
Statistics Made Easy
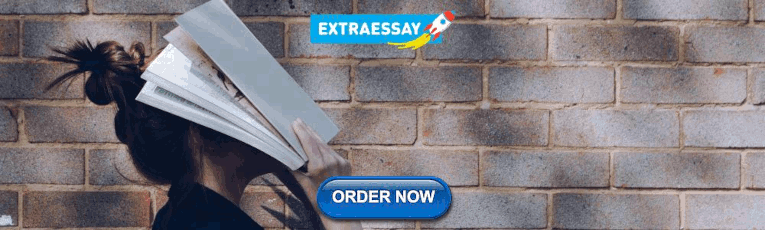
What is a Directional Hypothesis? (Definition & Examples)
A statistical hypothesis is an assumption about a population parameter . For example, we may assume that the mean height of a male in the U.S. is 70 inches.
The assumption about the height is the statistical hypothesis and the true mean height of a male in the U.S. is the population parameter .
To test whether a statistical hypothesis about a population parameter is true, we obtain a random sample from the population and perform a hypothesis test on the sample data.
Whenever we perform a hypothesis test, we always write down a null and alternative hypothesis:
- Null Hypothesis (H 0 ): The sample data occurs purely from chance.
- Alternative Hypothesis (H A ): The sample data is influenced by some non-random cause.
A hypothesis test can either contain a directional hypothesis or a non-directional hypothesis:
- Directional hypothesis: The alternative hypothesis contains the less than (“<“) or greater than (“>”) sign. This indicates that we’re testing whether or not there is a positive or negative effect.
- Non-directional hypothesis: The alternative hypothesis contains the not equal (“≠”) sign. This indicates that we’re testing whether or not there is some effect, without specifying the direction of the effect.
Note that directional hypothesis tests are also called “one-tailed” tests and non-directional hypothesis tests are also called “two-tailed” tests.
Check out the following examples to gain a better understanding of directional vs. non-directional hypothesis tests.
Example 1: Baseball Programs
A baseball coach believes a certain 4-week program will increase the mean hitting percentage of his players, which is currently 0.285.
To test this, he measures the hitting percentage of each of his players before and after participating in the program.
He then performs a hypothesis test using the following hypotheses:
- H 0 : μ = .285 (the program will have no effect on the mean hitting percentage)
- H A : μ > .285 (the program will cause mean hitting percentage to increase)
This is an example of a directional hypothesis because the alternative hypothesis contains the greater than “>” sign. The coach believes that the program will influence the mean hitting percentage of his players in a positive direction.
Example 2: Plant Growth
A biologist believes that a certain pesticide will cause plants to grow less during a one-month period than they normally do, which is currently 10 inches.
To test this, she applies the pesticide to each of the plants in her laboratory for one month.
She then performs a hypothesis test using the following hypotheses:
- H 0 : μ = 10 inches (the pesticide will have no effect on the mean plant growth)
- H A : μ < 10 inches (the pesticide will cause mean plant growth to decrease)
This is also an example of a directional hypothesis because the alternative hypothesis contains the less than “<” sign. The biologist believes that the pesticide will influence the mean plant growth in a negative direction.
Example 3: Studying Technique
A professor believes that a certain studying technique will influence the mean score that her students receive on a certain exam, but she’s unsure if it will increase or decrease the mean score, which is currently 82.
To test this, she lets each student use the studying technique for one month leading up to the exam and then administers the same exam to each of the students.
- H 0 : μ = 82 (the studying technique will have no effect on the mean exam score)
- H A : μ ≠ 82 (the studying technique will cause the mean exam score to be different than 82)
This is an example of a non-directional hypothesis because the alternative hypothesis contains the not equal “≠” sign. The professor believes that the studying technique will influence the mean exam score, but doesn’t specify whether it will cause the mean score to increase or decrease.
Additional Resources
Introduction to Hypothesis Testing Introduction to the One Sample t-test Introduction to the Two Sample t-test Introduction to the Paired Samples t-test
Featured Posts

Hey there. My name is Zach Bobbitt. I have a Masters of Science degree in Applied Statistics and I’ve worked on machine learning algorithms for professional businesses in both healthcare and retail. I’m passionate about statistics, machine learning, and data visualization and I created Statology to be a resource for both students and teachers alike. My goal with this site is to help you learn statistics through using simple terms, plenty of real-world examples, and helpful illustrations.
Leave a Reply Cancel reply
Your email address will not be published. Required fields are marked *
Join the Statology Community
Sign up to receive Statology's exclusive study resource: 100 practice problems with step-by-step solutions. Plus, get our latest insights, tutorials, and data analysis tips straight to your inbox!
By subscribing you accept Statology's Privacy Policy.

Directional Hypothesis: Definition and 10 Examples
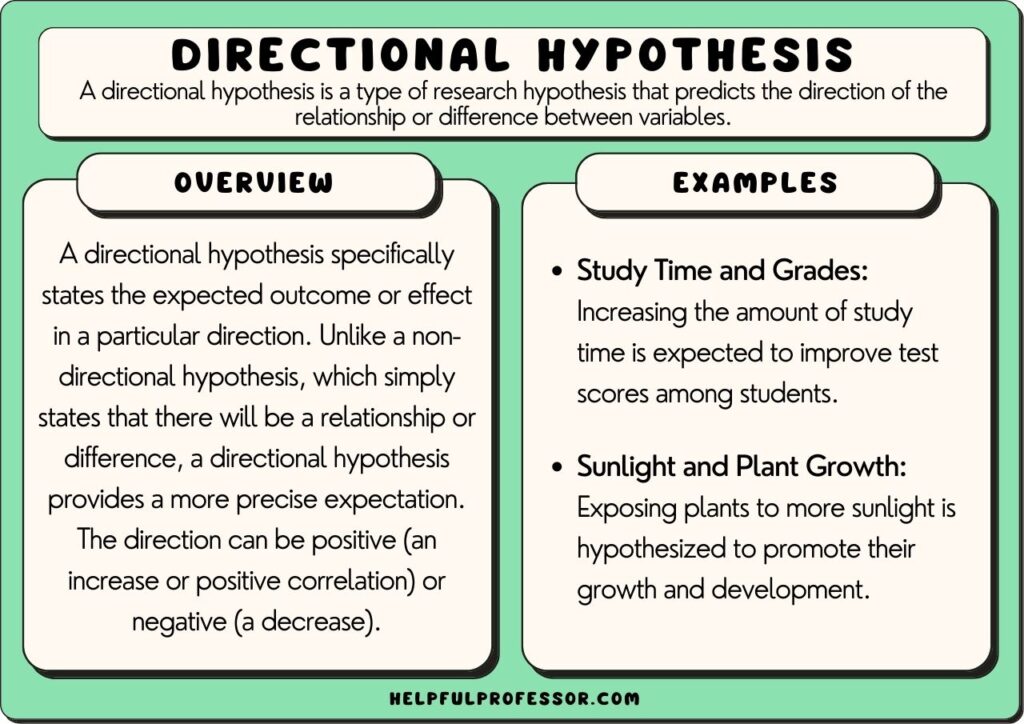
A directional hypothesis refers to a type of hypothesis used in statistical testing that predicts a particular direction of the expected relationship between two variables.
In simpler terms, a directional hypothesis is an educated, specific guess about the direction of an outcome—whether an increase, decrease, or a proclaimed difference in variable sets.
For example, in a study investigating the effects of sleep deprivation on cognitive performance, a directional hypothesis might state that as sleep deprivation (Independent Variable) increases, cognitive performance (Dependent Variable) decreases (Killgore, 2010). Such a hypothesis offers a clear, directional relationship whereby a specific increase or decrease is anticipated.
Global warming provides another notable example of a directional hypothesis. A researcher might hypothesize that as carbon dioxide (CO2) levels increase, global temperatures also increase (Thompson, 2010). In this instance, the hypothesis clearly articulates an upward trend for both variables.
In any given circumstance, it’s imperative that a directional hypothesis is grounded on solid evidence. For instance, the CO2 and global temperature relationship is based on substantial scientific evidence, and not on a random guess or mere speculation (Florides & Christodoulides, 2009).
Directional vs Non-Directional vs Null Hypotheses
A directional hypothesis is generally contrasted to a non-directional hypothesis. Here’s how they compare:
- Directional hypothesis: A directional hypothesis provides a perspective of the expected relationship between variables, predicting the direction of that relationship (either positive, negative, or a specific difference).
- Non-directional hypothesis: A non-directional hypothesis denotes the possibility of a relationship between two variables ( the independent and dependent variables ), although this hypothesis does not venture a prediction as to the direction of this relationship (Ali & Bhaskar, 2016). For example, a non-directional hypothesis might state that there exists a relationship between a person’s diet (independent variable) and their mood (dependent variable), without indicating whether improvement in diet enhances mood positively or negatively. Overall, the choice between a directional or non-directional hypothesis depends on the known or anticipated link between the variables under consideration in research studies.
Another very important type of hypothesis that we need to know about is a null hypothesis :
- Null hypothesis : The null hypothesis stands as a universality—the hypothesis that there is no observed effect in the population under study, meaning there is no association between variables (or that the differences are down to chance). For instance, a null hypothesis could be constructed around the idea that changing diet (independent variable) has no discernible effect on a person’s mood (dependent variable) (Yan & Su, 2016). This proposition is the one that we aim to disprove in an experiment.
While directional and non-directional hypotheses involve some integrated expectations about the outcomes (either distinct direction or a vague relationship), a null hypothesis operates on the premise of negating such relationships or effects.
The null hypotheses is typically proposed to be negated or disproved by statistical tests, paving way for the acceptance of an alternate hypothesis (either directional or non-directional).
Directional Hypothesis Examples
1. exercise and heart health.
Research suggests that as regular physical exercise (independent variable) increases, the risk of heart disease (dependent variable) decreases (Jakicic, Davis, Rogers, King, Marcus, Helsel, Rickman, Wahed, Belle, 2016). In this example, a directional hypothesis anticipates that the more individuals maintain routine workouts, the lesser would be their odds of developing heart-related disorders. This assumption is based on the underlying fact that routine exercise can help reduce harmful cholesterol levels, regulate blood pressure, and bring about overall health benefits. Thus, a direction – a decrease in heart disease – is expected in relation with an increase in exercise.
2. Screen Time and Sleep Quality
Another classic instance of a directional hypothesis can be seen in the relationship between the independent variable, screen time (especially before bed), and the dependent variable, sleep quality. This hypothesis predicts that as screen time before bed increases, sleep quality decreases (Chang, Aeschbach, Duffy, Czeisler, 2015). The reasoning behind this hypothesis is the disruptive effect of artificial light (especially blue light from screens) on melatonin production, a hormone needed to regulate sleep. As individuals spend more time exposed to screens before bed, it is predictably hypothesized that their sleep quality worsens.
3. Job Satisfaction and Employee Turnover
A typical scenario in organizational behavior research posits that as job satisfaction (independent variable) increases, the rate of employee turnover (dependent variable) decreases (Cheng, Jiang, & Riley, 2017). This directional hypothesis emphasizes that an increased level of job satisfaction would lead to a reduced rate of employees leaving the company. The theoretical basis for this hypothesis is that satisfied employees often tend to be more committed to the organization and are less likely to seek employment elsewhere, thus reducing turnover rates.
4. Healthy Eating and Body Weight
Healthy eating, as the independent variable, is commonly thought to influence body weight, the dependent variable, in a positive way. For example, the hypothesis might state that as consumption of healthy foods increases, an individual’s body weight decreases (Framson, Kristal, Schenk, Littman, Zeliadt, & Benitez, 2009). This projection is based on the premise that healthier foods, such as fruits and vegetables, are generally lower in calories than junk food, assisting in weight management.
5. Sun Exposure and Skin Health
The association between sun exposure (independent variable) and skin health (dependent variable) allows for a definitive hypothesis declaring that as sun exposure increases, the risk of skin damage or skin cancer increases (Whiteman, Whiteman, & Green, 2001). The premise aligns with the understanding that overexposure to the sun’s ultraviolet rays can deteriorate skin health, leading to conditions like sunburn or, in extreme cases, skin cancer.
6. Study Hours and Academic Performance
A regularly assessed relationship in academia suggests that as the number of study hours (independent variable) rises, so too does academic performance (dependent variable) (Nonis, Hudson, Logan, Ford, 2013). The hypothesis proposes a positive correlation , with an increase in study time expected to contribute to enhanced academic outcomes.
7. Screen Time and Eye Strain
It’s commonly hypothesized that as screen time (independent variable) increases, the likelihood of experiencing eye strain (dependent variable) also increases (Sheppard & Wolffsohn, 2018). This is based on the idea that prolonged engagement with digital screens—computers, tablets, or mobile phones—can cause discomfort or fatigue in the eyes, attributing to symptoms of eye strain.
8. Physical Activity and Stress Levels
In the sphere of mental health, it’s often proposed that as physical activity (independent variable) increases, levels of stress (dependent variable) decrease (Stonerock, Hoffman, Smith, Blumenthal, 2015). Regular exercise is known to stimulate the production of endorphins, the body’s natural mood elevators, helping to alleviate stress.
9. Water Consumption and Kidney Health
A common health-related hypothesis might predict that as water consumption (independent variable) increases, the risk of kidney stones (dependent variable) decreases (Curhan, Willett, Knight, & Stampfer, 2004). Here, an increase in water intake is inferred to reduce the risk of kidney stones by diluting the substances that lead to stone formation.
10. Traffic Noise and Sleep Quality
In urban planning research, it’s often supposed that as traffic noise (independent variable) increases, sleep quality (dependent variable) decreases (Muzet, 2007). Increased noise levels, particularly during the night, can result in sleep disruptions, thus, leading to poor sleep quality.
11. Sugar Consumption and Dental Health
In the field of dental health, an example might be stating as one’s sugar consumption (independent variable) increases, dental health (dependent variable) decreases (Sheiham, & James, 2014). This stems from the fact that sugar is a major factor in tooth decay, and increased consumption of sugary foods or drinks leads to a decline in dental health due to the high likelihood of cavities.
See 15 More Examples of Hypotheses Here
A directional hypothesis plays a critical role in research, paving the way for specific predicted outcomes based on the relationship between two variables. These hypotheses clearly illuminate the expected direction—the increase or decrease—of an effect. From predicting the impacts of healthy eating on body weight to forecasting the influence of screen time on sleep quality, directional hypotheses allow for targeted and strategic examination of phenomena. In essence, directional hypotheses provide the crucial path for inquiry, shaping the trajectory of research studies and ultimately aiding in the generation of insightful, relevant findings.
Ali, S., & Bhaskar, S. (2016). Basic statistical tools in research and data analysis. Indian Journal of Anaesthesia, 60 (9), 662-669. doi: https://doi.org/10.4103%2F0019-5049.190623
Chang, A. M., Aeschbach, D., Duffy, J. F., & Czeisler, C. A. (2015). Evening use of light-emitting eReaders negatively affects sleep, circadian timing, and next-morning alertness. Proceeding of the National Academy of Sciences, 112 (4), 1232-1237. doi: https://doi.org/10.1073/pnas.1418490112
Cheng, G. H. L., Jiang, D., & Riley, J. H. (2017). Organizational commitment and intrinsic motivation of regular and contractual primary school teachers in China. New Psychology, 19 (3), 316-326. Doi: https://doi.org/10.4103%2F2249-4863.184631
Curhan, G. C., Willett, W. C., Knight, E. L., & Stampfer, M. J. (2004). Dietary factors and the risk of incident kidney stones in younger women: Nurses’ Health Study II. Archives of Internal Medicine, 164 (8), 885–891.
Florides, G. A., & Christodoulides, P. (2009). Global warming and carbon dioxide through sciences. Environment international , 35 (2), 390-401. doi: https://doi.org/10.1016/j.envint.2008.07.007
Framson, C., Kristal, A. R., Schenk, J. M., Littman, A. J., Zeliadt, S., & Benitez, D. (2009). Development and validation of the mindful eating questionnaire. Journal of the American Dietetic Association, 109 (8), 1439-1444. doi: https://doi.org/10.1016/j.jada.2009.05.006
Jakicic, J. M., Davis, K. K., Rogers, R. J., King, W. C., Marcus, M. D., Helsel, D., … & Belle, S. H. (2016). Effect of wearable technology combined with a lifestyle intervention on long-term weight loss: The IDEA randomized clinical trial. JAMA, 316 (11), 1161-1171.
Khan, S., & Iqbal, N. (2013). Study of the relationship between study habits and academic achievement of students: A case of SPSS model. Higher Education Studies, 3 (1), 14-26.
Killgore, W. D. (2010). Effects of sleep deprivation on cognition. Progress in brain research , 185 , 105-129. doi: https://doi.org/10.1016/B978-0-444-53702-7.00007-5
Marczinski, C. A., & Fillmore, M. T. (2014). Dissociative antagonistic effects of caffeine on alcohol-induced impairment of behavioral control. Experimental and Clinical Psychopharmacology, 22 (4), 298–311. doi: https://psycnet.apa.org/doi/10.1037/1064-1297.11.3.228
Muzet, A. (2007). Environmental Noise, Sleep and Health. Sleep Medicine Reviews, 11 (2), 135-142. doi: https://doi.org/10.1016/j.smrv.2006.09.001
Nonis, S. A., Hudson, G. I., Logan, L. B., & Ford, C. W. (2013). Influence of perceived control over time on college students’ stress and stress-related outcomes. Research in Higher Education, 54 (5), 536-552. doi: https://doi.org/10.1023/A:1018753706925
Sheiham, A., & James, W. P. (2014). A new understanding of the relationship between sugars, dental caries and fluoride use: implications for limits on sugars consumption. Public health nutrition, 17 (10), 2176-2184. Doi: https://doi.org/10.1017/S136898001400113X
Sheppard, A. L., & Wolffsohn, J. S. (2018). Digital eye strain: prevalence, measurement and amelioration. BMJ open ophthalmology , 3 (1), e000146. doi: http://dx.doi.org/10.1136/bmjophth-2018-000146
Stonerock, G. L., Hoffman, B. M., Smith, P. J., & Blumenthal, J. A. (2015). Exercise as Treatment for Anxiety: Systematic Review and Analysis. Annals of Behavioral Medicine, 49 (4), 542–556. doi: https://doi.org/10.1007/s12160-014-9685-9
Thompson, L. G. (2010). Climate change: The evidence and our options. The Behavior Analyst , 33 , 153-170. Doi: https://doi.org/10.1007/BF03392211
Whiteman, D. C., Whiteman, C. A., & Green, A. C. (2001). Childhood sun exposure as a risk factor for melanoma: a systematic review of epidemiologic studies. Cancer Causes & Control, 12 (1), 69-82. doi: https://doi.org/10.1023/A:1008980919928
Yan, X., & Su, X. (2009). Linear regression analysis: theory and computing . New Jersey: World Scientific.
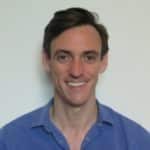
Chris Drew (PhD)
Dr. Chris Drew is the founder of the Helpful Professor. He holds a PhD in education and has published over 20 articles in scholarly journals. He is the former editor of the Journal of Learning Development in Higher Education. [Image Descriptor: Photo of Chris]
- Chris Drew (PhD) https://helpfulprofessor.com/author/chris-drew-phd/ Social-Emotional Learning (Definition, Examples, Pros & Cons)
- Chris Drew (PhD) https://helpfulprofessor.com/author/chris-drew-phd/ What is Educational Psychology?
- Chris Drew (PhD) https://helpfulprofessor.com/author/chris-drew-phd/ What is IQ? (Intelligence Quotient)
- Chris Drew (PhD) https://helpfulprofessor.com/author/chris-drew-phd/ 5 Top Tips for Succeeding at University
Leave a Comment Cancel Reply
Your email address will not be published. Required fields are marked *
Research Hypothesis In Psychology: Types, & Examples
Saul Mcleod, PhD
Editor-in-Chief for Simply Psychology
BSc (Hons) Psychology, MRes, PhD, University of Manchester
Saul Mcleod, PhD., is a qualified psychology teacher with over 18 years of experience in further and higher education. He has been published in peer-reviewed journals, including the Journal of Clinical Psychology.
Learn about our Editorial Process
Olivia Guy-Evans, MSc
Associate Editor for Simply Psychology
BSc (Hons) Psychology, MSc Psychology of Education
Olivia Guy-Evans is a writer and associate editor for Simply Psychology. She has previously worked in healthcare and educational sectors.
On This Page:
A research hypothesis, in its plural form “hypotheses,” is a specific, testable prediction about the anticipated results of a study, established at its outset. It is a key component of the scientific method .
Hypotheses connect theory to data and guide the research process towards expanding scientific understanding
Some key points about hypotheses:
- A hypothesis expresses an expected pattern or relationship. It connects the variables under investigation.
- It is stated in clear, precise terms before any data collection or analysis occurs. This makes the hypothesis testable.
- A hypothesis must be falsifiable. It should be possible, even if unlikely in practice, to collect data that disconfirms rather than supports the hypothesis.
- Hypotheses guide research. Scientists design studies to explicitly evaluate hypotheses about how nature works.
- For a hypothesis to be valid, it must be testable against empirical evidence. The evidence can then confirm or disprove the testable predictions.
- Hypotheses are informed by background knowledge and observation, but go beyond what is already known to propose an explanation of how or why something occurs.
Predictions typically arise from a thorough knowledge of the research literature, curiosity about real-world problems or implications, and integrating this to advance theory. They build on existing literature while providing new insight.
Types of Research Hypotheses
Alternative hypothesis.
The research hypothesis is often called the alternative or experimental hypothesis in experimental research.
It typically suggests a potential relationship between two key variables: the independent variable, which the researcher manipulates, and the dependent variable, which is measured based on those changes.
The alternative hypothesis states a relationship exists between the two variables being studied (one variable affects the other).
A hypothesis is a testable statement or prediction about the relationship between two or more variables. It is a key component of the scientific method. Some key points about hypotheses:
- Important hypotheses lead to predictions that can be tested empirically. The evidence can then confirm or disprove the testable predictions.
In summary, a hypothesis is a precise, testable statement of what researchers expect to happen in a study and why. Hypotheses connect theory to data and guide the research process towards expanding scientific understanding.
An experimental hypothesis predicts what change(s) will occur in the dependent variable when the independent variable is manipulated.
It states that the results are not due to chance and are significant in supporting the theory being investigated.
The alternative hypothesis can be directional, indicating a specific direction of the effect, or non-directional, suggesting a difference without specifying its nature. It’s what researchers aim to support or demonstrate through their study.
Null Hypothesis
The null hypothesis states no relationship exists between the two variables being studied (one variable does not affect the other). There will be no changes in the dependent variable due to manipulating the independent variable.
It states results are due to chance and are not significant in supporting the idea being investigated.
The null hypothesis, positing no effect or relationship, is a foundational contrast to the research hypothesis in scientific inquiry. It establishes a baseline for statistical testing, promoting objectivity by initiating research from a neutral stance.
Many statistical methods are tailored to test the null hypothesis, determining the likelihood of observed results if no true effect exists.
This dual-hypothesis approach provides clarity, ensuring that research intentions are explicit, and fosters consistency across scientific studies, enhancing the standardization and interpretability of research outcomes.
Nondirectional Hypothesis
A non-directional hypothesis, also known as a two-tailed hypothesis, predicts that there is a difference or relationship between two variables but does not specify the direction of this relationship.
It merely indicates that a change or effect will occur without predicting which group will have higher or lower values.
For example, “There is a difference in performance between Group A and Group B” is a non-directional hypothesis.
Directional Hypothesis
A directional (one-tailed) hypothesis predicts the nature of the effect of the independent variable on the dependent variable. It predicts in which direction the change will take place. (i.e., greater, smaller, less, more)
It specifies whether one variable is greater, lesser, or different from another, rather than just indicating that there’s a difference without specifying its nature.
For example, “Exercise increases weight loss” is a directional hypothesis.
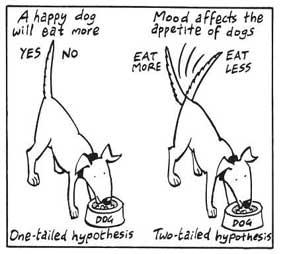
Falsifiability
The Falsification Principle, proposed by Karl Popper , is a way of demarcating science from non-science. It suggests that for a theory or hypothesis to be considered scientific, it must be testable and irrefutable.
Falsifiability emphasizes that scientific claims shouldn’t just be confirmable but should also have the potential to be proven wrong.
It means that there should exist some potential evidence or experiment that could prove the proposition false.
However many confirming instances exist for a theory, it only takes one counter observation to falsify it. For example, the hypothesis that “all swans are white,” can be falsified by observing a black swan.
For Popper, science should attempt to disprove a theory rather than attempt to continually provide evidence to support a research hypothesis.
Can a Hypothesis be Proven?
Hypotheses make probabilistic predictions. They state the expected outcome if a particular relationship exists. However, a study result supporting a hypothesis does not definitively prove it is true.
All studies have limitations. There may be unknown confounding factors or issues that limit the certainty of conclusions. Additional studies may yield different results.
In science, hypotheses can realistically only be supported with some degree of confidence, not proven. The process of science is to incrementally accumulate evidence for and against hypothesized relationships in an ongoing pursuit of better models and explanations that best fit the empirical data. But hypotheses remain open to revision and rejection if that is where the evidence leads.
- Disproving a hypothesis is definitive. Solid disconfirmatory evidence will falsify a hypothesis and require altering or discarding it based on the evidence.
- However, confirming evidence is always open to revision. Other explanations may account for the same results, and additional or contradictory evidence may emerge over time.
We can never 100% prove the alternative hypothesis. Instead, we see if we can disprove, or reject the null hypothesis.
If we reject the null hypothesis, this doesn’t mean that our alternative hypothesis is correct but does support the alternative/experimental hypothesis.
Upon analysis of the results, an alternative hypothesis can be rejected or supported, but it can never be proven to be correct. We must avoid any reference to results proving a theory as this implies 100% certainty, and there is always a chance that evidence may exist which could refute a theory.
How to Write a Hypothesis
- Identify variables . The researcher manipulates the independent variable and the dependent variable is the measured outcome.
- Operationalized the variables being investigated . Operationalization of a hypothesis refers to the process of making the variables physically measurable or testable, e.g. if you are about to study aggression, you might count the number of punches given by participants.
- Decide on a direction for your prediction . If there is evidence in the literature to support a specific effect of the independent variable on the dependent variable, write a directional (one-tailed) hypothesis. If there are limited or ambiguous findings in the literature regarding the effect of the independent variable on the dependent variable, write a non-directional (two-tailed) hypothesis.
- Make it Testable : Ensure your hypothesis can be tested through experimentation or observation. It should be possible to prove it false (principle of falsifiability).
- Clear & concise language . A strong hypothesis is concise (typically one to two sentences long), and formulated using clear and straightforward language, ensuring it’s easily understood and testable.
Consider a hypothesis many teachers might subscribe to: students work better on Monday morning than on Friday afternoon (IV=Day, DV= Standard of work).
Now, if we decide to study this by giving the same group of students a lesson on a Monday morning and a Friday afternoon and then measuring their immediate recall of the material covered in each session, we would end up with the following:
- The alternative hypothesis states that students will recall significantly more information on a Monday morning than on a Friday afternoon.
- The null hypothesis states that there will be no significant difference in the amount recalled on a Monday morning compared to a Friday afternoon. Any difference will be due to chance or confounding factors.
More Examples
- Memory : Participants exposed to classical music during study sessions will recall more items from a list than those who studied in silence.
- Social Psychology : Individuals who frequently engage in social media use will report higher levels of perceived social isolation compared to those who use it infrequently.
- Developmental Psychology : Children who engage in regular imaginative play have better problem-solving skills than those who don’t.
- Clinical Psychology : Cognitive-behavioral therapy will be more effective in reducing symptoms of anxiety over a 6-month period compared to traditional talk therapy.
- Cognitive Psychology : Individuals who multitask between various electronic devices will have shorter attention spans on focused tasks than those who single-task.
- Health Psychology : Patients who practice mindfulness meditation will experience lower levels of chronic pain compared to those who don’t meditate.
- Organizational Psychology : Employees in open-plan offices will report higher levels of stress than those in private offices.
- Behavioral Psychology : Rats rewarded with food after pressing a lever will press it more frequently than rats who receive no reward.

