central enabler for access control authorizations in the IoT. In: GLOBECOM 2017—2017 IEEE global communications conference; 2017. pp. 1–6.
Mandrita B, Junghee L, Choo KKR. A blockchain future for internet of things security: a position paper. Dig Commun Netw. 2018;4(3):149–60.
Kshetri N. Blockchain’s roles in strengthening cybersecurity and protecting privacy. Celebrating 40 years of telecommunications policy—a retrospective and prospective view. Telecommun Policy. 2017;41(10):1027–38.
Ali M, Nelson J, Shea R, Freedman Freedman MJ. Blockstack: a global naming and storage system secured by blockchains. In: 2016 USENIX annual technical conference (USENIX ATC 16), pp 181–194. Denver, CO, 2016. USENIX:Association. 2016.
Shahaab A, Lidgey B, Hewage C, Khan I. Applicability and appropriateness of distributed ledgers consensus protocols in public and private sectors: a systematic review. IEEE Access. 2019;7:43622–36.
Taylor PJ, Dargahi T, Dehghantanha A, Prizi RM, Choo KKR. A systematic literature review of blockchain cybersecurity. Dig Commun Netw. 2020;6(2):147–56.
Alphand O, Amoretti M, Claeys T, Dall’Asta S, Duda A, Ferrari G, Rousseau F, Tourancheau B, Veltri L, Zanichelli F. IoT Chain: a blockchain security architecture for the internet of things. In: 2018 IEEE wireless communications and networking conference (WCNC); 2018. pp. 1–6.
Haque AB, Najmul Islam S, Hyrynsalmi AKM, Naqvi B, Smolander K. GDPR compliant blockchains-a systematic literature review. IEEE Access. 2021;9:50593–606.
Al-Zaben N, Hassan O, Mehedi M, Yang J, Lee NY, Kim CS. General data protection regulation complied blockchain architecture for personally identifiable information management. In: 2018 international conference on computing, electronics communications engineering (iCCECE); 2018. pp. 77–82.
Pletinckx S, Trap C, Doerr C. Malware coordination using the blockchain: an analysis of the cerber ransomware. In: 2018 IEEE conference on communications and network security (CNS); 2018. pp. 1–9.
Johny S, Priyadharsini C. Investigations on the implementation of blockchain technology in supplychain network. In: 2021 7th international conference on advanced computing and communication systems (ICACCS); 2021. pp. 1–6.
Qi X, Zhang Z, Jin C, Zhou A. A reliable storage partition for permissioned blockchain. IEEE Trans Knowl Data Eng. 2021;33(1):14–27.
Paruln K, Gulshan K, Geetha G. Exploring the potential of distributed ledger technology in publication industry—a technological review. In: CEUR Workshop Proceedings. 2021.
Kumar G, Saha R, Buchanan WJ, Geetha G, Thomas R, Rai MK, Kim T, Alazab M. Decentralized accessibility of e-commerce products through blockchain technology. Sustain Cities Soc. 2020;62:102361.
Download references
Author information
Authors and affiliations.
Cardiff School of Technologies, Cardiff Metropolitan University, CF5 2YB, Cardiff, UK
Vinden Wylde, Nisha Rawindaran, John Lawrence, Rushil Balasubramanian, Edmond Prakash, Imtiaz Khan, Chaminda Hewage & Jon Platts
School of Information Systems and Technology, University of Canberra, Bruce, ACT 2617, Australia
Ambikesh Jayal
You can also search for this author in PubMed Google Scholar
Corresponding author
Correspondence to Edmond Prakash .
Ethics declarations
Conflict of interest.
Authors declare that they have no conflicts of interest.
Additional information
Publisher's note.
Springer Nature remains neutral with regard to jurisdictional claims in published maps and institutional affiliations.
This article is part of the topical collection “Cyber Security and Privacy in Communication Networks” guest edited by Rajiv Misra, R. K. Shyamsunder, Alexiei Dingli, Natalie Denk, Omer Rana, Alexander Pfeiffer, Ashok Patel and Nishtha Kesswani.
Rights and permissions
Open Access This article is licensed under a Creative Commons Attribution 4.0 International License, which permits use, sharing, adaptation, distribution and reproduction in any medium or format, as long as you give appropriate credit to the original author(s) and the source, provide a link to the Creative Commons licence, and indicate if changes were made. The images or other third party material in this article are included in the article's Creative Commons licence, unless indicated otherwise in a credit line to the material. If material is not included in the article's Creative Commons licence and your intended use is not permitted by statutory regulation or exceeds the permitted use, you will need to obtain permission directly from the copyright holder. To view a copy of this licence, visit http://creativecommons.org/licenses/by/4.0/ .
Reprints and permissions
About this article
Wylde, V., Rawindaran, N., Lawrence, J. et al. Cybersecurity, Data Privacy and Blockchain: A Review. SN COMPUT. SCI. 3 , 127 (2022). https://doi.org/10.1007/s42979-022-01020-4
Download citation
Received : 04 August 2021
Accepted : 03 January 2022
Published : 12 January 2022
DOI : https://doi.org/10.1007/s42979-022-01020-4
Share this article
Anyone you share the following link with will be able to read this content:
Sorry, a shareable link is not currently available for this article.
Provided by the Springer Nature SharedIt content-sharing initiative
- Data privacy
- Smart Contracts
Advertisement
- Find a journal
- Publish with us
- Track your research
Insights Into Privacy Protection Research in AI
Ieee account.
- Change Username/Password
- Update Address
Purchase Details
- Payment Options
- Order History
- View Purchased Documents
Profile Information
- Communications Preferences
- Profession and Education
- Technical Interests
- US & Canada: +1 800 678 4333
- Worldwide: +1 732 981 0060
- Contact & Support
- About IEEE Xplore
- Accessibility
- Terms of Use
- Nondiscrimination Policy
- Privacy & Opting Out of Cookies
A not-for-profit organization, IEEE is the world's largest technical professional organization dedicated to advancing technology for the benefit of humanity. © Copyright 2024 IEEE - All rights reserved. Use of this web site signifies your agreement to the terms and conditions.
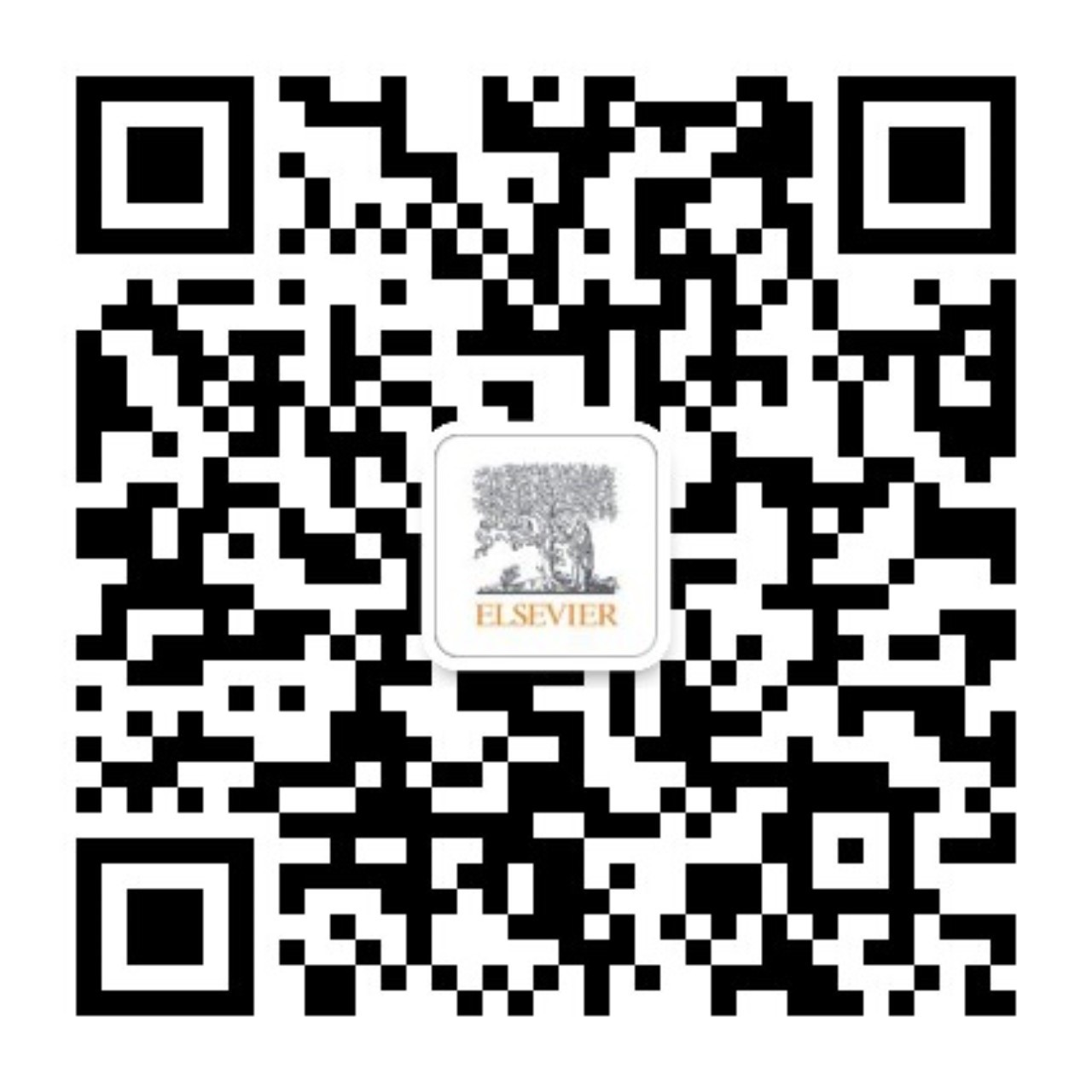
- Research Process
Confidentiality and Data Protection in Research
- 4 minute read
- 44.3K views
Table of Contents
Data protection issues in research remain at the top of researchers’ and institutional awareness, especially in this day and age where confidential information can be hacked and disseminated. When you are conducting research on human beings, whether its clinical trials or psychological inquiries, the importance of privacy and confidentiality cannot be understated. In the past, it was as easy as a lockable file cabinet. But now, it’s more and more challenging to maintain confidentiality and data protection in research.
In this article, we’ll talk about the implications of confidentiality in research, and how to protect privacy and confidentiality in research. We’ll also touch on ways to secure electronically stored data, as well as third-party data protection services.
Data Protection and Confidentiality in Research
How can you protect privacy and confidentiality in research? The answer, in some ways, is quite simple. However, the means of protecting sensitive data can often, by design, be complex.
In the research time, the Principal Investigator is ultimately responsible for the integrity of the stored data. The data protections and confidentiality protocols should be in place before the project starts, and includes aspects like theft, loss or tampering of the data. The easy way to do this is to limit access to the research data. The Principal Investigator should limit access to this information to the fewest individuals possible, including which research team members are authorized to manage and access any data.
For example, any hard-copies of notebooks, questionnaires, surveys and other paper documentation should be kept in a secure location, where there is no public access. A locked file cabinet, away from general access areas of the institution, for instance. Names and other personal information can be coded, with the encoding key kept in a separate and secure location.
It is the Principal Investigator’s responsibility to make sure that every member of the research team is fully trained and educated on the importance of data protection and confidentiality, as well as the procedures and protocols related to private information.
Check more about the Team Structure and Responsibilities .
Implications of Confidentiality in Research
Even if paper copies of questionnaires, notes, etc., are stored in a safe, locked location, typically all of that information is also stored in some type of electronic database. This fulfills the need to have data available for statistical analysis, as well as information accessible for developing conclusions and implications of the research project.
You’ve certainly heard about the multitude of data breaches and hacks that occur, even in highly sophisticated data protection systems. Since research projects can often involve data around human subjects, they can also be a target to hackers. Restoring, reproducing and/or replacing data that’s been stolen, including the time and resources needed to do so, can be prohibitively expensive. That doesn’t even take into consideration the cost to the human subjects themselves.
Therefore, it’s up to the entire research team to ensure that data, especially around the private information of human beings, is strongly protected.
How Can Electronic Data Be Protected?
Frankly, it’s easier said than done to ensure confidentiality and the protection of research data. There are several well-established protocols, however, that can guide you and your team:
- Just like for any hard-copy records, limit who has access to any electronic records to the bare minimum
- Continually evaluate and limit access rights as the project proceeds
- Protect access to data with strong passwords that can’t be easily hacked, and have those passwords change often
- Access to data files should be done through a centralized, protected process
- Most importantly, make sure that wireless devices can’t access your data and your network system
- Protect your data system by updating antivirus software for every computer that has access to the data and confidential information
- If your data system is connected via the cloud, use a very strong firewall, and test it regularly
- Use intrusion detection software to find any unauthorized access to your system
- Utilize encryption software, electronic signatures and/or watermarking to keep track of any changes made to data files and authorship
- Back up any and all electronic databases (on and offsite), and have hard and soft copies of every aspect of your data, analysis, etc.
- When applicable, make sure any data is properly and completely destroyed
Check more about: Why Manage Research Data?
Using Third-Party Data Protection Services
If your institution does not have built-in systems to assure confidentiality and data protection in research, you may want to consider a third party. An outside information technology organization, or a team member specifically tasked to ensure data protection, might be a good idea. Also look into different protections that are often featured within database programs themselves.
Elsevier Author Services
Helping you publish your research is our job. If you need assistance with translating services, proofreading, editing, graphics and illustrations services, look no further than Elsevier Author Services .
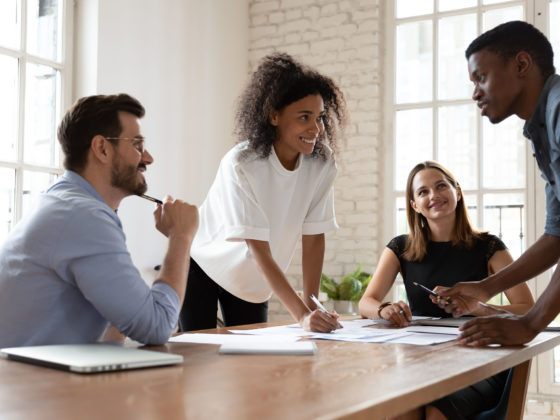
Research Team Structure
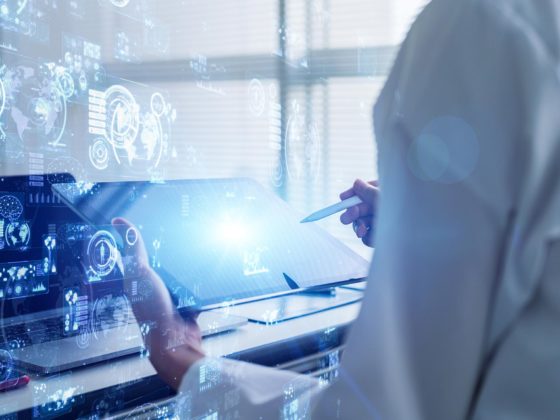
- Publication Process
Research Data Storage and Retention
You may also like.
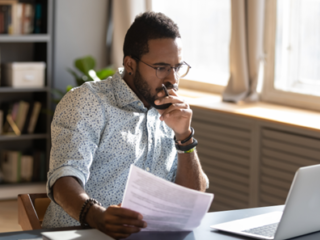
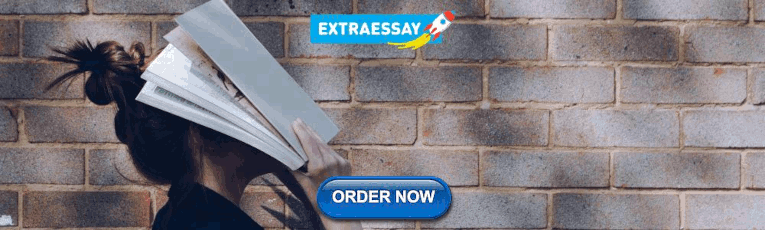
Descriptive Research Design and Its Myriad Uses
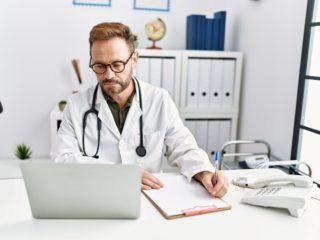
Five Common Mistakes to Avoid When Writing a Biomedical Research Paper
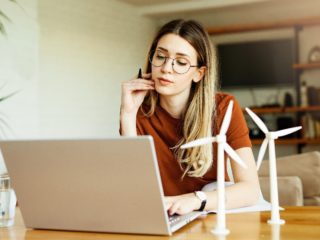
Making Technical Writing in Environmental Engineering Accessible
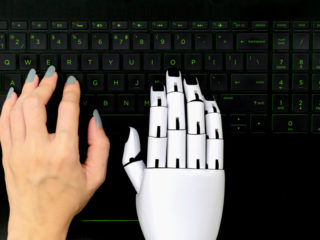
To Err is Not Human: The Dangers of AI-assisted Academic Writing
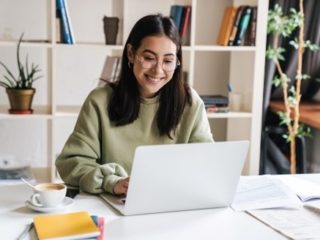
When Data Speak, Listen: Importance of Data Collection and Analysis Methods
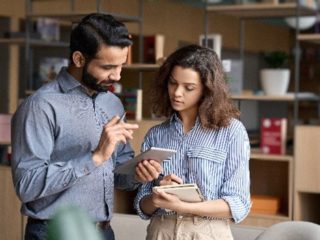
Choosing the Right Research Methodology: A Guide for Researchers
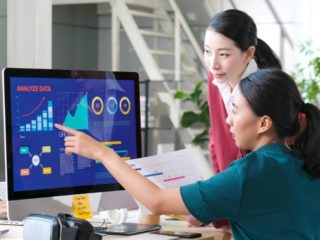
Why is data validation important in research?
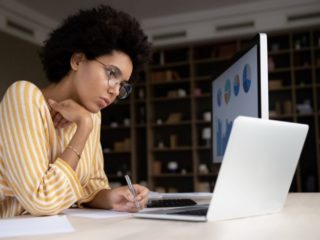
Writing a good review article
Input your search keywords and press Enter.
This paper is in the following e-collection/theme issue:
Published on 29.3.2024 in Vol 26 (2024)
#ProtectOurElders: Analysis of Tweets About Older Asian Americans and Anti-Asian Sentiments During the COVID-19 Pandemic
Authors of this article:

