
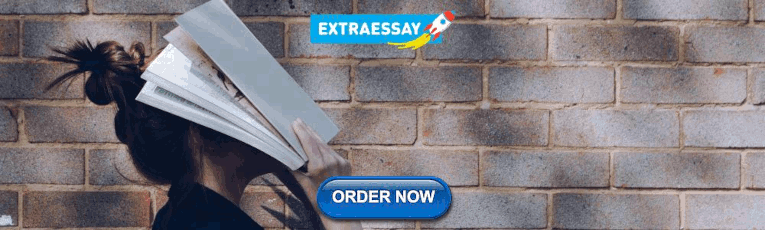
Critical Writing 101
Descriptive vs analytical vs critical writing.
By: Derek Jansen (MBA) | Expert Reviewed By: Dr Eunice Rautenbach | April 2017
Across the thousands of students we work with , descriptive writing (as opposed to critical or analytical writing) is an incredibly pervasive problem . In fact, it’s probably the biggest killer of marks in dissertations, theses and research papers . So, in this post, we’ll explain the difference between descriptive and analytical writing in straightforward terms, along with plenty of practical examples.
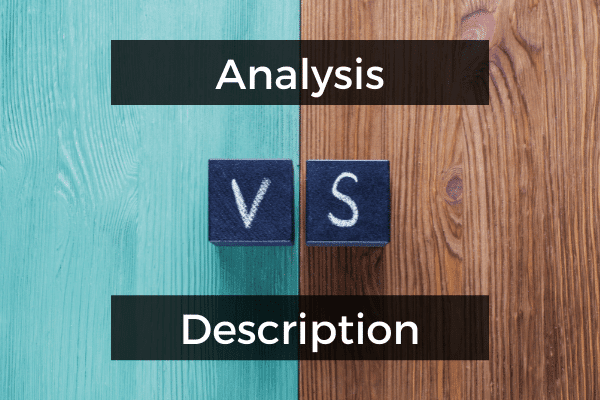
Descriptive vs Analytical Writing
Writing critically is one of the most important skills you’ll need to master for your academic journey, but what exactly does this mean?
Well, when it comes to writing, at least for academic purposes, there are two main types – descriptive writing and critical writing. Critical writing is also sometimes referred to as analytical writing, so we’ll use these two terms interchangeably.
To understand what constitutes critical (or analytical) writing, it’s useful to compare it against its opposite, descriptive writing. At the most basic level, descriptive writing merely communicates the “ what ”, “ where ”, “ when ” or “ who ”. In other words, it describes a thing, place, time or person. It doesn’t consider anything beyond that or explore the situation’s impact, importance or meaning. Here’s an example of a descriptive sentence:
“Yesterday, the president unexpectedly fired the minister of finance.”
As you can see, this sentence just states what happened, when it happened and who was involved. Classic descriptive writing.
Contrasted to this, critical writing takes things a step further and unveils the “ so what? ” – in other words, it explains the impact or consequence of a given situation. Let’s stick with the same event and look at an example of analytical writing:
“The president’s unexpected firing of the well-respected finance minister had an immediate negative impact on investor confidence. This led to a sharp decrease in the value of the local currency, especially against the US dollar. This devaluation means that all dollar-based imports are now expected to rise in cost, thereby raising the cost of living for citizens, and reducing disposable income.”
As you can see in this example, the descriptive version only tells us what happened (the president fired the finance minister), whereas the critical version goes on to discuss some of the impacts of the president’s actions.
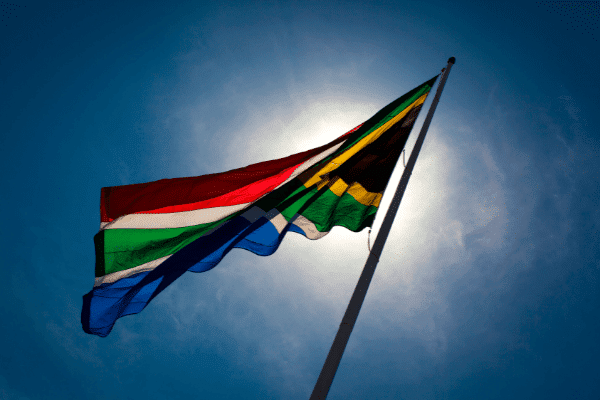
Ideally, critical writing should always link back to the broader objectives of the paper or project, explaining what each thing or event means in relation to those objectives. In a dissertation or thesis, this would involve linking the discussion back to the research aims, objectives and research questions – in other words, the golden thread .
Sounds a bit fluffy and conceptual? Let’s look at an example:
If your research aims involved understanding how the local environment impacts demand for specialty imported vegetables, you would need to explain how the devaluation of the local currency means that the imported vegetables would become more expensive relative to locally farmed options. This in turn would likely have a negative impact on sales, as consumers would turn to cheaper local alternatives.
As you can see, critical (or analytical) writing goes beyond just describing (that’s what descriptive writing covers) and instead focuses on the meaning of things, events or situations, especially in relation to the core research aims and questions.
Need a helping hand?
But wait, there’s more.
This “ what vs so what” distinction is important in understanding the difference between description and analysis, but it is not the only difference – the differences go deeper than this. The table below explains some other key differences between descriptive and analytical writing.
Should I avoid descriptive writing altogether?
Not quite. For the most part, you’ll need some descriptive writing to lay the foundation for the critical, analytical writing. In other words, you’ll usually need to state the “what” before you can discuss the “so what”. Therefore, description is simply unavoidable and in fact quite essential , but you do want to keep it to a minimum and focus your word count on the analytical side of things.
As you write, a good rule of thumb is to identify every what (in other words, every descriptive point you make) and then check whether it is accompanied by a so what (in other words, a critical conclusion regarding its meaning or impact).
Of course, this won’t always be necessary as some conclusions are fairly obvious and go without saying. But, this basic practice should help you minimise description, maximise analysis, and most importantly, earn you marks!
Let’s recap.
So, the key takeaways for this post are as follows:
- Descriptive writing focuses on the what , while critical/analytical writing focuses on the so what .
- Analytical writing should link the discussion back to the research aims, objectives or research questions (the golden thread).
- Some amount of description will always be needed, but aim to minimise description and maximise analysis to earn higher marks.
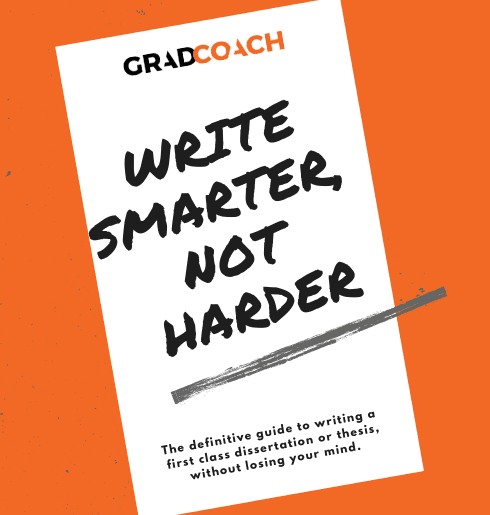
Psst… there’s more (for free)
This post is part of our dissertation mini-course, which covers everything you need to get started with your dissertation, thesis or research project.
You Might Also Like:
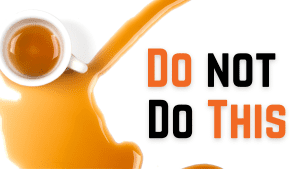
19 Comments
Thank you so much. This was helpful and a switch from the bad writing habits to the good habits.
Great to hear that, Sarah. Glad you found it useful!
I am currently working on my Masters Thesis and found this extremely informative and helpful. Thank you kindly.
I’m currently a University student and this is so helpful. Thank you.
It really helped me to get the exact meaning of analytical writing. Differences between the two explains it well
Thank you! this was very useful
With much appreciation, I say thank you. Your explanations are down to earth. It has been helpful.
Very helpful towards my theses journey! Many thanks 👍
very helpful
very helpful indeed
Thanks Derek for the useful coaching
Thank you for sharing this. I was stuck on descriptive now I can do my corrections. Thank you.
I was struggling to differentiate between descriptive and analytical writing. I googled and found this as it is so helpful. Thank you for sharing.
I am glad to see this differences of descriptive against analytical writing. This is going to improve my masters dissertation
Thanks in deed. It was helpful
Thank you so much. I’m now better informed
Busy with MBA in South Africa, this is very helpful as most of the writing requires one to expound on the topics. thanks for this, it’s a salvation from watching the blinking cursor for hours while figuring out what to write to hit the 5000 word target 😂
It’s been fantastic and enriching. Thanks a lot, GRAD COACH.
Wonderful explanation of descriptive vs analytic writing with examples. This is going to be greatly helpful for me as I am writing my thesis at the moment. Thank you Grad Coach. I follow your YouTube videos and subscribed and liked every time I watch one.
Very useful piece. thanks
Submit a Comment Cancel reply
Your email address will not be published. Required fields are marked *
Save my name, email, and website in this browser for the next time I comment.
- Print Friendly
- Translators
- Graphic Designers
- Editing Services
- Academic Editing Services
- Admissions Editing Services
- Admissions Essay Editing Services
- AI Content Editing Services
- APA Style Editing Services
- Application Essay Editing Services
- Book Editing Services
- Business Editing Services
- Capstone Paper Editing Services
- Children's Book Editing Services
- College Application Editing Services
- College Essay Editing Services
- Copy Editing Services
- Developmental Editing Services
- Dissertation Editing Services
- eBook Editing Services
- English Editing Services
- Horror Story Editing Services
- Legal Editing Services
- Line Editing Services
- Manuscript Editing Services
- MLA Style Editing Services
- Novel Editing Services
- Paper Editing Services
- Personal Statement Editing Services
- Research Paper Editing Services
- Résumé Editing Services
- Scientific Editing Services
- Short Story Editing Services
- Statement of Purpose Editing Services
- Substantive Editing Services
- Thesis Editing Services
Proofreading
- Proofreading Services
- Admissions Essay Proofreading Services
- Children's Book Proofreading Services
- Legal Proofreading Services
- Novel Proofreading Services
- Personal Statement Proofreading Services
- Research Proposal Proofreading Services
- Statement of Purpose Proofreading Services
Translation
- Translation Services
Graphic Design
- Graphic Design Services
- Dungeons & Dragons Design Services
- Sticker Design Services
- Writing Services
Please enter the email address you used for your account. Your sign in information will be sent to your email address after it has been verified.
Analytical vs. Descriptive Writing: Definitions and Examples
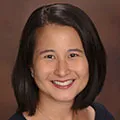
Scholars at all levels are expected to write. People who are not students or scholars often engage in writing for work, or to communicate with friends, family, and strangers through email, text messages, and social media. Academia recognizes two major types of writing—descriptive writing and analytical writing—which are both used in non-academic situations as well. As you might expect, descriptive writing focuses on clear descriptions of facts or things that have happened, while analytical writing provides additional analysis.
Descriptive writing is the most straightforward type of academic writing. It provides accurate information about "who", "what", "where", and "when". Examples of descriptive writing include:
- Summarizing an article (without offering additional insight)
- Stating the results of an experiment (without analyzing the implications)
- Describing a newsworthy event (without discussing possible long-term consequences)
High school students and undergraduates are most commonly asked to write descriptively, to show that they understand the key points of a specific topic (e.g. the major causes of World War II).
Analytical writing goes beyond summarizing information and instead provides evaluation, comparison, and possible conclusions. It addresses the questions of "why?", "so what?", and "what next?". Examples of analytical writing include:
- The discussion section of research papers
- Opinion pieces about the likely consequences of newsworthy events and the steps that should be taken in response.
High school students and undergraduates are sometimes asked to write analytically to "stretch their thinking". Possible topics might include "Could World War II have been avoided?" and "How can CRISPR-Cas9 technology improve human health?". The value of any such analysis is entirely dependent on the writer's ability to understand and clearly explain relevant information, which would be explained through descriptive writing. For graduate students and professional researchers, the quality of their work is at least partially based on the quality of their analysis.
The following table from The Study Skills Handbook by Stella Cottrell (2013, 4th edition, Palgrave Macmillan, page 198) is commonly used to summarize the differences between descriptive writing and analytical writing.
Description and analysis are also used in spoken communication such as presentations and conversations, and in visual communication such as diagrams and memes. In all of these cases, it is important to communicate clearly and effectively, and to use reliable sources of information.
Descriptive writing and analytical writing are often used in combination. In job application cover letters and essays for university admission, adding analytical text can provide context for otherwise unremarkable statements.
- Descriptive text: "I graduated from Bear University in 2020 with a B.S. in Chemistry and a cumulative GPA of 3.056."
- Analytical text: "While I struggled with some of my introductory courses, I proactively sought help to fill gaps in my understanding, and earned an "A" grade for all five of my senior year science courses. Therefore, I believe I am a strong candidate for . . ."
Combining description and analysis can also be very effective when discussing the significance of research results.
- Descriptive text: "Our study found significant (>2 ug/L) concentrations of polyfluoroalkyl substances (PFAS) in blood samples from all 5,478 study participants."
- Analytical text: "These results are alarming because the sample population included people who range in age from 1 month old to 98 years old, who live on five different continents, who reside in extremely rural areas and in urban areas, and who have little to no direct contact with products containing PFAS. PFAS are called "forever chemicals" because they are estimated to take hundreds or thousands of years to degrade. According to the US Centers for Disease Control (CDC), PFAS can move through soils to contaminate drinking water, and bioaccumulate in animals. Further research is urgently needed to better understand the adverse effects that PFAS have on human health, to identify the source of PFAS in rural communities, and to develop a method to sequester or destroy PFAS that have already entered the environment."
In both of the examples above, the analytical text includes additional facts (e.g. "A" grade for senior science courses; 1 month old to 98 years old) that help strengthen the argument. The student's transcript and the research paper's results section would contain these same facts—along with many others—written descriptively or presented in graphs, tables, or lists. For the analytical text, the author is trying to persuade the reader, and has therefore selected relevant facts to support their argument.
In the example about PFAS, the author's argument is further strengthened by citing additional information from a reputable source (the CDC). In reports where the author is supposed to be unbiased (e.g. a journalist writing descriptively), a similar effect can be obtained by quoting reputable sources. For example, "Professor of environmental science Kim Lee explains that PFAS are. . ." In these situations, it is often appropriate to present opposing views, as long as they come from reputable sources. This strategy of quoting or citing reputable sources can also be effective for students and professionals who do not have strong credentials in the topic under discussion.
Analytical writing supports a point of view
People cannot choose their own facts, but the same facts can be used to support very different points of view. Let's consider some different points of view that can be supported by the PFAS example from above.
- Scientific point of view: "Further research is urgently needed to better understand the adverse effects that PFAS have on human health, to identify the source of PFAS in rural communities, and to develop a method to sequester or destroy PFAS that have already entered the environment."
- Policy point of view: "Legislative action is urgently needed to ban the use of all PFAS, instead of banning new PFAS one at a time. Abundant and reliable data strongly indicates that all PFAS have similar effects, even if they have small differences in chemical composition. Given such evidence, the impetus must be on the chemical industry to prove safety, rather than on the general public to prove harm."
- Legal point of view: "Chemical companies have known about the danger of PFAS for years, but hid the evidence and continued to use these chemicals. Therefore, individuals and communities who have been harmed have the right to sue for damages."
These three points of view focus on three different fields (science, policy, and law), but all have a negative view of PFAS. The next example shows how the same factual information can be used to support opposing views.
- Descriptive text: " According to Data USA , the average fast food worker in 2019 was 26.1 years old, and earned a salary of $12,294 a year."
- Point of view #1: "These data show why raising the minimum wage is unnecessary. Most fast food workers are young, with many being teenagers who are making extra money while living with their parents. The majority will eventually transition to jobs that require more skills, and that are rewarded with higher pay. If we mandate that companies pay low-skill workers more than required by the free market, then more highly skilled workers will also demand a pay raise. This will hurt businesses, contribute to inflation, and have no net benefit."
- Point of view #2: "These data show why raising the minimum wage is so important. On average, for every 16-year-old working in fast food for extra money, there is a 36-year-old trying to make ends meet. As factory jobs have moved overseas, employees without specialized skills have turned to fast food for steady employment. According to the UC Berkeley Labor Center , for families with someone working full-time (40 hours/week) in fast food, more than half are enrolled in public assistance programs. These include Medicaid, food stamps, and the Earned Income Tax Credit. Therefore, taxpayers are subsidizing companies that pay poverty wages, so that their employees can have access to basic necessities like food and healthcare."
A primary purpose of analytical writing is to show how facts (explained through descriptive writing) support a particular conclusion or a particular path forward. This often requires explaining why an alternative interpretation is less satisfactory. This is how scholarly work—and good discussions in less formal situations—contribute to our collective understanding of the world.
Related Posts
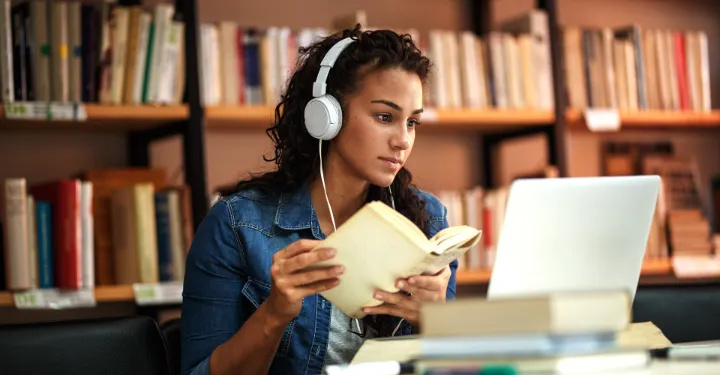
Using Logical Reasoning in Academic Writing
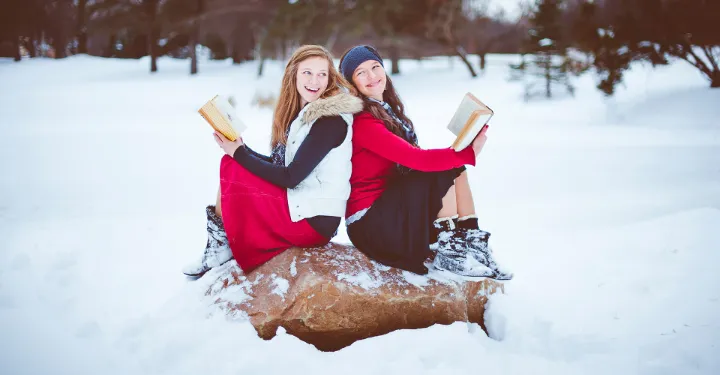
How to Write Outstanding College Level Book Reports
- Academic Writing Advice
- All Blog Posts
- Writing Advice
- Admissions Writing Advice
- Book Writing Advice
- Short Story Advice
- Employment Writing Advice
- Business Writing Advice
- Web Content Advice
- Article Writing Advice
- Magazine Writing Advice
- Grammar Advice
- Dialect Advice
- Editing Advice
- Freelance Advice
- Legal Writing Advice
- Poetry Advice
- Graphic Design Advice
- Logo Design Advice
- Translation Advice
- Blog Reviews
- Short Story Award Winners
- Scholarship Winners
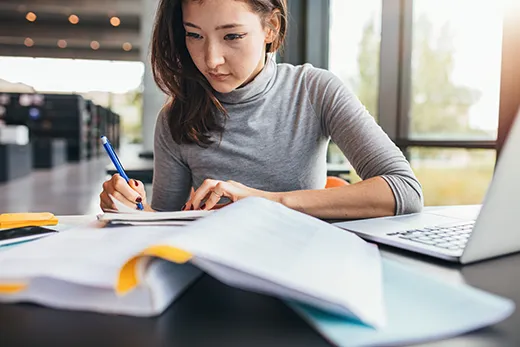
Need an academic editor before submitting your work?