Related Articles
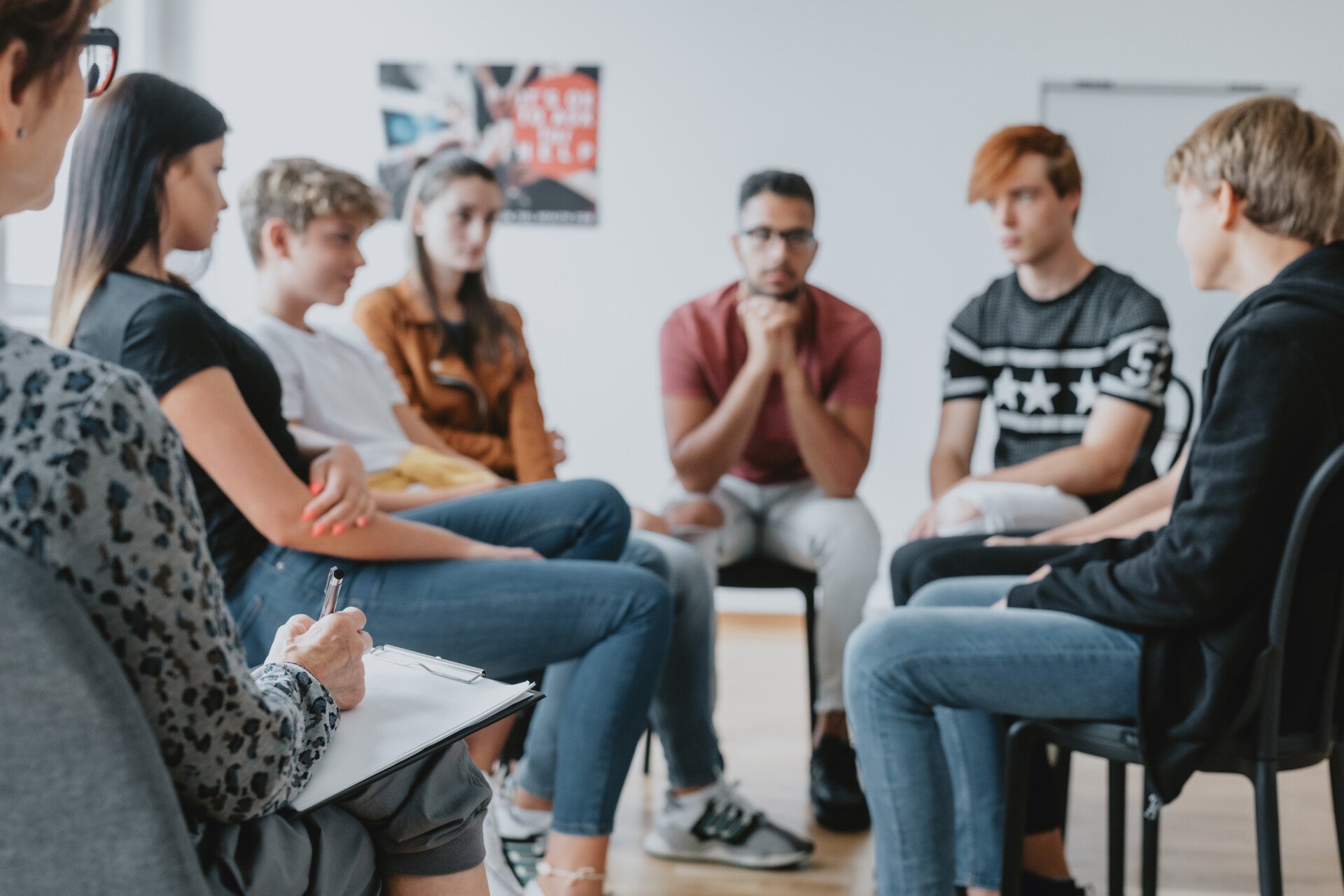
Research Methodology
What Is a Focus Group?
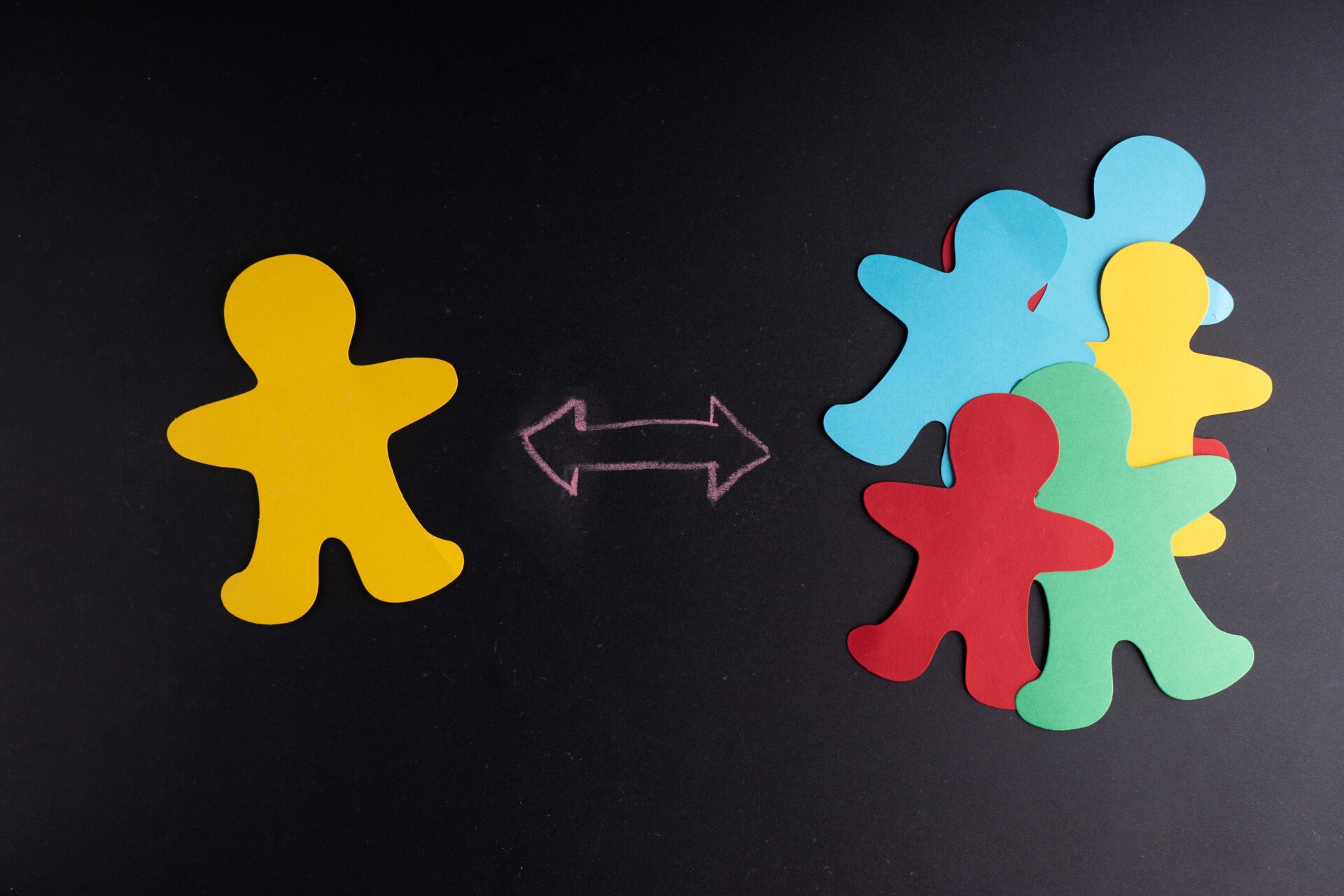
Cross-Cultural Research Methodology In Psychology
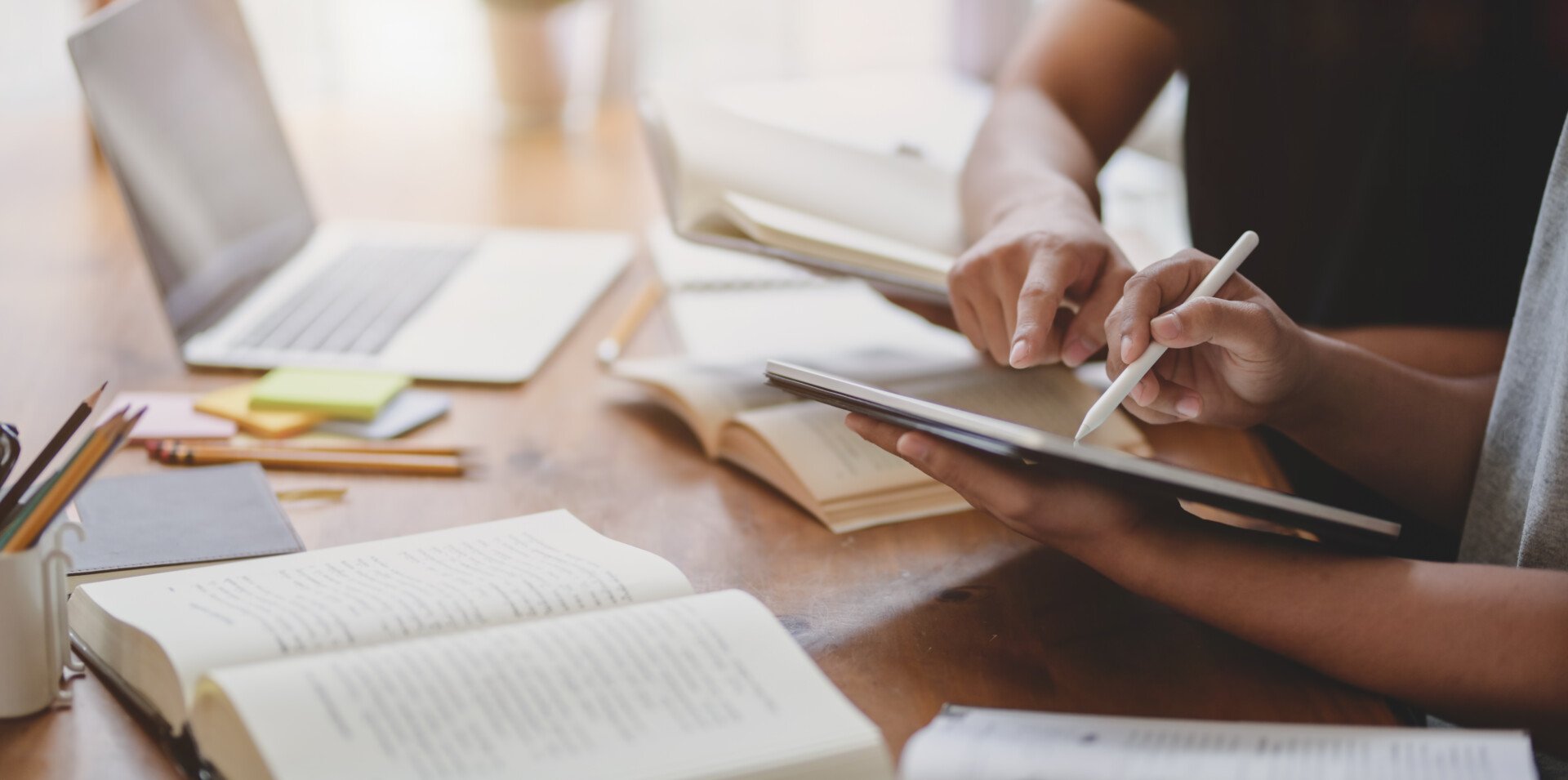
What Is Internal Validity In Research?
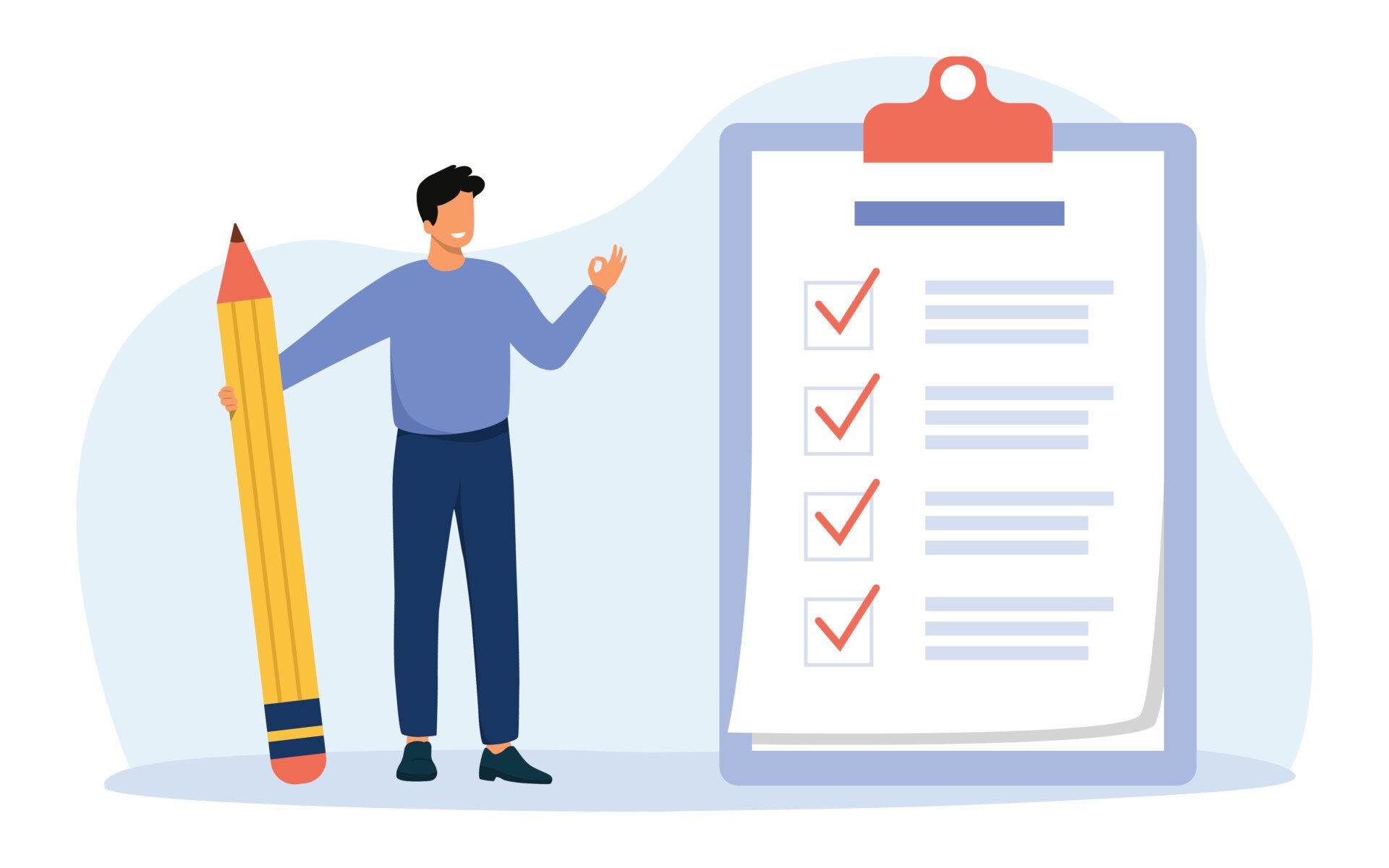
Research Methodology , Statistics
What Is Face Validity In Research? Importance & How To Measure
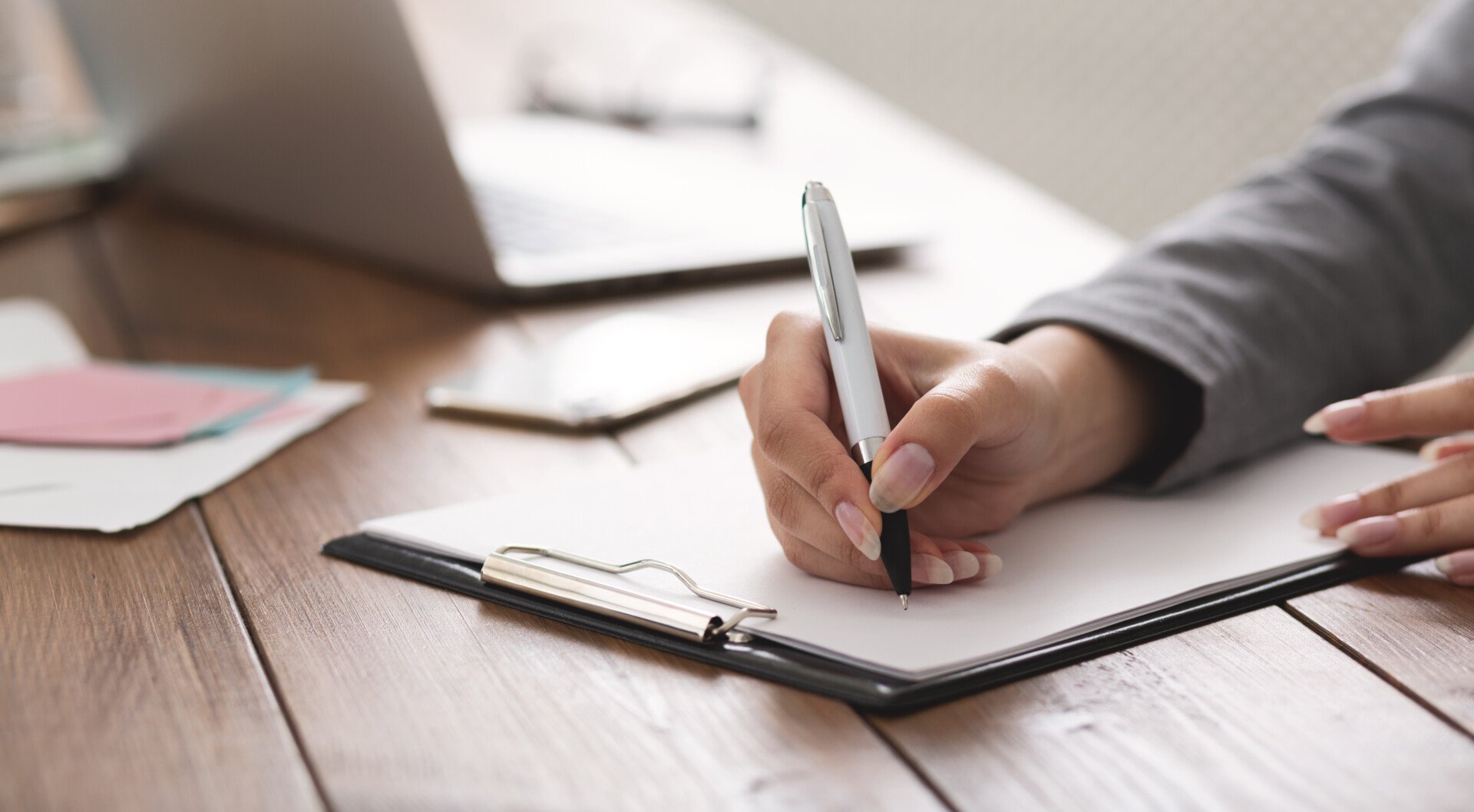
Criterion Validity: Definition & Examples
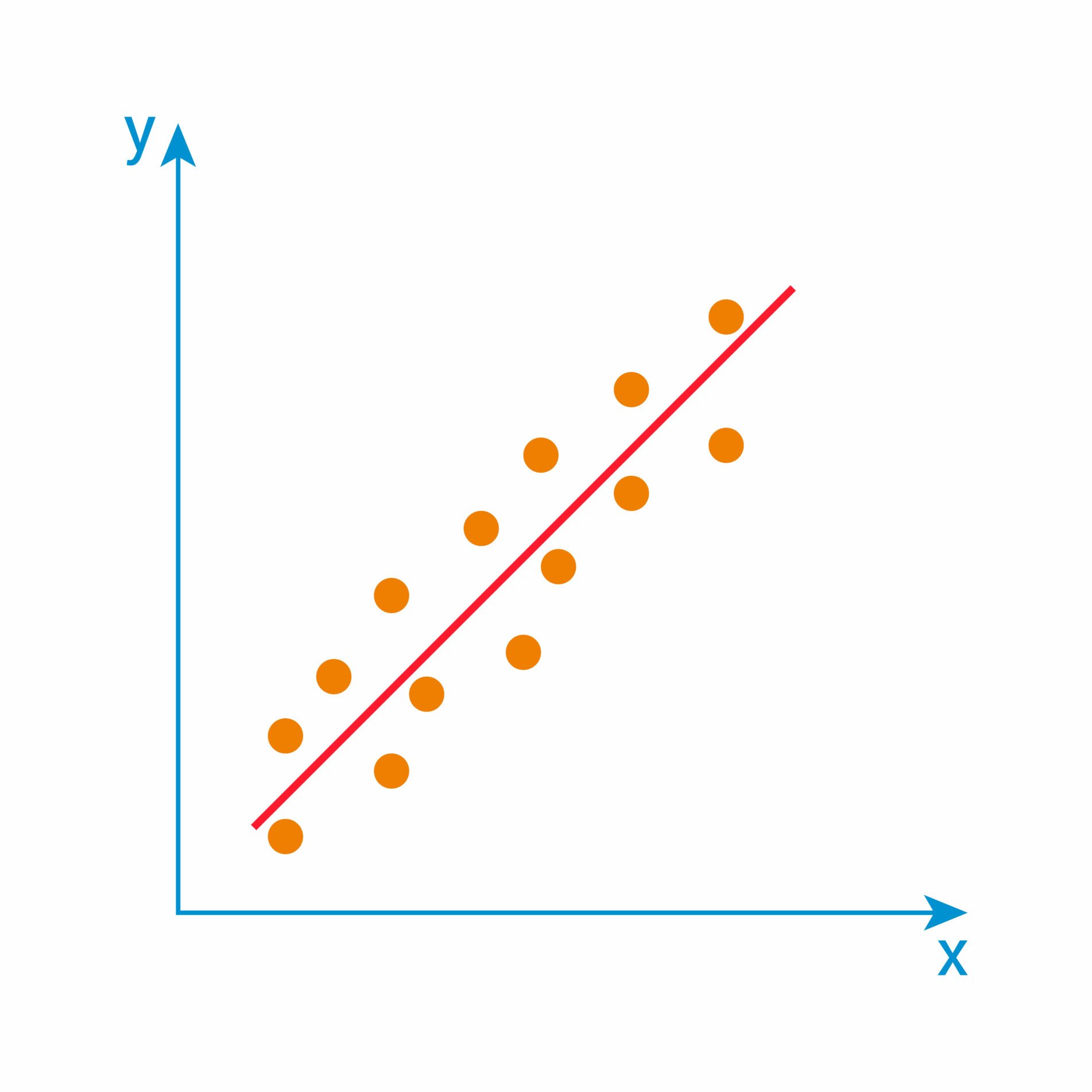
Convergent Validity: Definition and Examples
Directional and non-directional hypothesis: A Comprehensive Guide
Karolina Konopka
Customer support manager
In the world of research and statistical analysis, hypotheses play a crucial role in formulating and testing scientific claims. Understanding the differences between directional and non-directional hypothesis is essential for designing sound experiments and drawing accurate conclusions. Whether you’re a student, researcher, or simply curious about the foundations of hypothesis testing, this guide will equip you with the knowledge and tools to navigate this fundamental aspect of scientific inquiry.
Understanding Directional Hypothesis
Understanding directional hypotheses is crucial for conducting hypothesis-driven research, as they guide the selection of appropriate statistical tests and aid in the interpretation of results. By incorporating directional hypotheses, researchers can make more precise predictions, contribute to scientific knowledge, and advance their fields of study.
Definition of directional hypothesis
Directional hypotheses, also known as one-tailed hypotheses, are statements in research that make specific predictions about the direction of a relationship or difference between variables. Unlike non-directional hypotheses, which simply state that there is a relationship or difference without specifying its direction, directional hypotheses provide a focused and precise expectation.
A directional hypothesis predicts either a positive or negative relationship between variables or predicts that one group will perform better than another. It asserts a specific direction of effect or outcome. For example, a directional hypothesis could state that “increased exposure to sunlight will lead to an improvement in mood” or “participants who receive the experimental treatment will exhibit higher levels of cognitive performance compared to the control group.”
Directional hypotheses are formulated based on existing theory, prior research, or logical reasoning, and they guide the researcher’s expectations and analysis. They allow for more targeted predictions and enable researchers to test specific hypotheses using appropriate statistical tests.
The role of directional hypothesis in research
Directional hypotheses also play a significant role in research surveys. Let’s explore their role specifically in the context of survey research:
- Objective-driven surveys : Directional hypotheses help align survey research with specific objectives. By formulating directional hypotheses, researchers can focus on gathering data that directly addresses the predicted relationship or difference between variables of interest.
- Question design and measurement : Directional hypotheses guide the design of survey question types and the selection of appropriate measurement scales. They ensure that the questions are tailored to capture the specific aspects related to the predicted direction, enabling researchers to obtain more targeted and relevant data from survey respondents.
- Data analysis and interpretation : Directional hypotheses assist in data analysis by directing researchers towards appropriate statistical tests and methods. Researchers can analyze the survey data to specifically test the predicted relationship or difference, enhancing the accuracy and reliability of their findings. The results can then be interpreted within the context of the directional hypothesis, providing more meaningful insights.
- Practical implications and decision-making : Directional hypotheses in surveys often have practical implications. When the predicted relationship or difference is confirmed, it informs decision-making processes, program development, or interventions. The survey findings based on directional hypotheses can guide organizations, policymakers, or practitioners in making informed choices to achieve desired outcomes.
- Replication and further research : Directional hypotheses in survey research contribute to the replication and extension of studies. Researchers can replicate the survey with different populations or contexts to assess the generalizability of the predicted relationships. Furthermore, if the directional hypothesis is supported, it encourages further research to explore underlying mechanisms or boundary conditions.
By incorporating directional hypotheses in survey research, researchers can align their objectives, design effective surveys, conduct focused data analysis, and derive practical insights. They provide a framework for organizing survey research and contribute to the accumulation of knowledge in the field.
Examples of research questions for directional hypothesis
Here are some examples of research questions that lend themselves to directional hypotheses:
- Does increased daily exercise lead to a decrease in body weight among sedentary adults?
- Is there a positive relationship between study hours and academic performance among college students?
- Does exposure to violent video games result in an increase in aggressive behavior among adolescents?
- Does the implementation of a mindfulness-based intervention lead to a reduction in stress levels among working professionals?
- Is there a difference in customer satisfaction between Product A and Product B, with Product A expected to have higher satisfaction ratings?
- Does the use of social media influence self-esteem levels, with higher social media usage associated with lower self-esteem?
- Is there a negative relationship between job satisfaction and employee turnover, indicating that lower job satisfaction leads to higher turnover rates?
- Does the administration of a specific medication result in a decrease in symptoms among individuals with a particular medical condition?
- Does increased access to early childhood education lead to improved cognitive development in preschool-aged children?
- Is there a difference in purchase intention between advertisements with celebrity endorsements and advertisements without, with celebrity endorsements expected to have a higher impact?
These research questions generate specific predictions about the direction of the relationship or difference between variables and can be tested using appropriate research methods and statistical analyses.
Definition of non-directional hypothesis
Non-directional hypotheses, also known as two-tailed hypotheses, are statements in research that indicate the presence of a relationship or difference between variables without specifying the direction of the effect. Instead of making predictions about the specific direction of the relationship or difference, non-directional hypotheses simply state that there is an association or distinction between the variables of interest.
Non-directional hypotheses are often used when there is no prior theoretical basis or clear expectation about the direction of the relationship. They leave the possibility open for either a positive or negative relationship, or for both groups to differ in some way without specifying which group will perform better or worse.
Advantages and utility of non-directional hypothesis
Non-directional hypotheses in survey s offer several advantages and utilities, providing flexibility and comprehensive analysis of survey data. Here are some of the key advantages and utilities of using non-directional hypotheses in surveys:
- Exploration of Relationships : Non-directional hypotheses allow researchers to explore and examine relationships between variables without assuming a specific direction. This is particularly useful in surveys where the relationship between variables may not be well-known or there may be conflicting evidence regarding the direction of the effect.
- Flexibility in Question Design : With non-directional hypotheses, survey questions can be designed to measure the relationship between variables without being biased towards a particular outcome. This flexibility allows researchers to collect data and analyze the results more objectively.
- Open to Unexpected Findings : Non-directional hypotheses enable researchers to be open to unexpected or surprising findings in survey data. By not committing to a specific direction of the effect, researchers can identify and explore relationships that may not have been initially anticipated, leading to new insights and discoveries.
- Comprehensive Analysis : Non-directional hypotheses promote comprehensive analysis of survey data by considering the possibility of an effect in either direction. Researchers can assess the magnitude and significance of relationships without limiting their analysis to only one possible outcome.
- S tatistical Validity : Non-directional hypotheses in surveys allow for the use of two-tailed statistical tests, which provide a more conservative and robust assessment of significance. Two-tailed tests consider both positive and negative deviations from the null hypothesis, ensuring accurate and reliable statistical analysis of survey data.
- Exploratory Research : Non-directional hypotheses are particularly useful in exploratory research, where the goal is to gather initial insights and generate hypotheses. Surveys with non-directional hypotheses can help researchers explore various relationships and identify patterns that can guide further research or hypothesis development.
It is worth noting that the choice between directional and non-directional hypotheses in surveys depends on the research objectives, existing knowledge, and the specific variables being investigated. Researchers should carefully consider the advantages and limitations of each approach and select the one that aligns best with their research goals and survey design.
- Share with others
- Twitter Twitter Icon
- LinkedIn LinkedIn Icon
Related posts
How to implement nps surveys: a step-by-step guide, 15 best website survey questions to ask your visitors, how to write a good survey introduction, 7 best ai survey generators, multiple choice questions: types, examples & samples, how to make a gdpr compliant survey, get answers today.
- No credit card required
- No time limit on Free plan
You can modify this template in every possible way.
All templates work great on every device.

An official website of the United States government
The .gov means it’s official. Federal government websites often end in .gov or .mil. Before sharing sensitive information, make sure you’re on a federal government site.
The site is secure. The https:// ensures that you are connecting to the official website and that any information you provide is encrypted and transmitted securely.
- Publications
- Account settings
Preview improvements coming to the PMC website in October 2024. Learn More or Try it out now .
- Advanced Search
- Journal List
- J Korean Med Sci
- v.37(16); 2022 Apr 25

A Practical Guide to Writing Quantitative and Qualitative Research Questions and Hypotheses in Scholarly Articles
Edward barroga.
1 Department of General Education, Graduate School of Nursing Science, St. Luke’s International University, Tokyo, Japan.
Glafera Janet Matanguihan
2 Department of Biological Sciences, Messiah University, Mechanicsburg, PA, USA.
The development of research questions and the subsequent hypotheses are prerequisites to defining the main research purpose and specific objectives of a study. Consequently, these objectives determine the study design and research outcome. The development of research questions is a process based on knowledge of current trends, cutting-edge studies, and technological advances in the research field. Excellent research questions are focused and require a comprehensive literature search and in-depth understanding of the problem being investigated. Initially, research questions may be written as descriptive questions which could be developed into inferential questions. These questions must be specific and concise to provide a clear foundation for developing hypotheses. Hypotheses are more formal predictions about the research outcomes. These specify the possible results that may or may not be expected regarding the relationship between groups. Thus, research questions and hypotheses clarify the main purpose and specific objectives of the study, which in turn dictate the design of the study, its direction, and outcome. Studies developed from good research questions and hypotheses will have trustworthy outcomes with wide-ranging social and health implications.
INTRODUCTION
Scientific research is usually initiated by posing evidenced-based research questions which are then explicitly restated as hypotheses. 1 , 2 The hypotheses provide directions to guide the study, solutions, explanations, and expected results. 3 , 4 Both research questions and hypotheses are essentially formulated based on conventional theories and real-world processes, which allow the inception of novel studies and the ethical testing of ideas. 5 , 6
It is crucial to have knowledge of both quantitative and qualitative research 2 as both types of research involve writing research questions and hypotheses. 7 However, these crucial elements of research are sometimes overlooked; if not overlooked, then framed without the forethought and meticulous attention it needs. Planning and careful consideration are needed when developing quantitative or qualitative research, particularly when conceptualizing research questions and hypotheses. 4
There is a continuing need to support researchers in the creation of innovative research questions and hypotheses, as well as for journal articles that carefully review these elements. 1 When research questions and hypotheses are not carefully thought of, unethical studies and poor outcomes usually ensue. Carefully formulated research questions and hypotheses define well-founded objectives, which in turn determine the appropriate design, course, and outcome of the study. This article then aims to discuss in detail the various aspects of crafting research questions and hypotheses, with the goal of guiding researchers as they develop their own. Examples from the authors and peer-reviewed scientific articles in the healthcare field are provided to illustrate key points.
DEFINITIONS AND RELATIONSHIP OF RESEARCH QUESTIONS AND HYPOTHESES
A research question is what a study aims to answer after data analysis and interpretation. The answer is written in length in the discussion section of the paper. Thus, the research question gives a preview of the different parts and variables of the study meant to address the problem posed in the research question. 1 An excellent research question clarifies the research writing while facilitating understanding of the research topic, objective, scope, and limitations of the study. 5
On the other hand, a research hypothesis is an educated statement of an expected outcome. This statement is based on background research and current knowledge. 8 , 9 The research hypothesis makes a specific prediction about a new phenomenon 10 or a formal statement on the expected relationship between an independent variable and a dependent variable. 3 , 11 It provides a tentative answer to the research question to be tested or explored. 4
Hypotheses employ reasoning to predict a theory-based outcome. 10 These can also be developed from theories by focusing on components of theories that have not yet been observed. 10 The validity of hypotheses is often based on the testability of the prediction made in a reproducible experiment. 8
Conversely, hypotheses can also be rephrased as research questions. Several hypotheses based on existing theories and knowledge may be needed to answer a research question. Developing ethical research questions and hypotheses creates a research design that has logical relationships among variables. These relationships serve as a solid foundation for the conduct of the study. 4 , 11 Haphazardly constructed research questions can result in poorly formulated hypotheses and improper study designs, leading to unreliable results. Thus, the formulations of relevant research questions and verifiable hypotheses are crucial when beginning research. 12
CHARACTERISTICS OF GOOD RESEARCH QUESTIONS AND HYPOTHESES
Excellent research questions are specific and focused. These integrate collective data and observations to confirm or refute the subsequent hypotheses. Well-constructed hypotheses are based on previous reports and verify the research context. These are realistic, in-depth, sufficiently complex, and reproducible. More importantly, these hypotheses can be addressed and tested. 13
There are several characteristics of well-developed hypotheses. Good hypotheses are 1) empirically testable 7 , 10 , 11 , 13 ; 2) backed by preliminary evidence 9 ; 3) testable by ethical research 7 , 9 ; 4) based on original ideas 9 ; 5) have evidenced-based logical reasoning 10 ; and 6) can be predicted. 11 Good hypotheses can infer ethical and positive implications, indicating the presence of a relationship or effect relevant to the research theme. 7 , 11 These are initially developed from a general theory and branch into specific hypotheses by deductive reasoning. In the absence of a theory to base the hypotheses, inductive reasoning based on specific observations or findings form more general hypotheses. 10
TYPES OF RESEARCH QUESTIONS AND HYPOTHESES
Research questions and hypotheses are developed according to the type of research, which can be broadly classified into quantitative and qualitative research. We provide a summary of the types of research questions and hypotheses under quantitative and qualitative research categories in Table 1 .
Research questions in quantitative research
In quantitative research, research questions inquire about the relationships among variables being investigated and are usually framed at the start of the study. These are precise and typically linked to the subject population, dependent and independent variables, and research design. 1 Research questions may also attempt to describe the behavior of a population in relation to one or more variables, or describe the characteristics of variables to be measured ( descriptive research questions ). 1 , 5 , 14 These questions may also aim to discover differences between groups within the context of an outcome variable ( comparative research questions ), 1 , 5 , 14 or elucidate trends and interactions among variables ( relationship research questions ). 1 , 5 We provide examples of descriptive, comparative, and relationship research questions in quantitative research in Table 2 .
Hypotheses in quantitative research
In quantitative research, hypotheses predict the expected relationships among variables. 15 Relationships among variables that can be predicted include 1) between a single dependent variable and a single independent variable ( simple hypothesis ) or 2) between two or more independent and dependent variables ( complex hypothesis ). 4 , 11 Hypotheses may also specify the expected direction to be followed and imply an intellectual commitment to a particular outcome ( directional hypothesis ) 4 . On the other hand, hypotheses may not predict the exact direction and are used in the absence of a theory, or when findings contradict previous studies ( non-directional hypothesis ). 4 In addition, hypotheses can 1) define interdependency between variables ( associative hypothesis ), 4 2) propose an effect on the dependent variable from manipulation of the independent variable ( causal hypothesis ), 4 3) state a negative relationship between two variables ( null hypothesis ), 4 , 11 , 15 4) replace the working hypothesis if rejected ( alternative hypothesis ), 15 explain the relationship of phenomena to possibly generate a theory ( working hypothesis ), 11 5) involve quantifiable variables that can be tested statistically ( statistical hypothesis ), 11 6) or express a relationship whose interlinks can be verified logically ( logical hypothesis ). 11 We provide examples of simple, complex, directional, non-directional, associative, causal, null, alternative, working, statistical, and logical hypotheses in quantitative research, as well as the definition of quantitative hypothesis-testing research in Table 3 .
Research questions in qualitative research
Unlike research questions in quantitative research, research questions in qualitative research are usually continuously reviewed and reformulated. The central question and associated subquestions are stated more than the hypotheses. 15 The central question broadly explores a complex set of factors surrounding the central phenomenon, aiming to present the varied perspectives of participants. 15
There are varied goals for which qualitative research questions are developed. These questions can function in several ways, such as to 1) identify and describe existing conditions ( contextual research question s); 2) describe a phenomenon ( descriptive research questions ); 3) assess the effectiveness of existing methods, protocols, theories, or procedures ( evaluation research questions ); 4) examine a phenomenon or analyze the reasons or relationships between subjects or phenomena ( explanatory research questions ); or 5) focus on unknown aspects of a particular topic ( exploratory research questions ). 5 In addition, some qualitative research questions provide new ideas for the development of theories and actions ( generative research questions ) or advance specific ideologies of a position ( ideological research questions ). 1 Other qualitative research questions may build on a body of existing literature and become working guidelines ( ethnographic research questions ). Research questions may also be broadly stated without specific reference to the existing literature or a typology of questions ( phenomenological research questions ), may be directed towards generating a theory of some process ( grounded theory questions ), or may address a description of the case and the emerging themes ( qualitative case study questions ). 15 We provide examples of contextual, descriptive, evaluation, explanatory, exploratory, generative, ideological, ethnographic, phenomenological, grounded theory, and qualitative case study research questions in qualitative research in Table 4 , and the definition of qualitative hypothesis-generating research in Table 5 .
Qualitative studies usually pose at least one central research question and several subquestions starting with How or What . These research questions use exploratory verbs such as explore or describe . These also focus on one central phenomenon of interest, and may mention the participants and research site. 15
Hypotheses in qualitative research
Hypotheses in qualitative research are stated in the form of a clear statement concerning the problem to be investigated. Unlike in quantitative research where hypotheses are usually developed to be tested, qualitative research can lead to both hypothesis-testing and hypothesis-generating outcomes. 2 When studies require both quantitative and qualitative research questions, this suggests an integrative process between both research methods wherein a single mixed-methods research question can be developed. 1
FRAMEWORKS FOR DEVELOPING RESEARCH QUESTIONS AND HYPOTHESES
Research questions followed by hypotheses should be developed before the start of the study. 1 , 12 , 14 It is crucial to develop feasible research questions on a topic that is interesting to both the researcher and the scientific community. This can be achieved by a meticulous review of previous and current studies to establish a novel topic. Specific areas are subsequently focused on to generate ethical research questions. The relevance of the research questions is evaluated in terms of clarity of the resulting data, specificity of the methodology, objectivity of the outcome, depth of the research, and impact of the study. 1 , 5 These aspects constitute the FINER criteria (i.e., Feasible, Interesting, Novel, Ethical, and Relevant). 1 Clarity and effectiveness are achieved if research questions meet the FINER criteria. In addition to the FINER criteria, Ratan et al. described focus, complexity, novelty, feasibility, and measurability for evaluating the effectiveness of research questions. 14
The PICOT and PEO frameworks are also used when developing research questions. 1 The following elements are addressed in these frameworks, PICOT: P-population/patients/problem, I-intervention or indicator being studied, C-comparison group, O-outcome of interest, and T-timeframe of the study; PEO: P-population being studied, E-exposure to preexisting conditions, and O-outcome of interest. 1 Research questions are also considered good if these meet the “FINERMAPS” framework: Feasible, Interesting, Novel, Ethical, Relevant, Manageable, Appropriate, Potential value/publishable, and Systematic. 14
As we indicated earlier, research questions and hypotheses that are not carefully formulated result in unethical studies or poor outcomes. To illustrate this, we provide some examples of ambiguous research question and hypotheses that result in unclear and weak research objectives in quantitative research ( Table 6 ) 16 and qualitative research ( Table 7 ) 17 , and how to transform these ambiguous research question(s) and hypothesis(es) into clear and good statements.
a These statements were composed for comparison and illustrative purposes only.
b These statements are direct quotes from Higashihara and Horiuchi. 16
a This statement is a direct quote from Shimoda et al. 17
The other statements were composed for comparison and illustrative purposes only.
CONSTRUCTING RESEARCH QUESTIONS AND HYPOTHESES
To construct effective research questions and hypotheses, it is very important to 1) clarify the background and 2) identify the research problem at the outset of the research, within a specific timeframe. 9 Then, 3) review or conduct preliminary research to collect all available knowledge about the possible research questions by studying theories and previous studies. 18 Afterwards, 4) construct research questions to investigate the research problem. Identify variables to be accessed from the research questions 4 and make operational definitions of constructs from the research problem and questions. Thereafter, 5) construct specific deductive or inductive predictions in the form of hypotheses. 4 Finally, 6) state the study aims . This general flow for constructing effective research questions and hypotheses prior to conducting research is shown in Fig. 1 .

Research questions are used more frequently in qualitative research than objectives or hypotheses. 3 These questions seek to discover, understand, explore or describe experiences by asking “What” or “How.” The questions are open-ended to elicit a description rather than to relate variables or compare groups. The questions are continually reviewed, reformulated, and changed during the qualitative study. 3 Research questions are also used more frequently in survey projects than hypotheses in experiments in quantitative research to compare variables and their relationships.
Hypotheses are constructed based on the variables identified and as an if-then statement, following the template, ‘If a specific action is taken, then a certain outcome is expected.’ At this stage, some ideas regarding expectations from the research to be conducted must be drawn. 18 Then, the variables to be manipulated (independent) and influenced (dependent) are defined. 4 Thereafter, the hypothesis is stated and refined, and reproducible data tailored to the hypothesis are identified, collected, and analyzed. 4 The hypotheses must be testable and specific, 18 and should describe the variables and their relationships, the specific group being studied, and the predicted research outcome. 18 Hypotheses construction involves a testable proposition to be deduced from theory, and independent and dependent variables to be separated and measured separately. 3 Therefore, good hypotheses must be based on good research questions constructed at the start of a study or trial. 12
In summary, research questions are constructed after establishing the background of the study. Hypotheses are then developed based on the research questions. Thus, it is crucial to have excellent research questions to generate superior hypotheses. In turn, these would determine the research objectives and the design of the study, and ultimately, the outcome of the research. 12 Algorithms for building research questions and hypotheses are shown in Fig. 2 for quantitative research and in Fig. 3 for qualitative research.

EXAMPLES OF RESEARCH QUESTIONS FROM PUBLISHED ARTICLES
- EXAMPLE 1. Descriptive research question (quantitative research)
- - Presents research variables to be assessed (distinct phenotypes and subphenotypes)
- “BACKGROUND: Since COVID-19 was identified, its clinical and biological heterogeneity has been recognized. Identifying COVID-19 phenotypes might help guide basic, clinical, and translational research efforts.
- RESEARCH QUESTION: Does the clinical spectrum of patients with COVID-19 contain distinct phenotypes and subphenotypes? ” 19
- EXAMPLE 2. Relationship research question (quantitative research)
- - Shows interactions between dependent variable (static postural control) and independent variable (peripheral visual field loss)
- “Background: Integration of visual, vestibular, and proprioceptive sensations contributes to postural control. People with peripheral visual field loss have serious postural instability. However, the directional specificity of postural stability and sensory reweighting caused by gradual peripheral visual field loss remain unclear.
- Research question: What are the effects of peripheral visual field loss on static postural control ?” 20
- EXAMPLE 3. Comparative research question (quantitative research)
- - Clarifies the difference among groups with an outcome variable (patients enrolled in COMPERA with moderate PH or severe PH in COPD) and another group without the outcome variable (patients with idiopathic pulmonary arterial hypertension (IPAH))
- “BACKGROUND: Pulmonary hypertension (PH) in COPD is a poorly investigated clinical condition.
- RESEARCH QUESTION: Which factors determine the outcome of PH in COPD?
- STUDY DESIGN AND METHODS: We analyzed the characteristics and outcome of patients enrolled in the Comparative, Prospective Registry of Newly Initiated Therapies for Pulmonary Hypertension (COMPERA) with moderate or severe PH in COPD as defined during the 6th PH World Symposium who received medical therapy for PH and compared them with patients with idiopathic pulmonary arterial hypertension (IPAH) .” 21
- EXAMPLE 4. Exploratory research question (qualitative research)
- - Explores areas that have not been fully investigated (perspectives of families and children who receive care in clinic-based child obesity treatment) to have a deeper understanding of the research problem
- “Problem: Interventions for children with obesity lead to only modest improvements in BMI and long-term outcomes, and data are limited on the perspectives of families of children with obesity in clinic-based treatment. This scoping review seeks to answer the question: What is known about the perspectives of families and children who receive care in clinic-based child obesity treatment? This review aims to explore the scope of perspectives reported by families of children with obesity who have received individualized outpatient clinic-based obesity treatment.” 22
- EXAMPLE 5. Relationship research question (quantitative research)
- - Defines interactions between dependent variable (use of ankle strategies) and independent variable (changes in muscle tone)
- “Background: To maintain an upright standing posture against external disturbances, the human body mainly employs two types of postural control strategies: “ankle strategy” and “hip strategy.” While it has been reported that the magnitude of the disturbance alters the use of postural control strategies, it has not been elucidated how the level of muscle tone, one of the crucial parameters of bodily function, determines the use of each strategy. We have previously confirmed using forward dynamics simulations of human musculoskeletal models that an increased muscle tone promotes the use of ankle strategies. The objective of the present study was to experimentally evaluate a hypothesis: an increased muscle tone promotes the use of ankle strategies. Research question: Do changes in the muscle tone affect the use of ankle strategies ?” 23
EXAMPLES OF HYPOTHESES IN PUBLISHED ARTICLES
- EXAMPLE 1. Working hypothesis (quantitative research)
- - A hypothesis that is initially accepted for further research to produce a feasible theory
- “As fever may have benefit in shortening the duration of viral illness, it is plausible to hypothesize that the antipyretic efficacy of ibuprofen may be hindering the benefits of a fever response when taken during the early stages of COVID-19 illness .” 24
- “In conclusion, it is plausible to hypothesize that the antipyretic efficacy of ibuprofen may be hindering the benefits of a fever response . The difference in perceived safety of these agents in COVID-19 illness could be related to the more potent efficacy to reduce fever with ibuprofen compared to acetaminophen. Compelling data on the benefit of fever warrant further research and review to determine when to treat or withhold ibuprofen for early stage fever for COVID-19 and other related viral illnesses .” 24
- EXAMPLE 2. Exploratory hypothesis (qualitative research)
- - Explores particular areas deeper to clarify subjective experience and develop a formal hypothesis potentially testable in a future quantitative approach
- “We hypothesized that when thinking about a past experience of help-seeking, a self distancing prompt would cause increased help-seeking intentions and more favorable help-seeking outcome expectations .” 25
- “Conclusion
- Although a priori hypotheses were not supported, further research is warranted as results indicate the potential for using self-distancing approaches to increasing help-seeking among some people with depressive symptomatology.” 25
- EXAMPLE 3. Hypothesis-generating research to establish a framework for hypothesis testing (qualitative research)
- “We hypothesize that compassionate care is beneficial for patients (better outcomes), healthcare systems and payers (lower costs), and healthcare providers (lower burnout). ” 26
- Compassionomics is the branch of knowledge and scientific study of the effects of compassionate healthcare. Our main hypotheses are that compassionate healthcare is beneficial for (1) patients, by improving clinical outcomes, (2) healthcare systems and payers, by supporting financial sustainability, and (3) HCPs, by lowering burnout and promoting resilience and well-being. The purpose of this paper is to establish a scientific framework for testing the hypotheses above . If these hypotheses are confirmed through rigorous research, compassionomics will belong in the science of evidence-based medicine, with major implications for all healthcare domains.” 26
- EXAMPLE 4. Statistical hypothesis (quantitative research)
- - An assumption is made about the relationship among several population characteristics ( gender differences in sociodemographic and clinical characteristics of adults with ADHD ). Validity is tested by statistical experiment or analysis ( chi-square test, Students t-test, and logistic regression analysis)
- “Our research investigated gender differences in sociodemographic and clinical characteristics of adults with ADHD in a Japanese clinical sample. Due to unique Japanese cultural ideals and expectations of women's behavior that are in opposition to ADHD symptoms, we hypothesized that women with ADHD experience more difficulties and present more dysfunctions than men . We tested the following hypotheses: first, women with ADHD have more comorbidities than men with ADHD; second, women with ADHD experience more social hardships than men, such as having less full-time employment and being more likely to be divorced.” 27
- “Statistical Analysis
- ( text omitted ) Between-gender comparisons were made using the chi-squared test for categorical variables and Students t-test for continuous variables…( text omitted ). A logistic regression analysis was performed for employment status, marital status, and comorbidity to evaluate the independent effects of gender on these dependent variables.” 27
EXAMPLES OF HYPOTHESIS AS WRITTEN IN PUBLISHED ARTICLES IN RELATION TO OTHER PARTS
- EXAMPLE 1. Background, hypotheses, and aims are provided
- “Pregnant women need skilled care during pregnancy and childbirth, but that skilled care is often delayed in some countries …( text omitted ). The focused antenatal care (FANC) model of WHO recommends that nurses provide information or counseling to all pregnant women …( text omitted ). Job aids are visual support materials that provide the right kind of information using graphics and words in a simple and yet effective manner. When nurses are not highly trained or have many work details to attend to, these job aids can serve as a content reminder for the nurses and can be used for educating their patients (Jennings, Yebadokpo, Affo, & Agbogbe, 2010) ( text omitted ). Importantly, additional evidence is needed to confirm how job aids can further improve the quality of ANC counseling by health workers in maternal care …( text omitted )” 28
- “ This has led us to hypothesize that the quality of ANC counseling would be better if supported by job aids. Consequently, a better quality of ANC counseling is expected to produce higher levels of awareness concerning the danger signs of pregnancy and a more favorable impression of the caring behavior of nurses .” 28
- “This study aimed to examine the differences in the responses of pregnant women to a job aid-supported intervention during ANC visit in terms of 1) their understanding of the danger signs of pregnancy and 2) their impression of the caring behaviors of nurses to pregnant women in rural Tanzania.” 28
- EXAMPLE 2. Background, hypotheses, and aims are provided
- “We conducted a two-arm randomized controlled trial (RCT) to evaluate and compare changes in salivary cortisol and oxytocin levels of first-time pregnant women between experimental and control groups. The women in the experimental group touched and held an infant for 30 min (experimental intervention protocol), whereas those in the control group watched a DVD movie of an infant (control intervention protocol). The primary outcome was salivary cortisol level and the secondary outcome was salivary oxytocin level.” 29
- “ We hypothesize that at 30 min after touching and holding an infant, the salivary cortisol level will significantly decrease and the salivary oxytocin level will increase in the experimental group compared with the control group .” 29
- EXAMPLE 3. Background, aim, and hypothesis are provided
- “In countries where the maternal mortality ratio remains high, antenatal education to increase Birth Preparedness and Complication Readiness (BPCR) is considered one of the top priorities [1]. BPCR includes birth plans during the antenatal period, such as the birthplace, birth attendant, transportation, health facility for complications, expenses, and birth materials, as well as family coordination to achieve such birth plans. In Tanzania, although increasing, only about half of all pregnant women attend an antenatal clinic more than four times [4]. Moreover, the information provided during antenatal care (ANC) is insufficient. In the resource-poor settings, antenatal group education is a potential approach because of the limited time for individual counseling at antenatal clinics.” 30
- “This study aimed to evaluate an antenatal group education program among pregnant women and their families with respect to birth-preparedness and maternal and infant outcomes in rural villages of Tanzania.” 30
- “ The study hypothesis was if Tanzanian pregnant women and their families received a family-oriented antenatal group education, they would (1) have a higher level of BPCR, (2) attend antenatal clinic four or more times, (3) give birth in a health facility, (4) have less complications of women at birth, and (5) have less complications and deaths of infants than those who did not receive the education .” 30
Research questions and hypotheses are crucial components to any type of research, whether quantitative or qualitative. These questions should be developed at the very beginning of the study. Excellent research questions lead to superior hypotheses, which, like a compass, set the direction of research, and can often determine the successful conduct of the study. Many research studies have floundered because the development of research questions and subsequent hypotheses was not given the thought and meticulous attention needed. The development of research questions and hypotheses is an iterative process based on extensive knowledge of the literature and insightful grasp of the knowledge gap. Focused, concise, and specific research questions provide a strong foundation for constructing hypotheses which serve as formal predictions about the research outcomes. Research questions and hypotheses are crucial elements of research that should not be overlooked. They should be carefully thought of and constructed when planning research. This avoids unethical studies and poor outcomes by defining well-founded objectives that determine the design, course, and outcome of the study.
Disclosure: The authors have no potential conflicts of interest to disclose.
Author Contributions:
- Conceptualization: Barroga E, Matanguihan GJ.
- Methodology: Barroga E, Matanguihan GJ.
- Writing - original draft: Barroga E, Matanguihan GJ.
- Writing - review & editing: Barroga E, Matanguihan GJ.
User Preferences
Content preview.
Arcu felis bibendum ut tristique et egestas quis:
- Ut enim ad minim veniam, quis nostrud exercitation ullamco laboris
- Duis aute irure dolor in reprehenderit in voluptate
- Excepteur sint occaecat cupidatat non proident
Keyboard Shortcuts
5.2 - writing hypotheses.
The first step in conducting a hypothesis test is to write the hypothesis statements that are going to be tested. For each test you will have a null hypothesis (\(H_0\)) and an alternative hypothesis (\(H_a\)).
When writing hypotheses there are three things that we need to know: (1) the parameter that we are testing (2) the direction of the test (non-directional, right-tailed or left-tailed), and (3) the value of the hypothesized parameter.
- At this point we can write hypotheses for a single mean (\(\mu\)), paired means(\(\mu_d\)), a single proportion (\(p\)), the difference between two independent means (\(\mu_1-\mu_2\)), the difference between two proportions (\(p_1-p_2\)), a simple linear regression slope (\(\beta\)), and a correlation (\(\rho\)).
- The research question will give us the information necessary to determine if the test is two-tailed (e.g., "different from," "not equal to"), right-tailed (e.g., "greater than," "more than"), or left-tailed (e.g., "less than," "fewer than").
- The research question will also give us the hypothesized parameter value. This is the number that goes in the hypothesis statements (i.e., \(\mu_0\) and \(p_0\)). For the difference between two groups, regression, and correlation, this value is typically 0.
Hypotheses are always written in terms of population parameters (e.g., \(p\) and \(\mu\)). The tables below display all of the possible hypotheses for the parameters that we have learned thus far. Note that the null hypothesis always includes the equality (i.e., =).