Original Paper
- Reuben Ng 1, 2 , PhD ;
- Nicole Indran 1 , BSocSci (Hons)
1 Lee Kuan Yew School of Public Policy, National University of Singapore, Singapore, Singapore
2 Lloyd's Register Foundation Institute for the Public Understanding of Risk, National University of Singapore, Singapore, Singapore
Corresponding Author:
Reuben Ng, PhD
Lee Kuan Yew School of Public Policy
National University of Singapore
469C Bukit Timah Road
Singapore, 259772
Phone: 65 66013967
Email: [email protected]
Background: A silver lining to the COVID-19 pandemic is that it cast a spotlight on a long-underserved group. The barrage of attacks against older Asian Americans during the crisis galvanized society into assisting them in various ways. On Twitter, now known as X, support for them coalesced around the hashtag #ProtectOurElders. To date, discourse surrounding older Asian Americans has escaped the attention of gerontologists—a gap we seek to fill. Our study serves as a reflection of the level of support that has been extended to older Asian Americans, even as it provides timely insights that will ultimately advance equity for them.
Objective: This study explores the kinds of discourse surrounding older Asian Americans during the COVID-19 crisis, specifically in relation to the surge in anti-Asian sentiments. The following questions guide this study: What types of discourse have emerged in relation to older adults in the Asian American community and the need to support them? How do age and race interact to shape these discourses? What are the implications of these discourses for older Asian Americans?
Methods: We retrieved tweets (N=6099) through 2 search queries. For the first query, we collated tweets with the hashtag #ProtectOurElders. For the second query, we collected tweets with an age-based term, for example, “elderly” or “old(er) adults(s)” and either the hashtag #StopAAPIHate or #StopAsianHate. Tweets were posted from January 1, 2020, to August 1, 2023. After applying the exclusion criteria, the final data set contained 994 tweets. Inductive and deductive approaches informed our qualitative content analysis.
Results: A total of 4 themes emerged, with 50.1% (498/994) of posts framing older Asian Americans as “vulnerable and in need of protection” (theme 1). Tweets in this theme either singled them out as a group in need of protection because of their vulnerable status or discussed initiatives aimed at safeguarding their well-being. Posts in theme 2 (309/994, 31%) positioned them as “heroic and resilient.” Relevant tweets celebrated older Asian Americans for displaying tremendous strength in the face of attack or described them as individuals not to be trifled with. Tweets in theme 3 (102/994, 10.2%) depicted them as “immigrants who have made selfless contributions and sacrifices.” Posts in this section referenced the immense sacrifices made by older Asian Americans as they migrated to the United States, as well as the systemic barriers they had to overcome. Posts in theme 4 (85/994, 8.5%) venerated older Asian Americans as “worthy of honor.”
Conclusions: The COVID-19 crisis had the unintended effect of garnering greater support for older Asian Americans. It is consequential that support be extended to this group not so much by virtue of their perceived vulnerability but more so in view of their boundless contributions and sacrifices.
Introduction
Not unlike other public health crises, the COVID-19 pandemic brought with it a disconcerting onslaught of racism and xenophobia [ 1 ]. The number of anti-Asian hate crimes in the United States quadrupled in 2021, escalating from the already significant uptick it experienced in 2020, when the COVID-19 outbreak was declared a global pandemic [ 2 ]. In the Asian American and Pacific Islanders (AAPI) community, those aged 60 years or older accounted for 7.3% of the 2808 self-reported incidents in 2020 [ 3 ]. Though not a particularly large figure, underreporting in this community is fairly common [ 4 ]. Moreover, older adults have reported being physically assaulted and having to deal with civil rights violations more than the general AAPI community [ 3 ]. When the crisis first emerged, older Asian Americans were beleaguered by increased economic insecurity [ 5 ] and poorer health outcomes [ 6 ] due to a confluence of structural inequities [ 5 ].
A silver lining to the COVID-19 pandemic is that it cast a spotlight on a long-underserved group. The barrage of attacks against older Asian Americans galvanized both individuals and organizations into assisting them in various ways, such as by distributing safety whistles and meal vouchers [ 7 ]. On Twitter, now known as X, support for them coalesced around the hashtag #ProtectOurElders [ 4 ]. The objective of this study is to explore the kinds of discourse surrounding older Asian Americans during the COVID-19 crisis, specifically in relation to the surge in anti-Asian sentiments.
Dating back to the nineteenth century, one of the most pervasive stereotypes of Asian Americans is that they are a high-achieving demographic [ 8 ]. While seemingly innocuous, this myth of them as a “model minority” has been criticized as highly problematic. Not only does it run counter to their lived realities—plenty of evidence has exposed the widespread inequalities confronted by various subgroups within the community [ 8 , 9 ]—it also delegitimizes their struggles and feeds the misconception that they require no assistance whatsoever [ 5 ].
Racial discrimination is well known to be a key social determinant of health [ 6 , 10 ]. Among Asian Americans in the United States, experiences of discrimination are linked to poorer mental health outcomes, including anxiety, depression, hypertension, and elevated blood pressure [ 10 ]. Racism may exacerbate health issues brought about by the aging process, such as the onset of chronic diseases or functional impairment [ 11 ], rendering older Asian Americans more susceptible to detrimental health outcomes.
Studies have indicated that social support has a positive impact on both the mental and physical health of older adults [ 12 ]. Social support likewise serves as a protective buffer against the negative effects of racial discrimination on one’s health [ 13 , 14 ]. The role of social support may be especially critical for Asian Americans. Although the Asian American populace includes a diverse array of ethnicities, cultures, and languages, collectivism appears to be a cultural orientation shared among many Asian American groups [ 15 ]. Evidence revealed that social support improved health outcomes among Asian Americans during the start of the pandemic, when anti-Asian sentiments were rampant [ 14 ].
It is widely acknowledged that in Asian societies, attitudes toward older adults are typically informed by values of respect and filial piety [ 11 , 16 ]. Old age bespeaks knowledge and wisdom, and younger people are expected to honor and respect their older counterparts [ 11 ]. Despite concerns that such values have eroded, there is evidence that they continue to resonate with Asian Americans [ 17 ]. One study concluded that Asian Americans are twice as likely as the general population to care for their parents [ 18 ]. Even so, ageism has been discovered to be pan-cultural [ 19 ]. A meta-analysis comparing Western and Eastern attitudes toward older adults revealed that Easterners actually harbored more negative views of older adults than Westerners [ 20 ]. In this analysis, Western countries included anglophone countries in the West such as Australia, Canada, the United Kingdom, and the United States, as well as Western European countries like Switzerland and France. Eastern countries covered countries in different regions of Asia, such as East Asia, South Asia, and Southeast Asia [ 20 ].
First proposed in 2002, the stereotype content model maintains that people stereotype others on the basis of warmth and competence [ 21 ]. The dimension of warmth includes qualities such as friendliness and sincerity, while the dimension of competence includes traits such as intelligence and skillfulness [ 21 ]. According to the stereotype content model, perceptions of social groups can be categorized into four clusters: (1) warm and competent, (2) incompetent and cold, (3) competent and cold, and (4) warm and incompetent. These 4 combinations of stereotypes produce distinct emotional responses among those who hold them. Groups stereotyped as warm and competent elicit admiration. Those evaluated as incompetent and cold elicit contempt. Groups stereotyped as competent and cold evoke envy. Those evaluated as warm but incompetent evoke pity [ 21 ].
A large body of work has evinced that older adults are generally stereotyped as warm but incompetent [ 21 ]. Although they elicit feelings of admiration occasionally, they predominantly evoke pity. Evidence attests to the universality of these stereotypes in both individualistic and collectivistic societies [ 19 ]. The evaluation of older adults as warm but lacking in competence may lend itself to benevolent ageism—a paternalistic form of prejudice founded on the assumption that older adults are helpless or pitiful [ 22 ]. Benevolent ageism has intensified over the course of the pandemic owing to recurring depictions of older adults as an at-risk group [ 23 ].
Asian Americans—older or otherwise—are one of the most underresearched ethnic groups in peer-reviewed literature [ 24 , 25 ]. In spite of the discomfiting rise in violence directed at them during the COVID-19 outbreak, discourse surrounding older adults from the Asian American community has escaped the attention of gerontologists. Most social media analyses conducted before and during the pandemic have focused on the discursive construction of the older population as a whole [ 26 - 28 ]. Other social media analyses have concentrated on the general Asian American population [ 29 - 31 ]. This study is therefore conceptually significant in that it is the first to dissect the content of tweets posted about older Asian Americans during the COVID-19 crisis.
At the heart of the concept of intersectionality is the notion that various social positions—such as race, age, gender, and socioeconomic status—interact to shape the types of biases one confronts [ 32 ]. From an intersectional standpoint, age and race may converge in ways that worsen the experience of discrimination for older Asian Americans [ 33 ]. In addition to being part of a racial group that faces more systemic challenges compared to White people, older Asian Americans also face age-related hurdles [ 34 ]. In terms of practical significance, this study serves as a reflection of the level of support being extended to older Asian Americans, even as it provides timely insights that will ultimately advance equity for them.
This study pivots around the following questions: What types of discourse have emerged in relation to older Asian Americans and the need to support them? How do age and race interact to shape these discourses? What are the implications of these discourses for older Asian Americans?
We retrieved the data using version 2 of Twitter’s application programming interface (API) [ 35 ], which was accessed through Twitter’s academic research product track [ 36 ]. Compared to what was achievable with the standard version 1.1 API, the version 2 API grants users a higher monthly tweet cap and access to more precise filters [ 37 ].
To build an extensive data set, we collected the tweets using 2 search queries. For both queries, “retweets” were excluded, and only English tweets posted from January 1, 2020, to August 1, 2023, were collated. We excluded retweets to avoid including duplicate content in the data set, which could skew the significance of particular topics. Tweets collected through the first query (n=1549) contained the hashtag #ProtectOurElders. For the second query (n=4550), we gathered tweets that met the following inclusion criteria: (1) contained either the hashtag #StopAAPIHate or #StopAsianHate; (2) included “elder,” “elderly,” “old(er) adult(s),” “old(er) people,” “old(er) person(s),” “senior(s),” “aged,” “old folk(s),” “grandparent(s),” “grandfather(s),” “grandmother(s),” “grandpa,” or “grandma.” The 2 queries yielded a total of 6099 tweets.
We removed posts that were (1) contextually irrelevant, that is, discussed content not pertaining to anti-Asian attacks, such as tweets related to getting vaccinated to protect older people, or tweets related to protecting older adults from cybercrime (n=1384); (2) repeated in the 2 queries (n=20); (3) incorrectly retrieved by the API, that is, they did not fulfill the inclusion criteria of either search query (n=258); and (4) informative, factual, or descriptive (eg, tweets that were newspaper headlines) or that brought up the older person in a tangential fashion (eg, tweets that mentioned older Asian Americans alongside several other groups; n=3443). After applying the aforementioned exclusion criteria, the data set consisted of 994 tweets. Figure 1 provides a flowchart of the data collection process.