Have a language expert improve your writing
Run a free plagiarism check in 10 minutes, generate accurate citations for free.
- Knowledge Base
Methodology
- Descriptive Research | Definition, Types, Methods & Examples
Descriptive Research | Definition, Types, Methods & Examples
Published on May 15, 2019 by Shona McCombes . Revised on June 22, 2023.
Descriptive research aims to accurately and systematically describe a population, situation or phenomenon. It can answer what , where , when and how questions , but not why questions.
A descriptive research design can use a wide variety of research methods to investigate one or more variables . Unlike in experimental research , the researcher does not control or manipulate any of the variables, but only observes and measures them.
Table of contents
When to use a descriptive research design, descriptive research methods, other interesting articles.
Descriptive research is an appropriate choice when the research aim is to identify characteristics, frequencies, trends, and categories.
It is useful when not much is known yet about the topic or problem. Before you can research why something happens, you need to understand how, when and where it happens.
Descriptive research question examples
- How has the Amsterdam housing market changed over the past 20 years?
- Do customers of company X prefer product X or product Y?
- What are the main genetic, behavioural and morphological differences between European wildcats and domestic cats?
- What are the most popular online news sources among under-18s?
- How prevalent is disease A in population B?
Here's why students love Scribbr's proofreading services
Discover proofreading & editing
Descriptive research is usually defined as a type of quantitative research , though qualitative research can also be used for descriptive purposes. The research design should be carefully developed to ensure that the results are valid and reliable .
Survey research allows you to gather large volumes of data that can be analyzed for frequencies, averages and patterns. Common uses of surveys include:
- Describing the demographics of a country or region
- Gauging public opinion on political and social topics
- Evaluating satisfaction with a company’s products or an organization’s services
Observations
Observations allow you to gather data on behaviours and phenomena without having to rely on the honesty and accuracy of respondents. This method is often used by psychological, social and market researchers to understand how people act in real-life situations.
Observation of physical entities and phenomena is also an important part of research in the natural sciences. Before you can develop testable hypotheses , models or theories, it’s necessary to observe and systematically describe the subject under investigation.
Case studies
A case study can be used to describe the characteristics of a specific subject (such as a person, group, event or organization). Instead of gathering a large volume of data to identify patterns across time or location, case studies gather detailed data to identify the characteristics of a narrowly defined subject.
Rather than aiming to describe generalizable facts, case studies often focus on unusual or interesting cases that challenge assumptions, add complexity, or reveal something new about a research problem .
If you want to know more about statistics , methodology , or research bias , make sure to check out some of our other articles with explanations and examples.
- Normal distribution
- Degrees of freedom
- Null hypothesis
- Discourse analysis
- Control groups
- Mixed methods research
- Non-probability sampling
- Quantitative research
- Ecological validity
Research bias
- Rosenthal effect
- Implicit bias
- Cognitive bias
- Selection bias
- Negativity bias
- Status quo bias
Cite this Scribbr article
If you want to cite this source, you can copy and paste the citation or click the “Cite this Scribbr article” button to automatically add the citation to our free Citation Generator.
McCombes, S. (2023, June 22). Descriptive Research | Definition, Types, Methods & Examples. Scribbr. Retrieved March 27, 2024, from https://www.scribbr.com/methodology/descriptive-research/
Is this article helpful?
Shona McCombes
Other students also liked, what is quantitative research | definition, uses & methods, correlational research | when & how to use, descriptive statistics | definitions, types, examples, unlimited academic ai-proofreading.
✔ Document error-free in 5minutes ✔ Unlimited document corrections ✔ Specialized in correcting academic texts
What are Analytical Study Designs?
- Research Process
- Peer Review
Analytical study designs can be experimental or observational and each type has its own features. In this article, you'll learn the main types of designs and how to figure out which one you'll need for your study.
Updated on September 19, 2022

A study design is critical to your research study because it determines exactly how you will collect and analyze your data. If your study aims to study the relationship between two variables, then an analytical study design is the right choice.
But how do you know which type of analytical study design is best for your specific research question? It's necessary to have a clear plan before you begin data collection. Lots of researchers, sadly, speed through this or don't do it at all.
When are analytical study designs used?
A study design is a systematic plan, developed so you can carry out your research study effectively and efficiently. Having a design is important because it will determine the right methodologies for your study. Using the right study design makes your results more credible, valid, and coherent.
Descriptive vs. analytical studies
Study designs can be broadly divided into either descriptive or analytical.
Descriptive studies describe characteristics such as patterns or trends. They answer the questions of what, who, where, and when, and they generate hypotheses. They include case reports and qualitative studies.
Analytical study designs quantify a relationship between different variables. They answer the questions of why and how. They're used to test hypotheses and make predictions.
Experimental and observational
Analytical study designs can be either experimental or observational. In experimental studies, researchers manipulate something in a population of interest and examine its effects. These designs are used to establish a causal link between two variables.
In observational studies, in contrast, researchers observe the effects of a treatment or intervention without manipulating anything. Observational studies are most often used to study larger patterns over longer periods.
Experimental study designs
Experimental study designs are when a researcher introduces a change in one group and not in another. Typically, these are used when researchers are interested in the effects of this change on some outcome. It's important to try to ensure that both groups are equivalent at baseline to make sure that any differences that arise are from any introduced change.
In one study, Reiner and colleagues studied the effects of a mindfulness intervention on pain perception . The researchers randomly assigned participants into an experimental group that received a mindfulness training program for two weeks. The rest of the participants were placed in a control group that did not receive the intervention.
Experimental studies help us establish causality. This is critical in science because we want to know whether one variable leads to a change, or causes another. Establishing causality leads to higher internal validity and makes results reproducible.
Experimental designs include randomized control trials (RCTs), nonrandomized control trials (non-RCTs), and crossover designs. Read on to learn the differences.
Randomized control trials
In an RCT, one group of individuals receives an intervention or a treatment, while another does not. It's then possible to investigate what happens to the participants in each group.
Another important feature of RCTs is that participants are randomly assigned to study groups. This helps to limit certain biases and retain better control. Randomization also lets researchers pinpoint any differences in outcomes to the intervention received during the trial. RTCs are considered the gold standard in biomedical research and are considered to provide the best kind of evidence.
For example, one RCT looked at whether an exercise intervention impacts depression . Researchers randomly placed patients with depressive symptoms into intervention groups containing different types of exercise (i.e., light, moderate, or strong). Another group received usual medications or no exercise interventions.
Results showed that after the 12-week trial, patients in all exercise groups had decreased depression levels compared to the control group. This means that by using an RCT design, researchers can now safely assume that the exercise variable has a positive impact on depression.
However, RCTs are not without drawbacks. In the example above, we don't know if exercise still has a positive impact on depression in the long term. This is because it's not feasible to keep people under these controlled settings for a long time.
Advantages of RCTs
- It is possible to infer causality
- Everything is properly controlled, so very little is left to chance or bias
- Can be certain that any difference is coming from the intervention
Disadvantages of RCTs
- Expensive and can be time-consuming
- Can take years for results to be available
- Cannot be done for certain types of questions due to ethical reasons, such as asking participants to undergo harmful treatment
- Limited in how many participants researchers can adequately manage in one study or trial
- Not feasible for people to live under controlled conditions for a long time
Nonrandomized controlled trials
Nonrandomized controlled trials are a type of nonrandomized controlled studies (NRS) where the allocation of participants to intervention groups is not done randomly . Here, researchers purposely assign some participants to one group and others to another group based on certain features. Alternatively, participants can sometimes also decide which group they want to be in.
For example, in one study, clinicians were interested in the impact of stroke recovery after being in an enriched versus non-enriched hospital environment . Patients were selected for the trial if they fulfilled certain requirements common to stroke recovery. Then, the intervention group was given access to an enriched environment (i.e. internet access, reading, going outside), and another group was not. Results showed that the enriched group performed better on cognitive tasks.
NRS are useful in medical research because they help study phenomena that would be difficult to measure with an RCT. However, one of their major drawbacks is that we cannot be sure if the intervention leads to the outcome. In the above example, we can't say for certain whether those patients improved after stroke because they were in the enriched environment or whether there were other variables at play.
Advantages of NRS's
- Good option when randomized control trials are not feasible
- More flexible than RCTs
Disadvantages of NRS's
- Can't be sure if the groups have underlying differences
- Introduces risk of bias and confounds
Crossover study
In a crossover design, each participant receives a sequence of different treatments. Crossover designs can be applied to RCTs, in which each participant is randomly assigned to different study groups.
For example, one study looked at the effects of replacing butter with margarine on lipoproteins levels in individuals with cholesterol . Patients were randomly assigned to a 6-week butter diet, followed by a 6-week margarine diet. In between both diets, participants ate a normal diet for 5 weeks.
These designs are helpful because they reduce bias. In the example above, each participant completed both interventions, making them serve as their own control. However, we don't know if eating butter or margarine first leads to certain results in some subjects.
Advantages of crossover studies
- Each participant serves as their own control, reducing confounding variables
- Require fewer participants, so they have better statistical power
Disadvantages of crossover studies
- Susceptible to order effects, meaning the order in which a treatment was given may have an effect
- Carry-over effects between treatments
Observational studies
In observational studies, researchers watch (observe) the effects of a treatment or intervention without trying to change anything in the population. Observational studies help us establish broad trends and patterns in large-scale datasets or populations. They are also a great alternative when an experimental study is not an option.
Unlike experimental research, observational studies do not help us establish causality. This is because researchers do not actively control any variables. Rather, they investigate statistical relationships between them. Often this is done using a correlational approach.
For example, researchers would like to examine the effects of daily fiber intake on bone density . They conduct a large-scale survey of thousands of individuals to examine correlations of fiber intake with different health measures.
The main observational studies are case-control, cohort, and cross-sectional. Let's take a closer look at each one below.
Case-control study
A case-control is a type of observational design in which researchers identify individuals with an existing health situation (cases) and a similar group without the health issue (controls). The cases and the controls are then compared based on some measurements.
Frequently, data collection in a case-control study is retroactive (i.e., backwards in time). This is because participants have already been exposed to the event in question. Additionally, researchers must go through records and patient files to obtain the records for this study design.
For example, a group of researchers examined whether using sleeping pills puts people at risk of Alzheimer's disease . They selected 1976 individuals that received a dementia diagnosis (“cases”) with 7184 other individuals (“controls”). Cases and controls were matched on specific measures such as sex and age. Patient data was consulted to find out how much sleeping pills were consumed over the course of a certain time.
Case-control is ideal for situations where cases are easy to pick out and compare. For instance, in studying rare diseases or outbreaks.
Advantages of case-control studies
- Feasible for rare diseases
- Cheaper and easier to do than an RCT
Disadvantages of case-control studies
- Relies on patient records, which could be lost or damaged
- Potential recall and selection bias
Cohort study (longitudinal)
A cohort is a group of people who are linked in some way. For instance, a birth year cohort is all people born in a specific year. In cohort studies, researchers compare what happens to individuals in the cohort that have been exposed to some variable compared with those that haven't on different variables. They're also called longitudinal studies.
The cohort is then repeatedly assessed on variables of interest over a period of time. There is no set amount of time required for cohort studies. They can range from a few weeks to many years.
Cohort studies can be prospective. In this case, individuals are followed for some time into the future. They can also be retrospective, where data is collected on a cohort from records.
One of the longest cohort studies today is The Harvard Study of Adult Development . This cohort study has been tracking various health outcomes of 268 Harvard graduates and 456 poor individuals in Boston from 1939 to 2014. Physical screenings, blood samples, brain scans and surveys were collected on this cohort for over 70 years. This study has produced a wealth of knowledge on outcomes throughout life.
A cohort study design is a good option when you have a specific group of people you want to study over time. However, a major drawback is that they take a long time and lack control.
Advantages of cohort studies
- Ethically safe
- Allows you to study multiple outcome variables
- Establish trends and patterns
Disadvantages of cohort studies
- Time consuming and expensive
- Can take many years for results to be revealed
- Too many variables to manage
- Depending on length of study, can have many changes in research personnel
Cross-sectional study
Cross-sectional studies are also known as prevalence studies. They look at the relationship of specific variables in a population in one given time. In cross-sectional studies, the researcher does not try to manipulate any of the variables, just study them using statistical analyses. Cross-sectional studies are also called snapshots of a certain variable or time.
For example, researchers wanted to determine the prevalence of inappropriate antibiotic use to study the growing concern about antibiotic resistance. Participants completed a self-administered questionnaire assessing their knowledge and attitude toward antibiotic use. Then, researchers performed statistical analyses on their responses to determine the relationship between the variables.
Cross-sectional study designs are ideal when gathering initial data on a research question. This data can then be analyzed again later. By knowing the public's general attitudes towards antibiotics, this information can then be relayed to physicians or public health authorities. However, it's often difficult to determine how long these results stay true for.
Advantages of cross-sectional studies
- Fast and inexpensive
- Provides a great deal of information for a given time point
- Leaves room for secondary analysis
Disadvantages of cross-sectional studies
- Requires a large sample to be accurate
- Not clear how long results remain true for
- Do not provide information on causality
- Cannot be used to establish long-term trends because data is only for a given time
So, how about your next study?
Whether it's an RCT, a case-control, or even a qualitative study, AJE has services to help you at every step of the publication process. Get expert guidance and publish your work for the world to see.

The AJE Team
See our "Privacy Policy"
- Skip to main content
- Skip to primary sidebar
- Skip to footer
- QuestionPro

- Solutions Industries Gaming Automotive Sports and events Education Government Travel & Hospitality Financial Services Healthcare Cannabis Technology Use Case NPS+ Communities Audience Contactless surveys Mobile LivePolls Member Experience GDPR Positive People Science 360 Feedback Surveys
- Resources Blog eBooks Survey Templates Case Studies Training Help center

Home Market Research Research Tools and Apps
Analytical Research: What is it, Importance + Examples
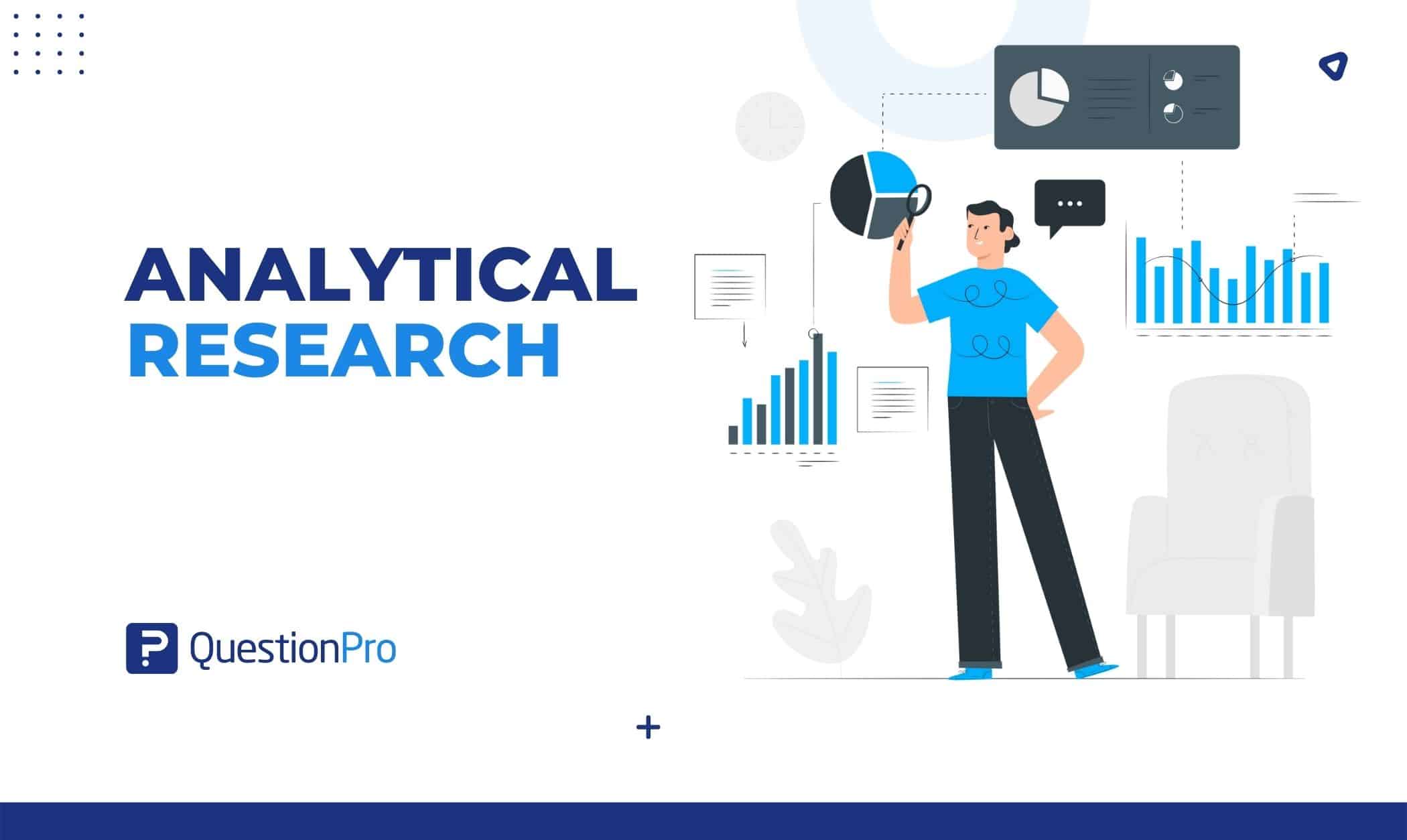
Finding knowledge is a loose translation of the word “research.” It’s a systematic and scientific way of researching a particular subject. As a result, research is a form of scientific investigation that seeks to learn more. Analytical research is one of them.
