Thank you for visiting nature.com. You are using a browser version with limited support for CSS. To obtain the best experience, we recommend you use a more up to date browser (or turn off compatibility mode in Internet Explorer). In the meantime, to ensure continued support, we are displaying the site without styles and JavaScript.
- View all journals
- Explore content
- About the journal
- Publish with us
- Sign up for alerts
- Review Article
- Published: 02 May 2012
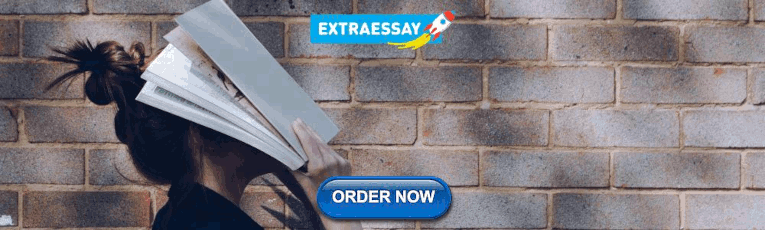
Mining electronic health records: towards better research applications and clinical care
- Peter B. Jensen 1 ,
- Lars J. Jensen 1 &
- Søren Brunak 1 , 2
Nature Reviews Genetics volume 13 , pages 395–405 ( 2012 ) Cite this article
24k Accesses
969 Citations
78 Altmetric
Metrics details
- Bioinformatics
- Clinical genetics
- Data mining
- Disease genetics
- Genetic databases
- Pharmacogenetics
Electronic health record (EHR) systems are increasingly being implemented all over the world, but represent a vast, underused data resource for biomedical research.
Structured EHR data, such as encoded diagnosis and medication information, are the easiest data sources to process, but advances in text-mining methods has made it possible to also use the narrative parts of patient records.
Statistical studies of the distribution and co-occurrence of clinical features in large collections of patient records enables identification of correlations between, for example, diseases (comorbidities) or between medications and adverse drug reactions.
Knowledge-discovery and machine-learning methods can be used both for discovering novel patterns in patient data and for classification and predictive purposes, such as outcome or risk assessment. This has the potential to extend current EHR decision support systems, which integrate available patient data with clinical guidelines to provide assistance to the physician at the point of care.
Research platforms built on EHR data, alone or coupled to genotype data, provide an inexpensive and timely way to sample relevant case and control cohorts based on relevant clinical features. As EHR and DNA databases become increasingly interlinked, genotype–phenotype association studies may be designed and conducted by re-using existing data.
The growing political focus on the adoption of EHR systems must be accompanied by funding and strategic research into data standards, interoperability and security. Legal matters such as data ownership, privacy and consent need to be addressed to find the right balance between public demands for autonomy and privacy, and manageable procedures for researchers to access data.
Fulfilling the full potential of electronic health data for scientific discovery and improved public health will require collaboration across stakeholders and research groups.
Clinical data describing the phenotypes and treatment of patients represents an underused data source that has much greater research potential than is currently realized. Mining of electronic health records (EHRs) has the potential for establishing new patient-stratification principles and for revealing unknown disease correlations. Integrating EHR data with genetic data will also give a finer understanding of genotype–phenotype relationships. However, a broad range of ethical, legal and technical reasons currently hinder the systematic deposition of these data in EHRs and their mining. Here, we consider the potential for furthering medical research and clinical care using EHR data and the challenges that must be overcome before this is a reality.
This is a preview of subscription content, access via your institution
Access options
Subscribe to this journal
Receive 12 print issues and online access
176,64 € per year
only 14,72 € per issue
Buy this article
- Purchase on Springer Link
- Instant access to full article PDF
Prices may be subject to local taxes which are calculated during checkout
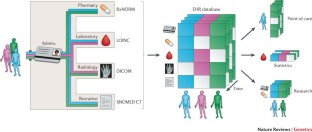
Similar content being viewed by others
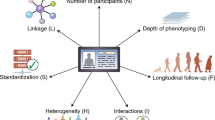
Axes of a revolution: challenges and promises of big data in healthcare
Smadar Shilo, Hagai Rossman & Eran Segal
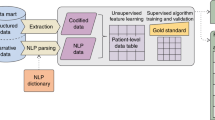
High-throughput phenotyping with electronic medical record data using a common semi-supervised approach (PheCAP)
Yichi Zhang, Tianrun Cai, … Katherine P. Liao
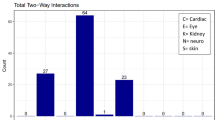
Cluster analysis and visualisation of electronic health records data to identify undiagnosed patients with rare genetic diseases
Daniel Moynihan, Sean Monaco, … Saumya Shekhar Jamuar
Stewart, W. F., Shah, N. R., Selna, M. J., Paulus, R. A. & Walker, J. M. Bridging the inferential gap: the electronic health record and clinical evidence. Health Aff. 26 , w181–w191 (2007).
Article Google Scholar
Hillestad, R. et al. Can electronic medical record systems transform health care? Potential health benefits, savings, and costs. Health Aff. 24 , 1103–1117 (2005).
Prokosch, H.-U. & Ganslandt, T. Perspectives for medical informatics. Reusing the electronic medical record for clinical research. Methods Inf. Med. 1 , 38–44 (2009).
Google Scholar
Kohane, I. S. Using electronic health records to drive discovery in disease genomics. Nature Rev. Genet. 12 , 417–428 (2011).
Article CAS PubMed Google Scholar
Kush, R. D., Helton, E., Rockhold, F. W. & Hardison, C. D. Electronic health records, medical research, and the Tower of Babel. N. Eng. J. Med. 358 , 1738–1740 (2008).
Article CAS Google Scholar
Taylor, P. When consent gets in the way. Nature 456 , 32–33 (2008).
Himmelstein, D. U., Wright, A. & Woolhandler, S. Hospital computing and the costs and quality of care: a national study. Am. J. Med. 123 , 40–46 (2010).
Article PubMed Google Scholar
Buntin, M. B., Burke, M. F., Hoaglin, M. C. & Blumenthal, D. The benefits of health information technology: a review of the recent literature shows predominantly positive results. Health Aff. 30 , 464–471 (2011).
Sarkar, I. N. Biomedical informatics and translational medicine. J. Transl. Med. 8 , 22 (2010).
Article PubMed PubMed Central Google Scholar
Blumenthal, D. Launching HITECH. N. Eng. J. Med. 362 , 382–385 (2010).
Hunter, J. The Innovative Medicines Initiative: a pre-competitive initiative to enhance the biomedical science base of Europe to expedite the development of new medicines for patients. Drug Discov. Today 13 , 371–373 (2008).
Coiera, E. Building a National Health IT System from the middle out. J. Am. Med. Inform. Assoc. 16 , 271–273 (2009).
Morrison, Z., Robertson, A., Cresswell, K., Crowe, S. & Sheikh, A. Understanding contrasting approaches to nationwide implementations of electronic health record systems: England, the USA and Australia. J. Healthc. Engin. 2 , 25–41 (2010).
Jha, A. K., DesRoches, C. M., Kralovec, P. D. & Joshi, M. S. A progress report on electronic health records in US hospitals. Health Aff. 29 , 1951–1957 (2010).
Serdén, L., Lindqvist, R. & Rosén, M. Have DRG-based prospective payment systems influenced the number of secondary diagnoses in health care administrative data? Health Policy 65 , 101–107 (2003).
Thygesen, L. C., Daasnes, C., Thaulow, I. & Bronnum-Hansen, H. Introduction to Danish (nationwide) registers on health and social issues: structure, access, legislation, and archiving. Scand. J. Public Health 39 , 12–16 (2011). An overview of Danish health and socio-economic registries and research possibilities as an example of extensive population-wide registration.
Frank, L. When an entire country is a cohort. Science 287 , 2398–2399 (2000).
Øyen, N. et al. Recurrence of congenital heart defects in families. Circulation 120 , 295–301 (2009).
Masutani, Y., MacMahon, H. & Doi, K. Computerized detection of pulmonary embolism in spiral CT angiography based on volumetric image analysis. IEEE Trans. Med. Imaging. 21 , 1517–1523 (2002).
Hoffman, M. The genome-enabled electronic medical record. J. Biomed. Inform. 40 , 44–46 (2007).
Sax, U. & Schmidt, S. Integration of genomic data in Electronic Health Records — opportunities and dilemmas. Methods Inform. Med. 44 , 546–550 (2005).
Meystre, S. M., Savova, G. K., Kipper-Schuler, K. C. & Hurdle, J. F. Extracting information from textual documents in the electronic health record: a review of recent research. Yearb. Med. Inform. 2008 , 128–144 (2008). An introduction to NLP and information extraction in the challenging clinical context, which also reviews the relevant research in the field.
Rosenbloom, S. T. et al. Data from clinical notes: a perspective on the tension between structure and flexible documentation. J. Am. Med. Inform. Assoc. 8 , 181–186 (2011). A summary of the conflicting views on structured and narrative health data in the context of how to produce valuable and reusable data.
Johnson, S. B. et al. An electronic health record based on structured narrative. J. Am. Med. Inform. Assoc. 15 , 54–65 (2008).
The International Health Terminology Standards Development Organisation. Systematized Nomenclature of Medicine-Clinical Terms (SNOMED CT). [online]
Bodenreider, O. The Unified Medical Language System (UMLS): integrating biomedical terminology. Nucleic Acids Res. 32 , D267–D270 (2004).
Article CAS PubMed PubMed Central Google Scholar
Savova, G. K. et al. Mayo clinical Text Analysis and Knowledge Extraction System (cTAKES): architecture, component evaluation and applications. J. Am. Med. Inform. Assoc. 17 , 507–513 (2010).
Zeng, Q. T. et al. Extracting principal diagnosis, co-morbidity and smoking status for asthma research: evaluation of a natural language processing system. BMC Med. Inform. Decis. Mak. 6 , 30 (2006).
Friedman, C., Alderson, P. O., Austin, J. H. M., Cimino, J. J. & Johnson, S. B. A general natural-language text processor for clinical radiology. J. Am. Med. Inform. Assoc. 1 , 161–174 (1994).
Friedman, C., Shagina, L., Lussier, Y. & Hripcsak, G. Automated encoding of clinical documents based on natural language processing. J. Am. Med. Inform. Assoc. 11 , 392–402 (2004).
Xu, H. et al. MedEx: a medication information extraction system for clinical narratives. J. Am. Med. Inform. Assoc. 17 , 19–24 (2010).
Ohno-Machado, L. Realizing the full potential of electronic health records: the role of natural language processing. J. Am. Med. Inform. Assoc. 18 , 539 (2011).
Evans, R. S. et al. A computer-assisted management program for antibiotics and other antiinfective agents. N. Eng. J. Med. 338 , 232–238 (1998).
Demner-Fushman, D., Chapman, W. W. & McDonald, C. J. What can natural language processing do for clinical decision support? J. Biomed. Inform. 42 , 760–772 (2009).
Bellazzi, R. & Zupan, B. Predictive data mining in clinical medicine: current issues and guidelines. Int. J. Med. Inform. 77 , 81–97 (2008). A review of the use of predictive methods in medicine with a special focus on temporal data.
Bellazzi, R., Ferrazzi, F. & Sacchi, L. Predictive data mining in clinical medicine: a focus on selected methods and applications. WIREs Data Mining Knowl. Discov. 1 , 416–430 (2011).
Lavrac, N. Selected techniques for data mining in medicine. Artif. Intell. Med. 16 , 3–23 (1999).
Degroot, V., Beckerman, H., Lankhorst, G. & Bouter, L. How to measure comorbidity. A critical review of available methods. J. Clin. Epidemiol. 56 , 221–229 (2003).
Hanauer, D., Rhodes, D. R. & Chinnaiyan, A. M. Exploring clinical associations using “-omics” based enrichment analyses. PLoS ONE 4 , e5203 (2009).
Roque, F. S. et al. Using electronic patient records to discover disease correlations and stratify patient cohorts. PLoS Comput. Biol. 7 , e1002141 (2011). Patient stratification and discovery of disease comorbidities and their causes at the molecular level using structured data and text mining on a psychiatric cohort.
Holmes, A. B. et al. Discovering disease associations by integrating electronic clinical data and medical literature. PLoS ONE 6 , e21132 (2011).
Hidalgo, C., Blumm, N., Barabási, A.-L. & Christakis, N. A dynamic network approach for the study of human phenotypes. PLoS Comput. Biol. 5 , e1000353 (2009).
Gibbons, R. D. et al. Post-approval drug safety surveillance. Annu. Rev. Public Health 2010 , 419–437 (2010).
Lopez-Gonzalez, E., Herdeiro, M. T. & Figueiras, A. Determinants of under-reporting of adverse drug reactions: a systematic review. Drug Saf. 32 , 19–31 (2009).
Wang, X., Hripcsak, G., Markatou, M. & Friedman, C. Active computerized pharmacovigilance using natural language processing, statistics, and electronic health records: a feasibility study. J. Am. Med. Inform. Assoc. 16 , 328–337 (2009). An example of how text mining of bulk EHR data can be used to uncover statistical correlations between clinical concepts, specifically between medications and ADEs.
Gini, R., Herings, R., Coloma, P. M., Schuemie, M. J. & Trifiro, G. Combining electronic healthcare databases in Europe to allow for large-scale drug safety monitoring : the EU-ADR Project. Pharmacoepidemiol. Drug Saf. 20 , 1–11 (2011).
Yao, L., Zhang, Y., Li, Y., Sanseau, P. & Agarwal, P. Electronic health records: implications for drug discovery. Drug Discov. Today 16 , 594–599 (2011).
Mullins, I. M. et al. Data mining and clinical data repositories: Insights from a 667,000 patient data set. Comput. Biol. Med. 36 , 1351–1377 (2006).
Wright, A., Chen, E. S. & Maloney, F. L. An automated technique for identifying associations between medications, laboratory results and problems. J. Biomed. Inform. 43 , 891–901 (2010).
Harpaz, R., Chase, H. S. & Friedman, C. Mining multi-item drug adverse effect associations in spontaneous reporting systems. BMC Bioinformatics 11 (Suppl. 9), S7 (2010).
Swanson, D. R. Fish oil, Raynaud's syndrome, and undiscovered public knowledge. Perspect. Biol. Med. 30 , 7–18 (1986).
Tsuruoka, Y., Miwa, M., Hamamoto, K., Tsujii, J. & Ananiadou, S. Discovering and visualizing indirect associations between biomedical concepts. Bioinformatics 27 , 111–119 (2011).
Oztekin, A., Delen, D. & Kong, Z. J. Predicting the graft survival for heart-lung transplantation patients: an integrated data mining methodology. Int. J. Med. Inform. 78 , e84–e96 (2009).
Delen, D., Walker, G. & Kadam, A. Predicting breast cancer survivability: a comparison of three data mining methods. Artif. Intell. Med. 34 , 113–127 (2005).
Kurt, I., Ture, M. & Kurum, A. T. Comparing performances of logistic regression, classification and regression tree, and neural networks for predicting coronary artery disease. Expert Syst. Appl. 34 , 366–374 (2008).
Valentino-Devries, J. May the best algorithm win. The Wall Street Journal [online] , (2011).
Ohlsson, M., Peterson, C. & Dictor, M. Using hidden Markov models to characterize disease trajectories. Proc. Neural Networks and Expert Systems in Medicine and Healthcare Conference 2001 , 324–326 (2001).
Chen, L. L., Blumm, N., Christakis, N. A., Barabási, A.-L. & Deisboeck, T. S. Cancer metastasis networks and the prediction of progression patterns. Br. J. Cancer 101 , 749–758 (2009).
Fu, T.-C. A review on time series data mining. Eng. Appl. Artif. Intell. 24 , 164–181 (2011).
Cao, H., Melton, G. B., Markatou, M. & Hripcsak, G. Use abstracted patient-specific features to assist an information-theoretic measurement to assess similarity between medical cases. J. Biomed. Inform. 41 , 882–888 (2008).
Melton, G. B. et al. Inter-patient distance metrics using SNOMED CT defining relationships. J. Biomed. Inform. 39 , 697–705 (2006).
Murphy, S. et al. Instrumenting the health care enterprise for discovery research in the genomic era. Genome Res. 19 , 1675–1681 (2009).
Murphy, S. N. et al. Serving the enterprise and beyond with informatics for integrating biology and the bedside (i2b2). J. Am. Med. Inform. Assoc. 17 , 124–130 (2010). A thorough description of the architecture and capabilities of the i2b2 research platform for biomedical research based on EHR data.
McCarty, C. A. et al. The eMERGE Network: A consortium of biorepositories linked to electronic medical records data for conducting genomic studies. BMC Med. Genomics 4 , 13 (2011).
Kho, A. N. et al. Electronic medical records for genetic research: results of the eMERGE consortium. Science Transl. Med. 3 , 79re1 (2011).
Schildcrout, J. S. et al. An analytical approach to characterize morbidity profile dissimilarity between distinct cohorts using electronic medical records. J. Biomed. Inform. 43 , 914–923 (2010).
Kurreeman, F. et al. Genetic basis of autoantibody positive and negative rheumatoid arthritis risk in a multi-ethnic cohort derived from electronic health records. Am. J. Hum. Genet. 88 , 57–69 (2011). The i2b2 platform put to use for case–control generation and study design based on EHR and DNA data in a rheumatoid arthritis project.
Kullo, I. J. et al. Complement receptor 1 gene variants are associated with erythrocyte sedimentation rate. Am. J. Hum. Genet. 89 , 131–138 (2011).
Kullo, I. J., Ding, K., Jouni, H., Smith, C. Y. & Chute, C. G. A genome-wide association study of red blood cell traits using the electronic medical record. PLoS ONE 5 , 9 (2010).
Denny, J. C. et al. Variants near FOXE1 are associated with hypothyroidism and other thyroid conditions: using electronic medical records for genome- and phenome-wide studies. Am. J. Hum. Genet. 89 , 529–542 (2011).
Ritchie, M. D. et al. Robust replication of genotype-phenotype associations across multiple diseases in an electronic medical record. Am. J. Hum. Genet. 86 , 560–572 (2010).
Perlis, R. H. et al. Using electronic medical records to enable large-scale studies in psychiatry: treatment resistant depression as a model. Psychol. Med. 42 , 41–50 (2012).
Kho, A. N. et al. Use of diverse electronic medical record systems to identify genetic risk for type 2 diabetes within a genome-wide association study. J. Am. Med. Inform. Assoc. 19 , 212–218 (2011).
Himes, B. E., Dai, Y., Kohane, I. S., Weiss, S. T. & Ramoni, M. F. Prediction of chronic obstructive pulmonary disease (COPD) in asthma patients using electronic medical records. J. Am. Med. Inform. Assoc. 16 , 371–379 (2009).
Roden, D. M. et al. Development of a large-scale de-identified DNA biobank to enable personalized medicine. Clin. Pharmacol. Ther. 84 , 362–369 (2008). A description of the technical, scientific and legal aspects of the development of an EHR–DNA linked research database with an opt-out consent model.
Denny, J. C. et al. PheWAS: demonstrating the feasibility of a phenome-wide scan to discover gene-disease associations. Bioinformatics 26 , 1205–1210 (2010). A demonstration of how EHR data linked with DNA data can be used in a reversal of the normal GWAS approach to search for disease phenotypes associated with SNPs.
Wilke, R. et al. The emerging role of electronic medical records in pharmacogenomics. Clin. Pharmacol. Ther. 89 , 379–386 (2011).
Al Mallah, A., Guelpa, P., Marsh, S. & van Rooij, T. Integrating genomic-based clinical decision support into electronic health records. Personalized Med. 7 , 163–170 (2010).
McCarty, C. A. & Wilke, R. A. Biobanking and pharmacogenomics. Pharmacogenomics 11 , 637–641 (2010).
Schwarz, U. I. et al. Genetic determinants of response to warfarin during initial anticoagulation. N. Eng. J. Med. 358 , 999–1008 (2008).
Onitilo, A. et al. Estrogen receptor genotype is associated with risk of venous thromboembolism during tamoxifen therapy. Breast Cancer Res. Treat. 115 , 643–650 (2009).
Lage, K. et al. Dissecting spatio-temporal protein networks driving human heart development and related disorders. Mol. Syst. Biol. 6 , 1–9 (2010).
Greenblum, S., Turnbaugh, P. J. & Borenstein, E. Metagenomic systems biology of the human gut microbiome reveals topological shifts associated with obesity and inflammatory bowel disease. Proc. Natl Acad. Sci. USA. 109 , 594–599 (2012).
Rzhetsky, A., Wajngurt, D., Park, N. & Zheng, T. Probing genetic overlap among complex human phenotypes. Proc. Natl Acad. Sci. USA. 104 , 11694–11699 (2007).
Goh, K.-I. et al. The human disease network. Proc. Natl Acad. Sci. USA. 104 , 8685–8690 (2007).
Park, J., Lee, D.-S., Christakis, N. A. & Barabási, A.-L. The impact of cellular networks on disease comorbidity. Mol. Syst. Biol. 5 , 262 (2009).
Ashley, E. A. et al. Clinical assessment incorporating a personal genome. Lancet 375 , 1525–1535 (2010).
Hood, L., Heath, J. R., Phelps, M. E. & Lin, B. Systems biology and new technologies enable predictive and preventative medicine. Science 306 , 640–643 (2004).
Galas, D. J. & Hood, L. Systems biology and emerging technologies will catalyze the transition from reactive medicine to predictive, personalized, preventive and participatory (P4) medicine. Interdisciplinary Bio Central 1 , 6 (2009).
Hall, M. A. Property, privacy, and the pursuit of interconnected electronic medical records. Iowa Law Review 2010 , 631–663 (2010).
Noble, S. et al. Feasibility and cost of obtaining informed consent for essential review of medical records in large-scale health services research. J. Health Serv. Res. Policy 14 , 77–81 (2009).
Kho, M. E., Duffett, M., Willison, D. J., Cook, D. J. & Brouwers, M. C. Written informed consent and selection bias in observational studies using medical records: systematic review. BMJ 338 , 1–8 (2009).
Hoffman, S. Balancing privacy, autonomy, and scientific needs in electronic health records research. Case Research Paper Series in Legal Studies [online] , (2011). An extensive summary of legal and ethical issues encountered in health research and their potential consequences for conducting scientific research.
Meystre, S. M., Friedlin, F. J., South, B. R., Shen, S. & Samore, M. H. Automatic de-identification of textual documents in the electronic health record: a review of recent research. BMC Med. Res. Methodol. 10 , 1–16 (2010).
Benitez, K. & Malin, B. Evaluating re-identification risks with respect to the HIPAA privacy rule. J. Am. Med. Inform. Assoc. 17 , 169–177 (2010).
Heeney, C., Hawkins, N., de Vries, J., Boddington, P. & Kaye, J. Assessing the privacy risks of data sharing in genomics. Public Health Genomics 14 , 17–25 (2011).
Homer, N. et al. Resolving individuals contributing trace amounts of DNA to highly complex mixtures using high-density SNP genotyping microarrays. PLoS Genet. 4 , e1000167 (2008).
Malin, B. & Sweeney, L. Re-identification of DNA through an automated linkage process. Proc. AMIA Symp. 2001 , 423–427 (2001).
Arumugam, M. et al. Enterotypes of the human gut microbiome. Nature 473 , 174–180 (2011).
Rothstein, M. A. Is deidentification sufficient to protect health privacy in research? Am. J. Bioeth. 10 , 3–11 (2010).
Begoyan, A. An overview of interoperability standards for electronic health records. In Integrated Design and Process Technology (IDPT-2007) (2007).
Goossen, W., Goossen-Baremans, A. & van der Zel, M. Detailed clinical models: a review. Healthc. Inform. Res. 16 , 201–214 (2010). An introduction to modelling and representation of clinical concepts and meaning, which is important for data interoperability.
Knaup, P., Bott, O., Kohl, C., Lovis, C. & Garde, S. Electronic patient records: moving from islands and bridges towards electronic health records for continuity of care. Yearb. Med. Inform. 2007 , 34–46 (2007).
Garde, S., Knaup, P., Hovenga, E. & Heard, S. Towards semantic interoperability for electronic health records. Methods Inf. Med. 46 , 332–343 (2007).
Wicks, P., Vaughan, T. E., Massagli, M. P. & Heywood, J. Accelerated clinical discovery using self-reported patient data collected online and a patient-matching algorithm. Nature Biotech. 29 , 411–414 (2011).
Aronson, R. Effective mapping of biomedical text to the UMLS Metathesaurus: the MetaMap program. Proc. AMIA Symp. 2001 , 17–21 (2001).
Uzuner, O., Goldstein, I., Luo, Y. & Kohane, I. Identifying patient smoking status from medical discharge records. J. Am. Med. Inform. Assoc. 15 , 14–24 (2008).
Uzuner, O. Recognizing obesity and comorbidities in sparse data. J. Am. Med. Inform. Assoc. 16 , 561–570 (2009).
Uzuner, O., Solti, I. & Cadag, E. Extracting medication information from clinical text. J. Am. Med. Inform. Assoc. 17 , 514–518 (2010).
Uzuner, O., South, B. R., Shen, S. & Duvall, S. L. 2010 i2b2/VA challenge on concepts, assertions, and relations in clinical text. J. Am. Med. Inform. Assoc. 18 , 552–557 (2011).
Fung, K. W., McDonald, C. & Bray, B. E. RxTerms - a drug interface terminology derived from RxNorm. Proc. AMIA Symp. 2008 , 227–231 (2008).
PubMed Central Google Scholar
Steindel, S. J. International classification of diseases, 10th edition, clinical modification and procedure coding system: descriptive overview of the next generation HIPAA code sets. J. Am. Med. Inform. Assoc. 17 , 274–282 (2010).
Download references
Acknowledgements
We thank U. Buddrus for kindly providing unpublished data on the adoption of EHR systems in Europe. Any errors in communicating these insights are the sole responsibility of the authors. The authors were supported in part by the Villum Kann Rasmussen Foundation, the Novo Nordisk Foundation and the Danish Research Council for Strategic Research.
Author information
Authors and affiliations.
NNF Center for Protein Research, Faculty of Health Sciences, University of Copenhagen, Copenhagen, Denmark
Peter B. Jensen, Lars J. Jensen & Søren Brunak
Department of Systems Biology, Center for Biological Sequence Analysis, Technical University of Denmark, Lyngby, Denmark
Søren Brunak
You can also search for this author in PubMed Google Scholar
Corresponding author
Correspondence to Søren Brunak .
Ethics declarations
Competing interests.
The authors declare no competing financial interests.
(CDS). Software systems providing support for decision making to physicians through the application of health knowledge and logical rules to patient data.
Central repositories of biological material that are mainly used for research. They facilitate the re-use of collected samples in different research projects.
(EHRs). In this review we do not distinguish between EHRs, Electronic Patient Records (EPRs) and Electronic Medical Records (EMRs).
Part of the American Recovery and Reinvestment act from 2009. The Health Information Technology for Economic and Clinical Health (HITECH) act allocates funding and attention to HIT infrastructure and electronic health record adoption and research in the United States.
The International Classification of Diseases (ICD) published by the World Health Organization. It has been translated into numerous languages.
A US government health insurance programme primarily covering people aged 65 yearsand older.
A measure of the accumulated disease burden for a patient. It is calculated as a weighted sum of 22 selected medical conditions that are assigned scores depending on the severity of the condition.
Monitoring of adverse drug events during clinical trials and after marketing in order to prevent harm to patients. It is typically based on statistical pattern-finding in databases of reported adverse events.
(ADE). Used in pharmacology to describe any unexpected or harmful event associated with a given medication.
The representation of objects (patients) as vectors in the space of all relevant features. Each dimension of the vector specifies the association of a patient with a certain feature.
A common task in statistical data exploration using measures of similarity between data points, network topology or other methods to group data points with similar characteristics together in clusters.
A measure of the similarity of two concepts in terms of their meaning or semantic content. Often quantified using topological measures of distance in an ontology of concepts, such as WordNet or Systematized Nomenclature of Medicine — Clinical Terms (SNOMED CT).
(eMERGE Network). An institutional network that is exploring the potential of electronic health record data in genetic and medical research. Participating institutions are: GroupHealth, Geisinger, Marshfield Clinic, Mayo Clinic, Mount Sinai School of Medicine, Northwestern University and Vanderbilt University.
The study of how genetic variants influence the effects of drugs on, for example, drug metabolism, efficacy and toxicity, with the goal of improving and personalizing drug therapy.
A research project initiated by the Veterans Affairs Office of Research and Development that is aimed at establishing a database with DNA and health record data from one million people. Participation is opt-in.
The Kaiser Permanente Research Project on Genes, Environment and Health (Kaiser RPGEH). This project aims to establish a research database with genetic data, environment data and health record data from 500,000 people. Participation is opt-in.
Rights and permissions
Reprints and permissions
About this article
Cite this article.
Jensen, P., Jensen, L. & Brunak, S. Mining electronic health records: towards better research applications and clinical care. Nat Rev Genet 13 , 395–405 (2012). https://doi.org/10.1038/nrg3208
Download citation
Published : 02 May 2012
Issue Date : June 2012
DOI : https://doi.org/10.1038/nrg3208
Share this article
Anyone you share the following link with will be able to read this content:
Sorry, a shareable link is not currently available for this article.
Provided by the Springer Nature SharedIt content-sharing initiative
This article is cited by
Prediction of sjögren’s disease diagnosis using matched electronic dental-health record data.
- Grace Gomez Felix Gomez
- Thankam P. Thyvalikakath
BMC Medical Informatics and Decision Making (2024)
- Daniel Moynihan
- Sean Monaco
- Saumya Shekhar Jamuar
Scientific Reports (2024)
Systematic Review of Machine Learning Applied to the Secondary Prevention of Ischemic Stroke
- Dongbao Qian
- Chunying Pang
Journal of Medical Systems (2024)
Knowledge Graph Embeddings for ICU readmission prediction
- Ricardo M. S. Carvalho
- Daniela Oliveira
- Catia Pesquita
BMC Medical Informatics and Decision Making (2023)
Association study between drug prescriptions and Alzheimer’s disease claims in a commercial insurance database
- Tong Shu Li
- Andrew I. Su
Alzheimer's Research & Therapy (2023)
Quick links
- Explore articles by subject
- Guide to authors
- Editorial policies
Sign up for the Nature Briefing newsletter — what matters in science, free to your inbox daily.

- Research article
- Open access
- Published: 26 February 2019
Patient perspectives on use of electronic health records for research recruitment
- Laura M. Beskow ORCID: orcid.org/0000-0002-9314-1915 1 ,
- Kathleen M. Brelsford 1 &
- Catherine M. Hammack 1
BMC Medical Research Methodology volume 19 , Article number: 42 ( 2019 ) Cite this article
3614 Accesses
12 Citations
16 Altmetric
Metrics details
EHR phenotyping offers the ability to rapidly assemble a precisely defined cohort of patients prescreened for eligibility to participate in health-related research. Even so, stakeholders in the process must still contend with the practical and ethical challenges associated with research recruitment. Patient perspectives on these matters are particularly important given that the success of research recruitment depends on patients’ willingness to participate.
We conducted 15 focus groups ( n = 110 participants) in four counties in diverse regions of the southeastern US: Appalachia, the Mississippi Delta, and the Piedmont area of North Carolina. Based on a hypothetical study of a behavioral intervention for type 2 diabetes, we asked about the acceptability and appropriateness of direct investigator versus physician-mediated contact with patients for research recruitment, and whether patients should be asked to opt in or opt out of further contact in response to recruitment letters.
For initial contact, nearly all participants said it would be acceptable for researchers to contact patients directly and three-fourths said that it would be acceptable for researchers to contact patients through their physicians. When we asked which would be most appropriate, a substantial majority chose direct contact. Themes that arose in the discussion included trust and transparency, decision-making power, the effect on research, and the effect on patient care. For response expectations, the vast majority of participants said both opt-in and opt-out would be acceptable—typically finding neither especially problematic and noting that both afford patients the opportunity to make their own decisions.
Conclusions
External validity relies heavily on researchers’ success enrolling eligible patients and failure to reach accrual targets is a costly and common barrier to advancing scientific knowledge. Our results suggest that patients recognize multiple advantages and disadvantages of different research recruitment strategies and place value on the implications not just for themselves, but also for researchers and healthcare providers. Our findings, including rich qualitative detail, contribute to the body of empirical and ethical literature on improving research recruitment and suggest specific ways forward as well as important areas for future research.
Peer Review reports
The widespread adoption and use of electronic health records (EHRs) [ 1 , 2 ], together with the development of “big data” tools to mine, assimilate, and analyze information [ 3 ], has led to the ability to identify cohorts of patients with precise attributes. This process, known as EHR phenotyping, applies algorithms to electronic data to classify patients based on exact constellations of information, such as demographics, diagnoses, procedures, lab values, vital signs, medications, and environmental and behavioral factors [ 4 , 5 , 6 , 7 ].
EHR phenotyping is used to support an array of research, including studies involving contact with patients for the purposes of research recruitment [ 8 , 9 , 10 , 11 , 12 , 13 ]. After querying EHR data to identify those who meet inclusion/exclusion criteria, researchers could approach patients about their interest in participation either directly or through their physicians, asking for either an opt-in or opt-out response regarding further recruitment communication. Each of these strategies raises both ethical and practical challenges [ 14 , 15 ] and studies suggest that institutional policies vary considerably [ 16 , 17 , 18 ]. Further, little is known about patients’ opinions concerning these different strategies. Their perspectives are particularly important given that the ultimate success of recruitment endeavors depends on building and maintaining patients’ trust and willingness to participate in research.
To contribute to the development of ethical policy and practice, we conducted a mixed methods study in diverse regions of the southeastern US—including Appalachia, the Mississippi Delta, and the Piedmont area of North Carolina—to better understand patients’ attitudes about research use of their EHRs. This included focus group research exploring the acceptability and appropriateness of direct investigator versus physician-mediated contact for research recruitment, and opt-in versus opt-out response expectations.
Participants
We conducted focus groups with patients in four locations selected to maximize demographic diversity (Additional file 1 : Table S1–1): Cabarrus County (CC), North Carolina; Durham County (DC), North Carolina; Mingo County (MC), West Virginia; and Quitman County (QC), Mississippi. Additional focus groups were conducted in Cabarrus County with research participants enrolled in the MURDOCK Study (MU), a population-based biobank [ 19 ].
We worked with commercial vendors to mail recruitment letters to a random selection of residential addresses ( n = 3000) in each county. The sampling frames included PO boxes designated as “only way we get mail” and rural route delivery addresses; it excluded business addresses, educational housing, and addresses identified as vacant or seasonal. Quitman county did not have 3000 addresses meeting these criteria and thus our mailing was sent to all eligible addresses ( n = 2447) in the area. In addition, in Quitman and Mingo counties, both of which are sparsely-populated rural areas, we also used word-of-mouth [ 20 ], i.e. , invited enrolled participants to share study information with others who might be interested. MURDOCK study staff mailed letters to a random selection of participants and also reached out through social media. Among individuals who contacted us to learn more about the study, we used purposive selection and scheduling to maximize demographic diversity within groups.
English-speaking adults who had seen a healthcare provider in the past two years were eligible. Those who had participated in more than two medical research studies in the past year or whose jobs involved clinical research of regular access to patient medical records were excluded.
Instrument development
We reviewed relevant literature (as cited throughout this paper) and examined existing patient-facing materials from reputable sources (e.g. , https://www.healthit.gov/how-do-i/individuals , https://www.hhs.gov/hipaa/for-individuals/index.html ) to develop focus group instrumentation, including:
A questionnaire (Additional file 1 : Table S1–2) using established measures to elicit basic demographic information, trust in health care providers and organizations, attitudes toward research, and general level of concern about health information privacy.
Educational videos to convey basic information needed to support opinion formation. The first described EHRs, research use of clinical records and data, and oversight mechanisms and privacy protections employed in such research. The second introduced a hypothetical study (Table 1 ) as the basis for group discussion. In addition to using plain language principles [ 21 ] and attending to best practices for the verbal, visual, and textual display of information [ 22 , 23 ], these narrated slide shows were reviewed by a multidisciplinary group of expert advisors and an endocrinologist to assess accuracy and neutrality. We then undertook several rounds of cognitive testing to improve comprehension and clarity.
A moderator’s guide to explore participants’ opinions regarding several vignettes in which researchers conducting the hypothetical study might have reason to consider contact with patients. Participants’ reactions to vignettes involving the discovery of discrepant information (e.g. , a potentially undiagnosed health condition) were reported elsewhere [ 24 ]; here we report reactions to a vignette about contact for the purpose of research recruitment.
A worksheet for participants to record their individual responses to key closed-ended questions prior to full group discussion, with the goal of enhancing engagement and generating limited quantitative data for comparison [ 25 ].
We conducted two pilot focus groups to test and finalize the instruments (available upon request), process, and logistics.