Directional Hypothesis
Definition:
A directional hypothesis is a specific type of hypothesis statement in which the researcher predicts the direction or effect of the relationship between two variables.
Key Features
1. Predicts direction:
Unlike a non-directional hypothesis, which simply states that there is a relationship between two variables, a directional hypothesis specifies the expected direction of the relationship.
2. Involves one-tailed test:
Directional hypotheses typically require a one-tailed statistical test, as they are concerned with whether the relationship is positive or negative, rather than simply whether a relationship exists.
3. Example:
An example of a directional hypothesis would be: “Increasing levels of exercise will result in greater weight loss.”
4. Researcher’s prior belief:
A directional hypothesis is often formed based on the researcher’s prior knowledge, theoretical understanding, or previous empirical evidence relating to the variables under investigation.
5. Confirmatory nature:
Directional hypotheses are considered confirmatory, as they provide a specific prediction that can be tested statistically, allowing researchers to either support or reject the hypothesis.
6. Advantages and disadvantages:
Directional hypotheses help focus the research by explicitly stating the expected relationship, but they can also limit exploration of alternative explanations or unexpected findings.
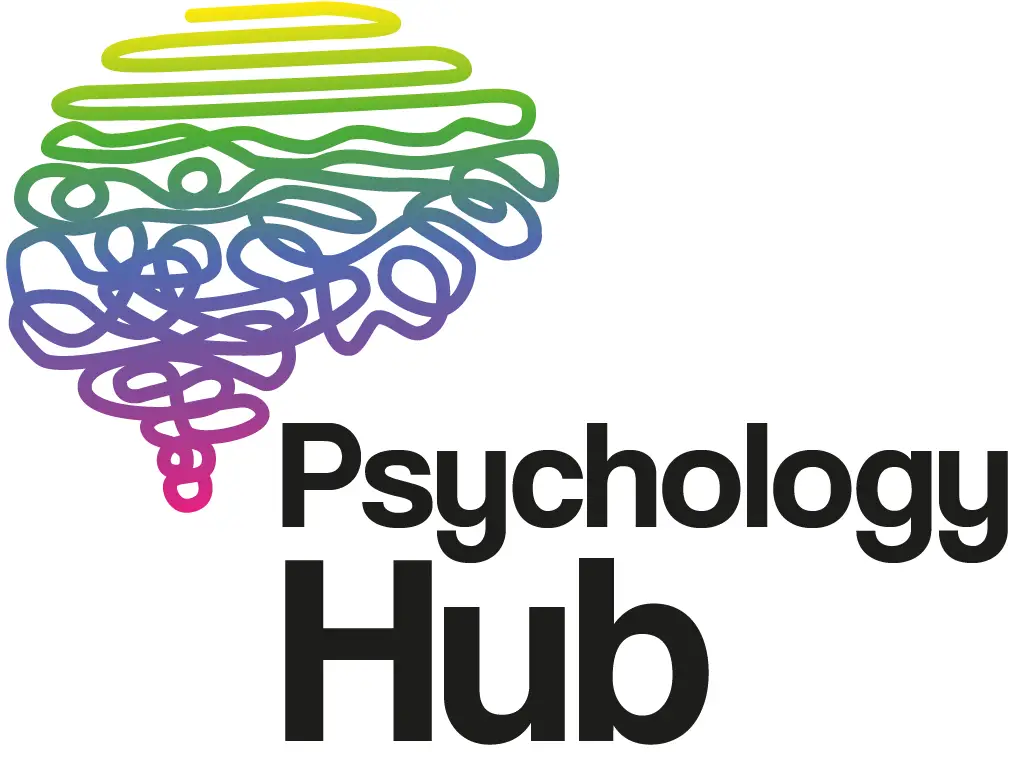
Aims And Hypotheses, Directional And Non-Directional
March 7, 2021 - paper 2 psychology in context | research methods.
- Back to Paper 2 - Research Methods
In Psychology, hypotheses are predictions made by the researcher about the outcome of a study. The research can chose to make a specific prediction about what they feel will happen in their research (a directional hypothesis) or they can make a ‘general,’ ‘less specific’ prediction about the outcome of their research (a non-directional hypothesis). The type of prediction that a researcher makes is usually dependent on whether or not any previous research has also investigated their research aim.
Variables Recap:
The independent variable (IV) is the variable that psychologists manipulate/change to see if changing this variable has an effect on the depen dent variable (DV).
The dependent variable (DV) is the variable that the psychologists measures (to see if the IV has had an effect).
It is important that the only variable that is changed in research is the independent variable (IV), all other variables have to be kept constant across the control condition and the experimental conditions. Only then will researchers be able to observe the true effects of just the independent variable (IV) on the dependent variable (DV).
Research/Experimental Aim(S):

An aim is a clear and precise statement of the purpose of the study. It is a statement of why a research study is taking place. This should include what is being studied and what the study is trying to achieve. (e.g. “This study aims to investigate the effects of alcohol on reaction times”.
It is important that aims created in research are realistic and ethical.
Hypotheses:
This is a testable statement that predicts what the researcher expects to happen in their research. The research study itself is therefore a means of testing whether or not the hypothesis is supported by the findings. If the findings do support the hypothesis then the hypothesis can be retained (i.e., accepted), but if not, then it must be rejected.
Three Different Hypotheses:

We're not around right now. But you can send us an email and we'll get back to you, asap.
Start typing and press Enter to search
Cookie Policy - Terms and Conditions - Privacy Policy

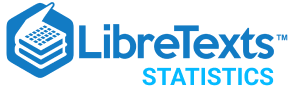
- school Campus Bookshelves
- menu_book Bookshelves
- perm_media Learning Objects
- login Login
- how_to_reg Request Instructor Account
- hub Instructor Commons
Margin Size
- Download Page (PDF)
- Download Full Book (PDF)
- Periodic Table
- Physics Constants
- Scientific Calculator
- Reference & Cite
- Tools expand_more
- Readability
selected template will load here
This action is not available.
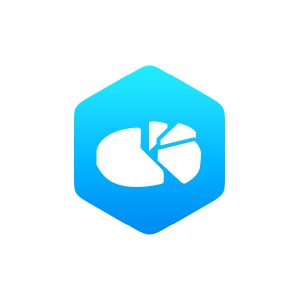
7.5.1: Critical Values
- Last updated
- Save as PDF
- Page ID 22071
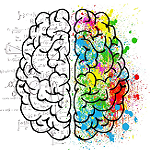
- Michelle Oja
- Taft College
\( \newcommand{\vecs}[1]{\overset { \scriptstyle \rightharpoonup} {\mathbf{#1}} } \)
\( \newcommand{\vecd}[1]{\overset{-\!-\!\rightharpoonup}{\vphantom{a}\smash {#1}}} \)
\( \newcommand{\id}{\mathrm{id}}\) \( \newcommand{\Span}{\mathrm{span}}\)
( \newcommand{\kernel}{\mathrm{null}\,}\) \( \newcommand{\range}{\mathrm{range}\,}\)
\( \newcommand{\RealPart}{\mathrm{Re}}\) \( \newcommand{\ImaginaryPart}{\mathrm{Im}}\)
\( \newcommand{\Argument}{\mathrm{Arg}}\) \( \newcommand{\norm}[1]{\| #1 \|}\)
\( \newcommand{\inner}[2]{\langle #1, #2 \rangle}\)
\( \newcommand{\Span}{\mathrm{span}}\)
\( \newcommand{\id}{\mathrm{id}}\)
\( \newcommand{\kernel}{\mathrm{null}\,}\)
\( \newcommand{\range}{\mathrm{range}\,}\)
\( \newcommand{\RealPart}{\mathrm{Re}}\)
\( \newcommand{\ImaginaryPart}{\mathrm{Im}}\)
\( \newcommand{\Argument}{\mathrm{Arg}}\)
\( \newcommand{\norm}[1]{\| #1 \|}\)
\( \newcommand{\Span}{\mathrm{span}}\) \( \newcommand{\AA}{\unicode[.8,0]{x212B}}\)
\( \newcommand{\vectorA}[1]{\vec{#1}} % arrow\)
\( \newcommand{\vectorAt}[1]{\vec{\text{#1}}} % arrow\)
\( \newcommand{\vectorB}[1]{\overset { \scriptstyle \rightharpoonup} {\mathbf{#1}} } \)
\( \newcommand{\vectorC}[1]{\textbf{#1}} \)
\( \newcommand{\vectorD}[1]{\overrightarrow{#1}} \)
\( \newcommand{\vectorDt}[1]{\overrightarrow{\text{#1}}} \)
\( \newcommand{\vectE}[1]{\overset{-\!-\!\rightharpoonup}{\vphantom{a}\smash{\mathbf {#1}}}} \)
Okay, this whole chapter is full of complex theoretical ideas. Critical values and null hypothesis significance testing is a TOUGH concept to get. Here's another description of critical values, p-values, and significance. Everyone learns differently, so hopefully this slightly different explanation will help understand the prior section.
We understand that we need a research hypothesis that predicts the relationship between two groups on an measured outcome (DV), and that each research hypothesis has a null hypothesis that says that there is no relationship between the two groups on the measured outcome (the means of the DV are similar). If we reject the null hypothesis (which says that the means are similar), we are saying that the means are different; this may or may not be in the direction that we predicted in the research hypothesis so we may or may not support the research hypothesis. If we retain (fail to reject) the null hypothesis, we are saying that the means are similar and then we cannot support the research hypothesis. Got it?
But how do we decide again to retain or reject the null hypothesis? We compare a calculated statistic (there are many, depending on your variables, that we'll cover for the rest of this textbook) to a critical value from a table. The table uses probability (p-values) to tell us what calculated values are so extreme to be absolutely unlikely.
Critical Regions and Critical Values
The critical region of any test corresponds to those values of the test statistic that would lead us to reject null hypothesis (which is why the critical region is also sometimes called the rejection region). How do we find this critical region? Well, let’s consider what we know:
- The test statistic should be very big or very small (extreme) in order to reject the null hypothesis.
- If α=.05 (α is "alpha," and is just another notation for probability; we'll talk about it more in the section on errors), the critical region must cover 5% of a Standard Normal Distribution.
It’s only important to make sure you understand this last point when you are dealing with non-directional hypotheses (which we will only do for Confidence Intervals). The critical region corresponds to those values of the test statistic for which we would reject the null hypothesis, and the Standard Normal Distribution describes the probability that we would obtain a particular value if the null hypothesis (that the means are similar) were actually true. Now, let’s suppose that we chose a critical region that covers 20% of the Standard Normal Distribution, and suppose that the null hypothesis is actually true. What would be the probability of incorrectly rejecting the null (saying that there is a difference between the means when there really isn't a difference)? The answer is 20%. And therefore, we would have built a test that had an α level of 0.2. If we want α=.05, the critical region only covers 5% of the Standard Normal Distribution.
Huh? Let's draw that out. Figure \(\PageIndex{1}\) shows the critical region associated with a non-directional hypothesis test (also called a "two-sided test" because the calculated value might be in either tail of the distribution). Figure \(\PageIndex{1}\) itself shows the sampling distribution of X (the scores we got). The grey bars correspond to those values for which we would retain the null hypothesis. The blue (dark) bars show the critical region: those values for which we would reject the null. In this example, the research hypothesis was non-directional, so the critical region covers both tails of the distribution. To ensure an α level of .05, we need to ensure that each of the two regions encompasses 2.5% of the sampling distribution.
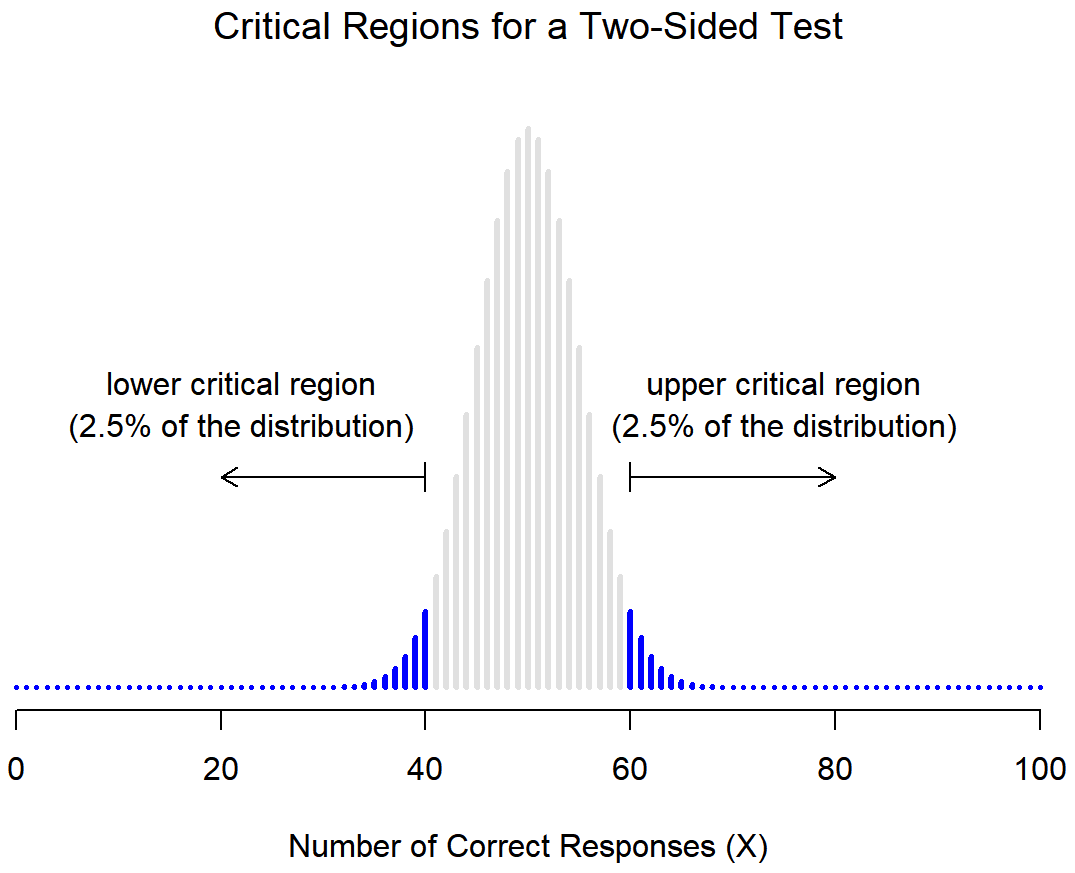
Our critical region consists of the most extreme values , known as the tails of the distribution.
At this point, our hypothesis test is essentially complete: (1) we choose an α level (e.g., α=.05, (2) come up with some test statistic (more on this step later) that does a good job (in some meaningful sense) of comparing the null hypothesis to the research hypothesis, (3) calculate the critical region that produces an appropriate α level, and then (4) calculate the value of the test statistic for the real data and then compare it to the critical values to make our decision. If we reject the null hypothesis, we say that the test has produced a significant result.
A note on statistical “significance”
Like other occult techniques of divination, the statistical method has a private jargon deliberately contrived to obscure its methods from non-practitioners. – Attributed to G. O. Ashley*
A very brief digression is in order at this point, regarding the word “significant”. The concept of statistical significance is actually a very simple one, but has a very unfortunate name. If the data allow us to reject the null hypothesis, we say that “the result is statistically significant ”, which is often shortened to “the result is significant”. This terminology is rather old, and dates back to a time when “significant” just meant something like “indicated”, rather than its modern meaning, which is much closer to “important”. As a result, a lot of modern readers get very confused when they start learning statistics, because they think that a “significant result” must be an important one. It doesn’t mean that at all. All that “statistically significant” means is that the data allowed us to reject a null hypothesis. Whether or not the result is actually important in the real world is a very different question, and depends on all sorts of other things.
Directional and Non-Directional Hypotheses
There’s one more thing to point out about the hypothesis test that we’ve just constructed. In statistical language, this is an example of a non-directional hypothesis, also known as a two-sided test. It’s called this because the alternative hypothesis covers the area on both “sides” of the null hypothesis, and as a consequence the critical region of the test covers both tails of the sampling distribution (2.5% on either side if α=.05), as illustrated earlier in Figure \(\PageIndex{1}\).
However, that’s not the only possibility, and not the situation that we'll be using with our directional research hypotheses (the ones that predict which group will have a higher mean). A directional research hypothesis would only cover the possibility that p>.5, and as a consequence the null hypothesis now becomes p≤.5. When this happens, we have what’s called a one-sided test , and when this happens the critical region only covers one tail of the sampling distribution. This is illustrated in Figure \(\PageIndex{2}\). In this case, we would only reject the null hypothesis for large values of of our test statistic (values that are more extreme but only in one direction). As a consequence, the critical region only covers the upper tail of the sampling distribution; specifically the upper 5% of the distribution. Contrast this to the two-sided version earlier in Figure \(\PageIndex{1}\).
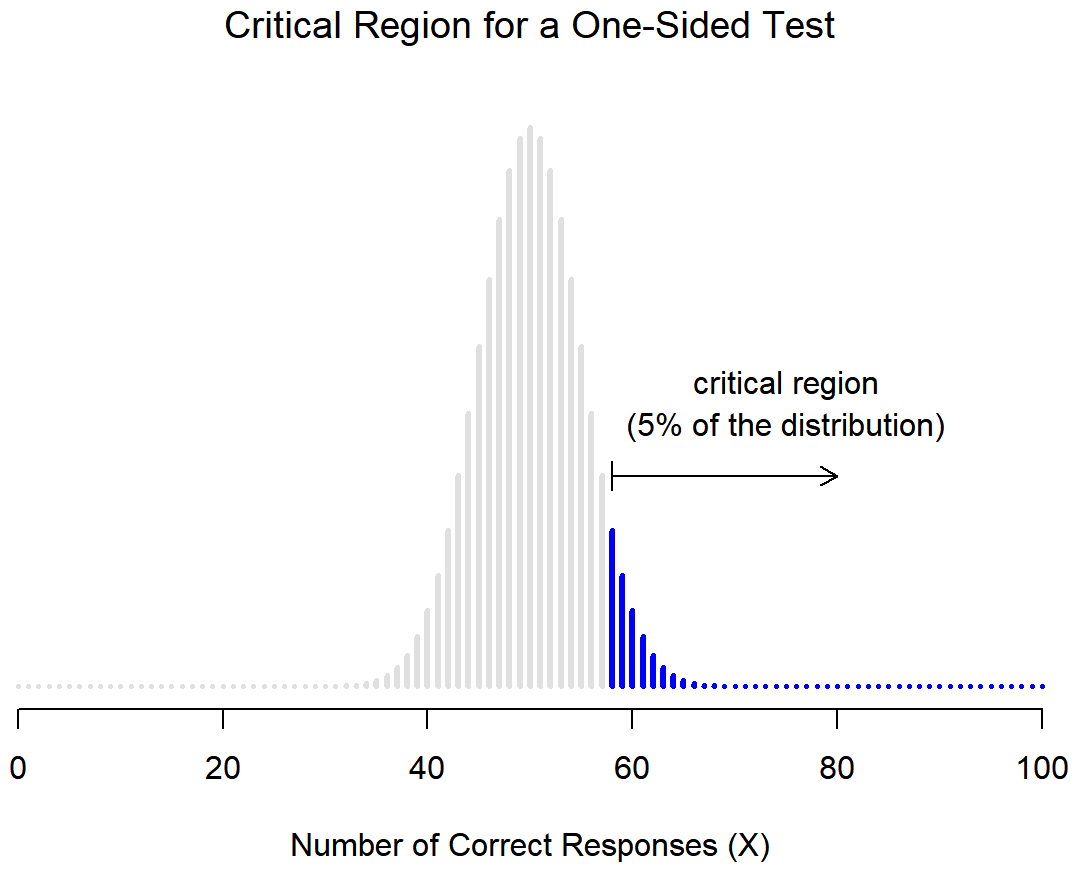
Clear as mud? Let's try one more way to describe how research hypotheses, null hypotheses, p-values, and null hypothesis significance testing work.
*The internet seems fairly convinced that Ashley said this, though I can’t for the life of me find anyone willing to give a source for the claim.
Contributors and Attributions
- Danielle Navarro ( University of New South Wales )
Dr. MO ( Taft College )
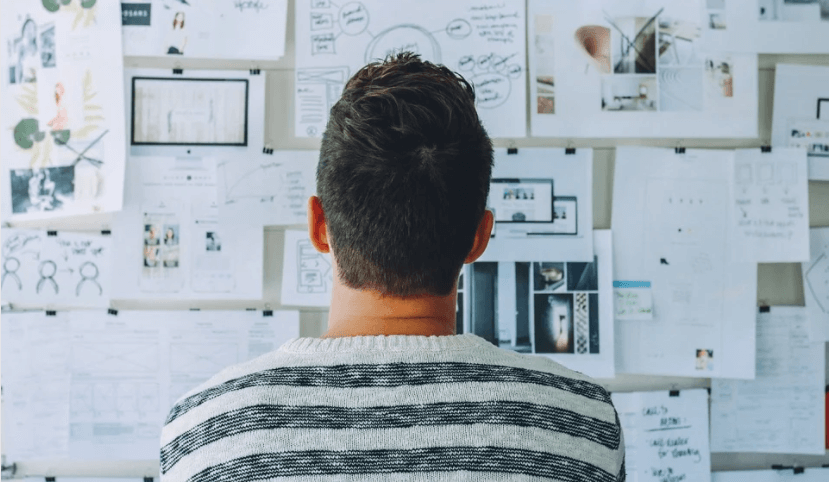
Directional vs Non-Directional Hypothesis – Collect Feedback More Effectively
To conduct a perfect survey, you should know the basics of good research . That’s why in Startquestion we would like to share with you our knowledge about basic terms connected to online surveys and feedback gathering . Knowing the basis you can create surveys and conduct research in more effective ways and thanks to this get meaningful feedback from your customers, employees, and users. That’s enough for the introduction – let’s get to work. This time we will tell you about the hypothesis .
What is a Hypothesis?
A Hypothesis can be described as a theoretical statement built upon some evidence so that it can be tested as if it is true or false. In other words, a hypothesis is a speculation or an idea, based on insufficient evidence that allows it further analysis and experimentation.
The purpose of a hypothetical statement is to work like a prediction based on studied research and to provide some estimated results before it ha happens in a real position. There can be more than one hypothesis statement involved in a research study, where you need to question and explore different aspects of a proposed research topic. Before putting your research into directional vs non-directional hypotheses, let’s have some basic knowledge.
Most often, a hypothesis describes a relation between two or more variables. It includes:
An Independent variable – One that is controlled by the researcher
Dependent Variable – The variable that the researcher observes in association with the Independent variable.
Try one of the best survey tools for free!
Start trial period without any credit card or subscription. Easily conduct your research and gather feedback via link, social media, email, and more.
Create first survey
No credit card required · Cancel any time · GDRP Compilant
How to write an effective Hypothesis?
To write an effective hypothesis follow these essential steps.
- Inquire a Question
The very first step in writing an effective hypothesis is raising a question. Outline the research question very carefully keeping your research purpose in mind. Build it in a precise and targeted way. Here you must be clear about the research question vs hypothesis. A research question is the very beginning point of writing an effective hypothesis.
Do Literature Review
Once you are done with constructing your research question, you can start the literature review. A literature review is a collection of preliminary research studies done on the same or relevant topics. There is a diversified range of literature reviews. The most common ones are academic journals but it is not confined to that. It can be anything including your research, data collection, and observation.
At this point, you can build a conceptual framework. It can be defined as a visual representation of the estimated relationship between two variables subjected to research.
Frame an Answer
After a collection of literature reviews, you can find ways how to answer the question. Expect this stage as a point where you will be able to make a stand upon what you believe might have the exact outcome of your research. You must formulate this answer statement clearly and concisely.
Build a Hypothesis
At this point, you can firmly build your hypothesis. By now, you knew the answer to your question so make a hypothesis that includes:
- Applicable Variables
- Particular Group being Studied (Who/What)
- Probable Outcome of the Experiment
Remember, your hypothesis is a calculated assumption, it has to be constructed as a sentence, not a question. This is where research question vs hypothesis starts making sense.
Refine a Hypothesis
Make necessary amendments to the constructed hypothesis keeping in mind that it has to be targeted and provable. Moreover, you might encounter certain circumstances where you will be studying the difference between one or more groups. It can be correlational research. In such instances, you must have to testify the relationships that you believe you will find in the subject variables and through this research.
Build Null Hypothesis
Certain research studies require some statistical investigation to perform a data collection. Whenever applying any scientific method to construct a hypothesis, you must have adequate knowledge of the Null Hypothesis and an Alternative hypothesis.
Null Hypothesis:
A null Hypothesis denotes that there is no statistical relationship between the subject variables. It is applicable for a single group of variables or two groups of variables. A Null Hypothesis is denoted as an H0. This is the type of hypothesis that the researcher tries to invalidate. Some of the examples of null hypotheses are:
– Hyperactivity is not associated with eating sugar.
– All roses have an equal amount of petals.
– A person’s preference for a dress is not linked to its color.
Alternative Hypothesis:
An alternative hypothesis is a statement that is simply inverse or opposite of the null hypothesis and denoted as H1. Simply saying, it is an alternative statement for the null hypothesis. The same examples will go this way as an alternative hypothesis:
– Hyperactivity is associated with eating sugar.
– All roses do not have an equal amount of petals.
– A person’s preference for a dress is linked to its color.
Start your research right now: use professional survey templates
- Brand Awareness Survey
- Survey for the thesis
- Website Evaluation Survey
See more templates
Types of Hypothesis
Apart from null and alternative hypotheses, research hypotheses can be categorized into different types. Let’s have a look at them:
Simple Hypothesis:
This type of hypothesis is used to state a relationship between a particular independent variable and only a dependent variable.
Complex Hypothesis:
A statement that states the relationship between two or more independent variables and two or more dependent variables, is termed a complex hypothesis.
Associative and Causal Hypothesis:
This type of hypothesis involves predicting that there is a point of interdependency between two variables. It says that any kind of change in one variable will cause a change in the other one. Similarly, a casual hypothesis says that a change in the dependent variable is due to some variations in the independent variable.
Directional vs non-directional hypothesis
Directional hypothesis:.
A hypothesis that is built upon a certain directional relationship between two variables and constructed upon an already existing theory, is called a directional hypothesis. To understand more about what is directional hypothesis here is an example, Girls perform better than boys (‘better than’ shows the direction predicted)
Non-directional Hypothesis:
It involves an open-ended non-directional hypothesis that predicts that the independent variable will influence the dependent variable; however, the nature or direction of a relationship between two subject variables is not defined or clear.
For Example, there will be a difference in the performance of girls & boys (Not defining what kind of difference)
As a professional, we suggest you apply a non-directional alternative hypothesis when you are not sure of the direction of the relationship. Maybe you’re observing potential gender differences on some psychological test, but you don’t know whether men or women would have the higher ratio. Normally, this would say that you are lacking practical knowledge about the proposed variables. A directional test should be more common for tests.
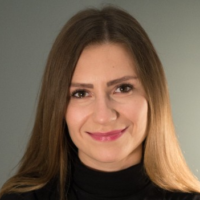
Author: Ula Kamburov-Niepewna
Updated: 18 November 2022
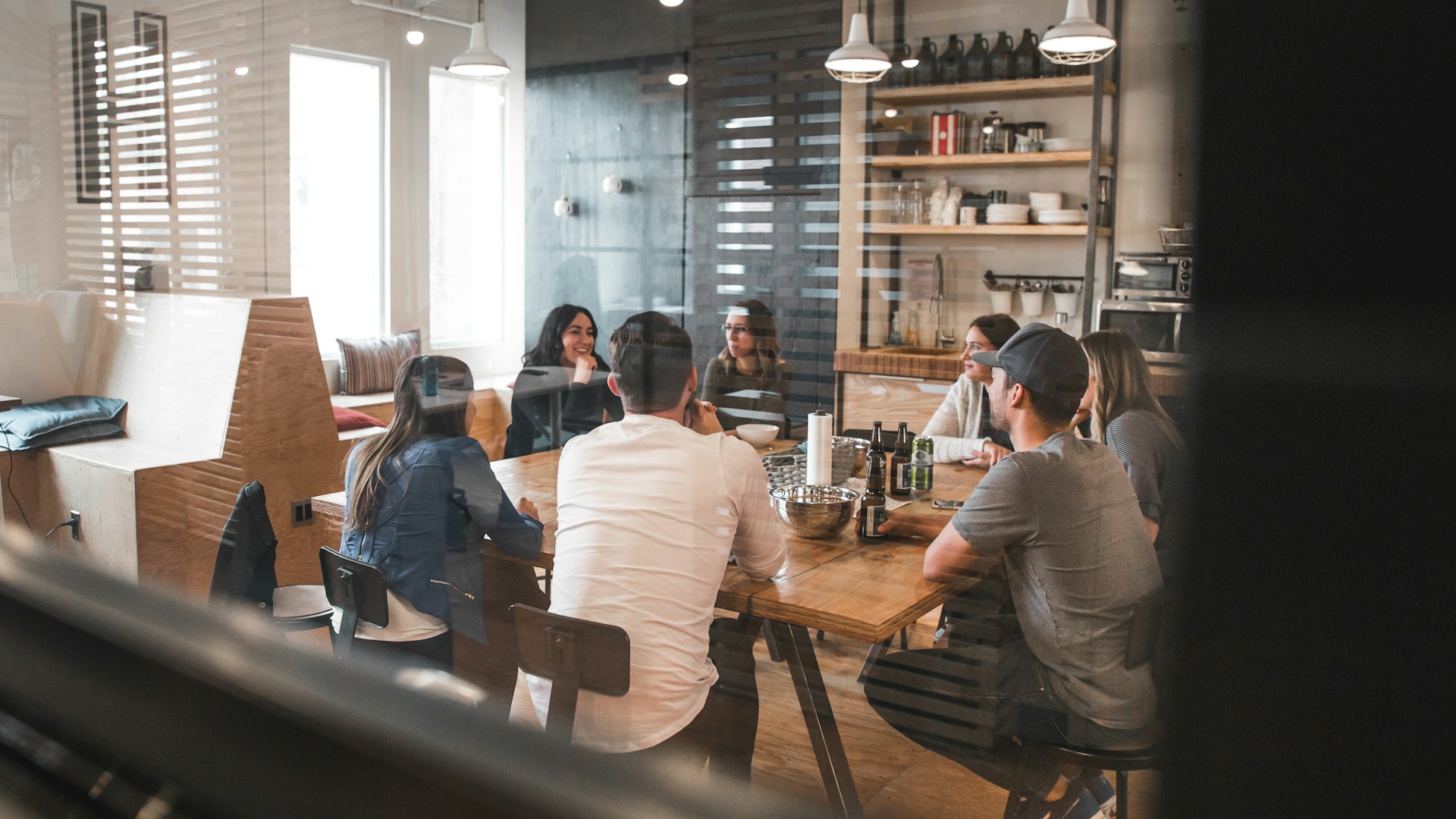
Top 10 Useful Employee Pulse Survey Tools
This guide explores the goal of pulse surveys, reviews the top tools available for conducting them, and contrasts their benefits with traditional survey methods.
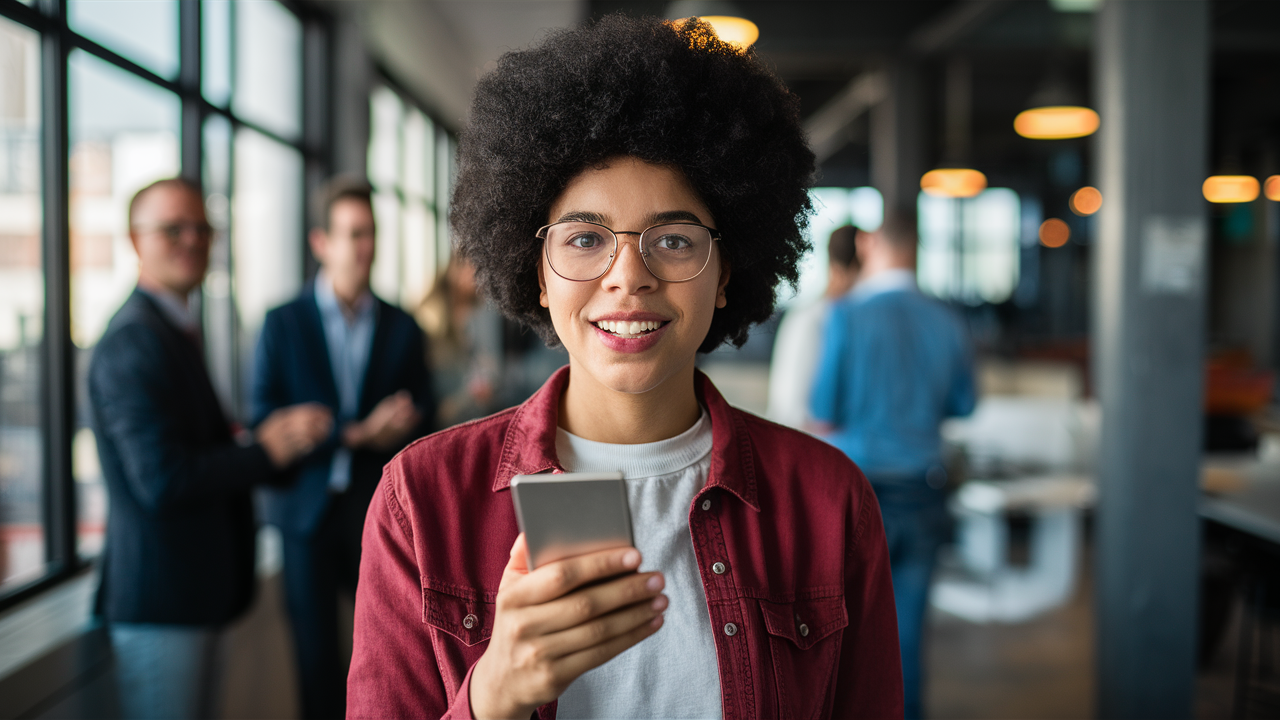
12 Post Event Survey Questions to Ask
After your meticulously planned event concludes, there’s one crucial step left: gathering feedback. Post-event surveys are invaluable tools for understanding attendee experiences, identifying areas for improvement, and maintaining attendee satisfaction.
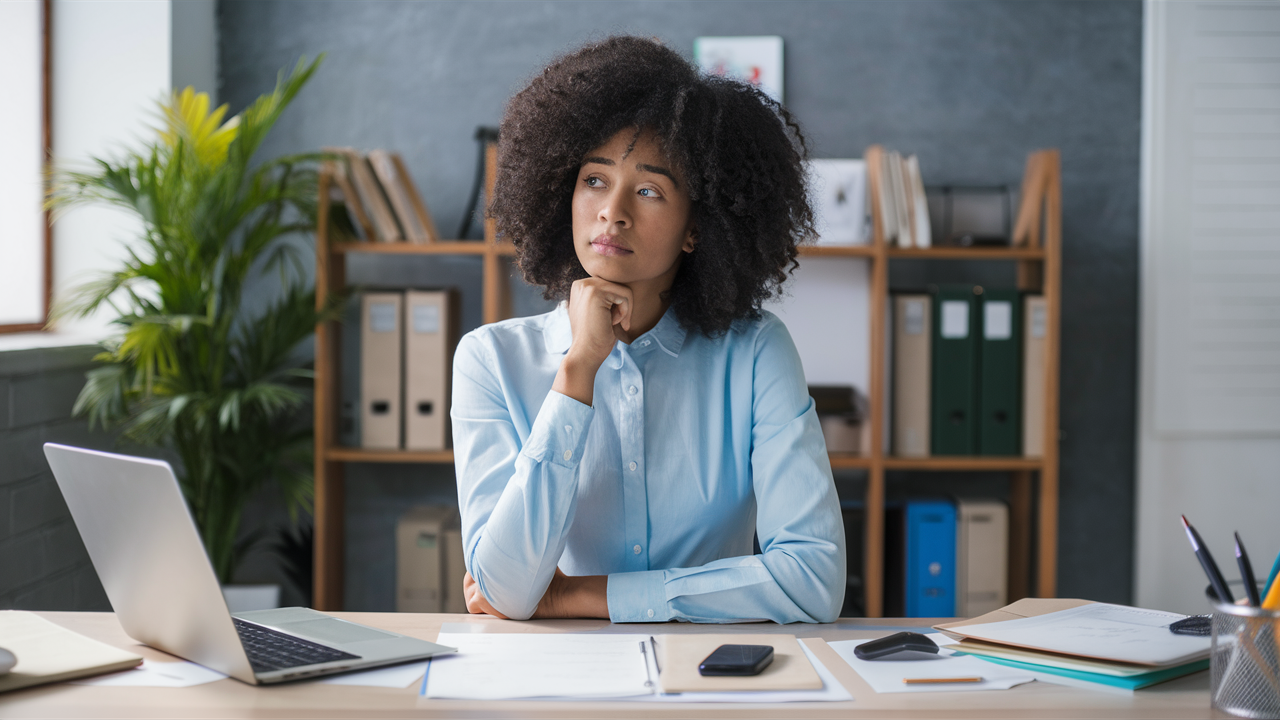
Yes or No Questions in Online Surveys
This article will discuss the benefits of using yes or no questions, explore common examples, and provide practical tips for using them effectively in your surveys.
- Privacy Policy

Home » What is a Hypothesis – Types, Examples and Writing Guide
What is a Hypothesis – Types, Examples and Writing Guide
Table of Contents

Definition:
Hypothesis is an educated guess or proposed explanation for a phenomenon, based on some initial observations or data. It is a tentative statement that can be tested and potentially proven or disproven through further investigation and experimentation.
Hypothesis is often used in scientific research to guide the design of experiments and the collection and analysis of data. It is an essential element of the scientific method, as it allows researchers to make predictions about the outcome of their experiments and to test those predictions to determine their accuracy.
Types of Hypothesis
Types of Hypothesis are as follows:
Research Hypothesis
A research hypothesis is a statement that predicts a relationship between variables. It is usually formulated as a specific statement that can be tested through research, and it is often used in scientific research to guide the design of experiments.
Null Hypothesis
The null hypothesis is a statement that assumes there is no significant difference or relationship between variables. It is often used as a starting point for testing the research hypothesis, and if the results of the study reject the null hypothesis, it suggests that there is a significant difference or relationship between variables.
Alternative Hypothesis
An alternative hypothesis is a statement that assumes there is a significant difference or relationship between variables. It is often used as an alternative to the null hypothesis and is tested against the null hypothesis to determine which statement is more accurate.
Directional Hypothesis
A directional hypothesis is a statement that predicts the direction of the relationship between variables. For example, a researcher might predict that increasing the amount of exercise will result in a decrease in body weight.
Non-directional Hypothesis
A non-directional hypothesis is a statement that predicts the relationship between variables but does not specify the direction. For example, a researcher might predict that there is a relationship between the amount of exercise and body weight, but they do not specify whether increasing or decreasing exercise will affect body weight.
Statistical Hypothesis
A statistical hypothesis is a statement that assumes a particular statistical model or distribution for the data. It is often used in statistical analysis to test the significance of a particular result.
Composite Hypothesis
A composite hypothesis is a statement that assumes more than one condition or outcome. It can be divided into several sub-hypotheses, each of which represents a different possible outcome.
Empirical Hypothesis
An empirical hypothesis is a statement that is based on observed phenomena or data. It is often used in scientific research to develop theories or models that explain the observed phenomena.
Simple Hypothesis
A simple hypothesis is a statement that assumes only one outcome or condition. It is often used in scientific research to test a single variable or factor.
Complex Hypothesis
A complex hypothesis is a statement that assumes multiple outcomes or conditions. It is often used in scientific research to test the effects of multiple variables or factors on a particular outcome.
Applications of Hypothesis
Hypotheses are used in various fields to guide research and make predictions about the outcomes of experiments or observations. Here are some examples of how hypotheses are applied in different fields:
- Science : In scientific research, hypotheses are used to test the validity of theories and models that explain natural phenomena. For example, a hypothesis might be formulated to test the effects of a particular variable on a natural system, such as the effects of climate change on an ecosystem.
- Medicine : In medical research, hypotheses are used to test the effectiveness of treatments and therapies for specific conditions. For example, a hypothesis might be formulated to test the effects of a new drug on a particular disease.
- Psychology : In psychology, hypotheses are used to test theories and models of human behavior and cognition. For example, a hypothesis might be formulated to test the effects of a particular stimulus on the brain or behavior.
- Sociology : In sociology, hypotheses are used to test theories and models of social phenomena, such as the effects of social structures or institutions on human behavior. For example, a hypothesis might be formulated to test the effects of income inequality on crime rates.
- Business : In business research, hypotheses are used to test the validity of theories and models that explain business phenomena, such as consumer behavior or market trends. For example, a hypothesis might be formulated to test the effects of a new marketing campaign on consumer buying behavior.
- Engineering : In engineering, hypotheses are used to test the effectiveness of new technologies or designs. For example, a hypothesis might be formulated to test the efficiency of a new solar panel design.
How to write a Hypothesis
Here are the steps to follow when writing a hypothesis:
Identify the Research Question
The first step is to identify the research question that you want to answer through your study. This question should be clear, specific, and focused. It should be something that can be investigated empirically and that has some relevance or significance in the field.
Conduct a Literature Review
Before writing your hypothesis, it’s essential to conduct a thorough literature review to understand what is already known about the topic. This will help you to identify the research gap and formulate a hypothesis that builds on existing knowledge.
Determine the Variables
The next step is to identify the variables involved in the research question. A variable is any characteristic or factor that can vary or change. There are two types of variables: independent and dependent. The independent variable is the one that is manipulated or changed by the researcher, while the dependent variable is the one that is measured or observed as a result of the independent variable.
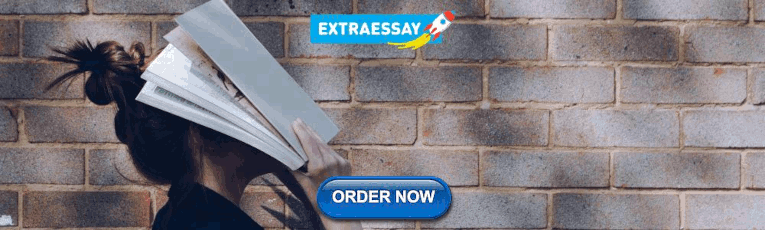
Formulate the Hypothesis
Based on the research question and the variables involved, you can now formulate your hypothesis. A hypothesis should be a clear and concise statement that predicts the relationship between the variables. It should be testable through empirical research and based on existing theory or evidence.
Write the Null Hypothesis
The null hypothesis is the opposite of the alternative hypothesis, which is the hypothesis that you are testing. The null hypothesis states that there is no significant difference or relationship between the variables. It is important to write the null hypothesis because it allows you to compare your results with what would be expected by chance.
Refine the Hypothesis
After formulating the hypothesis, it’s important to refine it and make it more precise. This may involve clarifying the variables, specifying the direction of the relationship, or making the hypothesis more testable.
Examples of Hypothesis
Here are a few examples of hypotheses in different fields:
- Psychology : “Increased exposure to violent video games leads to increased aggressive behavior in adolescents.”
- Biology : “Higher levels of carbon dioxide in the atmosphere will lead to increased plant growth.”
- Sociology : “Individuals who grow up in households with higher socioeconomic status will have higher levels of education and income as adults.”
- Education : “Implementing a new teaching method will result in higher student achievement scores.”
- Marketing : “Customers who receive a personalized email will be more likely to make a purchase than those who receive a generic email.”
- Physics : “An increase in temperature will cause an increase in the volume of a gas, assuming all other variables remain constant.”
- Medicine : “Consuming a diet high in saturated fats will increase the risk of developing heart disease.”
Purpose of Hypothesis
The purpose of a hypothesis is to provide a testable explanation for an observed phenomenon or a prediction of a future outcome based on existing knowledge or theories. A hypothesis is an essential part of the scientific method and helps to guide the research process by providing a clear focus for investigation. It enables scientists to design experiments or studies to gather evidence and data that can support or refute the proposed explanation or prediction.
The formulation of a hypothesis is based on existing knowledge, observations, and theories, and it should be specific, testable, and falsifiable. A specific hypothesis helps to define the research question, which is important in the research process as it guides the selection of an appropriate research design and methodology. Testability of the hypothesis means that it can be proven or disproven through empirical data collection and analysis. Falsifiability means that the hypothesis should be formulated in such a way that it can be proven wrong if it is incorrect.
In addition to guiding the research process, the testing of hypotheses can lead to new discoveries and advancements in scientific knowledge. When a hypothesis is supported by the data, it can be used to develop new theories or models to explain the observed phenomenon. When a hypothesis is not supported by the data, it can help to refine existing theories or prompt the development of new hypotheses to explain the phenomenon.
When to use Hypothesis
Here are some common situations in which hypotheses are used:
- In scientific research , hypotheses are used to guide the design of experiments and to help researchers make predictions about the outcomes of those experiments.
- In social science research , hypotheses are used to test theories about human behavior, social relationships, and other phenomena.
- I n business , hypotheses can be used to guide decisions about marketing, product development, and other areas. For example, a hypothesis might be that a new product will sell well in a particular market, and this hypothesis can be tested through market research.
Characteristics of Hypothesis
Here are some common characteristics of a hypothesis:
- Testable : A hypothesis must be able to be tested through observation or experimentation. This means that it must be possible to collect data that will either support or refute the hypothesis.
- Falsifiable : A hypothesis must be able to be proven false if it is not supported by the data. If a hypothesis cannot be falsified, then it is not a scientific hypothesis.
- Clear and concise : A hypothesis should be stated in a clear and concise manner so that it can be easily understood and tested.
- Based on existing knowledge : A hypothesis should be based on existing knowledge and research in the field. It should not be based on personal beliefs or opinions.
- Specific : A hypothesis should be specific in terms of the variables being tested and the predicted outcome. This will help to ensure that the research is focused and well-designed.
- Tentative: A hypothesis is a tentative statement or assumption that requires further testing and evidence to be confirmed or refuted. It is not a final conclusion or assertion.
- Relevant : A hypothesis should be relevant to the research question or problem being studied. It should address a gap in knowledge or provide a new perspective on the issue.
Advantages of Hypothesis
Hypotheses have several advantages in scientific research and experimentation:
- Guides research: A hypothesis provides a clear and specific direction for research. It helps to focus the research question, select appropriate methods and variables, and interpret the results.
- Predictive powe r: A hypothesis makes predictions about the outcome of research, which can be tested through experimentation. This allows researchers to evaluate the validity of the hypothesis and make new discoveries.
- Facilitates communication: A hypothesis provides a common language and framework for scientists to communicate with one another about their research. This helps to facilitate the exchange of ideas and promotes collaboration.
- Efficient use of resources: A hypothesis helps researchers to use their time, resources, and funding efficiently by directing them towards specific research questions and methods that are most likely to yield results.
- Provides a basis for further research: A hypothesis that is supported by data provides a basis for further research and exploration. It can lead to new hypotheses, theories, and discoveries.
- Increases objectivity: A hypothesis can help to increase objectivity in research by providing a clear and specific framework for testing and interpreting results. This can reduce bias and increase the reliability of research findings.
Limitations of Hypothesis
Some Limitations of the Hypothesis are as follows:
- Limited to observable phenomena: Hypotheses are limited to observable phenomena and cannot account for unobservable or intangible factors. This means that some research questions may not be amenable to hypothesis testing.
- May be inaccurate or incomplete: Hypotheses are based on existing knowledge and research, which may be incomplete or inaccurate. This can lead to flawed hypotheses and erroneous conclusions.
- May be biased: Hypotheses may be biased by the researcher’s own beliefs, values, or assumptions. This can lead to selective interpretation of data and a lack of objectivity in research.
- Cannot prove causation: A hypothesis can only show a correlation between variables, but it cannot prove causation. This requires further experimentation and analysis.
- Limited to specific contexts: Hypotheses are limited to specific contexts and may not be generalizable to other situations or populations. This means that results may not be applicable in other contexts or may require further testing.
- May be affected by chance : Hypotheses may be affected by chance or random variation, which can obscure or distort the true relationship between variables.
About the author
Muhammad Hassan
Researcher, Academic Writer, Web developer
You may also like

Data Collection – Methods Types and Examples

Delimitations in Research – Types, Examples and...