Tweet Content Coding
Consistent with past research [ 27 , 38 - 41 ], the codebook was designed through both deductive and inductive modes of reasoning [ 42 ]. Analyses led by a directed or deductive approach begin with the identification of an initial set of codes based on previous literature [ 43 ]. Conversely, in inductive content analyses, codes are derived directly from the data [ 43 ]. We used both deductive and inductive approaches to make sure certain pertinent assumptions guided the analysis while also being aware that new categories would surface inductively [ 42 ].
To create a preliminary codebook, we first identified a set of categories based on previous literature regarding the perceptions of older adults in Asia [ 44 ]. The content analysis was subsequently conducted in several stages, with each tweet read twice by 2 researchers trained in gerontology to ensure familiarity with and immersion in the data [ 43 ]. The goal of the first reading was to ascertain the validity of the initial set of categories as well as to generate codes systematically across the whole data set. Each researcher modified the codebook independently until all variables were refined and clearly defined. During this first reading, a new category was added whenever a post featured a particular trait that could not be suitably coded into any of the existing categories and which was recurrent in the data. During the second reading, the 2 coders had frequent discussions where any discrepancies were reviewed and adjudicated to ensure rigor in the analysis. At this point, both coders discussed what the codes meant, confirmed the relevance of the codes to the research question, and identified areas of significant overlap to finalize the coding rubric.
The percentage agreement between the 2 raters was 92.5% with a weighted Cohen κ of 0.89 (P<.001), indicating high interrater reliability. A total of 4 themes emerged from the whole process. The frequency of each theme was identified after the analysis. As mentioned in past scholarship, categories in a content analysis need not be mutually exclusive, although they should be internally homogeneous (ie, coherent within themes) and externally heterogeneous (ie, distinct from each other) as far as possible [ 27 , 45 ].
Ethical Considerations
Ethical approval was not deemed necessary for this study, as all the data used were publicly available and anonymized.
Summary of Insights From Content Analysis of Tweets
A total of 4 themes emerged from our content analysis of 994 tweets. Half of the posts (498/994, 50.1%) were filed under the theme “vulnerable and in need of protection” (theme 1). Tweets in this theme either singled out older Asian Americans as a group in need of protection because of their vulnerable status or discussed initiatives aimed at safeguarding their well-being. The theme “heroic and resilient” (theme 2) was present in 31.1% (309/994) of the posts. Relevant tweets celebrated older Asian Americans for displaying tremendous strength in the face of attack or described them as individuals not to be trifled with. The theme “immigrants who have made selfless contributions and sacrifices” (theme 3) appeared in 10.2% (102/994) of the posts. Posts in this section referenced the immense sacrifices made by older Asian Americans as they migrated to the United States, as well as the systemic barriers they had to overcome. Theme 4 “worthy of honor” (85/994, 8.5%) consisted of tweets that venerated older Asian Americans. Textbox 1 provides a summary of the themes.
Vulnerable and in need of protection (498/994, 50.1%)
- “Isn't it so cowardly that they attack the elderly mostly? Not that violence is acceptable for any age, but to hurt the defenseless only means they got loose screws. #StopAsianHate”
- “Conducting walking patrols everyday to protect our elders and community #StopAAPIHate #HateisaVirus #StopAsianHate #SFChinatown #SafeNeighborhood #ProtectOurElders #TogetherWeCan”
Heroic and resilient (309/994, 31.1%)
- “Underestimating the terror wrought by old Chinese ladies with sticks was his first mistake #grannygoals #StopAsianHate”
- “Don't mess with Asian grandmas. But also sad this is happening. #StopAsianHate #StopAAPIHate”
Immigrants who have made selfless contributions and sacrifices (102/994, 10.2%)
- “Come to America they said..
It's the land of Opportunities they said...
Feeling so sad seeing this video 2 underage over privileged girls get to do this to a man ,a father ,a grandfather and not even have their identities revealed ...devastating
#MuhammadAnwar #StopAsianHate”
- “These are my grandparents. They came to America to build a new life. (That's my dad on the right wearing a tie.) My grandfather was a very well respected doctor in the Chinese community. America is built on the backbone of hard-working immigrants. #StopAsianHate”
Worthy of honor (85/994, 8.5%)
- “What's been shocking to me about these increased attacks on #AAPI is how often the elderly have been the focus. It’s such a shock because one thing that has been common amongst #AAPI culture is the reverence/respect of elders. #StopAAPIHate #StopAsianHate”
- “It really makes me weak and cry seeing videos of those elderly being hit and hurt. We, Asians, value and esteem our elderly. We even live with them in the same house, take care of them. I can't imagine how someone can simply push them. Just like that. #StopAsianHate”
Theme 1: Vulnerable and in Need of Protection
The vulnerability of older adults was a throughline in this category (498/994, 50.1%). Although concern was directed at the entire Asian American population, older adults were singled out as deserving of more sympathy because of their advanced age. Adjectives commonly used to frame them include “infirm,” “weak,” “defenseless,” and “powerless.” A person described them as lacking “the strength to even unclasp a grip.” Sympathy for older adults was magnified in view of other challenges they had been confronting since the outbreak of COVID-19. For instance, one poster expressed sorrow over how older Asian Americans had to grapple with the “fear of getting attacked” on top of “already [being] really afraid of COVID-19 because it disproportionately affects” them.
What made the act “especially egregious” in the eyes of many was the fact that assailants targeted older adults of all people. Users lambasted attackers for their “coward[ice],” asserting that they should have “picked on someone [their] own size” instead of attacking “people who can’t even defend themselves.” Several posters insisted that it was incumbent upon society to “be watchdogs” for older adults since they are more vulnerable.
A large number of tweets featured a call-to-action aimed at mobilizing members of the Twitter community to assist older Asian Americans. Fundraising campaigns were conducted to raise money for “alarms and pepper spray” for older Asian Americans. Others lobbied for donations to causes that deliver food to this group. The following tweet is one such example: “Wondering how you can support elderly Asians and show you will not tolerate #Asianhate? Join me in making a contribution to @heartofdinner, which brings food to elderly Asians in NYC so they can eat safely in their homes #StopAsianHateCrimes #StopAAPIHate.” The Twitter audience was also invited to escort older persons who walk alone: “United Peace Collaborative protects the #SF Chinatown community with daily walking patrols, providing protection & assistance to the elderly & residents. Please join us & volunteer!”
There were many tweets concerning the suite of initiatives aimed at supporting older Asian Americans. The Yellow Whistle—a campaign involving the distribution of free whistles for Asian Americans to signal danger in the event of an assault—was held up as one such example to “keep older Asian Americans safe.” Select community partners also received plaudits for their “wonderful work in distributing and training use of the alarms to” older persons.
Theme 2: Heroic and Resilient
Tweets in this theme (309/994, 31.1%) mainly revolved around a high-profile incident in San Francisco in which Xiao Zhen Xie, an older woman of Asian descent, put her assailant on a stretcher in an unexpected turn of events. She earned kudos from the Twitter community for “hold[ing] her ground,” “fighting back,” and sending him “to the hospital with his face bloodied.” Many saluted her for being “feisty,” “resilient,” and “[as] tough as nails,” dubbing her a “hero” who made them feel “#HonoredToBeAsian.” One user used the hashtag “#GrannyGoals,” quipping that the attacker made a “mistake” “underestimating the terror” that “old Chinese ladies” could wreak. Xiao Zhen Xie was also applauded for “refusing to be a statistic” as well as for defying the image of older adults as a group most expect “not to fight back.”
This episode involving Xiao Zhen Xie set in motion a series of tweets in which users warned others not to get on their grandparents’ bad side. A user cautioned that the incident was a lesson to everyone not to “mess with ahjummas, lolas, and all the elderly Asian women.” Another claimed that Asian grandmothers possess a special kind of “Asian grandma strength.” Some took the opportunity to underline the importance of not belittling older adults, with one in particular commenting on how his or her grandparents embodied grit and “toughness” because they “lived through war.”
Besides Xiao Zhen Xie, a few other older Asian Americans were celebrated for their resilience. A Filipina immigrant, Vilma Kari, was lauded for saying she “forgives” and “prays” for her attacker. A handful of tweets focused on a group of older Asian Americans who made the headlines for having filmed a music video in which they condemned the racially motivated acts of violence targeting their community.
Theme 3: Immigrants Who Have Made Selfless Contributions and Sacrifices
Members of the Twitter community frequently shared stories of their grandparents’ immigration (102/994, 10.2%). A common thread running through these posts was that their forefathers made immense sacrifices, uprooting themselves to move to the United States in order that their children might receive “the best education they can get” and “enjoy a “better future.” A user portrayed his or her grandmother as a “fighter” who “worked two to three jobs” while struggling to acculturate in a new society at a time when she knew “very little English.”
Attention was drawn to how the string of attacks against Asian Americans was ironic given the national ethos of the country commonly touted as the “American dream.” A few posters implied that labeling the United States as a “land of opportunity” was a misnomer: “Come to America,’ they said... ‘It’s the land of opportunities,’ they said...” A user said that the Asian “elderly did not escape communism” only to become a target of racism.
Tweets in this theme also discussed the burden of racism that older Asian Americans had endured before the COVID-19 pandemic. Users commented on their grandparents’ day-to-day experiences of racial discrimination. A handful were dismayed by how their grandparents were survivors of “prejudice and xenophobia” during World War II when they were forcibly relocated to Japanese internment camps. Others bemoaned that their older family members were “imprisoned for being the wrong-colored Americans.” One user deplored the fact that his or her grandfather “could not come to [the United States] because of his race” due to the Chinese Exclusion Act of 1882, a law that suspended Chinese immigration for 10 years and declared Chinese immigrants ineligible for naturalization. Another poster pinpointed how his or her grandfather felt compelled to dress in an “extremely patriotic” manner in order to camouflage his Asian identity and better assimilate into America.
Users considered older Asian Americans as foundational to the growth of America and foregrounded the need to acknowledge that “America is built on the backbone of hardworking immigrants,” who “made 90%” of what society has. Examples of contributions made by those of Asian ancestry include how they “oversaw” the construction of the transcontinental railroad in the “Old West” as well as their “service in the #442RCT (442nd Infantry Regiment)”—a highly decorated infantry regiment that mainly comprised second-generation American soldiers of Japanese descent who served in World War II. One user mentioned Chien-Shiung Wu, a groundbreaking Chinese American physicist whose scientific accomplishments were a core part of “U.S. WW II efforts” and that “helped win Nobel Prizes for Americans,” without which the “country would be so much worse off.” Artworks inspired by “hustling, elderly Asian folks” were also broadcasted under a hashtag that deified them as “#ChinatownGods.”
Several attempts were made to deconstruct the myth of the model minority. Individuals were aggrieved at how the looming specter of anti-Asian violence compounded the plight of older Asian Americans, who had already been dealt multiple blows during the COVID-19 crisis. These posters raised awareness of how many of them are in “precarious living situations” or “working in low-wage jobs.” Some pleaded for the Asian American community to be seen and understood, as captured in the following tweet: “See what’s happening to our elderly and community. Understand us. Understand why no matter how model of a minority we seem to be... we are just like you. #StopAsianHate #StopAAPIHate #StandWithAsians.”
Theme 4: Worthy of Honor
Many users (85/994, 8.5%) were outraged at how older adults appeared to be prime targets of violence against the Asian American community, perceiving these acts as a flagrant transgression of Asian cultural mores that “revere” them as “the most important people” in society. Some tweets exalted them as wellsprings of “wisdom” and “thoughtful guidance”—one user even likened them to “gold”—to “value and esteem.” Tweets in this theme also alluded to how deference to the older community was practically nonnegotiable in the Asian household. A poster tweeted, “No one should be assaulted, especially the elderly. I grew up respecting my elders. You never even argued with them ... They pass on wisdom.”
Values of collectivism were prized by certain users. These posters made reference to the notion of intergenerational reciprocity by stressing that younger people had an obligation to “protect” the older generation in return. The idea of solidarity was also raised. For instance, some viewed the attack of an older adult—related or otherwise—as an affront to the entire Asian community: “Many are saying ‘she could've been MY grandma.’ To that I say, she is ALL OUR GRANDMAS. Fight hate, love justice, stand with our elders always. #ForTheLoveOfLolas #StopAsianHate #StopAAPIHate #StopAsianHateCrimes.”
This study serves as a substantive first step in understanding discourses surrounding older Asian Americans. In our content analysis of tweets posted about the rash of attacks targeting them during the COVID-19 crisis, 4 main discourses surfaced. The first positioned them as “vulnerable and in need of protection” (theme 1). The second characterized them as “heroic and resilient” (theme 2). The third portrayed them as “immigrants who have made selfless contributions and sacrifices” (theme 3), and the fourth extolled them as “worthy of honor” (theme 4).
Our findings demonstrate an outpouring of support for the older Asian American community, which manifested itself in various local initiatives such as the distribution of safety whistles and the delivery of food. Scholars have drawn attention to how social support is particularly crucial for those in their later years [ 12 ] as well as those who experience racial discrimination [ 13 , 14 ]. The fact that older Asian Americans are finally being given support and assistance is therefore a step in the right direction.
However, even well-intentioned acts may be met with negative repercussions. In the wake of the COVID-19 crisis, older adults were reduced to a uniform group of at-risk individuals [ 46 ]. Assumptions of their vulnerability led to paternalistic behaviors, which denied them their autonomy [ 23 ]. Our results indicate that the rise in violence toward older Asian Americans sparked much-needed dialogue regarding their everyday struggles. Nevertheless, an unfortunate corollary is that this may have predisposed them to being recipients of benevolent prejudice on the basis of both age and race. Older Asian Americans may have been viewed as especially defenseless or vulnerable, perhaps more so than the general older population. This was made amply clear in the findings, where half of the tweets branded older Asian Americans as “weak” and “powerless.”
Notwithstanding concerns that Asian values of respect and filial piety have become irrelevant in the face of modernization [ 17 ], findings from themes 2-4 show emphatically that older adults retain their revered status, at least among some in the Asian American community. Tweets in theme 2 featured users enthusing over the way Xiao Zhen Xie held her ground when she was attacked in San Francisco, which led to deliberations on the strength and tenacity of older Asian women in general. Discourses of gratitude emerged in theme 3 as users ruminated over the sacrifices their forefathers had made in migrating to the United States, as well as the attendant systemic challenges they had to navigate. Posts in theme 4 indicate that users perceived the violence against older Asian Americans as a contravention of cultural norms, which emphasize the importance of honoring older adults. These provide a countervailing force to the various ageist tropes that came to the fore during the COVID-19 pandemic, such as the #BoomerRemover hashtag, which saw the lives of older people being discounted [ 27 , 28 ].
Theoretical Contribution and Implications