Data collection
We conducted 15 focus groups between August 2015 and February 2016. One research team member moderated all the groups.
Participants completed the questionnaire, watched the educational videos, and were prompted often to ask any clarifying questions. For the research recruitment vignette (Table 2 ), the moderator introduced two general ways researchers could contact patients to invite them to participate in the hypothetical study: directly or through their physicians. To encourage participants to consider a wide range of views before forming their own opinions, the group was asked to generate a list of the advantages and disadvantages of each approach from patient, researcher, and provider perspectives. The moderator then led a group conversation during which participants answered questions on their individual worksheets and discussed their opinions regarding the acceptability of each approach, as well as which approach would be most appropriate .
We similarly described opt-in and opt-out as the two general responses that could be requested during initial contact (Table 2 ). We asked the group to generate the advantages and disadvantages of each, and then address the acceptability of each, individually on their worksheets and then in group discussion.
Data analysis
We used NVivo 11 (QSR International) and a standard iterative process [ 26 ], using two independent coders who reached > 80% inter-coder agreement, to code and analyze transcribed audio recordings of the focus groups. See Additional file 1 : Table S1–3 for additional qualitative methods details.
We conducted basic descriptive and comparative analyses of questionnaire and worksheet data, including Fisher’s exact test to examine differences by study location, using Stata 14.2 (StataCorp).
Participant characteristics
We conducted 15 focus groups comprising participants ( n = 110) representing substantial diversity (Table 3 ). Compared to census data, characteristics broadly mirrored those of the target counties, although our sample was slightly more educated and included a larger proportion of women and older individuals. Although many characteristics varied by study location, statistically significant differences were found only in self-reported race and having a regular healthcare provider. (See Additional file 2 : Table S2–1 for data on trust and attitudes toward research.)
Initial contact with prospective participants: Cross-cutting themes
We asked focus group participants their opinions about different ways researchers conducting a hypothetical study (Table 1 ) could contact potentially eligible patients—directly or through their physicians (Table 2 )—about their interest in participating. Throughout these discussions, four themes emerged: trust and transparency, decision-making power, the effect on research, and the effect on patient care. (Narrative segments presented in all sections are exemplary of frequently mentioned ideas unless stated otherwise; additional examples are provided in Additional file 2 : Table S2–2.)
Trust and transparency
Participants frequently raised issues of trust surrounding initial research contact: “Well, it gets down to the trust issue. How much would I trust these people?” (CC2_P3) They identified several advantages associated with contact by a known source, such as their physician, including the benefit of having an established relationship:
CC1_P3: It’d be less intimidating if a doctor asked you to participate in [a study], as opposed to the researcher. ‘Cause you know the doctor and you trust him, most of what he says. The doctor knows more about you.
Based on this existing relationship, they expected a physician “knows what patients are dealing with” (QC1_P6) and could predict “how they would react if you contact them” (MU1_P5) about research participation. Thus, physicians might be able to help circumvent negative reactions; for example, the possibility of patients misinterpreting the reason for research contact:
QC2_P2: Might scare ‘em.
QC2_P1: Might scare ‘em to death.
Moderator: It might scare people?
QC2_P1: Cause anxiety, you know. “I’m not sure I wanna be in this. Do you think it’s all right? Should I go talk to my doctor?” Some people are just nervous types and that would just give them something to think about, really. That might not be good for ‘em.
Another advantage of contact by a known source was the potential to reduce privacy concerns: “I don’t wanna get a call from someone I don’t know or an email from somebody I don’t know. ‘Cause then it feels like your privacy’s been invaded.” (DC2_P7).
Many participants extended these advantages to trusted healthcare organizations and entities known to patients as having a good reputation:
CC1_P1: I’ve been involved in studies where I did not go through my physician. And depending on who’s doing the study, if they have a good track record, then I’m comfortable with it.
Some suggested that even when a recruitment letter is not sent directly by a known source, it may be sufficient if the letter establishes a clear link to a trusted person or entity:
MU1_P8: You could [send the letter] on behalf of the doctor, couldn’t you? “I’m [Name] from the Duke study, on behalf of Dr. Such-and-Such.” And then you have a connection.
Indeed, transparency regarding the circumstances that led to patients being contacted about research was a common theme. The exchange below, for instance, hints at potential differences of opinion concerning how explicit versus obscure the recruitment letter should—or could—be about what researchers already know about patients:
CC3_P1: I have a question about contacting patients directly. When you do that, do you let them know that you know they have diabetes? Or do you just send out a generic “If you have it…”, [so patients] don’t know that you know?
CC3_P5: “Just a shot in the dark here: If you happen to have diabetes…”.
CC3_P4: We’re in the South.
CC3_P1: To be like, “We know you have it. Do you want to…” Or, you just happened to get something in the mail and be like, “Oh, okay. Yeah. I have that”—then you think everybody else got one too.
CC3_P4: “What a coincidence. I do have diabetes.”
Some participants suggested that raising awareness about the use of EHRs for research in general would facilitate patients’ understanding and acceptance of their use for recruitment purposes:
MC3_P4: When you go calling [people] and [they have] no idea that anything’s going on and you’re like, “Oh, hey, I’ve been searching through your stuff,” everyone—almost everyone finds that offensive.
DC2_P8: I guess if I know that the records are there and I know that researchers have access to them, then I’m okay with them contacting me directly for a study. Because that’s what researchers do. I would understand that.
Decision-making power
Participants expressed strong opinions about who should have the power to decide whether and which patients hear about research for which they might be eligible. Some felt physicians were best situated to make these decisions, whereas others located this power with patients.
Physician as decisionmaker
When discussing physicians’ role in determining whether patients hear about research opportunities, trust and established relationships were again common themes:
MC1_P2: If I hear from my doctor … I’m gonna listen to him. ‘Cause that’s who I trust. It’s who I’ve been seeing for many years.
Participants expected that physicians would apply their knowledge of a patient’s health and life in deciding “whether that person would be a good person for the study” (QC2_P1):
CC1_P2: Well, he just knows your situation. And I think if he does, he’s gonna recommend you for that research.
Some participants anticipated that physicians would be better able than patients to understand and evaluate research opportunities:
CC2_P2: The physician could possibly be able to know whether or not the research was a positive... Whether it was a research organization that was approved or recommended or etcetera.
Patient as decisionmaker
Participants also identified advantages to contacting patients directly—many of them based on the significant downsides they saw to physicians in the role of decisionmaker. For example, a common concern about physician involvement was the likelihood that some potentially eligible patients would not hear about the study: “Everybody won’t get the information—it’ll be up to the doctor” (CC1_P7) and “you may get skipped over” (MC3_P5).
Participants also raised concerns about the basis upon which physicians would decide which patients could be contacted. Some voiced general unease related to trust and bias: “He could be biased. He just may not be willing to let his patients know, or all of his patients know about a study” (QC2_P3). Others were more specific about the kinds of bias they thought could occur, based on characteristics of the patient as well as other influences on the physician:
DC1_P3: I would think, from the researcher’s standpoint, that would not be good. Because it would be a subjective selection. It would be just who the doctors felt were—.
DC1_P6: People who visit the doctors regularly.
DC1_P3: ... or might have lifestyle choices …
DC1_P2: The doctors … they know their patients, so they may guess how their patients would respond, and might have some bias in who they would invite or not invite because they would wanna affect the results, perhaps.
QC2_P2: If y’all are offering monetary value to the person to be a part of the study, the physician’s gonna know who needs the money and who don’t.
More fundamentally, many felt strongly that patients have a right to hear about research opportunities that should not be eclipsed by their physicians:
MC2_P2: The physician shouldn’t decide—if I want research done on this, my physician shouldn’t make my decision.
MU2_P5: I just don’t think that it should be left in the hands of the doctor to decide, to exclude me from being a part of a study… To take away that right from me to be a part of something—I think that’s unacceptable.
By not placing physicians in the middle of the process, participants highlighted the advantage that “definitely you would know that the patient is getting the information” (MC1_P2), rather than ending up being “that person [the doctor] just pushed to the side and said, ‘I don’t think they need to hear that’” (MU2_P7). By getting the information directly, patients would be empowered to make their own decisions about whether to learn more about a study:
MC3_P4: I’m sure, for the people being researched, it’s much more respectful.
MC3_P1: Well, the patient gets to decide instead of maybe the doctor deciding for them.
MC3_P4: Exactly. You’re cutting out the middle man.
MC3_P1: Letting the patient take responsibility for themself.
QC3_P1: I think that should be your choice and not the doctor’s choice. Because you might learn something from this study. If he excludes you, you just might miss out on learning something.
Effect on research
Participants discussed the possible impact of initial contact procedures on the research itself, articulating notably mixed views about the effect of different approaches on efficiency and research quality, as well as participation rates.
Efficiency and research quality
Many participants anticipated that contacting patients directly would be more efficient: “I look at it in terms of how many people you can contact” (DC1_P1). They pointed out that omitting physicians from the recruitment process would reduce delays…
MU2_P9: The researcher isn’t having to go to the physician to ask for permission… And you’re also not waiting for the physician to ask the patients, and then waiting on that turnaround time from the patient.
…as well as eliminate the potential obstacle of physician non-cooperation:
CC2_P5: The physician has other patients. I don’t think he’s really concerned about research. So he’s like, “Okay, I’ll get to it when I can.” If he ever gets to it.
Participants further noted that incurring the burdens associated with involving physicians might bring little if any gain in terms of the quality of the sample:
CC1_P4: I could see [physicians], if they were in a hurry, just kinda starting at the top of the alphabet and going, “Let’s give ‘em ten people. Here.” They’re all As and Bs. You know, you didn’t really go through, because of time, and look at each patient individually to see who would qualify for the study.
Some participants were specifically concerned that involving physicians—and their potential biases in determining which patients could be contacted—would in turn result in a biased sample:
DC3_P6: [When] the physician becomes a gatekeeper, you narrow your roster of eligible patients. It could have an impact on the validity of the study. Because someone that’s not associated with the study is deciding who’s going to be in your study.
DC3_P4: Despite what I said about the [advantage] being that the physician knew other things—whether it would be appropriate for an individual to be in the study—I still think if the physician is making the decision, it would skew the study.
A few participants, however, saw efficiency and research quality advantages to contacting patients through their physicians:
CC3_P4: [Physicians] may be able to filter it for you … to know what’s a best match for what you’re doing, the people most likely to accept, to kinda eliminate your turn-downs and stuff—to say, “These seven people will likely do it for you. Don’t bother calling the other three. I can’t even get them to come to the office” or something like that.
Participation rates
Some participants expected that contacting patients directly would result in higher participation rates:
MC3_P4: As a researcher, I’m sure you’d get more. I mean, I can imagine that the person who works at the physician’s office that already has the crappy job to begin with gets handed a list of 50 people that you want to participate in your research… I think your success rate would be much better with a person-to-person contact to the patient from the researcher.
However, many felt that patients’ enrollment decisions would be positively influenced by hearing about the study from their physicians:
DC2_P7: If you got a call from your physician, it would appear to be his giving you his blessing or his opinion that this is more important that you do it. I think that it’d be harder to turn your doctor down than an anonymous researcher.
DC2_P6: Yeah. I actually think you get a better response if the physician did ask the patient, because you’re gonna believe your doctor wouldn’t send you to something that wasn’t gonna be beneficial for your health.
DC2_P4: True.
DC2_P6: And your doctor knows you. Well, supposedly. I mean, if you have a good relationship with your primary care, you trust their opinion. And I would do it.
Participants also predicted negative consequences for enrollment if, when contacted directly, patients ignore communication from unrecognized sources:
MU2_P3: If you get emails, you might think it’s spam and just neglect it, and just toss it out.
MU2_P6: I was kinda thinking the same thing. In today’s world, you have to be so leery about emails from your bank, from your Target credit card, everything you do, even medical records now… Seems like there’s just so many scams… So I just thought hearing it from your physician’s office would seem more legitimate to me as a patient than just getting a random letter or email or phone call.
Effect on patient care
Some participants believed initial contact procedures could affect clinical care. A primary concern about involving physicians in the recruitment process was taking time away from patients:
DC1_P7: I don’t wanna take any time away from my physician. I’m serious. We get 15 min. That’s it.
However, by involving physicians as the connection between researchers and patients, some anticipated that physicians might be able to apply research-generated information in patient care:
CC2_P5: When the research is done, [researchers] can share information with the physicians and what they come up with, and this way it’s beneficial to the research and the physician.
Initial contact with prospective participants: Acceptability and most appropriate approach
After participants identified and discussed this wide range of considerations, we asked about the acceptability of the two basic ways researchers could contact potentially eligible patients to invite their participation in the hypothetical study. Nearly all (95%), particularly those in rural locations (Mingo and Quitman), said it would be acceptable for researchers to contact patients directly (Table 4 ). Three-fourths (75%) indicated that it would be acceptable for researchers to contact patients through their physicians.
When we asked which approach would be most appropriate , a large majority (70%) chose direct contact (Table 4 ; see also Additional file 2 : Figure S2). For these participants, patient choice and ability to make their own decisions emerged as pivotal. Approximately one-third (30%) chose contact through physicians as most appropriate. Advocates of this approach commonly referred to themes of trust and established relationships.
Over the course of these discussions, some participants suggested modifications to the two basic approaches, as well some caveats related to the nature of the particular study.
Modified approaches
As described above, participants acknowledged potential advantages of physician involvement in the recruitment process, but many were uncomfortable with physicians acting as a “gatekeeper” with the power to limit patient choice. This led some to suggest alternate roles for physicians; for example, one suggested that physicians could be required to let patients know about research opportunities:
CC1_P1: If you took out the words that the doctor had the option to let people know about the study or not, [that] would be okay. I’m saying that the doctor has an obligation to his patients to let them know about the study once you contact him.
More commonly, the idea arose that physicians could simply be informed about researcher contact with their patients: “I think it’s in the best interest of the patient for their physician to be aware of the study” (CC3_P3). Some participants envisioned this as an informal process whereby, upon hearing directly about a study, patients could choose to seek input from their physicians:
QC2_P1: If somebody got upset about getting a letter or an email or something, they’d probably go to the doctor anyway and say, “What is this all about? Do you know?”
Others pictured a more formal process in which researchers notify physicians of impending patient contact:
MC3_P4: Personally, I think that that needs to be “Contact patients directly, after letting the physician know of the study.” I think that’s probably a much more acceptable… I think that the best results would be contacting the patient after you’d contacted the physician to let them know that it’s going on.
The nature of the study
Our focus group discussions centered on a hypothetical study of the effect of telephone reminders on managing blood sugar levels. In discussing acceptable and most appropriate approaches to initial recruitment contact for this particular study, some participants volunteered that their opinions would be different if the study involved a higher-risk intervention, such as a drug:
CC3_P9: If it were like something like taking a medication, my opinion would probably be different. But just to participate in [this] study? I don’t think that that needs to go through a physician. But obviously if it’s something that’s going to impact their health like taking a medication or doing something that will actually affect their individual health, then I would think they would need to go through the physician.
Response requested to initial contact
We next queried our focus groups about whether patients should be asked to opt in or opt out of further contact in response to an initial recruitment letter (Table 2 ). Participants identified advantages and disadvantages of each, from the perspectives of both the researcher and the patient.
With an opt-in approach, participants recognized that researchers would be in the position of waiting for patients to call them in response to a recruitment letter. Some found this advantageous in terms of efficiency; rather than spending time calling all potentially eligible patients, researchers could simply rely on interested individuals to call in:
QC1_P3: You save time, you save money, everything. Because only the ones that’s gonna want to do it is gonna call… If they don’t want to, they’re gonna toss it in the garbage or just forget that it was ever there. You save time and money both.
Other participants, however, expected that there could be negative effects on efficiency. Researchers would have to send more letters and likely have difficulties achieving target sample sizes as they passively awaited calls:
MU2_P10: Lifestyles are so filled with … so many distractions. Something that you’re just getting in the mail, it’s so easy to put it down. And once you don’t think about it, then it’s gone; it’s done. So they’ve gotta be getting their mail at the moment that they’re in the mood to look at it—so your response rate’s just gonna be so low.
From the patient’s perspective, participants recognized that an opt-in approach would put the onus on patients to call researchers in response to a recruitment letter. Many found this appealing in terms of convenience…
QC2_P4: Well, to call the 800 number it would be on my time. I may not be at home if you call me, or I may be in an area that my phone didn’t pick up, if the researchers were doing the call.
QC2_P1: And that way you’d be kinda volunteering to find out more.
QC2_P2: Right.
QC2_P1: [With opt-out], you were just waiting to be called, and if you don’t wanna be called, you still have to call them. This way’s a lot less phone time.
…and “personal control” (DC2_P4):
QC1_P5: I think that when you get that information in the mail and you read it, and you have an option then to say yea or nay, basically that’s how people answer. That’s how they operate. They got their mind made up … if they intend to deal with it, they will. If not, in the garbage it go.
In particular, some commented favorably on the intentionality involved in patients taking the initiative to respond:
CC1_P8: If somebody really wanted to participate in it … they gonna take out the time to call the number. And have real desire to do it.
Other participants, however, were concerned about the likelihood that recruitment letters would be overlooked, resulting in patients missing the chance to hear about a study: “I’d throw away [the letter] and call it junk mail… That’s something I’d miss out on” (QC3_P5).
Not surprisingly, participants’ opinions about an opt-out approach were often the inverse of their reactions to opt-in. For instance, they recognized that researchers would be in the position of calling all potentially eligible patients (except those who took steps to opt out of further contact). Many felt this would reduce efficiency, increasing time and effort spent making calls—especially given that people often do not answer calls from unknown numbers:
DC2_P3: I kinda hate to go back to dollars and cents all the time, but if you do the opt-out, you’ve gotta have somebody that’s gonna pick up the phone and call everybody on your list. And that’s gonna cost you money.
QC3_P1: If it’s a number that’s not programmed and a name gonna come up with it, I don’t answer it.
Other participants, however, saw efficiency advantages in researchers being able to reach out proactively: “At least you made contact and then the person has the opportunity to say yes or no—I think that that would increase the sample size” (DC2_P6). These participants frequently expected that even interested individuals would not take the time to opt in upon receipt of the recruitment letter:
MC1_P3: I would take opt-out, because most of the time if we get something that we are to call an 800 number, I’m never gonna call it… But I’ve been made aware of the study; I’ve had a week to think about it; I’ve become familiar with it. So I would be more than likely to accept the phone call and talk to the person.
By having the chance to make personal contact, some even predicted that researchers could convince patients to participate who had not been interested based on the letter:
MU1_P8: Opt-out, you have the chance to change their mind too if you call ‘em. They might get the letter and be like, “I ain’t doing that.” But then [the researcher] gets them on the phone and she could change their mind… It’s more personal than a letter. So if you’re that type of person, it might change your mind.
From the patient’s perspective, participants recognized that an opt-out approach could involve awaiting a follow-up call from researchers. For some, not having to take any action in response to the recruitment letter was an advantage. They described that, upon receiving the follow-up call, “Patients, A, don’t have to answer or, B, [could] say ‘Don’t call me anymore.’ So at least for the researcher and the participant, I think it’s a win, ‘cause you can still say no.” (CC2_P6).
Some mentioned that a researcher following up with them would make them feel valued: “You feel more important, I guess, in this scenario. It feels like you’re needed; you’re necessary” (DC2_P4). In contrast, other participants felt that receiving calls would be intrusive:
MU1_P7: With the opt-out you’re gonna have a lotta people that are not interested but they for some reason don’t have time to call in… And they’ll just be irritated by the phone call. If somebody’s really interested, they’ll call.
Response requested to initial contact: Acceptable approaches
After participants raised and considered this variety of factors, nearly all (94%) said it was acceptable for recruitment letters to ask patients to opt in to learning more about the study (Table 4 ; see also Additional file 2 : Figure S2). A substantial majority (83%) also found opt-out to be an acceptable approach. The minority who found opt-out unacceptable commonly described it as intrusive and irritating for patients as well as ineffective and time-consuming for researchers. However, as the larger proportions indicate, most participants found both approaches acceptable because neither seemed particularly troubling:
DC1_P8: I just think they’re both—they’ve got their pros and cons, and none of them seem very problematic to me.
In addition, participants observed that, regardless of the approach, patients get to decide whether to participate in the study or not:
CC2_P3: I think it’s whichever way the people doing the research decide they wanna go.
Moderator: Okay. So whatever the researchers think is the best, you’re okay with.
CC2_P3: Yeah. And I’ll make the decision whether I wanna be involved in it.
EHR phenotyping offers the ability to rapidly assemble a precisely defined cohort of patients prescreened for eligibility to participate in health-related research. Even so, researchers, Institutional Review Boards (IRBs), patients, and healthcare providers must still contend with the challenges associated with research recruitment. Identifying and contacting individuals about their interest in participation must occur within the context of well-established requirements for ethically responsible research [ 27 ]. Although research recruitment is typically considered to involve fewer risks than research participation, there are concerns about researcher access to patients’ personal information prior to consent [ 28 ]. At the same time, external validity relies heavily on researchers’ success enrolling eligible patients [ 29 ] and failure to reach accrual targets is a costly and common barrier to advancing scientific knowledge [ 8 , 16 ].
Two central aspects of research recruitment—initial contact with patients and the response requested to this contact—are the subject of significant institutional variability and have ethical and practical implications [ 16 , 17 , 18 , 28 , 30 ]. To illuminate patients’ perspectives on these strategies, we conducted focus group research in diverse populations to gather opinions about direct investigator versus physician-mediated contact, and about opt-in versus opt-out response expectations.
Regarding initial contact, most of our participants said direct investigator and physician-mediated approaches are both acceptable; however, a large majority said direct contact would be most appropriate. In these discussions, participants raised considerations related to trust and transparency, the locus of decision-making power, and the effects on research and patient care. These issues have been the subject of some empirical and ethical inquiry. Studies have shown that requiring physician permission to contact patients results in a significantly smaller proportion of potentially eligible participants being accessible to researchers [ 29 , 31 ]. Some commentators have argued that paying this “price” may yield little upside [ 29 , 32 , 33 ]. It may be difficult for researchers to identify healthcare providers who are positioned to permit or deny contact for each prospective participant, and impractical to expect busy providers to familiarize themselves with the details of research protocols. Further, although IRBs place restrictions on researchers, they generally do not place procedural requirements on treating physicians—some physicians may contact patients to elicit their wishes and values concerning research participation, whereas others may rely on their own perceptions and biases. Thus, requiring physicians’ permission to contact patients may not necessarily enhance careful consideration of a patient’s eligibility or ensure added protection. Indeed, several have asserted that limiting patients’ opportunities to hear about studies is unduly paternalistic and violates principles of respect for persons and self-determination [ 29 , 32 , 33 , 34 ].
The experiences and perspectives of providers and researchers are essential to this debate. A few such studies have been conducted [ 35 , 36 ] but more are needed—particularly in the context of the unique features of the US healthcare system. In the meantime, our participants’ input about initial contact suggests several promising ways forward. First, concentrated efforts to raise patient and public awareness about research use of EHRs could increase trust and transparency and help facilitate acceptance of their use for recruitment purposes. These efforts could include education about the importance of research using EHRs for the overarching goal of improving health and healthcare; the importance of representativeness in research (i.e. , maximizing participation to reduce bias and increase quality and fairness); and the applicable system of oversight and protections.
Second, a “physician notification” approach is worthy of in-depth exploration. Rather than requiring active physician permission before contact can occur, physicians could be notified and given time to object, after which non-response is taken as passive approval [ 29 , 31 , 37 ]. This approach is likely an efficient way of involving patients’ physicians, providing opportunity for their input but avoiding the burdens associated with requiring their permission [ 31 ].
Third, the relative merits of involving treating physicians in research recruitment depends on the study. Requiring physicians’ permission to contact patients regardless of the nature of the study (from focus groups to drug trials) is neither sensible nor efficient [ 33 ]. Flexible policies are needed that tailor requirements for physician involvement—ranging from no role to passive notification to active approval—based on the risks associated with the research.
Finally, recruitment letters—particularly those coming directly from researchers—can also help increase trust and transparency by making a clear connection to persons or entities known to recipients. This should include an explanation of why the prospective participant is being contacted, how the investigator obtained knowledge about the individual relevant to recruitment, and what will happen to that information if the person decides not to participate [ 30 ].
Regarding the response requested to recruitment letters, our participants anticipated that opt-in and opt-out strategies both would have advantages and disadvantages for research efficiency. Available evidence supports their general intuition. A major systematic review of interventions to improve recruitment to randomized trials found with high certainty that telephone calls to people who do not reply to a mailed invitation improves enrollment [ 38 ]. A few studies have also documented the resources (e.g. , staff time making phone calls) required for follow-up activities associated with opt-out [ 39 , 40 ].
In addition to the value placed on research efficiency, our participants’ comments about opt-in and opt-out reflected several other patient considerations, including convenience, control, intentionality, and intrusiveness. There is a lack of empirical data on these outcomes, perhaps due in part to the fundamental challenges associated with recruiting an unbiased sample into a real-time study of reactions to research recruitment [ 41 ]. Ultimately, however, the vast majority of our participants expected both approaches would be acceptable—finding neither especially problematic and, importantly, noting that both afford patients the opportunity to make their own decisions.
In professional literature, conceptual arguments favoring opt-out echo many of our focus group findings—including that if patients and the public want certain kinds of research to be conducted, recruitment procedures must be designed to reduce bias and increase participation; that patients who receive a letter may not perceive a brief follow-up phone call to be an unjustifiable invasion of their privacy; that some might prefer opt-out because of the reassurance provided by personal contact; and that those who so wish can always avoid or ignore further contact [ 42 ].
Whichever strategy is used, a central goal should be that patients’ first crucial decision to opt in or opt out in response to a recruitment letter is adequately informed [ 43 ]. Research is needed to maximize the likelihood of recipients opening the letter, as well as the content and formatting of the letter so that it effectively communicates the information prospective participants identify as important to deciding whether they want to learn more. Examples might include the specific type and depth of detail they find helpful regarding researchers’ credentials and the importance of the study topic.
Our focus group study had several strengths, including diverse study locations (including rural areas); baseline educational efforts to enable participants to develop informed opinions; and asking participants not about personal preferences, but about acceptability and most appropriate actions, after considering advantages and disadvantages of competing strategies from multiple viewpoints.
Our findings are limited in certain ways. Our study was primarily qualitative and, due to feasibility constraints, limited geographically to the southeastern US and conducted only in English. In qualitative research, the goal is to elucidate the range of perspectives—including the nuance and rationale—as articulated by participants. Rather than statistical power, nonprobablistic sampling is guided by the concept of “saturation,” the point at which no new information or themes are observed in the data [ 44 ]. We provide some quantitative data, captured as a product of the questionnaire as well as the worksheets we used to help structure the discussions. These proportions should be viewed as an indicator of how commonly various themes and responses were expressed among our diverse group of participants. They do not necessarily provide an accurate forecast of the results if our findings were used to, for example, generate closed-ended items for a survey fielded in a sample drawn to be representative of an entire population. Future research, including both qualitative and quantitative approaches, should examine whether and to what extent opinions differ in other regions or populations. In addition, although patients are a vital source of input, they are only one of many stakeholder groups whose feedback is essential to the development of sound policy. Finally, our study used a hypothetical scenario premised on a minimal risk study of a behavioral intervention for type 2 diabetes. Future research should focus on elucidating stakeholders’ views on the array of research facilitated by next-generation EHR phenotyping [ 45 ], as well as assessing the outcomes of alternative policies in actual practice.
Our focus group results suggest that patients recognize multiple advantages and disadvantages of different research recruitment strategies and place value on the implications not just for themselves, but also for researchers and healthcare providers. Most of our participants said various ways to initiate contact with patients were each acceptable, but a substantial majority said direct contact by researchers was the most appropriate approach compared to physician-mediated contact. Similarly, nearly all found it acceptable for recruitment letters to ask for either an opt-in or opt-out response. These findings, including rich qualitative detail, contribute to the body of empirical and ethical literature on improving research recruitment and suggest specific ways forward as well as important areas for future research.
Abbreviations
Cabarrus County, North Carolina
Durham County, North Carolina
Electronic health record(s)
Institutional Review Boards
Mingo County, West Virginia
Quitman County, Mississippi
United States
Blumenthal D, Tavenner M. The “meaningful use” regulation for electronic health records. N Engl J Med. 2010;363(6):501–4.
Article CAS PubMed Google Scholar
Jha AK. Meaningful use of electronic health records: the road ahead. JAMA. 2010;304(15):1709–10.
Banda JM, Seneviratne M, Hernandez-Boussard T, Shah NH. Advances in electronic phenotyping: from rule-based definitions to machine learning models. Annu Rev Biomed Data Sci. 2018;1(1):53–68.
Article PubMed PubMed Central Google Scholar
Hripcsak G, Albers DJ. Next-generation phenotyping of electronic health records. J Am Med Inform Assoc. 2013;20(1):117–21.
Article PubMed Google Scholar
Pathak J, Kho AN, Denny JC. Electronic health records-driven phenotyping: challenges, recent advances, and perspectives. J Am Med Inform Assoc. 2013;20(e2):e206–11.
Richesson RL, Hammond WE, Nahm M, Wixted D, Simon GE, Robinson JG, et al. Electronic health records based phenotyping in next-generation clinical trials: a perspective from the NIH health care systems Collaboratory. J Am Med Inform Assoc. 2013;20(e2):e226–31.
Jensen PB, Jensen LJ, Brunak S. Mining electronic health records: towards better research applications and clinical care. Nat Rev Genet. 2012;13(6):395–405.
Visweswaran S, Becich MJ, D’Itri VS, Sendro ER, MacFadden D, Anderson NR, et al. Accrual to clinical trials (ACT): a clinical and translational science award consortium network. JAMIA Open. 2018;1(2):147–52.
Cowie MR, Blomster JI, Curtis LH, Duclaux S, Ford I, Fritz F, et al. Electronic health records to facilitate clinical research. Clin Res Cardiol. 2017;106(1):1–9.
Kopcke F, Prokosch HU. Employing computers for the recruitment into clinical trials: a comprehensive systematic review. J Med Internet Res. 2014;16(7):e161.
Miotto R, Weng C. Case-based reasoning using electronic health records efficiently identifies eligible patients for clinical trials. J Am Med Inform Assoc. 2015;22(e1):e141–50.
Shivade C, Raghavan P, Fosler-Lussier E, Embi PJ, Elhadad N, Johnson SB, et al. A review of approaches to identifying patient phenotype cohorts using electronic health records. J Am Med Inform Assoc. 2014;21(2):221–30.
Coorevits P, Sundgren M, Klein GO, Bahr A, Claerhout B, Daniel C, et al. Electronic health records: new opportunities for clinical research. J Intern Med. 2013;274(6):547–60.
Patterson S, Mairs H, Borschmann R. Successful recruitment to trials: a phased approach to opening gates and building bridges. BMC Med Res Methodol. 2011;11(1):73.
Treweek S, Lockhart P, Pitkethly M, et al. Methods to improve recruitment to randomised controlled trials: Cochrane systematic review and meta-analysis. BMJ Open. 2013;3:e002360. https://doi.org/10.1136/bmjopen-2012-002360 .
Stein MA, Shaffer M, Echo-Hawk A, Smith J, Stapleton A, Melvin A. Research START: A multimethod study of barriers and accelerators of recruiting research participants. Clin Transl Sci. 2015;8(6):647–54.
Kost RG, Mervin-Blake S, Hallarn R, Rathmann C, Kolb HR, Himmelfarb CD, et al. Accrual and recruitment practices at clinical and translational science award (CTSA) institutions: a call for expectations, expertise, and evaluation. Acad Med. 2014;89(8):1180–9.
Obeid JS, Beskow LM, Rape M, Gouripeddi R, Black T, Cimino JJ, et al. A survey of practices for the use of electronic health records to support research recruitment. J Clin Transl Med. 2017;1:246–52.
Google Scholar
Tenenbaum JD, Christian V, Cornish MA, Dolor RJ, Dunham AA, Ginsburg GS, et al. The MURDOCK study: a long-term initiative for disease reclassification through advanced biomarker discovery and integration with electronic health records. Am J Transl Res. 2012;4(3):291–301.
PubMed PubMed Central Google Scholar
Ellard-Gray A, Jeffrey NK, Choubak M, Crann SE. Finding the hidden participant: solutions for recruiting hidden, hard-to-reach, and vulnerable populations. Int J Qual Methods. 2015;14(5):1609406915621420.
Article Google Scholar
Ridpath JR, Greene SM, Wiese CJ. PRISM Readability Toolkit. 3rd ed. Seattle: Kaiser Permanente Washington Health Research Institute; 2007. Available from: https://www.kpwashingtonresearch.org/about-us/capabilities/research-communications/prism/ .
Ginns P. Integrating information: a meta-analysis of the spatial contiguity and temporal contiguity effects. Learn Instr. 2006;16(6):511–25.
Ware C. Information visualization: perception for design. 3rd ed. Waltham, MA: Morgan Kaufmann; 2013.
Brelsford KM, Spratt SE, Beskow LM. Research use of electronic health records: patients’ perspectives on contact by researchers. J Am Med Inform Assoc. 2018;25(9):1122–9.
Guest G, MacQueen KM, Namey EE. Applied thematic analysis. Los Angeles, CA: Sage Publications; 2012.
Book Google Scholar
MacQueen KM, McLellan E, Kay K, Milstein B. Codebook development for team-based qualitative analysis. Cult Anthropol Methods. 1998;10(2):31–6.
National Commission for the Protection of Human Subjects of Biomedical and Behavioral Research. The Belmont Report: Ethical Principles and Guidelines for the Protection of Human Subjects of Research. Washington DC: US Government Printing Office; 1979.
Beskow LM, Sandler RS, Weinberger M. Research recruitment through US central cancer registries: balancing privacy and scientific issues. Am J Public Health. 2006;96(11):1920–6.
Gurwitz JH, Guadagnoli E, Landrum MB, Silliman RA, Wolf R, Weeks JC. The treating physician as active gatekeeper in the recruitment of research subjects. Med Care. 2001;39(12):1339–44.
Beskow LM, Botkin JR, Daly M, Juengst ET, Lehmann LS, Merz JF, et al. Ethical issues in identifying and recruiting participants for familial genetic research. Am J Med Genet A. 2004;130A(4):424–31.
Beskow LM, Millikan RC, Sandler RS, Godley PA, Weiner BJ, Weinberger M. The effect of physician permission versus notification on research recruitment through cancer registries (United States). Cancer Causes Control. 2006;17(3):315–23.
Sharkey K, Savulescu J, Aranda S, Schofield P. Clinician gate-keeping in clinical research is not ethically defensible: an analysis. J Med Ethics. 2010;36(6):363–6.
Weng C, Appelbaum P, Hripcsak G, Kronish I, Busacca L, Davidson KW, et al. Using EHRs to integrate research with patient care: promises and challenges. J Am Med Inform Assoc. 2012;19(5):684–7.
Meslin EM. The recruitment of research participants and the role of the treating physician. Med Care. 2001;39(12):1270–2.
Newington L, Metcalfe A. Researchers’ and clinicians’ perceptions of recruiting participants to clinical research: a thematic meta-synthesis. J Clin Med Res. 2014;6(3):162–72.
Guillemin M, McDougall R, Martin D, Hallowell N, Brookes A, Gillam L. Primary care physicians’ views about gatekeeping in clinical research recruitment: a qualitative study. AJOB Empir Bioeth. 2017;8(2):99–105.
Beskow LM, Sandler RS, Millikan RC, Weinberger M. Patient perspectives on research recruitment through cancer registries. Cancer Causes Control. 2005;16(10):1171–5.
Treweek S, Pitkethly M, Cook J, Fraser C, Mitchell E, Sullivan F, et al. Strategies to improve recruitment to randomised trials. Cochrane Database of Syst Rev. 2018;2:Mr000013.
Miller CJ, Burgess JF Jr, Fischer EP, Hodges DJ, Belanger LK, Lipschitz JM, et al. Practical application of opt-out recruitment methods in two health services research studies. BMC Med Res Methodol. 2017;17(1):57.
Weiss D, Murchison A, Hark L, Collymore B, Casten R, Brawer R, et al. Comparing opt-in versus opt-out recruitment strategies for ophthalmology research. Invest Ophthalmol Vis Sci. 2013;54(15):2438.
Agre P, Rapkin B, Dougherty J, Wilson R. Barriers encountered conducting informed consent research. IRB. 2002;24(4):1–5.
Hewison J, Haines A. Overcoming barriers to recruitment in health research. BMJ. 2006;333(7562):300–2.
Williams B, Irvine L, McGinnis AR, McMurdo ME, Crombie IK. When “no” might not quite mean “no”; the importance of informed and meaningful non-consent: results from a survey of individuals refusing participation in a health-related research project. BMC Health Serv Res. 2007;7:59.