Research Process – Steps, Examples and Tips

Research Design – Types, Methods and Examples

Institutional Review Board – Application Sample...

Evaluating Research – Process, Examples and...
psychologyrocks
Hypotheses; directional and non-directional, what is the difference between an experimental and an alternative hypothesis.
Nothing much! If the study is a laboratory experiment then we can call the hypothesis “an experimental hypothesis”, where we make a prediction about how the IV causes an effect on the DV. If we have a non-experimental design, i.e. we are not able to manipulate the IV as in a natural or quasi-experiment , or if some other research method has been used, then we call it an “alternativehypothesis”, alternative to the null.
Directional hypothesis: A directional (or one tailed hypothesis) states which way you think the results are going to go, for example in an experimental study we might say…”Participants who have been deprived of sleep for 24 hours will have more cold symptoms in the following week after exposure to a virus than participants who have not been sleep deprived”; the hypothesis compares the two groups/conditions and states which one will ….have more/less, be quicker/slower, etc.
If we had a correlational study, the directional hypothesis would state whether we expect a positive or a negative correlation, we are stating how the two variables will be related to each other, e.g. there will be a positive correlation between the number of stressful life events experienced in the last year and the number of coughs and colds suffered, whereby the more life events you have suffered the more coughs and cold you will have had”. The directional hypothesis can also state a negative correlation, e.g. the higher the number of face-book friends, the lower the life satisfaction score “
Non-directional hypothesis: A non-directional (or two tailed hypothesis) simply states that there will be a difference between the two groups/conditions but does not say which will be greater/smaller, quicker/slower etc. Using our example above we would say “There will be a difference between the number of cold symptoms experienced in the following week after exposure to a virus for those participants who have been sleep deprived for 24 hours compared with those who have not been sleep deprived for 24 hours.”
When the study is correlational, we simply state that variables will be correlated but do not state whether the relationship will be positive or negative, e.g. there will be a significant correlation between variable A and variable B.
Null hypothesis The null hypothesis states that the alternative or experimental hypothesis is NOT the case, if your experimental hypothesis was directional you would say…
Participants who have been deprived of sleep for 24 hours will NOT have more cold symptoms in the following week after exposure to a virus than participants who have not been sleep deprived and any difference that does arise will be due to chance alone.
or with a directional correlational hypothesis….
There will NOT be a positive correlation between the number of stress life events experienced in the last year and the number of coughs and colds suffered, whereby the more life events you have suffered the more coughs and cold you will have had”
With a non-directional or two tailed hypothesis…
There will be NO difference between the number of cold symptoms experienced in the following week after exposure to a virus for those participants who have been sleep deprived for 24 hours compared with those who have not been sleep deprived for 24 hours.
or for a correlational …
there will be NO correlation between variable A and variable B.
When it comes to conducting an inferential stats test, if you have a directional hypothesis , you must do a one tailed test to find out whether your observed value is significant. If you have a non-directional hypothesis , you must do a two tailed test .
Exam Techniques/Advice
- Remember, a decent hypothesis will contain two variables, in the case of an experimental hypothesis there will be an IV and a DV; in a correlational hypothesis there will be two co-variables
- both variables need to be fully operationalised to score the marks, that is you need to be very clear and specific about what you mean by your IV and your DV; if someone wanted to repeat your study, they should be able to look at your hypothesis and know exactly what to change between the two groups/conditions and exactly what to measure (including any units/explanation of rating scales etc, e.g. “where 1 is low and 7 is high”)
- double check the question, did it ask for a directional or non-directional hypothesis?
- if you were asked for a null hypothesis, make sure you always include the phrase “and any difference/correlation (is your study experimental or correlational?) that does arise will be due to chance alone”
Practice Questions:
- Mr Faraz wants to compare the levels of attendance between his psychology group and those of Mr Simon, who teaches a different psychology group. Which of the following is a suitable directional (one tailed) hypothesis for Mr Faraz’s investigation?
A There will be a difference in the levels of attendance between the two psychology groups.
B Students’ level of attendance will be higher in Mr Faraz’s group than Mr Simon’s group.
C Any difference in the levels of attendance between the two psychology groups is due to chance.
D The level of attendance of the students will depend upon who is teaching the groups.
2. Tracy works for the local council. The council is thinking about reducing the number of people it employs to pick up litter from the street. Tracy has been asked to carry out a study to see if having the streets cleaned at less regular intervals will affect the amount of litter the public will drop. She studies a street to compare how much litter is dropped at two different times, once when it has just been cleaned and once after it has not been cleaned for a month.
Write a fully operationalised non-directional (two-tailed) hypothesis for Tracy’s study. (2)
3. Jamila is conducting a practical investigation to look at gender differences in carrying out visuo-spatial tasks. She decides to give males and females a jigsaw puzzle and will time them to see who completes it the fastest. She uses a random sample of pupils from a local school to get her participants.
(a) Write a fully operationalised directional (one tailed) hypothesis for Jamila’s study. (2) (b) Outline one strength and one weakness of the random sampling method. You may refer to Jamila’s use of this type of sampling in your answer. (4)
4. Which of the following is a non-directional (two tailed) hypothesis?
A There is a difference in driving ability with men being better drivers than women
B Women are better at concentrating on more than one thing at a time than men
C Women spend more time doing the cooking and cleaning than men
D There is a difference in the number of men and women who participate in sports
Revision Activity
writing-hypotheses-revision-sheet
Quizizz link for teachers: https://quizizz.com/admin/quiz/5bf03f51add785001bc5a09e
Share this:
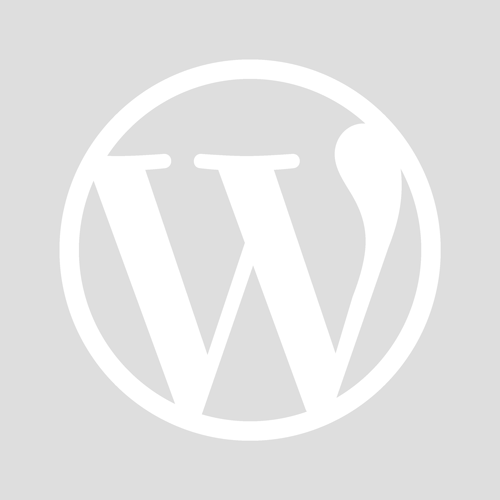
- Already have a WordPress.com account? Log in now.
- Subscribe Subscribed
- Copy shortlink
- Report this content
- View post in Reader
- Manage subscriptions
- Collapse this bar
Developing the research hypothesis
Affiliation.
- 1 Division of Abdominal Transplantation, Department of Surgery, University of North Carolina at Chapel Hill, USA. [email protected]
- PMID: 21867386
- DOI: 10.3109/08941939.2011.609449
The research hypothesis is needed for a sound and well-developed research study. The research hypothesis contributes to the solution of the research problem. Types of research hypotheses include inductive and deductive, directional and non-directional, and null and alternative hypotheses. Rejecting the null hypothesis and accepting the alternative hypothesis is the basis for building a good research study. This work reviews the most important aspects of organizing and establishing an efficient and complete hypothesis.
- Biomedical Research / methods*
- Data Interpretation, Statistical
- Research Design*
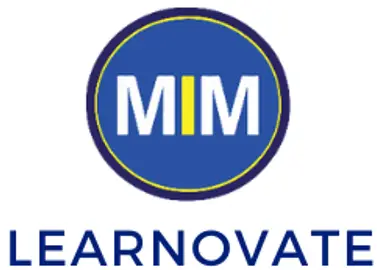
Directional vs. Non-Directional Hypothesis in Research
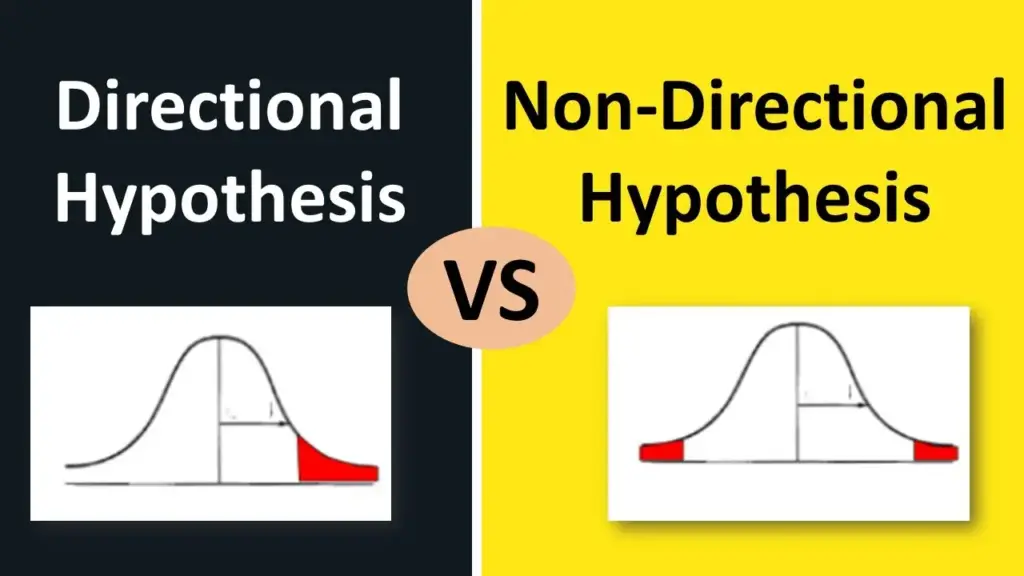
In the world of research and statistical analysis, formulating hypotheses is a crucial step in the scientific process. Hypotheses guide researchers in making predictions and testing relationships between variables. When it comes to hypotheses, there are two main types: directional and non-directional.
In this blog post, we will explore the differences between Directional vs. Non-Directional Hypothesis in Research and their implications in research.
- Table of Contents
Directional Hypothesis
A directional hypothesis, also known as a one-tailed hypothesis, is formulated with a specific predicted direction of the relationship between variables. It indicates an expectation of the relationship being either positive or negative.
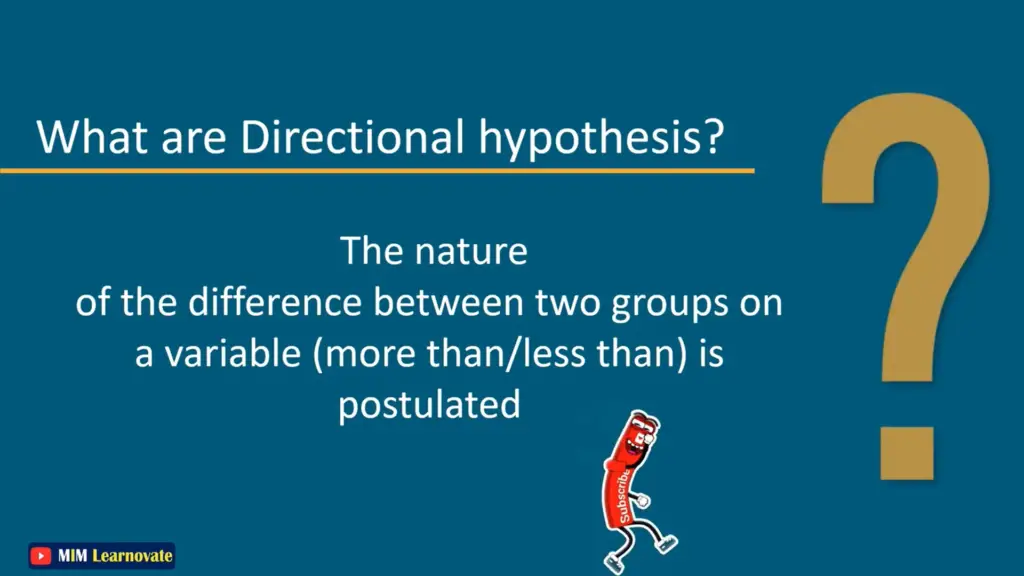
The directional hypothesis is often used when there is prior knowledge or theoretical reasoning supporting the predicted direction of the relationship. It allows researchers to make more specific predictions and draw conclusions based on the expected direction of the effect.
Example of Directional Hypothesis
For example, a directional hypothesis might state that “increased physical activity will lead to a decrease in body weight.” Here, the researcher expects a negative relationship between physical activity and body weight.
Advantages of Directional Hypothesis
- Specific predictions: Directional hypotheses provide a clear prediction of the expected relationship between variables, allowing for a focused investigation.
- Increased statistical power: By focusing on one direction of the relationship, researchers can allocate more statistical power to that specific direction, increasing the chances of detecting a significant effect if it exists.
Non-Directional Hypothesis
A non-directional hypothesis, also known as a two-tailed hypothesis, does not make a specific prediction about the direction of the relationship between variables. Instead, it states that there is a relationship, but without indicating whether it will be positive or negative.
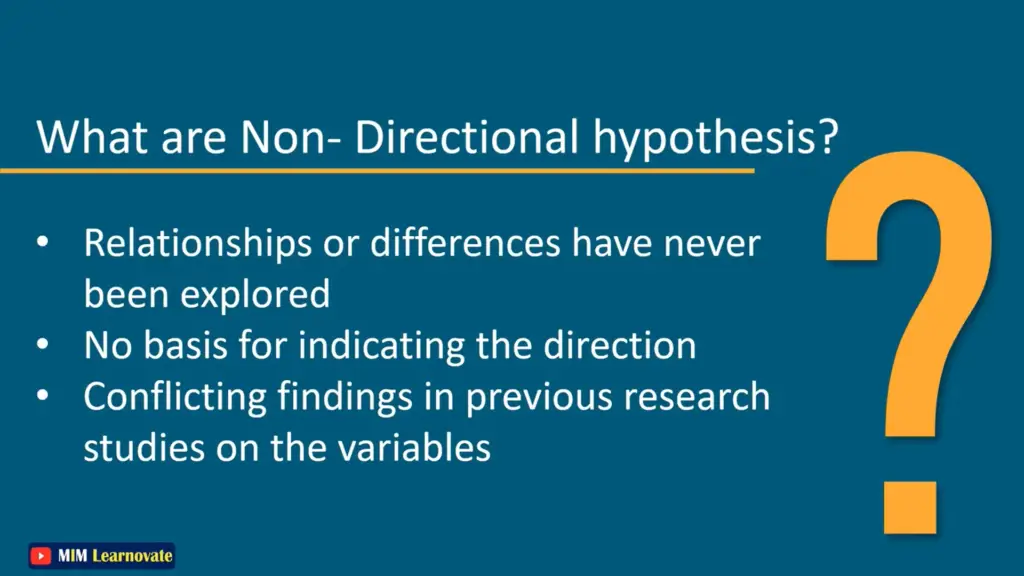
Non-directional hypotheses are often used when there is insufficient prior knowledge or theoretical basis to predict the direction of the relationship. It allows for a more exploratory approach, where the researcher is open to discovering the nature of the relationship through data analysis .
Read More: Internal Validity vs External Validity | Examples
Example of Non-Directional Hypothesis
For example, a non-directional hypothesis might state that “there is a relationship between caffeine consumption and reaction time.” Here, the researcher expects a relationship between the variables but does not specify the direction.
Read More: Population vs Sample | Examples
Advantages of Non-Directional Hypothesis:
- Flexibility: Non-directional hypotheses provide flexibility in exploring relationships between variables without preconceived notions about the direction of the effect.
- Open to unexpected findings : By not specifying the direction, researchers remain open to unexpected results or alternative explanations that may emerge during the analysis.
Difference Between Directional and Non-Directional Hypotheses
Choosing Between Directional and Non-Directional Hypotheses: The choice between a directional and non-directional hypothesis depends on the research question, existing knowledge, and theoretical background. Here are a few considerations for selecting the appropriate type of hypothesis:
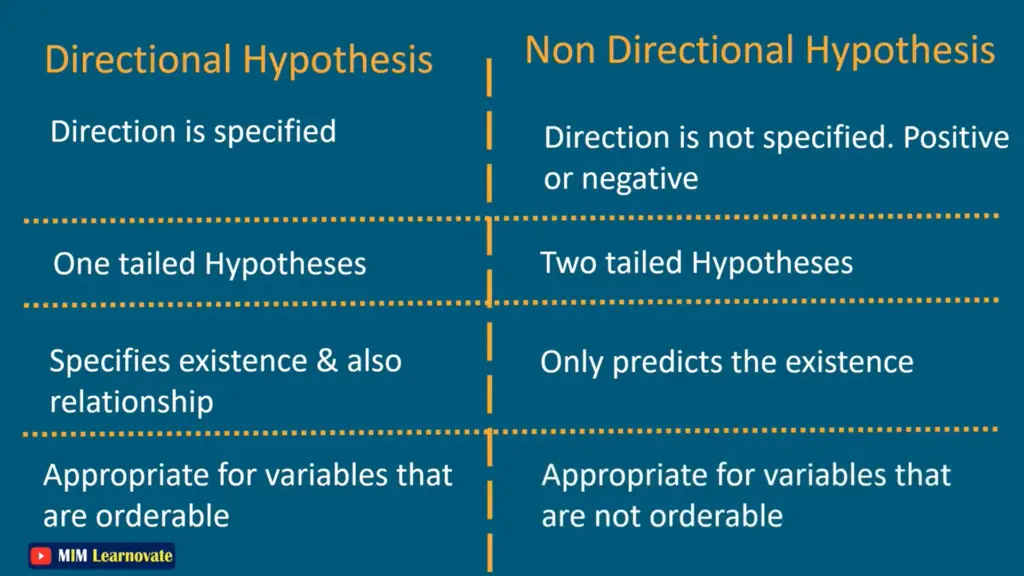
- Prior research: If previous studies have established a clear direction of the relationship, a directional hypothesis may be more appropriate.
- Theoretical reasoning: If there is a strong theoretical foundation supporting a specific direction, a directional hypothesis can provide a focused investigation.
- Exploratory nature: If the research question is exploratory or lacks prior knowledge, a non-directional hypothesis allows for a more open-ended investigation.
Read More: Reliability vs Validity | Examples
- Directional vs. Non-Directional Hypothesis
Formulating hypotheses is an essential step in the research process, guiding researchers in testing relationships between variables.
Directional hypotheses offer specific predictions about the expected direction of the relationship, whereas non-directional hypotheses allow for more exploratory investigations without preconceived notions of the direction.
The choice between these types of hypotheses depends on the research question, prior knowledge, and theoretical background.
By understanding the distinctions between directional and non-directional hypotheses, researchers can effectively formulate hypotheses that align with their research goals and contribute to the advancement of scientific knowledge.
Remember, hypotheses serve as a roadmap for research, and regardless of their type, they play a crucial role in scientific inquiry and the pursuit of knowledge.
Other articles
Please read through some of our other articles with examples and explanations if you’d like to learn more about research methodology.
Comparision
- Basic and Applied Research
- Cross-Sectional vs Longitudinal Studies
- Survey vs Questionnaire
- Open Ended vs Closed Ended Questions
- Experimental and Non-Experimental Research
- Inductive vs Deductive Approach
- Null and Alternative Hypothesis
- Reliability vs Validity
- Population vs Sample
- Conceptual Framework and Theoretical Framework
- Bibliography and Reference
- Stratified vs Cluster Sampling
- Sampling Error vs Sampling Bias
- Internal Validity vs External Validity
- Full-Scale, Laboratory-Scale and Pilot-Scale Studies
- Plagiarism and Paraphrasing
- Research Methodology Vs. Research Method
- Mediator and Moderator
- Type I vs Type II error
- Descriptive and Inferential Statistics
- Microsoft Excel and SPSS
- Parametric and Non-Parametric Test
- Independent vs. Dependent Variable – MIM Learnovate
- Research Article and Research Paper
- Proposition and Hypothesis
- Principal Component Analysis and Partial Least Squares
- Academic Research vs Industry Research
- Clinical Research vs Lab Research
- Research Lab and Hospital Lab
- Thesis Statement and Research Question
- Quantitative Researchers vs. Quantitative Traders
- Premise, Hypothesis and Supposition
- Survey Vs Experiment
- Hypothesis and Theory
- Independent vs. Dependent Variable
- APA vs. MLA
- Ghost Authorship vs. Gift Authorship
- Research Methods
- Quantitative Research
- Qualitative Research
- Case Study Research
- Survey Research
- Conclusive Research
- Descriptive Research
- Cross-Sectional Research
- Theoretical Framework
- Conceptual Framework
- Triangulation
- Grounded Theory
- Quasi-Experimental Design
- Mixed Method
- Correlational Research
- Randomized Controlled Trial
- Stratified Sampling
- Ethnography
- Ghost Authorship
- Secondary Data Collection
- Primary Data Collection
- Ex-Post-Facto
- Dissertation Topic
- Thesis Statement
- Research Proposal
- Research Questions
- Research Problem
- Research Gap
- Types of Research Gaps
- Operationalization of Variables
- Literature Review
- Research Hypothesis
- Questionnaire
- Reliability
- Measurement of Scale
- Sampling Techniques
- Acknowledgements
- PLS-SEM model
- Principal Components Analysis
- Multivariate Analysis
- Friedman Test
- Chi-Square Test (Χ²)
- Effect Size
Related Posts
Survey sampling: what it is, types & tips, cluster sampling | method and examples, who is a good peer reviewer, peer review | types of peer review, ethics in research: safeguarding integrity and credibility, advantages and disadvantages of snowball sampling, exploring qualitative researcher skills: what they are and how to develop them, difference between quota sampling and stratified sampling, how effective laboratory design impacts health, safety, and productivity, why is laboratory safety important in research, leave a reply cancel reply.
Save my name, email, and website in this browser for the next time I comment.
Live revision! Join us for our free exam revision livestreams Watch now →
Reference Library
Collections
- See what's new
- All Resources
- Student Resources
- Assessment Resources
- Teaching Resources
- CPD Courses
- Livestreams
Study notes, videos, interactive activities and more!
Psychology news, insights and enrichment
Currated collections of free resources
Browse resources by topic
- All Psychology Resources
Resource Selections
Currated lists of resources
Directional Hypothesis
A directional hypothesis is a one-tailed hypothesis that states the direction of the difference or relationship (e.g. boys are more helpful than girls).
- Share on Facebook
- Share on Twitter
- Share by Email
Research Methods: MCQ Revision Test 1 for AQA A Level Psychology
Topic Videos
Example Answers for Research Methods: A Level Psychology, Paper 2, June 2018 (AQA)
Exam Support
Example Answer for Question 14 Paper 2: AS Psychology, June 2017 (AQA)
Model answer for question 11 paper 2: as psychology, june 2016 (aqa), a level psychology topic quiz - research methods.
Quizzes & Activities
Our subjects
- › Criminology
- › Economics
- › Geography
- › Health & Social Care
- › Psychology
- › Sociology
- › Teaching & learning resources
- › Student revision workshops
- › Online student courses
- › CPD for teachers
- › Livestreams
- › Teaching jobs
Boston House, 214 High Street, Boston Spa, West Yorkshire, LS23 6AD Tel: 01937 848885
- › Contact us
- › Terms of use
- › Privacy & cookies
© 2002-2024 Tutor2u Limited. Company Reg no: 04489574. VAT reg no 816865400.
Economic complexity, greenfield investments, and energy innovation: policy implications for sustainable development goals in newly industrialised economies
- Research Article
- Published: 15 May 2024
Cite this article
- Muhammad Farhan Bashir ORCID: orcid.org/0000-0001-5103-4639 1 ,
- Roula Inglesi-Lotz 2 ,
- Ummara Razi 3 , 4 &
- Luqman Shahzad 5
The crucial role of environmental assessment quality has been recognised by environmental and sustainable development goals in addressing climate change challenges. By focusing on the key identifier of environmental assessment, progress can be made towards overcoming climate change issues effectively. The current study considers environmental commitments under COP28 to study the role of economic complexity, greenfield investments, and energy innovation in environmental degradation in newly industrialised economies from 1995 to 2021. We employ novel panel estimations from CS-ARDL, CS-DL, AMG, and CCEMG to confirm that economic growth and greenfield investments degrade environmental quality. On the other hand, energy innovation and urbanisation improve environmental sustainability. Lastly, we confirm the EKC hypothesis for economic complexity as well. Given the reported empirical findings, the study suggests policymakers must focus on economic complexity to transform industrial sectors’ economic potential. Furthermore, foreign investment projects must be linked with environmental goals to increase renewable energy capacity.
This is a preview of subscription content, log in via an institution to check access.
Access this article
Price includes VAT (Russian Federation)
Instant access to the full article PDF.
Rent this article via DeepDyve
Institutional subscriptions
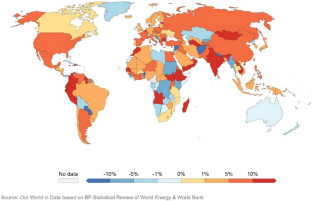
Data availability
Data and relevant materials will be available from the corresponding author.
Turkey, Thailand, South Africa, Philippines, Mexico, Malaysia, Indonesia, India, China, Brazil.
Hydrofluorocarbons sulphur, hexafluoride, and perfluorocarbons.
Abu Bakar NA (2019) Greenfield, mergers and acquisitions, energy consumption, and environmental performance in selected SAARC and ASEAN countries. Int J Energy Econ Policy 9(2):216–224
Adeel-Farooq RM, Abu Bakar NA, Olajide Raji J (2018) Green field investment and environmental performance: a case of selected nine developing countries of Asia. Environ Prog Sustain Energy 37:1085–1092
Article CAS Google Scholar
Adom PK, Opoku EEO, Yan IKM (2019) Energy demand–FDI nexus in Africa: do FDIs induce dichotomous paths? Energy Econ 81:928–941. https://doi.org/10.1016/j.eneco.2019.05.030
Article Google Scholar
Ahmed Z, Asghar MM, Malik MN, Nawaz K (2020a) Moving towards a sustainable environment: the dynamic linkage between natural resources, human capital, urbanization, economic growth, and ecological footprint in China. Resour Policy 67:101677
Ahmed Z, Zafar MW, Ali S (2020b) Linking urbanization, human capital, and the ecological footprint in G7 countries: an empirical analysis. Sustain Cities Soc 55:102064
Akadiri S saint, Adebayo TS, Asuzu OC et al (2022) Testing the role of economic complexity on the ecological footprint in China: a nonparametric causality-in-quantiles approach. Energy Environ 34(7):2290–2316
Al-Mulali U, Ozturk I (2015) The effect of energy consumption, urbanization, trade openness, industrial output, and the political stability on the environmental degradation in the MENA (Middle East and North African) region. Energy 84:382–389
Al-Mulali U, Weng-Wai C, Sheau-Ting L, Mohammed AH (2015) Investigating the environmental Kuznets curve (EKC) hypothesis by utilizing the ecological footprint as an indicator of environmental degradation. Ecol Indic 48:315–323
Aluko OA, Opoku EEO, Acheampong AO (2022) Economic complexity and environmental degradation: evidence from OECD countries. Bus Strategy Environ n/a. https://doi.org/10.1002/bse.3269
Álvarez-Herránz A, Balsalobre D, Cantos JM, Shahbaz M (2017) Energy innovations-GHG emissions nexus: fresh empirical evidence from OECD countries. Energy Policy 101:90–100. https://doi.org/10.1016/j.enpol.2016.11.030
Baek J (2016) A new look at the FDI–income–energy–environment nexus: dynamic panel data analysis of ASEAN. Energy Policy 91:22–27
Bashir MF (2022) Discovering the evolution of Pollution Haven Hypothesis: a literature review and future research agenda. Environ Sci Pollut Res 29:48210–48232. https://doi.org/10.1007/s11356-022-20782-1
Bashir MF, Ma B, Shahbaz M, Jiao Z (2020) The nexus between environmental tax and carbon emissions with the roles of environmental technology and financial development. PLoS One 15:e0242412
Bashir MF, MA B, Hussain HI et al (2022a) Evaluating environmental commitments to COP21 and the role of economic complexity, renewable energy, financial development, urbanization, and energy innovation: empirical evidence from the RCEP countries. Renew Energy 184:541–550. https://doi.org/10.1016/j.renene.2021.11.102
Bashir MF, Sadiq M, Talbi B et al (2022b) An outlook on the development of renewable energy, policy measures to reshape the current energy mix, and how to achieve sustainable economic growth in the post COVID-19 era. Environ Sci Pollut Res 29:43636–43647. https://doi.org/10.1007/s11356-022-20010-w
Bashir MF, Pan Y, Shahbaz M, Ghosh S (2023) How energy transition and environmental innovation ensure environmental sustainability? Contextual evidence from Top-10 manufacturing countries. Renew Energy 204:697–709. https://doi.org/10.1016/j.renene.2023.01.049
Bekun FV, Alola AA, Sarkodie SA (2019) Toward a sustainable environment: nexus between CO2 emissions, resource rent, renewable and nonrenewable energy in 16-EU countries. Sci Total Environ 657:1023–1029
Bilgili F, Kuşkaya S, Khan M et al (2021) The roles of economic growth and health expenditure on CO2 emissions in selected Asian countries: a quantile regression model approach. Environ Sci Pollut Res 28:44949–44972
Bukhari WAA, Pervaiz A, Zafar M et al (2023) Role of renewable and non-renewable energy consumption in environmental quality and their subsequent effects on average temperature: an assessment of sustainable development goals in South Korea. Environ Sci Pollut Res. https://doi.org/10.1007/s11356-023-30493-w
Can M, Gozgor G (2017) The impact of economic complexity on carbon emissions: evidence from France. Environ Sci Pollut Res 24:16364–16370
Charfeddine L (2017) The impact of energy consumption and economic development on ecological footprint and CO2 emissions: evidence from a Markov switching equilibrium correction model. Energy Econ 65:355–374
Charfeddine L, Mrabet Z (2017) The impact of economic development and social-political factors on ecological footprint: a panel data analysis for 15 MENA countries. Renew Sustain Energy Rev 76:138–154
Chu LK (2021) Economic structure and environmental Kuznets curve hypothesis: new evidence from economic complexity. Appl Econ Lett 28:612–616
Chu LK, Le NTM (2022) Environmental quality and the role of economic policy uncertainty, economic complexity, renewable energy, and energy intensity: the case of G7 countries. Environ Sci Pollut Res 29:2866–2882
Danish, Wang Z (2019) Investigation of the ecological footprint’s driving factors: what we learn from the experience of emerging economies. Sustain Cities Soc 49:101626
Destek MA, Manga M (2021) Technological innovation, financialization, and ecological footprint: evidence from BEM economies. Environ Sci Pollut Res 28:21991–22001
Dogan E, Ulucak R, Kocak E, Isik C (2020) The use of ecological footprint in estimating the environmental Kuznets curve hypothesis for BRICST by considering cross-section dependence and heterogeneity. Sci Total Environ 723:138063
Doğan B, Balsalobre-Lorente D, Nasir MA (2020) European commitment to COP21 and the role of energy consumption, FDI, trade and economic complexity in sustaining economic growth. J Environ Manag 273:111146
Doğan B, Driha OM, Balsalobre Lorente D, Shahzad U (2021) The mitigating effects of economic complexity and renewable energy on carbon emissions in developed countries. Sustain Dev 29:1–12
Doytch N (2020) The impact of foreign direct investment on the ecological footprints of nations. Environ Sustain Indic 8:100085
Google Scholar
Doytch N, Ashraf A (2022) The ecological footprints of greenfield FDI and cross-border M&A Sales. Environ Model Assess 27:935–951
Doytch N, Narayan S (2016) Does FDI influence renewable energy consumption? An analysis of sectoral FDI impact on renewable and non-renewable industrial energy consumption. Energy Econ 54:291–301
Dumitrescu EI, Hurlin C (2012) Testing for Granger non-causality in heterogeneous panels. Econ Model 29:1450–1460. https://doi.org/10.1016/j.econmod.2012.02.014
García-Quevedo J, Jové-Llopis E (2021) Environmental policies and energy efficiency investments. An industry-level analysis. Energy Policy 156:112461
Gormus S, Aydin M (2020) Revisiting the environmental Kuznets curve hypothesis using innovation: new evidence from the top 10 innovative economies. Environ Sci Pollut Res 27:27904–27913
Hassan ST, Wang P, Khan I, Zhu B (2023) The impact of economic complexity, technology advancements, and nuclear energy consumption on the ecological footprint of the USA: Towards circular economy initiatives. Gondwana Res 113:237–246
He K, Ramzan M, Awosusi AA et al (2021) Does globalization moderate the effect of economic complexity on CO2 emissions? Evidence from the top 10 energy transition economies. Front Environ Sci 9:778088
IEA (2020) World energy outlook 2020. International Energy Agency: Paris, France, p 2050
Ke H, Yang W, Liu X, Fan F (2020) Does innovation efficiency suppress the ecological footprint? Empirical evidence from 280 Chinese cities. Int J Environ Res Public Health 17:6826
Khezri M, Heshmati A, Khodaei M (2022) Environmental implications of economic complexity and its role in determining how renewable energies affect CO2 emissions. Appl Energy 306:117948
Kihombo S, Ahmed Z, Chen S et al (2021) Linking financial development, economic growth, and ecological footprint: what is the role of technological innovation? Environ Sci Pollut Res 28:61235–61245
Kosifakis G, Kampas A, Papadas CT (2020) Economic complexity and the environment: some estimates on their links. Int J Sustain Agric Manag Inform 6:261–271
Lee C-C, Chen M-P, Wu W (2022) The criticality of tourism development, economic complexity, and country security on ecological footprint. Environ Sci Pollut Res 29:37004–37040
Leitão NC, Balsalobre-Lorente D, Cantos-Cantos JM (2021) The impact of renewable energy and economic complexity on carbon emissions in BRICS countries under the EKC scheme. Energies (basel) 14:4908
Li S, Sun H, Sharif A et al (2024) Economic complexity, natural resource abundance and education: implications for sustainable development in BRICST economies. Resour Policy 89:104572. https://doi.org/10.1016/j.resourpol.2023.104572
Liang W, Yang M (2019) Urbanization, economic growth and environmental pollution: evidence from China. Sustai Comput: Inform Syst 21:1–9. https://doi.org/10.1016/j.suscom.2018.11.007
Long X, Ji X, Ulgiati S (2017) Is urbanization eco-friendly? An energy and land use cross-country analysis. Energy Policy 100:387–396
Luo W, Bai H, Jing Q et al (2018) Urbanization-induced ecological degradation in Midwestern China: an analysis based on an improved ecological footprint model. Resour Conserv Recycl 137:113–125
Ma B, Lin S, Bashir MF et al (2023a) Revisiting the role of firm-level carbon disclosure in sustainable development goals: research agenda and policy implications. Gondwana Res 117:230–242. https://doi.org/10.1016/j.gr.2023.02.002
Ma B, Sharif A, Bashir M, Bashir MF (2023b) The dynamic influence of energy consumption, fiscal policy and green innovation on environmental degradation in BRICST economies. Energy Policy 183:113823. https://doi.org/10.1016/j.enpol.2023.113823
Mahmood N, Zhao Y, Lou Q, Geng J (2022) Role of environmental regulations and eco-innovation in energy structure transition for green growth: evidence from OECD. Technol Forecast Soc Chang 183:121890
Majeed MT, Mazhar M, Samreen I, Tauqir A (2022) Economic complexities and environmental degradation: evidence from OECD countries. Environ Dev Sustain 24:5846–5866
Martins JM, Adebayo TS, Mata MN et al (2021) Modeling the relationship between economic complexity and environmental degradation: evidence from top seven economic complexity countries. Front Environ Sci 9(2021):744781
Mealy P, Teytelboym A (2022) Economic complexity and the green economy. Res Policy 51:103948
Mensah CN, Long X, Boamah KB et al (2018) The effect of innovation on CO2 emissions of OCED countries from 1990 to 2014. Environ Sci Pollut Res 25:29678–29698
Mrabet Z, AlSamara M, Hezam Jarallah S (2017) The impact of economic development on environmental degradation in Qatar. Environ Ecol Stat 24:7–38
Nathaniel S, Khan SAR (2020) The nexus between urbanization, renewable energy, trade, and ecological footprint in ASEAN countries. J Clean Prod 272:122709
Neagu O (2021) Economic complexity: a new challenge for the environment. Earth 2:1059–1076
Okamoto S (2013) Impacts of growth of a service economy on CO 2 emissions: Japan’s case. J Econ Struct 2:1–21
Pesaran MH (2007) A simple panel unit root test in the presence of cross-section dependence. J Appl Economet 22:265–312. https://doi.org/10.1002/jae.951
Ponce P, Álvarez-García J, Álvarez V, Irfan M (2022) Analysing the influence of foreign direct investment and urbanization on the development of private financial system and its ecological footprint. Environ Sci Pollut Res 30(4):9624–9641
Rafei M, Esmaeili P, Balsalobre-Lorente D (2022) A step towards environmental mitigation: How do economic complexity and natural resources matter? Focusing on different institutional quality level countries. Resour Policy 78:102848
Sadiq M, Wen F, Bashir MF, Amin A (2022) Does nuclear energy consumption contribute to human development? Modeling the effects of public debt and trade globalization in an OECD heterogeneous panel. J Clean Prod 375:133965. https://doi.org/10.1016/j.jclepro.2022.133965
Shahbaz M, Balsalobre D, Shahzad SJH (2019) The influencing factors of CO 2 emissions and the role of biomass energy consumption: statistical experience from G-7 countries. Environ Model Assess 24:143–161
Shahbaz M, Sinha A, Raghutla C, Vo XV (2022) Decomposing scale and technique effects of financial development and foreign direct investment on renewable energy consumption. Energy 238:121758
Swart J, Brinkmann L (2020) Economic complexity and the environment: evidence from Brazil. In: Universities and sustainable communities: meeting the goals of the agenda 2030. pp 3–45. Springer International Publishing, 2020
Wang Y, Kang L, Wu X, Xiao Y (2013) Estimating the environmental Kuznets curve for ecological footprint at the global level: a spatial econometric approach. Ecol Indic 34:15–21
World Bank (2022) World development indicators 2022. The World Bank 2022
Yahya F, Rafiq M (2020) Brownfield, greenfield, and renewable energy consumption: moderating role of effective governance. Energy Environ 31:405–423
Yilanci V, Pata UK (2020) Investigating the EKC hypothesis for China: the role of economic complexity on ecological footprint. Environ Sci Pollut Res 27:32683–32694
Yu Y, Jiang T, Li S et al (2020) Energy-related CO2 emissions and structural emissions’ reduction in China’s agriculture: an input–output perspective. J Clean Prod 276:124169
Download references
Moreover, we acknowledge funding support from Project (Grant No:2023JJ40061) supported by Natural Science Foundation of Hunan Province.
Author information
Authors and affiliations.
College of Management, Shenzhen University, Shenzhen, Guangzhou, People’s Republic of China
Muhammad Farhan Bashir
Department of Economics, University of Pretoria, Pretoria, South Africa
Roula Inglesi-Lotz
Department of Economic and Finance, Sunway Business School, Sunway University, Subang Jaya, Malaysia
Ummara Razi
Department of Business Administration, ILMA University, Karachi, Pakistan
Independent Researcher, Guangzhou, Guangdong, People’s Republic of China
Luqman Shahzad
You can also search for this author in PubMed Google Scholar
Contributions
Muhammad Farhan Bashir: conceptualization, methodology, writing draft, writing—revision; Roula Inglesi-Lotz: data curation, writing draft, methodology, data analysis; Ummara Razi: writing draft; Luqman Shahzad: data curation; methodology.
Corresponding author
Correspondence to Muhammad Farhan Bashir .
Ethics declarations
Ethics approval, consent to participate, consent for publication, competing interests.
The author declares no competing interest.
Additional information
Responsible Editor: Philippe Garrigues
Publisher's Note
Springer Nature remains neutral with regard to jurisdictional claims in published maps and institutional affiliations.
Rights and permissions
Springer Nature or its licensor (e.g. a society or other partner) holds exclusive rights to this article under a publishing agreement with the author(s) or other rightsholder(s); author self-archiving of the accepted manuscript version of this article is solely governed by the terms of such publishing agreement and applicable law.
Reprints and permissions
About this article
Bashir, M.F., Inglesi-Lotz, R., Razi, U. et al. Economic complexity, greenfield investments, and energy innovation: policy implications for sustainable development goals in newly industrialised economies. Environ Sci Pollut Res (2024). https://doi.org/10.1007/s11356-024-33433-4
Download citation
Received : 07 November 2023
Accepted : 18 April 2024
Published : 15 May 2024
DOI : https://doi.org/10.1007/s11356-024-33433-4
Share this article
Anyone you share the following link with will be able to read this content:
Sorry, a shareable link is not currently available for this article.
Provided by the Springer Nature SharedIt content-sharing initiative
- Newly industrialised countries
- Energy transition
- Environmental degradation
- Economics complexity
- Greenfield investment
- Find a journal
- Publish with us
- Track your research
BRIEF RESEARCH REPORT article
Alzheimer’s disease and oral manifestations: a bi-directional mendelian randomization study.
- 1 Department of Neurology, Laboratory of Neurodegenerative Disorders, National Clinical Research Center for Geriatric, West China Hospital, Sichuan University, Chengdu, China
- 2 Department of Neurology, West China Hospital, Sichuan University/West China School of Nursing, Sichuan University, Chengdu, China
- 3 Outpatient Department, West China Hospital, Sichuan University, Chengdu, China
Background: Epidemiological studies have provided evidence suggesting an association between Alzheimer’s disease (AD) and various oral manifestations. However, conflicting conclusions have been drawn, and whether a causal association truly exists remains unclear.
Methods: In order to investigate the potential causal association between AD and prevalent oral diseases, we conducted a bi-directional two-sample Mendelian randomization analysis based on summary statistics from genome-wide association studies of AD ( N = 63,926), as well as mouth ulcer ( N = 461,103), oral cavity cancer ( N = 4,151), and periodontal disease ( N = 527,652).
Results: We identified that one standard increase in the risk of AD was causally associated with a reduced risk of oral cavity cancer (OR = 0.76, 95% CI: 0.63–0.92, p = 3.73 × 10 −3 ). In the opposite direction, oral conditions were not causally associated with risk of AD.
Conclusion: The present findings contributed to a better understanding of the correlation between AD and oral conditions, specifically oral cavity cancer. These results also identified new avenues for exploring the underlying mechanisms of oral cavity cancer.
Introduction
The world’s older population is continuing to grow at an unprecedented rate. Around 8.5% of people worldwide are aged 65 and over nowadays, and this percentage is projected to nearly double over the next three decades. Oral health has been a neglected dimension of global health in the aging population ( 1 ). For example, oral cavity cancer is of particular concern for older adults, with around 70% diagnosed between the ages of 46 and 75 years old ( 2 ). The prevalence of periodontal disease increased gradually with age ( 3 ), and is highest in those aged 60 years and older ( 4 ). Mouth ulcers affect individuals of all ages, but are more common in older adults ( 5 ). Oral diseases and conditions that are associated with aging concomitantly result in an increased need for preventive, restorative, and periodontal dental care.
Alzheimer’s disease (AD) is the most common manifestation of elderly dementia, characterized by extracellular β-amyloid peptide, hyperphosphorylated tau protein, and neuronal or synaptic loss. Close correlation has been identified between AD and common oral conditions ( 6 , 7 ). For example, compared to older people who are cognitively intact, older people who develop dementia are at increased risk of establishing oral health problems, such as periodontitis and ulcers ( 8 ). Among those aged 65 years and over, AD incidence and mortality were consistently associated with periodontal disease ( 9 ), and previous study has shown that older people with periodontal disease may have an increased risk of AD ( 10 ). Meanwhile, inverse association has been identified between AD and various types of cancer, suggesting potential shared inverse etiological mechanisms ( 11 ). A previous longitudinal population-based cohort study identified that patients with oral cavity cancer were in higher risk of developing AD (HR = 1.74, 95% CI: 1.27–2.83) ( 12 ), though negative result has also been reported ( 13 ). The precise mechanism and aetiology of oral manifestations in AD is not clear. One hypothesis is the chronic infection and system inflammation ( 14 ). In oral diseases, the exacerbated host inflammatory response is associated with greater amount of tissue damage, while inflammation plays a key role in the pathophysiology of AD. Another mechanism that has been suggested to underlie association between poor oral health and AD is diet and nutrition. Chewing deficiency among the elderly affects quality of diet and nutrient intake by leading to reliance on mashed food, carbohydrate, and confectioneries, as well as fewer fruits and vegetables, which might be risk factor of AD ( 15 ). In addition, AD patients have greater impairment of oral health because of their progressive cognitive impairment, which would affect their oral hygiene habits. Poor oral hygiene, difficulty in wearing dentures, and the inability to self-care such as carrying out oral hygiene procedures are the probable causes of impaired oral health in AD ( 6 ). These multiple lines of evidence suggested important correlation between AD and oral health, yet the complex interplay between AD and oral conditions complicates the analysis. Observational studies face the inherent challenge of accounting for confounding biases without the benefit of randomization, making it difficult to establish causation. Therefore, the question of whether AD plays a causal role in these oral manifestations remains largely unknown.
In this context, we aimed to explore the causal association between AD and prevalent oral diseases with two-sample Mendelian randomization (MR) analysis, which is a statistical method increasingly utilized to establish causal relationship between an exposure and a specific outcome. This method relies on leveraging single nucleotide polymorphisms (SNPs) as instrumental variables to strengthen the validity of causal inference ( 16 , 17 ). By employing genetic variants as instrumental variables, MR offers a means to mitigate bias resulting from unobserved confounding between the exposure and outcome variables. In the current study, we investigated the causal relationship between AD and prevalent oral diseases, specifically mouth ulcers, periodontal disease, and oral cavity cancer, and identified that AD was associated with a decreased risk of oral cavity cancer.
GWAS summary datasets
The GWAS summary datasets utilized in the current study were provided in Supplementary Table S1 . We obtained summary statistics of AD from a previous genome-wide association study (GWAS) among 21,982 cases and 41,944 controls of European ancestry ( 18 ). To select instrumental variables, we identified SNPs that met the genome-wide significance threshold ( p < 5 × 10 −8 ) and exhibited low linkage disequilibrium ( r 2 < 0.001) with other SNPs within a clumping distance of 10,000 kb as exposure for each trait. We analyzed three oral diseases as outcomes, including mouth ulcer ( N = 461,103) ( 19 ), periodontal disease ( N = 527,652) ( 20 ), and oral cavity cancer ( N = 4,151) ( 21 ), based on available summary statistics from previous GWAS. The effects were harmonized to ensure that the effect estimates in both the exposure and outcome GWAS corresponded to the same allele for each SNP.
Two-sample Mendelian randomization analysis
Conducting MR analysis requires three fundamental assumptions: (A) relevance assumption: the instrumental variables used are associated with the exposure of interest; (B) independence assumption: the instrumental variables are independent of any confounding factors that may influence the association between the exposure and the outcome; and (C) exclusion-restriction assumption: the instrumental variables are conditionally independent of the outcome, given the exposure and the confounding factors. These assumptions serve as prerequisites for valid causal inference in MR analyses.
In our study, a two-sample MR analysis was conducted using the random effects inverse variance weighted (IVW) method. This approach allowed us to assess the causal effect of AD on the risk of oral diseases. Bonferroni-corrected thresholds (0.05/3 = 0.017) were adopted to account for multiple testing. Additionally, to validate the significant findings, we employed the weighted median and weighted mode methods as alternative approaches. These two methods were used as supplementary analyses to confirm the robustness and consistency of the results.
In the second stage, we investigated whether oral conditions, acting as a potential risk factor, could causally influence the risk of AD. We conducted MR analysis to assess the causal role of oral conditions in AD with the same workflow. SNPs meeting the genome-wide significance threshold ( p < 5 × 10 −8 ) and having low linkage disequilibrium ( r 2 < 0.001) with other SNPs within a clumping distance of 10,000 kb were chosen as exposure for each oral disease, while summary statistics of AD were used as the outcome. Given that no loci were significant ( p < 5 × 10 −8 ) for oral cavity cancer and periodontal disease, a more relaxed significance threshold ( p < 1 × 10 −5 ) was used.
In order to assess the potential violation of MR assumptions in the analysis, we conducted several sensitivity analyses to evaluate the robustness of our results and to account for any potential biases or violations of the MR assumptions. To assess the strength of the effect of each SNP on the exposure traits, we calculated the F-statistic. SNPs with an F-statistic below 10 were considered as weak instruments and subsequently excluded from the analysis. It is important to address the issue of pleiotropy, where the selected SNPs may have effects on multiple traits. To identify and mitigate the influence of horizontal pleiotropy outliers, we employed MR-PRESSO, a statistical method specifically designed for detecting and correcting for such outliers. The identified outliers were removed from the analysis to minimize the impact of horizontal pleiotropy on the results. Cochran’s Q statistic, which is derived from the IVW estimate, should follow a χ 2 distribution with degrees of freedom equal to the number of SNPs minus 1. In order to assess heterogeneity in the MR estimates, we conducted Cochran’s Q test, which allowed us to examine if there was significant variation across the instrumental variables in estimating the causal effect. Additionally, we employed MR-Egger regression, which is a weighted linear regression model that incorporates the SNP-outcome effects and SNP-exposure effects, allowing for the estimation of the intercept. The intercept in MR-Egger regression provides an estimate of the average pleiotropic bias, which can indicate the presence of potential pleiotropy in the analysis. The statistical power was calculated at http://cnsgenomics.com/shiny/mRnd/ . The R package TwoSampleMR 0.5.6 was used for the statistical analyses.
Using the two-sample MR approach, we investigated the potential role of AD in the risk of three common oral diseases. Results showed that one standard deviation increase in genetically determined higher risk of AD was significantly associated with a reduced risk of oral cavity cancer (OR = 0.76, 95% CI: 0.63–0.92, p = 3.73 × 10 −3 ) ( Figure 1 ). Such association was further verified by the weighted median (OR = 0.75, 95% CI: 0.59–0.96, p = 0.023) and weighted mode (OR = 0.75, 95% CI: 0.58–0.97, p = 0.042) methods ( Figure 2 ). The funnel plot demonstrated a visually symmetrical distribution, indicating the absence of significant directional pleiotropy that could influence the estimates ( Figure 2 ). In contrast, our analysis did not reveal a significant association between AD and the risk of mouth ulcers or periodontal disease. In the opposite direction, we did not find any significant associations suggesting that oral conditions act as risk factors for AD ( Figure 1 ; Supplementary Figures S2–S5 ).
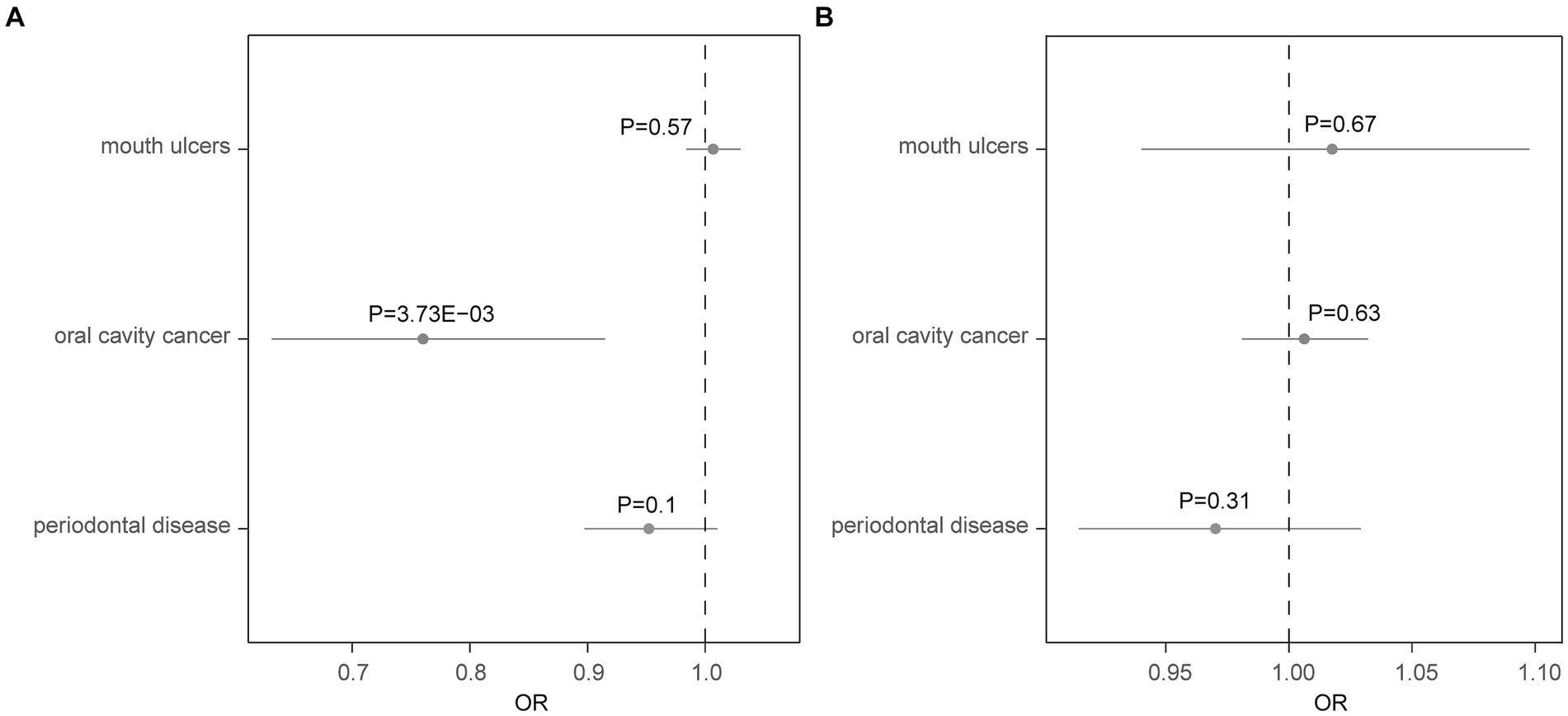
Figure 1 . Forest plot showing results from the Mendelian randomization analysis. Results from the Mendelian randomization (MR) analysis to evaluate causal role of (A) Alzheimer’s disease in three oral conditions, and (B) three oral conditions in Alzheimer’s disease. Estimates are per 1 standard deviation (SD) increase in the trait. Bold p -value denotes significant association ( p < 0.017).
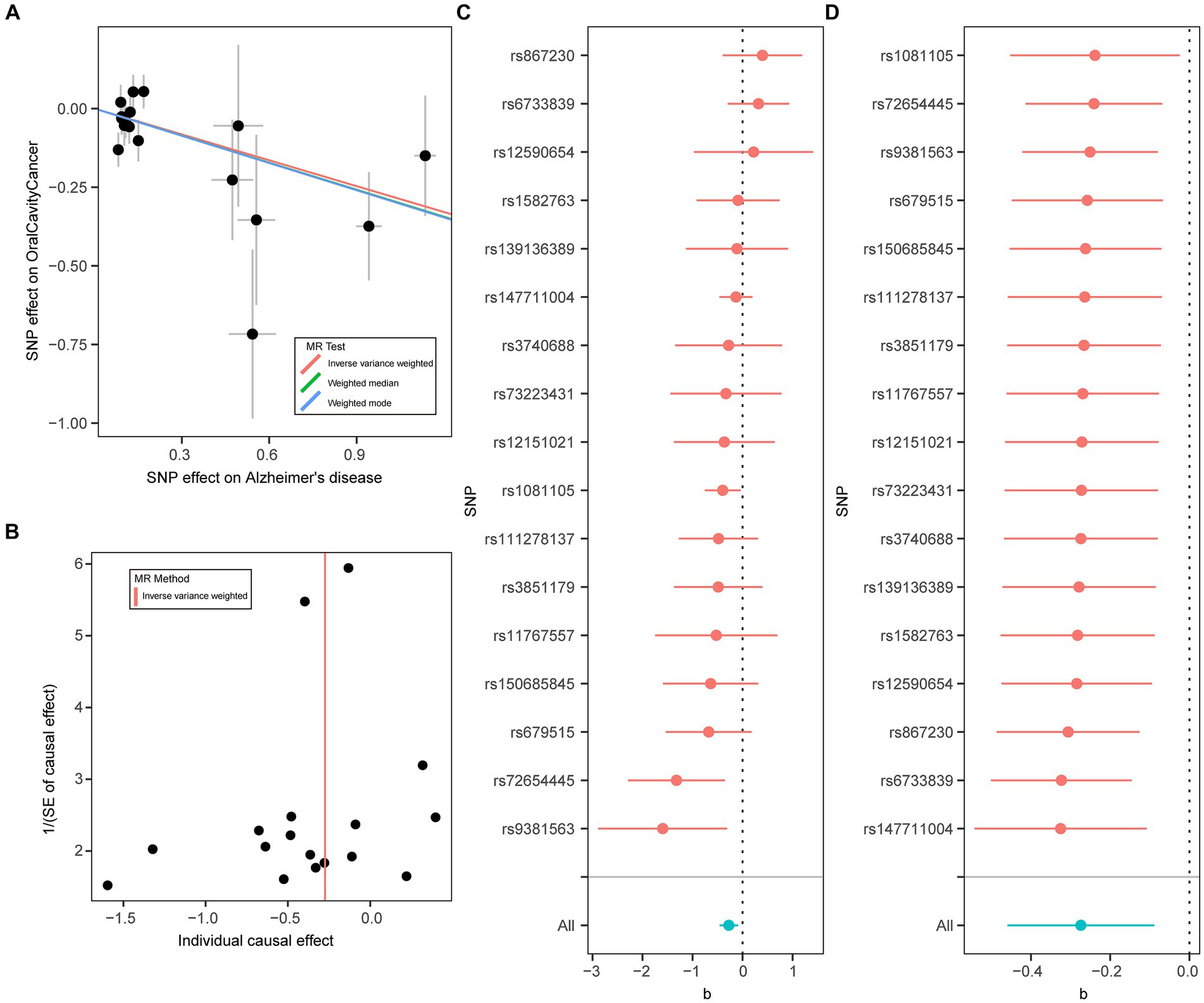
Figure 2 . Mendelian randomization analysis results for Alzheimer’s disease on risk of oral cavity cancer. (A) Scatter plot of genetic associations with Alzheimer’s disease (horizontal lines) against genetic associations with oral cavity cancer (vertical lines). Error bars for genetic associations are 95% confidence intervals. The slopes of each line in the scatter plot represent the causal association for each method. (B) Funnel plot of single-SNP effect estimates and corresponding inverse standard errors. (C) Forest plot of the association of individual SNPs with Alzheimer’s disease and oral cavity cancer, together with pooled estimates. (D) Forest plot of the results of the leave-one-out sensitivity analysis, where each SNP in the instrument was iteratively removed from the instrumental variables.
Furthermore, we performed a number of sensitivity analyses to validate the causal association between AD and the oral diseases. The Cochran’s Q test indicated no significant heterogeneity among the instrumental variables, suggesting consistent estimates of the causal association between AD and the oral diseases ( Table 1 ; Supplementary Table S2 ). The F statistics of all the instrumental variables were above 10 (ranging from 19 to 962), indicating that the selected instruments were sufficiently strong to provide reliable estimates. Furthermore, the MR-Egger regression analysis did not provide evidence of horizontal pleiotropy, as the intercept was not significantly deviated from zero. Moreover, the MR-PRESSO analysis did not detect any potential instrumental outliers, indicating that the instrumental variables used in the analysis were not influenced by pleiotropic effects. Overall, these results enhanced the validity and reliability of the MR analysis results.