Any kind of research is a way to learn new things. In this research, data and other pertinent information about a project are assembled; after the information is gathered and assessed, the sources are used to support a notion or prove a hypothesis.
An individual can successfully draw out minor facts to make more significant conclusions about the subject matter by using critical thinking abilities (a technique of thinking that entails identifying a claim or assumption and determining whether it is accurate or untrue).
What is analytical research?
This particular kind of research calls for using critical thinking abilities and assessing data and information pertinent to the project at hand.
Determines the causal connections between two or more variables. The analytical study aims to identify the causes and mechanisms underlying the trade deficit’s movement throughout a given period.
It is used by various professionals, including psychologists, doctors, and students, to identify the most pertinent material during investigations. One learns crucial information from analytical research that helps them contribute fresh concepts to the work they are producing.
Some researchers perform it to uncover information that supports ongoing research to strengthen the validity of their findings. Other scholars engage in analytical research to generate fresh perspectives on the subject.
Various approaches to performing research include literary analysis, Gap analysis , general public surveys, clinical trials, and meta-analysis.
Importance of analytical research
The goal of analytical research is to develop new ideas that are more believable by combining numerous minute details.
The analytical investigation is what explains why a claim should be trusted. Finding out why something occurs is complex. You need to be able to evaluate information critically and think critically.
This kind of information aids in proving the validity of a theory or supporting a hypothesis. It assists in recognizing a claim and determining whether it is true.
Analytical kind of research is valuable to many people, including students, psychologists, marketers, and others. It aids in determining which advertising initiatives within a firm perform best. In the meantime, medical research and research design determine how well a particular treatment does.
Thus, analytical research can help people achieve their goals while saving lives and money.
Methods of Conducting Analytical Research
Analytical research is the process of gathering, analyzing, and interpreting information to make inferences and reach conclusions. Depending on the purpose of the research and the data you have access to, you can conduct analytical research using a variety of methods. Here are a few typical approaches:
Quantitative research
Numerical data are gathered and analyzed using this method. Statistical methods are then used to analyze the information, which is often collected using surveys, experiments, or pre-existing datasets. Results from quantitative research can be measured, compared, and generalized numerically.
Qualitative research
In contrast to quantitative research, qualitative research focuses on collecting non-numerical information. It gathers detailed information using techniques like interviews, focus groups, observations, or content research. Understanding social phenomena, exploring experiences, and revealing underlying meanings and motivations are all goals of qualitative research.
Mixed methods research
This strategy combines quantitative and qualitative methodologies to grasp a research problem thoroughly. Mixed methods research often entails gathering and evaluating both numerical and non-numerical data, integrating the results, and offering a more comprehensive viewpoint on the research issue.
Experimental research
Experimental research is frequently employed in scientific trials and investigations to establish causal links between variables. This approach entails modifying variables in a controlled environment to identify cause-and-effect connections. Researchers randomly divide volunteers into several groups, provide various interventions or treatments, and track the results.
Observational research
With this approach, behaviors or occurrences are observed and methodically recorded without any outside interference or variable data manipulation . Both controlled surroundings and naturalistic settings can be used for observational research . It offers useful insights into behaviors that occur in the actual world and enables researchers to explore events as they naturally occur.
Case study research
This approach entails thorough research of a single case or a small group of related cases. Case-control studies frequently include a variety of information sources, including observations, records, and interviews. They offer rich, in-depth insights and are particularly helpful for researching complex phenomena in practical settings.
Secondary data analysis
Examining secondary information is time and money-efficient, enabling researchers to explore new research issues or confirm prior findings. With this approach, researchers examine previously gathered information for a different reason. Information from earlier cohort studies, accessible databases, or corporate documents may be included in this.
Content analysis
Content research is frequently employed in social sciences, media observational studies, and cross-sectional studies. This approach systematically examines the content of texts, including media, speeches, and written documents. Themes, patterns, or keywords are found and categorized by researchers to make inferences about the content.
Depending on your research objectives, the resources at your disposal, and the type of data you wish to analyze, selecting the most appropriate approach or combination of methodologies is crucial to conducting analytical research.
Examples of analytical research
Analytical research takes a unique measurement. Instead, you would consider the causes and changes to the trade imbalance. Detailed statistics and statistical checks help guarantee that the results are significant.
For example, it can look into why the value of the Japanese Yen has decreased. This is so that an analytical study can consider “how” and “why” questions.
Another example is that someone might conduct analytical research to identify a study’s gap. It presents a fresh perspective on your data. Therefore, it aids in supporting or refuting notions.
Descriptive vs analytical research
Here are the key differences between descriptive research and analytical research:
The study of cause and effect makes extensive use of analytical research. It benefits from numerous academic disciplines, including marketing, health, and psychology, because it offers more conclusive information for addressing research issues.
QuestionPro offers solutions for every issue and industry, making it more than just survey software. For handling data, we also have systems like our InsightsHub research library.
You may make crucial decisions quickly while using QuestionPro to understand your clients and other study subjects better. Make use of the possibilities of the enterprise-grade research suite right away!
LEARN MORE FREE TRIAL
MORE LIKE THIS
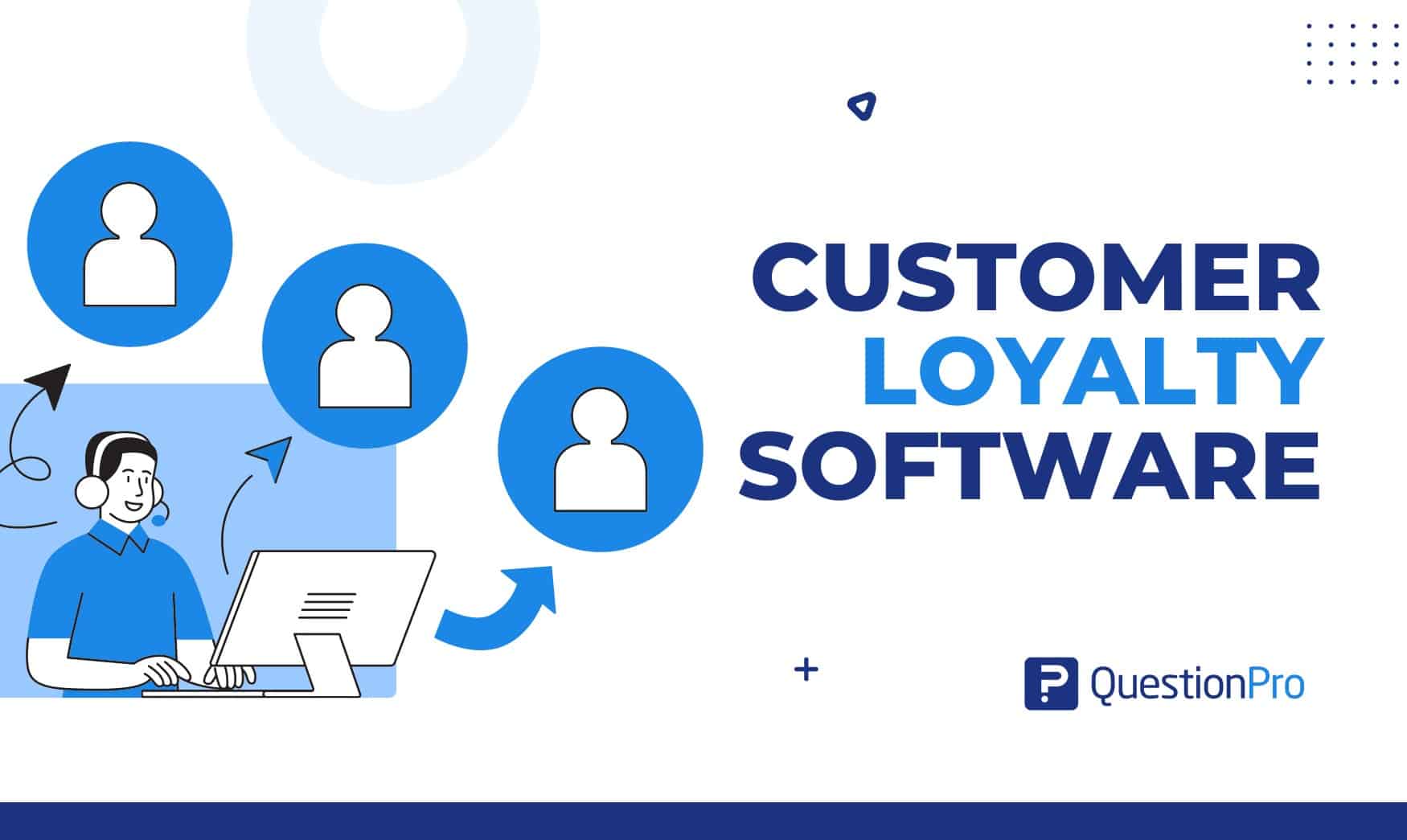
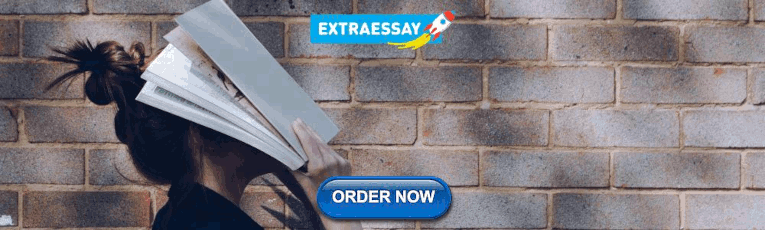
10 Top Customer Loyalty Software to Boost Your Business
Mar 25, 2024
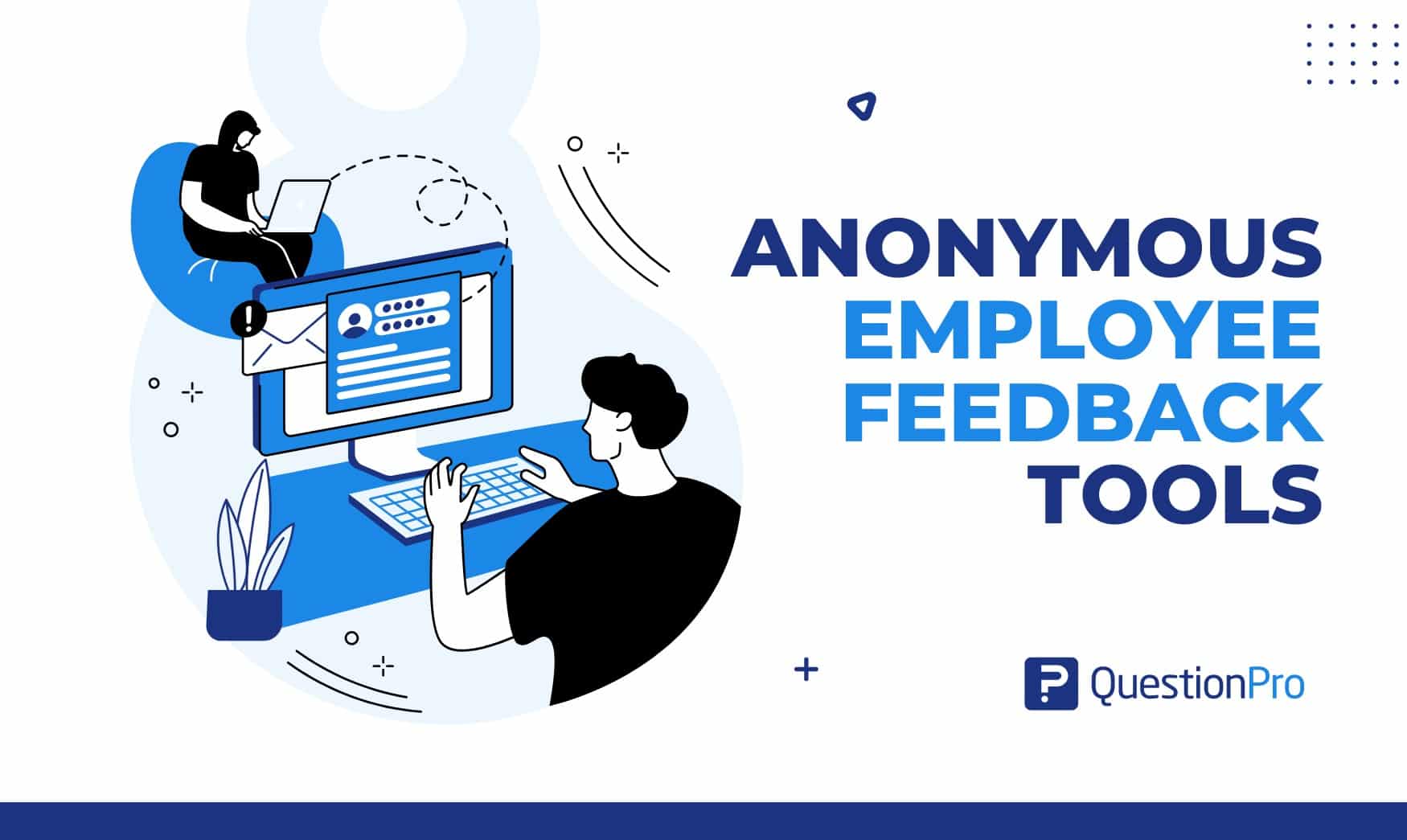
Top 13 Anonymous Employee Feedback Tools for 2024
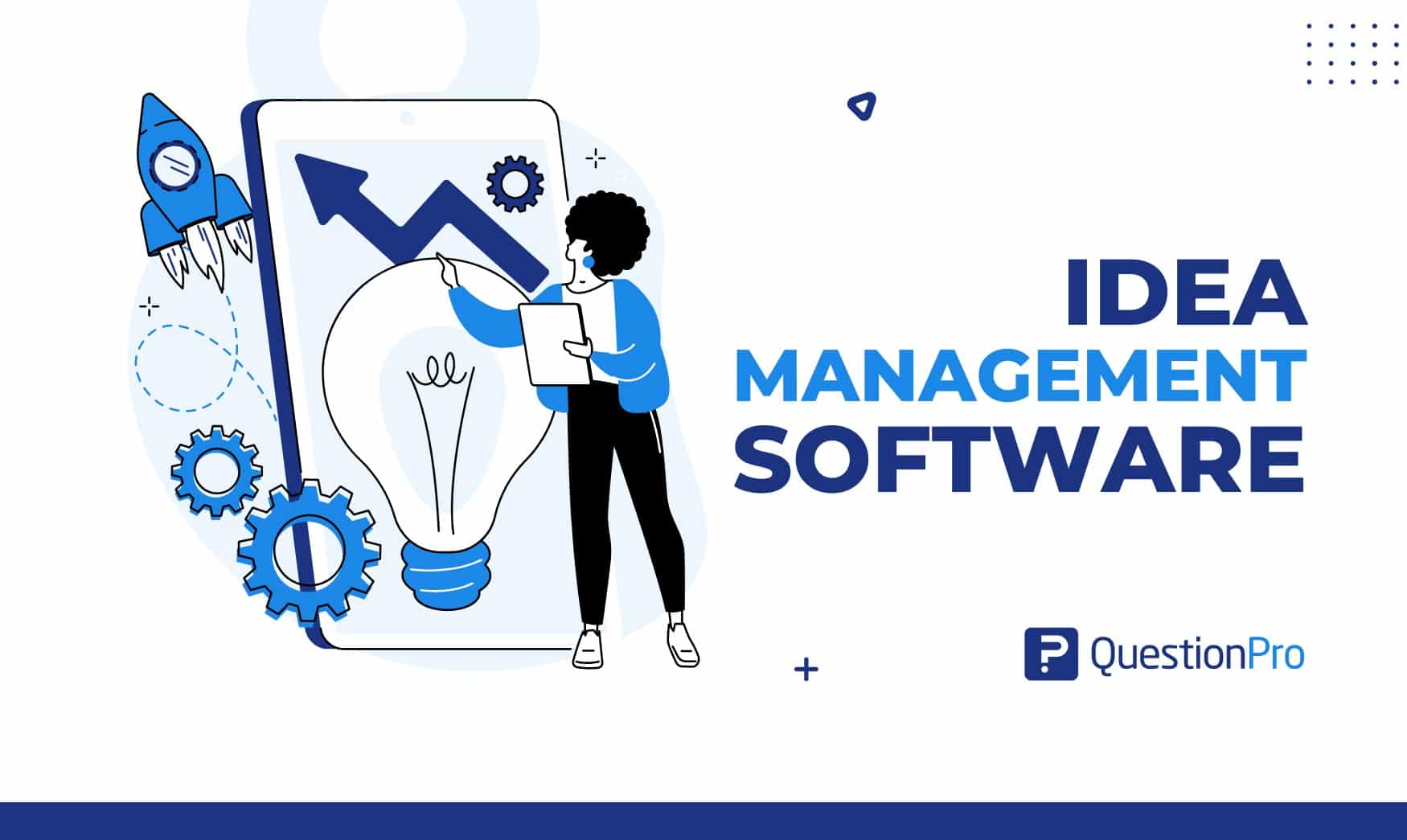
Unlocking Creativity With 10 Top Idea Management Software
Mar 23, 2024
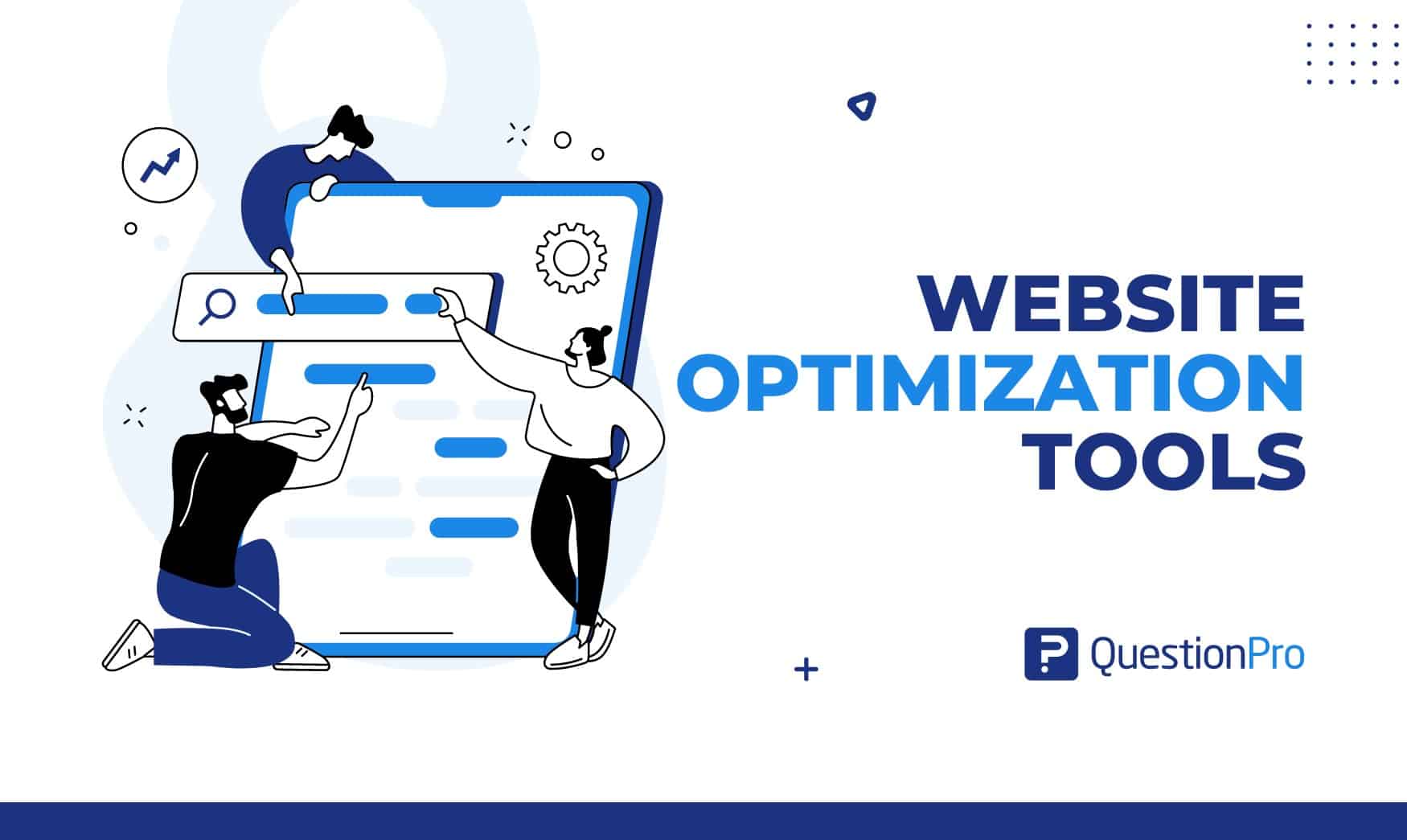
20 Best Website Optimization Tools to Improve Your Website
Mar 22, 2024
Other categories
- Academic Research
- Artificial Intelligence
- Assessments
- Brand Awareness
- Case Studies
- Communities
- Consumer Insights
- Customer effort score
- Customer Engagement
- Customer Experience
- Customer Loyalty
- Customer Research
- Customer Satisfaction
- Employee Benefits
- Employee Engagement
- Employee Retention
- Friday Five
- General Data Protection Regulation
- Insights Hub
- Life@QuestionPro
- Market Research
- Mobile diaries
- Mobile Surveys
- New Features
- Online Communities
- Question Types
- Questionnaire
- QuestionPro Products
- Release Notes
- Research Tools and Apps
- Revenue at Risk
- Survey Templates
- Training Tips
- Uncategorized
- Video Learning Series
- What’s Coming Up
- Workforce Intelligence
- Privacy Policy
Buy Me a Coffee

Home » Descriptive Analytics – Methods, Tools and Examples
Descriptive Analytics – Methods, Tools and Examples
Table of Contents

Descriptive Analytics
Definition:
Descriptive analytics focused on describing or summarizing raw data and making it interpretable. This type of analytics provides insight into what has happened in the past. It involves the analysis of historical data to identify patterns, trends, and insights. Descriptive analytics often uses visualization tools to represent the data in a way that is easy to interpret.