Guest G, Bunce A, Johnson L. How many interviews are enough? An experiment with data saturation and variability. Field Methods. 2006;18(1):59–82.
Boland MR, Hripcsak G, Shen Y, Chung WK, Weng C. Defining a comprehensive verotype using electronic health records for personalized medicine. J Am Med Inform Assoc. 2013;20(e2):e232–8.
Download references
Acknowledgments
Thank you to Martina Bresciani, Kevin McKenna, Li Lin, and Anh Nguyen for their assistance.
This work was supported by a grant from the National Library of Medicine (R01-LM-012178). The content is solely the responsibility of the authors and does not necessarily represent the official views of NLM or NIH. The funders had no role in the study design; the collection, analysis, or interpretation of data; the writing of the report; or the decision to submit this article for publication.
Availability of data and materials
The datasets generated and analyzed in this study are not publicly available due to privacy and confidentiality considerations, but are available upon reasonable request from qualified researchers conducting IRB-approved studies that fall within the scope of the study purpose and data use described to interviewees at the time of participation.
Author information
Authors and affiliations.
Center for Biomedical Ethics and Society, Vanderbilt University Medical Center, 2525 West End Avenue, Suite 400, Nashville, TN, 37203, USA
Laura M. Beskow, Kathleen M. Brelsford & Catherine M. Hammack
You can also search for this author in PubMed Google Scholar
Contributions
LB, Principal Investigator, obtained the funding; led the research design and methodology; led the development of study instruments and other materials; supervised collection, coding, analysis, and interpretation of the qualitative and quantitative data; and led the drafting and revising of the manuscript. KB collaborated on the research design and methodology, and on the development of study instruments and other materials; moderated the focus groups; assisted with coding, analysis, and interpretation of the data; and contributed substantively to the manuscript. CH collaborated on the development of study instruments and other materials; assisted with the conduct of the focus groups; assisted with the analysis and interpretation of the data; and contributed substantively to the manuscript. All authors have read and approved the final manuscript.
Corresponding author
Correspondence to Laura M. Beskow .
Ethics declarations
Ethics approval and consent to participate.
Institutional Review Boards at Duke University and Vanderbilt University deemed this research exempt under 45 CFR 46.101(b) (2) (2009), covering the collection and analysis of data from all locations. Participants received a study information sheet describing the purpose of the focus group and the risks, potential harms, and protections; after allowing sufficient time to read the information and an opportunity for questions, the moderator obtained participants’ verbal agreement to participate and to audio recording of the group discussion.
Consent for publication
Not applicable.
Competing interests
The authors declare that they have no competing interests.
Publisher’s Note
Springer Nature remains neutral with regard to jurisdictional claims in published maps and institutional affiliations.
Additional files
Additional file 1:.
Table S1–1. Study location (county) demographics. Table S1–2 . Participant questionnaire development. Table S1–3 . Consolidated Criteria for Reporting Qualitative Research (COREQ). (PDF 164 kb)
Additional file 2:
Table S2–1 . Questionnaire responses – trust, attitudes toward research. Table S2–2 . Additional illustrative quotes. Figure S2 . Summary – acceptable and most appropriate recruitment approaches. (PDF 187 kb)
Rights and permissions
Open Access This article is distributed under the terms of the Creative Commons Attribution 4.0 International License ( http://creativecommons.org/licenses/by/4.0/ ), which permits unrestricted use, distribution, and reproduction in any medium, provided you give appropriate credit to the original author(s) and the source, provide a link to the Creative Commons license, and indicate if changes were made. The Creative Commons Public Domain Dedication waiver ( http://creativecommons.org/publicdomain/zero/1.0/ ) applies to the data made available in this article, unless otherwise stated.
Reprints and permissions
About this article
Cite this article.
Beskow, L.M., Brelsford, K.M. & Hammack, C.M. Patient perspectives on use of electronic health records for research recruitment. BMC Med Res Methodol 19 , 42 (2019). https://doi.org/10.1186/s12874-019-0686-z
Download citation
Received : 09 December 2018
Accepted : 15 February 2019
Published : 26 February 2019
DOI : https://doi.org/10.1186/s12874-019-0686-z
Share this article
Anyone you share the following link with will be able to read this content:
Sorry, a shareable link is not currently available for this article.
Provided by the Springer Nature SharedIt content-sharing initiative
- Electronic health records
- Patient perspectives
- Research ethics
- Research subject recruitment
- Physician-patient relationship
BMC Medical Research Methodology
ISSN: 1471-2288
- General enquiries: [email protected]
Advertisement
Electronic health records to facilitate clinical research
- Open access
- Published: 24 August 2016
- Volume 106 , pages 1–9, ( 2017 )
Cite this article
You have full access to this open access article
- Martin R. Cowie 1 ,
- Juuso I. Blomster 2 , 3 ,
- Lesley H. Curtis 4 ,
- Sylvie Duclaux 5 ,
- Ian Ford 6 ,
- Fleur Fritz 7 ,
- Samantha Goldman 8 ,
- Salim Janmohamed 9 ,
- Jörg Kreuzer 10 ,
- Mark Leenay 11 ,
- Alexander Michel 12 ,
- Seleen Ong 13 ,
- Jill P. Pell 14 ,
- Mary Ross Southworth 15 ,
- Wendy Gattis Stough 16 ,
- Martin Thoenes 17 ,
- Faiez Zannad 18 , 19 &
- Andrew Zalewski 20
33k Accesses
334 Citations
308 Altmetric
41 Mentions
Explore all metrics
Electronic health records (EHRs) provide opportunities to enhance patient care, embed performance measures in clinical practice, and facilitate clinical research. Concerns have been raised about the increasing recruitment challenges in trials, burdensome and obtrusive data collection, and uncertain generalizability of the results. Leveraging electronic health records to counterbalance these trends is an area of intense interest. The initial applications of electronic health records, as the primary data source is envisioned for observational studies, embedded pragmatic or post-marketing registry-based randomized studies, or comparative effectiveness studies. Advancing this approach to randomized clinical trials, electronic health records may potentially be used to assess study feasibility, to facilitate patient recruitment, and streamline data collection at baseline and follow-up. Ensuring data security and privacy, overcoming the challenges associated with linking diverse systems and maintaining infrastructure for repeat use of high quality data, are some of the challenges associated with using electronic health records in clinical research. Collaboration between academia, industry, regulatory bodies, policy makers, patients, and electronic health record vendors is critical for the greater use of electronic health records in clinical research. This manuscript identifies the key steps required to advance the role of electronic health records in cardiovascular clinical research.
Similar content being viewed by others
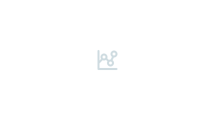
The Electronic Health Record for Translational Research
Luke V. Rasmussen
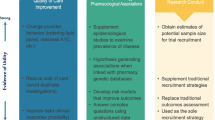
Harnessing Electronic Medical Records in Cardiovascular Clinical Practice and Research
Pishoy Gouda & Justin Ezekowitz
Electronic Medical Records and Their Use in Health Promotion and Population Research of Cardiovascular Disease
Bobbie J. Kite, Wilkister Tangasi, … Randi E. Foraker
Avoid common mistakes on your manuscript.
Introduction
Electronic health records (EHRs) provide opportunities to enhance patient care, to embed performance measures in clinical practice, and to improve the identification and recruitment of eligible patients and healthcare providers in clinical research. On a macroeconomic scale, EHRs (by enabling pragmatic clinical trials) may assist in the assessment of whether new treatments or innovation in healthcare delivery result in improved outcomes or healthcare savings.
Concerns have been raised about the current state of cardiovascular clinical research: the increasing recruitment challenges; burdensome data collection; and uncertain generalizability to clinical practice [ 1 ]. These factors add to the increasing costs of clinical research [ 2 ] and are thought to contribute to declining investment in the field [ 1 ].
The Cardiovascular Round Table (CRT) of the European Society of Cardiology (ESC) convened a two-day workshop among international experts in cardiovascular clinical research and health informatics to explore how EHRs could advance cardiovascular clinical research. This paper summarizes the key insights and discussions from the workshop, acknowledges the barriers to EHR implementation in clinical research, and identifies practical solutions for engaging stakeholders (i.e., academia, industry, regulatory bodies, policy makers, patients, and EHR vendors) in the implementation of EHRs in clinical research.
Overview of electronic health records
Broadly defined, EHRs represent longitudinal data (in electronic format) that are collected during routine delivery of health care [ 3 ]. EHRs generally contain demographic, vital statistics, administrative, claims (medical and pharmacy), clinical, and patient-centered (e.g., originating from health-related quality-of-life instruments, home-monitoring devices, and frailty or caregiver assessments) data. The scope of an EHR varies widely across the world. Systems originating primarily as billing systems were not designed to support clinical work flow. Moving forward, EHR should be designed to optimize diagnosis and clinical care, which will enhance their relevance for clinical research. The EHR may reflect single components of care (e.g., primary care, emergency department, and intensive care unit) or data from an integrated hospital-wide or inter-hospital linked system [ 4 ]. EHRs may also change over time, reflecting evolving technology capabilities or external influences (e.g., changes in type of data collected related to coding or reimbursement practices).
EHRs emerged largely as a means to improve healthcare quality [ 5 – 7 ] and to capture billing data. EHRs may potentially be used to assess study feasibility, facilitate patient recruitment, streamline data collection, or conduct entirely EHR-based observational, embedded pragmatic, or post-marketing randomized registry studies, or comparative effectiveness studies. The various applications of EHRs for observational studies, safety surveillance, clinical research, and regulatory purposes are shown in Table 1 [ 3 , 8 – 10 ].
Electronic health records for research applications
Epidemiologic and observational research.
EHR data have been used to support observational studies, either as stand-alone data or following linkage to primary research data or other administrative data sets [ 3 , 11 – 14 ]. For example, the initial Euro Heart Survey [ 15 ] and subsequent Eurobservational Research Program (EORP) [ 16 ], the American College of Cardiology National Cardiovascular Data Registry (ACC-NCDR) [ 14 ], National Registry of Myocardial Infarction (NRMI), and American Heart Association Get With the Guidelines (AHA GWTG) [ 17 ] represent clinical data (collected from health records into an electronic case report form [eCRF] designed for the specific registry) on the management of patients across a spectrum of different cardiovascular diseases. However, modern EHR systems can minimize or eliminate the need for duplicate data collection (i.e., in a separate registry-specific eCRF), are capable of integrating large amounts of medical information accumulated throughout the patient’s life, enabling longitudinal study of diseases using the existing informatics infrastructure [ 18 ]. For example, EHR systems increasingly house imaging data which provide more detailed disease characterization than previously available in most observational data sets. In some countries (e.g., Farr Institute in Scotland [ 19 ]), the EHR can be linked, at an individual level, to other data sets, including general population health and lifestyle surveys, disease registries, and data collected by other sectors (e.g., education, housing, social care, and criminal justice). EHR data support a wide range of epidemiological research on the natural history of disease, drug utilization, and safety, as well as health services research.
Safety surveillance and regulatory uses
Active post-marketing safety surveillance and signal detection are important, emerging applications for EHRs, because they can provide realistic rates of events (unlike spontaneous event reports) and information on real-world use of drugs [ 20 ]. The EU-ADR project linked 8 databases in four European countries (Denmark, Italy, The Netherlands, United Kingdom) to enable analysis of select target adverse drug events [ 21 ]. The European Medicines Agency (EMA) coordinates the European Network of Centres for Pharmacoepidemiology and Pharmacovigilance (ENCePP) which aims to conduct post-marketing risk assessment using various EHR sources [ 22 , 23 ]. In the United States, the Food and Drug Administration (FDA) uses EHR data from several different sources (e.g., Sentinel and Mini-Sentinel System [ 24 ], Centers for Medicare and Medicaid Services [CMS], Veterans Affairs, Department of Defense, Substance Abuse and Mental Health Services Administration) to support post-marketing safety investigations [ 25 ].
Prospective clinical research
National patient registries that contain data extracted from the EHR are an accepted modality to assess guideline adherence and the effectiveness of performance improvement initiatives [ 26 – 33 ]. However, the use of EHRs for prospective clinical research is still limited, despite the fact that data collected for routine medical care overlap considerably with data collected for research. The most straightforward and generally accepted application for EHR is assessing trial feasibility and facilitating patient recruitment, and EHRs are currently used for this purpose in some centers. Using EHR technology to generate lists of patients who might be eligible for research is recognized as an option to meet meaningful use standards for EHR in the United States [ 6 ]. However, incomplete data may prohibit screening for the complete list of eligibility criteria [ 34 ], but EHRs may facilitate pre-screening of patients by age, gender, and diagnosis, particularly for exclusion of ineligible patients, and reduce the overall screening burden in clinical trials [ 35 ]. A second, and more complex, step involves the reuse of information collected in EHRs for routine clinical care as source data for research. Using EHRs as the source for demographic information, co-morbidities, and concomitant medications has several advantages over separately recording these data into an eCRF. Transcription errors may be reduced, since EHR data are entered by providers directly involved in a patient’s care as opposed to secondary eCRF entry by study personnel. The eCRF may be a redundant and costly step in a clinical trial, since local health records (electronic or paper) are used to verify source data entered into the eCRF. Finally, EHRs might enhance patient safety and reduce timelines if real-time EHR systems are used in clinical trials, in contrast to delays encountered with manual data entry into an eCRF. The EHR may facilitate implementation of remote data monitoring, which has the potential to greatly reduce clinical trial costs. The Innovative Medicine Initiative (IMI) Electronic Health Records for Clinical Research (EHR4CR, http://www.ehr4cr.eu ) project is one example, where tools and processes are being developed to facilitate reuse of EHR data for clinical research purposes. Systems to assess protocol feasibility and identify eligible patients for recruitment have been implemented, and efforts to link EHRs with clinical research electronic data collection are ongoing [ 36 ].
A shift towards pragmatic trials has been proposed as a mechanism to improve clinical trial efficiency [ 37 ]. Most of the data in a pragmatic trial are collected in the context of routine clinical care, which reduce trial-specific clinic visits and assessments, and should also reduce costs [ 38 ]. This concept is being applied in the National Institutes of Health (NIH) Health Care Systems Research Collaboratory. Trials conducted within the NIH Collaboratory aim to answer questions related to care delivery and the EHR contains relevant data for this purpose. Studies may have additional data collection modules if variables not routinely captured in the EHR are needed for a specific study. Similarly, the Patient-Centered Outcomes Research Institute (PCORI) has launched PCORnet, a research network that uses a common data platform alongside the existing EHR to conduct observational and interventional comparative effectiveness research [ 9 , 39 , 40 ].
The integration of EHRs in the conventional randomized controlled trials intended to support a new indication is more complex. EHRs may be an alternative to eCRFs when data collection is focused and limited to critical variables that are consistently collected in routine clinical care. Regulatory feedback indicates that while a new indication for a marketed drug might be achieved through EHRs, first marketing authorization using data entirely from EHRs would most likely not be possible with current systems until validation studies are performed and reviewed by regulatory agencies. The EHR could also be used to collect serious adverse events (SAE) that result in hospitalization, or to collect endpoints that do not necessarily require blinded adjudication (e.g., death), although the utility of EHRs for this purpose is dependent on the type of endpoint, whether it can reliably be identified in the EHR, and the timeliness of EHR data availability. Events that are coded for reimbursement (e.g., hospitalizations, MI) or new diagnoses, where disease-specific therapy is initiated (e.g., initiation of glucose lowering drugs to define new onset diabetes) tend to be more reliable. The reliability of endpoint collection varies by region and depends on the extent of linkage between different databases.
Challenges to using electronic health records in clinical trials and steps toward solutions
Challenges to using EHRs in clinical trials have been identified, related to data quality and validation, complete data capture, heterogeneity between systems, and developing a working knowledge across systems (Table 2 ). Ongoing projects, such as those conducted within the NIH Collaboratory and PCORnet [ 39 , 41 ] in the United States or the Farr Institute of Health Informatics Research in Scotland, have demonstrated the feasibility of using EHRs for aspects of clinical research, particularly comparative effectiveness. The success of these endeavors is connected to careful planning by a multi-stakeholder group committed to patient privacy, data security, fair governance, robust data infrastructure, and quality science from the outset. The next hurdle is to adapt the accrued knowledge for application to a broader base of clinical trials.
Data quality and validation
Data quality and validation are key factors in determining whether EHRs might be suitable data sources in clinical trials. Concerns about coding inaccuracies or bias introduced by selection of codes driven by billing incentives rather than clinical care may be diminished when healthcare providers enter data directly into the EHRs or when EHRs are used throughout all areas of the health-system, but such systems have not yet been widely implemented [ 42 ]. Excessive or busy workloads may also contribute to errors in clinician data entry [ 43 ]. Indeed, errors in EHRs have been reported [ 43 – 45 ].
Complete data capture is also a critical aspect of using EHRs for clinical research, particularly if EHRs are used for endpoint ascertainment or SAE collection. Complete data capture can be a major barrier in regions, where patients receive care from different providers or hospitals operating in different EHR systems that are not linked.
Consistent, validated methods for assessing data quality and completeness have not yet been adopted [ 46 ], but validation is a critical factor for the regulatory acceptance of EHR data. Proposed validation approaches include using both an eCRF and EHRs in a study in parallel and comparing results using the two data collection methods. This approach will require collaborative efforts to embed EHR substudies in large cardiovascular studies conducted by several sponsors. Assessing selected outcomes of interest from several EHR-based trials to compare different methodologies with an agreed statistical framework will be required to gauge precision of data collection via EHRs. A hybrid approach has also been proposed, where the EHR is used to identify study endpoints (e.g., death, hospitalization, myocardial infarction, and cancer), followed by adjudication and validation of EHR findings using clinical data (e.g., electrocardiogram and laboratory data).
Validity should be defined a priori and should be specific to the endpoints of interest as well as relevant to the country or healthcare system. Validation studies should aim to assess both the consistency between EHR data and standard data collection methods, and also how identified differences influence a study’s results. Proposed uses of EHRs for registration trials and methods for their validation will likely be considered by regulatory agencies on a case-by-case basis, because of the limited experience with EHRs for this purpose at the current time. Collaboration among industry sponsors to share cumulative experiences with EHR validation studies might lead to faster acceptance by regulatory authorities.
The ESC-CRT recommends that initial efforts to integrate EHRs in clinical trials focus on a few efficacy endpoints of interest, preferably objective endpoints (e.g., all-cause or cause-specific mortality) that are less susceptible to bias or subjective interpretation. As noted above, mortality may be incompletely captured in EHRs, particularly if patients die outside of the hospital, or at another institution using a non-integrated EHR. Thus, methods to supplement endpoint ascertainment in the EHR may be necessary if data completeness is uncertain. Standardized endpoint definitions based on the EHR should be included in the study protocol and analysis plan. A narrow set of data elements for auditing should be prospectively defined to ensure the required variables which are contained in the EHR.
Early interaction between sponsors, clinical investigators, and regulators is recommended to enable robust designs for clinical trials aiming to use EHRs for endpoint ascertainment. Plans to translate Good Clinical Practice into an EHR facilitated research environment should be described. Gaps in personnel training and education should be identified and specific actions to address training deficiencies should be communicated to regulators and in place prior to the start of the trial.
Timely access to electronic health record data
The potential for delays in data access is an important consideration when EHRs are used in clinical trials. EHRs may contain data originally collected as free text that was later coded for the EHR. Thus, coded information may not be available for patient identification/recruitment during the admission. Similarly, coding may occur weeks or months after discharge. In nationally integrated systems, data availability may also be delayed. These delays may be critical depending on the purpose of data extracted from the EHR (e.g., SAE reporting, source data, or endpoints in a time-sensitive study).
Heterogeneity between systems
Patients may be treated by multiple healthcare providers who operate independently of one another. Such patients may have more than one EHR, and these EHRs may not be linked. This heterogeneity adds to the complexity of using EHRs for clinical trials, since data coordinating centres have to develop processes for interacting or extracting data from any number of different systems. Differences in quality [ 47 ], non-standardized terminology, incomplete data capture, issues related to data sharing and data privacy, lack of common data fields, and the inability of systems to be configured to communicate with each other may also be problematic. Achieving agreement on a minimum set of common data fields to enable cross communication between systems would be a major step forward towards enabling EHRs to be used in clinical trials across centers and regions [ 48 , 49 ].
Data security and privacy
Privacy issues and information governance are among the most complex aspects of implementing EHRs for clinical research, in part because attitudes and regulations related to data privacy vary markedly around the world. Data security and appropriate use are high priorities, but access should not be restricted to the extent that the data are of limited usefulness. Access to EHR data by regulatory agencies will be necessary for auditing purposes in registration trials. Distributed analyses have the advantage of allowing data to remain with the individual site and under its control [ 39 , 41 ].
Pre-trial planning is critical to anticipate data security issues and to develop optimal standards and infrastructure. For pivotal registration trials, patients should be informed during the consent process about how their EHRs will be used and by whom. Modified approaches to obtaining informed consent for comparative effectiveness research studies of commonly used clinical practices or interventions may be possible [ 50 ]. A general upfront consent stating that EHR data may be used for research is a proactive step that may minimize later barriers to data access, although revision of existing legislation or ethics board rules may be needed to allow this approach. Patients and the public should be recognized as important stakeholders, and they can be advocates for clinical research using EHRs and improve the quality of EHR-based research if they are educated and engaged in the process and the purpose and procedures for EHR use are transparent. Developing optimal procedures for ensuring patients that are informed and protected, balanced with minimizing barriers to research is a major consideration as EHR-based research advances.
System capabilities
EHRs for use in clinical research need a flexible architecture to accommodate studies of different interventions or disease states. EHR systems may be capable of matching eligibility criteria to relevant data fields and flagging potential trial subjects to investigators. Patient questionnaires and surveys can be linked to EHRs to provide additional context to clinical data. Pre-population of eCRFs has been proposed as a potential role for EHRs, but the proportion of fields in an EHR that can be mapped to an eCRF varies substantially across systems.
EHRs may be more suitable for pragmatic trials where data collection mirrors those variables collected in routine clinical care. Whether regulators would require collection of additional elements to support a new drug or new indication depends on the drug, intended indication, patient population, and potential safety concerns.
Sustainability
The sustainability of EHRs in clinical research will largely depend on the materialization of their promised efficiencies. Programs like the NIH Collaboratory [ 41 ] and PCORnet [ 39 , 41 ], and randomized registry trials [ 51 , 52 ] are demonstrating the feasibility of these more efficient approaches to clinical research. The sustainability of using EHRs for pivotal registration clinical trials will depend on regulatory acceptance of the approach and whether the efficiencies support a business case for their use.
Role of stakeholders
To make the vision of EHRs in clinical trials a reality, stakeholders should collaborate and contribute to the advancement of EHRs for research. Professional bodies, such as the ESC, can play a major role in the training and education of researchers and the public about the potential value of EHR. Clinical trialists and industry must be committed to advancing validation methodology [ 53 ]. Investigators should develop, conduct, and promote institutional EHR trials that change clinical practice; such experience may encourage EHR trial adoption by industry and the agencies. Development of core or minimal data sets could streamline the process, reduce redundancy and heterogeneity, and decrease start-up time for future EHR-based clinical trials. These and other stakeholder contributions are outlined in Table 3 .
Electronic health records are a promising resource to improve the efficiency of clinical trials and to capitalize on novel research approaches. EHRs are useful data sources to support comparative effectiveness research and new trial designs that may answer relevant clinical questions as well as improve efficiency and reduce the cost of cardiovascular clinical research. Initial experience with EHRs has been encouraging, and accruing knowledge will continue to transform the application of EHRs for clinical research. The pace of technology has produced unprecedented analytic capabilities, but these must be pursued with appropriate measures in place to manage security, privacy, and ensure adequacy of informed consent. Ongoing programs have implemented creative solutions for these issues using distributed analyses to allow organizations to retain data control and by engaging patient stakeholders. Whether EHRs can be successfully applied to the conventional drug development in pivotal, registration trials remains to be seen and will depend on demonstration of data quality and validity, as well as realization of expected efficiencies.
Jackson N, Atar D, Borentain M, Breithardt G, van Eickels M, Endres M, Fraass U, Friede T, Hannachi H, Janmohamed S, Kreuzer J, Landray M, Lautsch D, Le Floch C, Mol P, Naci H, Samani N, Svensson A, Thorstensen C, Tijssen J, Vandzhura V, Zalewski A, Kirchhof P (2016) Improving clinical trials for cardiovascular diseases: a position paper from the Cardiovascular Roundtable of the European Society of Cardiology. Eur Heart J 37:747–754
Article PubMed Google Scholar
Eisenstein EL, Collins R, Cracknell BS, Podesta O, Reid ED, Sandercock P, Shakhov Y, Terrin ML, Sellers MA, Califf RM, Granger CB, Diaz R (2008) Sensible approaches for reducing clinical trial costs. Clin Trials 5:75–84
Denaxas SC, Morley KI (2015) Big biomedical data and cardiovascular disease research: opportunities and challenges. European Heart Journal - Quality of Care and Clinical Outcomes 1:9–16
Article Google Scholar
Hayrinen K, Saranto K, Nykanen P (2008) Definition, structure, content, use and impacts of electronic health records: a review of the research literature. Int J Med Inform 77:291–304
Appari A, Eric JM, Anthony DL (2013) Meaningful use of electronic health record systems and process quality of care: evidence from a panel data analysis of U.S. acute-care hospitals. Health Serv Res 48:354–375
Blumenthal D, Tavenner M (2010) The “meaningful use” regulation for electronic health records. N Engl J Med 363:501–504
Article CAS PubMed Google Scholar
Roumia M, Steinhubl S (2014) Improving cardiovascular outcomes using electronic health records. Curr Cardiol Rep 16:451
Doods J, Botteri F, Dugas M, Fritz F (2014) A European inventory of common electronic health record data elements for clinical trial feasibility. Trials 15:18
Article PubMed Central PubMed Google Scholar
Collins FS, Hudson KL, Briggs JP, Lauer MS (2014) PCORnet: turning a dream into reality. J Am Med Inform Assoc 21:576–577
James S, Rao SV, Granger CB (2015) Registry-based randomized clinical trials–a new clinical trial paradigm. Nat Rev Cardiol 12:312–316
Krumholz HM, Normand SL, Wang Y (2014) Trends in hospitalizations and outcomes for acute cardiovascular disease and stroke, 1999-2011. Circulation 130:966–975
Hlatky MA, Ray RM, Burwen DR, Margolis KL, Johnson KC, Kucharska-Newton A, Manson JE, Robinson JG, Safford MM, Allison M, Assimes TL, Bavry AA, Berger J, Cooper-DeHoff RM, Heckbert SR, Li W, Liu S, Martin LW, Perez MV, Tindle HA, Winkelmayer WC, Stefanick ML (2014) Use of Medicare data to identify coronary heart disease outcomes in the Women’s Health Initiative. Circ Cardiovasc Qual Outcomes 7:157–162
Chung SC, Gedeborg R, Nicholas O, James S, Jeppsson A, Wolfe C, Heuschmann P, Wallentin L, Deanfield J, Timmis A, Jernberg T, Hemingway H (2014) Acute myocardial infarction: a comparison of short-term survival in national outcome registries in Sweden and the UK. Lancet 383:1305–1312
Brindis RG, Fitzgerald S, Anderson HV, Shaw RE, Weintraub WS, Williams JF (2001) The American College of Cardiology-National Cardiovascular Data Registry (ACC-NCDR): building a national clinical data repository. J Am Coll Cardiol 37:2240–2245
Scholte op Reimer W, Gitt A, Boersma E, Simoons Me (2006) Cardiovascular diseases in Europe. Euro Heart Survey−2006. European Society of Cardiology, . Sophia Antipolis
Ferrari R (2010) EURObservational research programme. Eur Heart J 31:1023–1031
Smaha LA (2004) The American Heart Association Get With The Guidelines program. Am Heart J 148:S46–S48
Krumholz HM (2014) Big data and new knowledge in medicine: the thinking, training, and tools needed for a learning health system. Health Aff (Millwood) 33:1163–1170
Wood R, Clark D, King A, Mackay D, Pell J (2013) Novel cross-sectoral linkage of routine health and education data at an all-Scotland level: a feasibility study. Lancet 382(Supplement 3):S10
Cederholm S, Hill G, Asiimwe A, Bate A, Bhayat F, Persson BG, Bergvall T, Ansell D, Star K, Noren GN (2015) Structured assessment for prospective identification of safety signals in electronic medical records: evaluation in the health improvement network. Drug Saf 38:87–100
Trifiro G, Fourrier-Reglat A, Sturkenboom MC, Diaz AC, Van Der Lei J (2009) The EU-ADR project: preliminary results and perspective. Stud Health Technol Inform 148:43–49
PubMed Google Scholar
Eichler HG, Pignatti F, Flamion B, Leufkens H, Breckenridge A (2008) Balancing early market access to new drugs with the need for benefit/risk data: a mounting dilemma. Nat Rev Drug Discov 7:818–826
Goedecke T, Arlett P (2014) A Description of the European Network of Centres for pharmacoepidemiology and pharmacovigilance as a global resource for pharmacovigilance and pharmacoepidemiology. Mann’s pharmacovigilance. Wiley, New York, pp 403–408
Google Scholar
Ball R, Robb M, Anderson SA, Dal Pan G (2016) The FDA’s sentinel initiative-A comprehensive approach to medical product surveillance. Clin Pharmacol Ther 99:265–268
Staffa JA, Dal Pan GJ (2012) Regulatory innovation in postmarketing risk assessment and management. Clin Pharmacol Ther 91:555–557
Peterson ED, Shah BR, Parsons L, Pollack CV Jr, French WJ, Canto JG, Gibson CM, Rogers WJ (2008) Trends in quality of care for patients with acute myocardial infarction in the National Registry of Myocardial Infarction from 1990 to 2006. Am Heart J 156:1045–1055
Chan PS, Maddox TM, Tang F, Spinler S, Spertus JA (2011) Practice-level variation in warfarin use among outpatients with atrial fibrillation (from the NCDR PINNACLE program). Am J Cardiol 108:1136–1140
Article CAS PubMed Central PubMed Google Scholar
Maddox TM, Chan PS, Spertus JA, Tang F, Jones P, Ho PM, Bradley SM, Tsai TT, Bhatt DL, Peterson PN (2014) Variations in coronary artery disease secondary prevention prescriptions among outpatient cardiology practices: insights from the NCDR (National Cardiovascular Data Registry). J Am Coll Cardiol 63:539–546
Jernberg T, Attebring MF, Hambraeus K, Ivert T, James S, Jeppsson A, Lagerqvist B, Lindahl B, Stenestrand U, Wallentin L (2010) The Swedish Web-system for enhancement and development of evidence-based care in heart disease evaluated according to recommended therapies (SWEDEHEART). Heart 96:1617–1621
Cleland JG, Swedberg K, Follath F, Komajda M, Cohen-Solal A, Aguilar JC, Dietz R, Gavazzi A, Hobbs R, Korewicki J, Madeira HC, Moiseyev VS, Preda I, van Gilst WH, Widimsky J, Freemantle N, Eastaugh J, Mason J (2003) The EuroHeart Failure survey programme: a survey on the quality of care among patients with heart failure in Europe. Part 1: patient characteristics and diagnosis. Eur Heart J 24:442–463
Nieminen MS, Brutsaert D, Dickstein K, Drexler H, Follath F, Harjola VP, Hochadel M, Komajda M, Lassus J, Lopez-Sendon JL, Ponikowski P, Tavazzi L (2006) EuroHeart Failure Survey II (EHFS II): a survey on hospitalized acute heart failure patients: description of population. Eur Heart J 27:2725–2736
Tofield A (2010) EURObservational research programme. Eur Heart J 31:1023–1031
McNamara RL, Herrin J, Bradley EH, Portnay EL, Curtis JP, Wang Y, Magid DJ, Blaney M, Krumholz HM (2006) Hospital improvement in time to reperfusion in patients with acute myocardial infarction, 1999 to 2002. J Am Coll Cardiol 47:45–51
Kopcke F, Trinczek B, Majeed RW, Schreiweis B, Wenk J, Leusch T, Ganslandt T, Ohmann C, Bergh B, Rohrig R, Dugas M, Prokosch HU (2013) Evaluation of data completeness in the electronic health record for the purpose of patient recruitment into clinical trials: a retrospective analysis of element presence. BMC Med Inform Decis Mak 13:37
Thadani SR, Weng C, Bigger JT, Ennever JF, Wajngurt D (2009) Electronic screening improves efficiency in clinical trial recruitment. J Am Med Inform Assoc 16:869–873
De Moor G, Sundgren M, Kalra D, Schmidt A, Dugas M, Claerhout B, Karakoyun T, Ohmann C, Lastic PY, Ammour N, Kush R, Dupont D, Cuggia M, Daniel C, Thienpont G, Coorevits P (2015) Using electronic health records for clinical research: the case of the EHR4CR project. J Biomed Inform 53:162–173
Fordyce CB, Roe MT, Ahmad T, Libby P, Borer JS, Hiatt WR, Bristow MR, Packer M, Wasserman SM, Braunstein N, Pitt B, DeMets DL, Cooper-Arnold K, Armstrong PW, Berkowitz SD, Scott R, Prats J, Galis ZS, Stockbridge N, Peterson ED, Califf RM (2015) Cardiovascular drug development: is it dead or just hibernating? J Am Coll Cardiol 65:1567–1582
New JP, Bakerly ND, Leather D, Woodcock A (2014) Obtaining real-world evidence: the Salford Lung Study. Thorax 69:1152–1154
Fleurence RL, Curtis LH, Califf RM, Platt R, Selby JV, Brown JS (2014) Launching PCORnet, a national patient-centered clinical research network. J Am Med Inform Assoc 21:578–582
Hernandez AF, Fleurence RL, Rothman RL (2015) The ADAPTABLE Trial and PCORnet: shining light on a new research paradigm. Ann Intern Med 163:635–636
Curtis LH, Brown J, Platt R (2014) Four health data networks illustrate the potential for a shared national multipurpose big-data network. Health Aff (Millwood) 33:1178–1186
Jha AK, DesRoches CM, Campbell EG, Donelan K, Rao SR, Ferris TG, Shields A, Rosenbaum S, Blumenthal D (2009) Use of electronic health records in U.S. hospitals. N Engl J Med 360:1628–1638
Hersh WR, Weiner MG, Embi PJ, Logan JR, Payne PR, Bernstam EV, Lehmann HP, Hripcsak G, Hartzog TH, Cimino JJ, Saltz JH (2013) Caveats for the use of operational electronic health record data in comparative effectiveness research. Med Care 51:S30–S37
Brennan L, Watson M, Klaber R, Charles T (2012) The importance of knowing context of hospital episode statistics when reconfiguring the NHS. BMJ 344:e2432
Green SM (2013) Congruence of disposition after emergency department intubation in the National Hospital Ambulatory Medical Care Survey. Ann Emerg Med 61:423–426
Weiskopf NG, Weng C (2013) Methods and dimensions of electronic health record data quality assessment: enabling reuse for clinical research. J Am Med Inform Assoc 20:144–151
Elnahal SM, Joynt KE, Bristol SJ, Jha AK (2011) Electronic health record functions differ between best and worst hospitals. Am J Manag Care 17:e121–e147
PubMed Central PubMed Google Scholar
Flynn MR, Barrett C, Cosio FG, Gitt AK, Wallentin L, Kearney P, Lonergan M, Shelley E, Simoons ML (2005) The Cardiology Audit and Registration Data Standards (CARDS), European data standards for clinical cardiology practice. Eur Heart J 26:308–313
Simoons ML, van der Putten N, Wood D, Boersma E, Bassand JP (2002) The Cardiology Information System: the need for data standards for integration of systems for patient care, registries and guidelines for clinical practice. Eur Heart J 23:1148–1152
Sugarman J, Califf RM (2014) Ethics and regulatory complexities for pragmatic clinical trials. JAMA 311:2381–2382
Frobert O, Lagerqvist B, Olivecrona GK, Omerovic E, Gudnason T, Maeng M, Aasa M, Angeras O, Calais F, Danielewicz M, Erlinge D, Hellsten L, Jensen U, Johansson AC, Karegren A, Nilsson J, Robertson L, Sandhall L, Sjogren I, Ostlund O, Harnek J, James SK (2013) Thrombus aspiration during ST-segment elevation myocardial infarction. N Engl J Med 369:1587–1597
Hess CN, Rao SV, Kong DF, Aberle LH, Anstrom KJ, Gibson CM, Gilchrist IC, Jacobs AK, Jolly SS, Mehran R, Messenger JC, Newby LK, Waksman R, Krucoff MW (2013) Embedding a randomized clinical trial into an ongoing registry infrastructure: unique opportunities for efficiency in design of the Study of Access site For Enhancement of Percutaneous Coronary Intervention for Women (SAFE-PCI for Women). Am Heart J 166:421–428
Barry SJ, Dinnett E, Kean S, Gaw A, Ford I (2013) Are routinely collected NHS administrative records suitable for endpoint identification in clinical trials? Evidence from the West of Scotland Coronary Prevention Study. PLoS One 8:e75379
Download references
Acknowledgments
This paper was generated from discussions during a cardiovascular round table (CRT) Workshop organized on 23–24 April 2015 by the European Society of Cardiology (ESC). The CRT is a strategic forum for high-level dialogues between academia, regulators, industry, and ESC leadership to identify and discuss key strategic issues for the future of cardiovascular health in Europe and other parts of the world. We acknowledge Colin Freer for his participation in the meeting. This article reflects the views of the authors and should not be construed to represent FDA’s views or policies. The opinions expressed in this paper are those of the authors and cannot be interpreted as the opinion of any of the organizations that employ the authors. MRC’s salary is supported by the National Institute for Health Research (NIHR) Cardiovascular Biomedical Research Unit at the Royal Brompton Hospital, London, UK.