Table 1 . Heterogeneity and horizontal pleiotropy analyses of Alzheimer’s disease in three oral conditions.
Previous clinical and epidemiological studies have indicated a close relationship between AD and various oral diseases. However, the conclusions drawn from these studies have been inconsistent. Moreover, unmeasured confounding factors in clinical studies can introduce biases that are difficult to account for. In light of these challenges, we conducted a study utilizing the MR approach to examine the causal role of AD in the risk of three oral diseases. Additionally, we investigated the reverse relationship between these oral diseases and AD. Our analysis revealed a significant association between AD and a decreased risk of oral cavity cancer. These findings contribute to a better understanding of the involvement of AD in oral diseases and provide insights that can aid in the investigation of the pathogenesis of oral cavity cancer.
Cancer and AD are common diseases in the aging population. Previous epidemiological studies have identified an inverse association between AD and various types of cancer, suggesting potential shared inverse etiological mechanisms ( 11 , 22 ). However, conflicting results have also been reported ( 23 ). In the current study, we identified that AD was associated with a decreased risk of oral cavity cancer using the MR approach. Though the precise aetiology of reduced risk of oral cavity cancer in AD remains unclear, the biological mechanisms involved in both diseases have been proposed, such as sustaining proliferative signaling, cell death, and grow suppressors ( 24 ). Additionally, processes related to cell growth and survival, as well as the production of specific molecules including the anti-stress response protein vimentin and the enzyme carbonic anhydrase, are all upregulated in cancer, while these processes and proteins are downregulated in AD ( 25 ). Another study found that the proteins p53 and PIN1 were implicated in both cancer and Alzheimer’s biomarkers ( 26 ). These accumulating evidence pointed towards potential shared pathogenesis between AD and oral cavity cancer. In the opposite direction, we did not identify association between oral cavity cancer and AD, suggesting oral cavity cancer might not causally influence the risk of AD from the genetic perspective. In contrast, one previous epidemiological study has identified higher risk of AD in patients with oral cavity cancer ( 12 ). However, the increased risk might be due to treatment for the cancer. A majority of patients with oral cavity cancer will receive radiation therapy, while radiation-related brain damage will be caused as the treatment site is adjacent to the brain when radiation therapy is administered. Consistently, one previous cohort study showed that patients with nasopharyngeal cancer treated with radiation therapy were more likely to develop dementia than normal controls ( 27 ). Nevertheless, the sample size of the GWAS on oral cavity cancer used in the current study was small, with no significant variants identified. The suggestively significant variants used as instrumental variables might limit the statistical power. Therefore, replication studies with larger sample sizes were still needed. Meanwhile, more in-depth studies are required to gain a comprehensive understanding of the role of AD in the development and progression of oral cavity cancer. Further investigations can explore the underlying mechanisms, such as common molecular pathways or genetic factors that may contribute to the observed association.
Periodontitis is a prevalent chronic inflammatory disease affecting the teeth-supporting tissues, ultimately leading to tooth-loss if not treated. Periodontitis is linked to several systemic conditions with underlying pathophysiological features related to chronic inflammation or altered immune system function such as rheumatoid arthritis and diabetes mellitus. There is also increasing evidence that inflammation plays a key role in the pathophysiology of AD ( 28 ). From the epidemiological perspective, one previous study found that periodontitis was associated with cognitive impairment among 2,355 participants aged 60 years or over ( 29 ). However, it is important to note that the limited statistical power of our study may have influenced the results. Given this limitation, further replication of our findings is warranted to draw more definitive conclusions regarding the association between AD and periodontal disease.
Previous study has shown that patients with AD had poorer oral health than the general population ( 7 ). Cognitive impairment in patients with AD may contribute to the worsening of oral hygiene thus aggravating oral infections ( 30 ). Meanwhile, AD could cause frequent dehydration, which leads to dry mouth from low saliva production. This puts patients with AD at higher risk of tooth decay, gum disease, and tooth loss if not treated properly. In the current study, we did not identify a causal association between AD and the risk of mouth ulcer. However, it is important to acknowledge that the proportion of variance explained by the instrumental variables used in our study for the exposures was relatively small ( Supplementary Table S3 ). This limited the statistical power to detect associations with moderate effect sizes. Therefore, it is important for future research to consider larger sample sizes or the inclusion of additional instrumental variables to enhance the power and detect potential associations with greater precision. In addition, the study results primarily derive from cohorts of European individuals, necessitating caution in generalizing these findings to other ethnic populations. Future research endeavors should prioritize the inclusion of diverse ancestral cohorts to validate and extend the observed associations.
In conclusion, our study revealed a significant association between AD and a decreased risk of oral cavity cancer. These findings contribute to a better understanding of the relationship between AD and oral conditions. By shedding light on the connection between AD and oral cavity cancer, our findings offer valuable insights that can inform further research and clinical practices.
Data availability statement
The original contributions presented in the study are included in the article/ Supplementary material , further inquiries can be directed to the corresponding authors.
Ethics statement
Ethical review and approval was not required for the study on human participants in accordance with the local legislation and institutional requirements. Written informed consent from the patients/participants or patients/participants’ legal guardian/next of kin was not required to participate in this study in accordance with the national legislation and the institutional requirements.
Author contributions
JH: Conceptualization, Data curation, Formal analysis, Methodology, Writing – original draft. AD: Writing – review & editing. YB: Writing – review & editing. CL: Validation, Visualization, Writing – review & editing. HS: Project administration, Supervision, Writing – review & editing.
The author(s) declare financial support was received for the research, authorship, and/or publication of this article. This research was supported by the funding of the National Key Research and Development Program of China (grant no. 2021YFC2501200).
Conflict of interest
The authors declare that the research was conducted in the absence of any commercial or financial relationships that could be construed as a potential conflict of interest.
The author(s) declared that they were an editorial board member of Frontiers, at the time of submission. This had no impact on the peer review process and the final decision.
Publisher’s note
All claims expressed in this article are solely those of the authors and do not necessarily represent those of their affiliated organizations, or those of the publisher, the editors and the reviewers. Any product that may be evaluated in this article, or claim that may be made by its manufacturer, is not guaranteed or endorsed by the publisher.
Supplementary material
The Supplementary material for this article can be found online at: https://www.frontiersin.org/articles/10.3389/fneur.2024.1391625/full#supplementary-material
Abbreviations
AD, Alzheimer’s disease; SNP, Single nucleotide polymorphism; LD, Linkage disequilibrium; IVW, Inverse variance weighted; GWAS, Genome-wide association study; MR, Mendelian randomization.
1. Patel, J, Wallace, J, Doshi, M, Gadanya, M, Ben Yahya, I, Roseman, J, et al. Oral health for healthy ageing. Lancet Healthy Longev . (2021) 2:e521–7. doi: 10.1016/S2666-7568(21)00142-2
PubMed Abstract | Crossref Full Text | Google Scholar
2. van Dijk, BA, Brands, MT, Geurts, SM, Merkx, MA, and Roodenburg, JL. Trends in oral cavity cancer incidence, mortality, survival and treatment in the Netherlands. Int J Cancer . (2016) 139:574–83. doi: 10.1002/ijc.30107
3. Kassebaum, NJ, Bernabé, E, Dahiya, M, Bhandari, B, Murray, CJ, and Marcenes, W. Global burden of severe periodontitis in 1990–2010: a systematic review and meta-regression. J Dent Res . (2014) 93:1045–53. doi: 10.1177/0022034514552491
4. Kassebaum, NJ, Bernabé, E, Dahiya, M, Bhandari, B, Murray, CJL, and Marcenes, W. Global burden of untreated caries: a systematic review and metaregression. J Dent Res . (2015) 94:650–8. doi: 10.1177/0022034515573272
Crossref Full Text | Google Scholar
5. Shi, L, Wan, K, Tan, M, Yin, G, Ge, M, Rao, X, et al. Risk factors of recurrent aphthous ulceration among university students. Int J Clin Exp Med . (2015) 8:6218–23.
PubMed Abstract | Google Scholar
6. Mancini, M, Grappasonni, I, Scuri, S, and Amenta, F. Oral health in Alzheimer’s disease: a review. Curr Alzheimer Res . (2010) 7:368–73. doi: 10.2174/156720510791162359
7. Ribeiro, GR, Costa, JLR, Bovi Ambrosano, GM, and Rodrigues Garcia, RCM. Oral health of the elderly with Alzheimer’s disease. Oral Surg Oral Med Oral Pathol Oral Radiol . (2012) 114:338–43. doi: 10.1016/j.oooo.2012.03.028
8. Delwel, S, Binnekade, TT, Perez, RSGM, Hertogh, CMPM, Scherder, EJA, and Lobbezoo, F. Oral hygiene and oral health in older people with dementia: a comprehensive review with focus on oral soft tissues. Clin Oral Investig . (2018) 22:93–108. doi: 10.1007/s00784-017-2264-2
9. Beydoun, MA, Beydoun, HA, Hossain, S, El-Hajj, ZW, Weiss, J, and Zonderman, AB. Clinical and bacterial markers of periodontitis and their association with incident all-cause and Alzheimer’s disease dementia in a large national survey. J Alzheimers Dis . (2020) 75:157–72. doi: 10.3233/jad-200064
10. Dominy, SS, Lynch, C, Ermini, F, Benedyk, M, Marczyk, A, Konradi, A, et al. Porphyromonas gingivalis in Alzheimer’s disease brains: evidence for disease causation and treatment with small-molecule inhibitors. Sci Adv . (2019) 5:eaau3333. doi: 10.1126/sciadv.aau3333
11. Ospina-Romero, M, Glymour, MM, Hayes-Larson, E, Mayeda, ER, Graff, RE, Brenowitz, WD, et al. Association between Alzheimer disease and cancer with evaluation of study biases: a systematic review and meta-analysis. JAMA Netw Open . (2020) 3:e2025515. doi: 10.1001/jamanetworkopen.2020.25515
12. Lee, IH, Yu, H, Ha, SS, Yang, HG, and Kim, DK. Increased risk of Alzheimer’s disease in patients with head and neck cancer. Cancers . (2023) 15:15 (23). doi: 10.3390/cancers15235516
13. Bowles, EJA, Walker, RL, Anderson, ML, Dublin, S, Crane, PK, and Larson, EB. Risk of Alzheimer’s disease or dementia following a cancer diagnosis. PLoS One . (2017) 12:e0179857. doi: 10.1371/journal.pone.0179857
14. Teixeira, FB, Saito, MT, Matheus, FC, Prediger, RD, Yamada, ES, Maia, CSF, et al. Periodontitis and Alzheimer’s disease: a possible comorbidity between oral chronic inflammatory condition and neuroinflammation. Front Aging Neurosci . (2017) 9:327. doi: 10.3389/fnagi.2017.00327
15. Morris, MC, Evans, DA, Bienias, JL, Tangney, CC, Bennett, DA, Aggarwal, N, et al. Dietary fats and the risk of incident Alzheimer disease. Arch Neurol . (2003) 60:194–200. doi: 10.1001/archneur.60.2.194
16. Sanderson, E, Glymour, MM, Holmes, MV, Kang, H, Morrison, J, Munafò, MR, et al. Mendelian randomization. Nat Rev Methods Primers . (2022) 2:6. doi: 10.1038/s43586-021-00092-5
17. Li, C, Liu, J, Lin, J, and Shang, H. Covid-19 and risk of neurodegenerative disorders: a Mendelian randomization study. Transl Psychiatry . (2022) 12:283. doi: 10.1038/s41398-022-02052-3
18. Kunkle, BW, Grenier-Boley, B, Sims, R, Bis, JC, Damotte, V, Naj, AC, et al. Genetic meta-analysis of diagnosed Alzheimer’s disease identifies new risk loci and implicates Aβ, tau, immunity and lipid processing. Nat Genet . (2019) 51:414–30. doi: 10.1038/s41588-019-0358-2
19. Dudding, T, Haworth, S, Lind, PA, Sathirapongsasuti, JF, Tung, JY, Mitchell, R, et al. Genome wide analysis for mouth ulcers identifies associations at immune regulatory loci. Nat Commun . (2019) 10:1052. doi: 10.1038/s41467-019-08923-6
20. Sakaue, S, Kanai, M, Tanigawa, Y, Karjalainen, J, Kurki, M, Koshiba, S, et al. A cross-population atlas of genetic associations for 220 human phenotypes. Nat Genet . (2021) 53:1415–24. doi: 10.1038/s41588-021-00931-x
21. Lesseur, C, Diergaarde, B, Olshan, AF, Wünsch-Filho, V, Ness, AR, Liu, G, et al. Genome-wide association analyses identify new susceptibility loci for oral cavity and pharyngeal cancer. Nat Genet . (2016) 48:1544–50. doi: 10.1038/ng.3685
22. Ma, LL, Yu, JT, Wang, HF, Meng, XF, Tan, CC, Wang, C, et al. Association between cancer and Alzheimer’s disease: systematic review and meta-analysis. J Alzheimers Dis . (2014) 42:565–73. doi: 10.3233/jad-140168
23. Frain, L, Swanson, D, Cho, K, Gagnon, D, Lu, KP, Betensky, RA, et al. Association of cancer and Alzheimer’s disease risk in a national cohort of veterans. Alzheimers Dement . (2017) 13:1364–70. doi: 10.1016/j.jalz.2017.04.012
24. Nudelman, KNH, McDonald, BC, Lahiri, DK, and Saykin, AJ. Biological hallmarks of cancer in Alzheimer’s disease. Mol Neurobiol . (2019) 56:7173–87. doi: 10.1007/s12035-019-1591-5
25. Shafi, O. Inverse relationship between Alzheimer’s disease and cancer, and other factors contributing to Alzheimer’s disease: a systematic review. BMC Neurol . (2016) 16:236. doi: 10.1186/s12883-016-0765-2
26. Lanni, C, Masi, M, Racchi, M, and Govoni, S. Cancer and Alzheimer’s disease inverse relationship: an age-associated diverging derailment of shared pathways. Mol Psychiatry . (2021) 26:280–95. doi: 10.1038/s41380-020-0760-2
27. Penn, IW, Chung, CH, Huang, YC, Chen, MC, Sun, CA, Yip, PK, et al. Increased risk of dementia in patients with nasopharyngeal cancer treated with radiation therapy: a nationwide population-based cohort study. Arch Gerontol Geriatr . (2021) 93:104303. doi: 10.1016/j.archger.2020.104303
28. Leng, F, and Edison, P. Neuroinflammation and microglial activation in Alzheimer disease: where do we go from here? Nat Rev Neurol . (2021) 17:157–72. doi: 10.1038/s41582-020-00435-y
29. Noble, JM, Borrell, LN, Papapanou, PN, Elkind, MSV, Scarmeas, N, and Wright, CB. Periodontitis is associated with cognitive impairment among older adults: analysis of NHANES-III. J Neurol Neurosurg Psychiatry . (2009) 80:1206–11. doi: 10.1136/jnnp.2009.174029
30. Syrjälä, AM, Ylöstalo, P, Sulkava, R, and Knuuttila, M. Relationship between cognitive impairment and oral health: results of the health 2000 health examination survey in Finland. Acta Odontol Scand . (2007) 65:103–8. doi: 10.1080/00016350601083521
Keywords: Alzheimer’s disease, oral conditions, oral cavity cancer, Mendelian randomization, causation analysis
Citation: Huang J, Deng A, Bai Y, Li C and Shang H (2024) Alzheimer’s disease and oral manifestations: a bi-directional Mendelian randomization study. Front. Neurol . 15:1391625. doi: 10.3389/fneur.2024.1391625
Received: 26 February 2024; Accepted: 03 May 2024; Published: 15 May 2024.
Reviewed by:
Copyright © 2024 Huang, Deng, Bai, Li and Shang. This is an open-access article distributed under the terms of the Creative Commons Attribution License (CC BY) . The use, distribution or reproduction in other forums is permitted, provided the original author(s) and the copyright owner(s) are credited and that the original publication in this journal is cited, in accordance with accepted academic practice. No use, distribution or reproduction is permitted which does not comply with these terms.
*Correspondence: Chunyu Li, [email protected] ; Huifang Shang, [email protected]
† These authors have contributed equally to this work
Disclaimer: All claims expressed in this article are solely those of the authors and do not necessarily represent those of their affiliated organizations, or those of the publisher, the editors and the reviewers. Any product that may be evaluated in this article or claim that may be made by its manufacturer is not guaranteed or endorsed by the publisher.
Thank you for visiting nature.com. You are using a browser version with limited support for CSS. To obtain the best experience, we recommend you use a more up to date browser (or turn off compatibility mode in Internet Explorer). In the meantime, to ensure continued support, we are displaying the site without styles and JavaScript.
- View all journals
- My Account Login
- Explore content
- About the journal
- Publish with us
- Sign up for alerts
- Open access
- Published: 13 May 2024
Structural anisotropy results in mechano-directional transport of proteins across nuclear pores
- Fani Panagaki 1 , 2 na1 ,
- Rafael Tapia-Rojo ORCID: orcid.org/0000-0002-9460-2859 1 , 2 na1 ,
- Tong Zhu ORCID: orcid.org/0000-0002-1804-2124 1 , 2 ,
- Natalie Milmoe 1 , 2 ,
- Patricia Paracuellos 1 , 2 ,
- Stephanie Board ORCID: orcid.org/0000-0002-1189-8173 1 , 2 ,
- Marc Mora ORCID: orcid.org/0000-0002-8130-2742 1 , 2 ,
- Jane Walker 1 , 2 ,
- Elena Rostkova 2 ,
- Andrew Stannard ORCID: orcid.org/0000-0001-6283-667X 2 ,
- Elvira Infante ORCID: orcid.org/0000-0002-9883-8391 1 , 2 &
- Sergi Garcia-Manyes ORCID: orcid.org/0000-0001-5140-2606 1 , 2
Nature Physics ( 2024 ) Cite this article
374 Accesses
76 Altmetric
Metrics details
- Biological physics
- Single-molecule biophysics
The nuclear pore complex regulates nucleocytoplasmic transport by means of a tightly synchronized suite of biochemical reactions. The physicochemical properties of the translocating cargos are emerging as master regulators of their shuttling dynamics. As well as being affected by molecular weight and surface-exposed amino acids, the kinetics of the nuclear translocation of protein cargos also depend on their nanomechanical properties, yet the mechanisms underpinning the mechanoselectivity of the nuclear pore complex are unclear. Here we show that proteins with locally soft regions in the vicinity of the nuclear-localization sequence exhibit higher nuclear-import rates, and that such mechanoselectivity is specifically impaired upon knocking down nucleoporin 153, a key protein in the nuclear pore complex. This allows us to design a short, easy-to-express and chemically inert unstructured peptide tag that accelerates the nuclear-import rate of stiff protein cargos. We also show that U2OS osteosarcoma cells expressing the peptide-tagged myocardin-related transcription factor import this mechanosensitive protein to the nucleus at higher rates and display faster motility. Locally unstructured regions lower the free-energy barrier of protein translocation and might offer a control mechanism for nuclear mechanotransduction.
Similar content being viewed by others
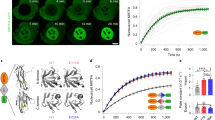
The mechanical stability of proteins regulates their translocation rate into the cell nucleus
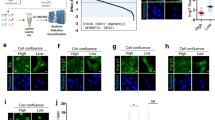
Mechanical control of nuclear import by Importin-7 is regulated by its dominant cargo YAP
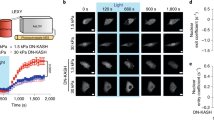
Mechanical force application to the nucleus regulates nucleocytoplasmic transport
During mechanotransduction, extracellular mechanical forces need to propagate across the cell to eventually reach the nucleus to activate force-induced transcriptional programs 1 , 2 , 3 , 4 . Several mechanosensitive transcriptional regulators, such as the myocardin-related transcription factor A (MRTFA) 5 , yes-associated protein 1 (YAP) 6 and nuclear factor kappa-light-chain-enhancer of activated B cells (NF-κB) 7 , which are in the cytoplasm under basal conditions, shuttle into the nucleus upon mechanical stimulation to initiate gene expression. Studding the nuclear envelope, the nuclear pore complex (NPC)—a 100-MDa protein complex formed by ~30 nucleoporin proteins (Nups)—is the main gateway in and out of the nucleus, and it orchestrates the continuous bidirectional nucleocytoplasmic traffic of RNA and proteins 8 , 9 , 10 .
Compared to other narrower biologically relevant pores, such as those found in mitochondria 11 , peroxisomes 12 or the proteolytic machinery in bacteria 13 , 14 , the width of the central pore of the NPC is seemingly large ( ∼ 40–70 nm) 15 , 16 , 17 , 18 , and yet it is incredibly permselective 19 , mainly due to an ensemble of intrinsically disordered and extremely dynamic Nups rich in phenylalanine and glycine (FG) repeats that line the inner surface of the pore and form an effective barrier to translocation 20 . In the canonical picture, small molecules (up to ∼ 5 nm ( ∼ 40 kDa), although there is a soft cutoff threshold 21 ) can diffuse through the NPC freely, whereas larger molecules, including ostensibly large cargos 22 , 23 , 24 , need to bind to nuclear transport receptors (NTRs) 23 , 25 , 26 . Briefly, in the importin-dependent ‘facilitated’ transport pathway, importin-α (Kapα) binds cargos containing a nuclear localization signal (NLS) sequence and importin-β (Kapβ), forming a trimeric complex in the cytoplasm. Conversely, in the nucleus, exportin binds to proteins featuring a nuclear export signal (NES). The reversibility and directionality of the process is fuelled by the nucleocytoplasmic Ran guanosine triphosphate gradient 27 . Although recent advances in cryo-electron microscopy (cryo-EM) 18 , 28 , 29 , 30 , 31 have provided a refined molecular picture of the structural components delineating the NPC 28 , a unified view on the molecular mechanisms underpinning the dynamics of nucleocytoplasmic transport across the NPC is still far from complete 32 .
Several enticing and complementary physicochemical models have been proposed to explain the highly sophisticated pore selectivity based on the interactions within the FG-Nups, as well as between the FG-Nups and the translocating protein complex 33 , 34 . From the cargo perspective, both molecular weight (larger proteins take longer to translocate, although a systematic analysis of the mass-dependency of nuclear translocation dynamics in facilitated transport is missing) 21 , 25 and the chemical composition of the exposed protein amino acids (hydrophobic residues exhibit a more favourable interaction with the surrounding FG-Nups, favouring translocation) have been mainly described as the key determinants of a protein’s passage rate across the NPC 35 . Experiments investigating this have highlighted, even in facilitated transport, and in addition to the NLS/NTR system, the much unappreciated role of the properties of the carried cargo in regulating nuclear translocation.
More recently, mechanical forces have been shown to regulate the dynamics of nuclear transport at the NPC at two different and independent levels 36 . First, external mechanical perturbations directly applied to the nucleus 37 , or internally applied from the cytoplasm by the LINC (linker of nucleoskeleton and cytoskeleton) complex, were able to elastically deform the NPC 38 , thereby increasing the nuclear import of the YAP transcription factor. Independently, a second (and orthogonal) new mechanism specifically related to the properties of the translocating cargo suggests that the mechanical stability of proteins regulates their nuclear import rate 39 . Yet the molecular mechanisms by which the NPC regulates the translocation of proteins by sensing their nanomechanical stability—and the functional consequences at the cellular level—remain unknown. How protein mechanics is linked to other relevant cargo physicochemical properties (namely mass and sequence) to collectively regulate their nuclear transport remains poorly understood. Given that transport is directional and that the mechanical resistance of proteins is a localized notion 40 , 41 , the challenge lies in establishing how a protein’s local mechanical stability regulates its nuclear import dynamics. Unravelling the physical rules underpinning the NPC mechanoselectivity jigsaw would potentially inspire the rational design of proteins with enhanced nuclear affinity, offering new molecular strategies towards the engineering of nuclear mechanotransduction.
We started by probing how the dynamics of nuclear entry are affected by the mechanical stability of an individual translocating protein monomer. We transiently transfected U2OS cells with a light-inducible nuclear export (LEXY) probe (AsLOV2-NES) 42 (Fig. 1a ), previously modified to contain a specific protein (X) mechanical marker, NLS-X-mCherry-LEXY 39 . As a first choice, we used X = Ig27 WT (titin’s 27th immunoglobulin (Ig) domain, a well-studied mechanical protein model that has been independently characterized by single-molecule atomic force microscopy (AFM) 43 ) and its two mutants, Ig27 V13P and Ig27 Y9P (ref. 40 ). The introduction of a single-point (V13P, Y9P) mutation to the Ig27 WT results in a substantial change in its mechanical stability while keeping its fold and sequence (and hence the exposed amino acids) intact (Fig. 1b ). Briefly, in the dark state, the NES of the LEXY probe is docked to the light-oxygen-voltage-sensing (LOV) domain, rendering it invisible to exportins. As a result, the construct mostly accumulates in the nucleus. Upon blue-light exposure, the NES motif is rapidly exposed, resulting in fast exclusion of the optogenetic probe from the nucleus. Switching off the light again re-docks the NES and triggers the construct to exponentially accumulate back into the nucleus (Fig. 1a ). Comparing the nuclear translocation kinetics (Supplementary Fig. 1 ) of the optogenetic tool tagged with the three Ig27 variants in the absence of light revealed that the import (but not the export, Supplementary Fig. 2 ) rate of the mechanically stable Ig27 Y9P mutant was significantly lower than that corresponding to the Ig27 WT and the mechanically labile Ig27 V13P form (Fig. 1c,d ). Altogether, these proof-of-principle experiments suggest that the rate of nuclear entry is inversely correlated with the mechanical stability of the translocating protein, in agreement with previous experiments 39 . This implies that, at least at the monomer level, the (at least partial) mechanical unfolding of Ig27 regulates its nuclear translocation rate.
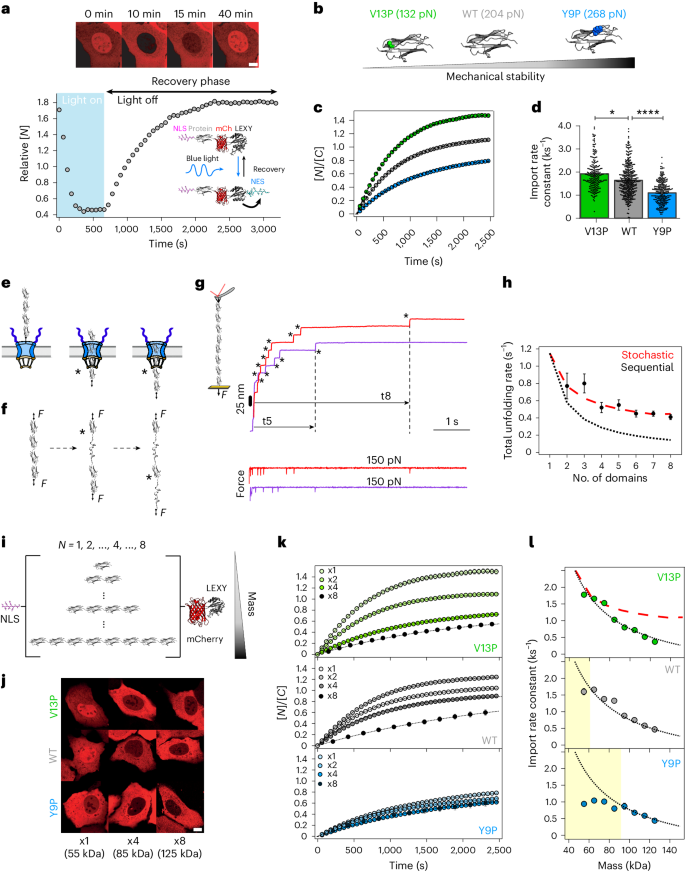
a , Optogenetics assay to measure the time course of the nucleus-to-cell localization of the NLS-X-mCherry-LEXY (here X = Ig27 WT ) probe, [ N ]( t ). Representative confocal images are shown at the top. Scale bar, 10 µm. b – d , Nuclear import kinetics of Ig27 WT (WT), Ig27 V13P (V13P) and Ig27 Y9P (Y9P): unfolding forces of each Ig27 variant obtained by AFM experiments 40 ( b ); average time courses of the nucleus/cytoplasm ratio, [ N ]/[ C ]( t ), for V13P, WT and Y9P ( c ); resulting import rate constants ( k I ) for WT ( k I = 1.62 ± 0.05 ks −1 , n = 249), V13P ( k I = 1.80 ± 0.09 ks −1 , n = 161) and Y9P ( k I = 1.09 ± 0.03 ks −1 , n = 279) ( d ). Significance levels (two-tailed Mann–Whitney non-parametric test): * P < 0.05; **** P < 0.0001. WT versus V13P, P = 0.04; WT versus Y9P, P = 6.60 × 10 −16 . e , Schematics of a polyprotein construct made of multiple identical protein domains as it sequentially translocates to the nucleus across the NPC, one domain at a time (black asterisk). f , By contrast, in an in vitro single polyprotein pulling experiment, in which each domain unfolds stochastically (black asterisks) with the same probability per unit time ( r U d t ), where r U is the characteristic unfolding rate of each individual domain. g , Force-clamp AFM unfolding trajectory of an individual (Ig27 WT ) 8 polyprotein stretched at 150 pN. The total unfolding time t N depends on N , the number of unfolding domains ( N = 5, purple trace; N = 8, red trace). h , Total unfolding rate r N of an Ig27 WT polyprotein as a function of N , calculated as r N = 1/ t N . The resulting unfolding kinetics can be reproduced by a stochastic model where r N = r U /Σ(1/ n ), with n = 1, …, N (red dashed line) and with r U = 1.15 s −1 . For comparison, a sequential unfolding process would exhibit a steeper dependence on N (black dotted line , r N = r U / N ). i , Schematics of the LEXY probe modified to include a varying number of Ig27 domains ( N = 1–8). j , Representative confocal images of U2OS cells expressing the LEXY construct with protein cargos of different mechanical stabilities (V13P, WT and Y9P) and molecular weights ( N = 1, 4 and 8 are shown), taken 30 min into the recovery phase. Scale bar, 10 µm. k , l , Average recovery curves of V13P (top), WT (middle) and Y9P (bottom) polyproteins ( k ) and the associated import rate constants ( l ) for N = 1–8. All points and bar plots indicate mean ± s.e.m.
To elucidate the mechanism by which structurally complex and larger protein cargos translocate into the nucleus across the NPC, we employed (homo)polyproteins made of identical repeats of the same protein monomer. It is tempting to speculate that, when entering the pore, a polyprotein made of several independent protein monomers will translocate one monomer at a time (sequential translocation) as they find the pore mouth (Fig. 1e ). This scenario is met in the ClpX bacterial proteolytic machine 14 , 44 , where individual mechanically stable domains independently unfold as they encounter the proteolytic pore, as shown by single-molecule techniques. For such a sequential translocation scenario, the total translocation rate of the entire polyprotein ( r N ) scales with the number of domains ( N ) as \({{r}_{{\mathrm{N}}}\left(N\right)}={\frac{{r}_{\rm{U}}}{N}}\) , where r U is the translocation rate of each monomer. However, given that the NPC is seemingly deep ( ∼ 40 nm) 45 , it is also possible that several domains enter the pore at once, thus being concomitantly exposed to mechanical force. This second scenario would be closer to that experienced by individual polyproteins when stretched in vitro using single-molecule techniques 46 (Fig. 1f ). In this situation, given that the force is rapidly propagated through the entire polyprotein backbone, all domains are equally exposed to force and hence their unfolding probability at a given time is identical, regardless of their position within the polyprotein chain (stochastic unfolding) 47 . Those two distinct unfolding scenarios can be singled out according to their associated dependency of the total unfolding rate r N with the number of domains ( N ). To experimentally evaluate the dependency of the total unfolding rate as a function of N in this ‘stochastic unfolding’ framework, we first studied the in vitro unfolding dynamics of an individual polyprotein formed by eight identical repeats, (Ig27 WT ) 8 , when stretched at a constant force of 150 pN by a single-molecule AFM operating in force-clamp mode (Fig. 1g ). A single unfolding trajectory resembles a staircase, where each individual step corresponds to the unfolding of an individual Ig27 WT domain within the polyprotein chain 48 . In those experiments, the protein is typically randomly picked up from the surface by the AFM tip from different positions within the chain 49 , resulting in unfolding trajectories of (up to) the full length ( N = 8 steps). We sorted individual trajectories with the same N (ensuring a long detachment time to avoid biasing the overall unfolding rate distribution 50 ), and we computed, in each case, the total unfolding rate (Fig. 1h ). The resulting unfolding rate follows a shallow dependency with N , namely \({r}_{\rm{N}}{(N\;)}={\frac{{r}_{\rm{U}}}{\mathop{\sum }\nolimits_{{\rm{n=1}}}^{N}\frac{1}{n}}}\) (ref. 47 ), compatible with a stochastic model where each domain unfolds independently (segmented red line).
To examine the mechanism of nuclear translocation of Ig27 polyproteins in U2OS cells, we used the optogenetic approach to systematically study the rate of nuclear translocation of Ig27 WT , Ig27 V13P and Ig27 Y9P polyproteins of increasing mass (Ig27) N , with 1 ≤ N ≤ 8 (Fig. 1i ). For each polyprotein length, we measured the dynamics of nuclear accumulation (Fig. 1j ) and calculated the associated nuclear import rate (Fig. 1k,l and Extended Data Figs. 1 – 3 ). In the case of Ig27 V13P , we observed a strong mass dependency that could be nicely reproduced by an ∼ (1/ N ) scaling relationship (mass law) across all probed protein lengths (1 ≤ N ≤ 8), reminiscent of a sequential import mechanism. An almost identical dependency was measured for the Ig27 WT polyproteins. However, in this case, the monomer data point ( ∼ 55 kDa) fell substantially below its expected mass law. This behaviour was exacerbated with the mechanically stronger I27 Y9P polyproteins, which showed a very significant slowing down of the rate of nuclear entry and an almost flat dependency for molecular weights below ∼ 95 kDa ( N = 5, pentamer). These experiments suggest that, for proteins of low mechanical stability (Ig27 V13P ), the sequential model for import applies for all polyprotein lengths (molecular weights) probed. This hypothesis was further corroborated for the mechanically labile spectrin R16 polyproteins 51 , which exhibit their own mass law, and also follow the ∼ (1/ N ) scaling across all polyprotein lengths (R16) N (1 ≤ N ≤ 8) (Supplementary Fig. 3 ). However, as the protein’s mechanical stability increases, a deviation from the protein’s mass law emerges at low molecular weights, globally suggesting a mass threshold value—which depends on the mechanical stability of the protein— below which the rate of nuclear entry is dominated by the mechanical stability of the protein, and beyond which it is uniquely modulated by mass. A deep implication of these data is that the mechanical stability of the first protein region (or first protein domain(s)) entering the pore mouth (leading protein) seems to be the bottleneck for import dynamics.
This naturally led us to systematically examine the role of the leading protein (in our case, the protein next to the NLS sequence in the LEXY optogenetic construct) in determining the overall protein import rate. We started by comparing the nuclear shuttling dynamics of a simple heterodimer composed of two domains with markedly different mechanical stabilities, R16-Ig27 WT (Fig. 2a ), and compared this to that of its specular image, Ig27 WT -R16 (Fig. 2b ). From the mechanical perspective, both proteins were indistinguishable when pulled by single-molecule magnetic tweezers (ideally suited to measure proteins with low mechanical stability 52 , 53 ) (Fig. 2c ). In both cases, the force-ramp unfolding trajectories showed first the unfolding of the mechanically labile R16 domain at low forces ( ∼ 30 pN, 21 nm step), followed by unfolding of the mechanically stable Ig27 WT at much higher forces ( ∼ 100 pN, 25 nm step). This was expected, because the mechanical unfolding of polyproteins composed of mechanically distinct monomers follows a hierarchy in their mechanical stability, implying that soft domains unfold first, irrespective of their sequence position within the polyprotein chain 46 , 54 . In addition to being mechanically analogous, both R16-Ig27 WT and Ig27 WT -R16 proteins have the same overall structure (and consequently the same exposed amino acids 35 ) and exactly the same mass, so one should a priori expect them to exhibit the same nuclear import rate.
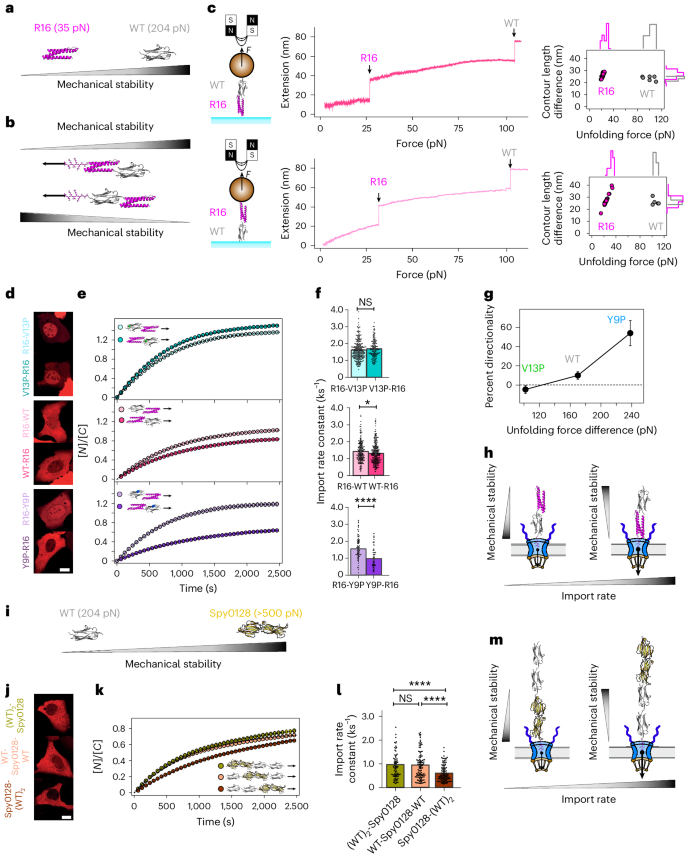
a , Unfolding forces (AFM force–extension, v = 400 nm s −1 ) 51 of the soft spectrin R16 domain and stiff titin Ig27 WT (WT). b , Heterodimer protein constructs alternatively placing the R16 or WT at the N-terminal position (after the NLS). c , Magnetic tweezers pulling experiments comparing the unfolding dynamics R16-WT (top) and WT-R16 (bottom) protein constructs. d – f , Nuclear import kinetics of heterodimers composed of the labile R16 domain, and an Ig27 variant (V13P, WT, Y9P) alternatively placed at the N- or C- terminus, to test the effect of protein mechano-directionality on the nuclear translocation kinetics. d , Representative confocal images of U2OS cells after 30 min into the recovery phase. Scale bar, 10 µm. e , Average time courses of the nucleus-to-cytoplasm localization of the respective protein construct. f , Import rates calculated from fits to the recovery time courses. R16-V13P ( k I = 1.64 ± 0.04 ks −1 , n = 276); V13P-R16 ( k I = 1.72 ± 0.04 ks −1 , n = 154); R16-WT ( k I = 1.42 ± 0.04 ks −1 , n = 192); WT-R16 ( k I = 1.29 ± 0.04 ks −1 , n = 260); R16-Y9P ( k I = 1.58 ± 0.07 ks −1 , n = 80); Y9P-R16 ( k I = 1.02 ± 0.08 ks −1 , n = 55). Significance levels for two-tailed the Mann–Whitney non-parametric test: NS , P > 0.05; * P < 0.05; ** P < 0.01; **** P < 0.0001. R16-V13P versus V13P-R16, P = 0.06; R16-WT versus WT-R16, P = 0.02; R16-Y9P versus Y9P-R16, P = 1.67 × 10 −6 . g , Relative directionality (calculated as the ratio between the import rates of the constructs with N-terminal R16 and C-terminal R16) compared to the difference in mechanical stability between each Ig27 variant and R16. h , Schematics highlighting the sensitivity of the NPC to the mechano-directionality of the translocating cargo. i , Unfolding forces (AFM force–extension, v = 400 nm s −1 ) 55 measured for the titin Ig27 WT (WT) and the pilin Spy0128 domain. Spy0128 is mechanically ultrastable; hence, WT plays in this case the role of the mechanically labile protein. j – l , Nuclear import kinetics of heteropolyproteins composed of two WT and one Spy0128 protein dimer. j , Representative confocal images of U2OS cells 30 min into the recovery phase. Scale bar, 10 µm. k , l , Average time courses of nucleus-to-cytoplasm localization ( k ) and associated import rates ( l ). (WT) 2 -Spy0128 ( k I = 0.89 ± 0.07 ks −1 , n = 70); WT-Spy01 2 8-WT ( k I = 0.99 ± 0.07 ks −1 , n = 118); Spy0128-(WT) 2 ( k I = 0.56 ± 0.03 ks −1 , n = 106). Significance levels for two-tailed Mann–Whitney non-parametric test: NS , P > 0.05; **** P < 0.0001. (WT) 2 -Spy0128 versus WT-Spy0128-WT, P = 0.80; WT-Spy0128-WT versus Spy0128-(WT) 2 , P = 5.70 × 10 −5 ; (WT) 2 -Spy0128 versus Spy0128-(WT) 2 , P = 4.45 × 10 −5 . m , Schematics of the dynamics of nuclear translocation of Spy0128-(WT) 2 versus (WT) 2 -Spy0128, highlighting the sensitivity of the NPC to the mechano-directionality of the shuttling protein cargo. All points and bar plots indicate mean ± s.e.m.
However, we observed, using the nuclear optogenetic approach (Fig. 2d–f ), that the construct starting with the mechanically soft R16 domain exhibited a significantly faster import rate and higher nuclear accumulation than the one starting with the mechanically rigid Ig27 WT domain. This was the case across the three Ig27 variants. Interestingly, for the constructs containing the mechanically rigid Ig27 Y9P mutant protein, the increase in nuclear accumulation of the R16-Ig27 Y9P as opposed to the reverse Ig27 Y9P -R16 construct was particularly enhanced (Fig. 2g,h ). The same result was independently observed in HeLa and NIH 3T3 cell lines (Supplementary Fig. 4 ).
These experiments suggest that the NPC senses the mechano-directionality of the translocating protein cargo, in the sense that those proteins exhibiting a mechanically soft region next to the NLS tag exhibit a faster and enhanced accumulation, and that this effect is more obvious for those constructs where the gap in the mechanical stability between the ‘soft’ and ‘stiff’ regions is larger. This behaviour was maintained for larger protein constructs such as (R16) 2 -(Ig27 WT ) versus (Ig27 WT )-(R16) 2 , (R16) 2 -(Ig27 WT ) 2 versus (Ig27 WT ) 2 -(R16) 2 , and (Ig27 WT ) 2 -(R16) 2 versus (Ig27 WT -R16) 2 , which, although they have the same mechanical stability (as measured with single-molecule AFM; Extended Data Fig. 4 ), they display markedly different nuclear import kinetics (Extended Data Fig. 5 and Supplementary Figs. 5 and 6 ) when the mechanically soft domain (R16) is next to the NLS in the LEXY probe. To explore the range of cargo mechanical stabilities for which the NPC exhibits mechano-directionality, we tested the effect of sequence (and mechanical) asymmetry on the dynamics of nuclear entry for the constructs (Spy0128)-(Ig27 WT ) 2 , (Ig27 WT ) 2 -Spy0128 and Ig27 WT -Spy0128-Ig27 WT , where Spy0128 is a two-domain pilin protein of Streptococcus pyogenes that exhibits ultra-high mechanical stability due to a covalent isopeptide bond close to the protein termini that renders the protein inextensible (Fig. 2i ) 55 . Note that, in these constructs, the Ig27 WT now acts as the soft protein, and the Spy0128 protein is the stiffer one. As before, despite their mass and overall structural identity, the (Ig27 WT ) 2 -Spy0128 construct exhibits a faster nuclear rate import than Spy0128-(Ig27 WT ) 2 (Fig. 2j–l ). Interestingly, the Ig27 WT -Spy0128-Ig27 WT construct (with only one Ig27 WT leading) displays a nuclear import rate very close to (Ig27 WT ) 2 -Spy0128 and much faster than Spy0128-(Ig27 WT ) 2 , again suggesting that although folded, inextensible proteins such as Spy0128 can translocate across the NPC, softer leading proteins increase their nuclear import kinetics, being the main determinant of the overall import kinetics of the whole protein construct (Fig. 2m ). This demonstrates that the NPC mechano-directionality is manifested for a wide range of protein mechanical stabilities.
In light of these findings, we wondered whether the addition of a soft protein region in front of a mechanically rigid one could be exploited as a strategy to accelerate the translocation of mechanically resilient proteins inside the cell nucleus. We hence compared (Fig. 3a–c ) the rate of nuclear entry of each Ig27 mutant (Ig27 V13P , Ig27 WT and Ig27 Y9P ) with the related construct resulting from the addition of the mechanically labile R16 protein in front (that is, R16-Ig27 V13P , R16-Ig27 WT and R16-Ig27 Y9P ). Consequently, in all constructs, the mass of the cargo was substantially increased (more than doubled) with respect to that of the Ig27 monomer alone. For both Ig27 V13P and Ig27 WT proteins, addition of the R16 did not significantly slow down their nuclear translocation dynamics in spite of their mass increase. Remarkably, in the case of the Ig27 Y9P , addition of the R16 protein not only did not slow down its nuclear import rate, it significantly accelerated it (Fig. 3d ). In all three cases, addition of the mechanically soft R16 domain compensated the mechanical stability penalty, bringing the import rate of the three constructs to their expected position in their respective mass-law relationship (Fig. 3e ). In summary, addition of the mechanically soft R16 in front of mechanically stiffer monomers has a net acceleration effect on those mechanically stiff proteins (Fig. 3f ).