Descriptive Analytics in Research
Descriptive analytics plays a crucial role in research, helping investigators understand and describe the data collected in their studies. Here’s how descriptive analytics is typically used in a research setting:
- Descriptive Statistics: In research, descriptive analytics often takes the form of descriptive statistics . This includes calculating measures of central tendency (like mean, median, and mode), measures of dispersion (like range, variance, and standard deviation), and measures of frequency (like count, percent, and frequency). These calculations help researchers summarize and understand their data.
- Visualizing Data: Descriptive analytics also involves creating visual representations of data to better understand and communicate research findings . This might involve creating bar graphs, line graphs, pie charts, scatter plots, box plots, and other visualizations.
- Exploratory Data Analysis: Before conducting any formal statistical tests, researchers often conduct an exploratory data analysis, which is a form of descriptive analytics. This might involve looking at distributions of variables, checking for outliers, and exploring relationships between variables.
- Initial Findings: Descriptive analytics are often reported in the results section of a research study to provide readers with an overview of the data. For example, a researcher might report average scores, demographic breakdowns, or the percentage of participants who endorsed each response on a survey.
- Establishing Patterns and Relationships: Descriptive analytics helps in identifying patterns, trends, or relationships in the data, which can guide subsequent analysis or future research. For instance, researchers might look at the correlation between variables as a part of descriptive analytics.
Descriptive Analytics Techniques
Descriptive analytics involves a variety of techniques to summarize, interpret, and visualize historical data. Some commonly used techniques include:
Statistical Analysis
This includes basic statistical methods like mean, median, mode (central tendency), standard deviation, variance (dispersion), correlation, and regression (relationships between variables).
Data Aggregation
It is the process of compiling and summarizing data to obtain a general perspective. It can involve methods like sum, count, average, min, max, etc., often applied to a group of data.
Data Mining
This involves analyzing large volumes of data to discover patterns, trends, and insights. Techniques used in data mining can include clustering (grouping similar data), classification (assigning data into categories), association rules (finding relationships between variables), and anomaly detection (identifying outliers).
Data Visualization
This involves presenting data in a graphical or pictorial format to provide clear and easy understanding of the data patterns, trends, and insights. Common data visualization methods include bar charts, line graphs, pie charts, scatter plots, histograms, and more complex forms like heat maps and interactive dashboards.
This involves organizing data into informational summaries to monitor how different areas of a business are performing. Reports can be generated manually or automatically and can be presented in tables, graphs, or dashboards.
Cross-tabulation (or Pivot Tables)
It involves displaying the relationship between two or more variables in a tabular form. It can provide a deeper understanding of the data by allowing comparisons and revealing patterns and correlations that may not be readily apparent in raw data.
Descriptive Modeling
Some techniques use complex algorithms to interpret data. Examples include decision tree analysis, which provides a graphical representation of decision-making situations, and neural networks, which are used to identify correlations and patterns in large data sets.
Descriptive Analytics Tools
Some common Descriptive Analytics Tools are as follows:
Excel: Microsoft Excel is a widely used tool that can be used for simple descriptive analytics. It has powerful statistical and data visualization capabilities. Pivot tables are a particularly useful feature for summarizing and analyzing large data sets.
Tableau: Tableau is a data visualization tool that is used to represent data in a graphical or pictorial format. It can handle large data sets and allows for real-time data analysis.
Power BI: Power BI, another product from Microsoft, is a business analytics tool that provides interactive visualizations with self-service business intelligence capabilities.
QlikView: QlikView is a data visualization and discovery tool. It allows users to analyze data and use this data to support decision-making.
SAS: SAS is a software suite that can mine, alter, manage and retrieve data from a variety of sources and perform statistical analysis on it.
SPSS: SPSS (Statistical Package for the Social Sciences) is a software package used for statistical analysis. It’s widely used in social sciences research but also in other industries.
Google Analytics: For web data, Google Analytics is a popular tool. It allows businesses to analyze in-depth detail about the visitors on their website, providing valuable insights that can help shape the success strategy of a business.
R and Python: Both are programming languages that have robust capabilities for statistical analysis and data visualization. With packages like pandas, matplotlib, seaborn in Python and ggplot2, dplyr in R, these languages are powerful tools for descriptive analytics.
Looker: Looker is a modern data platform that can take data from any database and let you start exploring and visualizing.
When to use Descriptive Analytics
Descriptive analytics forms the base of the data analysis workflow and is typically the first step in understanding your business or organization’s data. Here are some situations when you might use descriptive analytics:
Understanding Past Behavior: Descriptive analytics is essential for understanding what has happened in the past. If you need to understand past sales trends, customer behavior, or operational performance, descriptive analytics is the tool you’d use.
Reporting Key Metrics: Descriptive analytics is used to establish and report key performance indicators (KPIs). It can help in tracking and presenting these KPIs in dashboards or regular reports.
Identifying Patterns and Trends: If you need to identify patterns or trends in your data, descriptive analytics can provide these insights. This might include identifying seasonality in sales data, understanding peak operational times, or spotting trends in customer behavior.
Informing Business Decisions: The insights provided by descriptive analytics can inform business strategy and decision-making. By understanding what has happened in the past, you can make more informed decisions about what steps to take in the future.
Benchmarking Performance: Descriptive analytics can be used to compare current performance against historical data. This can be used for benchmarking and setting performance goals.
Auditing and Regulatory Compliance: In sectors where compliance and auditing are essential, descriptive analytics can provide the necessary data and trends over specific periods.
Initial Data Exploration: When you first acquire a dataset, descriptive analytics is useful to understand the structure of the data, the relationships between variables, and any apparent anomalies or outliers.
Examples of Descriptive Analytics
Examples of Descriptive Analytics are as follows:
Retail Industry: A retail company might use descriptive analytics to analyze sales data from the past year. They could break down sales by month to identify any seasonality trends. For example, they might find that sales increase in November and December due to holiday shopping. They could also break down sales by product to identify which items are the most popular. This analysis could inform their purchasing and stocking decisions for the next year. Additionally, data on customer demographics could be analyzed to understand who their primary customers are, guiding their marketing strategies.
Healthcare Industry: In healthcare, descriptive analytics could be used to analyze patient data over time. For instance, a hospital might analyze data on patient admissions to identify trends in admission rates. They might find that admissions for certain conditions are higher at certain times of the year. This could help them allocate resources more effectively. Also, analyzing patient outcomes data can help identify the most effective treatments or highlight areas where improvement is needed.
Finance Industry: A financial firm might use descriptive analytics to analyze historical market data. They could look at trends in stock prices, trading volume, or economic indicators to inform their investment decisions. For example, analyzing the price-earnings ratios of stocks in a certain sector over time could reveal patterns that suggest whether the sector is currently overvalued or undervalued. Similarly, credit card companies can analyze transaction data to detect any unusual patterns, which could be signs of fraud.
Advantages of Descriptive Analytics
Descriptive analytics plays a vital role in the world of data analysis, providing numerous advantages:
- Understanding the Past: Descriptive analytics provides an understanding of what has happened in the past, offering valuable context for future decision-making.
- Data Summarization: Descriptive analytics is used to simplify and summarize complex datasets, which can make the information more understandable and accessible.
- Identifying Patterns and Trends: With descriptive analytics, organizations can identify patterns, trends, and correlations in their data, which can provide valuable insights.
- Inform Decision-Making: The insights generated through descriptive analytics can inform strategic decisions and help organizations to react more quickly to events or changes in behavior.
- Basis for Further Analysis: Descriptive analytics lays the groundwork for further analytical activities. It’s the first necessary step before moving on to more advanced forms of analytics like predictive analytics (forecasting future events) or prescriptive analytics (advising on possible outcomes).
- Performance Evaluation: It allows organizations to evaluate their performance by comparing current results with past results, enabling them to see where improvements have been made and where further improvements can be targeted.
- Enhanced Reporting and Dashboards: Through the use of visualization techniques, descriptive analytics can improve the quality of reports and dashboards, making the data more understandable and easier to interpret for stakeholders at all levels of the organization.
- Immediate Value: Unlike some other types of analytics, descriptive analytics can provide immediate insights, as it doesn’t require complex models or deep analytical capabilities to provide value.
Disadvantages of Descriptive Analytics
While descriptive analytics offers numerous benefits, it also has certain limitations or disadvantages. Here are a few to consider:
- Limited to Past Data: Descriptive analytics primarily deals with historical data and provides insights about past events. It does not predict future events or trends and can’t help you understand possible future outcomes on its own.
- Lack of Deep Insights: While descriptive analytics helps in identifying what happened, it does not answer why it happened. For deeper insights, you would need to use diagnostic analytics, which analyzes data to understand the root cause of a particular outcome.
- Can Be Misleading: If not properly executed, descriptive analytics can sometimes lead to incorrect conclusions. For example, correlation does not imply causation, but descriptive analytics might tempt one to make such an inference.
- Data Quality Issues: The accuracy and usefulness of descriptive analytics are heavily reliant on the quality of the underlying data. If the data is incomplete, incorrect, or biased, the results of the descriptive analytics will be too.
- Over-reliance on Descriptive Analytics: Businesses may rely too much on descriptive analytics and not enough on predictive and prescriptive analytics. While understanding past and present data is important, it’s equally vital to forecast future trends and make data-driven decisions based on those predictions.
- Doesn’t Provide Actionable Insights: Descriptive analytics is used to interpret historical data and identify patterns and trends, but it doesn’t provide recommendations or courses of action. For that, prescriptive analytics is needed.
About the author
Muhammad Hassan
Researcher, Academic Writer, Web developer
You may also like

Digital Ethnography – Types, Methods and Examples

Predictive Analytics – Techniques, Tools and...

Big Data Analytics -Types, Tools and Methods

Diagnostic Analytics – Methods, Tools and...

Blockchain Research – Methods, Types and Examples

Social Network Analysis – Types, Tools and...
Distinguishing Features and Similarities Between Descriptive Phenomenological and Qualitative Description Research
Affiliations.
- 1 Boston College, Chestnut Hill, MA, USA [email protected].
- 2 New York University, New York City, USA.
- 3 University of North Carolina at Chapel Hill, USA.
- 4 University of Nebraska Medical Center, Omaha, USA.
- PMID: 27106878
- DOI: 10.1177/0193945916645499
Scholars who research phenomena of concern to the discipline of nursing are challenged with making wise choices about different qualitative research approaches. Ultimately, they want to choose an approach that is best suited to answer their research questions. Such choices are predicated on having made distinctions between qualitative methodology, methods, and analytic frames. In this article, we distinguish two qualitative research approaches widely used for descriptive studies: descriptive phenomenological and qualitative description. Providing a clear basis that highlights the distinguishing features and similarities between descriptive phenomenological and qualitative description research will help students and researchers make more informed choices in deciding upon the most appropriate methodology in qualitative research. We orient the reader to distinguishing features and similarities associated with each approach and the kinds of research questions descriptive phenomenological and qualitative description research address.
Keywords: phenomenology; qualitative methods.
© The Author(s) 2016.
- Nursing Methodology Research*
- Qualitative Research*
- Research Design*
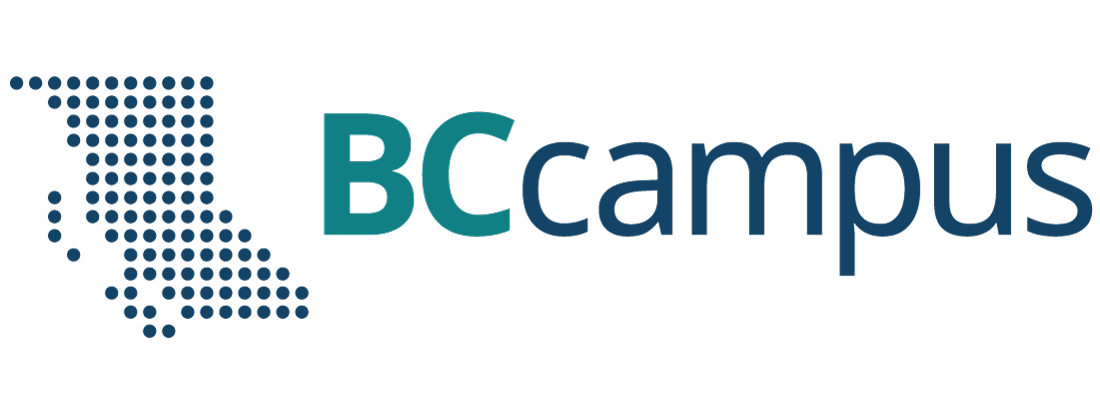
Want to create or adapt books like this? Learn more about how Pressbooks supports open publishing practices.
Chapter 3: Developing a Research Question
3.2 Exploration, Description, Explanation
As you can see, there is much to think about and many decisions to be made as you begin to define your research question and your research project. Something else you will need to consider in the early stages is whether your research will be exploratory, descriptive, or explanatory. Each of these types of research has a different aim or purpose, consequently, how you design your research project will be determined in part by this decision. In the following paragraphs we will look at these three types of research.
Exploratory research
Researchers conducting exploratory research are typically at the early stages of examining their topics. These sorts of projects are usually conducted when a researcher wants to test the feasibility of conducting a more extensive study; he or she wants to figure out the lay of the land with respect to the particular topic. Perhaps very little prior research has been conducted on this subject. If this is the case, a researcher may wish to do some exploratory work to learn what method to use in collecting data, how best to approach research participants, or even what sorts of questions are reasonable to ask. A researcher wanting to simply satisfy his or her own curiosity about a topic could also conduct exploratory research. Conducting exploratory research on a topic is often a necessary first step, both to satisfy researcher curiosity about the subject and to better understand the phenomenon and the research participants in order to design a larger, subsequent study. See Table 2.1 for examples.
Descriptive research
Sometimes the goal of research is to describe or define a particular phenomenon. In this case, descriptive research would be an appropriate strategy. A descriptive may, for example, aim to describe a pattern. For example, researchers often collect information to describe something for the benefit of the general public. Market researchers rely on descriptive research to tell them what consumers think of their products. In fact, descriptive research has many useful applications, and you probably rely on findings from descriptive research without even being aware that that is what you are doing. See Table 3.1 for examples.
Explanatory research
The third type of research, explanatory research, seeks to answer “why” questions. In this case, the researcher is trying to identify the causes and effects of whatever phenomenon is being studied. An explanatory study of college students’ addictions to their electronic gadgets, for example, might aim to understand why students become addicted. Does it have anything to do with their family histories? Does it have anything to do with their other extracurricular hobbies and activities? Does it have anything to do with the people with whom they spend their time? An explanatory study could answer these kinds of questions. See Table 3.1 for examples.