Conflict of interest
Martin R. Cowie: Research grants from ResMed, Boston Scientific, and Bayer; personal fees from ResMed, Boston Scientific, Bayer, Servier, Novartis, St. Jude Medical, and Pfizer. Juuso Blomster: Astra Zeneca employee. Lesley Curtis: Funding from FDA for work with the Mini-Sentinel program and from PCORI for work with the PCORnet program. Sylvie Duclaux: None. Ian Ford: None. Fleur Fritz: None. Samantha Goldman: None. Salim Janmohamed: GSK employee and shareholder. Jörg Kreuzer: Employee of Boehringer-Ingelheim. Mark Leenay: Employee of Optum. Alexander Michel: Bayer employee and shareholder. Seleen Ong: Employee of Pfizer. Jill Pell: None. Mary Ross Southworth: None. Wendy Gattis Stough: Consultant to European Society of Cardiology, Heart Failure Association of the European Society of Cardiology, European Drug Development Hub, Relypsa, CHU Nancy, Heart Failure Society of America, Overcome, Stealth BioTherapeutics, Covis Pharmaceuticals, University of Gottingen, and University of North Carolina. Martin Thoenes: Employee of Edwards Lifesciences. Faiez Zannad: Personal fees from Boston Scientific, Servier, Pfizer, Novartis, Takeda, Janssen, Resmed, Eli Lilly, CVRx, AstraZeneca, Merck, Stealth Peptides, Relypsa, ZS Pharma, Air Liquide, Quantum Genomics, Bayer for Steering Committee, Advisory Board, or DSMB member. Andrew Zalewski: Employee of GSK.
Author information
Authors and affiliations.
National Heart and Lung Institute, Imperial College London, Royal Brompton Hospital, Sydney Street, London, SW3 6HP, UK
Martin R. Cowie
Astra Zeneca R&D, Molndal, Sweden
Juuso I. Blomster
University of Turku, Turku, Finland
Duke Clinical Research Institute, Durham, NC, USA
Lesley H. Curtis
Servier, Paris, France
Sylvie Duclaux
Robertson Centre for Biostatistics, University of Glasgow, Glasgow, UK
University of Münster, Münster, Germany
Fleur Fritz
Daiichi-Sankyo, London, UK
Samantha Goldman
GlaxoSmithKline, Stockley Park, UK
Salim Janmohamed
Boehringer-Ingelheim, Pharma GmbH & Co KG, Ingelheim, Germany
Jörg Kreuzer
Optum International, London, UK
Mark Leenay
Bayer Pharma, Berlin, Germany
Alexander Michel
Pfizer Ltd., Surrey, UK
Institute of Health and Wellbeing, University of Glasgow, Glasgow, UK
Jill P. Pell
Food and Drug Administration, Silver Spring, MD, USA
Mary Ross Southworth
Campbell University College of Pharmacy and Health Sciences, Campbell, NC, USA
Wendy Gattis Stough
Edwards LifeSciences, Nyon, Switzerland
Martin Thoenes
INSERM, Centre d’Investigation Clinique 9501 and Unité 961, Centre Hospitalier Universitaire, Nancy, France
Faiez Zannad
Department of Cardiology, Nancy University, Université de Lorraine, Nancy, France
Glaxo Smith Kline, King of Prussia, Pennsylvania, USA
Andrew Zalewski
You can also search for this author in PubMed Google Scholar
Corresponding author
Correspondence to Martin R. Cowie .
Rights and permissions
Open Access This article is distributed under the terms of the Creative Commons Attribution 4.0 International License ( http://creativecommons.org/licenses/by/4.0/ ), which permits unrestricted use, distribution, and reproduction in any medium, provided you give appropriate credit to the original author(s) and the source, provide a link to the Creative Commons license, and indicate if changes were made.
Reprints and permissions
About this article
Cowie, M.R., Blomster, J.I., Curtis, L.H. et al. Electronic health records to facilitate clinical research. Clin Res Cardiol 106 , 1–9 (2017). https://doi.org/10.1007/s00392-016-1025-6
Download citation
Received : 04 May 2016
Accepted : 05 August 2016
Published : 24 August 2016
Issue Date : January 2017
DOI : https://doi.org/10.1007/s00392-016-1025-6
Share this article
Anyone you share the following link with will be able to read this content:
Sorry, a shareable link is not currently available for this article.
Provided by the Springer Nature SharedIt content-sharing initiative
- Electronic health records
- Clinical trials as topic
- Pragmatic clinical trials as topic
- Cardiovascular diseases
- Find a journal
- Publish with us
- Track your research
Suggestions or feedback?
MIT News | Massachusetts Institute of Technology
- Machine learning
- Social justice
- Black holes
- Classes and programs
Departments
- Aeronautics and Astronautics
- Brain and Cognitive Sciences
- Architecture
- Political Science
- Mechanical Engineering
Centers, Labs, & Programs
- Abdul Latif Jameel Poverty Action Lab (J-PAL)
- Picower Institute for Learning and Memory
- Lincoln Laboratory
- School of Architecture + Planning
- School of Engineering
- School of Humanities, Arts, and Social Sciences
- Sloan School of Management
- School of Science
- MIT Schwarzman College of Computing
Toward a smarter electronic health record
Press contact :, media download.
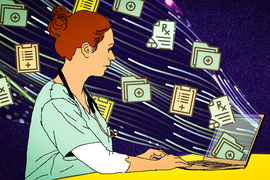
*Terms of Use:
Images for download on the MIT News office website are made available to non-commercial entities, press and the general public under a Creative Commons Attribution Non-Commercial No Derivatives license . You may not alter the images provided, other than to crop them to size. A credit line must be used when reproducing images; if one is not provided below, credit the images to "MIT."
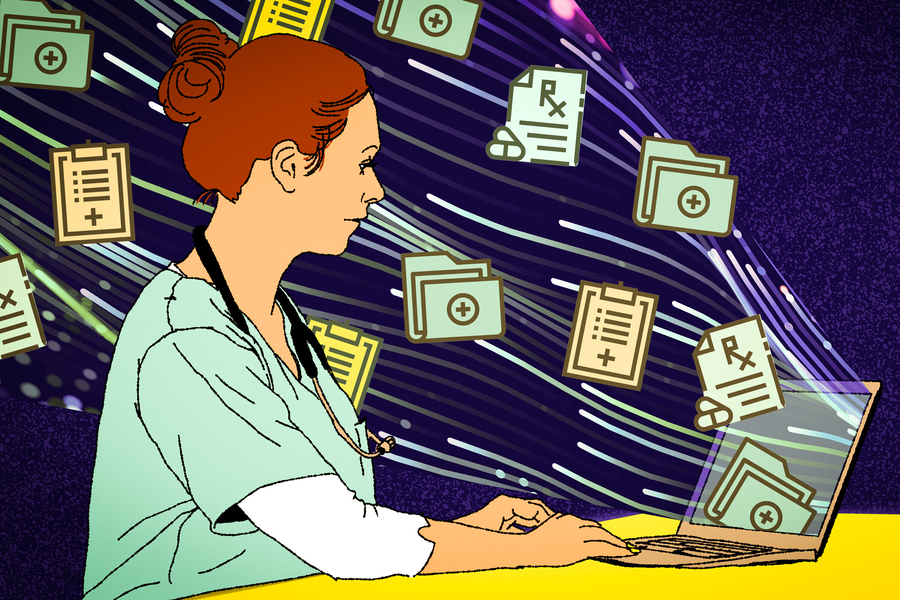
Previous image Next image
Electronic health records have been widely adopted with the hope they would save time and improve the quality of patient care. But due to fragmented interfaces and tedious data entry procedures, physicians often spend more time navigating these systems than they do interacting with patients.
Researchers at MIT and the Beth Israel Deaconess Medical Center are combining machine learning and human-computer interaction to create a better electronic health record (EHR). They developed MedKnowts, a system that unifies the processes of looking up medical records and documenting patient information into a single, interactive interface.
Driven by artificial intelligence, this “smart” EHR automatically displays customized, patient-specific medical records when a clinician needs them. MedKnowts also provides autocomplete for clinical terms and auto-populates fields with patient information to help doctors work more efficiently.
“In the origins of EHRs, there was this tremendous enthusiasm that getting all this information organized would be helpful to be able to track billing records, report statistics to the government, and provide data for scientific research. But few stopped to ask the deep questions around whether they would be of use for the clinician. I think a lot of clinicians feel they have had this burden of EHRs put on them for the benefit of bureaucracies and scientists and accountants. We came into this project asking how EHRs might actually benefit clinicians,” says David Karger, professor of computer science in the Computer Science and Artificial Intelligence Laboratory (CSAIL) and senior author of the paper.
The research was co-authored by CSAIL graduate students Luke Murray, who is the lead author, Divya Gopinath, and Monica Agrawal. Other authors include Steven Horng, an emergency medicine attending physician and clinical lead for machine learning at the Center for Healthcare Delivery Science of Beth Israel Deaconess Medical Center, and David Sontag, associate professor of electrical engineering and computer science at MIT and a member of CSAIL and the Institute for Medical Engineering and Science, and a principal investigator at the Abdul Latif Jameel Clinic for Machine Learning in Health. It will be presented at the Association for Computing Machinery Symposium on User Interface Software and Technology next month.
A problem-oriented tool
To design an EHR that would benefit doctors, the researchers had to think like doctors.
They created a note-taking editor with a side panel that displays relevant information from the patient’s medical history. That historical information appears in the form of cards that are focused on particular problems or concepts.
For instance, if MedKnowts identifies the clinical term “diabetes” in the text as a clinician types, the system automatically displays a “diabetes card” containing medications, lab values, and snippets from past records that are relevant to diabetes treatment.
Most EHRs store historical information on separate pages and list medications or lab values alphabetically or chronologically, forcing the clinician to search through data to find the information they need, Murray says. MedKnowts only displays information relevant to the particular concept the clinician is writing about.
“This is a closer match to the way doctors think about information. A lot of times, doctors will do this subconsciously. They will look through a medications page and only focus on the medications that are relevant to the current conditions. We are helping to do that process automatically and hopefully move some things out of the doctor’s head so they have more time to think about the complex part, which is determining what is wrong with the patient and coming up with a treatment plan,” Murray says.
Pieces of interactive text called chips serve as links to related cards. As a physician types a note, the autocomplete system recognizes clinical terms, such as medications, lab values, or conditions, and transforms them into chips. Each chip is displayed as a word or phrase that has been highlighted in a certain color depending on its category (red for a medical condition, green for a medication, yellow for a procedure, etc.)
Through the use of autocomplete, structured data on the patient’s conditions, symptoms, and medication usage is collected with no additional effort from the physician.
Sontag says he hopes the advance will “change the paradigm of how to create large-scale health datasets for studying disease progression and assessing the real-world effectiveness of treatments.”
In practice
After a year-long iterative design process, the researchers tested MedKnowts by deploying the software in the emergency department at Beth Israel Deaconess Medical Center in Boston. They worked with an emergency physician and four hospital scribes who enter notes into the electronic health record.
Deploying the software in an emergency department, where doctors operate in a high-stress environment, involved a delicate balancing act, Agrawal says.
“One of the biggest challenges we faced was trying to get people to shift what they currently do. Doctors who have used the same system, and done the same dance of clicks so many times, form a sort of muscle memory. Whenever you are going to make a change, there is a question of is this worth it? And we definitely found that some features had greater usage than others,” she says.
The Covid-19 pandemic complicated the deployment, too. The researchers had been visiting the emergency department to get a sense of the workflow, but were forced to end those visits due to Covid-19 and were unable to be in the hospital while the system was being deployed.
Despite those initial challenges, MedKnowts became popular with the scribes over the course of the one-month deployment. They gave the system an average rating of 83.75 (out of 100) for usability.
Scribes found the autocomplete function especially useful for speeding up their work, according to survey results. Also, the color-coded chips helped them quickly scan notes for relevant information.
Those initial results are promising, but as the researchers consider the feedback and work on future iterations of MedKnowts, they plan to proceed with caution.
“What we are trying to do here is smooth the pathway for doctors and let them accelerate. There is some risk there. Part of the purpose of bureaucracy is to slow things down and make sure all the i’s are dotted and all the t’s are crossed. And if we have a computer dotting the i’s and crossing the t’s for doctors, that may actually be countering the goals of the bureaucracy, which is to force doctors to think twice before they make a decision. We have to be thinking about how to protect doctors and patients from the consequences of making the doctors more efficient,” Karger says.
A longer-term vision
The researchers plan to improve the machine learning algorithms that drive MedKnowts so the system can more effectively highlight parts of the medical record that are most relevant, Agrawal says.
They also want to consider the needs of different medical users. The researchers designed MedKnowts with an emergency department in mind — a setting where doctors are typically seeing patients for the first time. A primary care physician who knows their patients much better would likely have some different needs.
In the longer-term, the researchers envision creating an adaptive system that clinicians can contribute to. For example, perhaps a doctor realizes a certain cardiology term is missing from MedKnowts and adds that information to a card, which would update the system for all users.
The team is exploring commercialization as an avenue for further deployment.
“We want to build tools that let doctors create their own tools. We don’t expect doctors to learn to be programmers, but with the right support they might be able to radically customize whatever medical applications they are using to really suit their own needs and preferences,” Karger says.
This research was funded by the MIT Abdul Latif Jameel Clinic for Machine Learning in Health.
Share this news article on:
Related links.
- David Karger
- David Sontag
- Computer Science and Artificial Intelligence Laboratory
- Abdul Latif Jameel Clinic for Machine Learning in Health
- Institute for Medical Engineering and Science
- Department of Electrical Engineering and Computer Science
Related Topics
- Health care
- Artificial intelligence
- Electrical Engineering & Computer Science (eecs)
- Computer Science and Artificial Intelligence Laboratory (CSAIL)
- Institute for Medical Engineering and Science (IMES)
Related Articles
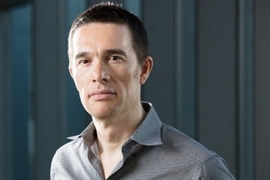
3 Questions: John Van Reenen on the impact of technology on health care workers
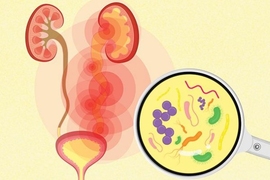
Algorithm reduces use of riskier antibiotics for UTIs
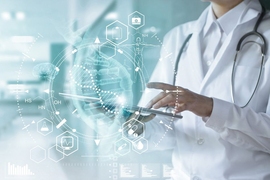
How well can computers connect symptoms to diseases?
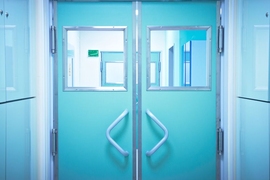
Model improves prediction of mortality risk in ICU patients
Previous item Next item
More MIT News
A biomedical engineer pivots from human movement to women’s health
Read full story →
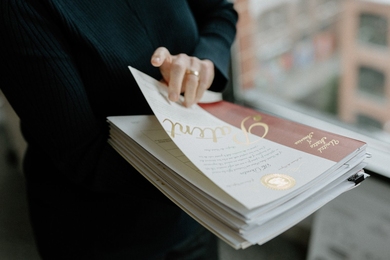
MIT tops among single-campus universities in US patents granted
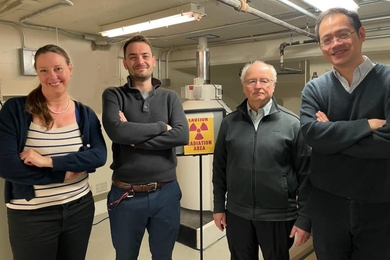
A new way to detect radiation involving cheap ceramics
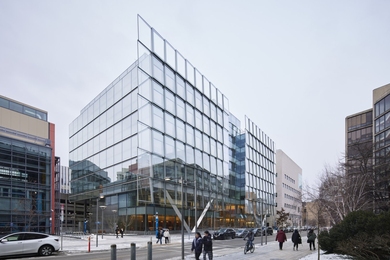
A crossroads for computing at MIT
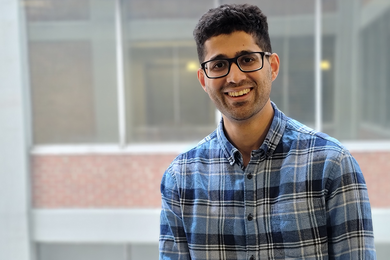
Growing our donated organ supply
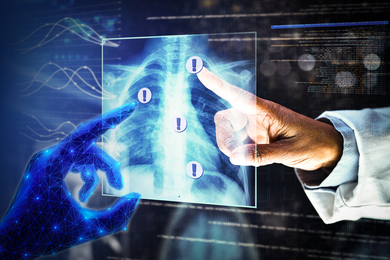
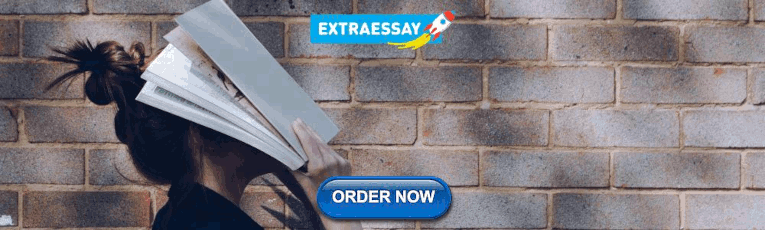
New AI method captures uncertainty in medical images
- More news on MIT News homepage →
Massachusetts Institute of Technology 77 Massachusetts Avenue, Cambridge, MA, USA
- Map (opens in new window)
- Events (opens in new window)
- People (opens in new window)
- Careers (opens in new window)
- Accessibility
- Social Media Hub
- MIT on Facebook
- MIT on YouTube
- MIT on Instagram
- Research article
- Open access
- Published: 04 September 2014
Implementing electronic health records in hospitals: a systematic literature review
- Albert Boonstra 1 ,
- Arie Versluis 2 &
- Janita F J Vos 1
BMC Health Services Research volume 14 , Article number: 370 ( 2014 ) Cite this article
93k Accesses
169 Citations
55 Altmetric
Metrics details
The literature on implementing Electronic Health Records (EHR) in hospitals is very diverse. The objective of this study is to create an overview of the existing literature on EHR implementation in hospitals and to identify generally applicable findings and lessons for implementers.
A systematic literature review of empirical research on EHR implementation was conducted. Databases used included Web of Knowledge, EBSCO, and Cochrane Library. Relevant references in the selected articles were also analyzed. Search terms included Electronic Health Record (and synonyms), implementation, and hospital (and synonyms). Articles had to meet the following requirements: (1) written in English, (2) full text available online, (3) based on primary empirical data, (4) focused on hospital-wide EHR implementation, and (5) satisfying established quality criteria.
Of the 364 initially identified articles, this study analyzes the 21 articles that met the requirements. From these articles, 19 interventions were identified that are generally applicable and these were placed in a framework consisting of the following three interacting dimensions: (1) EHR context, (2) EHR content, and (3) EHR implementation process.
Conclusions
Although EHR systems are anticipated as having positive effects on the performance of hospitals, their implementation is a complex undertaking. This systematic review reveals reasons for this complexity and presents a framework of 19 interventions that can help overcome typical problems in EHR implementation. This framework can function as a reference for implementers in developing effective EHR implementation strategies for hospitals.
Peer Review reports
In recent years, Electronic Health Records (EHRs) have been implemented by an ever increasing number of hospitals around the world. There have, for example, been initiatives, often driven by government regulations or financial stimulations, in the USA [ 1 ], the United Kingdom [ 2 ] and Denmark [ 3 ]. EHR implementation initiatives tend to be driven by the promise of enhanced integration and availability of patient data [ 4 ], by the need to improve efficiency and cost-effectiveness [ 5 ], by a changing doctor-patient relationship toward one where care is shared by a team of health care professionals [ 5 ], and/or by the need to deal with a more complex and rapidly changing environment [ 6 ].
EHR systems have various forms, and the term can relate to a broad range of electronic information systems used in health care. EHR systems can be used in individual organizations, as interoperating systems in affiliated health care units, on a regional level, or nationwide [ 1 , 2 ]. Health care units that use EHRs include hospitals, pharmacies, general practitioner surgeries, and other health care providers [ 7 ].
The implementation of hospital-wide EHR systems is a complex matter involving a range of organizational and technical factors including human skills, organizational structure, culture, technical infrastructure, financial resources, and coordination [ 8 , 9 ]. As Grimson et al. [ 5 ] argue, implementing information systems (IS) in hospitals is more challenging than elsewhere because of the complexity of medical data, data entry problems, security and confidentiality concerns, and a general lack of awareness of the benefits of Information Technology (IT). Boonstra and Govers [ 10 ] provide three reasons why hospitals differ from many other industries, and these differences might also affect EHR implementations. The first reason is that hospitals have multiple objectives, such as curing and caring for patients, and educating new physicians and nurses. Second, hospitals have complicated and highly varied structures and processes. Third, hospitals have a varied workforce including medical professionals who possess high levels of expertise, power, and autonomy. These distinct characteristics justify a study that focuses on the identification and analysis of the findings of previous studies on EHR implementation in hospitals.
Study aim, theoretical framework, and terminology
In dealing with the complexity of EHR implementation in hospitals, it is helpful to know which factors are seen as important in the literature and to capture the existing knowledge on EHR implementation in hospitals. As such, the objective of this research is to identify, categorize, and analyze the existing findings in the literature on EHR implementation processes in hospitals. This could contribute to greater insight into the underlying patterns and complex relationships involved in EHR implementation and could identify ways to tackle EHR implementation problems. In other words, this study focusses on the identification of factors that determine the progress of EHR implementation in hospitals. The motives behind implementing EHRs in hospitals and the effects on performance of implemented EHR systems are beyond the scope of this paper.
To our knowledge, there have been no systematic reviews of the literature concerning EHR implementation in hospitals and this article therefore fills that gap. Two interesting related review studies on EHR implementation are Keshavjee et al. [ 11 ] and McGinn et al. [ 12 ]. The study of Keshavjee et al. [ 11 ] develops a literature based integrative framework for EHR implementation. McGinn et al. [ 12 ] adopt an exclusive user perspective on EHR and their study is limited to Canada and countries with comparable socio-economic levels. Both studies are not explicitly focused on hospitals and include other contexts such as small clinics and national or regional EHR initiatives.
This systematic review is explicitly focused on hospital-wide, single hospital EHR implementations and identifies empirical studies (that include collected primary data) that reflect this situation. The categorization of the findings from the selected articles draws on Pettigrew’s framework for understanding strategic change [ 13 ]. This model has been widely applied in case study research into organizational contexts [ 14 ], as well as in studies on the implementation of health care innovations [ 15 ]. It generates insights by analyzing three interactive dimensions – context , content , and process – that together shape organizational change. Pettigrew’s framework [ 13 ] is seen as applicable because implementing an EHR artefact is an organization-wide effort. This framework was specifically selected for its focus on organizational change, its ease of understanding, and its relatively general dimensions allowing a broad range of findings to be included. The framework structures and focusses the analysis of the findings from the selected articles.
An organization’s context can be divided into internal and external components. External context refers to the social, economic, political, and competitive environments in which an organization operates. The internal context refers to the structure, culture, resources, capabilities, and politics of an organization. The content covers the specific areas of the transformation under examination. In an EHR implementation, these are the EHR system itself (both hardware and software), the work processes, and everything related to these (e.g. social conditions). The process dimension concerns the processes of change, made up of the plans, actions, reactions, and interactions of the stakeholders, rather than work processes in general. It is important to note that Pettigrew [ 13 ] does not see strategic change as a rational analytical process but rather as an iterative, continuous, multilevel process. This highlights that the outcome of an organizational change will be determined by the context, content, and process of that change. The framework with its three categories, shown in Figure 1 , illustrates the conceptual model used to categorize the findings of this systematic literature review.
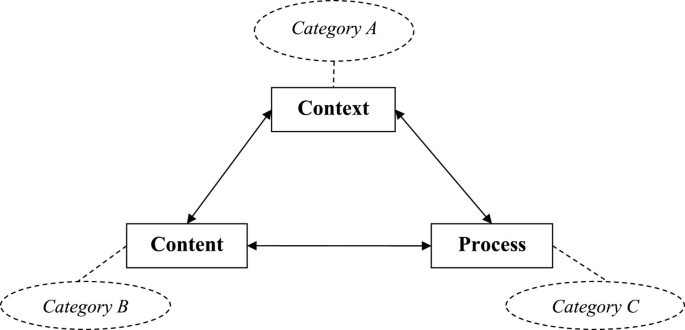
Pettigrew ’ s framework [ 13 ] ] and the corresponding categories.
In the literature, several terms are used to refer to electronic medical information systems. In this article, the term Electronic Health Record (EHR) is used throughout. Commonly used terms identified by ISO (the International Organization for Standardization) [ 16 ] plus another not identified by ISO are outlined below and used in our search. ISO considers Electronic Health Record (EHR) to be an overall term for “ a repository of information regarding the health status of a subject of care , in computer processable form ” [ 16 ], p. 13. ISO uses different terms to describe various types of EHRs. These include Electronic Medical Record (EMR), which is similar to an EHR but restricted to the medical domain. The terms Electronic Patient Record (EPR) and Computerized Patient Record (CPR) are also identified. Häyrinen et al. [ 17 ] view both terms as having the same meaning and referring to a system that contains clinical information from a particular hospital. Another term seen is Electronic Healthcare Record (EHCR) which refers to a system that contains all the available health information on a patient [ 17 ] and can thus be seen as synonymous with EHR [ 16 ]. A term often found in the literature is Computerized Physician Order Entry (CPOE). Although this term is not mentioned by ISO [ 16 ] or by Häyrinen et al. [ 17 ], we included CPOE for three reasons. First, it is considered by many to be a key hospital-wide function of an EHR system e.g. [ 8 , 18 ]. Second, from a preliminary analysis of our initial results, we found that, from the perspective of the implementation process, comparable issues and factors emerged from both CPOEs and EHRs. Third, the implementation of a comprehensive electronic medical record requires physicians to make direct order entries [ 19 ]. Kaushal et al. define a CPOE as “ a variety of computer - based systems that share the common features of automating the medication ordering process and that ensure standardized , legible , and complete orders ” [ 18 ], p. 1410. Other terms found in the literature were not included in this review as they were considered either irrelevant or too broadly defined. Examples of such terms are Electronic Client Record (ECR), Personal Health Record (PHR), Digital Medical Record (DMR), Health Information Technology (HIT), and Clinical Information System (CIS).
Search strategies
In order for a systematic literature review to be comprehensive, it is essential that all terms relevant to the aim of the research are covered in the search. Further, we need to include relevant synonyms and related terms, both for electronic medical information systems and for hospitals. By adding an * to the end of a term, the search engines pick out other forms, and by adding “ “ around words one ensures that only the complete term is searched for. Further, by including a ? as a wildcard character, every possible combination is included in the search.
The search used three categories of keywords. The first category included the following terms as approximate synonyms for hospital: “hospital*”, “healthcare”, and “clinic*”. The second category concerned implementation and included the term “implement*”. For the third category, electronic medical information systems, the following search terms were used: “Electronic Health Record*”, “Electronic Patient Record*”, “Electronic Medical Record*”, “Computeri?ed Patient Record*”, “Electronic Healthcare Record*”, “Computeri?ed Physician Order Entry”.
This relatively large set of keywords was necessary to ensure that articles were not missed in the search, and required a large number of search strategies to cover all those keywords. As we were seeking papers about the implementation of electronic medical information systems in hospitals , the search strategies included the terms shown in Table 1 .
The following three search engines were chosen based on their relevance to the field and their accessibility by the researcher: Web of knowledge, EBSCO, and The Cochrane Library. Most search engines use several databases but not all of them were relevant for this research as they serve a wide range of fields. Appendix A provides an overview of the databases used. The reference lists included in articles that met the selection criteria were checked for other possibly relevant studies that had not been identified in the database search.
The articles identified from the various search strategies had to be academic peer-reviewed articles if they were to be included in our review. Further, they were assessed and had to satisfy the following criteria to be included: (1) written in English, (2) full text available online, (3) based on primary empirical data, (4) focused on hospital-wide EHR implementation, and (5) meeting established quality criteria. A long list of abstracts was generated, and all of them were independently reviewed by two of the authors. They independently reviewed the abstracts, eliminated duplicates and shortlisted abstracts for detailed review. When opinions differed, a final decision over inclusion was made following a discussion between the researchers.
Data analysis
The quality of the articles that survived this filtering was assessed by the first two authors using the Standard Quality Assessment Criteria for Evaluating Primary Research Papers [ 18 ]. In other words, the quality of the articles was jointly assessed by evaluating whether specific criteria had been addressed, resulting in a rating of 2 (fully addressed), 1 (partly addressed), or 0 (not addressed) for each criteria. Different questions are posed for qualitative and quantitative research and, in the event of a mixed-method study, both questionnaires were used. Papers were included if they received at least half of the total possible points, admittedly a relatively liberal cut-off point given comments in the Standard Quality Assessment Criteria for Evaluating Primary Research Papers [ 20 ].
The next step was to extract the findings of the reviewed articles and to analyze these with the aim of reaching general findings on the implementation of EHR systems in hospitals. Categorizing these general findings can increase clarity. The earlier introduced conceptual model, based on Pettigrew’s framework for understanding strategic change, includes three categories: context (A), content (B), and process (C). As our review is specifically aimed at identifying findings related to the implementation process, possible motives for introducing such a system, as well as its effects and outcomes, are outside its scope. The authors held frequent discussions between themselves to discuss the meaning and the categorization of the general findings.
Paper selection
Applying the 18 search strategies listed in Table 1 with the various search engines resulted in 364 articles being identified. The searches were carried out on 12 March 2013 for search strategies 1–15 and on 18 April 2013 for search strategies 16–18. The latter three strategies were added following a preliminary analysis of the first set of results which highlighted several other terms and descriptions for information technology in health care. Not surprisingly, many duplicates were included in the 364 articles, both within and between search engines. Using the Refworks functions for identifying exact and close duplicates, 160 duplicates were found. However, this procedure did not identify all the duplicates present and the second author carried out a manual check that identified an additional 23 duplicates. When removing duplicates, we retained the link to the first search engine that identified the article and, as the Web of Knowledge was the first search engine used, most articles appear to have stemmed from this search engine. This left 181 different articles which were screened on title and abstract to check whether they met the selection criteria. When this was uncertain, the contents of the paper were further investigated. This screening resulted in just 13 articles that met all the selection criteria. We then performed two additional checks for completeness. First, checking the references of these articles identified another nine articles. Second, as suggested by the referees of this paper, we also used the term “introduc*” instead of “implement*”, together with the other two original categories of terms, and the term “provider” instead of “physician”, as part of CPOE. Each of these two searches identified one additional article (see Table 1 ). Of these resulting 24 articles, two proved to be almost identical so one was excluded, resulting in 23 articles for a final quality assessment.The results of the quality assessment can be found in Appendix B. The results show that two articles failed to meet the quality threshold and so 21 articles remained for in-depth analysis. Figure 2 displays the steps taken in this selection procedure.
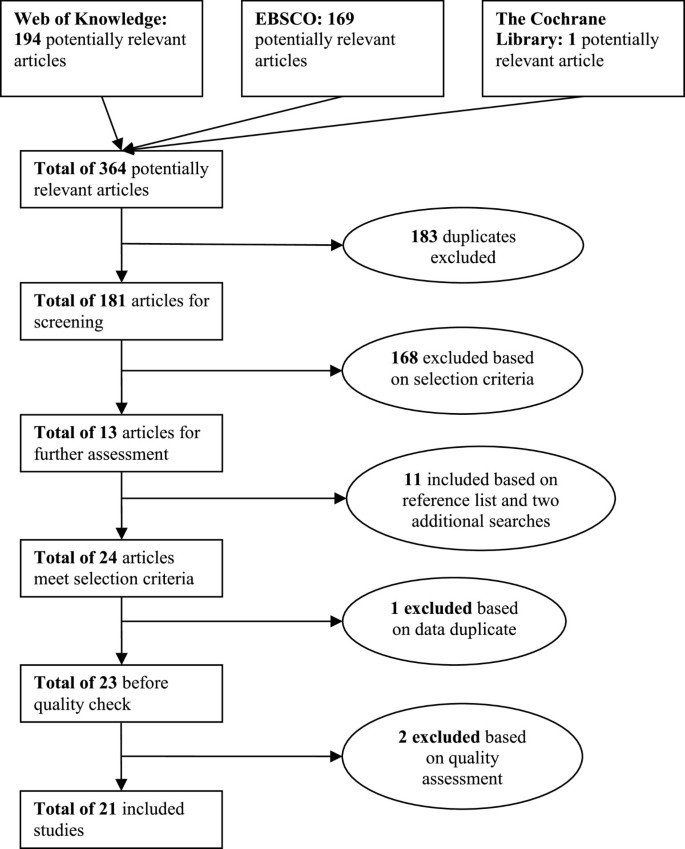
Selection procedure.
To provide greater insight into the context and nature of the 21 remaining articles, an overview is provided in Table 2 . All the studies except one were published after 2000. This reflects the recent increase in effort to implement organization-wide information systems, such as EHR systems, and also increasing incentives from governments to make use of EHR systems in hospitals. Of the 21 studies, 14 can be classified as qualitative, 6 as quantitative, and 1 as a mixed-method study. Most studies were conducted in the USA, with eight in various European countries. Teaching and non-teaching hospitals are almost equally the subject of inquiry, and some researchers have focused on specific types of hospitals such as rural, critical access, or psychiatric hospitals. Ten of the articles were in journals with a five-year impact factor in the Journal Citation Reports 2011 database. There is a huge difference in the number of citations but one should never forget that newer studies have had fewer opportunities to be cited.
Theoretical perspectives of reviewed articles
In research, it is common to use theoretical frameworks when designing an academic study [ 41 ]. Theoretical frameworks provide a way of thinking about and looking at the subject matter and describe the underlying assumptions about the nature of the subject matter [ 42 ]. By building on existing theories, research becomes focused in aiming to enrich and extend the existing knowledge in that particular field [ 42 ]. To provide a more thorough understanding of the selected articles, their theoretical frameworks, if present, are outlined in Table 3 .
It is striking that no specific theoretical frameworks have been used in the research leading to 13 of the 21 selected articles. Most articles simply state their objective as gaining insight into certain aspects of EHR implementation (as shown in Table 1 ) and do not use a particular theoretical approach to identify and categorize findings. As such, these articles add knowledge to the field of EHR implementation but do not attempt to extend existing theories.
Aarts et al. [ 21 ] introduce the notion of the sociotechnical approach: emphasizing the importance of focusing both on the social aspects of an EHR implementation and on the technical aspects of the system. Using the concept of emergent change, they argue that an implementation process is far from linear and predictable due to the contingencies and the organizational complexity that influences the process. A sociotechnical approach and the concept of emergent change are also included in the theoretical framework of Takian et al. [ 37 ]. Aarts et al. [ 21 ] elaborate on the sociotechnical approach when stating that the fit between work processes and the information technology determines the success of the implementation. Aarts and Berg [ 22 ] introduce a model of success or failure in information system implementation. They see creating synergy among the medical work practices, the information system, and the hospital organization as necessary for implementation, and argue that this will only happen if sufficient people accept a change in work practices. Cresswell et al.’s study [ 26 ] is also influenced by sociotechnical principles and draws on Actor-Network Theory. Gastaldi et al. [ 28 ] perceive Electronic Health Records as knowledge management systems and question how such systems can be used to develop knowledge assets. Katsma et al. [ 31 ] focus on implementation success and elaborate on the notion that implementation success is determined by system quality and acceptance through participation. As such, they adopt more of a social view on implementation success rather than a sociotechnical approach. Rivard et al. [ 34 ] examine the difficulties in EHR implementation from a cultural perspective. They not only view culture as a set of assumptions shared by an entire collective (an integration perspective) but also expect subcultures to exist (a differentiation perspective), as well as individual assumptions not shared by a specific (sub-) group (fragmentation perspective). Ford et al. [ 27 ] focus on an entirely different topic and investigate the IT adoption strategies of hospitals using a framework that identifies three strategies. These are the single-vendor strategy (in which all IT is purchased from a single vendor), the best-of-breed strategy (integrating IT from multiple vendors), and the best-of-suit strategy (a hybrid approach using a focal system from one vendor as the basis plus other applications from other vendors).