Findings from this study show that during the COVID-19 pandemic, age and race interfaced in complex ways to shape discourses on older Asian Americans. Specifically, our content analysis demonstrates that the stereotypes of warmth and incompetence, which are often thought to shape evaluations of older adults, cannot be applied indiscriminately to older Asian Americans as a subcategory of the older demographic. Theme 1, which positions older Asian Americans as vulnerable and in need of protection, does indeed align with traditional evaluations of older adults as warm and incompetent. However, the remaining themes celebrate older Asian Americans for their numerous contributions to society, the sacrifices they have made, and their unwavering resilience during the pandemic, all of which challenge the stereotype of incompetence under the stereotype content model. These findings add complexity to the commonly held notion of older adults as a pitiful social group by highlighting that older Asian Americans evoke not just pity but also admiration. The stereotype content model should therefore be expanded or modified in a way that accounts for attitudes toward older adults of different ethnicities.
Additionally, gerontological scholarship would benefit from a cross-cultural analysis of benevolent ageism. At present, little is known about how displays of benevolent ageism are affected by cultural norms of parental respect and filial piety and the extent to which these norms affect one’s perception of an older adult’s competence. Several studies have been conducted to make sense of ageism in different cultures [ 47 , 48 ], but there has been limited research on the cross-cultural differences in benevolent ageism specifically. The ways in which evaluations of older Asian Americans may be complicated by the deeply ingrained myth of the model minority as well as the pandemic-induced rise in anti-Asian hate are important avenues for future study.
This study has a number of implications for policy and practice. First, although care toward one’s parents or grandparents is not the prerogative of Asians [ 49 ], Asia’s adherence to collectivism nonetheless offers a useful learning point for the West. Many of the posters were Asian Americans, who held older adults in high regard, whether related or otherwise. Fostering a cultural emphasis on solidarity and interconnectedness in the West may promote respect not only for one’s parents but also for older adults outside of one’s family [ 44 ]. Second, ongoing efforts to reframe aging [ 50 ] could highlight the need to respect older adults, not in a way that advances their supremacy or absolves them from wrongdoing, but in a way that teaches society to view them as people whose experience may render them wise and worth learning from. Educators could also incorporate lessons on age-related stereotypes in schools to guard against the formation of ageist beliefs [ 51 ].
Third, current moves to redress the longstanding omission of Asian American history from national curricula [ 52 ] should ensure that students in every state are taught about the sacrifices, struggles, and contributions of older Asian Americans. Public campaigns could be organized as well to raise awareness of the aforementioned. This will help counter the myth of the model minority and get more people to acknowledge older Asian Americans as a significant part of America’s social fabric. Fourth, our findings underscore the need to reflect on the diversity of the older population in terms of socioeconomic status. Older adults—particularly those from the baby boomer generation—have been stereotyped as having made significant financial gains compared to their predecessors, at times even seen as stealing resources from the young [ 53 ]. However, as highlighted by some of the Twitter users as well as scholars, many older Asian Americans are in dire economic straits [ 5 ]. Rectifying the structural inequities that have contributed to their immiseration should hence be a key component of the agenda moving forward.
There are limitations inherent in this study. First, we acknowledge that Twitter users might not be representative of the wider population and that only publicly available tweets were included in the data set. Some of the users whose tweets were included in the study appeared to be Asian Americans, who are likely to be more passionate about supporting individuals in their community. Relatedly, as we did not collect information regarding users’ demographics—not all users publish demographic information, and there are certain limitations to using publicly provided demographic information on social media [ 54 ]—we could not contextualize the motivations of those whose tweets were included in the analysis. Ultimately, social support for older Asian Americans—whether from the Asian American community or society as a whole—has important implications for their well-being [ 14 ]. Subsequent research could focus on conducting interviews among individuals from different ethnic groups to tease out any differences in the level of support extended to older Asian Americans.
Second, we queried the hashtag #StopAAPIHate as a way to understand sentiments toward Asian Americans, even though the term “AAPI” refers to 2 different racial groups: Asian Americans and Pacific Islanders. As the tweets analyzed paid more attention to older Asian Americans, we were not able to offer insight into the types of discourses that emerged in relation to older Pacific Islanders. Future studies are needed to expound on such discourses. Third, it is vital to highlight that both the Asian American community and the older population are heterogeneous. The Asian American community encompasses numerous ethnicities, all with distinct languages, cultures, immigration histories, values, and beliefs [ 34 ]. The older demographic, too, is a diverse group composed of people with vastly different health trajectories [ 55 ]. Given the brevity of the tweets uploaded, we were unable to assess how discourses on older Asian Americans vary across different ethnicities. Finally, we collected only textual data, although tweets often contain visual elements such as photos, videos, and GIFs. This is a drawback that can be overcome in the future when multimodal techniques are developed to analyze both textual and visual content on Twitter.
Another direction for future inquiry involves an assessment of how discourses surrounding older Asian Americans have changed over time. The level of support shown to this group is likely to fluctuate over time, depending on the frequency at which anti-Asian attacks are reported in the news as well as other types of news being covered. Sentiment and narrative analyses [ 56 - 58 ] could be performed to glean such insights.
Even as older Asian Americans contended with a rise in racism alongside other struggles during the COVID-19 pandemic, our findings reveal that the crisis had the unintended effect of garnering greater support for this group. In the future, it is important that support be extended to older Asian Americans not so much by virtue of their perceived vulnerability but more so in view of their boundless contributions and sacrifices.
Acknowledgments
The authors would like to thank L Liu for preprocessing the data. We gratefully acknowledge support from the Social Science Research Council SSHR Fellowship (MOE2018-SSHR-004). The funder had no role in study design, data collection, analysis, writing, or the decision to publish this study.
Data Accessibility
Data are publicly available on Twitter [ 59 ].
Authors' Contributions
RN designed the study, developed the methodology, analyzed the data, wrote the paper, acquired the funding. RN and NI analyzed the data and wrote the paper.
Conflicts of Interest
None declared.
- Elias A, Ben J, Mansouri F, Paradies Y. Racism and nationalism during and beyond the COVID-19 pandemic. Ethn Racial Stud. 2021;44(5):783-793. [ CrossRef ]
- Yamauchi N. Anti-Asian hate crimes quadrupled in U.S. last year. Nikkei Asia. 2022. URL: https://asia.nikkei.com/Spotlight/Society/Anti-Asian-hate-crimes-quadrupled-in-U.S.-last-year [accessed 2022-02-16]
- Turton N. Stop AAPI Hate: new data on anti-Asian hate incidents against elderly and total national incidents in 2020. Stop AAPI Hate. 2021. URL: https://stopaapihate.org/wp-content/uploads/2021/04/Stop-AAPI-Hate-Press-Statement-Bay-Area-Elderly-Incidents-210209.pdf [accessed 2024-02-23]
- Huang J. How the #ProtectOurElders movement helped create a wave of first-time Asian American Activists. LAist. 2021. URL: https://laist.com/news/how-protectourelders-helped-created-a-wave-of-first-time-asian-american-activists [accessed 2022-04-03]
- Ma KPK, Bacong AM, Kwon SC, Yi SS, Ðoàn LN. The impact of structural inequities on older Asian Americans during COVID-19. Front Public Health. 2021;9:690014. [ FREE Full text ] [ CrossRef ] [ Medline ]
- Chen JA, Zhang E, Liu CH. Potential impact of COVID-19-related racial discrimination on the health of Asian Americans. Am J Public Health. 2020;110(11):1624-1627. [ CrossRef ] [ Medline ]
- Lee E. Asian American community rallies to support elders. AARP. 2021. URL: https://www.aarp.org/home-family/friends-family/info-2021/asian-american-support-communities.html [accessed 2022-02-16]
- Yi V, Museus S. Model minority myth. In: Smith AD, Hou X, Stone J, Dennis RM, Rizova P, editors. The Wiley Blackwell Encyclopedia of Race, Ethnicity, and Nationalism. Oxford, UK. John Wiley & Sons, Ltd; 2015.
- Li G, Wang L. Model Minority Myth Revisited: An Interdisciplinary Approach to Demystifying Asian American Educational Experiences. Charlotte, NC. Information Age Publishing; 2008.
- Paradies Y, Ben J, Denson N, Elias A, Priest N, Pieterse A, et al. Racism as a determinant of health: a systematic review and meta-analysis. PLoS One. 2015;10(9):e0138511. [ FREE Full text ] [ CrossRef ] [ Medline ]
- Min J, Moon A. Older Asian Americans. In: Berkman B, D'Ambruoso S, editors. Handbook of Social Work in Health and Aging. New York, NY. Oxford University Press; 2006.
- Antonucci TC, Ajrouch KJ, Birditt KS. The convoy model: explaining social relations from a multidisciplinary perspective. Gerontologist. 2014;54(1):82-92. [ FREE Full text ] [ CrossRef ] [ Medline ]
- Ajrouch KJ, Reisine S, Lim S, Sohn W, Ismail A. Perceived everyday discrimination and psychological distress: does social support matter? Ethn Health. 2010;15(4):417-434. [ FREE Full text ] [ CrossRef ] [ Medline ]
- Lee S, Waters SF. Asians and Asian Americans’ experiences of racial discrimination during the COVID-19 pandemic: impacts on health outcomes and the buffering role of social support. Stig Health. Feb 2021;6(1):70-78. [ CrossRef ]
- Markus HR, Kitayama S. The cultural construction of self and emotion: implications for social behavior. In: Emotion and Culture: Empirical Studies of Mutual Influence. Washington, DC. American Psychological Association; 1994;89-130.
- Ingersoll-Dayton B, Saengtienchai C. Respect for the elderly in Asia: stability and change. Int J Aging Hum Dev. 1999;48(2):113-130. [ CrossRef ] [ Medline ]
- Harrington B. "It's more us helping them instead of them helping us": how class disadvantage motivates Asian American college students to help their parents. J Fam Issues. 2022;44(7):1773-1795. [ CrossRef ]
- Montenegro X. Caregiving among Asian Americans and Pacific Islanders age 50+. AARP Research. 2014. URL: https://www.aarp.org/pri/topics/ltss/family-caregiving/caregiving-asian-americans-pacific-islanders/ [accessed 2024-02-23]
- Cuddy AJC, Norton MI, Fiske ST. This old stereotype: the pervasiveness and persistence of the elderly stereotype. J Social Issues. 2005;61(2):267-285. [ CrossRef ]
- North MS, Fiske ST. Modern attitudes toward older adults in the aging world: a cross-cultural meta-analysis. Psychol Bull. 2015;141(5):993-1021. [ CrossRef ] [ Medline ]
- Fiske ST, Cuddy AJC, Glick P, Xu J. A model of (often mixed) stereotype content: competence and warmth respectively follow from perceived status and competition. J Pers Soc Psychol. 2002;82(6):878-902. [ CrossRef ]
- Cary LA, Chasteen AL, Remedios J. The ambivalent ageism scale: developing and validating a scale to measure benevolent and hostile ageism. Gerontologist. 2017;57(2):e27-e36. [ FREE Full text ] [ CrossRef ] [ Medline ]
- Vervaecke D, Meisner B. Caremongering and assumptions of need: the spread of compassionate ageism during COVID-19. Gerontologist. 2021;61(2):159-165. [ FREE Full text ] [ CrossRef ] [ Medline ]
- Yi SS. Taking action to improve Asian American Health. Am J Public Health. 2020;110(4):435-437. [ CrossRef ] [ Medline ]
- Ðoàn LN, Takata Y, Sakuma KLK, Irvin VL. Trends in clinical research including Asian American, Native Hawaiian, and Pacific Islander participants funded by the US National Institutes of Health, 1992 to 2018. JAMA Netw Open. 2019;2(7):e197432. [ FREE Full text ] [ CrossRef ] [ Medline ]
- Levy BR, Chung PH, Bedford T, Navrazhina K. Facebook as a site for negative age stereotypes. Gerontologist. 2014;54(2):172-176. [ FREE Full text ] [ CrossRef ] [ Medline ]
- Sipocz D, Freeman JD, Elton J. "A toxic trend?": generational conflict and connectivity in Twitter discourse under the #BoomerRemover hashtag. Gerontologist. 2021;61(2):166-175. [ FREE Full text ] [ CrossRef ] [ Medline ]
- Skipper AD, Rose DJ. #BoomerRemover: COVID-19, ageism, and the intergenerational twitter response. J Aging Stud. 2021;57:100929. [ FREE Full text ] [ CrossRef ] [ Medline ]
- Hswen Y, Xu X, Hing A, Hawkins JB, Brownstein JS, Gee GC. Association of "#covid19" versus "#chinesevirus" with Anti-Asian sentiments on Twitter: march 9-23, 2020. Am J Public Health. 2021;111(5):956-964. [ FREE Full text ] [ CrossRef ] [ Medline ]
- Nguyen TT, Criss S, Dwivedi P, Huang D, Keralis J, Hsu E, et al. Exploring U.S. shifts in Anti-Asian sentiment with the emergence of COVID-19. Int J Environ Res Public Health. 2020;17(19):7032. [ FREE Full text ] [ CrossRef ] [ Medline ]
- Darling-Hammond S, Michaels EK, Allen AM, Chae DH, Thomas MD, Nguyen TT, et al. After "The China Virus" went viral: racially charged coronavirus coverage and trends in bias against Asian Americans. Health Educ Behav. 2020;47(6):870-879. [ FREE Full text ] [ CrossRef ] [ Medline ]
- Krekula C, Nikander P, Wilińska M. Multiple marginalizations based on age: gendered ageism and beyond. In: Ayalon L, Tesch-Römer C, editors. Contemporary Perspectives on Ageism. Cham, Switzerland. Springer Open; 2018;33-50.
- Gutterman AS. Ageism, race and ethnicity. SSRN Journal. Preprint posted online on December 8 2021. 2021. [ FREE Full text ] [ CrossRef ]
- Kim G, Wang SY, Park S, Yun SW. Mental health of Asian American older adults: contemporary issues and future directions. Innov Aging. 2020;4(5):igaa037. [ FREE Full text ] [ CrossRef ] [ Medline ]
- Twitter API v2: early access. Twitter. 2021. URL: https://developer.twitter.com/en/docs/twitter-api/early-access [accessed 2021-10-13]
- Tornes A, Trujillo L. Enabling the future of academic research with the Twitter API. Twitter Developer Platform Blog. 2021. URL: https://blog.twitter.com/developer/en_us/topics/tools/2021/enabling-the-future-of-academic-research-with-the-twitter-api [accessed 2021-10-13]
- Barrie C, Ho JC. academictwitteR: an R package to access the Twitter Academic Research Product Track v2 API endpoint. JOSS. 2021;6(62):3272. [ FREE Full text ] [ CrossRef ]
- Ng R, Indran N. Does age matter? Tweets about gerontocracy in the United States. J Gerontol B Psychol Sci Soc Sci. 2023;78(11):1870-1878. [ CrossRef ] [ Medline ]
- Ng R, Indran N. Innovations for an aging society through the lens of patent data. Gerontologist. 2024;64(2). [ FREE Full text ] [ CrossRef ] [ Medline ]
- Ng R, Indran N. Not too old for TikTok: how older adults are reframing aging. Gerontologist. 2022;62(8):1207-1216. [ FREE Full text ] [ CrossRef ] [ Medline ]
- Ng R, Indran N. Age advocacy on Twitter over 12 years. Gerontologist. 2024;64(1). [ CrossRef ] [ Medline ]
- Armat MR, Assarroudi A, Rad M, Sharifi H, Heydari A. Inductive and deductive: ambiguous labels in qualitative content analysis. TQR. 2018;23(1):219-221. [ FREE Full text ] [ CrossRef ]
- Hsieh HF, Shannon SE. Three approaches to qualitative content analysis. Qual Health Res. 2005;15(9):1277-1288. [ CrossRef ] [ Medline ]
- Hwang KK. Filial piety and loyalty: two types of social identification in Confucianism. Asian J of Social Psycho. 2002;2(1):163-183. [ CrossRef ]
- Bengtsson M. How to plan and perform a qualitative study using content analysis. NursingPlus Open. 2016;2:8-14. [ FREE Full text ] [ CrossRef ]
- Ayalon L. There is nothing new under the sun: ageism and intergenerational tension in the age of the COVID-19 outbreak. Int Psychogeriatr. 2020;32(10):1221-1224. [ FREE Full text ] [ CrossRef ] [ Medline ]
- Ng R, Lim-Soh JW. Ageism linked to culture, not demographics: evidence from an 8-billion-word corpus across 20 countries. J Gerontol B Psychol Sci Soc Sci. 2021;76(9):1791-1798. [ FREE Full text ] [ CrossRef ] [ Medline ]
- Löckenhoff CE, De Fruyt F, Terracciano A, McCrae RR, De Bolle M, Costa PT, et al. Perceptions of aging across 26 cultures and their culture-level associates. Psychol Aging. 2009;24(4):941-954. [ FREE Full text ] [ CrossRef ] [ Medline ]
- Lim AJ, Lau CYH, Cheng CY. Applying the Dual Filial Piety Model in the United States: a comparison of filial piety between Asian Americans and Caucasian Americans. Front Psychol. 2021;12:786609. [ FREE Full text ] [ CrossRef ] [ Medline ]
- Sweetland J, Volmert A, O'Neil M. Finding the frame: an empirical approach to reframing aging and ageism. FrameWorks Institute. Washington, DC.; 2017. URL: https://www.frameworksinstitute.org/wp-content/uploads/2020/05/aging_research_report_final_2017.pdf [accessed 2024-02-23]
- Russell ER, Thériault ÉR, Colibaba A. Facilitating age-conscious student development through lecture-based courses on aging. Can J Aging. 2022;41(2):283-293. [ FREE Full text ] [ CrossRef ] [ Medline ]
- Chavez N. New Jersey becomes second state to require Asian American history to be taught in schools. CNN. 2022. URL: https://www.cnn.com/2022/01/18/us/new-jersey-schools-asian-american-history/index.html [accessed 2022-04-11]
- Ng R, Indran N. Hostility toward baby Boomers on TikTok. Gerontologist. 2022;62(8):1196-1206. [ FREE Full text ] [ CrossRef ] [ Medline ]
- Sloan L. Who Tweets in the United Kingdom? Profiling the Twitter population using the British Social Attitudes Survey 2015. Soc Media Soc. 2017;3(1):205630511769898. [ FREE Full text ] [ CrossRef ]
- Diehl M, Smyer MA, Mehrotra CM. Optimizing aging: a call for a new narrative. Am Psychol. 2020;75(4):577-589. [ FREE Full text ] [ CrossRef ] [ Medline ]
- Ng R, Indran N, Suarez P. Communicating risk perceptions through Batik art. JAMA. 2023;330(9):790-791. [ CrossRef ] [ Medline ]