Table 3.1 Exploratory, descriptive and explanatory research differences (Adapted from Adjei, n.d.).
Research Methods for the Social Sciences: An Introduction Copyright © 2020 by Valerie Sheppard is licensed under a Creative Commons Attribution-NonCommercial-ShareAlike 4.0 International License , except where otherwise noted.
Share This Book
404 Not found
Symptom burden and relief in palliative care units of German Comprehensive Cancer Center and other hospitals
- Open access
- Published: 27 March 2024
- Volume 150 , article number 160 , ( 2024 )
Cite this article
You have full access to this open access article
- Julia Berendt ORCID: orcid.org/0000-0002-1203-6786 1 na1 ,
- Sarah Brunner 1 , 2 na1 ,
- Maria Heckel 1 ,
- Mitra Tewes 3 ,
- Christoph Ostgathe 1 &
- Susanne Gahr 1
The National Hospice and Palliative Registry contains patient data from German hospice and palliative care facilities about symptoms. The aim of the study at hand is to differentiate symptom burden of patients in palliative care units between Comprehensive Cancer Center (CCC) and other hospitals regarding symptom burden and relief of patients in palliative care units.
The registry analysis provided data of patients in palliative care units (2014–2018). We analyzed characteristic and symptom-related data on 18 symptoms, with considerable symptom-burdened patients (moderate or severe). We followed a cancer (yes/no) and facility-specific descriptive analysis ( f , %, μ , Mdn, SD, V , r ) using SPSS.
We evaluated 10,447 patient records (CCC: 4234 pts/non CCC 6,213 pts), 82% with a cancer diagnosis. For cancer patients, the mean age in CCC-affiliated palliative care units was 68 (SD 19–99) years, in others 73 (SD 23–104) years ( p < 0.05; V = 0.2). The proportion of patients with significant symptom burden is lower in CCC-affiliated than in other palliative care units. The difference between facilities shows a significant weak effect in pain, vomiting and constipation, depressiveness, anxiety, and tension. The proportion of cases which symptom burden could be alleviated is higher in CCC-affiliated palliative care units with significant weak/medium effect in pain, nausea, vomiting, shortness of breath, constipation, wound care problems, depressiveness, anxiety, tension, confusion, and problems in organizing care.
We found differences in symptom burden and symptom relief between CCC-affiliated and other palliative care units. CCCs should continue to feel responsible for sharing knowledge about symptom relief, such as through standard operating procedures and education.
Avoid common mistakes on your manuscript.
Introduction
In 2009, the German Cancer Aid founded the Comprehensive Cancer Center (CCC) Network: an alliance of oncological centers of excellence. Oncological centers offer patients and their families optimal, individualized health care based on the most recent evidence (Albreht et al. 2017 ). CCCs are high-performance medical centers, especially for modern cancer therapy, located in high population density areas and highly specialized in treating complex and difficult cases. Those certified centers declare a survival benefit of treatment (Schoffer et al. 2022 ). CCCs develop a role model effect for patient-centered cancer care of stakeholders in cancer care (Brandts 2019 ). Regarding the interest of interdisciplinary care palliative care facilities are affiliated with a CCC (Berendt et al. 2016 ). The Palliative Care Guideline from the National Cancer Center Network (NCCN) reports that high symptom burden may require palliative care consultation (Dans et al. 2022 ). The World Health Organization defines palliative care as “an approach that improves the quality of life of patients and their families facing the problems associated with life-threatening illness. It prevents and relieves suffering through the early identification, correct assessment and treatment of pain and other problems […]. Palliative care is required for a wide range of diseases […]” (WHO 2020 ). The concept of palliative care explicitly contains the relief of physical, psychological, social, and other problems (Mori et al. 2011 ; Radbruch et al. 2020 ). For high-quality standards in cancer care, CCC develop clinical practice guidelines for health care professionals and keep them up to date, especially to manage symptoms and problems according to current research findings (Berendt et al. 2017 ; Lödel et al. 2020 ). Symptom relief is highly successful at all settings in palliative care (Delgado-Guay et al. 2018 ; Jung et al. 2022 ; Ullrich et al. 2017 ). CCC are usually integrated within an university hospital. Therefore, palliative care is also provided to patients without cancer. As patients differ substantially (Stiel et al. 2014 ; Ostgathe et al. 2011 ), it is always important to consider both groups, on the one hand to better understand the specificity of needs of palliative care patients with cancer, to better taylor services and to mutually learn in regard, e.g., to symptom assessment and management.
In 2009, the German Association for Palliative Medicine (DGP) and the German Hospice and Palliative Association (DHPV) established the German National Hospice and Palliative Registry. It based on the Edmonton Symptom Assessment Scale (ESAS) (Hui and Bruera 2017 ; Stiel et al. 2010 ) and underwent some revisions after validation (Stiel et al. 2012 ). The title of the validated data-collecting instrument was Hospice and Palliative Care Evaluation Symptom and Problem Checklist (HOPE-SP-CL). The documentation contains a symptom and problem checklist on physical, psychological, social, and care-related symptoms (Stiel et al. 2012 ), and is noted essential in the Best Practice Recommendations for Integrating Palliative Care into CCCs funded by the German Cancer Aid (Berendt et al. 2016 ). Documented information came from palliative care teams, inpatient hospices, hospital palliative care support teams, and palliative care units. The current literature presents limited evidence on institution-specific analysis.
The aim of this study is to report (1) the symptom burden of patients on admission to palliative care units of CCCs and other hospitals, and (2) the success in symptom relief between admission and the end of treatment in each institution type. According to our hypothesis, palliative care units of CCCs treat patients with more considerable symptoms than other hospitals.
Register data
Our reporting is based on RECORD items for “Routinely collected health Data” as we use data from the German National Hospice and Palliative Registry. In February 2020, anonymized data (2014–2018) were provided by Smart-Q GmbH that collects and manages registry data of 184 institutions. With the given data set, it was only possible to determine whether the data came from the palliative care unit or the palliative care consultation service of a hospital. Eleven German CCCs agreed to declare the datasets back to the institution type “CCC”. Another reference to the institution "hospital" does not exist. The data set for the consultation services was eliminated due to missing data. We labeled the source of the remaining data sets as "palliative care unit of other hospitals", if they were obtained from palliative care units, e.g., of other university hospitals not certified as CCC.
Besides demographic, disease-specific, and treatment-related information, the core data set of the registry contains the validated symptom and problem checklist HOPE-SP-CL (Stiel et al. 2012 ). The HOPE-SP-CL encompasses proxy assessed data on pain, nausea, vomiting, dyspnea, constipation, weakness, loss of appetite, tiredness, wound care problems, need of assistance with activities of daily living, feeling depressed, anxiety, tension, restlessness, disorientation/confusion, problems with organization of care, overburdening of family, and other problems. These symptoms’ intensities are ranked on a scale from "0 = none", "1 = mild", "2 = moderate", to "3 = severe" and were assessed at two time points: at (1) admission, and (2) at the end of the treatment (hospital discharge or death).
This study analyzed data sets of patients hospitalized in German palliative care units between 2014 and 2018 entered in the register by the institution providing palliative care.
Transformation of scale
We declared all patients who rated their symptoms with 2 (moderate) or 3 (severe) as considerably burdened. The change of symptom burden was measured by the numerical difference between symptom burden at the beginning and end of treatment. In the registry, the end of treatment is defined as last information before discharge or death. Neutral values referred to a constant state. If there were positive values (symptom intensity increased), a decrease in symptom intensity was indicated. Negative values (symptom intensity decreased) showed an improvement, designated as symptom burden relief.
Study design
The study design is quantitative and descriptive, using exploratory data analysis (cohort study). A correlation analysis (rank correlation) between two variables is conducted: institution type (independent variable) and each symptom (dependent variable).
The data set was adjusted at baseline for missing information regarding age, sex, cancer diagnosis, and ECOG (Eastern Cooperative Oncology Group) Performance Status (see Fig. 1 ).
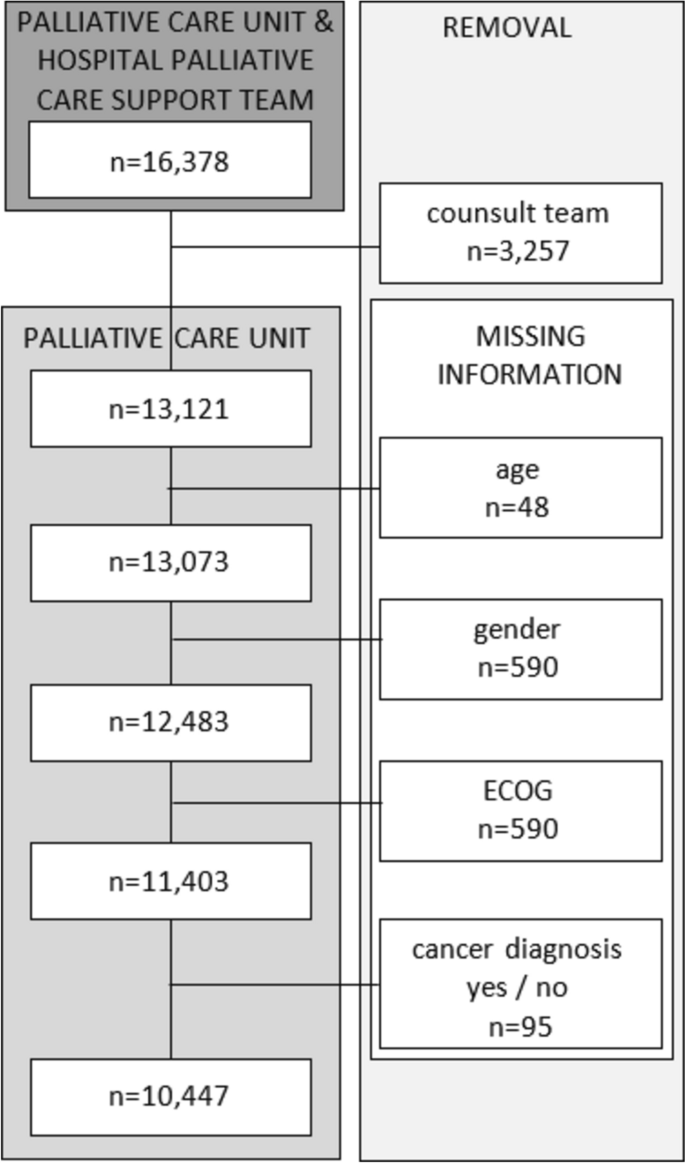
Data cleaning; removal of cases with missing data in different categories
Data were collected using IBM SPSS Statistics 24. The frequencies ( n ), percent (%), mean ( M ), standard deviation (SD), median (mdn), significance ( p ), Cramér’s V ( V ), and Pearson's r ( r ) were calculated. The level of significance was set at 5%. Because of a large sample size, Chi 2 tests and Chi 2 -based measures such as Cramér’s V according to Cohen (Cohen 1988 ) and Pearson’s correlations r were performed to derive insights for practical relevance. Cramér’s V is based on the contingency coefficient phi φ . We differentiated between two evaluation streams for analysis of patient clientele and symptom relief: cancer-directed (cancer and non-cancer), and institution-specific (CCC-affiliated palliative care units vs. other palliative care units). Percent differences were considered above a 5% deviation and were analyzed in relation to effect size.
We analyzed 10,447 patient records: 4234 cases of CCC-affiliated palliative care units (41%) and 6213 cases of other palliative care units (59%). The total data set included 8600 cases with cancer (82%) and 1847 patients with a non-cancer diagnosis (18%) (Table 1 ).
Characteristics of cancer patients
Among cancer patients in CCC-affiliated palliative care units, 46.0% are female, compared with 48.1% in other palliative care units. There is no correlation between institution type and gender or ECOG. Cancer patients in a CCC-affiliated palliative care unit are five years younger within a detectable weak effect and have fewer symptoms at the time of admission. Cancer patients remained hospitalized in CCC-affiliated palliative care unit one day shorter on median than in other palliative care units, at a moderate effect. There was a higher proportion of cancer patients dying in the palliative care unit of CCCs than other hospitals showing a weak significant effect. People not diagnosed with cancer but receiving treatment in a CCC are also younger, but have a shorter duration of stay (Table 2 ).
Symptom burden for cancer patients according to institution type
The rate of considerably symptom-burdened patients is more than 10% higher for each symptom in other palliative care units than in CCC-affiliated palliative care units. Thus, in CCC-affiliated palliative care units, significantly fewer cancer patients are affected by pain, vomiting and constipation, depressiveness, anxiety, tension at a weak effect between institution types (Table 3 ).
Symptom burden relief for cancer patients according to institution type
In CCC-affiliated palliative care units, symptom burden reduced to a higher rate among significantly symptom-burdened cancer patients compared with other palliative care units, especially with significant weak or moderate effect in pain, nausea, vomiting, dyspnea, constipation, wound care problems, feeling depressed, anxiety, tension, disorientation/confusion, and problems with organization of care (Table 4 ).
The study at hand is the first using nationwide registry data for cross-institutional analysis regarding symptom burden and relief in palliative care of German CCC and other hospitals. Interestingly and at the first sight somewhat counterintuitive, we found a larger proportion of considerably symptom-burdened cancer patients being treated in palliative care units of other hospitals compared to CCC-affiliated palliative care units for a range of symptoms. A result contrasting with our hypothesis and previous findings (Brunner et al. 2022 ; Delgado-Guay et al. 2018 ). However, Brunner et al. ( 2022 ) considered all patients over all classifications for symptom intensity, whereas we focused on cases with moderate and severe symptom burden.
CCC-affiliated palliative care units treat patients younger on average of five years. The lower proportion of considerably symptom-burdened cancer patients may be explained for CCC-affiliated palliative care units by younger patients with similar variance. One reason is the generally young patient clientele in CCCs. Internationally, the average age of cancer patients and in CCCs is low regarding 59–68 years (Bryson et al. 2010 ; Jung et al. 2022 ; Hui et al. 2010 ). Urban centers and metropolises imply a young demographic population. For another reason, comorbidities that may impact symptom burden occur primarily at an older age (Canoui-Poitrine et al. 2022 ; Williams et al. 2016 ).The interpretation of the data is challenging. The observation that in CCCs the number of considerably burdened patients is lower may also be an effect of timely integration of specialized palliative care alongside cancer treatment on the oncological wards positively impacting on the quality of symptom management. On the other hand, we see more patients dying in CCC palliative care units, even with a somewhat younger age structure. As CCCs apply novel cancer therapies and research-based procedures patients may often referred to inpatient specialized palliative care at a rather advanced stage of their disease (Hui et al. 2017 ). As a result, more cancer patients may die in the palliative care unit of a CCC despite a younger age structure. Palliative care units present very severe, complex cases as shown according to ECOG status in both: CCC-affiliated palliative care units and other palliative care units (Brunner et al. 2022 ). Every second cancer patient reports moderate or severe pain in German palliative care units. Internationally, the ratio of patients with moderate and severe pain is lower, on average 38% (32–43%) (van den Beuken-van Everdingen et al. 2016 ). The guideline "Palliative Care" of the NCCN refers to a high symptom burden, as a decisive factor to consult palliative care (Dans et al. 2022 ). Pain, depression, anxiety, and tension are increased reported by cancer patients in other palliative care units. That often occur as cluster (Öhlen et al. 2017 ; Stiel et al. 2014 ). Depressiveness and anxiety are pronounced, especially when the cancer has been diagnosed (Vogt et al. 2021 ).
CCC-affiliated palliative care units predominantly improve symptoms in considerably distressed patients. This is consistent with previous findings (Delgado-Guay et al. 2018 ). The difference between institutions in favor of CCC-affiliated palliative care units was shown for pain, anxiety, lack of appetite, as well as sleep quality (Jung et al. 2022 ). We show how CCC-affiliated palliative care units also reduce other distressing problems among cancer patients, such as nausea, vomiting, shortness of breath, constipation, wound care problems, depressive symptoms, tension, confusion, and problems in the care setting. CCCs understand their role in treating symptoms according to the latest scientific findings and sharing guidelines, standards, and recommendations. It is important to create, update, disseminate and use guidelines and Standard Operating Procedures for general and specialized palliative care. Relieving physical symptoms is also reflected in the Standard Operating Procedures developed by the Palliative Care Working Group in the CCC Network, where the largest proportion is symptom-based (Lödel et al. 2020 ; Ostgathe et al. 2017 ; Stachura et al. 2017 ). The Standard Operating Procedures are primarily focused on cancer patients because they were developed within the framework of the CCCs. They may also be beneficial for patients who suffer from other diseases than cancer in particular when no other Standard Operating Procedures are available. The use of a Standard Operating Procedure must be critically reflected in each individual case, since the pathophysiology of, e.g., symptoms can be very different and not all strategies can be transferred 1 to 1 to others, e.g., more careful administration of opioids in cardiac insufficiency (Gaertner et al. 2023 ).
However, not only physical symptoms should be considered in Standard Operating Procedures. Emotional problems such as and tension often occur (van den Beuken-van Everdingen et al. 2016 ), especially for younger patients (Abdelaal et al. 2023 ). So the working group members developed Standard Operating Procedures for depression (Schwartz et al. 2022 ) and anxiety (Hornemann et al. 2022 ). Psychological symptom burden, in particular anxiety, must be well documented to provide appropriate multiprofessional offers at an early stage. Besides the importance of the documentation of psychosocial symptoms, collaboration with psychooncology as soon as symptoms become apparent could benefit synergies (Zucca et al. 2015 ).
The findings focus on cancer patients. Nevertheless, the results may serve as a motivation for CCCs and non-CCCs to collaborate more and share knowledge to better taylor support and services for both groups.
Overall, the study shows that palliative inpatient care reduces symptom burden, independent of CCC-affiliated or other palliative care units. Providing palliative care that achieves consistent, all-encompassing symptom relief on a physical, psychological, social, and spiritual level is a way to enhance or maintain the patient's quality of life (Radbruch et al. 2020 ). All institution types satisfy the requirement of care. Both CCC-affiliated and other palliative care units improve symptom burden to a significant degree, especially in the physical, psychological, and social dimension. This is achieved among cancer patients even in CCC-affiliated palliative care units at a median shorter duration of care. The average length of care is similar in palliative care of NCCN (Brunner et al. 2022 ; Calton et al. 2016 ). Thus, palliative care reduces symptom distress effectively (Gomes et al. 2016 ), cost-effectively (Smith et al. 2014 ), and consequently regardless of place of care. CCCs are responsible for providing structures, information and training in palliative care to overcome hurdles (Anderson et al. 2022 ).