To summarize, the articles by Aarts et al. [ 21 ], Aarts and Berg [ 22 ], Cresswell et al. [ 26 ], and Takian et al. [ 37 ] apply a sociotechnical framework to focus their research. Gastaldi et al. [ 28 ] see EHRs as a means to renew organizational capabilities. Katsma et al. [ 31 ] use a social framework by focusing on the relevance of an IT system as perceived by the user and the participation of users in the implementation process. Rivard et al. [ 34 ] analyze how organizational cultures can be receptive to EHR implementation. Ford et al. [ 27 ] look at adoption strategies, leading them to focus on the selection procedure for Electronic Health Records. The 13 other studies did not use an explicit theoretical lens in their research.
Implementation-related findings
The process of categorization started by assessing whether a specific finding from a study should be placed in Category A, B, or C. Thirty findings were placed in Category A (context), 31 in Category B (content), and 66 in Category C (process). Comparing and combining the specific findings resulted in several general findings within each category. The general findings are each given a code (category character plus number) and the related code is indicated alongside each specific finding in Appendix C. Findings that were only seen in one article, and thus were lacking support, were discarded.
Category A - context
The context category of an EHR implementation process includes both internal variables (such as resources, capabilities, culture, and politics) and external variables (such as economic, political, and social variables). Six general findings were identified, all but one related to internal variables. An overview of the findings and corresponding articles can be found in Table 4 . The lack of general findings related to external variables reflects our decision to exclude the underlying reasons (e.g. political or social pressures) for implementing an EHR system from this review. Similarly, internal findings related to aspects such as perceived financial benefits or improved quality of care, are outside our scope.
A1: Large (or system-affiliated), urban, not-for-profit, and teaching hospitals are more likely to have implemented an EHR system due to having greater financial capabilities, a greater change readiness, and less focus on profit
The research reviewed shows that larger or system-affiliated hospitals are more likely to have implemented an EHR system, and that this can be explained by their easier access to the large financial resources required. Larger hospitals have more financial resources than smaller hospitals [ 30 ] and system-affiliated hospitals can share costs [ 27 ]. Hospitals situated in urban areas more often have an EHR system than rural hospitals, which is attributed to less knowledge of EHR systems and less support from medical staff in rural hospitals [ 29 ]. The fact that not-for-profit hospitals more often have an EHR system fully implemented and teaching hospitals slightly more often than private hospitals is attributed to the latter’s more wait-and-see approach and the more progressive change-ready nature of public and teaching hospitals [ 27 , 32 ].
A2: EHR implementation requires the selection of a mature vendor who is committed to providing a system that fits the hospital’s specific needs
Although this finding is not a great surprise, it is relevant to discuss it further. A hospital selecting its own vendor can ensure that the system will match the specific needs of that hospital [ 32 ]. Further, it is important to deal with a vendor that has proven itself on the EHR market with mature and successful products. The vendor must also be able to identify hospital workflows and adapt its product accordingly, and be committed to a long-term trusting relationship with the hospital [ 33 ]. With this in mind, the initial price of the system should not be the overriding consideration: the organization should be willing to avoid purely cost-oriented vendors [ 28 ], as costs soon mount if problems arise.
A3: The presence of hospital staff with previous experience of health information technology increases the likelihood of EHR implementation as less uncertainty is experienced by the end-users
In order to be able to work with an EHR system, users must be capable of using information technology such as computers and have adequate typing skills [ 19 , 32 ]. Knowledge of, and previous experience with, EHR systems or other medical information systems reduces uncertainty and disturbance for users, and this results in a more positive attitude towards the system [ 29 , 32 , 37 , 38 ].
A4: An organizational culture that supports collaboration and teamwork fosters EHR implementation success because trust between employees is higher
The influence of organizational culture on the success of organizational change is addressed in almost all the popular approaches to change management, as well as in several of the articles in this literature review. Ash et al. [ 23 , 24 ] and Scott et al. [ 35 ] highlight that a strong culture with a history of collaboration, teamwork, and trust between different stakeholder groups minimizes resistance to change. Boyer et al. [ 25 ] suggest creating a favorable culture that is more adaptive to EHR implementation. However, creating a favorable culture is not necessarily easy: a comprehensive approach including incentives, resource allocation, and a responsible team was used in the example of Boyer et al. [ 25 ].
A5: EHR implementation is most likely in an organization with little bureaucracy and considerable flexibility as changes can be rapidly made
A highly bureaucratic organizational structure hampers change: it slows the process and often leads to inter-departmental conflict [ 19 ]. Specifically, appointing a multidisciplinary team to deal with EHR-related issues can prevent conflict and stimulate collaboration [ 25 ].
A6: EHR system implementation is difficult because cure and care activities must be ensured at all times
During the process of implementing an EHR system, it is of the utmost importance that all relevant information is always available [ 28 , 34 , 39 ]. Ensuring the continuity of quality care while implementing an EHR system is difficult and is an important distinction from many other IT implementations.
Category B - content
The content of the EHR implementation process consists of the EHR system and the corresponding objectives, assumptions, and complementary services. Table 5 lists the five extracted general findings. These focus on both the hardware and software of the EHR system, and its relation to work practices and privacy.
B1: Creating a fit by adapting both the technology and work practices is a key factor in the implementation of EHR
This finding elaborates on the sociotechnical approach identified in the earlier section on the theories adopted in the articles. Several authors [ 21 , 26 , 31 , 37 ] make clear that creating a fit between the EHR system and the existing work practices requires an initial acknowledgement that an EHR implementation is not just a technical project and that existing work practices will change due to the new system. By customizing and adapting the system to meet specific needs, users will become more open to using it [ 19 , 26 , 28 ].
B2: Hardware availability and system reliability, in terms of speed, availability, and a lack of failures, are necessary to ensure EHR use
In several articles, authors highlight the importance of having sufficient hardware. A system can only be used if it is available to the users, and a system will only be used if it works without problems. Ash et al. [ 24 ], Scott et al. [ 35 ], and Weir et al. [ 19 ] refer to the speed of the system as well as to the availability of a sufficient number of adequate terminals see also [ 40 ] in various locations. Systems must be logically structured [ 29 ], reliable [ 32 ], and provide safe information access [ 37 ]. Boyer et al. [ 25 ] also mention the importance of technical aspects but add that these are not sufficient for EHR implementation.
B3: To ensure EHR implementation, the software needs to be user-friendly with regard to ease of use, efficiency in use, and functionality
Some authors distinguish between technical availability and reliability, and the user-friendliness of the software [ 19 , 24 , 32 ]. They argue that it is not sufficient for a system to be available and reliable, it should also be easy and efficient in use, and provide the functionality required for medical staff to give good care. If a system fails to do this, staff will not use the system and will stick to their old ways of working.
B4: An EHR implementation should contain adequate safeguards for patient privacy and confidentiality
Concerns over privacy and confidentiality are recognized by Boyer et al. [ 25 ] and Houser and Johnson [ 29 ] and are considered as a barrier to EHR implementation. Yoon-Flannery et al. [ 40 ] and Takian et al. [ 37 ] also recognize the importance of patient privacy and the need to address this issue by providing training and creating adequate safeguards.
B5: EHR implementation requires a vendor who is willing to adapt its product to hospital work processes
A vendor must be responsive and enable the hospital to develop its product to ensure a good and usable EHR system [ 32 , 33 ]. By so doing, dependence on the vendor decreases and concerns that arise within the hospital can be addressed [ 32 ]. This finding is related to A2 in the sense that an experienced, cooperative, and flexible vendor is needed to deal with the range of interest groups found in hospitals.
Category C - process
This category refers to the actual process of implementing the EHR system. Variables considered are time, change approach, and change management. In our review, this category produced the largest number of general findings (see Table 6 ), as might be expected given our focus on the implementation process. EHR implementation often leads to anxiety, uncertainty, and concerns about a possible negative impact of the EHR on work processes and quality. The process findings, including leadership, resource availability, communication and participation are explicitly aimed at overcoming resistance to EHR implementation. These interventions help to create a positive atmosphere of goal directedness, co-creation and partnership.
C1: Due to their influential position, management’s active involvement and support is positively associated with EHR implementation, and also counterbalances the physicians’ medical dominance
Several authors note the important role that managers play in EHR implementation. Whereas some authors refer to supportive leadership [ 19 , 24 ], others emphasize that strong and active management involvement is needed [ 25 , 32 – 35 ]. Strong leadership is relevant as it effectively counterbalances the physicians’ medical dominance. For instance, Rivard et al. [ 34 ] observe that physicians’ medical dominance and the status and autonomy of other health professionals hinder collaboration and teamwork, and that this complicates EHR implementation. Poon et al. [ 33 ] acknowledge this aspect and argue for strong leadership in order to deal with the otherwise dominant physicians. They also claim that leaders have to set an example and use the system themselves. At the same time, it is motivating that the implementation is managed by leaders who are recognized by the medical staff, for instance by head nurses and physicians or by former physicians and nurses [ 25 , 33 ]. Ovretveit et al. [ 32 ] argue that it helps the implementation if senior management repeatedly declares the EHR implementation to be of the highest priority and supports this with sufficient financial and human resources. Poon et al. [ 33 ] add to this by highlighting that, especially during uncertainties and setbacks, the common vision that guides the EHR implementation has to be communicated to hospital staff. Sufficient human resources include the selection of competent and experienced project leaders who are familiar with EHR implementation. Scott et al. [ 35 ] identify leadership styles for different phases: participatory leadership is valued in selection decisions, whereas a more hierarchical leadership style is preferable in the actual implementation.
C2: Participation of clinical staff in the implementation process increases support for and acceptance of the EHR implementation
Participation of end-users (the clinical staff) generates commitment and enables problems to be quickly solved [ 25 , 26 , 36 ]. Especially because it is very unlikely that the system will be perfect for all, it is important that the clinical staff become the owner, rather than customers, of the system. Clinical staff should participate at all levels and in all steps [ 19 , 28 , 32 , 36 ] from initial system selection onwards [ 35 ]. Ovretveit et al. [ 32 ] propose that this involvement should have an extensive timeframe, starting in the early stages of implementation, when initial vendor requirements are formulated (‘consultation before implementation’), through to the beginning of the use phase. Creating multidisciplinary work groups which determine the content of the EHR and the rules regarding the sharing of information contributes to EHR acceptance [ 25 ] and ensures realistic approaches acceptable to the clinical staff [ 36 ].
C3: Training end-users and providing real-time support is important for EHR implementation success
Frequently, the end-users of a new EHR system lack experience with the specific EHR system or with EHR systems in general. Although it is increasingly hard to imagine society or workplaces without IT, a large specific system, such as an EHR, still requires considerable training on how to use it properly. The importance of training is often underestimated, and inadequate training will create a barrier to EHR use [ 19 , 29 ]. Consequently, adequate training, of appropriate quantity and quality, must be provided at the right times and locations [ 19 , 32 , 36 ]. Simon et al. [ 36 ] add to this the importance of real-time support, preferably provided by peers and super-users.
C4: A comprehensive implementation strategy, offering both clear guidance and room for emergent change, is needed for implementing an EHR system
Several articles highlight aspects of an EHR implementation strategy. A good strategy facilitates EHR implementation [ 19 , 25 ] and consists of careful planning and preparation [ 36 ], a sustainable business plan, effective communication [ 28 , 40 ] and mandatory implementation [ 19 ]. Emergent change is perceived as a key characteristic of EHR implementation in complex organizations such as hospitals [ 21 ], and this suggests an implementation approach based on a development paradigm [ 31 ], which may initially even involve parallel use of paper [ 26 ]. The notion of emergent change has been variously applied, including in the theoretical frameworks of Aarts et al. [ 21 ] and Katsma et al. [ 31 ]. These studies recognize that EHR implementation is relatively unpredictable due to unforeseen contingencies for which one cannot plan. With their emphasis on emergent change with unpredictable outcomes, Aarts et al. [ 21 ] make a case for acknowledging that unexpected and unplanned contingencies will influence the implementation process. They argue that the changes resulting from these contingencies often manifest themselves unexpectedly and must then be dealt with. Additionally, Takian et al. [ 37 ] state that it is crucial to contextualize an EHR implementation so as to be better prepared for unexpected changes.
C5: Establishing an interdisciplinary implementation group consisting of developers, members of the IT department, and end-users fosters EHR implementation success
In line with the arguments for management support and for the participation of clinical staff, Ovretveit et al. [ 32 ], Simon et al. [ 36 ] and Weir et al. [ 19 ] build a case for using an interdisciplinary implementation group. By having all the direct stakeholders working together, a better EHR system can be delivered faster and with fewer problems.
C6: Resistance of clinical staff, in particular of physicians, is a major barrier to EHR implementation, but can be reduced by addressing their concerns
Clinical staff’s attitude is a crucial factor in EHR implementation [ 36 ]. Particularly, the physicians constitute an important group in hospitals. As such, their possible resistance to EHR implementation will form a major barrier [ 29 , 33 ] and may lead to workarounds [ 26 ]. Whether physicians accept or reject an EHR implementation depends on their acceptance of their work practices being transformed [ 22 ]. The likelihood of acceptance will be increased if implementers address the concerns of physicians [ 24 , 28 , 32 , 33 ], but also of other members of clinical staff [ 36 ].
C7: Identifying champions among clinical staff reduces resistance
The previous finding already elaborated on clinical staff resistance and suggested reducing this by addressing their concerns. Another way to reduce their resistance is related to the process of implementation and involves identifying physician champions, typically physicians that are well respected due to their knowledge and contacts [ 32 , 33 ]. Simon et al. [ 36 ] emphasize the importance of identifying champions among each stakeholder group. These champions can provide reassurance to their peers.
C8: Assigning a sufficient number of staff and other resources to the EHR implementation process is important in adequately implementing the system
Implementing a large EHR system requires considerable resources, including human ones. Assigning appropriate people, such as super-users [ 36 ] and a sufficient number of them to that process will increase the likelihood of success [ 19 , 32 , 33 , 36 ]. Further, it is important to have sufficient time and financial resources [ 26 , 32 ]. This finding is also relevant in relation to finding A6 (ensuring good care during organizational change).
These 19 general findings have been identified from the individual findings within the 20 analyzed articles. These findings are all related to one of the three main and interacting dimensions of the framework: six to context, five to content, and eight to process. This identification and explanation of the general findings concludes the results section of this systematic literature review and forms the basis for the discussion below.
This review of the existing academic literature sheds light on the current knowledge regarding EHR implementation. The 21 selected articles all originate from North America or Europe, perhaps reflecting a greater governmental attention to EHR implementation in these regions and, of course, our inclusion of only articles written in English. Two articles were rejected for quality reasons [ 43 , 44 ], see Appendix B. All but one of the selected articles have been published since 2000, reflecting the growing interest in implementing EHR systems in hospitals. Eight articles built their research on a theoretical framework, four of which use the same general lens of the sociotechnical approach [ 21 , 22 , 26 , 37 ]. Katsma et al. [ 31 ] and Rivard et al. [ 34 ] focus more on the social and cultural aspects of EHR implementation, the former on the relevance for, and participation of, users, the latter on three different cultural perspectives. Ford et al. [ 27 ] researched adoption strategies for EHR systems and Gastaldi et al. [ 26 ] consider them as a means to renew organizational capabilities. It is notable that the other reviewed articles did not use a theoretical framework to analyze EHR implementation and made no attempt to elaborate on existing theories.
A total of 127 findings were extracted from the articles, and these findings were categorized using Pettigrew’s framework for strategic change [ 13 ] as a conceptual model including the three dimensions of context, content, and process. To ensure a tight focus, the scope of the review was explicitly limited to findings related to the EHR implementation process, thus excluding the reasons for, barriers to, and outcomes of an EHR implementation.
Some of the findings require further interpretation. Contextual finding A1 relates to the demographics of a hospital. One of the assertions is that privately owned hospitals are less likely than public hospitals to invest in an EHR. The former apparently perceive the costs of EHR implementation to outweigh the benefits. This seems remarkable given that there is a general belief that information technology increases efficiency and reduces process costs, so more than compensating for the high initial investments. It is however important to note that the literature on EHR is ambivalent when it comes to efficiency; several authors record a decrease in the efficiency of work practices [ 25 , 33 , 35 , 38 ], whereas others mention an increase [ 29 , 31 ]. Finding A2 is a reminder of the importance of carefully selecting an appropriate vendor, taking into account experience with the EHR market and the maturity of their products rather than, for example, focussing on the cost price of the system. Given the huge investment costs, the price of an EHR system tends to have a major influence on vendor selection, an aspect that is also promoted by the current European tendering regulations that oblige (semi-) public institutions, like many hospitals, to select the lowest bidder, or the bidder that is economically the most preferable [ 45 ]. The finding that EHR system implementation is difficult because good medical care needs to be ensured at all times (A6) also deserves mention. Essentially, many system implementations in hospitals are different from IT implementations in other contexts because human lives are at stake in hospitals. This not only complicates the implementation process because medical work practices have to continue, it also requires a system to be reliable from the moment it is launched.
The findings regarding the content of the EHR system (Category B) highlight the importance of a suitable software product. A well-defined selection process of the software package and its associated vendor (discussed in A2) is seen as critical (B5). Selection should be based on a careful requirements analysis and an analysis of the experience and quality of the vendor. An important requirement is a sufficient degree of flexibility to customize and adapt the software to meet the needs of users and the work practices of the hospital (finding B1). At the same time the software product should challenge the hospital to rethink and improve its processes. A crucial condition for the acceptance by the diverse user groups of hospitals is the robustness of the EHR system in terms of availability, speed, reliability and flexibility (B2). This also requires adequate hardware in terms of access to computers, and mobile equipment to enable availability at all the locations of the hospital. Perceived ease of use of the system (B4) and the protection of patients’ privacy (B4) are other content factors that can make or break EHR implementation in hospitals.
The findings on the implementation process, our Category C, highlight four aspects that are commonly mentioned in change management approaches as important success factors in organizational change. The active involvement and support of management (C1), the participation of clinical staff (C2), a comprehensive implementation strategy (C4), and using an interdisciplinary implementation group (C5) correspond with three of the ten guidelines offered by Kanter et al. [ 46 ]. These three guidelines are: (1) support a strong leader role; (2) communicate, involve people, and be honest; and (3) craft an implementation plan. As the implementation of an EHR system is an organizational change process it is no surprise that these commonalities are identified in several of the analyzed articles. Three Category C findings (C2, C6, and C7) concern dealing with clinical staff given their powerful positions and potential resistance. Physicians are the most influential medical care providers, and their resistance can delay an EHR implementation [ 23 ], lead to at least some of it being dropped [ 21 , 22 , 34 ], or to it not being implemented at all [ 33 ]. Thus, there is ample evidence of the crucial importance of physicians’ acceptance of an EHR for it to be implemented. This means that clinicians and other key personnel should be highly engaged and motivated to contribute to EHR. Prompt feedback on requests, and high quality support during the implementation, and an EHR that clearly supports clinical work are key issues that contribute to a motivated clinical staff.
Analyzing and comparing the findings enables us to categorize them in terms of subject matter (see Table 7 ). By categorizing the findings in terms of subject, and by totaling the number of articles related to the individual findings on that subject, one can deduce how much attention has been given in the literature to the different topics. This analysis highlights that the involvement of physicians in the implementation process, the quality of the system, and a comprehensive implementation strategy are considered the crucial elements in EHR implementation.
Notwithstanding the useful results, this review and analysis has some limitations. Although we carefully developed and executed the search strategy, we cannot be sure that we found all the relevant articles. Since we focused narrowly on keywords, and these had to be part of an article’s title, we could have excluded relevant articles that used different terminology in their titles. Although searching the reference lists of identified articles did result in several additional articles, some relevant articles might still have been missed. Another limitation is the exclusion of publications in languages other than English. Further, the selection and categorization of specific findings, and the subsequent extraction of general findings, is subjective and depends on the interpretations of the authors, and other researchers might have made different choices. A final limitation is inherent to literature reviews in that the authors of the studies included may have had different motives and aims, and used different methods and interpretative means, in drawing their conclusions.
The existing literature fails to provide evidence of there being a comprehensive approach to implementing EHR systems in hospitals that integrates relevant aspects into an ‘EHR change approach’. The literature is diffuse, and articles seldom build on earlier ones to increase the theoretical knowledge on EHR implementation, notable exceptions being Aarts et al. [ 21 ], Aarts and Berg [ 22 ], Cresswell et al. [ 26 ], and Takian et al. [ 37 ]. The earlier discussion on the various results summarizes the existing knowledge and reveals gaps in the knowledge associated with EHR implementation. The number of EHR implementations in hospitals is growing, as well as the body of literature on this subject. This systematic review of the literature has produced 19 general findings on EHR implementation, which were each placed in one of three categories. A number of these general findings are in line with the wider literature on change management, and others relate to the specific nature of EHR implementation in hospitals.
The findings presented in this article can be viewed as an overview of important subjects that should be addressed in implementing an EHR system. It is clear that EHR systems have particular complexities and should be implemented with great care, and with attention given to context, content, and process issues and to interactions between these issues. As such, we have achieved our research goal by creating a systematic review of the literature on EHR implementation. This paper’s academic contribution is in providing an overview of the existing literature with regard to important factors in EHR implementation in hospitals. Academics interested in this specific field can now more easily access knowledge on EHR implementation in hospitals and can use this article as a starting point and build on the existing knowledge. The managerial contribution lies in the general findings that can be applied as guidelines when implementing EHR in hospitals. We have not set out to provide a single blueprint for implementing an EHR system, but rather to provide guidelines and to highlight points that deserve attention. Recognizing and addressing these aspects can increase the likelihood of getting an EHR system successfully implemented.
Appendix A - List of databases
This appendix provides an overview of all databases included in the used search engines. The databases in italic were excluded for the research as these databases focus on fields not relevant for the subject of EHR implementations.
Web of Knowledge
Web of Science
Biological Abstracts
Journal Citation Reports
Academic Search Premier
AMED - The Allied and Complementary Medicine Database
America : History & Life
American Bibliography of Slavic and East European Studies
Arctic & Antarctic Regions
Art Full Text ( H.W. Wilson )
Art Index Retrospective ( H.W. Wilson )
ATLA Religion Database with ATLASerials
Business Source Premier
Communication & Mass Media Complete
eBook Collection ( EBSCOhost )
Funk & Wagnalls New World Encyclopedia
Historical Abstracts
L ’ Annéephilologique
Library, Information Science & Technology Abstracts
MAS Ultra - School Edition
Military & Government Collection
MLA Directory of Periodicals
MLA International Bibliography
New Testament Abstracts
Old Testament Abstracts
Philosopher ’ s Index
Primary Search
PsycARTICLES
PsycCRITIQUES
Psychology and Behavioral Sciences Collection
Regional Business News
Research Starters - Business
RILM Abstracts of Music Literature
The Cochrane Library
Cochrane Database of Systematic Reviews
Cochrane Central Register of Controlled Trials
Cochrane Methodology Register
Database of Abstracts of Reviews of Effects
Health Technology Assessment Database
NHS Economic Evaluation Database
About The Cochrane Collaboration
Appendix B - Quality assessment
The quality of the articles was assessed with the Standard Quality Assessment Criteria for Evaluating Primary Research Papers [ 18 ]. Assessment was done by questioning whether particular criteria had been addressed, resulting in a rating of 2 (completely addressed), 1 (partly addressed), or 0 (not addressed) points. Table 8 provides the overview of the scores of the articles, (per question) for qualitative studies; Table 9 for quantitative studies; and Table 10 for mixed methods studies. Articles were included if they scored 50% or higher of the total amount of points possible. Based on this assessment, two articles were excluded from the search.
Appendix C - All findings
Table 11 displays all findings from the selected articles. The category number is related to the general finding as discussed in the Results section.
Abramson EL, McGinnis S, Edwards A, Maniccia DM, Moore J, Kaushal R: Electronic health record adoption and health information exchange among hospitals in New York State. J Eval Clin Pract. 2011, 18: 1156-1162.
Article PubMed Google Scholar
Robertson A, Cresswell K, Takian A, Petrakaki D, Crowe S, Cornford T, Sheikh A: Implementation and adoption of nationwide electronic health records in secondary care in England: qualitative analysis of interim results from a prospective national evaluation. Br Med J. 2010, 341: c4564-10.1136/bmj.c4564.
Article Google Scholar
Rigsrevisionen: Extract from the report to the Public Accounts Committee on the implementation of electronic patient records at Danish hospitals. 2011, http://uk.rigsrevisionen.dk/media/1886186/4-2010.pdf , 2011
Google Scholar
Hartswood M, Procter R, Rouncefield M, Slack R: Making a Case in Medical Work: Implications for the Electronic Medical Record. Comput Supported Coop Work. 2003, 12: 241-266. 10.1023/A:1025055829026.
Grimson J, Grimson W, Hasselbring W: The SI Challenge in Health Care. Commun ACM. 2000, 43 (6): 49-55.
Mantzana V, Themistocleous M, Irani Z, Morabito V: Identifying healthcare actors involved in theadoption of information systems. Eur J Inf Syst. 2007, 16: 91-102. 10.1057/palgrave.ejis.3000660.
Boonstra A, Boddy D, Bell S: Stakeholder management in IOS projects: analysis of an attempt to implement an electronic patient file. Eur J Inf Syst. 2008, 17 (2): 100-111. 10.1057/ejis.2008.2.
Jha A, DesRoches CM, Campbell EG, Donelan K, Rao SR, Ferris TF, Shields A, Rosenbaum S, Blumenthal D: Use of Electronic Health Records in US hospitals. N Engl J Med. 2009, 360: 1628-1638. 10.1056/NEJMsa0900592.
Article CAS PubMed Google Scholar
Heeks R: Health information systems: Failure, success and improvisation. Int J Med Inform. 2006, 75: 125-137. 10.1016/j.ijmedinf.2005.07.024.
Boonstra A, Govers MJ: Understanding ERP system implementation in a hospital by analysing stakeholders. N Technol Work Employ. 2009, 24 (2): 177-193. 10.1111/j.1468-005X.2009.00227.x.
Keshavjee K, Bosomworth J, Copen J, Lai J, Kucukyazici B, Liani R, Holbrook AM: Best practices in EMR implementation: a systematic review. Proceed of the 11th International Symposium on Health Information Mangement Research – iSHIMR. 2006, 1-15.
McGinn CA, Grenier S, Duplantie J, Shaw N, Sicotte C, Mathieu L, Leduc Y, Legare F, Gagnon MP: Comparison of use groups perspectives of barriers and facilitator to implementing EHR – a systematic review. BMC Med. 2011, 9: 46-10.1186/1741-7015-9-46.
Article PubMed PubMed Central Google Scholar
Pettigrew AM: Context and action in the transformation of the firm. J Manag Stud. 1987, 24 (6): 649-670. 10.1111/j.1467-6486.1987.tb00467.x.
Hartley J: Case Study Research. Chapter 26. Essential Guide to Qualitative Methods in Organizational Research. Edited by: Cassel C, Symon G. 2004, London: Sage
Hage E, Roo JP, Offenbeek MAG, Boonstra A: Implementation factors and their effect on e-health service adoption in rural communities: a systematic literature review. BMC Health Serv Res. 2013, 13 (19): 1-16.
ISO: Health informatics: Electronic health record - Definition, scope and context. Draft Tech Report. 2004, 03-16. ISO/DTR 20514. available at https://www.iso.org/obp/ui/#iso:std:iso:tr:20514:ed-1:v1:en
Häyrinen K, Saranto K, Nykänen P: Definition, structure, content, use and impacts of electronic health records: A review of the research literature. Int J Med Inform. 2008, 77: 291-304. 10.1016/j.ijmedinf.2007.09.001.
Kaushal R, Shojania KG, Bates DW: Effects of computerized physician order entry and clinical decision support systems on medication safety: a systematic review. Arch Intern Med. 2003, 163 (12): 1409-1416. 10.1001/archinte.163.12.1409.
Weir C, Lincoln M, Roscoe D, Turner C, Moreshead G: Dimensions associated with successful implementation of a hospital based integrated order entry system. Proc Annu Symp Comput Appl [Sic] in Med Care Symp Comput Appl Med Care. 1994, 653: 7.
Kmet LM, Lee RC, Cook LS: Standard quality assessment criteria for evaluating primary research papers from a variety of fields. 2004, Alberta Heritage Foundation for Medical Research, http://www.ihe.ca/documents/HTA-FR13.pdf .
Aarts J, Doorewaard H, Berg M: Understanding implementation: The case of a computerized physician order entry system in a large dutch university medical center. J Am Med Inform Assoc. 2004, 11 (3): 207-216. 10.1197/jamia.M1372.
Aarts J, Berg M: Same systems, different outcomes - Comparing the implementation of computerized physician order entry in two Dutch hospitals. Methods Inf Med. 2006, 45 (1): 53-61.
CAS PubMed Google Scholar
Ash J, Gorman P, Lavelle M, Lyman J, Fournier L: Investigating physician order entry in the field: lessons learned in a multi-center study. Stud Health Technol Inform. 2001, 84 (2): 1107-1111.
Ash JS, Gorman PN, Lavelle M, Payne TH, Massaro TA, Frantz GL, Lyman JA: A cross-site qualitative study of physician order entry. J Am Med Inform Assoc. 2003, 10 (2): 188-200. 10.1197/jamia.M770.
Boyer L, Samuelian J, Fieschi M, Lancon C: Implementing electronic medical records in a psychiatric hospital: A qualitative study. Int J Psychiatry Clin Pract. 2010, 14 (3): 223-227. 10.3109/13651501003717243.
Cresswell KM, Worth A, Sheikh A: Integration of a nationally procured electronic health record system into user work practices. BMC Med Inform Decis Mak. 2012, 12: 15-10.1186/1472-6947-12-15.
Ford EW, Menachemi N, Huerta TR, Yu F: Hospital IT Adoption Strategies Associated with Implementation Success: Implications for Achieving Meaningful Use. J Healthc Manag. 2010, 55 (3): 175-188.
PubMed Google Scholar
Gastaldi L, Lettieri E, Corso M, Masella C: Performance improvement in hospitals: leveraging on knowledge assets dynamics through the introduction of an electronic medical record. Meas Bus Excell. 2012, 16 (4): 14-30. 10.1108/13683041211276410.
Houser SH, Johnson LA: Perceptions regarding electronic health record implementation among health information management professionals in Alabama: a statewide survey and analysis. Perspect Health Inf Manage/AHIMA, Am Health Inf Manage Assoc. 2008, 5: 6-6.
Jaana M, Ward MM, Bahensky JA: EMRs and Clinical IS Implementation in Hospitals: A Statewide Survey. J Rural Health. 2012, 28: 34-43. 10.1111/j.1748-0361.2011.00386.x.
Katsma CP, Spil TAM, Ligt E, Wassenaar A: Implementation and use of an electronic health record: measuring relevance and participation in four hospitals. Int J Healthc Technol Manag. 2007, 8 (6): 625-643. 10.1504/IJHTM.2007.014194.
Ovretveit J, Scott T, Rundall TG, Shortell SM, Brommels M: Improving quality through effective implementation of information technology in healthcare. Int J Qual Health Care. 2007, 19 (5): 259-266. 10.1093/intqhc/mzm031.
Poon EG, Blumenthal D, Jaggi T, Honour MM, Bates DW, Kaushal R: Overcoming barriers to adopting and implementing computerized physician order entry systems in US hospitals. Health Aff. 2004, 23 (4): 184-190. 10.1377/hlthaff.23.4.184.
Rivard S, Lapointe L, Kappos A: An Organizational Culture-Based Theory of Clinical Information Systems Implementation in Hospitals. J Assoc Inf Syst. 2011, 12 (2): 123-162.
Scott JT, Rundall TG, Vogt TM, Hsu J: Kaiser Permanente’s experience of implementing an electronic medical record: a qualitative study. Br Med J. 2005, 331 (7528): 1313-1316. 10.1136/bmj.38638.497477.68.
Simon SR, Keohane CA, Amato M, Coffey M, Cadet M, Zimlichman E: Lessons learned from implementation of computerized provider order entry in 5 community hospitals: a qualitative study. BMC Med Inform Decis Mak. 2013, 13: 67-10.1186/1472-6947-13-67.
Takian A, Sheikh A, Barber N: We are bitter, but we are better off: case study of the implementation of an electronic health record system into a mental health hospital in England. BMC Health Serv Res. 2012, 12: 484-10.1186/1472-6963-12-484.
Ward MM, Vartak S, Schwichtenberg T, Wakefield DS: Nurses’ Perceptions of How Clinical Information System Implementation Affects Workflow and Patient Care. Cin-Comput Inform Nurs. 2011, 29 (9): 502-511. 10.1097/NCN.0b013e31822b8798.
Ward MM, Vartak S, Loes JL, O’Brien J, Mills TR, Halbesleben JRB, Wakefield DS: CAH Staff Perceptions of a Clinical Information System Implementation. Am J Manage Care. 2012, 18 (5): 244-252.
Yoon-Flannery K, Zandieh SO, Kuperman GJ, Langsam DJ, Hyman D, Kaushal R: A qualitative analysis of an electronic health record (EHR) implementation in an academic ambulatory setting. Inform Prim Care. 2008, 16 (4): 277-284.
Van Aken J, Berends H, Van der Bij H: Problem solving in organizations. 2012, New York, USA: Cambridge University Press
Book Google Scholar
Botha ME: Theory development in perspective: the role of conceptual frameworks and models in theory development. J Adv Nurs. 1989, 14 (1): 49-55. 10.1111/j.1365-2648.1989.tb03404.x.
Spetz J, Keane D: Information Technology Implementation in a Rural Hospital: A Cautionary Tale. J Healthc Manag. 2009, 54 (5): 337-347.
Massaro TA: Introducing Physician Order Entry at a Major Academic Medical-Center. Impact Organ Culture Behav Acad Med. 1993, 68 (1): 20-25.
CAS Google Scholar
Lundberg S, Bergman M: Tender evaluation and supplier selection methods in public procurement. J Purch Supply Manage. 2013, 19 (2): 73-83. 10.1016/j.pursup.2013.02.003.
Kanter RM, Stein BA, Jick TD: The Challenge of Organizational Change. 1992, New York, USA: Free Press
Pre-publication history
The pre-publication history for this paper can be accessed here: http://www.biomedcentral.com/1472-6963/14/370/prepub
Download references
Acknowledgement
We acknowledge the Master degree program Change Management at the University of Groningen for supporting this study. We also thank the referees for their valuable comments.
Author information
Authors and affiliations.
Faculty of Economics and Business, University of Groningen, Groningen, The Netherlands
Albert Boonstra & Janita F J Vos
Deloitte Consulting, Amsterdam, The Netherlands
Arie Versluis
You can also search for this author in PubMed Google Scholar
Corresponding author
Correspondence to Albert Boonstra .
Additional information
Competing interests.
The authors declare that they have no competing interests.
Authors’ contributions
AB and JV established the research design and made significant contributions to the interpretation of the results. They supervised AV throughout the study, and participated in writing the final version of this paper. AV contributed substantially to the selection and analysis of included papers, and wrote a preliminary draft of this article. All authors have read and approved the final manuscript.
Authors’ original submitted files for images
Below are the links to the authors’ original submitted files for images.
Authors’ original file for figure 1
Authors’ original file for figure 2, rights and permissions.
This article is published under license to BioMed Central Ltd. This is an Open Access article distributed under the terms of the Creative Commons Attribution License ( http://creativecommons.org/licenses/by/2.0 ), which permits unrestricted use, distribution, and reproduction in any medium, provided the original work is properly credited. The Creative Commons Public Domain Dedication waiver ( http://creativecommons.org/publicdomain/zero/1.0/ ) applies to the data made available in this article, unless otherwise stated.
Reprints and permissions
About this article
Cite this article.
Boonstra, A., Versluis, A. & Vos, J.F.J. Implementing electronic health records in hospitals: a systematic literature review. BMC Health Serv Res 14 , 370 (2014). https://doi.org/10.1186/1472-6963-14-370
Download citation
Received : 23 September 2013
Accepted : 11 August 2014
Published : 04 September 2014
DOI : https://doi.org/10.1186/1472-6963-14-370
Share this article
Anyone you share the following link with will be able to read this content:
Sorry, a shareable link is not currently available for this article.