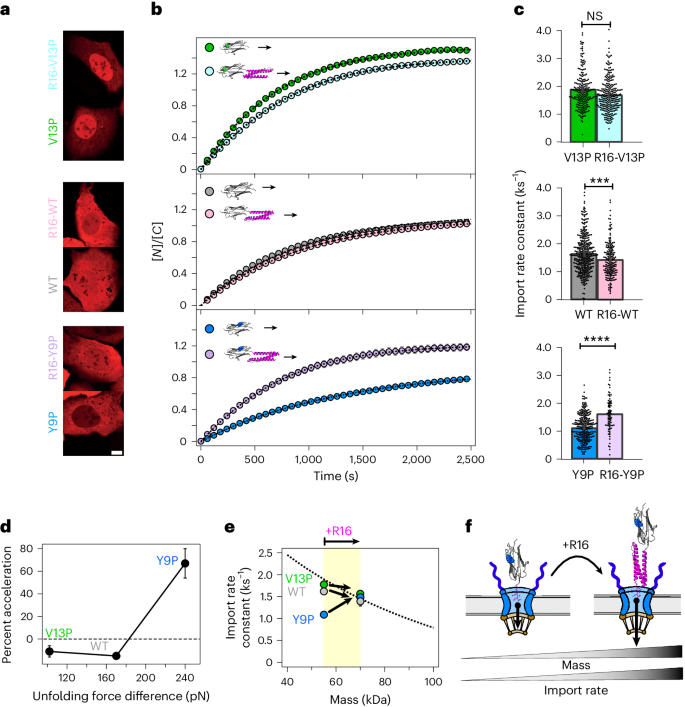
a – c , Nuclear import kinetics of R16-(V13P/WT/Y9P) compared to their Ig27 respective monomer variant: representative confocal images of U2OS cells after 30 min into the recovery phase (scale bar, 10 µm) ( a ); average time courses of the relative nucleus-to-cytoplasm localization of each protein construct ( b ); related import rates for V13P ( k I = 1.80 ± 0.09 ks −1 , n = 161), R16-V13P ( k I = 1.64 ± 0.04 ks −1 , n = 276); WT ( k I = 1.62 ± 0.05 ks −1 , n = 249), R16-WT ( k I = 1.42 ± 0.04 ks −1 , n = 192); Y9P ( k I = 1.09 ± 0.03 ks −1 , n = 279), R16-Y9P ( k I = 1.58 ± 0.07 ks −1 , n = 80) ( c ). Significance levels for two-tailed Mann–Whitney non-parametric test: NS, P > 0.05; ** P < 0.001; **** P < 0.0001. V13P versus R16-V13P, P = 0.10; WT versus R16-WT, P = 3.84 × 10 −3 ; Y9P versus R16-Y9P, P = 3.70 × 10 −10 . d , Relative acceleration resulting from the addition of an N-terminal R16 domain as a function of the difference in mechanical stability between R16 and the Ig27 variant (V13P, WT or Y9P). e , Import kinetics of the V13P, WT and Y9P monomers (55 kDa) versus the construct with an N-terminal R16 domain (70 kDa). In all cases, the import kinetics are brought up to the rate set by their respective mass law. In the case of mechanically stiff proteins (Y9P), this results in a net acceleration of the import kinetics relative to the monomer alone. f , Schematics of the dynamics of nuclear translocation of a Y9P monomer (left) versus the R16-Y9P construct (right). Adding the soft R16 domain next to the NLS accelerates the nuclear passage of mechanically stiff cargos, despite increasing their mass. All points and bar plots indicate mean ± s.e.m.
Given the markedly low mechanical stability of the R16 monomer, we then conjectured that the addition of shorter polypeptides with similarly low mechanical stability (mechanically labile tags) could potentially play the same role as R16, with the advantages of featuring a much lower molecular weight and, most importantly, being easy to introduce to essentially any target protein 56 , 57 . As a first candidate, we chose the GS-based tag given that it is classically used in protein engineering to create flexible loops due to its low propensity to form residual structures and that its chemical composition (glycines and serines) is not expected to substantially enhance or delay nuclear import 35 . We introduced GS-tags of different lengths, ranging from (GS) to (GS) 25 , in the N-terminus of the Ig27 WT protein (Fig. 4a ), and compared the nuclear accumulation (Fig. 4b ) and associated nuclear import rate (Fig. 4c ) of each resulting construct to that of the Ig27 WT monomer. We first found that introducing the shortest (GS) tag did not have a significant effect, whereas the longer (GS) 25 significantly slowed the rate of nuclear translocation, probably due to its increased mass. By contrast, we observed that those constructs composed of GS sequences of intermediate lengths—especially (GS) 2 and (GS) 4 —were able to significantly accelerate the nuclear translocation of Ig27 WT (Fig. 4d ).
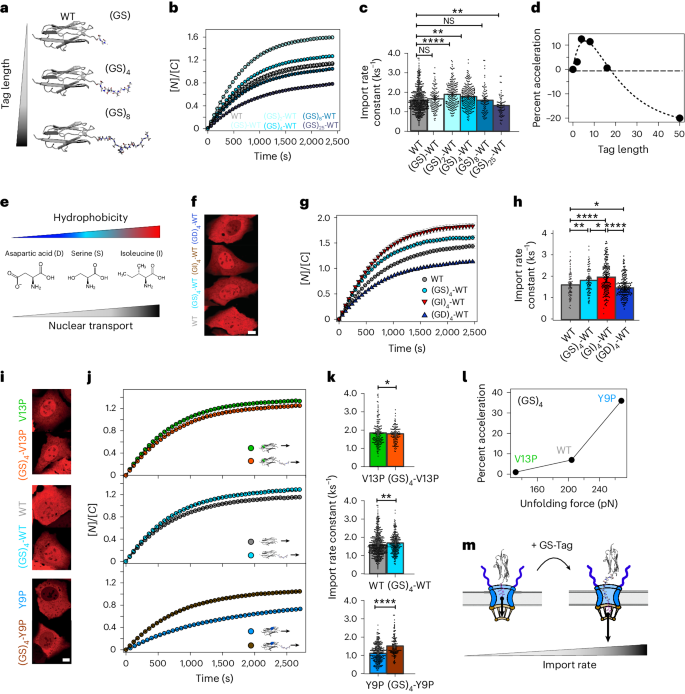
a , Addition of an N-terminal unstructured and flexible glycine–serine peptide tag to accelerate nuclear import. b , c , Average time courses of the nucleus-to-cytoplasm mCherry localization of LEXY constructs containing a (GS) X -WT protein cargo, ( X = 1, 2, 4, 8, 25) ( b ) and their associated import rates ( c ): WT ( k I = 1.64 ± 0.05 ks −1 , n = 249), (GS)-WT ( k I = 1.65 ± 0.07 ks −1 , n = 131), (GS) 2 -WT ( k I = 1.88 ± 0.04 ks −1 , n = 131), (GS) 4 -WT ( k I = 1.82 ± 0.03 ks −1 , n = 357), (GS) 8 -WT ( k I = 1.61 ± 0.07 ks −1 , n = 89), (GS) 25 -WT ( k I = 1.29 ± 0.06 ks −1 , n = 70). Significance levels for the two-tailed Mann–Whitney non-parametric test: NS, P > 0.05; ** P < 0.001; **** P < 0.0001. WT versus (GS)-WT, P = 0.44; WT versus (GS) 2 -WT, P = 1.67 × 10 −10 ; WT versus (GS) 4 -WT, P = 2.46 × 10 −3 ; WT versus (GS) 8 -WT, P = 0.94; WT versus (GS)25-WT, P = 1.91 × 10 −3 . d , Relative acceleration of the WT monomer as a function of the GS-tag length (number of amino acids). The WT is optimally accelerated when adding a flexible peptide of four to eight amino acids. e , The chemical properties of translocating amino acids regulate transport kinetics through the NPC, whereas charged residues like aspartic acid (D) impede NPC passage, and highly hydrophobic ones like isoleucine (I) facilitate nuclear import. f – h , Nuclear import kinetics of WT, (GS) 4 -(WT), (GI) 4 -(WT) and (GD) 4 -(WT). f , Representative confocal images of U2OS cells after 30 min into the recovery phase. Scale bar, 10 µm. g , Average time courses of the relative nucleus-to-cytoplasm localization of the respective protein construct. h , Associated import rates corresponding to WT ( k I = 1.60 + −0.06 ks −1 , n = 80), (GS) 4 -WT ( k I = 1.82 ± 0.07 ks −1 , n = 357), (GI) 4 -WT ( k I = 1.97 ± 0.06 ks −1 , n = 87), (GD) 4 -WT ( k I = 1.45 ± 0.04 ks −1 , n = 98). Significance levels for the two-tailed Mann–Whitney non-parametric test: * P < 0.05; ** P < 0.001; **** P < 0.0001. WT versus (GS) 4 -WT, P = 2.46 × 10 −3 ; WT versus (GI) 4 -WT, P = 1.11 × 10 −6 ; WT versus (GD) 4 -WT, P = 0.02; (GS) 4 -WT versus (GI) 4 -WT, P = 0.04; (GI) 4 -WT versus (GD) 4 -WT, P = 2.74 × 10 −11 . i – k , Nuclear import kinetics of (GS) 4 -(V13P/WT/Y9P) compared to the respective monomers. i , Representative confocal images of U2OS cells after 30 min into the recovery phase. Scale bar, 10 µm. j , Time courses of the relative nucleus-to-cytoplasm localization of the respective protein construct. k , Related import rates: (GS) 4 -V13P ( k I = 1.90 ± 0.05 ks −1 , n = 127), V13P ( k I = 1.87 ± 0.08 ks −1 , n = 170); (GS) 4 -WT ( k I = 1.82 ± 0.07 ks −1 , n = 357), WT ( k I = 1.62 ± 0.05 ks −1 , n = 249); (GS) 4 -Y9P ( k I = 1.48 ± 0.05 ks −1 , n = 132), Y9P ( k I = 1.09 ± 0.03 ks −1 , n = 279). Significance levels for the two-tailed Mann–Whitney non-parametric test: * P < 0.05; ** P < 0.01; **** P < 0.0001. V13P versus (GS) 4 -V13P, P = 0.02; WT versus (GS) 4 -WT, P = 2.46 × 10 −3 ; Y9P versus (GS) 4 -Y9P, P = 2.98 × 10. The mechanically labile (GS) 4 tag accelerates the nuclear import of the Ig27 variants. l , Relative acceleration of the (GS) 4 -tagged Ig27 variant as a function of its mechanical stability. m , Schematic showing that the addition of a mechanically labile peptide tag next to the NLS substantially increases the kinetics of the nuclear import of protein cargos across the NPC. Significance levels for the Mann–Whitney non-parametric test: NS, P > 0.05; * P < 0.05; *** P < 0.001; **** P < 0.0001. All points and bar plots indicate mean ± s.e.m.
We then explored how the chemical properties of the mechanically labile tag could further regulate the nuclear import rate of the protein cargo. We replaced the chemically inert serine (S) in the (GS) 4 tag with the negatively charged aspartic acid (D) and the highly hydrophobic isoleucine (I), which, according to recent literature 35 , should drastically delay or enhance, respectively, nuclear import across the NPC (Fig. 4e ). We compared the import kinetics of the resulting (GD) 4 -Ig27 WT and (GI) 4 -Ig27 WT proteins and found that adding the (GD) 4 tag significantly slows down (−12%) the kinetics of nuclear entry (and nuclear accumulation) of the Ig27 WT , whereas addition of the (GI) 4 tag significantly increases (+20%) the Ig27 WT ’s nuclear import rate, being also ~8% faster than the (GS) 4 -tagged form (Fig. 4f–h ). These experiments conclude that, at least for the specific protein cargos measured in our optogenetic assay, the ‘mechanical’ and ‘chemical’ contributions to the nuclear import rate—and also the mass contribution (Fig. 1l )—are comparable in magnitude, and can be conveniently combined to finely tune the rate of nuclear import.
We next compared the acceleration effect of the chemically inert (GS) 2 (Extended Data Fig. 6 ) and (GS) 4 in the three Ig27 variants (Fig. 4i–k ), and, as before, the acceleration was substantially exacerbated for the mechanically rigid Ig27 Y9P mutant (Fig. 4l ). It is noteworthy that the addition of GS-tags in larger (and hence slower) constructs formed by the mechanically rigid Spy0128 protein also increased their nuclear import dynamics (Extended Data Fig. 7 ). Consequently, these experiments demonstrate that engineering short, unstructured and floppy GS-tags is a successful molecular strategy to accelerate the nuclear import of proteins (Fig. 4m ). Similar results were obtained in HeLa cells (Supplementary Fig. 7 ). Altogether, the global picture that emerges suggests that low-mass, mechanically soft proteins follow the kinetics dictated by their mass law, whereas mechanically rigid ones exhibit notably slowed kinetics. Such a mechanical penalty can be reversed or bypassed by the addition of a mechanically soft protein (such as the R16) at the leading end, or by adding a mechanically labile, chemically inert 35 and conformationally disordered tag.
To begin to investigate plausible molecular mechanisms underpinning the NPC sensitivity to the cargo mechano-directionality, we turned our attention to the FG-Nups (Fig. 5a ), which represent about a third of nucleoporins 58 , 59 , 60 , fill the central channel of the NPC, and collectively create an effective and selective barrier for cargo translocation. We started by investigating Nup153, Nup98 and Nup214, which have been shown to play a particularly important role in nucleocytoplasmic transport 61 , 62 , 63 , despite being placed in different locations within the NPC, and have also been successfully used in biomimetic NPC systems 64 . It is particularly compelling that Nup153, while being in the nuclear basket, has been found to explore cytoplasmic regions of the NPC as well 65 , by potentially acting as a molecular spring that undergoes fast transitions between extended and collapsed conformations 66 . This rapid exchange between two separate locations within the NPC is suggestive of a Nup-mediated molecular motion mechanism able to facilitate transport across the pore 67 . Consequently, we conjectured that Nup153 might be involved in the mechanoselective transport of proteins across the NPC.
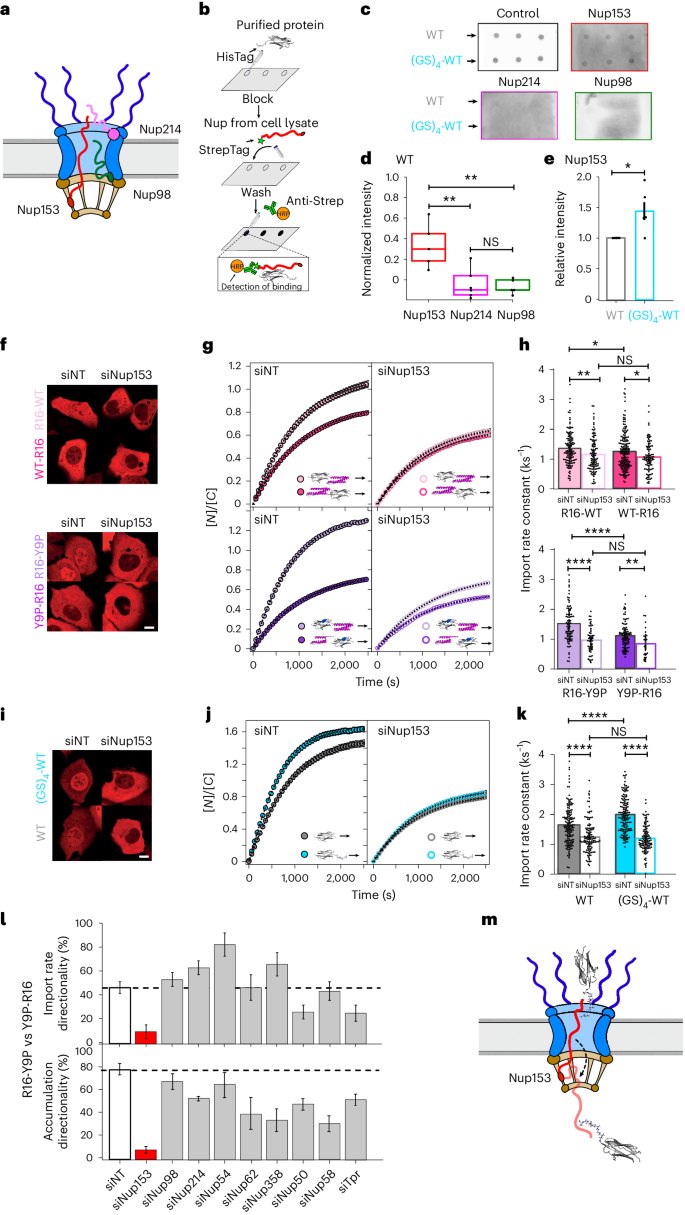
a , Schematics of the NPC, showing the approximate location of Nup153, Nup98 and Nup214. b , Schematics of the dot blot assay. c , Dot blot membranes to assess protein binding to Nup153, Nup98 and Nup214, including a control (no Nup present). d , Dot blot intensity for each of the Nups interacting with Ig27 WT , normalized by the control experiment. Each data point corresponds to the average intensity of three dots per membrane ( N = 5 independent dot blot experiments). Significance levels for two-tailed t -test: NS, P > 0.05; ** P < 0.01; Nup153 versus Nup214, P = 6.94 × 10 −3 ; Nup214 versus Nup98, P = 0.76; Nup153 versus Nup98, P = 1.42 × 10 −3 . Boxes span from the first to the third quartile, and the horizontal line indicates the median. e , Dot blot intensity for Nup153 binding to either Ig27 WT or (GS) 4 -Ig27 WT . Significance levels fo the two-tailed paired t -test: * P < 0.05. WT versus (GS) 4 -WT, P = 0.04. f , Representative confocal images of U2OS cells 30 min into the recovery phase. Scale bar, 10 µm. g , Time courses of the relative nucleus-to-cytoplasm localization of R16-WT versus WT-R16 (top) and R16-Y9P versus Y9P-R16 (bottom) under normal conditions (siNT) and Nup153 knockdown (siNup153). h , Associated import rates: R16-WT (siNT) ( k I = 1.35 ± 0.05 ks −1 , n = 130), R16-WT (siNup153) ( k I = 1.15 ± 0.05 ks −1 , n = 108); WT-R16 (siNT) ( k I = 1.20 ± 0.05 ks −1 , n = 179), WT-R16 (siNup153) ( k I = 1.09 ± 0.05 ks −1 , n = 98); R16-Y9P (siNT) ( k I = 1.51 ± 0 . 06 ks −1 , n = 92), R16-Y9P (siNup153) ( k I = 0.93 ± 0.06 ks −1 , n = 57); Y9P-R16 (siNT) ( k I = 1.07 ± 0.04 ks −1 , n = 100), Y9P-R16 (siNup153) ( k I = 0.83 ± 0.09 ks −1 , n = 33). Significance levels for the two-tailed Mann–Whitney non-parametric test: NS, P > 0.05; * P < 0.05; ** P < 0.01; **** P < 0.0001; siNT R16-WT versus siNup153 R16-WT, P = 2.14 × 10 −3 ; siNT WT-R16 versus siNup153 WT-R16, P = 0.02; siNT R16-WT versus siNT WT-R16, P = 0.03; siNup153 R16-WT versus siNUP153 WT-R16, P = 0.25. siNT R16-Y9P versus siNup153 R16-Y9P, P = 5.70 × 10 −9 ; siNT Y9P-R16 versus siNup153 Y9P-R16, P = 2.50 × 10 −3 ; siNT R16-Y9P versus siNT Y9P-R16, P = 1.26 × 10 −7 ; siNup153 R16-Y9P versus siNUP153 Y9P-R16, P = 0.17. i , Representative confocal image of U2OS cells. Scale bar, 10 µm. j , Time courses of the relative nucleus-to-cytoplasm localization of WT and (GS) 4 -WT under normal conditions (siNT) and Nup153 knockdown (siNup153). k , Associated import rates; WT (siNT) ( k I = 1.61 ± 0.05 ks −1 , n = 147), WT (siNup153) ( k I = 1.22 ± 0.05 ks −1 , n = 118); (GS) 4 -WT (siNT) ( k I = 2.02 ± 0.05 ks −1 , n = 169), (GS) 4 -WT (siNup153) ( k I = 1.28 ± 0.04 ks −1 , n = 122). Significance levels for the two-tailed Mann–Whitney non-parametric test: NS, P > 0.05; **** P < 0.0001; siNT WT versus siNup153 WT, P = 7.74 × 10 −7 ; siNT (GS) 4 -WT versus siNUP153 (GS) 4 -WT, P = 2.50 × 10 −28 ; siNT WT versus siNT (GS) 4 -WT, P = 1.42 × 10 −6 ; siNup153 WT versus siNup153 (GS) 4 -WT, P = 0.59. l , Directionality calculated as the ratio between the average import rate (top) and accumulation (bottom) for translocating R16-Ig27 Y9P versus Ig27 Y9P -R16 constructs, measured when silencing individual FG-Nups. Error bars indicate s.e.m. m , Schematic of the proposed role of Nup153 in identifying locally unstructured protein regions. Significance levels for two-tailed t -test ( d ), two-tailed paired t -test ( e ), Mann–Whitney non-parametric test ( h , k ): NS, P > 0.05; * P < 0.05; ** P < 0.01; *** P < 0.001; **** P < 0.0001. All points and bar plots indicate mean ± s.e.m.
We first queried whether Nup153 specifically binds to protein cargos. We conducted a dot blot assay 68 , where Ig27 WT and (GS) 4 -Ig27 WT were immobilized on a nitrocellulose blotting membrane and then exposed to a bacterial cell lysate containing overexpressed StrepII-tagged FG-rich domains of Nup153, Nup214 and Nup98 (Fig. 5b ). Our results demonstrate that Nup153 significantly binds Ig27 WT , whereas Nup214 and Nup98 do not (Fig. 5c,d ). It is noteworthy that Nup153 binds significantly ( P = 0.04) better to the tagged (GS) 4 -Ig27 WT protein, suggesting a potential role of Nup153 in recognizing unstructured protein cargos (Fig. 5e ).
We then tested whether these biochemical evidences would provide a molecular basis for the cellular translocation experiments by directly probing the effect of transiently silencing Nup153 with small interfering RNA (siNup153) on the kinetics of nuclear accumulation of the Ig27 WT and Ig27 V13P mutant (Extended Data Fig. 8 ). In both cases, we observed a marked decrease in nuclear entry upon Nup153 depletion, suggesting a clear role of Nup153 in regulating transport. However, the relative decrease in the rate of nuclear import with siNup153 was maintained for both proteins of different mechanical stabilities, suggesting that Nup153 does not dictate the mechanoselectivity of the NPC. To explore whether it affected the NPC sensitivity to the cargo mechano-directionality instead, we tested the effect of Nup153 depletion on the R16-Ig27 WT /Ig27 WT -R16 and R16-Ig27 Y9P /Ig27 Y9P -R16 construct pairs (Fig. 5f–h ). Surprisingly, we observed that, in both cases, the mechano-directionality effect disappeared, entailing that, in the absence of Nup153, the rate of nuclear entry for each pair of constructs was independent of the mechanical stability of the leading protein. The same effect was observed when comparing the Ig27 WT /(GS) 4 -Ig27 WT protein pair after Nup153 silencing (Fig. 5i–k ), resulting in the abrogation of the GS-tag acceleration effect. Noteworthy, this effect was maintained upon exposing cells to amphiphilic agents such as trans -1,2-cyclohexanediol (CHD) 60 and Pitstop-2 69 , which are known to disrupt FG-Nup interactions (Supplementary Figs. 8 and 9 ). Moreover, silencing Nup153 did not abrogate the NPC sensitivity to the cargo (R16-Ig27 Y9P /Ig27 Y9P -R16) mechano-directionality during export (Supplementary Fig. 10 ), hence restricting this particular mechanosensitive function of Nup153 to protein import.
To investigate whether other FG-Nups exhibit a similar mechanosensitive function, we repeated the optogenetic import experiments using the R16-Ig27 Y9P /Ig27 Y9P -R16 protein pair upon systematically silencing the majority of the individual FG-Nups essential for maintaining the permeability barrier, namely Nup98 62 , 64 , 70 , Nup214 71 , 72 , Nup54, Nup62, Nup358, Nup50, Nup58 and Tpr 58 , 59 . Remarkably, our measurements did not show any noticeable effect on the NPC’s sensitivity to the mechano-directionality of the shuttling cargos induced by any of the other FG-Nups (Fig. 5l and Supplementary Figs. 11 – 19 ). These results suggest that Nup153 plays a fundamentally specific and unique role in determining the NPC’s ability to sense the mechano-directionality of translocating proteins, probably by recognizing unstructured, mechanically labile, disordered protein regions as a first step during protein translocation across the NPC (Fig. 5m ).
Given that our findings focused on artificially devised cargo systems, we wondered whether the newly uncovered fundamental rules could serve as lessons to inspire the molecular engineering of naturally occurring proteins so as to rationally modify their dynamics of nuclear shuttling. We hence introduced the (GS) 4 tag into the N-terminus of a green fluorescent protein (GFP)-tagged MRTFA 73 , close to its bipartite NLS sequence 74 . In U2OS doubly transfected with (GS) 4 -MRTFA-GFP and MRTFA-mCherry constructs (to provide an internal normalization in each cell), we found that the (GS) 4 -tagged MRTFA variant exhibited a significantly ( P = 5 × 10 −5 ) higher import rate and nuclear accumulation than the unmodified MRTFA (Fig. 6a–c ). We also confirmed that (R16-Ig27 Y9P )-MRTFA exhibits a significantly higher import rate than (Ig27 Y9P -R16)-MRTFA, supporting our findings on the mechano-directionality of the translocating cargo (Extended Data Fig. 9 ). We then tested whether the enhanced nuclear accumulation of (GS) 4 -MRTFA with respect to the bare MRTFA could trigger genetic and functional knock-on effects. Because MRTFA is involved in the expression of genes related to adhesion and motility 75 , 76 , we conducted real-time quantitative polymerase chain reaction (RT–qPCR) experiments on stable U2OS cell lines expressing MRTFA-GFP and (GS) 4 -MRTFA-GFP and measured in each case, 4 h after serum stimulation, the levels of MYL9 and SRF messenger RNA (Fig. 6d ). We found that cells stably expressing (GS) 4 -MRTFA-GFP exhibited significantly higher levels of MYL9 (25% increase, P = 0.044) and SRF (23% increase, P = 0.007) mRNA expression compared with a MRTFA-GFP stable cell line. Finally, we performed a classical cell motility wound-healing assay that showed that U2OS cells stably expressing (GS) 4 -MRTFA closed a wound significantly faster than their MRTFA counterparts (Fig. 6e,f ).
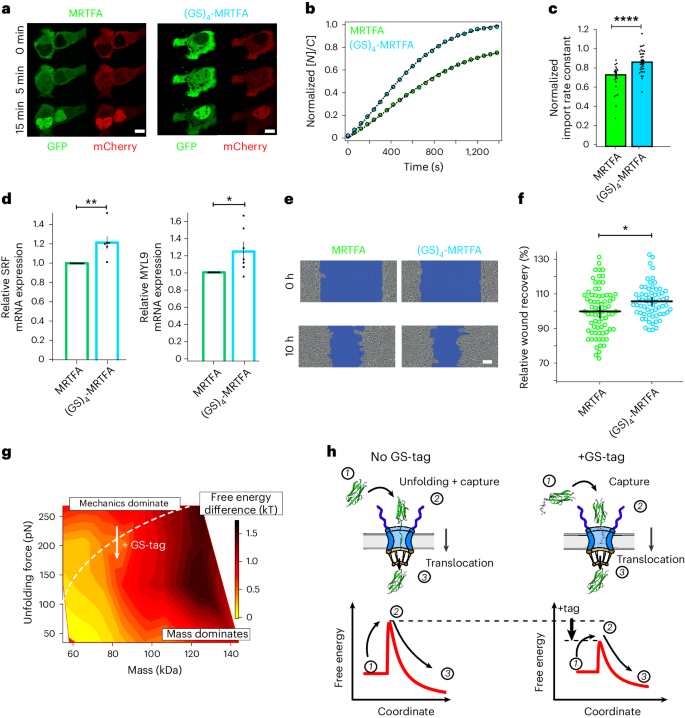
a , Representative confocal microscopy image gallery of U2OS doubly transfected with MRTFA-GFP–MRTFA-mCherry (left) and (GS) 4 -MRTFA-GFP–MRTFA-mCherry (right) at different time points after serum stimulation. Scale bars, 10 µm. b , Normalized average time courses of the relative nucleus-to-cytoplasm localization of MRTFA and (GS) 4 -MRTFA. The average time course is normalized by the average nucleus-to-cytoplasm accumulation ( K e ) of the co-translocating MRTFA-mCherry construct. c , Associated normalized nuclear import rates: MRTFA ( \({\widetilde{k}}_{\rm{I}}\) = 0.68 ± 0.03, n = 21); (GS) 4 -MRTFA ( \({\widetilde{k}}_{\rm{I}}\) = 0.87 ± 0.03, n = 34), where \({\widetilde{k}}_{\rm{I}}\) is the normalized import rate calculated as k I (GFP)/ k I (mCherry). Significance levels for the two-tailed Mann–Whitney non-parametric test: **** P < 0.0001; MRTFA versus (GS) 4 -MRTFA, P = 3.33 × 10 −5 . d , RT–qPCR in U2OS cells stably expressing the MRTFA-GFP and (GS) 4 -MRTFA-GFP, 4 h after serum stimulation ( N = 7 independent experiments). Bars show mean ± s.d. Significance levels for the two-tailed paired t -test after log transformation: * P < 0.01; ** P < 0.001; SRF, P = 7.16 × 10 −3 , MYL9, P = 0.04. e , f , U2OS cells stably expressing GS-tagged MRTFA exhibit increased cell motility: representative wound-healing assays on U2OS stable cell lines expressing MRTFA or (GS) 4 -MRTFA (scale bar, 100 µm) ( e ); relative wound-healing recovery calculated from the velocity of the cell front during the first 10 h for MRTFA ( n = 74) and (GS) 4 -MRTFA ( n = 62) ( f ). Significance levels for the two-tailed t -test: * P < 0.05 ( P = 0.03). g , Free-energy surface for active nucleoplasmic transport projected against the molecular weight and the unfolding force (characterized by AFM) of the translocating protein. The free-energy difference was calculated as \({\Delta G(M,\,{F}_{\rm{U}})}={-{kT}\,\mathrm{ln}\frac{{k}_{\rm{I}}(M,\,{F}_{\rm{U}})}{{k}_{\rm{I}}^{\rm{R}16}}}\) , where k I is the import rate for a protein of mass M and an unfolding force of F U , and where the import rate of the R16 monomer k I R16 has been used as reference. The dotted white line separates the regimes where mechanics or mass dominate the kinetics of nuclear import. h , Cartoon representation describing the passage of protein cargos across the NPC, in the absence (left) and presence (right) of a mechanically labile peptide tag. This results in the lowering of the free energy barrier and therefore in faster nuclear import kinetics. All points and bar plots indicate mean ± s.e.m.
Although the biochemical mechanisms at play during nucleocytoplasmic transport have been investigated in depth, the role that the translocating cargo plays in determining its shuttling kinetics has attracted comparatively less attention. We previously reported that, in addition to molecular weight and exposed sequence, the mechanical stability of proteins emerges as an additional property to regulate nuclear trafficking dynamics 39 . Here we have extended these initial proof-of-principle studies by analysing the interplay between mass and mechanics. By systematically increasing the mass of the protein, we have found that proteins follow a ‘sequential’ scaling or ‘mass’ law when entering the NPC; however, strong deviations emerged at a certain threshold mass value, below which the mechanical stability—and not its mass—dominates nuclear import kinetics (Fig. 6g ). That naturally led us to conjecture a direct relationship between the localized mechanical stability of a polyprotein (ultimately resulting from its local structure) and its nuclear transport dynamics. Specifically, we have demonstrated that mechanically labile, locally unstructured protein regions in the vicinity of the NLS increase the protein’s nuclear import rate across the NPC. These findings enable us to add the role of the protein cargo—based on the general physical principles regulating the translocation of polymers through a pore 77 —into the current description of the energy landscape governing protein traffic across the NPC. This view is based on the thermodynamic description of the ‘entropic exclusion’ model 78 —mostly centred on the interplay between reducing the conformational entropy of the FG-Nnups as cargos enter the pore (which increases with cargo size) and the attractive term stemming from the hydrophobic interactions between the FG-Nups and the translocating cargo, which counteracts the entropic penalty, reducing the total free energy and making cargo passage favourable. (Fig. 6h ). The experiments presented here suggest that the physicochemical properties of the translocating protein cargo (namely its mass and its local mechanical stability) should be considered in this energetic treatment. From the cargo perspective, we propose that the first step in NPC translocation involves the capture of the protein by the NPC mouth. Such an initial capture process has an associated free-energy barrier that results from the entropic contribution of narrowing the conformational space and the energy penalty related to the creation of a mechanically unfolded protein segment that helps position the protein cargo for translocation. Crucially, the presence of either an engineered soft protein such as R16 (Fig. 3 ) or a mechanically labile tag such as a GS polypeptide (Fig. 4 ) bypasses the requirement of (partial) mechanical unfolding, and acts as a molecular handle to translocate into the nucleus, hence substantially lowering the underpinning energy barrier. Regardless of the molecular origin of the locally loose protein structure, Nup153 specifically binds to these disordered regions, reflective of an underpinning attractive interaction potential that lowers the energy barrier to translocation. This is consistent, at least in part, with the directional motion reported for Nup153, involving successive cycles of collapse and release 67 , that might be able to overcome the presence of the other barrier of Nups forming the entropic brush. Other translocation scenarios are, of course, also possible. For example, it has also been reported that Nup153 creates a dense meshwork or ‘hydrogel’, compatible with a selective phase mechanism where Nups physically interact with each other 79 . The resulting protein meshwork is predicted to be ‘dissolved’ 80 by cargo complexes. We conjecture that the enhanced ability of Nup153 to specifically bind unstructured cargos will facilitate their penetration through the Nup meshwork, because, at least from a steric perspective, partially unfolded protein conformations are likely to exhibit enhanced capabilities to navigate through small pore constrictions.
Fundamentally, our results suggest that asymmetric transport seems to be a direct consequence of the mechanical asymmetry of the translocating polyproteins. It remains to be addressed whether local structural anisotropy occurring within an even smaller, single protein domain might also influence the protein’s (mechano)-directional transport.
In a broader context, the results presented here hold large similarities with the mechanisms of protein translocation through other—arguably less complex and structurally narrower—biological pores. For example, in the bacterial ClpXP and ClpAP proteases, an unstructured region of the protein substrate (a degradation tag, or ‘degron’, residing at the C-terminus of a natively folded protein) is required to initially engage the protein substrate in the pore mouth, before the ATPase ring begins to pull and mechanically unfold the protein to enable its translocation across the pore for degradation 44 . Notably, the length and composition of the degron tag precisely define the specificity and degradation kinetics 81 . Similarly, during mitochondrial import, proteins are usually preceded by targeting precursor sequences 82 . The efficiency of protein import depends on the targeting sequence as well as on the local mechanical resistance of the protein structures adjacent to the targeting tags 11 , 83 . Consequently, our results demonstrate that, similar to mitochondria or the bacterial proteolytic machinery, the admittedly much more complex NPC is also able to sense intrinsically disordered protein tags as well as the local mechanical stability of the protein adjacent to the tag (leading protein). However, in contrast to these examples where proteins are mechanically unfolded by specific ATP-dependent unfoldases, we still do not know the precise molecular origin of the force required to release the unstructured protein segments before translocation, where specific molecular machines have not been explicitly identified. We conjecture that the directional, spring-like 67 motion of specific Nups (such as Nup153) might help capture unstructured and mechanically weak protein regions as they enter the pore. It is possible that, similar to the role played by surface-exposed hydrophobic residues in artificial cargos 35 , the newly unfolded protein region of the engineered mechanical tag might be able to favourably interact with the different Nups encountered while being threaded through the pore, thereby not only helping in the initial capture step, but also the subsequent translocation stage. Future systematic sequence optimization of the polypeptide tag might result in further enhancement of the cargo’s nuclear import rate. It is also plausible that the diminished steric hindrance when locally unfolded protein regions (or unstructured tags) adjacent to the NLS are present increase the binding affinity for Kapα:Kapβ, thus favouring the binding of Kapβ with the FG-repeats, ultimately resulting in enhanced nuclear import.
It is thus enticing to speculate that transcription factors that need to constantly cross the NPC have evolved to exhibit intrinsically disordered regions. In fact, it is intriguing that transcription factors (such as MRTFA, for which the full X-ray crystal structure is lacking) are statistically enriched 84 with intrinsically disordered and flexible regions. Consequently, one might expect that, in general, transcription factors will exhibit low mechanical resistance. For example, YAP is mechanically weak, as revealed by single-molecule AFM experiments 38 . From the biological viewpoint, structurally disordered regions in transcription factors are emerging as key determinants to guide protein–protein interactions in transcriptional condensates, actively contributing to DNA-binding specificity 85 . It is appealing to conjecture that these protein regions might include, or be close to, the NLS sequence, underscoring yet another layer of nuclear transport-based functionality.
In short, our work resonates with the rapidly emerging data unravelling the structural and functional complexity of the NPC 16 , 17 , 18 , 29 , 45 and provides a cargo perspective, complementing pore-centric studies 66 , 86 , 87 , on the regulation of nucleocytoplasmic transport at the nanoscale. More generally, our approach might be useful as a biotechnological tool to deliver a wide range of cargos to the cell nucleus.
Plasmid construct and polyprotein engineering
For the optogenetic experiments using the NLS-mCherry-LEXY, the original pDN122 plasmid (Addgene 72655 ref. 42 ) was modified to remove the BglII site and the multicloning site. A cassette containing an NheI-NLS-BamHI-Ig27-KpnI-EheI sequence was inserted into the modified pDN122 between the NheI and AjiI sites before mCherry to create NLS-Ig27-mCherry-LEXY, allowing any DNA sequence to be swapped into the vector using BamHI-KpnI sites. Cloning was achieved using FastDigest Enzymes (Thermo Fisher Scientific) and T4 DNA ligase (New England Biolabs) unless otherwise stated.
Polymers were engineered by restriction digest using compatible cohesive ends restriction enzymes BamHI and BglII, with KpnI. For the final constructs, the terminal domain BamHI-X-KpnI was inserted between BglII and KpnI in the cleaved plasmid. To create GS-tagged proteins, a cassette of varying lengths of GS peptides, including an N-terminal BamHI site and C-terminal BglII and KpnI sites, was inserted into the modified pDN122 vector. Subsequent polymers were engineered as previously described. Cloning was achieved using FastDigest Enzymes (Thermo Fisher Scientific) and T4 DNA ligase (New England Biolabs). For MRTFA translocation experiments, (GS) 4 was inserted in front of the MRTFA sequence (MRTFA-GFP vector kindly provided by M. Vartiainen 88 ), by Gibson cloning after an L92M modification on the original plasmid to produce (GS) 4 -MRTFA-GFP (Gibson Assembly Master Mix, New England BioLabs). Recombinant plasmids were transformed into TOP10 competent cells (Thermo Fisher Scientific). Selected colonies were grown in Luria broth (LB) supplemented with 100 μg ml −1 ampicillin (or kanamycin 50 μg ml −1 for MRTFA constructs) at 37 °C, and the plasmid DNA was purified on GeneJet purification columns (Thermo Fisher Scientific), according to the manufacturer’s instructions.
For single-molecule experiments, polyproteins were engineered as previously described into pQE80L (Qiagen) between the BamHI and KpnI restriction sites. For AFM, polymers were designed with two additional cysteine residues at the C-terminus. For magnetic tweezers (MT) experiments, constructs were subcloned into a modified pFN18a vector (Promega) engineered with the AviTag (Avidity) (sequence GLNDIFEAQKIEWHE). Polymers were inserted between the HaloTag at the N-terminus and the histidine tag adjacent to the AviTag at the C-terminus using BamHI and KpnI restriction sites. Recombinant plasmids were transformed into TOP10 competent cells (Thermo Fisher Scientific). Selected colonies were grown in LB supplemented with 100 μg ml −1 carbenicillin at 37 °C, and the plasmid DNA was purified on GeneJet purification columns (Thermo Fisher Scientific), according to the manufacturer’s instructions. DNA sequences were verified by GENEWIZ (Azenta Life Sciences) or Source BioScience.
For the dot blot assays, DNA sequences encoding the FG-rich domains of Nup153 (residues 901–1475), Nup214 (residues 1400–2090) and Nup98 (residues 1–480) with a StrepII tag (located at the N terminus for Nup153 and Nup214 and at the C-terminus for Nup98) were synthesized by GeneArt (Thermo Fisher Scientific). These sequences were designed to incorporate NdeI and XhoI restriction sites for subsequent insertion into the pET50b+ plasmid. The coding sequences for I27 WT and (GS) 4 -I27 WT , all tagged with 6x His at the C-terminus, were also cloned into the pET50b+ vector using NdeI and XhoI as restriction sites.
Polyprotein expression and purification
Constructs encoding for the polyproteins of interest were expressed in Escherichia coli T7 Express cells (New England Biolabs) and grown in LB supplemented with 100 μg ml −1 carbenicillin at 37 °C. For the polyproteins used in MT experiments, biotinylation of the avidin tag was performed either in vivo (by co-expression of pBirAcm in medium supplemented with 34 mg ml −1 chloramphenicol) or post-elution through a purified BirA ligase (both Avidity). After reaching an optical density at 600 nm (OD 600 ) of 0.6, cultures were induced with 1 mM isopropyl-β- d -thiogalactopyranoside (IPTG) and, for in vivo biotinylation, 50 μM d -biotin was also added. Cultures expressing polyproteins were incubated in a shaker incubator for 16 h at 20 °C. Cells were collected by centrifugation at 3,000 g for 20 min and bacterial pellets of 500 ml of culture were resuspended in 25 ml of lysis buffer containing 20 mM HEPES pH 7.5, 300 mM NaCl, in the presence of protease inhibitor cocktail tablets (Roche), supplemented with 0.8 mg ml −1 lysozyme, 8 µg ml −1 DNase, 8 µg ml −1 RNase, 4 mM phenylmethylsulfonyl fluoride and 10 mM MgCl 2 . After incubation on ice for 30 min, the cells were disrupted by a French press (G. Heinemann) and the lysate was cleared by centrifugation at 39,000 g for 45 min. The supernatant containing the soluble His-tagged protein was filtered with a cell strainer (40-µm filter) and then mixed with a HisPur Cobalt resin (Thermo Fisher Scientific) previously equilibrated in a buffer containing 20 mM HEPES pH 7.5 and 300 mM NaCl (buffer A) for 1 h at 4 °C under rotation.
The lysate/cobalt resin mixture was washed with 100 column volumes with buffer A containing 10 mM imidazole. Protein elution was carried out with buffer A containing 500 mM imidazole. The eluted fractions were collected and analysed by SDS–PAGE.
Fractions containing the protein were then collected and dialysed overnight against a buffer containing 20 mM HEPES pH 7.5 and 150 mM NaCl. The protein sample was concentrated using a Vivaspin centrifugal device (Sartorious) and further purified on a Superdex 200 Increase 10/300 GL column (Cytiva) using buffer A supplemented with 10% glycerol as running buffer. Elutions were analysed by SDS–PAGE, and pure protein samples were flash-frozen and stored at −80 °C.
For the dot blot assay, plasmids encoding for I27 WT -6His and (GS) 4 -I27 WT -6His as well as the StrepII-tagged FG-rich domains of Nups 153, 214 and 98 were transformed into E. coli T7 Express cells (New England Biolabs) and cultured in LB medium supplemented with 50 µg ml −1 kanamycin at 37 °C. When the cultures reached an OD 600 of ∼ 0.6, they were induced with 1 mM IPTG and allowed to grow at 20 °C for 16 h.
The optical densities (ODs) of cell cultures expressing the StrepII-tagged FG-rich domains of Nups 153, 214 and 98 were measured after induction with IPTG, and an adjusted volume containing precisely 750 Optical Density Units for each culture was pelleted. Bacterial cultures were subsequently subjected to centrifugation at 3,000 g for 20 min at 4 °C, and the pellets were diluted in 25 ml of binding buffer (buffer A). Cells were disrupted using a French press. I27 WT -6His and (GS)4-I27 WT -6His protein purifications were conducted as described above.
Cell culture, stable and transient transfection, and siRNA transfection and generation of stable cell lines
U2OS (American Type Culture Collection), NIH 3T3 (Francis Crick Institute) and HeLa (Francis Crick Institute) cells were grown in Dulbecco’s modified Eagle medium (DMEM), high glucose (Merck), supplemented with 10% fetal bovine serum (Merck) (for U2OS and HeLa cells) or 10% calf bovine serum (Merck) (for NIH 3T3 cells), 100 U ml −1 penicillin, 100 mg ml −1 streptomycin and 2 mM glutamine (Invitrogen). For transient protein expression, cells were transfected with 1 μg of constructs using HD FuGENE (Promega) according to the manufacturer’s protocol. To produce stable cell lines of MRTFA-GFP and (GS) 4 -MRTFA-GFP in U2OS, transiently transfected cells were selected with 500 μg ml −1 geneticin (Thermo Fisher Scientific), following fluorescence-activated cell sorting. Polyclonal cell lines were obtained and cultured in Complete medium supplemented with 500 μg ml −1 geneticin. For protein knockdown experiments, siRNA (non-targeting; D-001810-10-05, Nup153 (L-005283-00-0005), Nup98 (L-013078-00-0005), and Nup214 (L-011980-00-0005), Tpr (L-010548-00-0005), Nup62 (L-012468-00-0005), Nup50 (L-012369-01-0005), Nup58 (L-013864-01-0005), Nup358 (L-004746-00-0005), Nup54 (L-017570-01-0005); Horizon Discovery) was transfected into cells with HiPerFect (Qiagen) using the manufacturer’s protocol.
For MRTFA double transfection, cells were transfected with 1 μg of constructs (in total) using HD FuGENE (Promega) according to the manufacturer’s protocol. Cells were starved by withdrawing serum and incubating in a serum-starved medium containing 0.3% fetal bovine serum for 24 h. Starved cells were stimulated with 15% serum before imaging.
Drug treatments
Pitstop-2 (abcam, ab120687) or dimethylsulfoxide (DMSO; control group) was added to the cells 30 min before image acquisition at a concentration of 30 µM, or, for DMSO, a concentration of 1:1,000. Samples were then imaged as described above. We added trans -1,2-cyclohexanediol (CHD; Merck, 141712) at a concentration of 20 mM 30 min before image acquisition. Saline buffer was added 30 min before image acquisition for the controls.
Protein extraction and immunoblotting
Cells were lysed using radioimmunoprecipitation assay buffer with a protease inhibitor cocktail. The protein concentration of the lysates was determined with a Pierce BCA assay (Thermo Fisher Scientific), following separation by SDS–PAGE and transferal to a nitrocellulose membrane. For TPr and Nup358(RanBP2), NuPAGE 3–8% Tris-acetate gel (23060670, Thermo Fisher) was used, and Mini-PROTEAN TGX gel (4561096, Bio-Rad) was used for all other Nups. Membranes were incubated in blocking buffer (5% milk in TBS containing 0.2% Tween-20) for 1 h at room temperature and then incubated overnight with antibodies against Nup153 (A301-788A-T, Bethyl Laboratories), Nup98 (ab125980, Abcam), Nup214 (A300-717A-T, Bethyl Laboratories), Tpr (A300-828A, Bethyl Laboratories), RANBP2 (nup358) (16232-1-AP, Proteintech), Nup54 (27606-1-AP, Proteintech), Nup62 (13916-1-AP, Proteintech), NupL1 (Nup58) (19907-1-AP, Proteintech), Nup50 (20798-1-AP, Proteintech) and glyceraldehyde-3-phosphate dehydrogenase (GAPDH, Abcam). Antibodies were visualized using a chemiluminescence detection system (Bio-Rad). Densitometric analysis was performed using ImageJ.
Wound-healing assay
U2OS cells stably expressing MRTFA-GFP and (GS) 4 -MRTFA-GFP were seeded onto ImageLock 96-well plates, which allow wound position tracking using Incucyte Zoom (Essen Bioscience). At 20 h before the beginning of the assay, the cells were serum-starved (0.3% serum). Scratch wounds were made using Woundmaker 96 (Essen Bioscience). The plate was washed once with phosphate-buffered saline (PBS), to remove any non-attached cells, then the cells were stimulated by the addition of cell medium containing 10% serum. Image acquisition and wound-length measurements from the acquired images were performed with Incucyte software. The closure of the wound was followed for 24 h (acquisition every 30 min). The wound-healing velocity was estimated from a linear fit to the first 10 h of the wound length versus time evolution. Final values were normalized with respect to MRTFA-GFP. Data were collected from six independent experiments.
Live-cell image acquisition
Before image acquisition, HEPES pH 7 was added (to a final concentration of 50 mM) to the cells. Live-cell imaging was performed in an enclosed environment chamber (37 °C) with a confocal Nikon A1R inverted microscope with a ×60 NA 1.40 oil-immersion objective. Up to six sample positions were recorded for at least three independent replicates. The microscope was operated with the Nikon Perfect Focus System and controlled by NIS Elements software.
For optogenetic polyprotein experiments, image acquisition was performed throughout the activation and recovery phases. Activation of the NLS-X-mCherry-LEXY constructs was performed with a constant blue light (488 nm) illuminating the sample (10 min, a frame every min). The duration of the recovery period was increased with the number of protein domains in the construct, as higher molecular weights are associated with lower translocation rates and require longer observation times for accurate quantification (Supplementary Table 1 ).