Besides the difference in symptom burden between CCC-affiliated and other palliative care units, the differences could also be caused due to other factors, such as the distribution of principal diagnoses. More research is needed to address this. Knowledge of the differences in symptom burden among patients in CCC-affiliated and other palliative care units could be helpful to improve the approach to symptom burden as well as the structures of palliative care for oncology patients in CCCs and other hospitals. It should raise the awareness that symptom burden relief in the context of palliative care makes a significant contribution to maintaining the quality of life of cancer patients.
The Registry does not contain institution-specific information, such as staff, beds or type and hence no comparison in regard to further structural data (e.g., staffing, multiprofessionality, independent or integrated department, hospital size) was possible and, therefore, possible structural confounders may be overseen. The group “other hospitals” includes general hospitals, and also university hospitals, and oncology centers. The data composition affects the interpretation of results. Also a misclassification bias or reporting bias may impact the data. The focus of the analysis is not on the patient’s diagnosis. But, we are clear about cancer diagnoses may differ between the settings as patients with rare cancers are preferably treated in specialized centers. More details are given in another publication (Brunner et al. 2022 ). The analysis rather pursues an overview of the patient data: physical, psychological, and social care situation. Spiritual aspects are not documented in the registry, although via "other problems". Nevertheless, physical and emotional problems can be well compared. Excessive demand seems to be an indirect indicator for a stressed family.
The patient datasets varies within each CCC (from 13 to 1438) and per documentation year. Technical, financial and human resources influence data quality and transmission of data sets to the registry. Documenter's subjective assessment, level of training, and professional group assignment distort data. Information may not always be complete in visits or admission conversations. Symptoms were assessed by proxies, reducing data quality. However, in many patients on the palliative care units due to cognitive impairment and increasing frailty due to the disease self-assessment is not possible. Proxy assessment is particularly challenging for patients who are nearing death, but also beneficial. First, it is simpler to identify, rank, and monitor goals for problem-solving. Second, it ensures complete data collection and raises awareness of general needs and symptoms (Campbell et al. 2022 ). For consistent data analysis, we decided to consider only data that was collected completely (both at the beginning and at the end of treatment) and exclude patient registrations with missing data regarding one or the other point in time. We assume that the main symptoms are included. But it is obvious that measurements of symptom burden relief in dying patients may not be comparable to measurements in patients discharged from the unit. This also distorts the results. Aspects of timing for palliative care integration in the palliative care units are not considered. Nonetheless, this analysis is the first multicenter analysis of patient data. Using a large sample, we prove and disprove findings from the literature and validate them with the additional calculated effect size. We are interested to conduct more multicenter studies to identify specifics in the care of critically ill patients among institutions and further improve care across all institution types.
The role of CCCs in the treatment of special patient collectives (younger cancer patients), timely integration and transferring knowledge to other areas is evident from the result, for example via Standard Operating Procedures. Furthermore, it is important to ensure general and specialized palliative care being systematically integrated in all hospitals and not left to oncological competence centers alone. Moreover, it shows to promote general palliative care in hospital institutions to better address physical and emotional symptoms and problems of care organization already during therapy. The results should be used in each institution to deepen the knowledge of patients' symptom burden and needs, and thus to improve treatment options with regard to symptoms, but also patient care, and to respond appropriately to patients' needs.
Data availability
Data are only available upon request.
Code availability
Not applicable.
Abdelaal M, Avery J, Chow R et al (2023) Palliative care for adolescents and young adults with advanced illness: a scoping review. Palliat Med. https://doi.org/10.1177/02692163221136160
Article Google Scholar
Anderson TM, Farrell MM, Moss G et al (2022) The perspectives of oncology healthcare providers on the role of palliative care in a comprehensive cancer center. BMC Palliat Care 21:148–154. https://doi.org/10.1186/s12904-022-01039-7
Berendt J, Stiel S, Simon ST et al (2016) Integrating palliative care into comprehensive cancer centers: consensus-based development of best practice recommendations. Oncologist 21(10):1241–1249. https://doi.org/10.1634/theoncologist.2016-0063
Berendt J, Stiel S, Nauck F et al (2017) Early palliative care: current status of integration within German comprehensive cancer centers. Support Care Cancer 25(8):2577–2580. https://doi.org/10.1007/s00520-017-3667-y
Brandts (2019) Innovating the outreach of comprehensive cancer centers. Mol Oncol 13(3):619–623. https://doi.org/10.1002/1878-0261.12451
Brunner S, Heckel M, Zenz D, Ostgathe C, Gahr S et al (2022) Health- and disease-related data of inpatients in palliative care units of the Comprehensive Cancer Centers and other hospitals in comparison - data from the Hospice and Palliative Care Register. Wien Med Wochenschr 172:172–180. https://doi.org/10.1007/s10354-021-00910-1
Bryson J, Coe G, Swami N et al (2010) Administrative outcomes five years after opening an acute palliative care unit at a comprehensive cancer center. J Palliat Med 13:559–565. https://doi.org/10.1089/jpm.2009.0373
Calton BA, Alvarez-Perez A, Portman DG et al (2016) The current state of palliative care for patients cared for at leading US cancer centers: the 2015 NCCN palliative care survey. J Natl Compr Cancer Netw 14:859–866. https://doi.org/10.6004/jnccn.2016.0090
Campbell R, Ju A, King MT, Rutherford C (2022) Perceived benefits and limitations of using patient-reported outcome measures in clinical practice with individual patients: a systematic review of qualitative studies. Qual Life Res 31:1597–1620. https://doi.org/10.1007/s11136-021-03003-z
Canoui-Poitrine F, Segaux L, Benderra M-A et al (2022) The prognostic value of eight comorbidity indices in older patients with cancer: the ELCAPA cohort study. Cancers 14:2236. https://doi.org/10.3390/cancers14092236
Cohen J (1988) Statistical power analysis for the behavioral sciences, 2nd edn. Lawrence Erlbaum Associates, New York
Google Scholar
Coym A, Ullrich A, Hackspiel LK et al (2020) Systematic symptom and problem assessment at admission to the palliative care ward–perspectives and prognostic impacts. BMC Palliat Care 19(1):1–11. https://doi.org/10.1186/s12904-020-00576-3
Gaertner J, Fusi-Schmidhauser T, Stock S et al (2023) Effect of opioids for breathlessness in heart failure: a systematic review and meta-analysis. Heart 109(14):1064–1071. https://doi.org/10.1136/heartjnl-2022-322074
Article CAS Google Scholar
Gomes B, Calanzani N, Curiale V, McCrone P, Higginson IJ (2016) Effectiveness and cost-effectiveness of home palliative care services for adults with advanced illness and their caregivers. Cochrane Database Syst Rev 2013(6):Cd007760. https://doi.org/10.1002/14651858.CD007760.pub2
Hornemann B, Müller T, Hentschel L et al (2022) SOP—Angst. Onkologie 28:1015–1021. https://doi.org/10.1007/s00761-022-01238-0
Hui D, Bruera E (2017) The Edmonton symptom assessment system 25 years later: past, present, and future developments. J Pain Symptom Manag 53:630–643. https://doi.org/10.1016/j.jpainsymman
Hui D, Elsayem A, Palla S et al (2010) Discharge outcomes and survival of patients with advanced cancer admitted to an acute palliative care unit at a comprehensive cancer center. J Palliat Med 13:49–57. https://doi.org/10.1001/jama.2010.258
Hui D, Cherny N, Latino N, Strasser F (2017) The “critical mass” survey of palliative care programme at ESMO designated centres of integrated oncology and palliative care. Ann Oncol 28:2057–2066. https://doi.org/10.1093/annonc/mdx280
Jung EH, Lee SW, Kim YJ et al (2022) Clinical outcomes of operating an acute palliative care unit at a comprehensive cancer center. JCO Oncol Pract 18:e1661–e1671. https://doi.org/10.1200/op.22.00163
Lödel S, Ostgathe C, Heckel M et al (2020) Standard Operating Procedures (SOPs) for Palliative Care in German Comprehensive Cancer Centers - an evaluation of the implementation status. BMC Palliat Care 19(1):1–9. https://doi.org/10.1186/s12904-020-00565-6
Lustbader D, Mudra M, Romano C et al (2017) The impact of a home-based palliative care program in an accountable care organization. J Palliat Med 20:23–28. https://doi.org/10.1089/jpm.2016.0265
Öhlén J, Russell L, Håkanson C et al (2017) Variations in care quality outcomes of dying people: latent class analysis of an adult national register population. J Pain Symptom Manag 53:13–24. https://doi.org/10.1016/j.jpainsymman.2016.08.006
Perpiñá-Galvañ J, Orts-Beneito N, Fernández-Alcántara M et al (2019) Level of burden and health-related quality of life in caregivers of palliative care patients. IJERPH 16:4806. https://doi.org/10.3390/ijerph16234806
Radbruch L, De Lima L, Knaul F et al (2020) Redefining palliative care-a new consensus-based definition. J Pain Symptom Manag 60:754–764. https://doi.org/10.1016/j.jpainsymman.2020.04.027
Schwartz J, Neukirchen M, Hornemann B et al (2022) SOP - Depression in der Palliativmedizin. Onkologie 28:931–936. https://doi.org/10.1007/s00761-022-01182-z
Smith S, Brick A, O’Hara S, Normand C (2014) Evidence on the cost and cost-effectiveness of palliative care: a literature review. Palliat Med 28(2):130–150. https://doi.org/10.1177/0269216313493466
Stiel S, Matthes ME, Bertram L et al (2010) Validation of the new version of the minimal documentation system (MIDOS) for patients in palliative care: the German version of the edmonton symptom assessment scale (ESAS). Schmerz 24:596–604. https://doi.org/10.1007/s00482-010-0972-5
Stiel S, Pollok A, Elsner F et al (2012) Validation of the symptom and problem checklist of the German Hospice and Palliative Care Evaluation (HOPE). J Pain Manag 43:593–605. https://doi.org/10.1016/j.jpainsymman.2011.04.021
Stiel S, Matthies DM, Seuß D et al (2014) Symptoms and problem clusters in cancer and non-cancer patients in specialized palliative care—is there a difference? J Pain Symptom Manag 48:26–35
Tipseankhum N, Tongprateep T, Forrester DA, Silpasuwun P (2016) Experiences of people with advanced cancer in home-based palliative care. PRIJNR 20:238–251. https://doi.org/10.1177/00302228211058307
Ullrich A, Ascherfeld L, Marx G et al (2017) Quality of life, psychological burden, needs, and satisfaction during specialized inpatient palliative care in family caregivers of advanced cancer patients. BMC Palliat Care 16:31. https://doi.org/10.1186/s12904-017-0206-z
van den Beuken-van Everdingen MH, Hochstenbach LM, Joosten EA, Tjan-Heijnen VC, Janssen DJ (2016) Update on prevalence of pain in patients with cancer: systematic review and meta-analysis. J Pain Symptom Manag 51:1070-1090.e1079. https://doi.org/10.1016/j.jpainsymman.2015.12.340
Vogt J, Beyer F, Sistermanns J et al (2021) Symptom burden and palliative care needs of patients with incurable cancer at diagnosis and during the disease course. Oncologist 26:e1058–e1065. https://doi.org/10.1002/onco.13751
Williams GR, Mackenzie A, Magnuson A et al (2016) Comorbidity in older adults with cancer. J Geriatr Oncol 7:249–257. https://doi.org/10.1016/j.jgo.2015.12.002
Zucca A, Sanson-Fisher R, Waller A et al (2015) Medical oncology patients: are they offered help and does it provide relief? J Pain Symptom Manag 50:436–444. https://doi.org/10.1016/j.jpainsymman.2015.04.018
Albreht T, Amati C, Angelastro A et al (2017) Integrated cancer control: the case for comprehensive cancer care networks (CCCN). Chapter 5. In: Albreht T, Kiasuwa R, Van den Bulcke M (eds) EUROPEAN guide on quality improvement in comprehensive cancer control, Ljubljana: National Institute of Public Health; Brussels: Scientific Institute, pp 1–27. https://www.iccp-portal.org/news/cancon-guide-and-policy-papers . Accessed 3 Mar 2023
Dans M, Kutner JS, Agarwal R et al (2022) NCCN clinical practice guidelines in oncology: palliative care. Version 1.2022; 2022
Delgado-Guay MO, Ferrer J, Ochoa J et al (2018) Characteristics and outcomes of advanced cancer patients who received palliative care at a public hospital compared with those at a comprehensive cancer center. J Palliat Med 21:678–685. https://doi.org/10.1089/jpm.2009.0166
Mori M, Parsons HA, De la Cruz M et al (2011) Changes in symptoms and inpatient mortality: a study in advanced cancer patients admitted to an acute palliative care unit in a comprehensive cancer center. J Palliat Med 14:1034–1041. https://doi.org/10.1089/jpm.2010.0544
Ostgathe C, Alt-Epping B, Golla H et al, Hospice and Palliative Care Evaluation (HOPE) Working Group (2011) Non-cancer patients in specialized palliative care in Germany: what are the problems? Palliat Med 25(2):148–152. https://doi.org/10.1177/0269216310385370
Ostgathe C, Thomas M, Berendt J (2017) Standard operating procedures (SOPs) for palliative care of patients in the network of German Comprehensive Cancer Centers (CCCs). Onkologe 23:211–212. https://doi.org/10.1007/s00761-017-0201-2
Schoffer O, Klinkhammer-Schalke M, Schmitt J (2022) WiZen-Studie: Überlebensvorteile bei Behandlung in zertifizierten Krebszentren. GGW 22(4):7–15
Stachura, P, Berendt J, Stiel S et al (2017) [Standard operating procedures (SOPs) for palliative care. Presence and relevance of palliative SOPs within the network of German Comprehensive Cancer Centers (CCCs) funded by the German Cancer Aid]. Der Schmerz 31(1):47–53. https://doi.org/10.1007/s00482-016-0132-7
World Health Organization, WHO (2020) Palliative care. https://www.who.int/news-room/fact-sheets/detail/palliative-care
Download references
Open Access funding enabled and organized by Projekt DEAL. We thank the German Cancer Aid [70113949] to support the Palliative Care Working Group of the CCC Network, the German Association for Palliative Medicine (DGP) and the steering group to approve data usage, SMART-Q GmbH, Daniel Zenz, to centralize data sets, and the institutions to submit their data to registry.
Author information
Julia Berendt and Sarah Brunner contributed equally.
Authors and Affiliations
Department of Palliative Medicine and Comprehensive Cancer Center, CCC Erlangen-EMN, University Hospital Erlangen Friedrich-Alexander-Universität Erlangen-Nürnberg, Krankenhausstraße 12, 91054, Erlangen, Germany
Julia Berendt, Sarah Brunner, Maria Heckel, Christoph Ostgathe & Susanne Gahr
Medical Informatics and Communication Center and Comprehensive Cancer Center, CCC Erlangen-EMN, University Hospital Erlangen Friedrich-Alexander-Universität Erlangen-Nürnberg, Erlangen, Germany
Sarah Brunner
Department of Palliative Medicine, West German Cancer Center, University Hospital Essen, University of Duisburg-Essen, Essen, Germany
Mitra Tewes
You can also search for this author in PubMed Google Scholar
Contributions
All authors contributed to the study conception and design. Data collection was performed by SB. Data analysis was done by JB, SB and MH. JB prepared all figures and tables with correction of CO. The first draft of the manuscript was written by JB, SB and SG, and all authors commented on previous versions of the manuscript. All authors read and approved the final manuscript.
Corresponding author
Correspondence to Julia Berendt .
Ethics declarations
Conflict of interest.
The centers in employment of the authors are funded by the German Cancer Aid. There are no other conflicts of interest.
Ethical approval
Ethical approval for the study was granted by the regional ethical review board of the Friedrich-Alexander-Universität (FAU) Erlangen-Nürnberg (application no.: 320_19 Bc).
Consent to participate
Not required.
Consent to publish
All authors approve the manuscript for publication.
Additional information
Publisher's note.
Springer Nature remains neutral with regard to jurisdictional claims in published maps and institutional affiliations.
Rights and permissions
Open Access This article is licensed under a Creative Commons Attribution 4.0 International License, which permits use, sharing, adaptation, distribution and reproduction in any medium or format, as long as you give appropriate credit to the original author(s) and the source, provide a link to the Creative Commons licence, and indicate if changes were made. The images or other third party material in this article are included in the article's Creative Commons licence, unless indicated otherwise in a credit line to the material. If material is not included in the article's Creative Commons licence and your intended use is not permitted by statutory regulation or exceeds the permitted use, you will need to obtain permission directly from the copyright holder. To view a copy of this licence, visit http://creativecommons.org/licenses/by/4.0/ .
Reprints and permissions
About this article
Berendt, J., Brunner, S., Heckel, M. et al. Symptom burden and relief in palliative care units of German Comprehensive Cancer Center and other hospitals. J Cancer Res Clin Oncol 150 , 160 (2024). https://doi.org/10.1007/s00432-023-05557-6
Download citation
Received : 03 July 2023
Accepted : 27 November 2023
Published : 27 March 2024
DOI : https://doi.org/10.1007/s00432-023-05557-6
Share this article
Anyone you share the following link with will be able to read this content:
Sorry, a shareable link is not currently available for this article.
Provided by the Springer Nature SharedIt content-sharing initiative
- Symptom burden
- Symptom relief
- Palliative care
- Find a journal
- Publish with us
- Track your research
Read our research on: Abortion | Podcasts | Election 2024
Regions & Countries
What the data says about abortion in the u.s..
Pew Research Center has conducted many surveys about abortion over the years, providing a lens into Americans’ views on whether the procedure should be legal, among a host of other questions.
In a Center survey conducted nearly a year after the Supreme Court’s June 2022 decision that ended the constitutional right to abortion , 62% of U.S. adults said the practice should be legal in all or most cases, while 36% said it should be illegal in all or most cases. Another survey conducted a few months before the decision showed that relatively few Americans take an absolutist view on the issue .
Find answers to common questions about abortion in America, based on data from the Centers for Disease Control and Prevention (CDC) and the Guttmacher Institute, which have tracked these patterns for several decades:
How many abortions are there in the U.S. each year?
How has the number of abortions in the u.s. changed over time, what is the abortion rate among women in the u.s. how has it changed over time, what are the most common types of abortion, how many abortion providers are there in the u.s., and how has that number changed, what percentage of abortions are for women who live in a different state from the abortion provider, what are the demographics of women who have had abortions, when during pregnancy do most abortions occur, how often are there medical complications from abortion.