Provided by the Springer Nature SharedIt content-sharing initiative
- Clinical Staff
- Electronic Health Record
- Electronic Patient Record
- Health Information Technology
- Computerize Physician Order Entry
BMC Health Services Research
ISSN: 1472-6963
- General enquiries: [email protected]
- Search Menu
- Advance articles
- Editor's Choice
- Focus Issue Archive
- Open Access Articles
- Author Guidelines
- Submission Site
- Open Access
- Call for Papers
- About Journal of the American Medical Informatics Association
- About the American Medical Informatics Association
- Journals Career Network
- Editorial Board
- Advertising and Corporate Services
- Self-Archiving Policy
- Dispatch Dates
- For Reviewers
- Journals on Oxford Academic
- Books on Oxford Academic
Article Contents
Introduction, author contributions, supplementary material, conflicts of interest, data availability, large language models facilitate the generation of electronic health record phenotyping algorithms.

- Article contents
- Figures & tables
- Supplementary Data
Chao Yan, Henry H Ong, Monika E Grabowska, Matthew S Krantz, Wu-Chen Su, Alyson L Dickson, Josh F Peterson, QiPing Feng, Dan M Roden, C Michael Stein, V Eric Kerchberger, Bradley A Malin, Wei-Qi Wei, Large language models facilitate the generation of electronic health record phenotyping algorithms, Journal of the American Medical Informatics Association , 2024;, ocae072, https://doi.org/10.1093/jamia/ocae072
- Permissions Icon Permissions
Phenotyping is a core task in observational health research utilizing electronic health records (EHRs). Developing an accurate algorithm demands substantial input from domain experts, involving extensive literature review and evidence synthesis. This burdensome process limits scalability and delays knowledge discovery. We investigate the potential for leveraging large language models (LLMs) to enhance the efficiency of EHR phenotyping by generating high-quality algorithm drafts.
We prompted four LLMs—GPT-4 and GPT-3.5 of ChatGPT, Claude 2, and Bard—in October 2023, asking them to generate executable phenotyping algorithms in the form of SQL queries adhering to a common data model (CDM) for three phenotypes (ie, type 2 diabetes mellitus, dementia, and hypothyroidism). Three phenotyping experts evaluated the returned algorithms across several critical metrics. We further implemented the top-rated algorithms and compared them against clinician-validated phenotyping algorithms from the Electronic Medical Records and Genomics (eMERGE) network.
GPT-4 and GPT-3.5 exhibited significantly higher overall expert evaluation scores in instruction following, algorithmic logic, and SQL executability, when compared to Claude 2 and Bard. Although GPT-4 and GPT-3.5 effectively identified relevant clinical concepts, they exhibited immature capability in organizing phenotyping criteria with the proper logic, leading to phenotyping algorithms that were either excessively restrictive (with low recall) or overly broad (with low positive predictive values).
GPT versions 3.5 and 4 are capable of drafting phenotyping algorithms by identifying relevant clinical criteria aligned with a CDM. However, expertise in informatics and clinical experience is still required to assess and further refine generated algorithms.
Electronic health record (EHR) phenotyping, which involves creating algorithms to identify and correctly classify a patient’s observable characteristics by integrating complex clinical data, has become pivotal in observational health research. 1 Developing EHR phenotypes is an intricate and labor-intensive process that demands extensive expertise in both the clinical and informatics domains. 2 , 3 While phenotyping includes the identification of individuals with specific characteristics, it also necessitates the selection of suitable controls for meaningful comparisons with the identified cases. 4
Rule-based computable phenotyping algorithms rely on clinical experts to select specialized criteria (eg, diagnosis codes, medications, and laboratory values) likely to define a phenotype of interest. When subjected to detailed refinement and thorough validation, these algorithms often exhibit enhanced performance compared to high-throughput methods, which typically employ machine learning or data mining-based approaches to provide automated and rapid categorization of numerous disease phenotypes. 5–8 However, the iterative nature of this process often requires substantial literature review and discussions with clinical experts to generate a single phenotyping algorithm, thereby limiting the scalability of this approach in practice. 2 Furthermore, implementation of phenotyping algorithms by secondary sites requires additional informatics expertise, manual effort, and time to adapt the existing code to different databases and EHR systems.
Recently, large language models (LLMs) have demonstrated effectiveness in information extraction and summarization, 9 indicating a potential benefit in phenotyping by reducing the time required for literature review and synthesis during the phenotype generation process. Previous studies investigating the application of LLMs to phenotyping tasks have primarily evaluated the ability of LLMs to extract phenotypic information from unstructured clinical notes. 10 For example, Alsentzer et al. 11 found that the open-source LLM Flan-T5 could effectively extract concepts from discharge summaries to create a postpartum hemorrhage phenotype. In this preliminary report, we investigate the novel application of LLMs for generating computable phenotyping algorithms to assess whether such tools can effectively expedite the development of EHR phenotypes based on structured data. We appraised four LLMs—GPT-4 (powering ChatGPT), 12 GPT-3.5 (powering ChatGPT), 13 Claude 2, 14 and PaLM 2-powered Bard 15 —to generate phenotyping algorithms for three clinical phenotypes—type 2 diabetes mellitus (T2DM), dementia, and hypothyroidism. We subsequently implemented the top-rated algorithms as adjudicated by phenotyping experts using multiple critical metrics from each LLM and compared them against the clinician-validated phenotyping algorithms from the Electronic Medical Records and Genomics (eMERGE) network. 16 , 17
We selected four LLMs in their default configurations to test their phenotyping algorithm generation capacity for three common clinical phenotypes. These LLMs were: (1) GPT-4 and (2) GPT-3.5 (both powering ChatGPT by OpenAI), 12 , 13 (3) Claude 2 (developed by Anthropic), 14 and (4) Bard (created by Google and based on PaLM 2). 15 These models were chosen because of their widespread use, easy accessibility, extensive evaluation, robust computational capabilities, and proficiency in handling and generating lengthy texts—qualities crucial for sustainably supporting phenotyping tasks.
This pilot study specifically focused on three clinical phenotypes: T2DM, 18 , 19 dementia, 20 and hypothyroidism. 21 , 22 We chose these phenotypes because they have existing algorithms that have undergone extensive validation processes and demonstrated highly accurate and consistent performances with well-documented results. Data collection via web-based interactions with the LLMs occurred in October 2023, with subsequent data analysis completed in November 2023. This study was approved by the institutional review boards at Vanderbilt University Medical Center (IRB #: 201434).
Figure 1 illustrates an overview of the study pipeline, which was comprised of two main components—prompting (steps 1-3, highlighted in pink) and evaluating (steps 4-9, highlighted in blue) LLMs.

An architectural overview of the study pipeline.
Prompting large language models to generate phenotyping algorithms
We prompted the LLMs to generate executable SQL queries to identify phenotype cases from structured EHR data organized according to the Observational Medical Outcomes Partnership (OMOP) Common Data Model (CDM), a standard EHR data framework that enables efficient data analysis and sharing across institutions. 23 , 24 There are multiple methods to search for concepts in an OMOP database, including specifying “concept_code”, “concept_name”, or “concept_id”. In this study, we focused on using International Classification of Diseases (ICD) codes as concept codes for diagnosis concepts and using concept names for non-diagnosis concepts. This design was based on our observations that LLMs were (1) able to generate ICD diagnosis codes that are relevant to the target phenotype; (2) less likely to identify meaningful non-diagnosis concepts using “concept_code” compared to using “concept_name”; and (3) unable to generate applicable“concept_id” in general. The variability in the capacity of LLMs to identify relevant concepts through different methods can be attributed to discrepancies in the amount of information they have encountered about these methods to during pretraining.
We designed two distinct prompting strategies, hereafter termed α-prompting and β-prompting. The α-prompting strategy (steps 1 and 2 in Figure 1 ) had two steps. The first step focused on obtaining a pseudocode version of the phenotyping algorithm (referred to as a pseudo-phenotyping algorithm), which emphasized identifying and integrating critical phenotyping criteria, as well as determining the strategy for combining these criteria. We utilized the chain-of-thought (CoT) prompting strategy, 25 an effective method for directing LLMs through a series of reasoning steps to resolve complex problems like humans. Specifically, we framed our instruction to guide reasoning as follows: “Let’s think step by step: (1) List the critical criteria to consider. (2) Determine how these criteria should be combined. (3) Derive the final algorithm” ( Supplementary Table S1 ). Additionally, multiple detailed instructions were specified so that the produced pseudo-phenotyping algorithm adhered to the OMOP concepts, including diagnosis codes (in ICD-9-CM and ICD-10-CM), symptoms, procedures, laboratory tests, and medications (both generic and brand names). We also mandated that the pseudo-phenotyping algorithm maintain a style consistent with the SQL logic, to facilitate generation of the SQL query in the second step.
Using the response of an LLM in the initial step, the second step involved converting the pseudo-phenotyping algorithm into an executable SQL query (referred to as an SQL-formatted phenotyping algorithm) for implementation in an OMOP CDM-based EHR database for subsequent validation ( Supplementary Table S1 ). Due to the probabilistic nature of LLMs, variations in the generated phenotyping algorithms are guaranteed. Consequently, we executed α-prompting five times independently for each phenotype and for each LLM to account for response variability.
The β-prompting strategy (step 3 in Figure 1 ) was designed to first present the LLM with the five SQL-formatted phenotyping algorithms generated from the α-prompting strategy and then instruct the LLM to assess the quality of these algorithms and generate an improved one ( Supplementary Table S1 ). This strategy, proven to effectively mitigate hallucinations 26–28 (ie, in the context of EHR phenotyping, generating column names that do not follow OMOP CDM or producing concept names, concept IDs, or even logics that are irrelevant to the target phenotype), leverages an LLM’s ability to evaluate scientific texts based on the extensive knowledge encoded during model pretraining. As a result, the LLM can produce an updated version of the phenotyping algorithm through analytical reflections. We also deployed the CoT strategy in β-prompting, which involved initially identifying the correct, incorrect, and missing criteria for each previously generated algorithm. The β-prompting strategy was executed in an independent session of the LLM (distinct from and subsequent to those used for α-prompting) and was executed only once.
Evaluating the quality of LLM-generated phenotyping algorithms
We then performed a comprehensive analysis, encompassing both qualitative and quantitative assessments, to evaluate the efficacy of the phenotyping algorithms generated by the four different LLMs on the three diseases of focus as described above. For the qualitative analysis, three experts (W.Q.W., M.E.G., and V.E.K.) in EHR phenotyping and clinical medicine, each with significant experience in clinical and informatics research, independently reviewed and rated the LLM-generated SQL-formatted phenotyping algorithms in a blind manner. All experts had authored numerous papers related to clinical phenotyping. We further compared the concepts utilized in these algorithms with those found in clinician-validated phenotyping algorithms developed by the eMERGE network. 16 , 17 The algorithms selected 18–22 were developed among large patient populations, validated across multiple research centers, and widely recognized for their reliability. Specifically for dementia, the chosen eMERGE algorithm excludes mild cognitive impairment codes and does not filter out delirium diagnoses, reflecting the reality that dementia patients may also present with delirium. For quantitative assessment, we implemented the top-rated SQL-formatted phenotyping algorithms using EHR data from Vanderbilt University Medical Center (VUMC) and assessed their performance against established eMERGE algorithms. 18–22
Expert assessment
Each of the three experts conducted independent reviews for every SQL-formatted phenotyping algorithm (4 LLMs, 3 phenotypes, and 2 strategies, for 24 algorithms in total). The evaluation focused on three dimensions: (1) adherence to instructions, which assessed how well the LLM conformed to predefined formatting rules; (2) the generation of proficient phenotyping algorithms based on knowledge, which evaluated the LLM’s ability to synthesize and organize phenotyping-related information effectively; and (3) presentation in executable SQL format, which measured the potential of an LLM to reduce the labor-intensive human efforts required for EHR implementation and validation. Detailed guidelines for expert evaluation can be found in Supplementary Table S2 . Experts assigned categorical scores (“Good [3],” “Medium [2],” or “Poor [1]”) for each axis based on predefined criteria, providing justifications accordingly. Interrater reliability was assessed using the weighted Cohen’s Kappa score. 29 We compared LLMs’ rated scores using the Wilcoxon signed-rank test 30 with a significance level of 0.05.
Comparison of concept coverage with eMERGE phenotyping algorithms
This analysis provided a comprehensive comparison of the concepts within phenotyping algorithms generated by LLMs and established EHR algorithms. We systematically reviewed and compared all the concepts employed in the algorithms for diagnoses, laboratory tests, procedures, medications, symptoms, and exclusions, and then summarized the noteworthy findings.
Implementation of LLM-generated algorithms and performance evaluation
We deployed the highest-rated phenotyping algorithms for each phenotype in a research cohort at VUMC ( n = 84 821). This cohort, extensively utilized in phenotyping research, has been a significant resource for phenotypic studies. 5 , 31 We summarized implementation details in Supplementary section S.3 , where we list the general edits we made as well as specific changes for each implemented algorithm ( Supplementary Table S3 ). As a benchmark, we implemented three eMERGE algorithms updated with current ICD-10-CM codes 5 to identify phenotype cases and controls. The cases and controls identified by the eMERGE algorithms served as a reference standard to assess the effectiveness of the LLM-generated algorithms. In our subsequent analysis, we excluded patients not categorized as either case or control by the eMERGE algorithm, as their data did not meet the criteria for either situation. Each of the top-rated LLM-generated algorithms required some modifications to be executable in a cloud-based platform to securely query VUMC’s research clinical databases that follow the OMOP CDM. We limited changes to technical domain knowledge, as opposed to clinical domain knowledge. For example, we edited the database names, but did not edit drug names or ICD codes.
We used the following metrics for evaluation: (1) positive predictive values (PPV), defined as the number of cases mutually identified by the eMERGE algorithm and an LLM over the total number of identified cases by the LLM; (2) recall, defined as the number of cases mutually identified by the eMERGE algorithm and an LLM over the total number of cases identified by the eMERGE algorithm; and (3) false positive rate (FPR), defined as the number of patients identified as cases by an LLM but identified as controls by the eMERGE algorithm (false positives) over the sum of false positives and number of cases identified by the eMERGE algorithm.
Expert assessments
The average interrater reliability was 0.59 [0.50-0.68], indicating a moderate to substantial agreement among experts, considering the categorical nature of the data (rather than dichotomous) and the variations in scoring criteria among different experts. 32
By mapping the experts’ assessments of “Good”, “Medium”, or “Poor” to numerical scores of 3, 2, and 1, respectively, GPT-4 (mean [95% confidence interval]: 2.57 [2.40-2.75]) and GPT-3.5 (2.43 [2.25-2.60]) exhibited significantly higher overall expert evaluation scores than Claude 2 (1.91 [1.68-2.13]) and Bard (1.20 [1.09-1.31]) ( Figure 2A ). GPT-4 marginally outperformed GPT-3.5, though the differences were not statistically significant. Moreover, the β-prompting strategy did not significantly differ from the α-prompting, according to experts’ evaluation ( Figure 2B ). Furthermore, experts assigned higher scores to LLMs for their effectiveness in generating phenotyping algorithms for T2DM and hypothyroidism compared to dementia ( Figure 2C ). The radar plot shown in Figure 2D displays the average scores for each involved LLM across the three axes of evaluation. There are two key findings. First, GPT-4 and GPT-3.5 were rated consistently rated better than Claude 2 and Bard in following instructions, algorithmic logic, and SQL executability. Second, GPT-4 was considered to be on par with GPT-3.5 in its ability to follow instructions and SQL executability, yet it surpassed GPT-3.5 in its algorithmic logic. Based on these findings, we continued our investigation with GPT-4 and GPT-3.5.
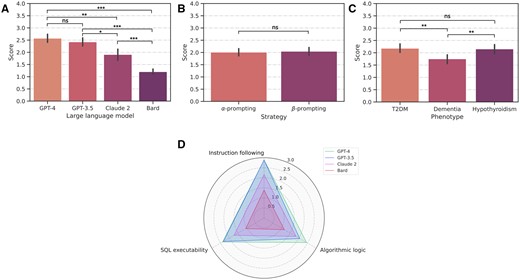
A comparative analysis based on expert evaluations focusing on ( A ) four large language models, ( B ) two prompting strategies, ( C ) three phenotypes, and ( D ) three individual evaluation axes. Numeric scores of 3, 2, and 1 correspond to expert assessments of “Good”, “Medium”, and “Poor”, respectively. ***, **, and * denote P < .001, P < .01, and P < .05, respectively. ns = not significant.
Comparison with eMERGE phenotyping algorithms
Table 1 summarizes the clinical concepts identified by the eMERGE phenotyping algorithms and LLM-produced algorithms. A full comparison of concepts can be found in Supplementary Table S4 . Given that the phenotyping algorithms produced by both GPT-4 and GPT-3.5 from the β-prompting strategy were rated similarly to those from the α-prompting strategy, we focused further analyses on the β-prompting results.
Shared and non-shared concepts from the eMERGE, GPT-4 (β-prompting), and GPT-3.5 (β-prompting) phenotyping algorithms for T2DM, dementia, and hypothyroidism.
Abbreviations: BG, blood glucose; BMI, body mass index; CPT, current procedural terminology; GTT, glucose tolerance test; HgbA1c, hemoglobin A1c; ICD, International Classification of Diseases; MMSE, Mini-Mental State Exam; MRI, magnetic resonance imaging; TSH, thyroid stimulating hormone; TPO, thyroperoxidase.
Inaccurately includes IC9-CM codes for type 1 diabetes mellitus with 250*.
All three phenotyping algorithms use a HgbA1c cutoff >6.5.
eMERGE and ChatGPT-4 use the correct fasting BG > 126 mg/dL and ChatGPT-3.5 incorrectly uses cutoff >6.5 mg/dL.
This denotes a unique concept example not used in any other phenotyping algorithm.
Different TSH cutoffs used by each phenotyping algorithm (eMERGE: >5, ChatGPT-4: >upper limit of normal; ChatGPT-3.5: >4.5).
Inaccurately includes amiodarone, which can cause hypothyroidism, as a treatment for hypothyroidism.
Rather than specifying specific procedure codes, queried the concept table for concepts exactly matching “Thyroid surgery” or “Radioactive iodine treatment” (returned five procedure codes including 2 SNOMED, 1 Nebraska Lexicon, 1 HemOnc, and 1 MeSH).
There are several notable observations. For the T2DM phenotyping algorithm, GPT-4 and GPT-3.5 identified relevant diagnosis codes (both ICD-9-CM and ICD-10-CM), lab tests (hemoglobin A1c, fasting blood glucose), and two generic medications (metformin and glipizide) that were also used in the eMERGE phenotyping algorithm. The eMERGE phenotyping algorithm also included medications not identified by either GPT-4 or GPT-3.5 ( n = 27). Only GPT-4 and GPT-3.5 included symptoms; both eMERGE and GPT-4 provided exclusionary criteria (ICD codes). Notably, the GPT-3.5 model incorrectly included ICD-9-CM codes for type 1 diabetes and applied an incorrect cutoff value for fasting blood glucose (>6.5 mg/dL instead of >125 mg/dL).
For the dementia phenotyping algorithm, GPT-4 and GPT-3.5 included relevant diagnosis codes (ICD-9-CM and ICD-10-CM, as well as one ICD-10). While there were several overlapping diagnosis codes between each pair of phenotyping algorithms, no diagnosis codes were shared across all three algorithms. GPT-4 and GPT-3.5 included symptoms potentially related to dementia while the eMERGE algorithm did not. All three phenotyping algorithms shared four medications (two generic: donepezil and memantine, and two brand names: Aricept and Namenda), although the eMERGE algorithm specified additional medications that did not appear in either LLM-generated algorithm ( n = 7). Only the GPT-4 phenotyping algorithm included exclusion criteria (eg, vitamin B12 level).
For the hypothyroidism phenotyping algorithm, GPT-4 and GPT-3.5 identified relevant diagnosis codes (both ICD-9-CM and ICD-10-CM), lab tests (thyroid stimulating hormone), and medications (generic levothyroxine and brand name Synthroid) used in the eMERGE algorithm. Only the eMERGE algorithm used thyroid autoantibodies (eg, thyroid antiperoxidase). Notably, the GPT-4 algorithm specified the largest number of medications ( n = 15), including nine medications that were not included in either the eMERGE algorithm or the GPT-3.5 algorithm. Once again, only GPT-4 and GPT-3.5 included symptoms. The eMERGE algorithm included 113 exclusions, including 18 ICD codes, 79 CPT codes, and 16 medications, while GPT-4 and GPT-3.5 specified only 2 and 0 exclusions, respectively.
Implementation and evaluation in VUMC
Along with the eMERGE algorithms, we successfully deployed all algorithms generated by GPT-4 and GPT-3.5 from the β-prompting strategy except the T2DM algorithm generated by GPT-3.5 ( Table 2 ). The failure of this phenotyping algorithm stemmed from its restrictive logic which accumulated LEFT JOINs across various tables, including the Person, Condition_Occurrence, Measurement, Drug_Exposure, and Observation tables. The algorithm required a LEFT JOIN on a list of people who had a record of symptoms in the Observation table (Polyuria, Polydipsia, Unexplained weight loss) and had a value of zero in the “value_as_concept_id” column. A value of zero did not exist for these symptoms, therefore the algorithm could not identify any individuals who met this criterion for T2DM.
Performance of the phenotyping algorithms generated by GPT-4 and GPT-3.5 from the β-prompting strategy when applied to VUMC data, as measured against clinician-validated algorithms for the eMERGE phenotype cases and controls.
TP, true positive; FP, false positive; FDR, false discovery rate; T2DM, type 2 diabetes mellitus.
The case (and control) prevalences for the eMERGE algorithms in our population were 10.9% (28.0%), 3.5% (91.5%), and 2.4% (30.4%) for T2DM, dementia, and hypothyroidism, respectively. The GPT-4 generated dementia algorithm achieved the highest PPV of 96.3% at the expense of a low recall (24.4%). This result was primarily attributable to the relatively restrictive inclusion criteria, which required medication prescription coupled with the co-occurrence of either diagnosis codes, symptoms, or cognitive assessment tests or procedures. The GPT-4 generated algorithms for T2DM and hypothyroidism, as well as the GPT-3.5 generated algorithm for hypothyroidism, achieved high recall but at the cost of lower PPV. In contrast, the GPT-3.5 generated algorithm for dementia achieved balanced PPV and recall.
EHR phenotyping is a critical area of modern observational clinical research, yet it commonly demands substantial resources. In this study, we explored the effectiveness of LLMs in creating preliminary versions of computable phenotyping algorithms, with the ultimate goal of streamlining the EHR phenotyping process.
Not all LLMs we tested were well-suited for phenotyping. GPT-4 and GPT-3.5 significantly outperformed both Claude 2 and Bard in their ability to generate executable and accurately SQL-formatted phenotyping algorithms. One of the reasons for this discrepancy was Claude 2’s tendency to represent concepts using numerical concept codes, without specifying what clinical criteria these concept codes were intended to capture. Five of the algorithms generated by Bard did not follow the OMOP CDM. Four of these algorithms referenced columns that did not exist in the OMOP CDM. These corresponded to “patient_id” for both prompting strategies of dementia, “observation_fact” and “measurement_fact” for the α-prompting strategies of T2DM, and “occurrence_age” for the β-prompting strategy of T2DM. Additionally, two of the algorithms searched for concepts in the wrong tables. The α-prompting algorithm for dementia attempted to use the “person” table to extract all concepts, including diagnosis codes and medications, which would not be found in this table, whereas the β-prompting algorithm for hypothyroidism looked for signs and symptoms in the Measurement table. We consider these behaviors to be indicative of LLM hallucinations in the context of EHR phenotyping. Given the poor performance of the phenotyping algorithms generated by Claude 2 and Bard, we focused the remaining analysis on phenotyping algorithms generated by GPT-4 and GPT-3.5.
Both GPT models were able to follow instructions and identify relevant concepts for the three selected phenotypes. The phenotyping algorithms generated by these models contained reasonably accurate diagnosis codes, related lab tests, and key medications. The concepts identified largely overlapped with the ones used by domain experts. We found that GPT-4 generally demonstrated slightly superior performance compared to GPT-3.5 by identifying more medications and providing more appropriate thresholds for lab values ( Table 1 ). Moreover, the GPT-4 and GPT-3.5 generated algorithms were able to identify additional potentially useful criteria, including a variety of symptoms and clinical signs for each phenotype, which have not usually been incorporated in any eMERGE algorithms.
Despite the merits of GPT-4 and GPT-3.5, they produced phenotyping algorithms containing incorrect criteria. For example, both GPT-4 and GPT-3.5 erroneously considered the ICD-10 code F00 as a relevant diagnosis code for dementia, whereas our prompts specifically required ICD-10-CM codes. Additionally, GPT-3.5 occasionally selected inappropriately broad ICD-9-CM codes, such as 250* for T2DM, inadvertently encompassing diagnoses related to other types of diabetes. Also, both GPT models missed some key ICD codes and medications. For example, while the query correctly identified patients with ICD-10-CM code G30 as dementia cases, they overlooked patients with more specific codes such as G30.0, G30.1, G30.8, and G30.9. The algorithms produced by both GPT models generally generated a shorter list of medications compared to their corresponding eMERGE algorithms, with the exception of the GPT-4 produced algorithm for hypothyroidism. In this case, GPT-4 interpreted the hypothyroidism phenotype more broadly to include not only endogenous causes of hypothyroidism (as in the eMERGE algorithm) but also exogenous causes (not included in the eMERGE algorithm). As a result, the medications specified by the GPT-4 hypothyroidism algorithm included both drugs used for treatment of hypothyroidism and drugs with potential to cause hypothyroidism (ie, lithium, amiodarone, Lithobid, Cordarone, Nexterone, Pacerone). Moreover, certain thresholds set for lab values were inaccurate, such as “fasting blood glucose ≥6.5” for T2DM.
As noted above, compared to the eMERGE algorithms, both GPT-4 and GPT-3.5 introduced some new concepts, including signs and symptoms such as fatigue, cold intolerance, and weight gain. Many of these concepts are infrequently used by domain experts in algorithm generation due to their low specificity. Including these low-specificity concepts might not substantially enhance the recall of the algorithms and could potentially diminish the algorithm’s PPV. Despite this, they showed promise in identifying noteworthy concepts, such as the Mini-Mental State Examination and Montreal Cognitive Assessment for dementia.
Finally, the evaluated LLMs exhibited immature capability in organizing phenotyping criteria with the proper logic. The SQL queries generated by GPT-4 and GPT-3.5 were predominantly characterized by a single logical operator (AND or OR), resulting in phenotyping algorithms that were either excessively restrictive or overly broad.
Collectively, our findings highlight that LLMs have the potential to produce helpful preliminary phenotyping algorithms by identifying relevant clinical concepts and criteria. As a result, we believe they have the potential to accelerate the creation of EHR-based phenotypes; however, clinical phenotyping expertise, familiarity with EHR data models, and SQL programming skills remain critical for assessing and subsequently enhancing the efficacy of LLM-produced algorithms. At present, LLMs fall short of creating ready-to-use algorithms without revision. Therefore, incorporating a human-in-the-loop approach is necessary at this stage to ensure the algorithms’ practical applicability and accuracy. Still, this preliminary step would allow for a shift from traditional approaches where domain experts independently conduct comprehensive literature review and evidence synthesis—a process that is both time-intensive and challenging—to a more efficient and manageable model, where the domain experts’ role evolves to assessing and refining algorithms generated by LLMs.
Limitations and future work
Several limitations need to be highlighted as potential opportunities for future improvement. First, this pilot study was limited in scope and did not investigate all the possible tools, options, and capabilities of the LLMs listed here. Additionally, we did not fully explore the improvements, if any, of different prompt-engineering strategies, in-context learning, or fine-tuning. We are reporting initial observations from using the most basic and widely accessible version of these LLMs, ie, the chat box interface with default settings available through the websites of each of the LLMs. Using an API would allow for selection of additional parameters, which may affect performance. Second, as a pilot study, this research focused on prompting LLMs to generate algorithms for identifying phenotype cases. The capability of LLMs in generating algorithms to identify controls also needs to be evaluated. Third, we tested solely on proprietary models and their default configurations. It is important to assess both proprietary models and the leading open-source models (eg, Llama 2), especially when they are enhanced with fine-tuning capabilities and knowledge integration. Fourth, our design of prompts did not consider optimizing for execution efficiency of the SQL queries. Consequently, LLMs often produced SQL queries with suboptimal query structures. Fifth, this study only considered phenotypes for three common diseases. Phenotyping rare diseases may present different challenges particularly when there is limited relevant online content for a particular disease. Sixth, there is a chance that the phenotyping algorithms produced by LLMs might include clinical concepts that are not universally adopted by the OMOP databases of all healthcare organizations. As a result, this could lead to uncertainty in the algorithms’ effectiveness in patient identification in such situations. Nevertheless, we believe this issue can be addressed through a human-in-the-loop approach, which involves initially determining the concepts that are either not implemented or included, followed by re-prompting the LLMs to fine-tune the algorithm to exclude these concepts. Seventh, the consistency of responses generated by proprietary LLMs varies over time, warranting consideration for potential future investigations. New LLMs are being rapidly deployed, and our future efforts will involve exploring alternative advanced models, such as ResearchGPT 33 and Google Gemini. 34 Additionally, we will be delving into more refined control and customization in the generation process through prompt engineering to achieve desired performance levels.
GPT-4 and GPT-3.5 of ChatGPT are capable of producing phenotyping algorithm drafts that align with a standard CDM. These models can reasonably identify relevant clinical inclusion and exclusion criteria that can be used in an initial draft phenotype algorithm. Nevertheless, expertise in informatics and clinical experience is still required to assess and further refine LLM-generated phenotyping algorithms for improving EHR phenotyping accuracy.
C.Y. designed the interactions with the large language models (LLM), collected data, and analyzed the results. W.Q.W. and H.H.O. implemented the algorithms produced by LLMs. M.E.G., V.E.K., and W.Q.W. performed expert review for the produced algorithms. M.E.G., C.Y., H.H.O., V.E.K., and M.S.K. drafted the paper. W.Q.W., B.A.M., W.C.S., A.L.D., Q.P.F., and C.M.S. critically revised the paper. D.M.R. and J.F.P. reviewed the paper and provided important intellectual content. W.Q.W. supervised this study. All authors approved this study.
Supplementary material is available at Journal of the American Medical Informatics Association online.
This work was supported by the National Institute of Health grants R01GM139891, R01AG069900, R01HL163854, R01LM012806, R01HG012748, F30AG080885, T32GM007347, K01HL157755, U01HG011181, and UL1TR002243.
None declared.
Source code for results, LLM-generated SQL-formatted algorithms, and implemented SQL code are shared at https://github.com/The-Wei-Lab/LLM-Phenotyping-2024 .
Wei WQ , Denny JC. Extracting research-quality phenotypes from electronic health records to support precision medicine . Genome Med . 2015 ; 7 ( 1 ): 41 .
Google Scholar
Banda JM , Seneviratne M , Hernandez-Boussard T , Shah NH. Advances in electronic phenotyping: from rule-based definitions to machine learning models . Annu Rev Biomed Data Sci . 2018 ; 1 ( 1 ): 53 - 68 .
Pacheco JA , Rasmussen LV , Wiley K Jr , et al. Evaluation of the portability of computable phenotypes with natural language processing in the eMERGE network . Sci Rep . 2023 ; 13 ( 1 ): 1971 .
Newton KM , Peissig PL , Kho AN , et al. Validation of electronic medical record-based phenotyping algorithms: results and lessons learned from the eMERGE network . J Am Med Inform Assoc . 2013 ; 20 ( e1 ): e147 - e154 .
Zheng NS , Feng Q , Kerchberger VE , et al. PheMap: a multi-resource knowledge base for high-throughput phenotyping within electronic health records . J Am Med Inform Assoc . 2020 ; 27 ( 11 ): 1675 - 1687 .
Wu P , Gifford A , Meng X , et al. Mapping ICD-10 and ICD-10-CM codes to phecodes: workflow development and initial evaluation . JMIR Med Inform . 2019 ; 7 ( 4 ): e14325 .
Grabowska ME , Van Driest SL , Robinson JR , et al. Developing and evaluating pediatric phecodes (Peds-phecodes) for high-throughput phenotyping using electronic health records . J Am Med Inform Assoc . 2024 ; 31 ( 2 ): 386 - 395 .
Zhang Y , Cai T , Yu S , et al. High-throughput phenotyping with electronic medical record data using a common semi-supervised approach (PheCAP) . Nat Protoc . 2019 ; 14 ( 12 ): 3426 - 3444 .
Clusmann J , Kolbinger FR , Muti HS , et al. The future landscape of large language models in medicine . Commun Med (Lond) . 2023 ; 3 ( 1 ): 141 - 148 .
Yang J , Liu C , Deng W , et al. Enhancing phenotype recognition in clinical notes using large language models: PhenoBCBERT and PhenoGPT . Patterns (N Y) . 2024 ; 5 ( 1 ): 100887 .
Alsentzer E , Rasmussen MJ , Fontoura R , et al. Zero-shot interpretable phenotyping of postpartum hemorrhage using large language models . NPJ Digit Med . 2023 ; 6 ( 1 ): 212 .
OpenAI . 2023 . GPT-4 Technical Report, arXiv, arXiv:2303.08774, preprint: not peer reviewed.
Introducing ChatGPT. 2022 . OpenAI. Accessed December 14, 2023. https://openai.com/blog/chatgpt
Introducing Claude . 2023 . Anthropic . Accessed December 14, 2023. https://www.anthropic.com/index/introducing-claude
Pichai S. 2023 . Google AI updates: Bard and new AI features in Search. Accessed December 14, 2023. https://blog.google/technology/ai/bard-google-ai-search-updates/
Gottesman O , Kuivaniemi H , Tromp G , et al. ; eMERGE Network . The electronic medical records and genomics (eMERGE) network: past, present, and future . Genet Med . 2013 ; 15 ( 10 ): 761 - 771 .
McCarty CA , Chisholm RL , Chute CG , et al. ; eMERGE Team . The eMERGE Network: a consortium of biorepositories linked to electronic medical records data for conducting genomic studies . BMC Med Genomics . 2011 ; 4 : 13 - 11 .
Kho AN , Hayes MG , Rasmussen-Torvik L , et al. Use of diverse electronic medical record systems to identify genetic risk for type 2 diabetes within a genome-wide association study . J Am Med Inform Assoc . 2012 ; 19 ( 2 ): 212 - 218 .
Pacheco J , Thompson W. 2012 . Northwestern University. Type 2 diabetes mellitus. Phekb.org. Accessed December 14, 2023. https://phekb.org/phenotype/type-2-diabetes-mellitus
Carlson C. 2012 . Group Health Cooperative. Dementia. PheKB . org . Accessed December 14, 2023. https://phekb.org/phenotype/10
Denny J. 2012 . Group Health Cooperative, Marshfield Clinic Research Foundation, Mayo Medical School College of Medicine, Northwestern University, Vanderbilt University. Hypothyroidism. PheKB.org . Accessed December 14, 2023. https://phekb.org/phenotype/14
Denny JC , Crawford DC , Ritchie MD , et al. Variants near FOXE1 are associated with hypothyroidism and other thyroid conditions: using electronic medical records for genome- and phenome-wide studies . Am J Hum Genet . 2011 ; 89 ( 4 ): 529 - 542 .
OMOP Common Data Model. Github.io . Accessed December 14, 2023. https://ohdsi.github.io/CommonDataModel/
OHDSI—observational health data sciences and informatics . Ohdsi.org. Accessed December 14, 2023. https://www.ohdsi.org/
Wei J , Wang X , Schuurmans D , et al. Chain-of-thought prompting elicits reasoning in large language models. Proc of the 37 th Conference on Neural Information Processing Systems. New Orleans; 2022 ; 35 : 24824 - 24837 .
Madaan A , Tandon N , Gupta P , et al. Self-refine: iterative refinement with self-feedback. Proc of the 38 th Conference on Neural Information Processing Systems. New Orleans; 2023;36: 46534 - 46594 .
Pan L , Saxon M , Xu W , Nathani D , Wang X , Wang WY. 2023 . Automatically correcting large language models: surveying the landscape of diverse self-correction strategies. arXiv, 2308.03188, preprint: not peer reviewed.
Samwald M , Praas R , Hebenstreit K. Towards unified objectives for self-reflective AI . SSRN J . 2023 . http://dx.doi.org/10.2139/ssrn.4446991 .
Vanbelle S. A new interpretation of the weighted kappa coefficients . Psychometrika . 2016 ; 81 ( 2 ): 399 - 410 .
Woolson RF. Wilcoxon signed‐rank test . In: Wiley Encyclopedia of Clinical Trials . John Wiley & Sons; 2007 : 1 - 3 .