For the MRTFA serum-stimulated experiments, the cells were incubated in serum-starved medium (0.3% serum) 16–20 h before the experiments. MRTFA translocation was triggered by the addition of 15% serum. Live-cell imaging was performed in an enclosed environment chamber (37 °C) with a confocal Nikon A1R inverted microscope with a ×60 NA 1.40 oil-immersion objective and laser and emission filter wavelengths of 488.2 nm and 540/30 nm for GFP and 561.9 nm and 595/50 nm, respectively for mCherry. Images were typically acquired every 60 s over 45 min.
To study protein export, we used a related optogenetic construct, NES-X-mCherry-AsLOV2-NLS, where the position of the NES and NLS tags has been swapped. In this construct, upon blue-light exposure, the protein cargo shuttles into the nucleus. Under dark conditions, the NES tag excludes the protein from the nucleus, hence increasing the cytoplasm/nucleus ratio, enabling direct quantification of the export rate. To activate protein export, cells were exposed to constant blue-light illumination (20 min, frame every min). The duration of the recovery period for the tested export constructs was 45 min, acquiring one image frame per minute.
RNA extraction and qPCR
RNA was extracted from U2OS cells using an RNeasy Mini kit (Qiagen) according to the manufacturer’s instructions, and was then treated with DNase (Invitrogen). The RNA concentration was determined and the purity checked by measuring the ratio of absorbance at 260 and 280 nm. RNA was converted to complementary DNA (cDNA) using iScript reverse transcription supermix for RT–qPCR (Bio-Rad) according to the manufacturer’s instructions. The relative mRNA expression of genes was determined by RT–qPCR assay using SYBR-Green detection chemistry (Applied Biosystems) and a QuantStudio 3 RT–qPCR system (Thermo Fisher). The primers used are detailed in Supplementary Table 2 . The relative abundance of template cDNA was calculated by the comparative CT (ΔΔCT) method. Each sample was normalized to the level of GAPDH.
Dot blot assay
Four small nitrocellulose blotting membranes (Amersham Protran 0.2-µm NC) were cut into quadrangular shapes, each measuring ~5.5 × 5.5 cm. The purified proteins were diluted to immobilize an equivalent amount of I27 WT -6His and (GS) 4 -I27 WT -6His, respectively. Spots containing these purified proteins were then fixed in triplicate onto three respective rows for each membrane and dried at room temperature for ~20 min.
The control membrane was blocked in a Petri dish with 5% (wt/vol) dry skimmed milk in TBS solution with 0.1% Tween (TBS-T), and the other three were blocked with 3% bovine serum albumin (BSA) in PBS-T (0.5% Tween in PBS) for a duration of 16 h at 4 °C.
The blots were washed twice for 5 min in TBS-T and PBS-T, respectively. Subsequently, they were incubated overnight at 4 °C with 750 OD units of bacterial lysates from cultures containing the overexpressed StrepII-Nup153, StrepII-Nup214 and StrepII-Nup98, respectively. The control membrane was incubated with the binding buffer.
The membranes were then washed three times in TBS-T and PBS-T, respectively. Afterwards, they were incubated at room temperature for 1 h with the corresponding antibodies. On the control membrane, horseradish peroxidase (HRP)-conjugated anti-His-Tag antibody was used at a 1:5,000 dilution in TBS-T. For the other three membranes, the Strep•Tag II antibody HRP conjugate was used at a 1:4,000 dilution in PBS-T to detect the binding of Nup to immobilized proteins.
Six additional washes were completed before membrane development, which was performed using a SuperSignal West Pico chemiluminescent detection kit from Thermo Scientific, following the manufacturer’s recommendations.
To quantify the dot blot assay, dot blot intensity was calculated as the pixel intensity (in black) of the dot region after substracting its background. For each experiment (membrane), the dot blot intensity for a particular group (for example, Ig27 WT - with Nup153) was calculated as the average intensity of the three dots normalized by the average intensity of the three dots in the corresponding control membrane (for example, Ig27 WT -). A total of five of five dot blot experiments were conducted.
Live-cell quantification
Image quantification was performed using a custom-made MATLAB script. The image collections for each recorded position were initially background-corrected, and then the total fluorescent intensities of the nucleus and cell were defined manually. For each frame, the nuclear or cytoplasmic signals were divided by the total cellular signal, and this quantity was used as a proxy for the nuclear or cytoplasmic protein concentrations, respectively. To quantify the nuclear import kinetics, we assumed a simple first-order kinetic process. For large LEXY constructs (>45 kDa), (1) passive transport is negligible and (2) there is no active export, because the NES sequence docked onto the LOV domain and is not accessible to the exportins 42 . Therefore, the recovery kinetics arise from the NLS-driven active import of the protein cargo into the nucleus. That way, the nuclear protein concentration [ N ]( t ) will increase in time as
where [ N ] 0 and [ N ] e are the initial and steady-state nuclear concentrations, respectively, and k I is the import rate constant. Similarly, the cytoplasmatic concentration will evolve as
Because the nucleus and cytoplasm have different volumes (and therefore the same number of proteins in the nucleus or in the cytoplasm will correspond to a different concentration), we can define the nucleus-to-cytoplasmatic volume ratio as
because the total number of proteins is constant. Our initial conditions correspond to the end of the activation phase, during which blue light was applied for 10 min, releasing the docked NES sequence and triggering mobilization of the protein construct out of the nucleus. Due to the much higher strength of the NES sequence with respect to the NLS one, the activation phase is dominated by the export kinetics, with a negligible contribution of active import 42 . Therefore, we can assume [ N ] 0 → 0; [ C ] 0 → [ C ] 0 + v [ N ] 0 , which allows us to write the time course of the nucleus-to-cytoplasm protein concentration as
where K e = [ N ] e /[ C ] e is the relative nucleus-to-cytoplasm accumulation in the steady state. Importantly, K e and k I are two uncoupled (free) parameters because there is no active protein export that contributes to the relaxation to the steady state. Although in most of the measured protein constructs K e and k I appear correlated (a faster import rate is typically associated with a higher nuclear accumulation, although there are some notable exceptions), both parameters have a different physical meaning: k I is the rate constant that defines the import kinetics into the nucleus, while K e represents the fraction of mobile proteins, related to the efficiency of the transport process, and is also construct-dependent.
In the case of MRTFA, by contrast, active export is not negligible due to its NES sequence. Although typically the export rate is much lower than the import rate under serum stimulation (~10%) 39 , one should always explicitly account for the import and export rates in the kinetic model, which leads to the following expression for the relative nucleus-to-cytoplasm protein concentrations 39 :
Although, formally, both equations have the same shape, here K e = k I / k E and k = k E + vk I .
We quantify the live-cell images by measuring the nuclear and cytoplasmic signals. By fitting the time course of [ N ]/[ C ] during the recovery phase to equation ( 1 ), we extract the relative nuclear accumulation K e and import rate k I . At the start of the recovery phase, there is a cell-to-cell variation in the initial conditions, which we correct by fitting the raw nuclear signal to n 0 e −kt + n e (1 − e −kt ) and subtracting n 0 e −kt from each data point (Supplementary Fig. 1a,b ). We then fit the corrected [ N ]/[ C ]( t ) to equation ( 1 ) and extract K e and k I , which characterize the import dynamics for that specific cell (Supplementary Fig. 1b ). Spurious cell measurements are removed from the analysis by applying a semi-automatic three-step filtering protocol:
Unphysical fits and outliers are removed using an iterative algorithm (generalized extreme studentized deviate), with a tolerance of two standard deviations.
Poorly activated cells (not meeting the initial conditions requirement assumed in the model) are filtered by evaluating the export rate constant from a single exponential fit to the nucleus signal in the activation phase. If the obtained export rate constant is lower than 1.4 ks −1 (85% of the duration of the activation phase), the cell is assumed to be poorly activated and is discarded.
A human inspection of the cells that passed steps 1 and 2 is finally done to discard spurious data that escaped the automatic filtering steps.
For a given protein construct, we quantify its nuclear entry properties with the average time course of the nucleus-to-cytoplasm protein concentration (calculated as a point-by-point average of each single cell recovery curve; Supplementary Fig. 1c ) and by the distributions of K e and k I . Supplementary Fig. 1d shows the distributions of K e (accumulation) and k I (import rate constant) for the NLS-(Ig27 WT )-mCherry-LEXY protein construct. To perform statistical comparisons between pairs of interest in LEXY (or MRTFA) experiments, we use a Mann–Whitney non-parametric test on the distribution of import rates k I , given their markedly non-Gaussian shape.
Analysis of nuclear export experiments was conducted in an analogous way, using in this case the cytoplasm-to-nucleus ratio ([ C ]/[ N ]( t )) instead of the nucleus-to-cytoplasm ratio.
‘Stochastic’ versus sequential unfolding model
In a single-molecule force spectroscopy experiment, a polyprotein composed of N identical domains is stretched from the molecule termini, implying that all N domains are exposed to force. All domains will thus unfold stochastically following their unfolding rate r U , following first-order kinetics. According to this, the total rate to unfold the polyprotein r N (this is the inverse of the mean-first-passage time to the last unfolding event) scales with the number of domains N as
where this is a purely Markovian process.
By contrast, if a polyprotein unfolds sequentially, domain i can only unfold if domain i − 1 has previously unfolded, and the unfolding rate of the full polyprotein scales with the number of domains N as
AFM force spectroscopy
Single-molecule AFM experiments were conducted using a Luigs and Neumann instrument operating at room temperature, as previously described 89 . During sample preparation, 0.5–2 μl of protein (1–5 mg ml −1 in PBS pH 7.3) was spread onto a gold-coated coverslip, previously plasma-cleaned for 10 min. Before each experiment, the cantilever (Si 3 N 4 MLCT-C, Bruker) was calibrated using the equipartition theorem, obtaining a spring constant of ~12–20 pN nm −1 .
In the force–extension mode, the experiment was initiated by first pressing the cantilever tip against the protein-coated surface with high force ( ∼ 1,000 pN) to achieve non-specific binding between a protein and the tip. Then, for both polyprotein constructs ((Ig27 WT ) 2 -(R16) 2 and (R16) 2 -(Ig27 WT ) 2 ), the piezo-mounted surface was retracted at a constant velocity of 400 nm s −1 , resulting in application of an increasing force. Data were recorded and analysed using a custom-made software script in Igor Pro (WaveMetrics). During the recordings, only traces showing both Ig27 WT domains were selected for analysis. To obtain the increment in contour length, every protein unfolding event was fitted to the worm-like chain model of polymer elasticity. Unfolding forces were determined from the position of the peaks.
When studying the unfolding rate of (Ig27 WT ) N at constant force (force-clamp mode), the experiment was begun by pressing the cantilever against the surface ( ∼ 500–2,000 pN for 1 s) to allow protein adhesion. The piezoelectric actuator was then retracted to achieve a constant force of 150 pN, maintained by an active feedback system that corrected the position of the piezo on a timescale of ∼ 1–5 ms to maintain a constant cantilever deflection of the cantilever (and hence a constant force). All force traces were filtered using a pole Bessel filter at 1 kHz. To analyse the force-clamp trajectories, only recordings showing ≥2 steps of 25 nm were selected to ensure a robust molecular fingerprint. Additionally, only recordings showing a detachment time three times longer than the Ig27 unfolding time were further analysed, to ensure that all Ig27 domains exposed to force had unfolded.
MT force spectroscopy
Single-molecule MT experiments were conducted on a custom-made set-up, as described in ref. 90 . Briefly, the set-up was built on an inverted microscope (Nikon) with the magnets (N52) mounted on a voice coil (Equipment Solutions) to control their vertical position, and placed on top of a ×100 oil-immersion objective (Nikon) mounted on a piezoelectric actuator (PI). Illumination was provided by a white light-emitting diode cold light source (Thorlabs), while image acquisition was achieved with a complementary metal–oxide–semiconductor camera (Ximea). Control of the magnet’s position and the piezo was achieved with a multifunction DAQ card (National Instruments), using custom-made data-acquisition software.
The molecule of interest (R16-Ig27 WT and Ig27 WT -R16 in our case) was tethered to a superparamagnetic Dynabeads M-270 streptavidin-coated bead (Invitrogen), which typically resists forces up to 120 pN. The sample was prepared in custom-made fluid chambers consisting of two glass coverslips (Menzel–Glaser) separated by a laser-cut parafilm pattern. The fluid chamber was functionalized with HaloTag O4 ligand 90 to achieve covalent and specific anchoring of the N-terminal HaloTag protein constructs, and amino-coated non-magnetic beads were used as reference beads. The chamber was passivated using Tris blocking buffer (20 mM Tris-HCl pH 7.4, 150 mM NaCl, 2 mM MgCl 2 and 1% wt/vol sulfhydryl blocked BSA).
The protein was incubated in the fluid chamber for ∼ 30 min (1–5 nM) to ensure attachment, and the experiments were conducted in PBS containing 10 mM ascorbic acid pH 7.4 to minimize oxidative damage. M-270 beads ( ∼ 20 μl) were added in the chamber and incubated for 5 min before force application. To unfold the R16-Ig27 WT or Ig27 WT -R16 constructs, a linear force ramp at a loading rate of 1 pN s −1 between 4 and 110 pN was applied. The observed step size for each event was converted to a contour length increment using the freely jointed chain model assuming a Kuhn length of 1.1 nm, so each event was characterized by its unfolding force and contour length increment. On every occasion, R16 unfolded first at a force of ∼ 25–30 pN (showing a step size of ∼ 21 nm that indicates a contour length increment of ∼ 35 nm) and Ig27 WT second at a force of ∼ 95–105 pN (showing a step size of ∼ 25 nm, indicating a contour length of ∼ 28 nm).
Data availability
The data that support the plots within this paper and other findings of this study are available from the corresponding author upon reasonable request.
Code availability
The code is available from the corresponding author upon reasonable request.
Miroshnikova, Y. A. & Wickstrom, S. A. Mechanical forces in nuclear organization. Cold Spring Harb. Perspect. Biol. 14 , a039685 (2022).
Google Scholar
Iskratsch, T., Wolfenson, H. & Sheetz, M. P. Appreciating force and shape—the rise of mechanotransduction in cell biology. Nat. Rev. Mol. Cell Biol. 15 , 825–833 (2014).
Vogel, V. & Sheetz, M. Local force and geometry sensing regulate cell functions. Nat. Rev. Mol. Cell Biol. 7 , 265–275 (2006).
Kirby, T. J. & Lammerding, J. Emerging views of the nucleus as a cellular mechanosensor. Nat. Cell Biol. 20 , 373–381 (2018).
Medjkane, S., Perez-Sanchez, C., Gaggioli, C., Sahai, E. & Treisman, R. Myocardin-related transcription factors and SRF are required for cytoskeletal dynamics and experimental metastasis. Nat. Cell Biol. 11 , 257–268 (2009).
Dupont, S. et al. Role of YAP/TAZ in mechanotransduction. Nature 474 , 179–183 (2011).
Mammoto, A., Mammoto, T. & Ingber, D. E. Mechanosensitive mechanisms in transcriptional regulation. J. Cell Sci. 125 , 3061–3073 (2012).
Knockenhauer, K. E. & Schwartz, T. U. The nuclear pore complex as a flexible and dynamic gate. Cell 164 , 1162–1171 (2016).
Beck, M. & Hurt, E. The nuclear pore complex: understanding its function through structural insight. Nat. Rev. Mol. Cell Biol. 18 , 73–89 (2017).
Grunwald, D. & Singer, R. H. Multiscale dynamics in nucleocytoplasmic transport. Curr. Opin. Cell Biol. 24 , 100–106 (2012).
Wilcox, A. J., Choy, J., Bustamante, C. & Matouschek, A. Effect of protein structure on mitochondrial import. Proc. Natl Acad. Sci. USA 102 , 15435–15440 (2005).
ADS Google Scholar
Gao, Y., Skowyra, M. L., Feng, P. & Rapoport, T. A. Protein import into peroxisomes occurs through a nuclear pore-like phase. Science 378 , eadf3971 (2022).
Cordova, J. C. et al. Stochastic but highly coordinated protein unfolding and translocation by the ClpXP proteolytic machine. Cell 158 , 647–658 (2014).
Maillard, R. A. et al. ClpX(P) generates mechanical force to unfold and translocate its protein substrates. Cell 145 , 459–469 (2011).
Lin, D. H. et al. Architecture of the symmetric core of the nuclear pore. Science 352 , aaf1015 (2016).
Zimmerli, C. E. et al. Nuclear pores dilate and constrict in cellulo. Science 374 , eabd9776 (2021).
Schuller, A. P. et al. The cellular environment shapes the nuclear pore complex architecture. Nature 598 , 667–671 (2021).
Fontana, P. et al. Structure of cytoplasmic ring of nuclear pore complex by integrative cryo-EM and AlphaFold. Science 376 , eabm9326 (2022).
Schmidt, H. B. & Gorlich, D. Transport selectivity of nuclear pores, phase separation and membraneless organelles. Trends Biochem. Sci. 41 , 46–61 (2016).
Lemke, E. A. The multiple faces of disordered nucleoporins. J. Mol. Biol. 428 , 2011–2024 (2016).
Timney, B. L. et al. Simple rules for passive diffusion through the nuclear pore complex. J. Cell Biol. 215 , 57–76 (2016).
Tu, L. C., Fu, G., Zilman, A. & Musser, S. M. Large cargo transport by nuclear pores: implications for the spatial organization of FG-nucleoporins. EMBO J. 32 , 3220–3230 (2013).
Paci, G., Zheng, T., Caria, J., Zilman, A. & Lemke, E. A. Molecular determinants of large cargo transport into the nucleus. eLife 9 , e55963 (2020).
Lowe, A. R. et al. Selectivity mechanism of the nuclear pore complex characterized by single cargo tracking. Nature 467 , 600–603 (2010).
Paine, P. L. & Feldherr, C. M. Nucleocytoplasmic exchange of macromolecules. Exp. Cell. Res. 74 , 81–98 (1972).
Paine, P. L., Moore, L. C. & Horowitz, S. B. Nuclear envelope permeability. Nature 254 , 109–114 (1975).
Terry, L. J., Shows, E. B. & Wente, S. R. Crossing the nuclear envelope: hierarchical regulation of nucleocytoplasmic transport. Science 318 , 1412–1416 (2007).
von Appen, A. et al. In situ structural analysis of the human nuclear pore complex. Nature 526 , 140–143 (2015).
Mosalaganti, S. et al. AI-based structure prediction empowers integrative structural analysis of human nuclear pores. Science 376 , eabm9506 (2022).
Bley, C. J. et al. Architecture of the cytoplasmic face of the nuclear pore. Science 376 , eabm9129 (2022).
Petrovic, S. et al. Architecture of the linker-scaffold in the nuclear pore. Science 376 , eabm9798 (2022).
Matsuda, A. & Mofrad, M. R. K. On the nuclear pore complex and its emerging role in cellular mechanotransduction. APL Bioeng. 6 , 011504 (2022).
Stewart, M. Molecular mechanism of the nuclear protein import cycle. Nat. Rev. Mol. Cell Biol. 8 , 195–208 (2007).
Paci, G., Caria, J. & Lemke, E. A. Cargo transport through the nuclear pore complex at a glance. J. Cell Sci. 134 , jcs247874 (2021).
Frey, S. et al. Surface properties determining passage rates of proteins through nuclear pores. Cell 174 , 202–217 (2018).
Andreu, I., Granero-Moya, I., Garcia-Manyes, S. & Roca-Cusachs, P. Understanding the role of mechanics in nucleocytoplasmic transport. APL Bioeng. 6 , 020901 (2022).
Andreu, I. et al. Mechanical force application to the nucleus regulates nucleocytoplasmic transport. Nat. Cell Biol. 24 , 896–905 (2022).
Elosegui-Artola, A. et al. Force triggers YAP nuclear entry by regulating transport across nuclear pores. Cell 171 , 1397–1410 (2017).
Infante, E. et al. The mechanical stability of proteins regulates their translocation rate into the cell nucleus. Nat. Phys. 15 , 973–981 (2019).
Li, H., Carrion-Vazquez, M., Oberhauser, A. F., Marszalek, P. E. & Fernandez, J. M. Point mutations alter the mechanical stability of immunoglobulin modules. Nat. Struct. Biol. 7 , 1117–1120 (2000).
Lu, H. & Schulten, K. The key event in force-induced unfolding of Titin’s immunoglobulin domains. Biophys. J. 79 , 51–65 (2000).
Niopek, D., Wehler, P., Roensch, J., Eils, R. & Di Ventura, B. Optogenetic control of nuclear protein export. Nat. Commun. 7 , 10624 (2016).
Carrion-Vazquez, M. et al. Mechanical and chemical unfolding of a single protein: a comparison. Proc. Natl Acad. Sci. USA 96 , 3694–3699 (1999).
Olivares, A. O., Baker, T. A. & Sauer, R. T. Mechanical protein unfolding and degradation. Annu. Rev. Physiol. 80 , 413–429 (2018).
Fernandez-Martinez, J. & Rout, M. P. One ring to rule them all? Structural and functional diversity in the nuclear pore complex. Trends Biochem. Sci. 46 , 595–607 (2021).
Fisher, T. E., Oberhauser, A. F., Carrion-Vazquez, M., Marszalek, P. E. & Fernandez, J. M. The study of protein mechanics with the atomic force microscope. Trends Biochem. Sci. 24 , 379–384 (1999).
Brujic, J., Hermans, R. I., Garcia-Manyes, S., Walther, K. A. & Fernandez, J. M. Dwell-time distribution analysis of polyprotein unfolding using force-clamp spectroscopy. Biophys. J. 92 , 2896–2903 (2007).
Schlierf, M., Li, H. & Fernandez, J. M. The unfolding kinetics of ubiquitin captured with single-molecule force-clamp techniques. Proc. Natl Acad. Sci. USA 101 , 7299–7304 (2004).
Carrion-Vazquez, M., Marszalek, P. E., Oberhauser, A. F. & Fernandez, J. M. Atomic force microscopy captures length phenotypes in single proteins. Proc. Natl Acad. Sci. USA 96 , 11288–11292 (1999).
Garcia-Manyes, S., Brujic, J., Badilla, C. L. & Fernandez, J. M. Force-clamp spectroscopy of single-protein monomers reveals the individual unfolding and folding pathways of I27 and ubiquitin. Biophys. J. 93 , 2436–2446 (2007).
Randles, L. G., Rounsevell, R. W. & Clarke, J. Spectrin domains lose cooperativity in forced unfolding. Biophys. J. 92 , 571–577 (2007).
Tapia-Rojo, R., Alonso-Caballero, A. & Fernandez, J. M. Talin folding as the tuning fork of cellular mechanotransduction. Proc. Natl Acad. Sci. USA 117 , 21346–21353 (2020).
Stannard, A. et al. Molecular fluctuations as a ruler of force-induced protein conformations. Nano Lett. 21 , 2953–2961 (2021).
Li, H., Oberhauser, A. F., Fowler, S. B., Clarke, J. & Fernandez, J. M. Atomic force microscopy reveals the mechanical design of a modular protein. Proc. Natl Acad. Sci. USA 97 , 6527–6531 (2000).
Alegre-Cebollada, J., Badilla, C. L. & Fernandez, J. M. Isopeptide bonds block the mechanical extension of pili in pathogenic Streptococcus pyogenes . J. Biol. Chem. 285 , 11235–11242 (2010).
Chen, X., Zaro, J. L. & Shen, W. C. Fusion protein linkers: property, design and functionality. Adv. Drug Deliv. Rev. 65 , 1357–1369 (2013).
van Rosmalen, M., Krom, M. & Merkx, M. Tuning the flexibility of glycine-serine linkers to allow rational design of multidomain proteins. Biochemistry 56 , 6565–6574 (2017).
Li, C., Goryaynov, A. & Yang, W. The selective permeability barrier in the nuclear pore complex. Nucleus 7 , 430–446 (2016).
Lin, D. H. & Hoelz, A. The structure of the nuclear pore complex (an update). Annu. Rev. Biochem. 88 , 725–783 (2019).
Liashkovich, I., Meyring, A., Oberleithner, H. & Shahin, V. Structural organization of the nuclear pore permeability barrier. J. Control. Release 160 , 601–608 (2012).
Lowe, A. R. et al. Importin-beta modulates the permeability of the nuclear pore complex in a Ran-dependent manner. eLife 4 , e04052 (2015).
Hulsmann, B. B., Labokha, A. A. & Gorlich, D. The permeability of reconstituted nuclear pores provides direct evidence for the selective phase model. Cell 150 , 738–751 (2012).
Makise, M. et al. The Nup153-Nup50 protein interface and its role in nuclear import. J. Biol. Chem. 287 , 38515–38522 (2012).
Kowalczyk, S. W. et al. Single-molecule transport across an individual biomimetic nuclear pore complex. Nat. Nanotechnol. 6 , 433–438 (2011).
Fahrenkrog, B. et al. Domain-specific antibodies reveal multiple-site topology of Nup153 within the nuclear pore complex. J. Struct. Biol. 140 , 254–267 (2002).
Lim, R. Y. et al. Nanomechanical basis of selective gating by the nuclear pore complex. Science 318 , 640–643 (2007).
Cardarelli, F., Lanzano, L. & Gratton, E. Capturing directed molecular motion in the nuclear pore complex of live cells. Proc. Natl Acad. Sci. USA 109 , 9863–9868 (2012).
Colvin, K. M. et al. PelA deacetylase activity is required for Pel polysaccharide synthesis in Pseudomonas aeruginosa . J. Bacteriol. 195 , 2329–2339 (2013).
Liashkovich, I. et al. Clathrin inhibitor Pitstop-2 disrupts the nuclear pore complex permeability barrier. Sci. Rep. 5 , 9994 (2015).
Labokha, A. A. et al. Systematic analysis of barrier-forming FG hydrogels from Xenopus nuclear pore complexes. EMBO J. 32 , 204–218 (2013).
Marg, A. et al. Nucleocytoplasmic shuttling by nucleoporins Nup153 and Nup214 and CRM1-dependent nuclear export control the subcellular distribution of latent Stat1. J. Cell Biol. 165 , 823–833 (2004).
Port, S. A. et al. Structural and functional characterization of CRM1-Nup214 interactions reveals multiple FG-binding sites involved in nuclear export. Cell Rep. 13 , 690–702 (2015).
Miralles, F., Posern, G., Zaromytidou, A. I. & Treisman, R. Actin dynamics control SRF activity by regulation of its coactivator MAL. Cell 113 , 329–342 (2003).
Mouilleron, S., Langer, C. A., Guettler, S., McDonald, N. Q. & Treisman, R. Structure of a pentavalent G-actin*MRTF-A complex reveals how G-actin controls nucleocytoplasmic shuttling of a transcriptional coactivator. Sci. Signal 4 , ra40 (2011).
Olson, E. N. & Nordheim, A. Linking actin dynamics and gene transcription to drive cellular motile functions. Nat. Rev. Mol. Cell Biol. 11 , 353–365 (2010).
Connelly, J. T. et al. Actin and serum response factor transduce physical cues from the microenvironment to regulate epidermal stem cell fate decisions. Nat. Cell Biol. 12 , 711–718 (2010).
Muthukumar, M. in Polymer Translocation 354 (CRC Press, 2011).
Matsuda, A. & Mofrad, M. R. K. Free energy calculations shed light on the nuclear pore complex’s selective barrier nature. Biophys. J. 120 , 3628–3640 (2021).
Milles, S. & Lemke, E. A. Single molecule study of the intrinsically disordered FG-repeat nucleoporin 153. Biophys. J. 101 , 1710–1719 (2011).
Lim, R. Y., Ullman, K. S. & Fahrenkrog, B. Biology and biophysics of the nuclear pore complex and its components. Int. Rev. Cell Mol. Biol. 267 , 299–342 (2008).
Fei, X., Bell, T. A., Barkow, S. R., Baker, T. A. & Sauer, R. T. Structural basis of ClpXP recognition and unfolding of ssrA-tagged substrates. eLife 9 , e61496 (2020).
Sato, T., Esaki, M., Fernandez, J. M. & Endo, T. Comparison of the protein-unfolding pathways between mitochondrial protein import and atomic-force microscopy measurements. Proc. Natl Acad. Sci. USA 102 , 17999–18004 (2005).
Olivares, A. O., Kotamarthi, H. C., Stein, B. J., Sauer, R. T. & Baker, T. A. Effect of directional pulling on mechanical protein degradation by ATP-dependent proteolytic machines. Proc. Natl Acad. Sci. USA 114 , E6306–E6313 (2017).
Liu, J. et al. Intrinsic disorder in transcription factors. Biochemistry 45 , 6873–6888 (2006).
Brodsky, S., Jana, T. & Barkai, N. Order through disorder: the role of intrinsically disordered regions in transcription factor binding specificity. Curr. Opin. Struct. Biol. 71 , 110–115 (2021).
Bestembayeva, A. et al. Nanoscale stiffness topography reveals structure and mechanics of the transport barrier in intact nuclear pore complexes. Nat. Nanotechnol. 10 , 60–64 (2015).
Sakiyama, Y., Mazur, A., Kapinos, L. E. & Lim, R. Y. Spatiotemporal dynamics of the nuclear pore complex transport barrier resolved by high-speed atomic force microscopy. Nat. Nanotechnol. 11 , 719–723 (2016).
Ho, C. Y. et al. Lamin A/C and emerin regulate MKL1-SRF activity by modulating actin dynamics. Nature 497 , 507–511 (2013).
Popa, I. et al. Force dependency of biochemical reactions measured by single-molecule force-clamp spectroscopy. Nat. Protoc. 8 , 1261–1276 (2013).
Popa, I. et al. A HaloTag anchored ruler for week-long studies of protein dynamics. J. Am. Chem. Soc. 138 , 10546–10553 (2016).
Download references
Acknowledgements
This work was supported in part by the Francis Crick Institute, which receives its core funding from Cancer Research UK (CC0102), the UK Medical Research Council (CC0102) and the Wellcome Trust (CC0102). Cell lines used in this work were provided by the Cell Services of the Francis Crick Institute. The wound-healing assay was performed using equipment from the High Throughput Screening facility of the Francis Crick Institute. R.T.-R. is recipient of a King’s Prize Fellowship. T.Z. is recipient of a CSC-King’s doctoral studentship and funding through the KCL British Heart Foundation Centre of Research Excellence. This work is supported by the European Commission (Mechanocontrol, grant agreement 731957), BBSRC sLoLa (BB/V003518/1), a Leverhulme Trust Research Leadership Award (RL 2016-015), a Wellcome Trust Investigator Award (212218/Z/18/Z) and a Royal Society Wolfson Fellowship (RSWF/R3/183006) to S.G.-M.
Open Access funding provided by The Francis Crick Institute.
Author information
These authors contributed equally: Fani Panagaki, Rafael Tapia-Rojo.
Authors and Affiliations
Single Molecule Mechanobiology Laboratory, The Francis Crick Institute, London, UK
Fani Panagaki, Rafael Tapia-Rojo, Tong Zhu, Natalie Milmoe, Patricia Paracuellos, Stephanie Board, Marc Mora, Jane Walker, Elvira Infante & Sergi Garcia-Manyes
Department of Physics, Randall Centre for Cell and Molecular Biophysics, Centre for the Physical Science of Life and London Centre for Nanotechnology, King’s College London, London, UK
Fani Panagaki, Rafael Tapia-Rojo, Tong Zhu, Natalie Milmoe, Patricia Paracuellos, Stephanie Board, Marc Mora, Jane Walker, Elena Rostkova, Andrew Stannard, Elvira Infante & Sergi Garcia-Manyes
You can also search for this author in PubMed Google Scholar
Contributions
F.P., R.T.-R. and S.G.-M. designed the research. F.P., T.Z. and N.M. conducted and analysed the cellular optogenetic experiments. R.T.-R. analysed the cellular translocation data. R.T.-R. and M.M. conducted single-molecule mechanical experiments and analysed the data. S.B., J.W., E.R. and T.Z. engineered protein constructs for cellular experiments. P.P. conducted the biochemistry dot blot experiments. S.B. and J.W. expressed and purified protein constructs for single-molecule experiments. E.I. and A.S. conducted preliminary experiments and analysis. R.T.-R. and S.G.-M. wrote the paper. All authors contributed to revising and editing the manuscript.
Corresponding author
Correspondence to Sergi Garcia-Manyes .
Ethics declarations
Competing interests.
The authors declare no competing interests.
Peer review
Peer review information.
Nature Physics thanks Ulrich Kubitscheck, Victor Shahin and the other, anonymous, reviewer(s) for their contribution to the peer review of this work.
Additional information
Publisher’s note Springer Nature remains neutral with regard to jurisdictional claims in published maps and institutional affiliations.
Extended data
Extended data fig. 1 translocation kinetics of the ig27 v13p polyprotein..
(A) Nucleus-to-cytoplasm time course localization of the construct NLS-(Ig27 V13P ) X -mCherry-LEXY, being X the number of Ig27 V13P (V13P) domains. (B) Import rate constants for the V13P polyproteins. Each construct was measured on at least three independent experiments, with n = 105 (V13P); n = 138 (V13P) 2 ; n = 132 (V13P) 3 ; n = 88 (V13P) 4 ; n = 108 (V13P) 5 ; n = 80 (V13P) 6 ; n = 55 (V13P) 7 ; n = 22 (V13P) 8 . Horizontal bars indicate the average import rate constant, and vertical bars the SEM.
Extended Data Fig. 2 Translocation kinetics of the Ig27 WT polyprotein.
(A) Nucleus-to-cytoplasm time course of the localization of a NLS-(Ig27 W T ) X -mCherry-LEXY construct, being X the number of Ig27 WT (WT) domains. (B) Import rate constants for the Ig27 WT polyproteins. Each construct was measured on at least three independent experiments, with n = 249 (WT); n = 115 (WT) 2 ; n = 171 (WT) 3 ; n = 159 (WT) 4 ; n = 85 (WT) 5 ; n = 68 (WT) 6 ; n = 61 (WT) 7 ; n = 27 (WT) 8 . Horizontal bars indicate the average import rate constant, and vertical bars the SEM.
Extended Data Fig. 3 Translocation kinetics of the Ig27 Y9P polyprotein.
(A) Nucleus-to-cytoplasm time course localization of the NLS-(Ig27 Y9P ) X -mCherry-LEXY construct, being X the number of Ig27 Y9P (Y9P) domains. (B) Import rate constants for the Y9P polyproteins. Each construct was measured on at least three independent experiments, with n = 78 (Y9P); n = 127 (Y9P) 2 ; n = 83 (Y9P) 3 ; n = 58 (Y9P) 4 ; n = 50 (Y9P) 5 ; n = 56 (Y9P) 6 ; n = 40 (Y9P) 7 ; n = 27 (Y9P) 8 . Horizontal bars indicate the average import rate constant, and vertical bars the SEM.
Extended Data Fig. 4 The (R16) 2 -(Ig27 WT ) 2 and (Ig27 WT ) 2 -(R16) 2 specular polyprotein constructs are mechanically equivalent.
(A) Schematics of the (R16) 2 -(Ig27 WT ) 2 polyprotein construct under force. (B) Representative AFM force-extension unfolding trajectory of the (R16) 2 -(Ig27 WT ) 2 construct. The protein domains unfold following their mechanical hierarchy. The two first unfolding events, fingerprinted by an unfolding force ( F U ) of ∼ 35 pN and an increase in contour length ( ΔL C ) of ∼ 37 nm, correspond to the unfolding of the two mechanically labile R16 domains. The two subsequent events, hallmarked by F U ∼ 220 pN with ΔL C ∼ 27 nm, correspond to the unfolding of the stiffer Ig27 WT . (C) Scatter plot of unfolding force ( F U ) versus increase in contour length ( ΔL C ) for all unfolding events detected on the AFM force–extension trajectories for the (R16) 2 -(Ig27 WT ) 2 construct. Two clusters of events can be clearly identified by their unique F U and ΔL C signature, a first one with F U = 33 ± 12 pN and ΔL C = 37 ± 4 nm corresponding to the unfolding of the R16 domains (magenta), and a second one with F U = 204 ± 20 pN and ΔL C = 27 ± 2 nm corresponding to the Ig27 WT domains. Data collected from n = 61 (R16), n = 78 (Ig27 WT ), unfolding events from 41 individual force-extension unfolding trajectories. (D-F) Schematics, force–extension trace, and F U versus ΔL C scatter plot for the (Ig27 W T ) 2 -(R16) 2 construct, showing a first cluster of events characterized by F U = 35 ± 12 pN, and ΔL C = 35 ± 3 nm (R16, magenta) and a second one with F u = 222 ± 25 pN and ΔL C = 28 ± 2 nm (Ig27 W T , grey). When pulled in a single-molecule AFM, both specular constructs are mechanically indistinguishable. Data collected from n = 48 (R16), n = 68 (WT), unfolding events from 43 force-extension individual trajectories measured at 400 nm/s.
Extended Data Fig. 5 Polyproteins exhibiting a low mechanical stability domain at the N-terminus wi translocate faster to the nucleus.
( A ) Left: Representative confocal images of U2OS cells 30 min in the recovery phase. Scale bar 10 µm. Right: Average time courses of the relative nucleus-to-cytoplasm localization of (upper) (R16) 2 -WT and WT-(R16) 2 , and (lower) (R16) 2 -(WT) 2 and (WT) 2 -(R16) 2 protein constructs. (B) Protein import rate constants (mean ± SEM) calculated from the accumulation curves. (R16) 2 -WT ( k I = 1.29 ± 0.06 ks −1 , n = 69); WT-(R16) 2 ( k I = 1.02 ± 0.07 ks −1 , n = 42); (R16) 2 -(WT) 2 ( k I = 1.34 ± 0.03 ks −1 , n = 217); (WT) 2 -(R16) 2 , ( k I = 1.14 ± 0.03 ks −1 , n = 208). Significance levels for two-tailed Mann-Whitney non-parametric test. *** P ≤ 0.001, **** P ≤ 0.0001 (R16) 2 -(WT) 2 vs. (WT) 2 -(R16) 2 , P = 9.92 × 10 −3 ; (R16) 2 -(WT) 2 vs. (WT) 2 -(R16) 2 , P = 1.06 × 10 −4 . All points and bar plots indicate mean ± SEM.
Extended Data Fig. 6 The addition of a (GS) 2 tag accelerates nuclear import triggered by the addition of four (GS-amino acid-tag depends on the mechanical stability of the protein cargo.
(A) Left: Representative confocal images of U2OS cells 30 min in the recovery phase. Scale bar 10 µm. Right: Average time courses of the relative nucleus-to-cytoplasm localization of (upper) V13P and (GS) 2 -V13P; (middle) WT and (GS) 2 -WT; (lower) Y9P and (GS) 2 -Y9P. (B) Protein import rate constants (mean ± SEM) calculated from the accumulation curves. V13P ( k I = 1.80 ± 0.09 ks −1 , n = 160); (GS) 2 -V13P ( k I = 1.90 ± 0.05 ks −1 , n = 128); WT ( k I = 1.62 ± 0.05 ks −1 , n = 249); (GS) 2 -WT ( k I = 1.83 ± 0.04 ks −1 , n = 196); Y9P ( k I = 1.09 ± 0.03 ks −1 , n = 278); (GS) 2 -Y9P ( k I = 1.34 ± 0.04 ks −1 , n = 109). Significance levels for two-tailed Mann-Whitney non-parametric test ** P ≤ 0.001, *** P ≤ 0.0001, **** P ≤ 0.0001. V13P vs. (GS) 2 -V13P, P = 8.98 × 10 −3 ; WT vs. (GS) 2 -WT, P = 1.17 × 10 −4 ; Y9P vs. (GS) 2 -Y9P, P = 4.34 × 10 −5 . (C) Plot of the percentage acceleration induced by the (GS) 2 addition against the unfolding force of the Ig27 variants measured in AFM force-extension experiments ( v = 400 nm/s). All points and bar plots indicate mean ± SEM.
Extended Data Fig. 7 Addition of an unstructured GS-peptide tag accelerates the rate of nuclear shuttling of cargos of large mass and extremely high mechanical stability.
(A) Left: Representative confocal images of U2OS cells 30 min in the recovery phase. Scale bar 10 µm. Right: Average time courses of the relative nucleus-to-cytoplasm localization of Spy0128-(WT) 2 , (GS) 8 -Spy0128-(WT) 2 and (GS) 25 -Spy0128-(WT) 2 . The (GS)-tag accelerates the nuclear import kinetics of large protein cargos with extreme mechanical stability. (B) Protein import rate constants (mean ± SEM) calculated from the accumulation curves. Spy0128-(WT) 2 ( k I = 0.56 ± 0.03 ks −1 , n = 104); (GS) 8 -Spy0128-(WT) 2 ( k I = 0.83 ± 0.08 ks −1 , n = 43); (GS) 25 -Spy0128-(WT) 2 ( k I = 0.65 ± 0.04 ks −1 , n = 92). Significance levels for two-tailed Mann-Whitney non-parametric test NS P > 0.05, * P ≤ 0.05. Spy0128-(WT) 2 vs. (GS) 8 -Spy0128-(WT) 2 , P = 0.01; (GS) 8 -Spy0128-(WT) 2 vs. (GS) 50 -Spy0128-(WT) 2 , P = 0.07; Spy0128-(WT) 2 vs. (GS) 50 -Spy0128-(WT) 2 , P = 0.21. All points and bar plots indicate mean ± SEM.
Extended Data Fig. 8 Knockdown of Nup153 decreases nuclear import kinetics but does not affect NPC mechanosensitivity.
(A) Representative confocal images of U2OS cells 30 min in the recovery phase, scale bar 10 µm. (B) Average time courses of the relative nucleus-to-cytoplasm localization of V13P and WT under normal conditions (siNT) and Nup153 knockdown (siNup153). (C) Import rates calculated from fits to the recovery time courses. V13P (siNT) ( k I = 2.32 ± 0.09 ks −1 , n = 82), V13P (siNup153) ( k I = 1.35 ± 0.04 ks −1 , n = 100); WT (siNT) ( k I = 1.61 ± 0.05 ks −1 , n = 147), WT (siNup153) ( k I = 1.22 ± 0.05 ks −1 , n = 118). Significance levels for two-tailed Mann-Whitney non-parametric test NS > 0.05, *** P ≤ 0.001, **** P ≤ 0.0001. siNT V13P vs. siNup153 V13P, P = 3.21 × 10 −29 ; siNT WT vs. siNup153 WT, P = 9.80 × 10 −10 ; siNT V13P vs. siNT WT, P = 3.14 × 10 −11 . siNup153 V13P vs. siNup153 WT, P = 6.78 × 10 −5 . All points and bar plots indicate mean ± SEM.
Extended Data Fig. 9 Mechano-directionality in the nuclear import of mechanosensitive myocardin-related transcription factor (MRTFA).
(A) Representative confocal microscopy image gallery of U2OS doubly transfected with R16-Y9P-MRTFA-GFP – MRTFA-mCherry (left) and Y9P-R16-MRTFA-GFP – MRTFA-mCherry (right) at different time points after serum stimulation, scale bar 10 µm. The mCherry-tagged MRTFA is used for normalizing the nuclear import kinetics in each individual cell. (B) Normalized average time courses of the relative nucleus-to-cytoplasm localization of R16-Y9P-MRTFA and Y9P-R16-MRTFA. The average time course is normalized by the average nucleus-to-cytoplasm accumulation ( K e ) of the co-translocating MRTFA-mCherry construct. (C) Associated normalized nuclear import rates, R16-Y9P-MRTFA ( \({\widetilde{k}}_{I}\) = 0.90 ± 0.08 , n = 35); Y9P-R16-MRTFA ( \({\widetilde{k}}_{I}\) = 0.70 ± 0.06 , n = 25), where \({\widetilde{k}}_{I}\) is the normalized import rate calculated as k I (GFP)/ k I (mCherry). Significance levels for two-tailed Mann-Whitney non-parametric test **P ≤ 0.01, P = 4.45 × 10 −3 . All points and bar plots indicate mean ± SEM.
Supplementary information
Supplementary information.
Supplementary Figs. 1–19 and Tables.
Rights and permissions
Open Access This article is licensed under a Creative Commons Attribution 4.0 International License, which permits use, sharing, adaptation, distribution and reproduction in any medium or format, as long as you give appropriate credit to the original author(s) and the source, provide a link to the Creative Commons licence, and indicate if changes were made. The images or other third party material in this article are included in the article’s Creative Commons licence, unless indicated otherwise in a credit line to the material. If material is not included in the article’s Creative Commons licence and your intended use is not permitted by statutory regulation or exceeds the permitted use, you will need to obtain permission directly from the copyright holder. To view a copy of this licence, visit http://creativecommons.org/licenses/by/4.0/ .
Reprints and permissions
About this article
Cite this article.
Panagaki, F., Tapia-Rojo, R., Zhu, T. et al. Structural anisotropy results in mechano-directional transport of proteins across nuclear pores. Nat. Phys. (2024). https://doi.org/10.1038/s41567-024-02438-8
Download citation
Received : 08 February 2023
Accepted : 08 February 2024
Published : 13 May 2024
DOI : https://doi.org/10.1038/s41567-024-02438-8
Share this article
Anyone you share the following link with will be able to read this content:
Sorry, a shareable link is not currently available for this article.
Provided by the Springer Nature SharedIt content-sharing initiative
Quick links
- Explore articles by subject
- Guide to authors
- Editorial policies
Sign up for the Nature Briefing newsletter — what matters in science, free to your inbox daily.