This compilation of data on abortion in the United States draws mainly from two sources: the Centers for Disease Control and Prevention (CDC) and the Guttmacher Institute, both of which have regularly compiled national abortion data for approximately half a century, and which collect their data in different ways.
The CDC data that is highlighted in this post comes from the agency’s “abortion surveillance” reports, which have been published annually since 1974 (and which have included data from 1969). Its figures from 1973 through 1996 include data from all 50 states, the District of Columbia and New York City – 52 “reporting areas” in all. Since 1997, the CDC’s totals have lacked data from some states (most notably California) for the years that those states did not report data to the agency. The four reporting areas that did not submit data to the CDC in 2021 – California, Maryland, New Hampshire and New Jersey – accounted for approximately 25% of all legal induced abortions in the U.S. in 2020, according to Guttmacher’s data. Most states, though, do have data in the reports, and the figures for the vast majority of them came from each state’s central health agency, while for some states, the figures came from hospitals and other medical facilities.
Discussion of CDC abortion data involving women’s state of residence, marital status, race, ethnicity, age, abortion history and the number of previous live births excludes the low share of abortions where that information was not supplied. Read the methodology for the CDC’s latest abortion surveillance report , which includes data from 2021, for more details. Previous reports can be found at stacks.cdc.gov by entering “abortion surveillance” into the search box.
For the numbers of deaths caused by induced abortions in 1963 and 1965, this analysis looks at reports by the then-U.S. Department of Health, Education and Welfare, a precursor to the Department of Health and Human Services. In computing those figures, we excluded abortions listed in the report under the categories “spontaneous or unspecified” or as “other.” (“Spontaneous abortion” is another way of referring to miscarriages.)
Guttmacher data in this post comes from national surveys of abortion providers that Guttmacher has conducted 19 times since 1973. Guttmacher compiles its figures after contacting every known provider of abortions – clinics, hospitals and physicians’ offices – in the country. It uses questionnaires and health department data, and it provides estimates for abortion providers that don’t respond to its inquiries. (In 2020, the last year for which it has released data on the number of abortions in the U.S., it used estimates for 12% of abortions.) For most of the 2000s, Guttmacher has conducted these national surveys every three years, each time getting abortion data for the prior two years. For each interim year, Guttmacher has calculated estimates based on trends from its own figures and from other data.
The latest full summary of Guttmacher data came in the institute’s report titled “Abortion Incidence and Service Availability in the United States, 2020.” It includes figures for 2020 and 2019 and estimates for 2018. The report includes a methods section.
In addition, this post uses data from StatPearls, an online health care resource, on complications from abortion.
An exact answer is hard to come by. The CDC and the Guttmacher Institute have each tried to measure this for around half a century, but they use different methods and publish different figures.
The last year for which the CDC reported a yearly national total for abortions is 2021. It found there were 625,978 abortions in the District of Columbia and the 46 states with available data that year, up from 597,355 in those states and D.C. in 2020. The corresponding figure for 2019 was 607,720.
The last year for which Guttmacher reported a yearly national total was 2020. It said there were 930,160 abortions that year in all 50 states and the District of Columbia, compared with 916,460 in 2019.
- How the CDC gets its data: It compiles figures that are voluntarily reported by states’ central health agencies, including separate figures for New York City and the District of Columbia. Its latest totals do not include figures from California, Maryland, New Hampshire or New Jersey, which did not report data to the CDC. ( Read the methodology from the latest CDC report .)
- How Guttmacher gets its data: It compiles its figures after contacting every known abortion provider – clinics, hospitals and physicians’ offices – in the country. It uses questionnaires and health department data, then provides estimates for abortion providers that don’t respond. Guttmacher’s figures are higher than the CDC’s in part because they include data (and in some instances, estimates) from all 50 states. ( Read the institute’s latest full report and methodology .)
While the Guttmacher Institute supports abortion rights, its empirical data on abortions in the U.S. has been widely cited by groups and publications across the political spectrum, including by a number of those that disagree with its positions .
These estimates from Guttmacher and the CDC are results of multiyear efforts to collect data on abortion across the U.S. Last year, Guttmacher also began publishing less precise estimates every few months , based on a much smaller sample of providers.
The figures reported by these organizations include only legal induced abortions conducted by clinics, hospitals or physicians’ offices, or those that make use of abortion pills dispensed from certified facilities such as clinics or physicians’ offices. They do not account for the use of abortion pills that were obtained outside of clinical settings .
(Back to top)
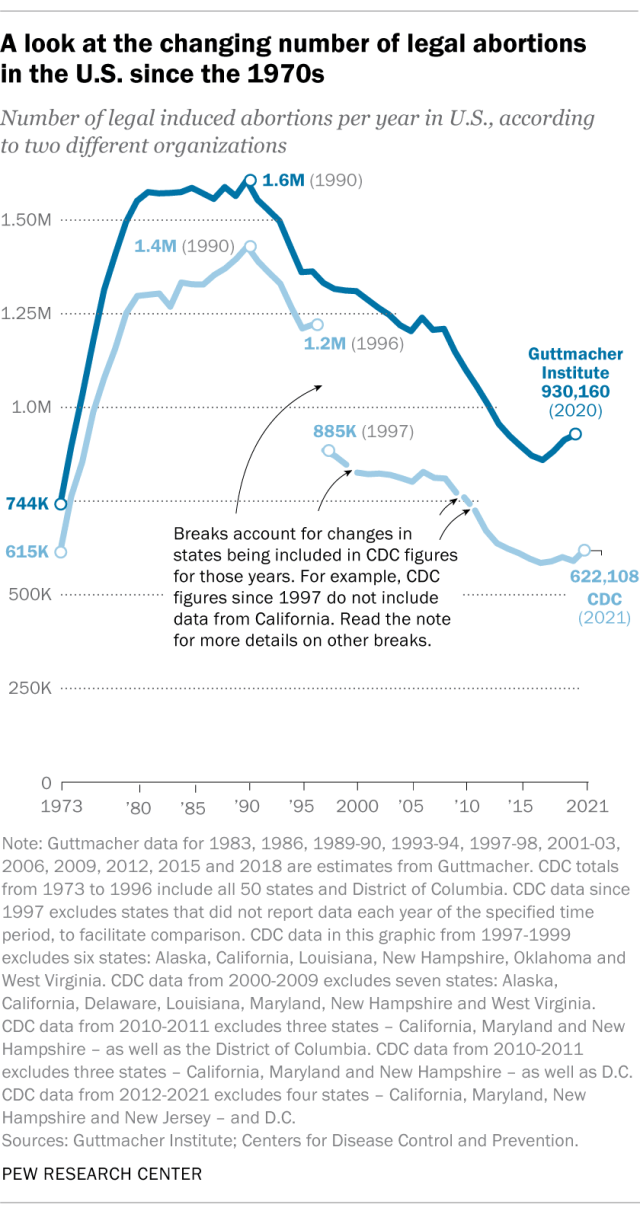
The annual number of U.S. abortions rose for years after Roe v. Wade legalized the procedure in 1973, reaching its highest levels around the late 1980s and early 1990s, according to both the CDC and Guttmacher. Since then, abortions have generally decreased at what a CDC analysis called “a slow yet steady pace.”
Guttmacher says the number of abortions occurring in the U.S. in 2020 was 40% lower than it was in 1991. According to the CDC, the number was 36% lower in 2021 than in 1991, looking just at the District of Columbia and the 46 states that reported both of those years.
(The corresponding line graph shows the long-term trend in the number of legal abortions reported by both organizations. To allow for consistent comparisons over time, the CDC figures in the chart have been adjusted to ensure that the same states are counted from one year to the next. Using that approach, the CDC figure for 2021 is 622,108 legal abortions.)
There have been occasional breaks in this long-term pattern of decline – during the middle of the first decade of the 2000s, and then again in the late 2010s. The CDC reported modest 1% and 2% increases in abortions in 2018 and 2019, and then, after a 2% decrease in 2020, a 5% increase in 2021. Guttmacher reported an 8% increase over the three-year period from 2017 to 2020.
As noted above, these figures do not include abortions that use pills obtained outside of clinical settings.
Guttmacher says that in 2020 there were 14.4 abortions in the U.S. per 1,000 women ages 15 to 44. Its data shows that the rate of abortions among women has generally been declining in the U.S. since 1981, when it reported there were 29.3 abortions per 1,000 women in that age range.
The CDC says that in 2021, there were 11.6 abortions in the U.S. per 1,000 women ages 15 to 44. (That figure excludes data from California, the District of Columbia, Maryland, New Hampshire and New Jersey.) Like Guttmacher’s data, the CDC’s figures also suggest a general decline in the abortion rate over time. In 1980, when the CDC reported on all 50 states and D.C., it said there were 25 abortions per 1,000 women ages 15 to 44.
That said, both Guttmacher and the CDC say there were slight increases in the rate of abortions during the late 2010s and early 2020s. Guttmacher says the abortion rate per 1,000 women ages 15 to 44 rose from 13.5 in 2017 to 14.4 in 2020. The CDC says it rose from 11.2 per 1,000 in 2017 to 11.4 in 2019, before falling back to 11.1 in 2020 and then rising again to 11.6 in 2021. (The CDC’s figures for those years exclude data from California, D.C., Maryland, New Hampshire and New Jersey.)
The CDC broadly divides abortions into two categories: surgical abortions and medication abortions, which involve pills. Since the Food and Drug Administration first approved abortion pills in 2000, their use has increased over time as a share of abortions nationally, according to both the CDC and Guttmacher.
The majority of abortions in the U.S. now involve pills, according to both the CDC and Guttmacher. The CDC says 56% of U.S. abortions in 2021 involved pills, up from 53% in 2020 and 44% in 2019. Its figures for 2021 include the District of Columbia and 44 states that provided this data; its figures for 2020 include D.C. and 44 states (though not all of the same states as in 2021), and its figures for 2019 include D.C. and 45 states.
Guttmacher, which measures this every three years, says 53% of U.S. abortions involved pills in 2020, up from 39% in 2017.
Two pills commonly used together for medication abortions are mifepristone, which, taken first, blocks hormones that support a pregnancy, and misoprostol, which then causes the uterus to empty. According to the FDA, medication abortions are safe until 10 weeks into pregnancy.
Surgical abortions conducted during the first trimester of pregnancy typically use a suction process, while the relatively few surgical abortions that occur during the second trimester of a pregnancy typically use a process called dilation and evacuation, according to the UCLA School of Medicine.
In 2020, there were 1,603 facilities in the U.S. that provided abortions, according to Guttmacher . This included 807 clinics, 530 hospitals and 266 physicians’ offices.
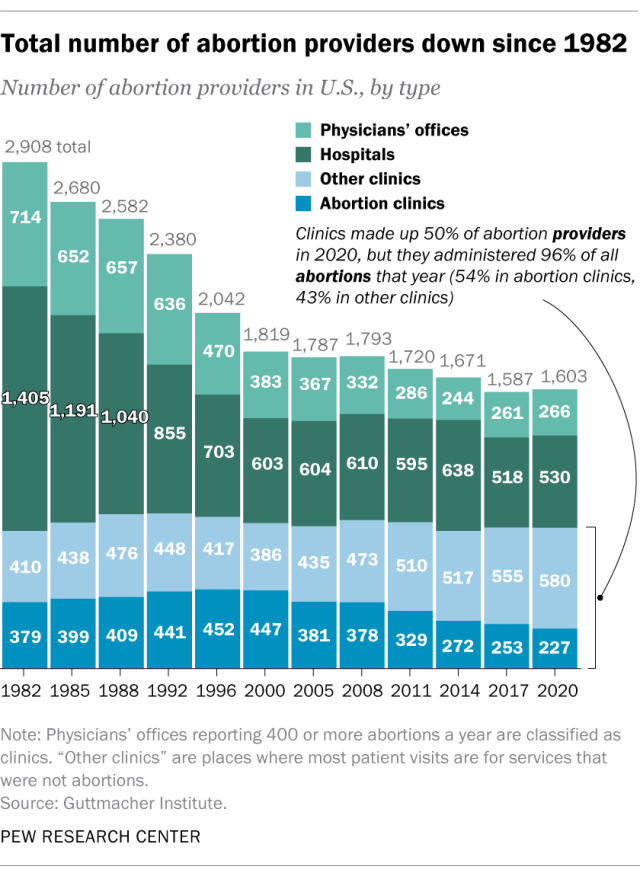
While clinics make up half of the facilities that provide abortions, they are the sites where the vast majority (96%) of abortions are administered, either through procedures or the distribution of pills, according to Guttmacher’s 2020 data. (This includes 54% of abortions that are administered at specialized abortion clinics and 43% at nonspecialized clinics.) Hospitals made up 33% of the facilities that provided abortions in 2020 but accounted for only 3% of abortions that year, while just 1% of abortions were conducted by physicians’ offices.
Looking just at clinics – that is, the total number of specialized abortion clinics and nonspecialized clinics in the U.S. – Guttmacher found the total virtually unchanged between 2017 (808 clinics) and 2020 (807 clinics). However, there were regional differences. In the Midwest, the number of clinics that provide abortions increased by 11% during those years, and in the West by 6%. The number of clinics decreased during those years by 9% in the Northeast and 3% in the South.
The total number of abortion providers has declined dramatically since the 1980s. In 1982, according to Guttmacher, there were 2,908 facilities providing abortions in the U.S., including 789 clinics, 1,405 hospitals and 714 physicians’ offices.
The CDC does not track the number of abortion providers.
In the District of Columbia and the 46 states that provided abortion and residency information to the CDC in 2021, 10.9% of all abortions were performed on women known to live outside the state where the abortion occurred – slightly higher than the percentage in 2020 (9.7%). That year, D.C. and 46 states (though not the same ones as in 2021) reported abortion and residency data. (The total number of abortions used in these calculations included figures for women with both known and unknown residential status.)
The share of reported abortions performed on women outside their state of residence was much higher before the 1973 Roe decision that stopped states from banning abortion. In 1972, 41% of all abortions in D.C. and the 20 states that provided this information to the CDC that year were performed on women outside their state of residence. In 1973, the corresponding figure was 21% in the District of Columbia and the 41 states that provided this information, and in 1974 it was 11% in D.C. and the 43 states that provided data.
In the District of Columbia and the 46 states that reported age data to the CDC in 2021, the majority of women who had abortions (57%) were in their 20s, while about three-in-ten (31%) were in their 30s. Teens ages 13 to 19 accounted for 8% of those who had abortions, while women ages 40 to 44 accounted for about 4%.
The vast majority of women who had abortions in 2021 were unmarried (87%), while married women accounted for 13%, according to the CDC , which had data on this from 37 states.
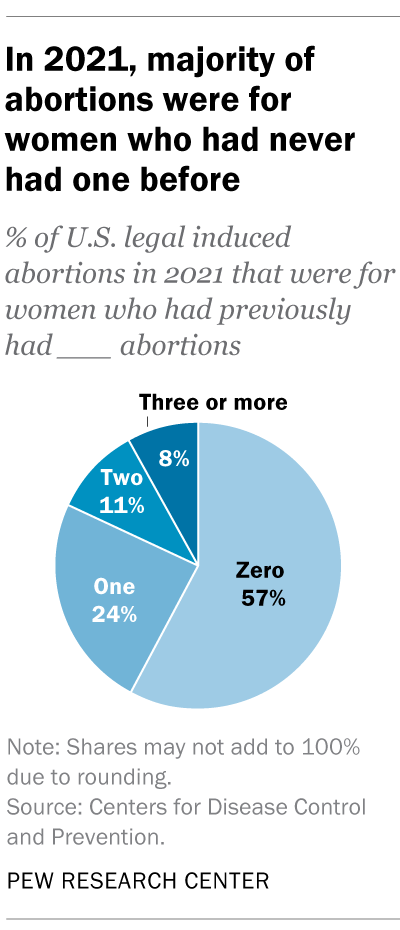
In the District of Columbia, New York City (but not the rest of New York) and the 31 states that reported racial and ethnic data on abortion to the CDC , 42% of all women who had abortions in 2021 were non-Hispanic Black, while 30% were non-Hispanic White, 22% were Hispanic and 6% were of other races.
Looking at abortion rates among those ages 15 to 44, there were 28.6 abortions per 1,000 non-Hispanic Black women in 2021; 12.3 abortions per 1,000 Hispanic women; 6.4 abortions per 1,000 non-Hispanic White women; and 9.2 abortions per 1,000 women of other races, the CDC reported from those same 31 states, D.C. and New York City.
For 57% of U.S. women who had induced abortions in 2021, it was the first time they had ever had one, according to the CDC. For nearly a quarter (24%), it was their second abortion. For 11% of women who had an abortion that year, it was their third, and for 8% it was their fourth or more. These CDC figures include data from 41 states and New York City, but not the rest of New York.
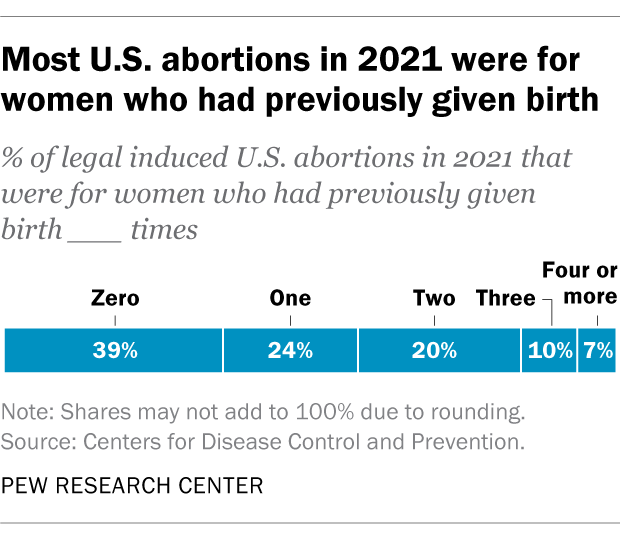
Nearly four-in-ten women who had abortions in 2021 (39%) had no previous live births at the time they had an abortion, according to the CDC . Almost a quarter (24%) of women who had abortions in 2021 had one previous live birth, 20% had two previous live births, 10% had three, and 7% had four or more previous live births. These CDC figures include data from 41 states and New York City, but not the rest of New York.