Wan NC , Yaqoob AA , Ong HH , Zhao J , Wei W-Q. Evaluating resources composing the PheMAP knowledge base to enhance high-throughput phenotyping . J Am Med Inform Assoc . 2023 ; 30 ( 3 ): 456 - 465 .
Landis JR , Koch GG. The measurement of observer agreement for categorical data . Biometrics . 1977 ; 33 ( 1 ): 159 - 174 .
ResearchGPT . 2023 . Researchgpt.com. Accessed December 14, 2023. https://www.researchgpt.com/
Gemini . 2023 . Google DeepMind. Accessed December 14, 2023. https://deepmind.google/technologies/gemini/
Supplementary data
Email alerts, citing articles via.
- Recommend to your Library
Affiliations
- Online ISSN 1527-974X
- Copyright © 2024 American Medical Informatics Association
- About Oxford Academic
- Publish journals with us
- University press partners
- What we publish
- New features
- Open access
- Institutional account management
- Rights and permissions
- Get help with access
- Accessibility
- Advertising
- Media enquiries
- Oxford University Press
- Oxford Languages
- University of Oxford
Oxford University Press is a department of the University of Oxford. It furthers the University's objective of excellence in research, scholarship, and education by publishing worldwide
- Copyright © 2024 Oxford University Press
- Cookie settings
- Cookie policy
- Privacy policy
- Legal notice
This Feature Is Available To Subscribers Only
Sign In or Create an Account
This PDF is available to Subscribers Only
For full access to this pdf, sign in to an existing account, or purchase an annual subscription.

Inherent Bias in Electronic Health Records: A Scoping Review of Sources of Bias
- Find this author on Google Scholar
- Find this author on PubMed
- Search for this author on this site
- ORCID record for Oriel Perets
- For correspondence: [email protected]
- ORCID record for Emanuela Stango
- ORCID record for Eyal Ben Yehuda
- ORCID record for Megan C McNichol
- ORCID record for Leo Anthony Celi
- ORCID record for Nadav Rappoport
- ORCID record for Matilda Dorotic
- Info/History
- Preview PDF
Objectives: Biases inherent in electronic health records (EHRs), and therefore in medical artificial intelligence (AI) models may significantly exacerbate health inequities and challenge the adoption of ethical and responsible AI in healthcare. Biases arise from multiple sources, some of which are not as documented in the literature. Biases are encoded in how the data has been collected and labeled, by implicit and unconscious biases of clinicians, or by the tools used for data processing. These biases and their encoding in healthcare records undermine the reliability of such data and bias clinical judgments and medical outcomes. Moreover, when healthcare records are used to build data-driven solutions, the biases are further exacerbated, resulting in systems that perpetuate biases and induce healthcare disparities. This literature scoping review aims to categorize the main sources of biases inherent in EHRs. Methods: We queried PubMed and Web of Science on January 19th, 2023, for peer-reviewed sources in English, published between 2016 and 2023, using the PRISMA approach to stepwise scoping of the literature. To select the papers that empirically analyze bias in EHR, from the initial yield of 430 papers, 27 duplicates were removed, and 403 studies were screened for eligibility. 196 articles were removed after the title and abstract screening, and 96 articles were excluded after the full-text review resulting in a final selection of 116 articles. Results: Systematic categorizations of diverse sources of bias are scarce in the literature, while the effects of separate studies are often convoluted and methodologically contestable. Our categorization of published empirical evidence identified the six main sources of bias: a) bias arising from past clinical trials; b) data-related biases arising from missing, incomplete information or poor labeling of data; human-related bias induced by c) implicit clinician bias, d) referral and admission bias; e) diagnosis or risk disparities bias and finally, (f) biases in machinery and algorithms. Conclusions: Machine learning and data-driven solutions can potentially transform healthcare delivery, but not without limitations. The core inputs in the systems (data and human factors) currently contain several sources of bias that are poorly documented and analyzed for remedies. The current evidence heavily focuses on data-related biases, while other sources are less often analyzed or anecdotal. However, these different sources of biases add to one another exponentially. Therefore, to understand the issues holistically we need to explore these diverse sources of bias. While racial biases in EHR have been often documented, other sources of biases have been less frequently investigated and documented (e.g. gender-related biases, sexual orientation discrimination, socially induced biases, and implicit, often unconscious, human-related cognitive biases). Moreover, some existing studies lack causal evidence, illustrating the different prevalences of disease across groups, which does not per se prove the causality. Our review shows that data-, human- and machine biases are prevalent in healthcare and they significantly impact healthcare outcomes and judgments and exacerbate disparities and differential treatment. Understanding how diverse biases affect AI systems and recommendations is critical. We suggest that researchers and medical personnel should develop safeguards and adopt data-driven solutions with a "bias-in-mind" approach. More empirical evidence is needed to tease out the effects of different sources of bias on health outcomes.
Competing Interest Statement
The authors have declared no competing interest.
Clinical Protocols
https://osf.io/skdm7
Funding Statement
LAC is funded by the National Institute of Health through NIBIB R01 EB017205.
Author Declarations
I confirm all relevant ethical guidelines have been followed, and any necessary IRB and/or ethics committee approvals have been obtained.
I confirm that all necessary patient/participant consent has been obtained and the appropriate institutional forms have been archived, and that any patient/participant/sample identifiers included were not known to anyone (e.g., hospital staff, patients or participants themselves) outside the research group so cannot be used to identify individuals.
I understand that all clinical trials and any other prospective interventional studies must be registered with an ICMJE-approved registry, such as ClinicalTrials.gov. I confirm that any such study reported in the manuscript has been registered and the trial registration ID is provided (note: if posting a prospective study registered retrospectively, please provide a statement in the trial ID field explaining why the study was not registered in advance).
I have followed all appropriate research reporting guidelines, such as any relevant EQUATOR Network research reporting checklist(s) and other pertinent material, if applicable.
Data Availability
A scoping review, all articles are available in the bibliography to this manuscript
View the discussion thread.
Thank you for your interest in spreading the word about medRxiv.
NOTE: Your email address is requested solely to identify you as the sender of this article.

Citation Manager Formats
- EndNote (tagged)
- EndNote 8 (xml)
- RefWorks Tagged
- Ref Manager
- Tweet Widget
- Facebook Like
- Google Plus One
- Addiction Medicine (316)
- Allergy and Immunology (621)
- Anesthesia (162)
- Cardiovascular Medicine (2291)
- Dentistry and Oral Medicine (280)
- Dermatology (202)
- Emergency Medicine (370)
- Endocrinology (including Diabetes Mellitus and Metabolic Disease) (809)
- Epidemiology (11607)
- Forensic Medicine (10)
- Gastroenterology (682)
- Genetic and Genomic Medicine (3606)
- Geriatric Medicine (338)
- Health Economics (620)
- Health Informatics (2320)
- Health Policy (916)
- Health Systems and Quality Improvement (865)
- Hematology (336)
- HIV/AIDS (756)
- Infectious Diseases (except HIV/AIDS) (13184)
- Intensive Care and Critical Care Medicine (760)
- Medical Education (360)
- Medical Ethics (101)
- Nephrology (391)
- Neurology (3384)
- Nursing (192)
- Nutrition (511)
- Obstetrics and Gynecology (652)
- Occupational and Environmental Health (651)
- Oncology (1773)
- Ophthalmology (526)
- Orthopedics (210)
- Otolaryngology (284)
- Pain Medicine (224)
- Palliative Medicine (66)
- Pathology (441)
- Pediatrics (1010)
- Pharmacology and Therapeutics (423)
- Primary Care Research (408)
- Psychiatry and Clinical Psychology (3090)
- Public and Global Health (6012)
- Radiology and Imaging (1231)
- Rehabilitation Medicine and Physical Therapy (716)
- Respiratory Medicine (813)
- Rheumatology (370)
- Sexual and Reproductive Health (357)
- Sports Medicine (318)
- Surgery (390)
- Toxicology (50)
- Transplantation (171)
- Urology (142)
- Download PDF
- Share X Facebook Email LinkedIn
- Permissions
Prediction Models and Clinical Outcomes—A Call for Papers
- 1 Department of Medicine, University of Washington, Seattle
- 2 Deputy Editor, JAMA Network Open
- 3 Epidemiology, Rutgers The State University of New Jersey, New Brunswick
- 4 Statistical Editor, JAMA Network Open
- 5 Department of Biostatistics, Harvard T. H. Chan School of Public Health, Boston, Massachusetts
- 6 Editor, JAMA Network Open
The need to classify disease and predict outcomes is as old as medicine itself. Nearly 50 years ago, the advantage of applying multivariable statistics to these problems became evident. 1 Since then, the increasing availability of databases containing often-complex clinical information from tens or even hundreds of millions of patients, combined with powerful statistical techniques and computing environments, has spawned exponential growth in efforts to create more useful, focused, and accurate prediction models. JAMA Network Open receives dozens of manuscripts weekly that present new or purportedly improved instruments intended to predict a vast array of clinical outcomes. Although we are able to accept only a small fraction of those submitted, we have, nonetheless, published nearly 2000 articles dealing with predictive models over the past 6 years.
The profusion of predictive models has been accompanied by the growing recognition of the necessity for standards to help ensure accuracy of these models. An important milestone was the publication of the Transparent Reporting of a Multivariable Prediction Model for Individual Prognosis or Diagnosis ( TRIPOD ) guidelines nearly a decade ago. 2 TRIPOD is a reporting guideline intended to enable readers to better understand the methods used in published studies but does not prescribe what actual methods should be applied. Since then, while the field has continued to advance and technology improve, many predictive models in widespread use, when critically evaluated, have been found to neither adhere to reporting standards nor perform as well as expected. 3 , 4
There are numerous reasons why performance of models falls short, even when efforts are made to adhere to methodologic standards. Despite the vast amounts of data that are often brought to bear, they may not be appropriate to the task, or they may have been collected and analyzed in ways that are biased. Additionally, that some models fall short may simply reflect the inherent difficulty of predicting relatively uncommon events that occur as a result of complex biological processes occurring within complex clinical environments. Moreover, clinical settings are highly variable, and predictive models typically perform worse outside of the environments in which they were developed. A comprehensive discussion of these issues is beyond the scope of this article, but as physicist Neils Bohr once remarked, “it is very difficult to predict—especially the future.” 5
Although problems with accuracy are well documented, hundreds of predictive models are in regular use in clinical practice and are frequently the basis for critically important decisions. Many such models have been widely adopted without subsequent efforts to confirm that they actually continue to perform as expected. That is not to say that such models are without utility, because even a suboptimal model may perform better than an unaided clinician. Nevertheless, we believe that a fresh examination of selected, well-established predictive models is warranted if not previously done. JAMA Network Open has published articles addressing prediction of relatively common clinical complications, such as recurrent gastrointestinal bleeding. 6 We think there remains considerable opportunity for research in this vein. In particular, we seek studies that examine current performance of commonly applied clinical prediction rules. We are particularly interested in studies using data from a variety of settings and databases as well as studies that simultaneously assess multiple models addressing the same or similar outcomes.
We also remain interested in the derivation of new models that address a clear clinical need. They should utilize data that are commonly collected as part of routine care, or in principle can be readily extracted from electronic health records. We generally require that prediction models be validated with at least 1 other dataset distinct from the development dataset. In practice, this means data from different health systems or different publicly available or commercial datasets. We note that internal validation techniques, such as split samples, hold-out, k -fold, and others, are not designed to overcome the intrinsic differences between data sources and, therefore, are not suited to quantifying performance externally. While the population to which the models apply should be described explicitly, ideally any such models should be applicable to patients from the wide range of races, ethnicities, and backgrounds commonly encountered in clinic practice. Most importantly, we are interested in examples of models that have been evaluated in clinical settings, assessing their feasibility and potential clinical benefit. This includes studies with negative as well as positive outcomes.
Please see the journal’s Instructions for Authors for information on manuscript preparation and submission. 7 This is not a time-limited call for studies on this topic.
Published: April 12, 2024. doi:10.1001/jamanetworkopen.2024.9640
Open Access: This is an open access article distributed under the terms of the CC-BY License . © 2024 Fihn SD et al. JAMA Network Open .
Corresponding Author: Stephan D. Fihn, MD, MPH, Department of Medicine, University of Washington, 325 Ninth Ave, Box 359780, Seattle, WA 98104 ( [email protected] ).
Conflict of Interest Disclosures: Dr Berlin reported receiving consulting fees from Kenvue related to acetaminophen outside the submitted work. No other disclosures were reported.
See More About
Fihn SD , Berlin JA , Haneuse SJPA , Rivara FP. Prediction Models and Clinical Outcomes—A Call for Papers. JAMA Netw Open. 2024;7(4):e249640. doi:10.1001/jamanetworkopen.2024.9640
Manage citations:
© 2024
Select Your Interests
Customize your JAMA Network experience by selecting one or more topics from the list below.
- Academic Medicine
- Acid Base, Electrolytes, Fluids
- Allergy and Clinical Immunology
- American Indian or Alaska Natives
- Anesthesiology
- Anticoagulation
- Art and Images in Psychiatry
- Artificial Intelligence
- Assisted Reproduction
- Bleeding and Transfusion
- Caring for the Critically Ill Patient
- Challenges in Clinical Electrocardiography
- Climate and Health
- Climate Change
- Clinical Challenge
- Clinical Decision Support
- Clinical Implications of Basic Neuroscience
- Clinical Pharmacy and Pharmacology
- Complementary and Alternative Medicine
- Consensus Statements
- Coronavirus (COVID-19)
- Critical Care Medicine
- Cultural Competency
- Dental Medicine
- Dermatology
- Diabetes and Endocrinology
- Diagnostic Test Interpretation
- Drug Development
- Electronic Health Records
- Emergency Medicine
- End of Life, Hospice, Palliative Care
- Environmental Health
- Equity, Diversity, and Inclusion
- Facial Plastic Surgery
- Gastroenterology and Hepatology
- Genetics and Genomics
- Genomics and Precision Health
- Global Health
- Guide to Statistics and Methods
- Hair Disorders
- Health Care Delivery Models
- Health Care Economics, Insurance, Payment
- Health Care Quality
- Health Care Reform
- Health Care Safety
- Health Care Workforce
- Health Disparities
- Health Inequities
- Health Policy
- Health Systems Science
- History of Medicine
- Hypertension
- Images in Neurology
- Implementation Science
- Infectious Diseases
- Innovations in Health Care Delivery
- JAMA Infographic
- Law and Medicine
- Leading Change
- Less is More
- LGBTQIA Medicine
- Lifestyle Behaviors
- Medical Coding
- Medical Devices and Equipment
- Medical Education
- Medical Education and Training
- Medical Journals and Publishing
- Mobile Health and Telemedicine
- Narrative Medicine
- Neuroscience and Psychiatry
- Notable Notes
- Nutrition, Obesity, Exercise
- Obstetrics and Gynecology
- Occupational Health
- Ophthalmology
- Orthopedics
- Otolaryngology
- Pain Medicine
- Palliative Care
- Pathology and Laboratory Medicine
- Patient Care
- Patient Information
- Performance Improvement
- Performance Measures
- Perioperative Care and Consultation
- Pharmacoeconomics
- Pharmacoepidemiology
- Pharmacogenetics
- Pharmacy and Clinical Pharmacology
- Physical Medicine and Rehabilitation
- Physical Therapy
- Physician Leadership
- Population Health
- Primary Care
- Professional Well-being
- Professionalism
- Psychiatry and Behavioral Health
- Public Health
- Pulmonary Medicine
- Regulatory Agencies
- Reproductive Health
- Research, Methods, Statistics
- Resuscitation
- Rheumatology
- Risk Management
- Scientific Discovery and the Future of Medicine
- Shared Decision Making and Communication
- Sleep Medicine
- Sports Medicine
- Stem Cell Transplantation
- Substance Use and Addiction Medicine
- Surgical Innovation
- Surgical Pearls
- Teachable Moment
- Technology and Finance
- The Art of JAMA
- The Arts and Medicine
- The Rational Clinical Examination
- Tobacco and e-Cigarettes
- Translational Medicine
- Trauma and Injury
- Treatment Adherence
- Ultrasonography
- Users' Guide to the Medical Literature
- Vaccination
- Venous Thromboembolism
- Veterans Health
- Women's Health
- Workflow and Process
- Wound Care, Infection, Healing
Get the latest research based on your areas of interest.
Others also liked.
- Register for email alerts with links to free full-text articles
- Access PDFs of free articles
- Manage your interests
- Save searches and receive search alerts
One way to speed up clinical trials: Skip right to the data with electronic medical records
S cientific knowledge, as measured by numbers of papers published, has been estimated to double every 17.3 years . However, it takes an average of about 17 years for health and medical research – going from basic lab studies on cell cultures and animals to clinical trials in people – to result in actual changes patients see in the clinic.
The typical process of medical research is generally not well equipped to respond effectively to quickly evolving pandemics. This has been especially evident for the COVID-19 pandemic, in part because the virus the causes COVID-19 mutates frequently. Scientists and public health officials are often left continually scrambling to develop and test new treatments to match emerging variants.
Fortunately, scientists may be able to bypass the typical research timeline and study treatments and interventions as they are used in the clinic nearly in real time by leveraging a common source of existing data – electronic medical records, or EMRs.
We are a team composed of an epidemiologist , pharmacist and cardiologist at the University of Pittsburgh Medical Center. During the COVID-19 pandemic, we realized the need to quickly study and disseminate accurate information on the most effective treatment approaches, especially for patients at high risk of hospitalization and death. In our recently published research , we used EMR data to show that early treatment with one or more of five different monoclonal antibodies substantially reduced the risk of hospitalization or death compared with delayed or no treatment.
Using EMR data for research
In the U.S., health care systems typically use EMR systems for documenting patient care and for administrative purposes like billing. While data collection is not uniform, these systems typically contain detailed records that can include sociodemographic information, medical history, test results, surgical and other procedures, prescriptions and billing charges.
Unlike single-payer health care systems that integrate data into a single EMR system, such as in the U.K. and in Scandinavian countries, many large health care systems in the U.S. collect patient data using multiple EMR systems .
Having multiple EMR systems adds a layer of complexity to using such data to conduct scientific research. To address this, the University of Pittsburgh Medical Center developed and maintains a clinical data warehouse that compiles and harmonizes data across the seven different EMR systems its 40 hospitals and outpatient clinics use.
Emulating clinical trials
Using EMR data for research is not new. More recently, researchers have been looking into ways to use these large health data systems to emulate randomized controlled trials , which are considered the gold standard study design yet are often costly and take years to complete.
Using this emulation framework, our team used the EMR data infrastructure at our institution to evaluate five different monoclonal antibodies for which the Food and Drug Administration granted emergency use authorization to treat COVID-19. Monoclonal antibodies are human-made proteins designed to prevent a pathogen – in this case the virus that causes COVID-19 – from entering human cells, replicating and causing serious illness. Initially the authorizations were based on clinical trial data. But as the virus mutated, subsequent evaluations based on cell culture studies suggested a loss of effectiveness.
We wanted to confirm that the findings of cell-based studies applied to actual patients. So we evaluated anonymous clinical data from 2,571 patients treated with these monoclonal antibodies within two days of COVID-19 infection, matching them with data from 5,135 patients with COVID-19 who were eligible for but either did not receive these treatments or received them three or more days after infection.
We found that overall, people who received monoclonal antibodies within two days of a positive COVID-19 test reduced their risk of hospitalization or death by 39% compared with those who did not receive the treatment or received delayed treatment. In addition, patients with compromised immune systems reduced their risk of hospitalization or death by 55%, regardless of their age.
Our near-real-time analysis of COVID-19 patients treated with monoclonal antibodies during the pandemic confirmed the findings of the cell culture studies. Our findings suggest that by using data in this way, researchers may be able to evaluate treatments in times of urgency without having to perform clinical trials.
Appropriate EMR data use
Many health care institutions have EMR systems that researchers can harness to rapidly answer important research questions as they arise. However, because this clinical data is not specifically collected for research purposes, researchers need to carefully design their studies and use rigorous data validation and analysis. They also need to take great care to harmonize data from different EMR systems, select appropriate patient samples and minimize all sources of potential bias.
New pandemics and significant public health challenges are likely to emerge abruptly and in unpredictable ways. Given the treasure trove of data routinely collected across U.S. health care systems, we believe that careful use of these data can help answer urgent health questions in ways that are representative of who’s actually receiving care.
- 90% of drugs fail clinical trials – here’s one way researchers can select better drug candidates
- Yes, Black patients do want to help with medical research – here are ways to overcome the barriers that keep clinical trials from recruiting diverse populations
Erin McCreary has served on scientific advisory boards for Shionogi, Inc and Merck.
Kevin Kip and Oscar Marroquin do not work for, consult, own shares in or receive funding from any company or organization that would benefit from this article, and have disclosed no relevant affiliations beyond their academic appointment.

An official website of the United States government
The .gov means it’s official. Federal government websites often end in .gov or .mil. Before sharing sensitive information, make sure you’re on a federal government site.
The site is secure. The https:// ensures that you are connecting to the official website and that any information you provide is encrypted and transmitted securely.
- Publications
- Account settings
Preview improvements coming to the PMC website in October 2024. Learn More or Try it out now .
- Advanced Search
- Journal List
- Medicine (Baltimore)
- v.95(19); 2016 May
Electronic Health Records and Quality of Care
Electronic health records (EHRs) were implemented to improve quality of care and patient outcomes. This study assessed the relationship between EHR-adoption and patient outcomes.
We performed an observational study using State Inpatient Databases linked to American Hospital Association survey, 2011. Surgical and medical patients from 6 large, diverse states were included. We performed univariate analyses and developed hierarchical regression models relating level of EHR utilization and mortality, readmission rates, and complications. We evaluated the effect of EHR adoption on outcomes in a difference-in-differences analysis, 2008 to 2011.
Medical and surgical patients sought care at hospitals reporting no EHR (3.5%), partial EHR (55.2%), and full EHR systems (41.3%). In univariate analyses, patients at hospitals with full EHR had the lowest rates of inpatient mortality, readmissions, and Patient Safety Indicators followed by patients at hospitals with partial EHR and then patients at hospitals with no EHR ( P < 0 .05). However, these associations were not robust when accounting for other patient and hospital factors, and adoption of an EHR system was not associated with improved patient outcomes ( P > 0 .05).
These results indicate that patients receiving medical and surgical care at hospitals with no EHR system have similar outcomes compared to patients seeking care at hospitals with a full EHR system, after controlling for important confounders.
To date, we have not yet seen the promised benefits of EHR systems on patient outcomes in the inpatient setting. EHRs may play a smaller role than expected in patient outcomes and overall quality of care.
INTRODUCTION
It is thought that health information technology, particularly electronic health records (EHR), will improve quality and efficiency of healthcare organizations, from small practices to large groups. 1 Given these potential benefits, the federal government encouraged EHR adoption under the Health Information Technology for Economic and Clinical Health (HITECH) Act. In response, many hospitals are striving to adopt these systems and demonstrate meaningful use. In 2013, 59% of US hospitals had some type of EHR system. 2 The federal incentive program defined 3 stages for timely adoption of EHR use: stage 1 is EHR adoption, stage 2 is EHR data exchange, and stage 3 is using EHRs to improve patient outcomes. 3 However, despite the widespread adoption of EHR systems, only about 6% of hospitals met all criteria of stage 2 meaningful use. 2 – 4 Thus, implementing and developing meaningful use for EHRs is still an ongoing process in the American healthcare system.
Electronic health records were originally built for billing purposes, not for research and quality improvement efforts. 5 Accordingly, the impact of EHRs on quality healthcare delivery has focused on physician performance and billing precision. 6 EHR studies often concentrate on process quality metrics, analyzing physician-level variability, and guideline compliance, rather than overall quality improvement or patient outcomes. 7 – 11 Some have suggested that EHRs have the potential to decrease medical errors by providing improved access to necessary information, better communication and integration of care between different providers and visits, and more efficient documentation and monitoring. 12 Many have used EHRs to decrease prescribing errors by providing real time clinical decision support. 13 – 16 Other recent studies have begun to use EHRs to track and monitor adverse patient outcomes such as catheter-associated urinary tract infections, deep vein thrombosis, or pulmonary embolism, providing critical data to improve patient safety outcomes. 17 , 18
Therefore, while several studies have looked at changes in quality attributed to electronic healthcare systems, overall improvements in patient outcomes associated with EHR implementation are still not yet well documented. In particular, the effect of the implementation of an EHR system on inpatient adverse events, inpatient mortality and 30-day all cause readmission for specific surgical, and medical conditions has yet to be explored. We thus sought to determine the association of hospital level-EHR systems with important patient outcomes.
The objective of this study was to determine whether hospitals with fully implemented EHR systems had better patient outcomes compared to hospitals with partial or no implemented EHR system after controlling for other important patient and hospital characteristics. Our study provides new information about the relationship between the implementation of an EHR system and the quality of healthcare delivered in the inpatient setting.
Data Source
We utilized discharge data from the 2011 State Inpatient Databases (SID), Healthcare Cost and Utilization Project (HCUP), Agency for Healthcare Research and Quality from Arkansas, California, Florida, Massachusetts, Mississippi, and New York. 19 SID is an all-capture state database that allows linkage of patients overtime and contains information on patient characteristics, primary and secondary diagnoses, and procedures received. The SID database was linked to the 2011 American Hospital Association (AHA) annual survey database, which contains information on EHR utilization in different hospitals along with other important hospital characteristics. 20
Patient safety indicators (PSI) are based on ICD-9-CM codes and Medicare severity diagnosis-related groups (DRGs), with specific inclusion and exclusion criteria determined by the Agency for Healthcare Research and Quality (AHRQ). 21 Using the PSI software (version 4.5), 22 we identified these adverse events in our dataset. Each PSI includes a unique denominator, numerator, and set of risk adjustors. 23 , 24
Study Population
Both surgical and medical patients from several diagnostic categories were included in the study. Specifically, surgical patients undergoing pulmonary lobectomy, open abdominal aortic aneurysm repair, endovascular abdominal aortic aneurysm repair, or colectomy were included. Medical patients receiving care for acute myocardial infarction, congestive heart failure, or pneumonia were included. These categories were chosen based on their frequency, contribution to patient comorbidities, and prevalence in the medical literature.
Outcome of Interest
Our main outcomes of interest were inpatient mortality, 30-day all cause readmission rates, PSIs, and length of stay.
Statistical Analysis
We utilized univariate regression analysis to develop descriptive statistics. A hierarchical regression model relating level of EHR utilization and quality of care was developed. The independent variables were level of EHR utilization (no EHR, partial EHR, or full EHR), patient demographics, comorbidities, and medical or surgical group. The dependent variables were mortality, readmissions, and complications (measured by PSIs). Relative-risk difference in differences analyses (DiD) were used to determine the effect of implementing an EHR system on quality of care. 25 The difference-in-differences analyses combined pre–post and treatment–control comparisons to eliminate some types of potential confounding. To do so, the hospitals lacking EHR systems in 2008 were selected and were then split into 3 groups: those that gained full EHRs by 2011 (treatment 1), those that gained only partial EHRs by 2011 (treatment 2), and those that still had no EHRs in 2011 (control). Direct comparison of these groups might be biased by confounders: there must be reasons why some of the hospitals adopted EHRs while others did not, and those reasons might also impact the outcomes of interest, both before and after adoption. Therefore, each hospital's event rates in 2008 are compared with the same hospital's rates in 2011, and the changes in those rates are then used to compare each treatment (i.e., EHR adoption level) with control (no EHR adoption). This analysis assumes that, in the absence of the intervention, the groups would have parallel trends and common external shocks. The use of a modified-Poisson model in these analyses enables estimation of risk ratios rather than odds ratios. 26 All statistical analyses were performed using STATA version 13.0, except for the difference-in-differences analyses, which were performed using both STATA and SAS version 9.3. 27
Patient characteristics are described in Tables Tables1 1 and and2. 2 . A total of 137,162 surgical patients and 311,605 medical patients were included. Medical and surgical patients sought care at hospitals reporting no EHR (3.5%), partial EHR (55.2%), and full EHR (41.3%). Of the surgical patients, 2.7% were treated in a hospital with no EHR, 55.9% were treated in a hospital with partial EHR, and 41.4% were treated in a hospital with full EHR. Of the medical patients, 3.9% were treated in a hospital with no EHR, 55.0% of patients were treated in a hospital with partial EHR, and 41.2% were treated in hospitals with full EHR.
Surgical Patient Demographics by EHR Status, 2011

Medical Patient Demographics by EHR Status, 2011

In the cross-sectional analyses, surgical patients treated at hospitals with full EHR had higher mortality rates (1.6%) than patients treated at hospitals with partial EHR (1.4%) or at hospitals with no EHR (1.6%) ( P = 0.0086). Surgical patients treated at hospitals with full EHR had higher readmission rates (11.9%) than patients treated at hospitals with partial EHR (11.2%) but lower readmission rates than patients treated at hospitals with no EHR (12.6%) ( P = 0.0005). Surgical patients treated at hospitals with full EHR had higher rates of complications measured by PSIs (3.7%) than patients treated at hospitals with partial EHR (3.0%) or no EHR (3.2%) ( P < 0.0001). Surgical patients treated at hospitals with full EHR had a shorter length of stay (LOS), measured in days (6.38) than patients treated at hospitals with partial EHR (6.85) or no EHR (7.89) ( P < 0.0001) (Table (Table3 3 ).
Cross-Sectional Univariate Analysis Surgical Patient Outcomes by EHR Status, 2011

Medical patients treated at hospitals with full EHR had a lower mortality rate (3.7%) than patients treated at hospitals with partial EHR (4.0%) or no EHR (4.4%) ( P < 0.0001). Medical patients treated at hospitals with full EHR did not have a statistically significant different readmission rate (19.4%) compared to patients treated at hospitals with partial EHR (19.6%) or no EHR (20.3%) ( P = 0.0548). Medical patients treated at hospitals with full EHR did not have a statistically significant difference in complications measured by PSIs (0.9%) compared to patients treated at hospitals with partial EHR (0.9%) or no EHR (0.8%) ( P = 0.196). Patients treated at hospitals with full EHR had a shorter length of stay (5.02) than patients treated at hospitals with partial EHR (5.28) or no EHR (5.76) ( P < 0.0001) (Table (Table4 4 ).
Cross-Sectional Univariate Analysis Medical Patient Outcomes by EHR Status, 2011

In the multiple regression analysis, there was no statistically significant difference in mortality rate among surgical patients treated at hospitals with full versus no EHR (odds ratio [OR] 1.24, P = 0.1442) or partial versus no EHR (OR 1.24, P = 0.1788) after controlling for important patient and hospital characteristics. There was no statistically significant difference in readmission rates among surgical patients treated at hospitals with full versus no EHR (OR 1.04, P = 0.5605) or partial versus no EHR (OR 1.04, P = 0.5158). There was a significant difference between rates of any complication measured by PSIs among surgical patients treated at hospitals with full versus no EHR (OR 1.22, P = 0.0452) but not between patients treated at hospitals with partial versus no EHR (OR 1.12, P = 0.2679).
There was no statistically significant difference between mortality rates among medical patients treated at hospitals with full EHR versus no EHR (OR 0.97, P = 0.4609) or partial versus no EHR (OR 1.01, P = 0.9375). There was no statistically significant difference between readmission rates among medical patients treated at hospitals with full versus no EHR (OR 0.97, P = 0.2834) or partial versus no EHR (OR 1.00, P = 0.8697). There was no statistically significant difference in complications measured by PSIs among medical patients treated at hospitals with full versus no EHR (OR 1.06, P = 0.6157) or partial versus no EHR (OR 1.13, P = 0.2516) (Table (Table5 5 ).
Association Between Patient Outcomes and EHR Implementation Status ∗

The difference-in-differences analysis allowed us to estimate the effect of implementing an EHR system on patient outcomes, assuming that the parallel trends and common shocks assumptions are correct. These analyses found statistically significant evidence of an effect in only three cases (Table (Table6 6 ).
The Relative Risk ∗ of EHR-Adoption on Patient Outcomes, 2008 and 2011

There was evidence of reduced risk of PSIs for medical patients in hospitals that had partially implemented EHRs by 2011 compared to those that still lacked EHRs in 2011 (risk ratio 0.673, 95% confidence intervals [CI] [0.45, 1.00], P -value 0.0492). There was also evidence of reduced risk of PSIs for medical patients in hospitals with fully implemented EHRs by 2011 compared with hospitals with no EHRs in 2011 (risk ratio 0.591, CI [0.38, 0.92], P -value 0.0198. Finally there was evidence of reduce risk of inpatient mortality for surgical patients in hospitals with partially implemented EHRs compared with no EHRs (risk ratio 2.031, CI [1.09, 3.79], P -value 0.0259) (Table (Table6 6 ).
This study tested the association between level of EHR implementation in inpatient settings and patient outcomes across 6 large, diverse states for both medical and surgical care. These results provided a preliminary glimpse at EHR meaningful use. Cross-sectional analysis found significant differences in rates of mortality, readmission, and complications between patients at hospitals with full EHR or partial EHR compared to hospitals with no EHR. However, these differences did not hold when adjusted for patient and hospital factors. Furthermore, the effect of EHR adoption was not associated with improved patient outcomes (specifically inpatient mortality, readmissions, and complications). Although EHR systems are thought to improve quality of care, this study suggests that in their current form, EHRs have not begun to reach meaningful use targets and may have a smaller impact than expected on patient outcomes.
This study builds on multiple studies highlighting the limitations of EHR systems on improving quality of care. Although EHRs have been extremely helpful for billing and physician compliance measurements, direct improvement of important patient outcomes have yet to be seen. A possible reason for this is that EHRs thus far have largely served as a recording mechanism after a patient care intervention rather than as an effective checking mechanism during the actual execution phase of patient care interventions. It has also been shown that while basic EHRs are associated with gains in quality measures, less benefit is associated with implementing advanced EHRs, suggesting that initial adoption of EHRs may actually be counterproductive by adding additional complexity into clinical settings. 28 Additionally, such improvements have yet to be translated to improvements in mortality. 29 Lack of improvement in other patient outcome measures has also been demonstrated. For example, 1 study demonstrated that although EHRs were associated with better rates of cholesterol testing, this did not translate to improvements in patients’ actual cholesterol levels. 9 In another study of ambulatory diabetes care in clinics with and without EHRs, patients at EHR enabled clinics actually did worse in rates of meeting 2 year hemoglobin A1c, cholesterol, and blood pressure goals. 30 Furthermore, data suggest that EHR implementation may actually increase the amount of time spent by patients during clinic visits. 31 These studies suggest that EHRs, with their increased documentation requirements, can have unintended consequences, including clinic inefficacy. All of these studies highlight the complexity of quality of care. Accurate documentation and billing is easily obtainable with EHRs but improving recognition of clinical problems and changing provider practice is much more challenging.
Our study does have some limitations. This study relied on administrative data derived from billing claims data, which has limited information on patients’ treatment courses that can affect outcomes. Additionally, this study uses hospital survey data to identify the level or EHR adoption, which is prone to reporting errors. Furthermore, changes in quality of care after the implementation of EHRs may be attributable in part to non-EHR factors, which cannot be fully accounted for in our analysis.
This population-based study builds on existing literature to demonstrate that EHRs are not yet associated with gains in measures of inpatient mortality, readmissions, and PSIs. Results here suggest that differences in outcomes at hospitals with different levels of EHR utilization may be attributable to other patient and hospital factors rather than EHR utilization itself. As federal incentives encourage EHR adoption and hospitals strive for meaningful use, it will be important to further characterize the benefits received from EHRs.
Acknowledgements
The authors thank National Cancer Institute of the National Institutes of Health under Award Number R01CA183962 and Agency for Healthcare Research and Quality (grant number R01HS024096) for the support. The content is solely the responsibility of the authors and does not necessarily represent the official views of the National Institutes of Health and Agency for Healthcare Research and Quality.
Abbreviations: AHA = american hospital association, CI = confidence interval, DiD = difference-in-differences, DRG = diagnosis-related group, EHR = electronic health record, HCUP = health care utilization project, LOS = length of stay, OR = odds ratio, PSI = patient safety indicator, SID = state inpatient database.
THB conceived and designed the study, supervised and contributed to the data analysis, interpreted results, and made substantial revisions to the paper; DM contributed to the study design, conducted the data analysis, and contributed to revisions of the paper; SY drafted the paper and contributed to the interpretation of the data analysis; KM contributed to the interpretation of the data analysis and revisions of the paper; and CC contributed to the interpretation of the data analysis and revisions of the paper.
Preliminary findings from this paper were presented at the Academy Health Research Meeting, San Diego, June 8–10, 2014 and at AMIA Annual Symposium, San Francisco, November 14–18, 2015.