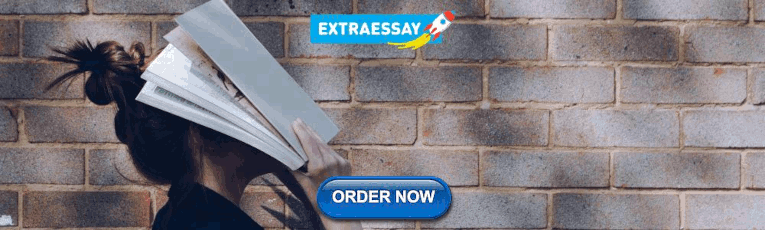
IMAGES
VIDEO
COMMENTS
A hypothesis test can either contain a directional hypothesis or a non-directional hypothesis: Directional hypothesis: The alternative hypothesis contains the less than ("<") or greater than (">") sign. This indicates that we're testing whether or not there is a positive or negative effect. Non-directional hypothesis: The alternative ...
Directional Hypothesis Examples. 1. Exercise and Heart Health. Research suggests that as regular physical exercise (independent variable) increases, the risk of heart disease (dependent variable) decreases (Jakicic, Davis, Rogers, King, Marcus, Helsel, Rickman, Wahed, Belle, 2016). In this example, a directional hypothesis anticipates that the ...
This can help streamline research efforts and increase the likelihood of finding meaningful results. Additionally, directional hypotheses are often used in experimental research, where researchers manipulate variables to observe their effects on outcomes. How to Write a Directional Hypothesis: A Step-by-Step Guide Step 1: Identify the Variables
A research hypothesis, in its plural form "hypotheses," is a specific, testable prediction about the anticipated results of a study, established at its outset. ... A non-directional hypothesis, also known as a two-tailed hypothesis, predicts that there is a difference or relationship between two variables but does not specify the direction of ...
Directional hypotheses, also known as one-tailed hypotheses, are statements in research that make specific predictions about the direction of a relationship or difference between variables. Unlike non-directional hypotheses, which simply state that there is a relationship or difference without specifying its direction, directional hypotheses ...
INTRODUCTION. Scientific research is usually initiated by posing evidenced-based research questions which are then explicitly restated as hypotheses.1,2 The hypotheses provide directions to guide the study, solutions, explanations, and expected results.3,4 Both research questions and hypotheses are essentially formulated based on conventional theories and real-world processes, which allow the ...
There are seven different types of research hypotheses. Simple Hypothesis. A simple hypothesis predicts the relationship between a single dependent variable and a single independent variable. Complex Hypothesis. A complex hypothesis predicts the relationship between two or more independent and dependent variables. Directional Hypothesis.
Abstract. Statistical hypothesis testing is common in research, but a conventional understanding sometimes leads to mistaken application and misinterpretation. The logic of hypothesis testing presented in this article provides for a clearer understanding, application, and interpretation. Key conclusions are that (a) the magnitude of an estimate ...
5.2 - Writing Hypotheses. The first step in conducting a hypothesis test is to write the hypothesis statements that are going to be tested. For each test you will have a null hypothesis ( H 0) and an alternative hypothesis ( H a ). When writing hypotheses there are three things that we need to know: (1) the parameter that we are testing (2) the ...
Definition: A directional hypothesis is a specific type of hypothesis statement in which the researcher predicts the direction or effect of the relationship between two variables. Key Features. 1. Predicts direction: Unlike a non-directional hypothesis, which simply states that there is a relationship between two variables, a directional ...
A research hypothesis is an assumption or a tentative explanation for a specific process observed during research. Unlike a guess, research hypothesis is a calculated, educated guess proven or disproven through research methods. ... Non-directional hypothesis: A non-directional hypothesis only claims an effect on the dependent variable. It does ...
A hypothesis (from the Greek, foundation) is a logical construct, interposed between a problem and its solution, which represents a proposed answer to a research question. It gives direction to the investigator's thinking about the problem and, therefore, facilitates a solution. Unlike facts and assumptions (presumed true and, therefore, not ...
Non-Directional Research Hypothesis. A non-directional hypothesis states that the means will be different, but does not specify which will be higher. In reality, there is rarely a situation in which we actually don't want one group to be higher than the other, so we will focus on directional research hypotheses. ...
It seeks to explore and understand a particular aspect of the research subject. In contrast, a research hypothesis is a specific statement or prediction that suggests an expected relationship between variables. It is formulated based on existing knowledge or theories and guides the research design and data analysis. 7.
The research can chose to make a specific prediction about what they feel will happen in their research (a directional hypothesis) or they can make a 'general,' 'less specific' prediction about the outcome of their research (a non-directional hypothesis). The type of prediction that a researcher makes is usually dependent on whether or ...
A directional research hypothesis would only cover the possibility that p>.5, and as a consequence the null hypothesis now becomes p≤.5. When this happens, we have what's called a one-sided test, and when this happens the critical region only covers one tail of the sampling distribution. This is illustrated in Figure \(\PageIndex{2}\).
Here you must be clear about the research question vs hypothesis. A research question is the very beginning point of writing an effective hypothesis. ... Non-directional Hypothesis: It involves an open-ended non-directional hypothesis that predicts that the independent variable will influence the dependent variable; however, the nature or ...
• Hypothesis or research questions: 1. May be used to compare the variables. 2. May be used to relate the variables. 3. May be used to describe the variables. • The research follows from a test of theory and the question and the hypothesis or the research questions are included in the theory.
Directional Hypothesis. A directional hypothesis is a statement that predicts the direction of the relationship between variables. For example, a researcher might predict that increasing the amount of exercise will result in a decrease in body weight. ... Guides research: A hypothesis provides a clear and specific direction for research. It ...
The directional hypothesis can also state a negative correlation, e.g. the higher the number of face-book friends, the lower the life satisfaction score ". Non-directional hypothesis: A non-directional (or two tailed hypothesis) simply states that there will be a difference between the two groups/conditions but does not say which will be ...
Abstract. The research hypothesis is needed for a sound and well-developed research study. The research hypothesis contributes to the solution of the research problem. Types of research hypotheses include inductive and deductive, directional and non-directional, and null and alternative hypotheses. Rejecting the null hypothesis and accepting ...
Conclusion. Formulating hypotheses is an essential step in the research process, guiding researchers in testing relationships between variables. Directional hypotheses offer specific predictions about the expected direction of the relationship, whereas non-directional hypotheses allow for more exploratory investigations without preconceived ...
A Level Psychology Topic Quiz - Research Methods. Quizzes & Activities. A directional hypothesis is a one-tailed hypothesis that states the direction of the difference or relationship (e.g. boys are more helpful than girls).
Terms to Know Directional hypothesis Nondirectional hypothesis Null hypothesis Research topic: broad and general, ask questions, who, what, where, when, how. Add geological , biological context or historical time) Narrow down your topic and focus on : a single even, a specific group, a limited time period, one cause or effect, one argument or viewpoint.
The integration of the EKC hypothesis posits a non-linear relationship between economic complexity and environmental degradation, reflecting an understanding that the relationship is not a straightforward, one-directional process but follows a more complex, inverted U-shaped curve.
Methods: In order to investigate the potential causal association between AD and prevalent oral diseases, we conducted a bi-directional two-sample Mendelian randomization analysis based on summary statistics from genome-wide association studies of AD (N = 63,926), as well as mouth ulcer (N = 461,103), oral cavity cancer (N = 4,151), and ...
Feature papers represent the most advanced research with significant potential for high impact in the field. A Feature Paper should be a substantial original Article that involves several techniques or approaches, provides an outlook for future research directions and describes possible research applications. ... Li, S.; Kang, R.; Wen, M.; Zu ...
This hypothesis was further corroborated for the mechanically labile spectrin R16 ... We conjecture that the directional, ... which receives its core funding from Cancer Research UK (CC0102), the ...