The vast majority of abortions occur during the first trimester of a pregnancy. In 2021, 93% of abortions occurred during the first trimester – that is, at or before 13 weeks of gestation, according to the CDC . An additional 6% occurred between 14 and 20 weeks of pregnancy, and about 1% were performed at 21 weeks or more of gestation. These CDC figures include data from 40 states and New York City, but not the rest of New York.
About 2% of all abortions in the U.S. involve some type of complication for the woman , according to an article in StatPearls, an online health care resource. “Most complications are considered minor such as pain, bleeding, infection and post-anesthesia complications,” according to the article.
The CDC calculates case-fatality rates for women from induced abortions – that is, how many women die from abortion-related complications, for every 100,000 legal abortions that occur in the U.S . The rate was lowest during the most recent period examined by the agency (2013 to 2020), when there were 0.45 deaths to women per 100,000 legal induced abortions. The case-fatality rate reported by the CDC was highest during the first period examined by the agency (1973 to 1977), when it was 2.09 deaths to women per 100,000 legal induced abortions. During the five-year periods in between, the figure ranged from 0.52 (from 1993 to 1997) to 0.78 (from 1978 to 1982).
The CDC calculates death rates by five-year and seven-year periods because of year-to-year fluctuation in the numbers and due to the relatively low number of women who die from legal induced abortions.
In 2020, the last year for which the CDC has information , six women in the U.S. died due to complications from induced abortions. Four women died in this way in 2019, two in 2018, and three in 2017. (These deaths all followed legal abortions.) Since 1990, the annual number of deaths among women due to legal induced abortion has ranged from two to 12.
The annual number of reported deaths from induced abortions (legal and illegal) tended to be higher in the 1980s, when it ranged from nine to 16, and from 1972 to 1979, when it ranged from 13 to 63. One driver of the decline was the drop in deaths from illegal abortions. There were 39 deaths from illegal abortions in 1972, the last full year before Roe v. Wade. The total fell to 19 in 1973 and to single digits or zero every year after that. (The number of deaths from legal abortions has also declined since then, though with some slight variation over time.)
The number of deaths from induced abortions was considerably higher in the 1960s than afterward. For instance, there were 119 deaths from induced abortions in 1963 and 99 in 1965 , according to reports by the then-U.S. Department of Health, Education and Welfare, a precursor to the Department of Health and Human Services. The CDC is a division of Health and Human Services.
Note: This is an update of a post originally published May 27, 2022, and first updated June 24, 2022.
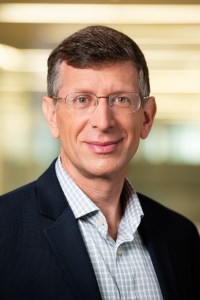
Sign up for our weekly newsletter
Fresh data delivered Saturday mornings
Key facts about the abortion debate in America
Public opinion on abortion, three-in-ten or more democrats and republicans don’t agree with their party on abortion, partisanship a bigger factor than geography in views of abortion access locally, do state laws on abortion reflect public opinion, most popular.
About Pew Research Center Pew Research Center is a nonpartisan fact tank that informs the public about the issues, attitudes and trends shaping the world. It conducts public opinion polling, demographic research, media content analysis and other empirical social science research. Pew Research Center does not take policy positions. It is a subsidiary of The Pew Charitable Trusts .
- Share full article
Advertisement
Supported by
What the Data Says About Pandemic School Closures, Four Years Later
The more time students spent in remote instruction, the further they fell behind. And, experts say, extended closures did little to stop the spread of Covid.

By Sarah Mervosh , Claire Cain Miller and Francesca Paris
Four years ago this month, schools nationwide began to shut down, igniting one of the most polarizing and partisan debates of the pandemic.
Some schools, often in Republican-led states and rural areas, reopened by fall 2020. Others, typically in large cities and states led by Democrats, would not fully reopen for another year.
A variety of data — about children’s academic outcomes and about the spread of Covid-19 — has accumulated in the time since. Today, there is broad acknowledgment among many public health and education experts that extended school closures did not significantly stop the spread of Covid, while the academic harms for children have been large and long-lasting.
While poverty and other factors also played a role, remote learning was a key driver of academic declines during the pandemic, research shows — a finding that held true across income levels.
Source: Fahle, Kane, Patterson, Reardon, Staiger and Stuart, “ School District and Community Factors Associated With Learning Loss During the COVID-19 Pandemic .” Score changes are measured from 2019 to 2022. In-person means a district offered traditional in-person learning, even if not all students were in-person.
“There’s fairly good consensus that, in general, as a society, we probably kept kids out of school longer than we should have,” said Dr. Sean O’Leary, a pediatric infectious disease specialist who helped write guidance for the American Academy of Pediatrics, which recommended in June 2020 that schools reopen with safety measures in place.
There were no easy decisions at the time. Officials had to weigh the risks of an emerging virus against the academic and mental health consequences of closing schools. And even schools that reopened quickly, by the fall of 2020, have seen lasting effects.
But as experts plan for the next public health emergency, whatever it may be, a growing body of research shows that pandemic school closures came at a steep cost to students.
The longer schools were closed, the more students fell behind.
At the state level, more time spent in remote or hybrid instruction in the 2020-21 school year was associated with larger drops in test scores, according to a New York Times analysis of school closure data and results from the National Assessment of Educational Progress , an authoritative exam administered to a national sample of fourth- and eighth-grade students.
At the school district level, that finding also holds, according to an analysis of test scores from third through eighth grade in thousands of U.S. districts, led by researchers at Stanford and Harvard. In districts where students spent most of the 2020-21 school year learning remotely, they fell more than half a grade behind in math on average, while in districts that spent most of the year in person they lost just over a third of a grade.
( A separate study of nearly 10,000 schools found similar results.)
Such losses can be hard to overcome, without significant interventions. The most recent test scores, from spring 2023, show that students, overall, are not caught up from their pandemic losses , with larger gaps remaining among students that lost the most ground to begin with. Students in districts that were remote or hybrid the longest — at least 90 percent of the 2020-21 school year — still had almost double the ground to make up compared with students in districts that allowed students back for most of the year.
Some time in person was better than no time.
As districts shifted toward in-person learning as the year went on, students that were offered a hybrid schedule (a few hours or days a week in person, with the rest online) did better, on average, than those in places where school was fully remote, but worse than those in places that had school fully in person.
Students in hybrid or remote learning, 2020-21
80% of students
Some schools return online, as Covid-19 cases surge. Vaccinations start for high-priority groups.
Teachers are eligible for the Covid vaccine in more than half of states.
Most districts end the year in-person or hybrid.
Source: Burbio audit of more than 1,200 school districts representing 47 percent of U.S. K-12 enrollment. Note: Learning mode was defined based on the most in-person option available to students.
Income and family background also made a big difference.
A second factor associated with academic declines during the pandemic was a community’s poverty level. Comparing districts with similar remote learning policies, poorer districts had steeper losses.
But in-person learning still mattered: Looking at districts with similar poverty levels, remote learning was associated with greater declines.
A community’s poverty rate and the length of school closures had a “roughly equal” effect on student outcomes, said Sean F. Reardon, a professor of poverty and inequality in education at Stanford, who led a district-level analysis with Thomas J. Kane, an economist at Harvard.
Score changes are measured from 2019 to 2022. Poorest and richest are the top and bottom 20% of districts by percent of students on free/reduced lunch. Mostly in-person and mostly remote are districts that offered traditional in-person learning for more than 90 percent or less than 10 percent of the 2020-21 year.
But the combination — poverty and remote learning — was particularly harmful. For each week spent remote, students in poor districts experienced steeper losses in math than peers in richer districts.
That is notable, because poor districts were also more likely to stay remote for longer .
Some of the country’s largest poor districts are in Democratic-leaning cities that took a more cautious approach to the virus. Poor areas, and Black and Hispanic communities , also suffered higher Covid death rates, making many families and teachers in those districts hesitant to return.
“We wanted to survive,” said Sarah Carpenter, the executive director of Memphis Lift, a parent advocacy group in Memphis, where schools were closed until spring 2021 .
“But I also think, man, looking back, I wish our kids could have gone back to school much quicker,” she added, citing the academic effects.
Other things were also associated with worse student outcomes, including increased anxiety and depression among adults in children’s lives, and the overall restriction of social activity in a community, according to the Stanford and Harvard research .
Even short closures had long-term consequences for children.
While being in school was on average better for academic outcomes, it wasn’t a guarantee. Some districts that opened early, like those in Cherokee County, Ga., a suburb of Atlanta, and Hanover County, Va., lost significant learning and remain behind.
At the same time, many schools are seeing more anxiety and behavioral outbursts among students. And chronic absenteeism from school has surged across demographic groups .
These are signs, experts say, that even short-term closures, and the pandemic more broadly, had lasting effects on the culture of education.
“There was almost, in the Covid era, a sense of, ‘We give up, we’re just trying to keep body and soul together,’ and I think that was corrosive to the higher expectations of schools,” said Margaret Spellings, an education secretary under President George W. Bush who is now chief executive of the Bipartisan Policy Center.
Closing schools did not appear to significantly slow Covid’s spread.
Perhaps the biggest question that hung over school reopenings: Was it safe?
That was largely unknown in the spring of 2020, when schools first shut down. But several experts said that had changed by the fall of 2020, when there were initial signs that children were less likely to become seriously ill, and growing evidence from Europe and parts of the United States that opening schools, with safety measures, did not lead to significantly more transmission.
“Infectious disease leaders have generally agreed that school closures were not an important strategy in stemming the spread of Covid,” said Dr. Jeanne Noble, who directed the Covid response at the U.C.S.F. Parnassus emergency department.
Politically, though, there remains some disagreement about when, exactly, it was safe to reopen school.
Republican governors who pushed to open schools sooner have claimed credit for their approach, while Democrats and teachers’ unions have emphasized their commitment to safety and their investment in helping students recover.
“I do believe it was the right decision,” said Jerry T. Jordan, president of the Philadelphia Federation of Teachers, which resisted returning to school in person over concerns about the availability of vaccines and poor ventilation in school buildings. Philadelphia schools waited to partially reopen until the spring of 2021 , a decision Mr. Jordan believes saved lives.
“It doesn’t matter what is going on in the building and how much people are learning if people are getting the virus and running the potential of dying,” he said.
Pandemic school closures offer lessons for the future.
Though the next health crisis may have different particulars, with different risk calculations, the consequences of closing schools are now well established, experts say.
In the future, infectious disease experts said, they hoped decisions would be guided more by epidemiological data as it emerged, taking into account the trade-offs.
“Could we have used data to better guide our decision making? Yes,” said Dr. Uzma N. Hasan, division chief of pediatric infectious diseases at RWJBarnabas Health in Livingston, N.J. “Fear should not guide our decision making.”
Source: Fahle, Kane, Patterson, Reardon, Staiger and Stuart, “ School District and Community Factors Associated With Learning Loss During the Covid-19 Pandemic. ”
The study used estimates of learning loss from the Stanford Education Data Archive . For closure lengths, the study averaged district-level estimates of time spent in remote and hybrid learning compiled by the Covid-19 School Data Hub (C.S.D.H.) and American Enterprise Institute (A.E.I.) . The A.E.I. data defines remote status by whether there was an in-person or hybrid option, even if some students chose to remain virtual. In the C.S.D.H. data set, districts are defined as remote if “all or most” students were virtual.
An earlier version of this article misstated a job description of Dr. Jeanne Noble. She directed the Covid response at the U.C.S.F. Parnassus emergency department. She did not direct the Covid response for the University of California, San Francisco health system.
How we handle corrections
Sarah Mervosh covers education for The Times, focusing on K-12 schools. More about Sarah Mervosh
Claire Cain Miller writes about gender, families and the future of work for The Upshot. She joined The Times in 2008 and was part of a team that won a Pulitzer Prize in 2018 for public service for reporting on workplace sexual harassment issues. More about Claire Cain Miller
Francesca Paris is a Times reporter working with data and graphics for The Upshot. More about Francesca Paris
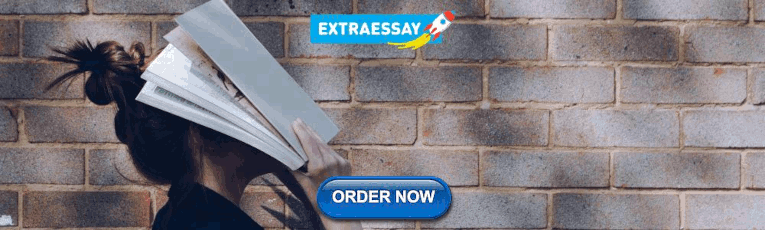
IMAGES
VIDEO
COMMENTS
Descriptive research classifies, describes, compares, and measures data. Meanwhile, analytical research focuses on cause and effect. For example, take numbers on the changing trade deficits between the United States and the rest of the world in 2015-2018. This is descriptive research.
Across the thousands of students we work with, descriptive writing (as opposed to critical or analytical writing) is an incredibly pervasive problem.In fact, it's probably the biggest killer of marks in dissertations, theses and research papers.So, in this post, we'll explain the difference between descriptive and analytical writing in straightforward terms, along with plenty of practical ...
Analytical writing is evaluative and critical. It seeks to go beyond the descriptive presentation of facts or details to the reader, and instead evaluates and investigates their significance. In other words, analytical writing demonstrates the 'why', 'how', and 'so what', interpreting the significance and meaning of the 'who ...
INTRODUCTION. In our previous article in this series, [ 1] we introduced the concept of "study designs"- as "the set of methods and procedures used to collect and analyze data on variables specified in a particular research question.". Study designs are primarily of two types - observational and interventional, with the former being ...
Research study design is a framework, or the set of methods and procedures used to collect and analyze data on variables specified in a particular research problem. ... Descriptive versus analytical studies. Descriptive (or nonanalytical) studies, as the name suggests, merely try to describe the data on one or more characteristics of a group of ...
Examples. Descriptive writing and analytical writing are often used in combination. In job application cover letters and essays for university admission, adding analytical text can provide context for otherwise unremarkable statements. Descriptive text: "I graduated from Bear University in 2020 with a B.S. in Chemistry and a cumulative GPA of 3 ...
Revised on June 22, 2023. Descriptive research aims to accurately and systematically describe a population, situation or phenomenon. It can answer what, where, when and how questions, but not why questions. A descriptive research design can use a wide variety of research methods to investigate one or more variables.
Descriptive vs. analytical studies. Study designs can be broadly divided into either descriptive or analytical. Descriptive studies describe characteristics such as patterns or trends. They answer the questions of what, who, where, and when, and they generate hypotheses. They include case reports and qualitative studies.
Another example is that someone might conduct analytical research to identify a study's gap. It presents a fresh perspective on your data. Therefore, it aids in supporting or refuting notions. Descriptive vs analytical research. Here are the key differences between descriptive research and analytical research:
Box 1. Descriptive Analysis Is a Critical Component of Research Box 2. Examples of Using Descriptive Analyses to Diagnose Need and Target Intervention on the Topic of "Summer Melt" Box 3. An Example of Using Descriptive Analysis to Evaluate Plausible Causes and Generate Hypotheses Box 4.
Here are some common methods of data analysis for descriptive research: Descriptive Statistics. ... This method involves comparing data across different groups or time periods to identify similarities and differences. Comparative analysis can help describe changes in attitudes or behaviors over time or differences between subgroups within a ...
Descriptive epidemiology assesses the burden and magnitude of health problems in a population, whereas analytical epidemiology identifies the causes and risk factors of health problems. This chapter provides the scopes, designs, data analytics approaches, ethical issues, and examples of various epidemiological studies.
Descriptive Studies. Characterize who, where, or when in relation to what (outcome) Person: characteristics (age, sex, occupation) of the individuals affected by the outcome. Place: geography (residence, work, hospital) of the affected individuals. Time: when events (diagnosis, reporting; testing) occurred.
Some common Descriptive Analytics Tools are as follows: Excel: Microsoft Excel is a widely used tool that can be used for simple descriptive analytics. It has powerful statistical and data visualization capabilities. Pivot tables are a particularly useful feature for summarizing and analyzing large data sets.
Abstract. In analytical observational studies, researchers try to establish an association between exposure (s) and outcome (s). Depending on the direction of enquiry, these studies can be directed forwards (cohort studies) or backwards (case-control studies). In this article, we examine the key features of these two types of studies.
Such choices are predicated on having made distinctions between qualitative methodology, methods, and analytic frames. In this article, we distinguish two qualitative research approaches widely used for descriptive studies: descriptive phenomenological and qualitative description.
In fact, descriptive research has many useful applications, and you probably rely on findings from descriptive research without even being aware that that is what you are doing. See Table 3.1 for examples. Explanatory research. The third type of research, explanatory research, seeks to answer "why" questions.
Qualitative research collects data qualitatively, and the method of analysis is also primarily qualitative. This often involves an inductive exploration of the data to identify recurring themes, patterns, or concepts and then describing and interpreting those categories. Of course, in qualitative research, the data collected qualitatively can ...
Descriptive employs observation and surveys; analytical uses statistical, mathematical, or computational techniques. Descriptive aims to identify patterns or trends, while analytical aims to establish causation. Descriptive research is often qualitative, whereas analytical can be both qualitative and quantitative.
Differences between descriptive research and analytical research. Analytical Research. Share ... There is a difference between Descriptive Research and Analytical Research as discussed here in ...
Descriptive press analytical research help used answer different models of question. Here's how they both help us understand the world.
This paper is in the following e-collection/theme issue: JMIR Theme Issue: COVID-19 Special Issue (2338) Telehealth and Telemonitoring (1461) Clinical Communication, Electronic Consultation and Telehealth (494) E-Health / Health Services Research and New Models of Care (383) Health Services Research (357) Focus Groups and Qualitative Research for Human Factors Research (689) Early Results from ...
Descriptive studies include case reports, case series reports, cross-sectional studies, surveillance studies, and ecological studies whereas analytical studies are either experimental or observational. Case-control and cohort studies are the type of observational studies out of which the latter is usually the prospective study.
The registry analysis provided data of patients in palliative care units (2014-2018). We analyzed characteristic and symptom-related data on 18 symptoms, with considerable symptom-burdened patients (moderate or severe). We followed a cancer (yes/no) and facility-specific descriptive analysis (f, %, μ, Mdn, SD, V, r) using SPSS.
The CDC says that in 2021, there were 11.6 abortions in the U.S. per 1,000 women ages 15 to 44. (That figure excludes data from California, the District of Columbia, Maryland, New Hampshire and New Jersey.) Like Guttmacher's data, the CDC's figures also suggest a general decline in the abortion rate over time.
The more time students spent in remote instruction, the further they fell behind. And, experts say, extended closures did little to stop the spread of Covid.