- Ng R, Indran N. Reframing aging: foregrounding familial and occupational roles of older adults is linked to decreased ageism over two centuries. J Aging Soc Policy. 2023.:1-18. [ CrossRef ] [ Medline ]
- Ng R, Indran N. Impact of old age on an occupation's image over 210 years: an age premium for doctors, lawyers, and soldiers. J Appl Gerontol. 2023;42(6):1345-1355. [ CrossRef ] [ Medline ]
- Twitter. 2021. URL: https://twitter.com/ [accessed 2021-10-13]
Abbreviations
Edited by A Mavragani; submitted 19.01.23; peer-reviewed by A Atalay, A Bacong; comments to author 22.02.23; revised version received 12.03.23; accepted 14.09.23; published 29.03.24.
©Reuben Ng, Nicole Indran. Originally published in the Journal of Medical Internet Research (https://www.jmir.org), 29.03.2024.
This is an open-access article distributed under the terms of the Creative Commons Attribution License (https://creativecommons.org/licenses/by/4.0/), which permits unrestricted use, distribution, and reproduction in any medium, provided the original work, first published in the Journal of Medical Internet Research, is properly cited. The complete bibliographic information, a link to the original publication on https://www.jmir.org/, as well as this copyright and license information must be included.
Thank you for visiting nature.com. You are using a browser version with limited support for CSS. To obtain the best experience, we recommend you use a more up to date browser (or turn off compatibility mode in Internet Explorer). In the meantime, to ensure continued support, we are displaying the site without styles and JavaScript.
- View all journals
- My Account Login
- Explore content
- About the journal
- Publish with us
- Sign up for alerts
- Open access
- Published: 21 March 2024
Expert review of the science underlying nature-based climate solutions
- B. Buma ORCID: orcid.org/0000-0003-2402-7737 1 , 2 na1 ,
- D. R. Gordon ORCID: orcid.org/0000-0001-6398-2345 1 , 3 na1 ,
- K. M. Kleisner 1 ,
- A. Bartuska 1 , 4 ,
- A. Bidlack 5 ,
- R. DeFries ORCID: orcid.org/0000-0002-3332-4621 6 ,
- P. Ellis ORCID: orcid.org/0000-0001-7933-8298 7 ,
- P. Friedlingstein ORCID: orcid.org/0000-0003-3309-4739 8 , 9 ,
- S. Metzger 10 nAff15 nAff16 ,
- G. Morgan 11 ,
- K. Novick ORCID: orcid.org/0000-0002-8431-0879 12 ,
- J. N. Sanchirico 13 ,
- J. R. Collins ORCID: orcid.org/0000-0002-5705-9682 1 , 14 ,
- A. J. Eagle ORCID: orcid.org/0000-0003-0841-2379 1 ,
- R. Fujita 1 ,
- E. Holst 1 ,
- J. M. Lavallee ORCID: orcid.org/0000-0002-3028-7087 1 ,
- R. N. Lubowski 1 nAff17 ,
- C. Melikov 1 nAff18 ,
- L. A. Moore ORCID: orcid.org/0000-0003-0239-6080 1 nAff19 ,
- E. E. Oldfield ORCID: orcid.org/0000-0002-6181-1267 1 ,
- J. Paltseva 1 nAff20 ,
- A. M. Raffeld ORCID: orcid.org/0000-0002-5036-6460 1 ,
- N. A. Randazzo 1 nAff21 nAff22 ,
- C. Schneider 1 ,
- N. Uludere Aragon 1 nAff23 &
- S. P. Hamburg 1
Nature Climate Change ( 2024 ) Cite this article
10k Accesses
43 Altmetric
Metrics details
- Climate-change ecology
- Climate-change mitigation
- Environmental impact
Viable nature-based climate solutions (NbCS) are needed to achieve climate goals expressed in international agreements like the Paris Accord. Many NbCS pathways have strong scientific foundations and can deliver meaningful climate benefits but effective mitigation is undermined by pathways with less scientific certainty. Here we couple an extensive literature review with an expert elicitation on 43 pathways and find that at present the most used pathways, such as tropical forest conservation, have a solid scientific basis for mitigation. However, the experts suggested that some pathways, many with carbon credit eligibility and market activity, remain uncertain in terms of their climate mitigation efficacy. Sources of uncertainty include incomplete GHG measurement and accounting. We recommend focusing on resolving those uncertainties before broadly scaling implementation of those pathways in quantitative emission or sequestration mitigation plans. If appropriate, those pathways should be supported for their cobenefits, such as biodiversity and food security.
Similar content being viewed by others
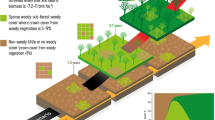
Australian human-induced native forest regeneration carbon offset projects have limited impact on changes in woody vegetation cover and carbon removals
Andrew Macintosh, Don Butler, … Paul Summerfield
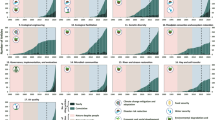
The evolution and future of research on Nature-based Solutions to address societal challenges
Thomas Dunlop, Danial Khojasteh, … Stefan Felder
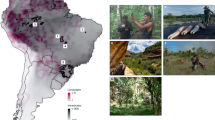
Contributions of human cultures to biodiversity and ecosystem conservation
Carolina Levis, Bernardo M. Flores, … Charles R. Clement
Nature-based climate solutions (NbCS) are conservation, restoration and improved management strategies (pathways) in natural and working ecosystems with the primary motivation to mitigate GHG emissions and remove CO 2 from the atmosphere 1 (similar to ecosystem-based mitigation 2 ). GHG mitigation through ecosystem stewardship is integral to meeting global climate goals, with the greatest benefit coming from near-term maximization of emission reductions, followed by CO 2 removal 3 . Many countries (for example, Indonesia, China and Colombia) use NbCS to demonstrate progress toward national climate commitments.
The scope of NbCS is narrower than that of nature-based solutions (NbS) which include interventions that prioritize non-climate benefits alongside climate (for example, biodiversity, food provisioning and water quality improvement) 4 . In many cases, GHG mitigation is considered a cobenefit that results from NbS actions focused on these other challenges 2 . In contrast, NbCS are broader than natural climate solutions, which are primarily focused on climate mitigation through conservation, restoration and improved land management, generally not moving ecosystems beyond their unmodified structure, function or composition 5 . NbCS may involve moving systems beyond their original function, for example by cultivating macroalgae in water deeper than their natural habitat.
The promise of NbCS has generated a proliferation of interest in using them in GHG mitigation plans 6 , 7 ; 104 of the 168 signatories to the Paris Accord included nature-based actions as part of their mitigation plans 8 . Success in long-term GHG management requires an accurate accounting of inputs and outputs to the atmosphere at scale, so NbCS credits must have robust, comprehensive and transparent scientific underpinnings 9 . Given the urgency of the climate problem, our goal is to identify NbCS pathways with a sufficient scientific foundation to provide broad confidence in their potential GHG mitigation impact, provide resources for confident implementation and identify priority research areas in more uncertain pathways. Evaluating implementation of mitigation projects is beyond our scope; this effort focuses on understanding the underlying science. The purpose is not evaluating any specific carbon crediting protocol or implementation framework but rather the current state of scientific understanding necessary to provide confidence in any NbCS.
In service of this goal, we first investigated nine biomes (boreal forests, coastal marine (salt marsh, mangrove, seagrass and coral reef), freshwater wetlands, grasslands, open ocean (large marine animal and mesopelagic zone biomass, seabed), peatlands, shrublands, temperate forests and tropical forests) and three cultivation types (agroforestry, croplands and macroalgae aquaculture); these were chosen because of their identified potential scale of global impact. In this context, impact is assessed as net GHG mitigation: the CO 2 sequestered or emissions reduced, for example, discounted by understood simultaneous emissions of other GHG (as when N 2 O is released simultaneously with carbon sequestration in cropland soils). From there, we identified 43 NbCS pathways which have been formally implemented (with or without market action) or informally proposed. We estimated the scale of mitigation impact for each pathway on the basis of this literature and, as a proxy measure of NbCS implementation, determined eligibility and activity under existing carbon crediting protocols. Eligibility means that the pathway is addressed by an existing GHG mitigation protocol; market activity means that credits are actively being bought under those eligibility requirements. We considered pathways across a spectrum from protection to improved management to restoration to manipulated systems, but some boundaries were necessary. We excluded primarily abiotically driven pathways (for example, ocean alkalinity enhancement) or where major land use or land-use trade-offs exist (for example, afforestation) 10 , 11 , 12 . Of the 43 pathways, 79% are at present eligible for carbon crediting (sometimes under several methodologies) and at least 65% of those have been implemented (Supplementary Table 1 ). This review was then appraised by 30 independent scholars (at least three per pathway; a complete review synthesis is given in the Supplementary Data ).
Consolidation of a broad body of scientific knowledge, with inherent variance, requires expert judgement. We used an expert elicitation process 13 , 14 , 15 with ten experts to place each proposed NbCS pathway into one of three readiness categories following their own assessment of the scientific literature, categorized by general sources of potential uncertainty: category 1, sufficient scientific basis to support a high-quality carbon accounting system or to support the development of such a system today; category 2, a >25% chance that focused research and reasonable funding would support development of high-quality carbon accounting (that is, move to category 1) within 5 years; or category 3, a <25% chance of development of high-quality carbon accounting within 5 years (for example, due to measurement challenges, unconstrained leakage, external factors which constrain viability).
If an expert ranked a pathway as category 2, they were also asked to rank general research needs to resolve: leakage/displacement (spillover to other areas), measuring, reporting and verification (the ability to quantify all salient stocks and fluxes), basic mechanisms of action (fundamental science), durability (ability to predict or compensate for uncertainty in timescale of effectiveness due to disturbances, climate change, human activity or other factors), geographic uncertainty (place-to-place variation), scaling potential (ability to estimate impact) and setting of a baseline (ability to estimate additionality over non-action; a counterfactual). To avoid biasing towards a particular a priori framework for evaluation of the scientific literature, reviewers could use their own framework for evaluating the NbCS literature about potential climate impact and so could choose to ignore or add relevant categorizations as well. Any pathway in category 1 would not need fundamental research for implementation; research gaps were considered too extensive for useful guidance on reducing uncertainty in category 3 pathways. Estimates of the global scale of likely potential impact (PgCO 2 e yr −1 ) and cobenefits were also collected from expert elicitors. See Methods and Supplementary Information for the survey instrument.
Four pathways with the highest current carbon market activity and high mitigation potential (tropical and temperate forest conservation and reforestation; Table 1 and Supplementary Data ), were consistently rated as high-confidence pathways in the expert elicitation survey. Other NbCS pathways, especially in the forestry sector, were rated relatively strongly by the experts for both confidence in scientific basis and scale of potential impact, with some spread across the experts (upper right quadrant, Fig. 1 ). Conversely, 13 pathways were consistently marked by experts as currently highly uncertain/low confidence (median score across experts: 2.5–3.0) and placed in category 3 (for example, cropland microbial amendments and coral reef restoration; Supplementary Tables 1 and 2 ). For the full review, including crediting protocols currently used, literature estimates of scale and details of sub-pathways, see Supplementary Data .
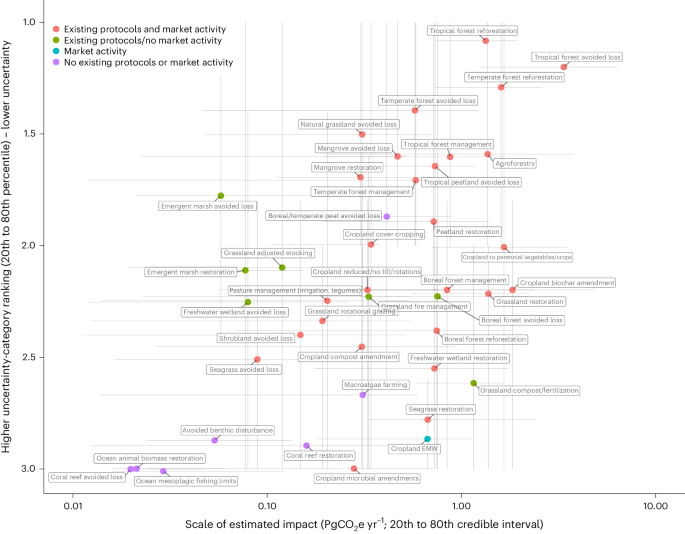
Pathways in the upper right quadrant have both high confidence in the scientific foundations and the largest potential scale of global impact; pathways in the lower left have the lowest confidence in our present scientific body of knowledge and an estimated smaller potential scale of impact. Designations of carbon credit eligibility under existing protocols and market activity at the present time are noted. Grassland enhanced mineral weathering (EMW) is not shown (mean category rating 2.9) as no scale of impact was estimated. See Supplementary Table 1 for specific pathway data. Bars represent 20th to 80th percentiles of individual estimates, if there was variability in estimates. A small amount of random noise was added to avoid overlap.
The experts assessed 26 pathways as having average confidence scores between 1.5 and 2.4, suggesting the potential for near-term resolution of uncertainties. This categorization arose from either consensus amongst experts on the uncertain potential (for example, boreal forest reforestation consistently rated category 2, with primary concerns about durability) or because experts disagreed, with some ranking category 1 and others category 3 (for example, pasture management). We note that where expert disagreement exists (seen as the spread of responses in Fig. 1 and Supplementary Table 1 ; also see Data availability for link to original data), this suggests caution against overconfidence in statements about these pathways. These results also suggest that confidence may be increased by targeted research on the identified sources of uncertainty (Supplementary Table 3 ).
Sources of uncertainty
Durability and baseline-setting were rated as high sources of uncertainty across all pathways ranked as category 2 by the experts (mean ratings of 3.6 and 3.4 out of 5, respectively; Supplementary Table 3 ). Understanding of mechanisms and geographic spread had the lowest uncertainty ratings (2.1 and 2.3, respectively), showing confidence in the basic science. Different subsets of pathways had different prioritizations, however, suggesting different research needs: forest-centric pathways were most uncertain in their durability and additionality (3.8 and 3.4, respectively), suggesting concerns about long-term climate and disturbance trajectories. Agricultural and grassland systems, however, had higher uncertainty in measurement methods and additionality (3.9 and 3.5 respectively). Although there were concerns about durability from some experts (for example, due to sea-level rise), some coastal blue carbon pathways such as mangrove restoration (mean category ranking: 1.7 (20th to 80th percentile 1.0–2.0)) have higher confidence than others (for example, seagrass restoration: mean category ranking 2.8, 20th to 80th percentile 2.6–3.0)), which are relatively poorly constrained in terms of net radiative forcing potential despite a potentially large carbon impact (seagrass median: 1.60 PgCO 2 e yr −1 ; see Supplementary Data for more scientific literature estimates).
Scale of impact
For those pathways with lower categorization by the expert elicitation (category 2 or 3) at the present time, scale of global impact is a potential heuristic for prioritizing further research. High variability, often two orders of magnitude, was evident in the mean estimated potential PgCO 2 e yr −1 impacts for the different pathways (Fig. 1 and Supplementary Table 2 ) and the review of the literature found even larger ranges produced by individual studies (Supplementary Data ). A probable cause of this wide range was different constraints on the estimated potential, with some studies focusing on potential maximum impact and others on more constrained realizable impacts. Only avoided loss of tropical forest and cropland biochar amendment were consistently estimated as having the likely potential to mitigate >2 PgCO 2 e yr −1 , although biochar was considered more uncertain by experts due to other factors germane to its overall viability as a climate solution, averaging a categorization of 2.2. The next four highest potential impact pathways, ranging from 1.6 to 1.7 PgCO 2 e yr −1 , spanned the spectrum from high readiness (temperate forest restoration) to moderate (cropland conversion from annual to perennial vegetation and grassland restoration) to low (seagrass restoration, with main uncertainties around scale of potential impact and durability).
There was high variability in the elicitors’ estimated potential scale of impact, even in pathways with strong support, such as tropical forest avoided loss (20th to 80th percentile confidence interval: 1–8 PgCO 2 e yr −1 ), again emphasizing the importance of consistent definitions and constraints on how NbCS are measured, evaluated and then used in broad-scale climate change mitigation planning and budgeting. Generally, as pathway readiness decreased (moving from category 1 to 3), the elicitor-estimated estimates of GHG mitigation potential decreased (Supplementary Fig. 1 ). Note that individual studies from the scientific literature may have higher or lower estimates (Supplementary Data ).
Expert elicitation meta-analyses suggest that 6–12 responses are sufficient for a robust and stable quantification of responses 15 . We tested that assumption via a Monte Carlo-based sensitivity assessment. Readiness categorizations by the ten experts were robust to a Monte Carlo simulation test, where further samples were randomly drawn from the observed distribution of responses: mean difference between the original and the boot-strapped data was 0.02 (s.d. = 0.05) with an absolute difference average of 0.06 (s.d. = 0.06). The maximum difference in readiness categorization means across all pathways was 0.20 (s.d. = 0.20) (Supplementary Table 2 ). The full dataset of responses is available online (see ʻData availabilityʼ).