Funding Research reported in this publication was supported by the National Cancer Institute of the National Institutes of Health under Award Number R01CA183962. The content is solely the responsibility of the authors and does not necessarily represent the official views of the National Institutes of Health. This project was supported by grant number R01HS024096 from the Agency for Healthcare Research and Quality. The content is solely the responsibility of the authors and does not necessarily represent the official views of the Agency for Healthcare Research and Quality.
The authors have no conflicts of interest to disclose.
- Methodology
- Open access
- Published: 15 April 2024
Natural language processing (NLP) to facilitate abstract review in medical research: the application of BioBERT to exploring the 20-year use of NLP in medical research
- Safoora Masoumi ORCID: orcid.org/0000-0002-7343-1771 1 ,
- Hossein Amirkhani 2 ,
- Najmeh Sadeghian 3 &
- Saeid Shahraz 4
Systematic Reviews volume 13 , Article number: 107 ( 2024 ) Cite this article
Metrics details
Abstract review is a time and labor-consuming step in the systematic and scoping literature review in medicine. Text mining methods, typically natural language processing (NLP), may efficiently replace manual abstract screening. This study applies NLP to a deliberately selected literature review problem, the trend of using NLP in medical research, to demonstrate the performance of this automated abstract review model.
Scanning PubMed, Embase, PsycINFO, and CINAHL databases, we identified 22,294 with a final selection of 12,817 English abstracts published between 2000 and 2021. We invented a manual classification of medical fields, three variables, i.e., the context of use (COU), text source (TS), and primary research field (PRF). A training dataset was developed after reviewing 485 abstracts. We used a language model called Bidirectional Encoder Representations from Transformers to classify the abstracts. To evaluate the performance of the trained models, we report a micro f1-score and accuracy.
The trained models’ micro f1-score for classifying abstracts, into three variables were 77.35% for COU, 76.24% for TS, and 85.64% for PRF.
The average annual growth rate (AAGR) of the publications was 20.99% between 2000 and 2020 (72.01 articles (95% CI : 56.80–78.30) yearly increase), with 81.76% of the abstracts published between 2010 and 2020. Studies on neoplasms constituted 27.66% of the entire corpus with an AAGR of 42.41%, followed by studies on mental conditions ( AAGR = 39.28%). While electronic health or medical records comprised the highest proportion of text sources (57.12%), omics databases had the highest growth among all text sources with an AAGR of 65.08%. The most common NLP application was clinical decision support (25.45%).
Conclusions
BioBERT showed an acceptable performance in the abstract review. If future research shows the high performance of this language model, it can reliably replace manual abstract reviews.
Peer Review reports
The history of natural language processing (NLP) is relatively short, but it has seen rapid growth through multiple fundamental revolutions. Alan Turing invented a test in the 1950s to determine whether computers could think like humans [ 1 ]. NLP scientists then applied universal linguistic rules to textual data to understand it. During this time, Noam Chomsky’s universal theory of language dominated NLP scientists’ attention. Computer scientists replaced this linguistic approach with computational models based on statistical analysis [ 1 ]. Increasing computational power for analyzing a large amount of textual information has contributed to our current understanding of NLP and its applications due to the invention of machine learning methods, especially deep learning [ 1 , 2 , 3 ]. Our intelligent machines now need natural language processing (NLP) to decipher meanings from human languages. With the widespread availability of smart gadgets in everyone’s life, NLP has become even more advanced over the past two decades [ 4 , 5 ]. Machines cannot recognize phrases and expressions without NLP in spoken and written languages. Moreover, the enormous amount of unstructured data produced daily highlights the need for NLP to assist professionals in sorting out their information [ 2 , 4 ]. Evidence-based medicine relies on systematic literature reviews to answer specific questions from a large amount of textual data, which can be challenging and time-consuming [ 6 ].
Machine learning and natural language processing can speed up and improve the SLR. In this context, text classification and data extraction are two NLP-based strategies. Abstract screening is an essential application of text classification in literature reviews. Alternatively, data extraction identifies information about a particular variable of interest. NLP can, for example, help extract the number of individuals who participated in particular clinical trials [ 6 , 7 ]. BERT (Bidirectional Encoder Representations from Transformers) is a transformer-based machine learning model for language modeling that has demonstrated significant success in various NLP tasks [ 8 ]. BioBERT, a BERT-based model pre-trained on biomedical texts, has outperformed other pre-trained language models in some biomedical datasets [ 9 ]. BioBERT has been highly performant in previous studies [ 10 , 11 , 12 , 13 ].
In this study, we deliberately analyze the evolution of medical NLP over the last two decades and benchmarked some of our findings against two similar studies published recently [ 14 , 15 ]. As an example of how NLP aids abstract review, we conducted an SLR using an automated method. Based on the results of SLR, a list of data sources used in medical NLP literature is provided, along with the type of NLP application and the related disease areas. We also show how the BioBERT model categorizes abstracts.
Developing training data
PubMed, Embase, PsycINFO, and CINAHL were searched using controlled vocabulary thesaurus (MesH in PubMed and Emtree in Embase) and free-text keywords. The search queries included “natural language processing” and “text mining.” Additional file 1 provides the full search queries. Also excluded were editorials, case reports, commentary, erratum, replies, and studies without abstracts. Before 2000, there were few NLP studies. Therefore, we included all abstracts published between January 2000 and December 2020. Multiple steps are involved in the study. First, we classified NLP abstracts based on their text source (e.g., social media versus clinical notes). After optimizing retrievable meaningful classes of abstracts, a finalized training dataset was created. Next, we calculated the classification accuracy of the computer algorithm using the entire corpus. As a final step, we applied the algorithm to obtain the classes and visualized them. The last author (S. S.) randomly selected 100 abstracts from PubMed and classified their text sources, the context of use (e.g., abstracts pertaining to clinical decision support vs. those related to NLP method development), and the type of medical conditions studied. Using these primary classes, the lead author (S. M.) and third author (N. S.) explored more classes and categories for each of these classes in further PubMed abstracts. By adding more abstracts, they continued to find more classes and subgroups until they were unable to find any more classes and subgroups. The saturation process was completed after reviewing 485 abstracts. All authors discussed and optimized the classification iteratively until they reached an agreement on the final classification. In Table 1 , the finalized classes and their definitions are described.
As depicted in Fig. 1 , machine learning algorithms were used to classify abstracts in the final corpus into those obtained from the trained dataset. By fine-tuning the pre-trained language models ubiquitous in modern NLP, we followed the favored approach. BERT, or Bidirectional Encoder Representations from Transformers, is a language model developed by Google [ 8 ]. The models are pre-trained on large corpora and then fine-tuned using task-specific training data by reusing the parameters from the pre-trained models. We used the BioBERT model [ 9 ] from the Hugging Face Transformers library [ 16 ], which was trained on abstracts from PubMed and full articles from PubMed Central. Then we fine-tuned three different models, one for each of our targets: text source, context of use, and primary research fields. The hyper-parameters, such as the learning rate and number of epochs, were selected using cross-validation. The final model was trained on the entire training data using the optimized hyperparameters. Since we utilized a pre-trained BioBERT model, a standard GPU, such as the Nvidia Tesla K80, was sufficient for fine-tuning the model during both the training and inference phases. All the experiments were conducted in a Google Colab environment leveraging this GPU.
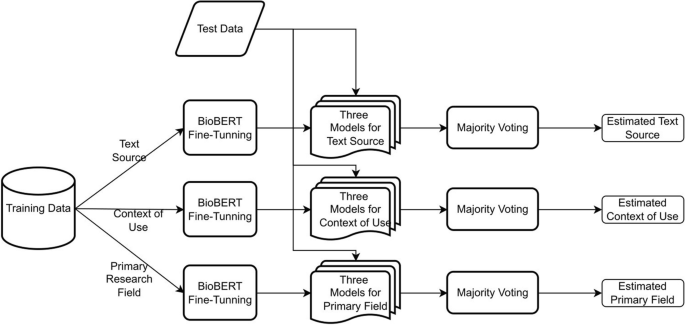
Overview of the proposed machine learning approach
For each target variable, we fine-tuned three different classifiers as an alternative method of improving the models’ accuracy. Repeating the fine-tuning process resulted in a different classifier due to different data batches used during training. The final prediction for an input article was obtained by majority voting of the base classifiers’ predictions. Afterwards, the trained models were applied to the entire corpus. A set of 362 randomly selected abstracts was manually annotated by the lead (S. M.), third (N. S.), and last author (S. S.) to evaluate the labels provided by the trained models. Next, the human annotations were compared to those provided by the models. The evaluation showed that the trained models’ accuracy in classifying abstracts into the text source, the context of use, and the primary research field was sufficient, mainly to track the time trends of the classes. Therefore, we assumed that misclassifications would remain constant over time. Our next step was to fit models that indicated publication growth rates for different study subgroups using ordinary least-squares regression. Citations were the dependent variable, and publication year was the predictor. Per year, the coefficient of the predictor showed an average increase in citations. A squared term for the publication year was added to the primary model to determine if the growth was linear or exponential. The increase in R 2 indicated logarithmic growth. The average annual growth rate (AAGR) was calculated by averaging all annual growth rates (AGR) over the study period (sum of AGRs/number of periods). We calculated AGR as the difference between the current year’s value and the past year’s value divided by the past year’s value.
We report a micro f1-score to evaluate the trained models. The f1-score is calculated as the harmonic mean of precision and recall for the positive class in a binary classification problem.
True positive (TP) and true negative (TN) are the numbers of samples correctly assigned to the positive and negative classes, respectively. On the other hand, false positive (FP) and false negative (FN) are the numbers of samples that are wrongly assigned to the positive and negative classes, respectively. Accuracy is the ratio of the samples correctly assigned to their respective classes.
Precision (P) and recall (R) are calculated as follows if TP, FP, and FN represent the number of true-positive, false-positive, and false-negative instances, respectively:
And f1-score will be as follows.
The average of the f1-scores obtained for different classes is computed for multiclass problems, such as ours. We report the weighted average considering the number of instances in each class in order to account for label imbalance.
Based on the evaluation, the trained models classified abstracts accurately into their text source, context, and primary research field (disease area) by 78.5%, 77.3%, and 87.6%, respectively. Accordingly, the trained models’ micro f1-scores for classifying abstracts into their text source, context of use, and primary research field were 77.35%, 76.24%, and 85.64%, respectively. We retrieved 22,294 English abstracts from the database. There were 12,817 references left after removing 8815 duplicates, 500 articles without abstracts, 32 errata, 31 commentaries, 31 editorials, and 68 veterinary-related abstracts. The selected analyses were based on 12,161 abstracts, excluding those published in 2021. Figure 2 illustrates the abstract selection process for creating the final abstract collection. NLP publications have increased logarithmically since 2000, as shown in Fig. 3 .
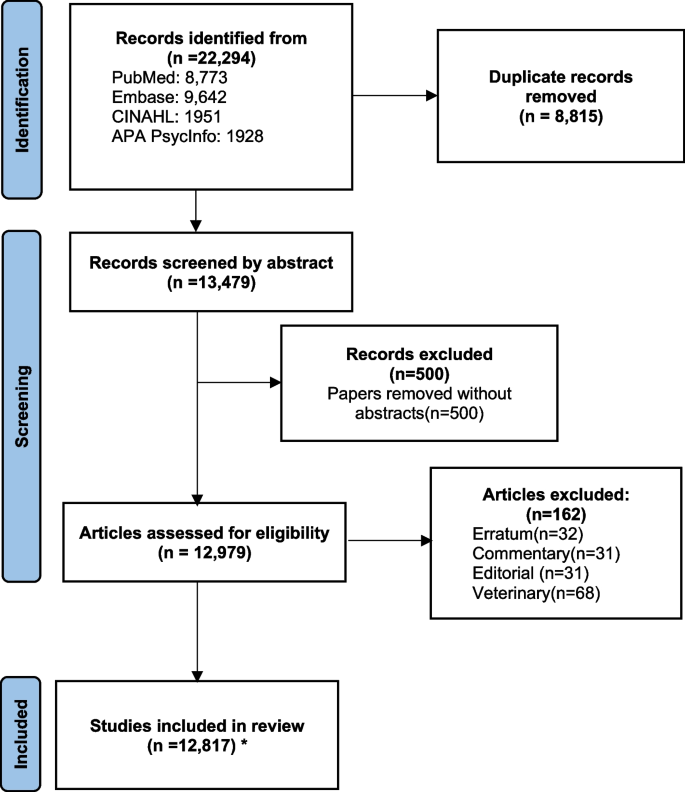
PRISMA flowchart illustrating the steps of abstract selection for building the final corpus. *For most analyses, we excluded abstracts for the year 2021, leaving 12,161 abstracts in the analysis data
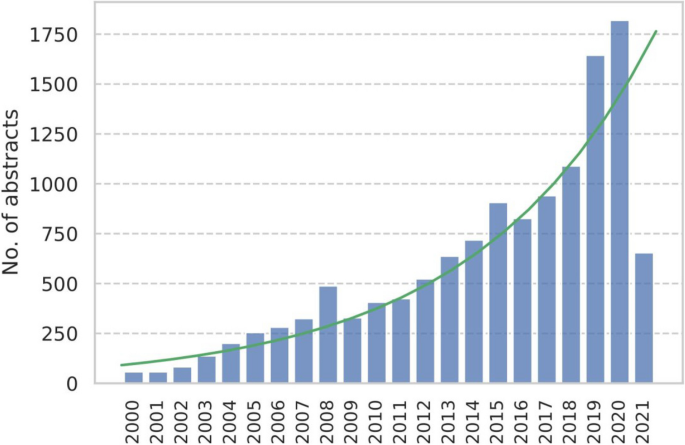
Trend analysis of 12,817 abstracts showing the overall trend of the growth and the number of articles per year
The Additional file 2 conveys the total number of abstracts retrieved for each subgroup. Table 2 shows the AAGR and average growth slope (coefficient) with a 95% confidence interval. It also displays the adjusted R2 of the regression model with and without a squared term for the publication year. The AAGR was 20.99%, with an average increase of 72 (95% CI : 56.80–78.30) publications per year. According to the adjusted R2 of 83%, the publication number is strongly affected by time. After adding a squared term for publication year, the indicator increased to 93%, indicating logarithmic growth. In all types of NLP text sources, electronic medical or health records or similar electronic clinical notes accounted for the highest percentage (57.12%). The addition of published articles and other sources of medical evidence accounted for 33.84% of all NLP text sources. Social media, including websites and databases with omics data (e.g., genomics), accounted for less than 10% of all NLP text sources (Table 2 ). Figure 4 displays the relative proportions and growth trends of four specific subgroups of text sources since the year 2000. Additionally, it presents the percentage representation of these chosen subgroups within the total for the “context of use” of the text sources. Despite comprising only 4.91% of publications, the so-called omics text data exhibited the fastest growth ( AAGR = 65.08%) among all other text sources.

Proportion and growth of four selected subgroups of text source since the year 2000 and percentage of selected subgroups of the “context of use” of the total for the subgroups of text source
Changes in the dominant primary research fields since 2000, along with the expansion rates, as well as the distribution percentages for specific subcategories within “context of use” and “text source,” are illustrated in Fig. 5 . Four medical fields accounted for slightly over 65% of all the research NLP researchers conducted and published (neoplasms, mental conditions, infectious diseases, and circulatory diseases). Neoplasms topped this list. The growth rates of all these medical fields were comparable (Table 2 and Fig. 5 ). NLP methods for clinical decision support were the most notable identifiable application among different aims (called “context of use”) of NLP studies, accounting for 25.45% of all publications. In contrast, bioinformatics-related discoveries showed the highest growth ( AAGR = 69.65%) among all medical NLP applications, in line with the highest growth of omics databases. Among the subgroups under “context of use,” the majority belonged to “other medical fields,” which included a wide range of medical applications. Changes in the “context of use” since the year 2000, including its proportion and growth, along with the percentage representation of specific subgroups within the “text source,” are depicted in Fig. 6 (Table 2 and Fig. 6 ).
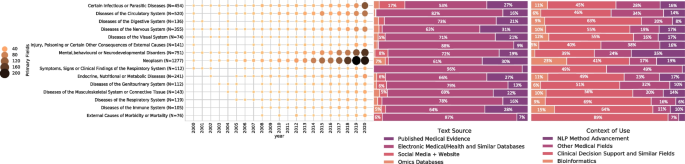
Proportion and growth of the most prevalent primary research fields since the year 2000 and the percentage of selected subgroups of the “context of use” and the “text source” of the total for the subgroups of the primary research field

Proportion and growth of the “context of use” since the year 2000 and the percentage of selected subgroups of the “text source” of the total for the subgroups of the “context of use”
According to Fig. 5 , clinical decision support applications and electronic medical/health records had the highest proportion of context of use and text source for each subgroup of primary research fields. The proportion of text source and context of use subtypes varied significantly across medical fields. For instance, published papers on NLP method advancement accounted for the highest percentage (35%) of ICD-11 codes for mental, behavioral, and neurodevelopmental disorders. Similarly, social media was used more frequently (17%) in certain infectious or parasitic diseases than in any diseases designated by ICD-11 codes.
Yu Zhu et al. [ 13 ] used output-modified BioBERT pre-trained with PubMed and PMC and obtained an f-score of 80.9, like ours. Elangovan et al. [ 11 ] found a lower f-score in a similar study. The other two systematic reviews observed a similar upward trend in using NLP in various medical fields over the last two decades [ 14 , 15 ]. In 2000, medical NLP publications began to appear prominently in peer-reviewed journals. This study shows BioBERT can spot an expected result reported in previous studies.
We were particularly interested in the type of text sources used in medial NLP, the type of medical conditions studied, and the motivation behind performing NLP. Three published bibliographic studies shared some features with ours. Using PubMed data, Chen et al. examined 1405 papers over 10 years (2007–2016) and reported country-region, author-affiliation, and thematic NLP research areas [ 14 ]. Using PubMed data from 1999 to 2018, Wang et al. identified 3498 publications. Additionally, country-regions, author affiliations, disease areas, and text sources were reported [ 15 ]. Similar to Wang [ 15 ] and Chen [ 14 ], Chen et al. [ 17 ] used NLP methods to explore a similar set of variables; however, the authors focused only on NLP-enhanced clinical trial research. PubMed, Web of Science, and Scopus were searched for 451 published articles from 2001 to 2018. We selected 12,817 peer-reviewed citations using a different approach than typical bibliographic methods. We systematically scanned four chief article datasets and manually classified citations based on three variables: primary research fields, text source used, and motivation for NLP (context of use). In addition, we used BioBERT of Google as a preferred NLP method to assign subgroups to our variables.
Unlike typical bibliometric research, we were not interested in regional or institutional distributions, typical features of bibliometric research. Instead, we explored the hows and whys of medical NLP research over the past two decades.
According to our results, annual medical NLP publications grew by roughly 21% between 2000 and 2020 on average, similar to the nearly 18% growth. Chen et al. reported between 2007 and 2016 [ 14 ]. According to Wang et al. [ 15 ] and Chen et al. [ 17 ], medical NLP publications increased rapidly between 1999 and 2017. The logarithmic progression of the citations in our study can partly be explained by the annual increase of over 65% in NLP studies using omics datasets. Nearly 27% of all NLP research was conducted on neoplasms, mental conditions, infectious diseases, and circulatory diseases. Similarly, Wang et al. retrieved around 25% of their citations from neoplasms [ 15 ]. Previous authors have not explained why medical NLP citations are unequally high in cancer and a limited number of other fields, like mental health. The same is valid for why particular medical conditions are at the center of medical community researchers, while EHR (electronic health records) or EMR (electronic medical records) massive data must be equally available for all medical conditions proportional to their prevalence. In the case of cancers and infectious lung disease, however, unstructured text may convey more information because of pathology or radiology reports. We can potentially apply medical NLP to broader clinical and research settings by studying the systematic differences across medical conditions from an NLP standpoint.
There are strengths and weaknesses to our approach. We began by categorizing medical conditions hierarchically using a systemic strategy. To identify primary research fields, we used ICD-11’s top-level taxonomy. In the future, if NLP studies follow the same procedure, the findings will remain comparable. We chose the BioBERT model from various pre-trained language models, including ClinicalBERT and BlueBERT. BioBERT can train with 4.5 billion biomedical words from PubMed abstracts and 13.5 billion words from PMC full-text articles. Compared to similar BERT models, NLP researchers are more involved with BioBERT. Hence, we recommend comparing the performance of various BERT models before selecting a model if an NLP specialist is not confident enough to choose the proper model. Finally, we publish the method for developing the analysis database (NLP corpus) based on medical systematic review guidelines. Future research can use this approach to confirm whether NLP can replace systematic literature reviews.
A potential shortcoming of our study was the idiosyncratic nature of the initial classification used for training the machine. Using our experience with observational datasets, such as electronic clinical notes and NLP applications, to analyze unstructured clinical data, we began building the initial subgroups. Nevertheless, to mitigate the risk of bias, we dissected the published studies cumulatively until more studies could not update the evolving classification. Our models’ estimated classification accuracy may have been adequate because of this strategy. The model can be fine-tuned based on more annotated articles, the hyper-parameters can be tuned more thoroughly, and multi-task learning can be explored instead of training separate models for each task. Additionally, the accuracy may improve further after the training dataset is expanded. Finally, we only included abstracts written in English. Results and conclusions may be influenced if relevant research published in languages other than English is excluded.
This study aimed to evaluate the performance of BioBERT as a tool to substitute manual abstract review using a language model. BioBERT is an acceptable method for abstract selection for systematic literature searches since it uses a uniform and human-independent algorithm that reduces the time required for manual abstract selection and increases inter-study reliability.
Availability of data and materials
Data sharing is not applicable, but the authors are happy to share the abstracts publicly if that helps the reviewers or readers.
Abbreviations
Natural language processing
Average annual growth rate
Electronic health records
Electronic medical records
International Classification of Diseases
Bidirectional Encoder Representations from Transformers
Johri P, Khatri S, Taani A, Sabharwal M, Suvanov S, Kumar A, editors. Natural language processing: history, evolution, application, and future work. 3rd International Conference on Computing Informatics and Networks; 2021. p.365–75.
Zhou M, Duan N, Liu S, Shum H. Progress in neural NLP: modeling, learning, and reasoning. Engineering. 2020;6(3):275–90.
Article Google Scholar
Jones KS. Natural language processing: a historical review. In: Zampolli A, Calzolari N, Palmer M, editors. Current Issues in Computational Linguistics: In Honour of Don Walker. Dordrecht: Springer; 1994. p. 3–16.
Locke S, Bashall A, Al-Adely S, Moore J, Wilson A, Kitchen G. Natural language processing in medicine: a review. Trends Anaesthesia Crit Care. 2021;38:4–9.
Manaris B. Natural language processing: a human-computer interaction perspective. Adv Comput. 1998;47:1–66.
Marshall IJ, Wallace BC. Toward systematic review automation: a practical guide to using machine learning tools in research synthesis. Syst Rev. 2019;8(1):163.
Article PubMed PubMed Central Google Scholar
Kim SN, Martinez D, Cavedon L, Yencken L. Automatic classification of sentences to support evidence based medicine. BMC Bioinformatics. 2011;12(2):S5.
Devlin J, Chang M-W, Lee K, Toutanova K, editors. BERT: Pre-training of deep bidirectional transformers for language understanding. Conference of the North American Chapter of the Association for Computational Linguistics: Human Language Technologies, Volume 1 (Long and Short Papers); 2019. p. 4171–86.
Lee J, Yoon W, Kim S, Kim D, Kim S, So CH, et al. BioBERT: a pre-trained biomedical language representation model for biomedical text mining. Bioinformatics. 2020;36(4):1234–40.
Article CAS PubMed Google Scholar
Giorgi JM, Bader GD. Transfer learning for biomedical named entity recognition with neural networks. Bioinformatics. 2018;34(23):4087–94.
Article CAS PubMed PubMed Central Google Scholar
Elangovan A, Li Y, Pires DEV, Davis MJ, Verspoor K. Large-scale protein-protein post-translational modification extraction with distant supervision and confidence calibrated BioBERT. BMC Bioinformatics. 2022;23(1):4.
Ji Z, Wei Q, Xu H. BERT-based ranking for biomedical entity normalization. AMIA Jt Summits Transl Sci Proc. 2020;2020:269–77.
PubMed PubMed Central Google Scholar
Zhu Y, Li L, Lu H, Zhou A, Qin X. Extracting drug-drug interactions from texts with BioBERT and multiple entity-aware attentions. J Biomed Inform. 2020;106:103451.
Article PubMed Google Scholar
Chen X, Xie H, Wang FL, Liu Z, Xu J, Hao T. A bibliometric analysis of natural language processing in medical research. BMC Med Inform Decis Mak. 2018;18(1):1–14.
Wang J, Deng H, Liu B, Hu A, Liang J, Fan L, et al. Systematic evaluation of research progress on natural language processing in medicine over the past 20 years: bibliometric study on PubMed. J Med Internet Res. 2020;22(1):e16816.
Wolf T, Chaumond J, Debut L, Sanh V, Delangue C, Moi A, et al., editors. Transformers: state-of-the-art natural language processing. Conference on Empirical Methods in Natural Language Processing: System Demonstrations; 2020. p. 38–45
Chen X, Xie H, Cheng G, Poon LK, Leng M, Wang FL. Trends and features of the applications of natural language processing techniques for clinical trials text analysis. Appl Sci. 2020;10(6):2157.
Article CAS Google Scholar
Download references
Acknowledgements
Not applicable
Not applicable.
Author information
Authors and affiliations.
Pediatric Infectious Diseases Research Center, Mazandaran University of Medical Sciences, Sari, Iran
Safoora Masoumi
Computer and Information Technology Department, University of Qom, Qom, Iran
Hossein Amirkhani
Student Research Committee, Mazandaran University of Medical Sciences, Sari, Iran
Najmeh Sadeghian
Institute for Clinical Research and Health Policy Studies, Tufts Medical Center, Boston, USA
Saeid Shahraz
You can also search for this author in PubMed Google Scholar
Contributions
SM drafted the manuscript, helped conduct the analysis, and contributed to the design of the study and data collection. HA conducted the analysis and contributed to the design of the study. NS contributed to data collection and critical review of the drafts. SSH designed the study, supervised the integrity and accuracy of the outputs, and critically reviewed the draft and revised it iteratively.
Corresponding author
Correspondence to Safoora Masoumi .
Ethics declarations
Ethics approval and consent to participate, consent for publication, competing interests.
The authors declare that they have no competing interests.
Additional information
Publisher’s note.
Springer Nature remains neutral with regard to jurisdictional claims in published maps and institutional affiliations.
Supplementary Information
Additional file 1.
: Appendix 1 . The three tables (a, b, and c) show the absolute number of abstracts retrieved between 2000 and 2020 (inclusive) for each of the three classes studied.
Additional file 2
: Appendix 2 . The search strategy used to received abstracts from four databases.
Rights and permissions
Open Access This article is licensed under a Creative Commons Attribution 4.0 International License, which permits use, sharing, adaptation, distribution and reproduction in any medium or format, as long as you give appropriate credit to the original author(s) and the source, provide a link to the Creative Commons licence, and indicate if changes were made. The images or other third party material in this article are included in the article's Creative Commons licence, unless indicated otherwise in a credit line to the material. If material is not included in the article's Creative Commons licence and your intended use is not permitted by statutory regulation or exceeds the permitted use, you will need to obtain permission directly from the copyright holder. To view a copy of this licence, visit http://creativecommons.org/licenses/by/4.0/ . The Creative Commons Public Domain Dedication waiver ( http://creativecommons.org/publicdomain/zero/1.0/ ) applies to the data made available in this article, unless otherwise stated in a credit line to the data.
Reprints and permissions
About this article
Cite this article.
Masoumi, S., Amirkhani, H., Sadeghian, N. et al. Natural language processing (NLP) to facilitate abstract review in medical research: the application of BioBERT to exploring the 20-year use of NLP in medical research. Syst Rev 13 , 107 (2024). https://doi.org/10.1186/s13643-024-02470-y
Download citation
Received : 23 July 2022
Accepted : 28 January 2024
Published : 15 April 2024
DOI : https://doi.org/10.1186/s13643-024-02470-y
Share this article
Anyone you share the following link with will be able to read this content:
Sorry, a shareable link is not currently available for this article.
Provided by the Springer Nature SharedIt content-sharing initiative
- Natural language processing (NLP)
- Trend analysis
- Machine learning
Systematic Reviews
ISSN: 2046-4053
- Submission enquiries: Access here and click Contact Us
- General enquiries: [email protected]
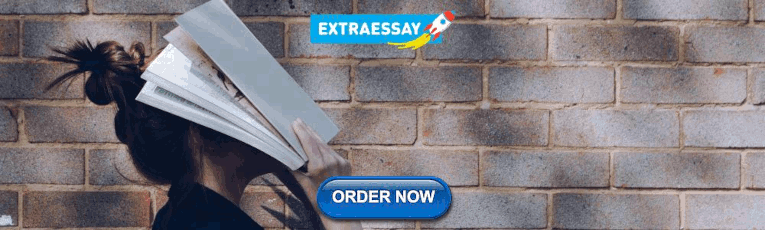
IMAGES
VIDEO
COMMENTS
Although the benefits of EHR are well-received and Health Information Technology for Economic and Clinical Health (HITECH) Act encourages the use of EHR to improve care quality and efficiency, prior studies show mixed results of implementing EHR. 3 Recent studies suggest that full adoption of EHR might not be sufficient to ensure the benefits of EHRs; instead, meaningful use 4 or meaningful ...
1. Introduction. In the early 1990s, a trend in the shift from paper-based health records to electronic records started; this was in response to advances in technology as well as the advocacy of the Institute of Medicine in the United States [1,2].As a result of the inadequacies of paper-based health records gradually becoming evident to the healthcare industry [], electronic records have ...
The proliferation of electronic health records (EHRs) spurred on by federal government incentives over the past few decades has resulted in greater than an 80% adoption-rate at hospitals [] and close to 90% in office-based practices [] in the United States.A natural consequence of the availability of electronic health data is the conduct of research with these data, both observational and ...
Abstract. Electronic health records (EHRs) provide opportunities to enhance patient care, embed performance measures in clinical practice, and facilitate clinical research. Concerns have been raised about the increasing recruitment challenges in trials, burdensome and obtrusive data collection, and uncertain generalizability of the results.
Abstract. Implementing an electronic health record (EHR) can be a difficult task to take on and planning the process is of utmost importance to minimize errors. Evaluating the selection criteria and implementation plan of an EHR system, intending interoperability, confidentiality, availability, and integrity of the patient health information ...
et. al., 2023, Gopal, et. al., 2019, Kumar, M., & Mos tafa, 2020). The purpose of this review is to provide a comprehensive overvi ew of the role of EHRs in modern healthcare and their. impact on ...
In the last decade, open-source Electronic Health Record systems (OS-EHRs) have gained notable recognition due to several factors, like, availability without financial barriers, wider compatibility, user-friendliness, and unrestricted modification and restructuring . 11,12 Open-source software in healthcare has also been quite successful as it provides development flexibility, involving users ...
Abstract. Background: The increased use of electronic health records (EHRs) has resulted in new opportunities for research, but also raises concerns regarding privacy, confidentiality, and patient awareness.Because public trust is essential to the success of the research enterprise, patient perspectives are essential to the development and implementation of ethical approaches to the research ...
Background One of the main objectives of Electronic Health Records (EHRs) is to enhance collaboration among healthcare professionals. However, our knowledge of how EHRs actually affect collaborative practices is limited. This study examines how an EHR facilitates and constrains collaboration in five outpatient clinics. Methods We conducted an embedded case study at five outpatient clinics of a ...
This Viewpoint posits suggestions to reform electronic health records (EHRs), including use of unique personal safety identifiers, reduction of administrative and regulatory content from clinical time, inclusion of patient-entered information into the EHR, and reinvention of the clinical note.
The use of electronic patient records for medical research: Conflicts and contradictions. BMC Health Services Research 15 (1): 124. Article Google Scholar Stevenson, F., N. Lloyd, L. Harrington, and P. Wallace. 2012. Use of electronic patient records for research: Views of patients and staff in general practice.
Health care innovations can influence patient care, and enhancements in health information technology have avowed to improve patient safety and reduce medical errors. 1 Studies to improve safety and decrease medical errors have been identified as research priorities by the National Academy of Medicine since publishing its report on building a safer health system in 1999. 2 Electronic health ...
The adoption of electronic health records will provide a rich resource for biomedical researchers. This Review discusses the potential for their use in informed decision making in the clinic, for ...
The widespread adoption and use of electronic health records (EHRs) [1, 2], together with the development of "big data" tools to mine, ... Spratt SE, Beskow LM. Research use of electronic health records: patients' perspectives on contact by researchers. J Am Med Inform Assoc. 2018;25(9):1122-9. Article PubMed PubMed Central ...
The interaction of physicians with electronic medical records (EMR) is the most relevant example of how our inventions have enslaved us. The focus is often on creating a perfect record on EMR, while patient interaction is relegated to the hazy periphery. ... and most importantly, data compilation for analysis and research, all with time ...
Electronic health records (EHRs) are now widely adopted in the United States, but health systems have barely begun using them to deliver high-value care. More directed and rigorous research is needed to fulfill the promise of EHRs to not only store information but also support the delivery of better care. This article describes 4 potential benefits of EHR-based research: improving clinical ...
Electronic health records (EHRs) provide opportunities to enhance patient care, embed performance measures in clinical practice, and facilitate clinical research. Concerns have been raised about the increasing recruitment challenges in trials, burdensome and obtrusive data collection, and uncertain generalizability of the results. Leveraging electronic health records to counterbalance these ...
This research was funded by the MIT Abdul Latif Jameel Clinic for Machine Learning in Health. MedKnowts, a "smart" electronic health record system, can help doctors work more efficiently by presenting relevant information from a patient's medical history, autocompleting medical terms as a clinician types, and auto-populating repetitive ...
Background The literature on implementing Electronic Health Records (EHR) in hospitals is very diverse. The objective of this study is to create an overview of the existing literature on EHR implementation in hospitals and to identify generally applicable findings and lessons for implementers. Methods A systematic literature review of empirical research on EHR implementation was conducted ...
The exact keyword "electronic health record" led to occurrences in all time and date segments. It appeared twice in the 1991-2000 period, six times during 2001-2010, 120 times in 2011-2020, and 05 times in the 2021-2021 period. The second most used keyword, "electronic medical record," also occurred for all the date segments.
Phenotyping is a core task in observational health research utilizing electronic health records (EHRs). Developing an accurate algorithm demands substantial input from domain experts, involving extensive literature review and evidence synthesis. This burdensome process limits scalability and delays knowledge discovery.
Objectives: Biases inherent in electronic health records (EHRs), and therefore in medical artificial intelligence (AI) models may significantly exacerbate health inequities and challenge the adoption of ethical and responsible AI in healthcare. Biases arise from multiple sources, some of which are not as documented in the literature. Biases are encoded in how the data has been collected and ...
Literature search based on "Electronic Health Record", "Medical Record", and "Medical Chart" using Medline, Google, Wikipedia Medical, and Cochrane Libraries resulted in an initial review of 2,356 abstracts and other information in papers and books. ... Scientific production of electronic health record research, 1991-2005. Comput ...
The need to classify disease and predict outcomes is as old as medicine itself. Nearly 50 years ago, the advantage of applying multivariable statistics to these problems became evident. 1 Since then, the increasing availability of databases containing often-complex clinical information from tens or even hundreds of millions of patients, combined with powerful statistical techniques and ...
Early Access to Electronic Health Records May Influence the Patient Experience. December 2023. ONCOLOGY 37 (12):477-478. DOI: 10.46883/2023.25921010. Authors: Mehmet Sitki Copur. Soe Min Tun. Adam ...
Public health agencies nationwide rely on timely and complete data from health care providers to detect infectious diseases and environmental hazards, helping to protect their communities from harm. But the electronic health records (EHRs) used by doctors' offices and health systems do not consistently send timely, granular, and complete data to these agencies.
Electronic health records: research into design and implementation - PMC. Journal List. Br J Gen Pract. v.61 (591); October 2011. PMC3177114. As a library, NLM provides access to scientific literature. Inclusion in an NLM database does not imply endorsement of, or agreement with, the contents by NLM or the National Institutes of Health.
Scientific knowledge, as measured by numbers of papers published, has been estimated to double every 17.3 years. However, it takes an average of about 17 years for health and medical research ...
Electronic health records (EHRs) were implemented to improve quality of care and patient outcomes. This study assessed the relationship between EHR-adoption and patient outcomes. We performed an observational study using State Inpatient Databases linked to American Hospital Association survey, 2011. Surgical and medical patients from 6 large ...
Abstract review is a time and labor-consuming step in the systematic and scoping literature review in medicine. Text mining methods, typically natural language processing (NLP), may efficiently replace manual abstract screening. This study applies NLP to a deliberately selected literature review problem, the trend of using NLP in medical research, to demonstrate the performance of this ...