These results highlight opportunities to accelerate implementation of NbCS in well-supported pathways and identify critical research needs in others (Fig. 1 ). We suggest focusing future efforts on resolving identified uncertainties for pathways at the intersection between moderate average readiness (for example, mean categorizations between ~1.5 and 2.0) and high potential impact (for example, median >0.5 PgCO 2 e yr −1 ; Supplementary Table 1 ): agroforestry, improved tropical and temperate forest management, tropical and boreal peatlands avoided loss and peatland restoration. Many, although not all, experts identified durability and baseline/additionality as key concerns to resolve in those systems; research explicitly targeted at those specific uncertainties (Supplementary Table 3 ) could rapidly improve confidence in those pathways.
We recommend a secondary research focus on the lower ranked (mean category 2.0 to 3.0) pathways with estimated potential impacts >1 PgCO 2 e yr −1 (Supplementary Fig. 2 ). For these pathways, explicit, quantitative incorporation into broad-scale GHG management plans will require further focus on systems-level carbon/GHG understandings to inspire confidence at all stages of action and/or identifying locations likely to support durable GHG mitigation, for example ref. 16 . Examples of this group include avoided loss and degradation of boreal forests (for example, fire, pests and pathogens and albedo 16 ) and effective mesopelagic fishery management, which some individual studies estimate would avoid future reductions of the currently sequestered 1.5–2.0 PgC yr −1 (refs. 17 , 18 ). These pathways may turn out to have higher or lower potential than the expert review suggests, on the basis of individual studies (Supplementary Data ) but strong support will require further, independent verification of that potential.
We note that category 3 rankings by expert elicitation do not necessarily imply non-viability but simply that much more research is needed to confidently incorporate actions into quantitative GHG mitigation plans. We found an unsurprising trend of lower readiness categorization with lower pathway familiarity (Supplementary Fig. 3 ). This correlation may result from two, non-exclusive potential causes: (1) lower elicitor expertise in some pathways (inevitable, although the panel was explicitly chosen for global perspectives, connections and diverse specialties) and (2) an actual lack of scientific evidence in the literature, which leads to that self-reported lack of familiarity, a common finding in the literature review (Supplementary Data ). Both explanations suggest a need to better consolidate, develop and disseminate the science in each pathway for global utility and recognition.
Our focus on GHG-related benefits in no way diminishes the substantial conservation, environmental and social cobenefits of these pathways (Supplementary Table 4 ), which often exceed their perceived climate benefits 1 , 19 , 20 , 21 . Where experts found climate impacts to remain highly uncertain but other NbS benefits are clear (for example, biodiversity and water quality; Supplementary Table 4 ), other incentives or financing mechanisms independent of carbon crediting should be pursued. While the goals here directly relate to using NbCS as a reliably quantifiable part of global climate action planning and thus strong GHG-related scientific foundations, non-climate NbS projects may provide climate benefits that are less well constrained (and thus less useful from a GHG budgeting standpoint) but also valuable. Potential trade-offs, if any, between ecosystem services and management actions, such as biodiversity and positive GHG outcomes, should be explored to ensure the best realization of desired goals 2 .
Finally, our focus in this study was on broad-scale NbCS potential in quantitative mitigation planning because of the principal and necessary role of NbCS in overall global warming targets. We recognize the range of project conditions that may increase, or decrease, the rigour of any pathway outside the global-scale focus here. We did not specifically evaluate the large and increasing number of crediting concepts (by pathway: Supplementary Data ), focusing rather on the underlying scientific body of knowledge within those pathways. Some broad pathways may have better defined sub-pathways within them, with a smaller potential scale of impact but potentially lower uncertainty (for example, macroalgae harvest cycling). Poorly enacted NbCS actions and/or crediting methodologies at project scales may result in loss of benefits even from high-ranking pathways 22 , 23 , 24 and attention to implementation should be paramount. Conversely, strong, careful project-scale methodologies may make lower readiness pathways beneficial for a given site.
Viable NbCS are vital to global climate change mitigation but NbCS pathways that lack strong scientific underpinnings threaten global accounting by potentially overestimating future climate benefits and eroding public trust in rigorous natural solutions. Both the review of the scientific literature and the expert elicitation survey identified high potential ready-to-implement pathways (for example, tropical reforestation), reinforcing present use of NbCS in planning.
However, uncertainty remains about the quantifiable GHG mitigation of some active and nascent NbCS pathways. On the basis of the expert elicitation survey and review of the scientific literature, we are concerned that large-scale implementation of less scientifically well-founded NbCS pathways in mitigation plans may undermine net GHG budget planning; those pathways require more study before they can be confidently promoted at broad scales and life-cycle analyses to integrate system-level emissions when calculating totals. The expert elicitation judgements suggest a precautionary approach to scaling lower confidence pathways until the scientific foundations are strengthened, especially for NbCS pathways with insufficient measurement and monitoring 10 , 24 , 25 or poorly understood or measured net GHG mitigation potentials 16 , 26 , 27 , 28 . While the need to implement more NbCS pathways for reducing GHG emissions and removing carbon from the atmosphere is urgent, advancing the implementation of poorly quantified pathways (in relation to their GHG mitigation efficacy) could give the false impression that they can balance ongoing, fossil emissions, thereby undermining overall support for more viable NbCS pathways. Explicitly targeting research to resolve these uncertainties in the baseline science could greatly bolster confidence in the less-established NbCS pathways, benefiting efforts to reduce GHG concentrations 29 .
The results of this study should inform both market-based mechanisms and non-market approaches to NbCS pathway management. Research and action that elucidates and advances pathways to ensure a solid scientific basis will provide confidence in the foundation for successfully implementing NbCS as a core component of global GHG management.
NbCS pathway selection
We synthesized scientific publications for nine biomes (boreal forests, coastal blue carbon, freshwater wetlands, grasslands, open ocean blue carbon, peatlands, shrublands, temperate forests and tropical forests) and three cultivation types (agroforestry, croplands and macroalgae aquaculture) (hereafter, systems) and the different pathways through which they may be able to remove carbon or reduce GHG emissions. Shrublands and grasslands were considered as independent ecosystems; nonetheless, we acknowledge that there is overlap in the numbers presented here because shrublands are often included with grasslands 5 , 30 , 31 , 32 , 33 .
The 12 systems were chosen because they have each been identified as having potential for emissions reductions or carbon removal at globally relevant scales. Within these systems, we identified 43 pathways which either have carbon credit protocols formally established or informally proposed for review (non-carbon associated credits were not evaluated). We obtained data on carbon crediting protocols from international, national and regional organizations and registries, such as Verra, American Carbon Registry, Climate Action Reserve, Gold Standard, Clean Development Mechanism, FAO and Nori. We also obtained data from the Voluntary Registry Offsets Database developed by the Berkeley Carbon Trading Project and Carbon Direct company 34 . While we found evidence of more Chinese carbon crediting protocols, we were not able to review these because of limited publicly available information. To maintain clarity and avoid misrepresentation, we used the language as written in each protocol. A full list of the organizations and registries for each system can be found in the Supplementary Data .
Literature searches and synthesis
We reviewed scientific literature and reviews (for example, IPCC special reports) to identify studies reporting data on carbon stocks, GHG dynamics and sequestration potential of each system. Peer-reviewed studies and meta-analyses were identified on Scopus, Web of Science and Google Scholar using simple queries combining the specific practice or pathway names or synonyms (for example, no-tillage, soil amendments, reduced stocking rates, improved forest management, avoided forest conversion and degradation, avoided mangrove conversion and degradation) and the following search terms: ‘carbon storage’, ‘carbon stocks’, ‘carbon sequestration’, ‘carbon sequestration potential’, ‘additional carbon storage’, ‘carbon dynamics’, ‘areal extent’ or ‘global’.
The full literature review was conducted between January and October 2021. We solicited an independent, external review of the syntheses (obtaining from at least three external reviewers per natural or working system; see p. 2 of the Supplementary Data ) as a second check against missing key papers or misinterpretation of data. The review was generally completed in March 2022. Data from additional relevant citations were added through October 2022 as they were discovered. For a complete list of all literature cited, see pp. 217–249 of the Supplementary Data .
From candidate papers, the papers were considered if their results/data could be applied to the following central questions:
How much carbon is stored (globally) at present in the system (total and on average per hectare) and what is the confidence?
At the global level, is the system a carbon source or sink at this time? What is the business-as-usual projection for its carbon dynamics?
Is it possible, through active management, to either increase net carbon sequestration in the system or prevent carbon emissions from that system? (Note that other GHG emissions and forcings were included here as well.)
What is the range of estimates for how much extra carbon could be sequestered globally?
How much confidence do we have in the present methods to detect any net increases in carbon sequestration in a system or net changes in areal extent of that?
From each paper, quantitative estimates for the above questions were extracted for each pathway, including any descriptive information/metadata necessary to understand the estimate. In addition, information on sample size, sampling scheme, geographic coverage, timeline of study, timeline of projections (if applicable) and specific study contexts (for example, wind-break agroforestry) were recorded.
We also tracked where the literature identified trade-offs between carbon sequestered or CO 2 emissions reduced and emissions of other GHG (for example, N 2 O or methane) for questions three and five above. For example, wetland restoration can result in increased CO 2 uptake from the atmosphere. However, it can also increase methane and N 2 O emissions to the atmosphere. Experts were asked to consider the uncertainty in assessing net GHG mitigation as they categorized the NbCS pathways.
Inclusion of each pathway in mitigation protocols and the specific carbon registries involved were also identified. These results are reported (grouped or individually as appropriate) in the Supplementary Data , organized by the central questions and including textual information for interpretation. The data and protocol summaries for each of the 12 systems were reviewed by at least three scientists each and accordingly revised.
These summaries were provided to the expert elicitation group as optional background information.
Unit conversions
Since this synthesis draws on literature from several sources that use different methods and units, all carbon measurements were standardized to the International System of Units (SI units). When referring to total stocks for each system, numbers are reported in SI units of elemental carbon (that is, PgC). When referring to mitigation potential, elemental carbon was converted to CO 2 by multiplying by 3.67. Differences in methodology, such as soil sampling depth, make it difficult to standardize across studies. Where applicable, the specific measurement used to develop each stock estimate is reported.
Expert elicitation process
To assess conclusions brought about by the initial review process described above, we conducted an expert elicitation survey to consolidate and add further, independent assessments to the original literature review. The expert elicitation survey design followed best practice recommendations 14 , with a focus on participant selection, explicitly defining uncertainty, minimizing cognitive and overconfidence biases and clarity of focus. Research on expert elicitation suggests that 6–12 responses are sufficient for a stable quantification of responses 15 . We identified >40 potential experts via a broad survey of leading academics, science-oriented NGO and government agency publications and products. These individuals have published on several NbCS pathways or could represent larger research efforts that spanned the NbCS under consideration. Careful attention was paid to the gender and sectoral breakdown of respondents to ensure equitable representation. Of the invitees, ten completed the full elicitation effort. Experts were offered compensation for their time.
Implementation of the expert elicitation process followed the IDEA protocol 15 . Briefly, after a short introductory interview, the survey was sent to the participants. Results were anonymized and standardized (methods below) and a meeting held with the entire group to discuss the initial results and calibrate understanding of questions. The purpose of this meeting was not to develop consensus on a singular answer but to discuss and ensure that all questions are being considered in the same way (for example, clarifying any potentially confusing language, discussing any questions that emerged as part of the process). The experts then revisited their initial rankings to provide final, anonymous rankings which were compiled in the same way. These final rankings are the results presented here and may be the same or different from the initial rankings, which were discarded.
Survey questions
The expert elicitation survey comprised five questions for each pathway. The data were collected via Google Forms and collated anonymously at the level of pathways, with each respondent contributing one datapoint for each pathway. The experts reported their familiarity (or the familiarity of the organization whose work they were representing) with the pathway and other cobenefits for the pathways.
The initial question ranked the NbCS pathway by category, from one to three.
Category 1 was defined as a pathway with sufficient scientific knowledge to support a high-quality carbon accounting system today (for example, meets the scientific criteria identified in the WWF-EDF-Oeko Institut and ICAO TAB) or to support the development of such a system today. The intended interpretation is that sufficient science is available for quantifying and verifying net GHG mitigation. Note that experts were not required to reference any given ‘high-quality’ crediting framework, which were provided only as examples. In other words, the evaluation was not intended to rank a given framework (for example, ref. 35 ) but rather expert confidence in the fundamental scientific understandings that underpin potential for carbon accounting overall. To this end, no categorization of uncertainty was required (reviewers could skip categorizations they felt were not necessary) and space was available to fill in new categories by individual reviewers (if they felt a category was missing or needed). Uncertainties at this category 1 level are deemed ‘acceptable’, for example, not precluding accounting now, although more research may further substantiate high-quality credits.
Category 2 pathways have a good chance (>25%) that with more research and within the next 5 years, the pathway could be developed into a high-quality pathway for carbon accounting and as a nature-based climate solution pathway. For these pathways, further understanding is needed for factors such as baseline processes, long-term stability, unconstrained fluxes, possible leakage or other before labelling as category 1 but the expert is confident that information can be developed, in 5 years or less, with more work. The >25% chance threshold and 5-year timeframe were determined a priori to reflect and identify pathways that experts identified as having the potential to meet the Paris Accord 2030 goal. Other thresholds (for example, longer timeframes) could have been chosen, which would impact the relative distribution of pathways in categories 2 and 3 (for example, a longer timeframe allowed could move some pathways from category 3 into category 2, for some reviewers). We emphasize that category 3 pathways do not necessarily mean non-valuable approaches but longer timeframes required for research than the one set here.
Category 3 responses denoted pathways that the expert thought had little chance (<25%) that with more research and within the next 5 years, this pathway could be developed into a suitable pathway for managing as a natural solutions pathway, either because present evidence already suggests GHG reduction is not likely to be viable, co-emissions or other biophysical feedbacks may offset those gains or because understanding of key factors is lacking and unlikely to be developed within the next 5 years. Notably, the last does not mean that the NbCS pathway is not valid or viable in the long-term, simply that physical and biological understandings are probably not established enough to enable scientific rigorous and valid NbCS activity in the near term.
The second question asked the experts to identify research gaps associated with those that they ranked as category 2 pathways to determine focal areas for further research. The experts were asked to rank concerns about durability (ability to predict or compensate for uncertainty in timescale of effectiveness due to disturbances, climate change, human activity or other factors), geographic uncertainty (place-to-place variation), leakage or displacement (spillover of activities to other areas), measuring, reporting and verification (MRV, referring to the ability to quantify all salient stocks and fluxes to fully assess climate impacts), basic mechanisms of action (fundamental science), scaling potential (ability to estimate potential growth) and setting of a baseline (ability to reasonably quantify additionality over non-action, a counterfactual). Respondents could also enter a different category if desired. For complete definitions of these categories, see the survey instrument ( Supplementary Information ). This question was not asked if the expert ranked the pathway as category 1, as those were deemed acceptable, or for category 3, respecting the substantial uncertainty in that rating. Note that responses were individual and so the same NbCS pathway could receive (for example) several individual category 1 rankings, which would indicate reasonable confidence from those experts, and several category 2 rankings from others, which would indicate that those reviewers have lingering concerns about the scientific basis, along with their rankings of the remaining key uncertainties in those pathways. These are important considerations, as they reflect the diversity of opinions and research priorities; individual responses are publicly available (anonymized: https://doi.org/10.5281/zenodo.7859146 ).
The third question involved quantification of the potential for moving from category 2 to 1 explicitly. Following ref. 14 , the respondents first reported the lowest plausible value for the potential likelihood of movement (representing the lower end of a 95% confidence interval), then the upper likelihood and then their best guess for the median/most likely probability. They were also asked for the odds that their chosen interval contained the true value, which was used to scale responses to standard 80% credible intervals and limit overconfidence bias 13 , 15 . This question was not asked if the expert ranked the pathway as category 3, respecting the substantial uncertainty in that rating.
The fourth question involved the scale of potential impact from the NbCS, given the range of uncertainties associated with effectiveness, area of applicability and other factors. The question followed the same pattern as the third, first asking about lowest, then highest, then best estimate for potential scale of impact (in PgCO 2 e yr −1 ). Experts were again asked to express their confidence in their own range, which was used to scale to a standard 80% credible interval. This estimate represents a consolidation of the best-available science by the reviewers. For a complete review including individual studies and their respective findings, see the Supplementary Data . This question was not asked if the expert ranked the pathway as category 3, respecting the substantial uncertainty in that rating.
Final results
After collection of the final survey responses, results were anonymized and compiled by pathway. For overall visualization and discussion purposes, responses were combined into a mean and 20th to 80th percentile range. The strength of the expert elicitation process lies in the collection of several independent assessments. Those different responses represent real differences in data interpretation and synthesis ascribed by experts. This can have meaningful impacts on decision-making by different individuals and organizations (for example, those that are more optimistic or pessimistic about any given pathway). Therefore, individual anonymous responses were retained by pathway to show the diversity of responses for any given pathway. The experts surveyed, despite their broad range of expertise, ranked themselves as less familiar with category 3 pathways than category 1 or 2 (linear regression, P < 0.001, F = 59.6 2, 394 ); this could be because of a lack of appropriate experts—although they represented all principal fields—or simply because the data are limited in those areas.
Sensitivity
To check for robustness against sample size variation, we conducted a Monte Carlo sensitivity analysis of the data on each pathway to generate responses of a further ten hypothetical experts. Briefly, the extra samples were randomly drawn from the observed category ranking mean and standard deviations for each individual pathway and appended to the original list; values <1 or >3 were truncated to those values. This analysis resulted in only minor differences in the mean categorization across all pathways: the mean difference between the original and the boot-strapped data was 0.02 (s.d. = 0.05) with an absolute difference average of 0.06 (s.d. = 0.06). The maximum difference in means across all pathways was 0.20 (s.d. = 0.20) (Supplementary Table 2 ). The results suggest that the response values are stable to additional responses.
All processing was done in R 36 , with packages including fmsb 37 and forcats 38 .
Data availability
Anonymized expert elicitation responses are available on Zenodo 39 : https://doi.org/10.5281/zenodo.7859146 .
Code availability
R code for analysis available on Zenodo 39 : https://doi.org/10.5281/zenodo.7859146 .
Novick, K. A. et al. Informing nature‐based climate solutions for the United States with the best‐available science. Glob. Change Biol. 28 , 3778–3794 (2022).
Article Google Scholar
Cohen-Shacham, E., Walters, G., Janzen, C. & Maginnis, S. (eds) Nature-based Solutions to Address Global Societal Challenges (IUCN, 2016).
IPCC Climate Change 2021: The Physical Science Basis (eds Masson-Delmotte, V. et al.) (Cambridge Univ. Press, 2021).
Seddon, N. et al. Understanding the value and limits of nature-based solutions to climate change and other global challenges. Philos. Trans. R. Soc. B 375 , 20190120 (2020).
Griscom, B. W. et al. Natural climate solutions. Proc. Natl Acad. Sci. USA 114 , 11645–11650 (2017).
Article CAS PubMed PubMed Central ADS Google Scholar
Blaufelder, C., Levy, C., Mannion, P. & Pinner, D. A. Blueprint for Scaling Voluntary Carbon Markets to Meet the Climate Challenge (McKinsey & Company, 2021).
Arcusa, S. & Sprenkle-Hyppolite, S. Snapshot of the carbon dioxide removal certification and standards ecosystem (2021–2022). Clim. Policy 22 , 1319–1332 (2022).
Seddon, N. et al. Global recognition of the importance of nature-based solutions to the impacts of climate change Glob. Sustain. 3 , pe15 (2020).
Anderegg, W. R. Gambling with the climate: how risky of a bet are natural climate solutions? AGU Adv. 2 , e2021AV000490 (2021).
Article ADS Google Scholar
Gattuso, J. P. et al. Ocean solutions to address climate change and its effects on marine ecosystems. Front. Mar. Sci. 5 , p337 (2018).
Bach, L. T., Gill, S. J., Rickaby, R. E., Gore, S. & Renforth, P. CO 2 removal with enhanced weathering and ocean alkalinity enhancement: potential risks and co-benefits for marine pelagic ecosystems. Front. Clim. 1 , 7 (2019).
Doelman, J. C. et al. Afforestation for climate change mitigation: potentials, risks and trade‐offs. Glob. Change Biol. 26 , 1576–1591 (2019).
Speirs-Bridge, A. et al. Reducing overconfidence in the interval judgments of experts. Risk Anal. 30 , 512–523 (2010).
Article PubMed Google Scholar
Morgan, M. G. Use (and abuse) of expert elicitation in support of decision making for public policy. Proc. Natl Acad. Sci. USA 111 , 7176–7184 (2014).
Hemming, V., Burgman, M. A., Hanea, A. M., McBride, M. F. & Wintle, B. C. A practical guide to structured expert elicitation using the IDEA protocol. Methods Ecol. Evol. 9 , 169–180 (2018).
Anderegg, W. R. et al. Climate-driven risks to the climate mitigation potential of forests. Science 368 , eaaz7005 (2020).
Article CAS PubMed Google Scholar
Boyd, P. W., Claustre, H., Levy, M., Siegel, D. A. & Weber, T. Multi-faceted particle pumps drive carbon sequestration in the ocean. Nature 568 , 327–335 (2019).
Article CAS PubMed ADS Google Scholar
Saba, G. K. et al. Toward a better understanding of fish-based contribution to ocean carbon flux. Limnol. Oceanogr. 66 , 1639–1664 (2021).
Article CAS ADS Google Scholar
Seddon, N., Turner, B., Berry, P., Chausson, A. & Girardin, C. A. Grounding nature-based climate solutions in sound biodiversity science. Nat. Clim. Change 9 , 84–87 (2019).
Soto-Navarro, C. et al. Mapping co-benefits for carbon storage and biodiversity to inform conservation policy and action. Philos. Trans. R. Soc. B 375 , 20190128 (2020).
Article CAS Google Scholar
Schulte, I., Eggers, J., Nielsen, J. Ø. & Fuss, S. What influences the implementation of natural climate solutions? A systematic map and review of the evidence. Environ. Res. Lett. 17 , p013002 (2022).
West, T. A., Börner, J., Sills, E. O. & Kontoleon, A. Overstated carbon emission reductions from voluntary REDD+ projects in the Brazilian Amazon. Proc. Natl Acad. Sci. USA 117 , 24188–24194 (2020).
Di Sacco, A. et al. Ten golden rules for reforestation to optimize carbon sequestration, biodiversity recovery and livelihood benefits. Glob. Change Biol. 27 , 1328–1348 (2021).
López-Vallejo, M. in Towards an Emissions Trading System in Mexico: Rationale, Design and Connections with the Global Climate Agenda (ed. Lucatello, S.) 191–221 (Springer, 2022)
Oldfield, E. E. et al. Realizing the potential of agricultural soil carbon sequestration requires more effective accounting. Science 375 , 1222–1225 (2022).
Burkholz, C., Garcias-Bonet, N. & Duarte, C. M. Warming enhances carbon dioxide and methane fluxes from Red Sea seagrass ( Halophila stipulacea ) sediments. Biogeosciences 17 , 1717–1730 (2020).
Guenet, B. et al. Can N 2 O emissions offset the benefits from soil organic carbon storage? Glob. Change Biol. 27 , 237–256 (2021).
Rosentreter, J. A., Al‐Haj, A. N., Fulweiler, R. W. & Williamson, P. Methane and nitrous oxide emissions complicate coastal blue carbon assessments. Glob. Biogeochem. Cycles 35 , pe2020GB006858 (2021).
Schwartzman, S. et al. Environmental integrity of emissions reductions depends on scale and systemic changes, not sector of origin. Environ. Res. Lett. 16 , p091001 (2021).
Crop and Livestock Products Database (FAO, 2022); https://www.fao.org/faostat/en/#data/QCL
Fargione, J. E. et al. Natural climate solutions for the United States. Sci. Adv. 4 , eaat1869 (2018).
Article PubMed PubMed Central ADS Google Scholar
Meyer, S. E. Is climate change mitigation the best use of desert shrublands? Nat. Resour. Environ. Issues 17 , 2 (2011).
Google Scholar
Lorenz, K. & Lal, R. Carbon Sequestration in Agricultural Ecosystems (Springer Cham, 2018).
Haya, B., So, I. & Elias, M. The Voluntary Registry Offsets Database (Univ. California, 2021); https://gspp.berkeley.edu/faculty-and-impact/centers/cepp/projects/berkeley-carbon-trading-project/offsets-database
Core Carbon Principles; CCP Attributes; Assessment Framework for Programs; and Assessment Procedure (ICVCM, 2023); https://icvcm.org/the-core-carbon-principles/
R Core Team. R: A Language and Environment for Statistical Computing (R Foundation for Statistical Computing, 2022).
Nakazawa, M. fmsb: Functions for medical statistics book with some demographic data. R package version 0.7.4 https://CRAN.R-project.org/package=fmsb (2022).
Wickham, H. forcats: Tools for working with categorical variables (factors). R package version 0.5.2 https://CRAN.R-project.org/package=forcats (2022)
Buma, B. Nature-based climate solutions: expert elicitation data and analysis code. Zenodo https://doi.org/10.5281/zenodo.7859146 (2023).
Download references
Acknowledgements
This research was supported through gifts to the Environmental Defense Fund from the Bezos Earth Fund, King Philanthropies and Arcadia, a charitable fund of L. Rausing and P. Baldwin. We thank J. Rudek for help assembling the review and 30 experts who reviewed some or all of those data and protocol summaries (Supplementary Data ). S.M. was supported by a cooperative agreement between the National Science Foundation and Battelle that sponsors the National Ecological Observatory Network programme.
Author information
Present address: Department of Atmospheric and Oceanic Sciences, University of Wisconsin-Madison, Madison, WI, USA
Present address: AtmoFacts, Longmont, CO, USA
R. N. Lubowski
Present address: Lombard Odier Investment Managers, New York, NY, USA
Present address: Ecological Carbon Offset Partners LLC, dba EP Carbon, Minneapolis, MN, USA
L. A. Moore
Present address: , San Francisco, CA, USA
J. Paltseva
Present address: ART, Arlington, VA, USA
N. A. Randazzo
Present address: NASA/GSFC, Greenbelt, MD, USA
Present address: University of Maryland, College Park, MD, USA
N. Uludere Aragon
Present address: Numerical Terradynamic Simulation Group, University of Montana, Missoula, MT, USA
These authors contributed equally: B. Buma, D. R. Gordon.
Authors and Affiliations
Environmental Defense Fund, New York, NY, USA
B. Buma, D. R. Gordon, K. M. Kleisner, A. Bartuska, J. R. Collins, A. J. Eagle, R. Fujita, E. Holst, J. M. Lavallee, R. N. Lubowski, C. Melikov, L. A. Moore, E. E. Oldfield, J. Paltseva, A. M. Raffeld, N. A. Randazzo, C. Schneider, N. Uludere Aragon & S. P. Hamburg
Department of Integrative Biology, University of Colorado, Denver, CO, USA
Department of Biology, University of Florida, Gainesville, FL, USA
D. R. Gordon
Resources for the Future, Washington, DC, USA
A. Bartuska
International Arctic Research Center, University of Alaska, Fairbanks, AK, USA
Department of Ecology Evolution and Environmental Biology and the Climate School, Columbia University, New York, NY, USA
The Nature Conservancy, Arlington, VA, USA
Faculty of Environment, Science and Economy, University of Exeter, Exeter, UK
P. Friedlingstein
Laboratoire de Météorologie Dynamique/Institut Pierre-Simon Laplace, CNRS, Ecole Normale Supérieure/Université PSL, Sorbonne Université, Ecole Polytechnique, Palaiseau, France
National Ecological Observatory Network, Battelle, Boulder, CO, USA
Department of Engineering and Public Policy, Carnegie Mellon University, Pittsburgh, PA, USA
O’Neill School of Public and Environmental Affairs, Indiana University, Bloomington, IN, USA
Department of Environmental Science and Policy, University of California, Davis, CA, USA
J. N. Sanchirico
Department of Marine Chemistry & Geochemistry, Woods Hole Oceanographic Institution, Woods Hole, MA, USA
J. R. Collins
You can also search for this author in PubMed Google Scholar
Contributions
D.R.G. and B.B. conceived of and executed the study design. D.R.G., K.M.K., J.R.C., A.J.E., R.F., E.H., J.M.L., R.N.L., C.M., L.A.M., E.E.O., J.P., A.M.R., N.A.R., C.S. and N.U.A. coordinated and conducted the literature review. G.M. and B.B. primarily designed the survey. A. Bartuska, A. Bidlack, B.B., J.N.S., K.N., P.E., P.F., R.D. and S.M. contributed to the elicitation. B.B. conducted the analysis and coding. S.P.H. coordinated funding. B.B. and D.R.G. were primary writers; all authors were invited to contribute to the initial drafting.
Corresponding author
Correspondence to B. Buma .
Ethics declarations
Competing interests.
The authors declare no competing interests. In the interest of full transparency, we note that while B.B., D.R.G., K.M.K., A.B., J.R.C., A.J.E., R.F., E.H., J.M.L., R.N.L., C.M., L.A.M., E.E.O., J.P., A.M.R., N.A.R., C.S., N.U.A., S.P.H. and P.E. are employed by organizations that have taken positions on specific NbCS frameworks or carbon crediting pathways (not the focus of this work), none have financial or other competing interest in any of the pathways and all relied on independent science in their contributions to the work.
Peer review
Peer review information.
Nature Climate Change thanks Camila Donatti, Connor Nolan and the other, anonymous, reviewer(s) for their contribution to the peer review of this work.
Additional information
Publisher’s note Springer Nature remains neutral with regard to jurisdictional claims in published maps and institutional affiliations.
Supplementary information
Supplementary information.
Supplementary Tables 1–4, Figs. 1–3 and survey instrument.
Supplementary Data
Literature review and list of reviewers.
Rights and permissions
Open Access This article is licensed under a Creative Commons Attribution 4.0 International License, which permits use, sharing, adaptation, distribution and reproduction in any medium or format, as long as you give appropriate credit to the original author(s) and the source, provide a link to the Creative Commons licence, and indicate if changes were made. The images or other third party material in this article are included in the article’s Creative Commons licence, unless indicated otherwise in a credit line to the material. If material is not included in the article’s Creative Commons licence and your intended use is not permitted by statutory regulation or exceeds the permitted use, you will need to obtain permission directly from the copyright holder. To view a copy of this licence, visit http://creativecommons.org/licenses/by/4.0/ .
Reprints and permissions
About this article
Cite this article.
Buma, B., Gordon, D.R., Kleisner, K.M. et al. Expert review of the science underlying nature-based climate solutions. Nat. Clim. Chang. (2024). https://doi.org/10.1038/s41558-024-01960-0
Download citation
Received : 24 April 2023
Accepted : 20 February 2024
Published : 21 March 2024
DOI : https://doi.org/10.1038/s41558-024-01960-0
Share this article
Anyone you share the following link with will be able to read this content:
Sorry, a shareable link is not currently available for this article.
Provided by the Springer Nature SharedIt content-sharing initiative
Quick links
- Explore articles by subject
- Guide to authors
- Editorial policies
Sign up for the Nature Briefing newsletter — what matters in science, free to your inbox daily.


- High contrast
- Our mandate
- Our history
- Annual report
- PRESS CENTRE
Search UNICEF

For every child, answers
Research and foresight that drive change for children
Latest work

Youth, Protests and the Polycrisis
Exploring how youth protests can help to build public support for change

Early Childhood Education Systems in Pacific Islands
Status report

Cash Plus Model for Safe Transitions to Healthy Adulthood
Examining the impacts of “Ujana Salama” (‘Safe Youth’ in Swahili), a cash plus programme targeting adolescents in the United Republic of Tanzania

The Impact of Valor Criança
Social cash transfer pilot programme in Angola

The Impact of the Cash Transfer Intervention
In the commune of Nsélé in Kinshasa, Democratic Republic of the Congo

Mitigating the socioeconomic impacts of COVID-19
With a cash transfer in peri-urban Kinshasa, Democratic Republic of the Congo

Prospects for Children: A Global Outlook 2024
Cooperation in a Fragmented World: Discover the eight trends that will define the year ahead for children and young people.

Data Must Speak: Chad
Reports and project briefs
Areas of work

Adolescent participation and civic engagement

Child protection

Climate crisis and the environment

Digital technology

Education and human capital

Health and well-being

Inclusion and equity

Poverty and social protection

Social and behaviour change
The State of the World’s Children
UNICEF’s flagship report – the most comprehensive analysis of global trends affecting children
Changing Childhood Project
What is childhood like today?
Prospects for Children: Global Outlook
An annual analysis of trends shaping the world and their impact on children
Report Card
Understanding child well-being everywhere
Our approach
UNICEF Innocenti works for and with children and young people to seek solutions to their most pressing challenges. As we focus on the rights and lives of children and young people, we always ask: Who else can we include? Will this work cause unintended harm? Are there events that could surprise us? Does this work drive change?
Events and insights

Six ways to make Loss and Damage finance work for children
Climate change is hurting kids. Here is how we can address the harm

The Antidote to Ageism
Understanding the importance of intergenerational collaboration

Celebrating women in education
A closer look at female teachers and school leaders

As they move
Child and youth experiences of migration, displacement and return in Afghanistan

Launch of UNICEF's Youth Foresight Playbook
28 November 2023, Dubai Future Forum

Expert consultation on age-related public expenditure
12-13 April 2023

The Third Annual KIX Symposium
12-13 October 2022

UNICEF at the International SBCC Summit 2022
5-9 December 2022

Become an Innocenti Insider
Receive the latest research and event invites in your inbox once a month
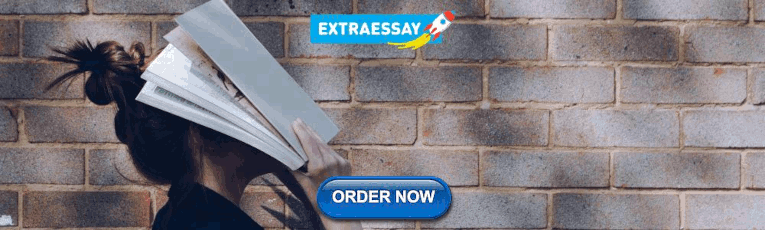
IMAGES
VIDEO
COMMENTS
Abstract. Against the background of the centrality of data for contemporary economies, the chapter contributes to a better understanding and contextualization of data protection and its interfaces ...
Introduction. This paper aims to critically assess the information duties set out in the General Data Protection Regulation (GDPR) and national adaptations when the purpose of processing is scientific research. Due to the peculiarities of the legal regime applicable to the research context, information about the processing plays a crucial role ...
B. Evolution of Personal Data Protection. The first data privacy legislation in the United States, 4 the Fair Credit Reporting Act (FCRA), was enacted in 1970. 5 The FCRA aimed to impose limits on data sharing in the consumer credit reporting industry and, in particular, to make it easier for individuals to correct reporting errors. 6 The FCRA ...
1. Introduction. The right to data protection enjoys a privileged position in the EU legal order. 1 The right is strictly interpreted by the Court of Justice of the EU (CJEU) and is given remarkable weight when balanced with other rights and interests. 2 While data protection sits alongside the more established right to respect for private life in the EU Charter, 3 it is data protection rather ...
The issue of data protection in research is becoming of pivotal importance, in particular in the last months with the pandemic emergency of COVID-19. 1 Studying the development of the outbreak on affected populations under a scientific and statistic perspective is necessary to understand the trend of contagion, the effectiveness of social distancing measures, the most vulnerable people who are ...
Data are today an asset more critical than ever for all organizations we may think of. Recent advances and trends, such as sensor systems, IoT, cloud computing, and data analytics, are making possible to pervasively, efficiently, and effectively collect data. However for data to be used to their full power, data security and privacy are critical. Even though data security and privacy have been ...
Five major streams of research inform our work in this paper: (1) technology adoption model (TAM), (2) consumer privacy paradox, (3) service failure, (4) protection motivation theory (PMT), and (5) trust. ... Third, most research on data breaches has focused mainly on post-breach analysis, that is, the impact of data breach. ... Both CCPA and ...
Purpose of the Study. This paper aims to examine the two case studies of the United States and India to show. that they do not have adequate data protection regulations to provide the right to privacy and. suggest ways that these two countries may move further towards the path of adopting adequate.
The increased dependency on technology brings national security to the forefront of concerns of the 21st century. It creates many challenges to developing and developed nations in their effort to counter cyber threats and adds to the inherent risk factors associated with technology. The failure to securely protect data would potentially give rise to far-reaching catastrophic consequences ...
The phrase "Big Data" refers to the vast and ever-increasing volumes of data that might overwhelm an organization (Ur Rehman et al., 2016).It gathers massive, broad, and multi-format data streams from disparate and independent data sources (X. Wu et al., 2014).Big Data is believed to have five properties, which are known as the five V's: volume, velocity, variety, veracity, and valence ...
Three strategies are summarized in this section. The first is for clinical data and provides a practical user access rating system, and the second is majority for genomic data and designs a network architecture to address both security access and potential risk of privacy disclosure and reidentification.
provides an introduction to data protection laws and an overview of considerations for Congress. (For a more detailed analysis, see CRS Report R45631, Data Protection Law: An Overview, by Stephen P. Mulligan, Wilson C. Freeman, and Chris D. Linebaugh.) Defining Data Protection As a legislative concept, data protection melds the fields of
Under the General Data Protection Regulation (GDPR), companies are obliged to protect personal data and safeguard the data protection rights of all individuals in the EU area. ... This research paper reviews the existing literature and open data sources related to cybersecurity and cyber risk, focusing on the datasets used to improve academic ...
33 LIBE Compromise, proposal for a Data Protection Regulation (this paper refers to the unofficial Consolidated Version after LIBE Committee Vote, provided by the Rapporteur, General Data Protection Regulation, 22 October 2013. The European Parliament is an EU body with legislative, supervisory, and budgetary responsibilities. ... Amsterdam Law ...
February 2019 regarding data protection at the EUI. President's Decision 10/2019 has adapted the EUI's Data Protection Policy to the new General Data Protection Regulation (GDPR) and Regulation 1725/2018. The goal of this guide is also to provide researchers with a handy tool to guide them through the daily work on their research project.
The Data Protection Act 2018 affords further protection by providing that the research condition (Article 9 2 . (j)) will only be met if the processing is in the public interest (Schedule 1. Part 1. 4. Public interest is a difficult concept to define and no attempt is made to do this in the Data Protection Act 2018.
This paper is divided into three parts with the first discussing the rights of users and responsibilities of companies as well as the established regulations in the protection of data. The second part of this work considers the issues surrounding data privacy and data protection and the challenges faced in ensuring the safety of users ...
This paper has examined a particular kind of research and publication misconduct, namely, the misuse of anonymity and confidentiality protections in the production of unreliable qualitative data. Analysis of this phenomenon included an exercise in PPPR that argued for the unreliability of the qualitative data in an article published in an ...
With data protection law, the UK and EU demonstrate cooperation, ethics, transparency with robust control methods in mitigating data privacy breaches. ... relatively new technology—just over a decade old—it seems to be revolutionary and there is a substantial number of research articles and white papers to justify this remark. Blockchain ...
This chapter explores the alignment of the EU data protection and consumer protection policy agendas through a discussion of the reference to the Unfair Contract Terms Directive in Recital 42 of the General Data Protection Regulation. ... Australian National University College of Law Legal Studies Research Paper Series. Subscribe to this free ...
This paper presents a systematic bibliometric analysis of the artificial intelligence (AI) domain to explore privacy protection research as AI technologies integrate and data privacy concerns rise. Understanding evolutionary patterns and current trends in this research is crucial. Leveraging bibliometric techniques, the authors analyze 8,322 papers from the Web of Science (WoS) database ...
In the research time, the Principal Investigator is ultimately responsible for the integrity of the stored data. The data protections and confidentiality protocols should be in place before the project starts, and includes aspects like theft, loss or tampering of the data. The easy way to do this is to limit access to the research data.
After applying the exclusion criteria, the final data set contained 994 tweets. Inductive and deductive approaches informed our qualitative content analysis. Results: A total of 4 themes emerged, with 50.1% (498/994) of posts framing older Asian Americans as "vulnerable and in need of protection" (theme 1).
Four pathways with the highest current carbon market activity and high mitigation potential (tropical and temperate forest conservation and reforestation; Table 1 and Supplementary Data), were ...
Research and foresight that drive change for children Our projects and reports. Latest work ... Data Must Speak: Chad Reports and project briefs See the full report. ... Poverty and social protection. Social and behaviour change. Explore our areas of work. Spotlight