An official website of the United States government
Official websites use .gov A .gov website belongs to an official government organization in the United States.
Secure .gov websites use HTTPS A lock ( Lock Locked padlock icon ) or https:// means you've safely connected to the .gov website. Share sensitive information only on official, secure websites.
- Publications
- Account settings
- Advanced Search
- Journal List

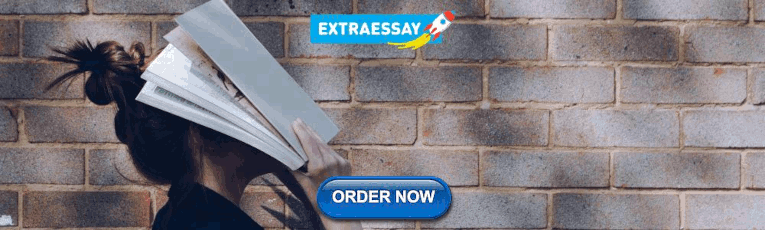
Cyberbullying as a Learned Behavior: Theoretical and Applied Implications
Christopher p barlett.
- Author information
- Article notes
- Copyright and License information
Received 2023 Jan 3; Revised 2023 Feb 2; Accepted 2023 Feb 3; Collection date 2023 Feb.
Licensee MDPI, Basel, Switzerland. This article is an open access article distributed under the terms and conditions of the Creative Commons Attribution (CC BY) license ( https://creativecommons.org/licenses/by/4.0/ ).
Cyberbullying perpetration has emerged as a world-wide societal issue. Interventions need to be continuously updated to help reduce cyberbullying perpetration. We believe that data derived from theory can best accomplish this objective. Here, we argue for the importance of learning theory to understand cyberbullying perpetration. The purpose of this manuscript is to firstly describe the various learning theories that are applicable to describe cyberbullying perpetration, such as social learning, operant conditioning, the general learning model, and others. Second, we delve into the Barlett Gentile Cyberbullying Model, which integrates learning postulates and distinguishes cyber from traditional bullying. Finally, we offer a learning perspective on interventions and future research.
Keywords: cyberbullying, learning, theory
1. Introduction
Technological innovation has paved the way for near instantaneous world-wide communication via the Internet. The adoption of the Internet, juxtaposed with (a) increased technology capabilities, (b) affordable software and hardware, and (c) accessibility, have changed nearly every sector in the industrialized world, such as education, medicine, banking, business, and others. As such, the Internet is used frequently by adults and youth alike. Recent survey data show that 97% of US youth [ 1 ] and 93% of US adults are online daily [ 2 ]. While most Internet behavior is likely unharmful, there are some who use the Internet for nefarious purposes, such as hacking, sending unwanted sexual depictions, and illegally downloading content. While continued empirical attention to these harmful online behaviors is needed, the purpose of the current paper will be on cyberbullying—defined as repeated unwanted and harmful behavior via online technology [ 3 ]. Results from a systematic review of the literature estimate the prevalence of cyberbullying perpetration to be between 6.3% and 32% [ 4 ].
Meta-analytic reviews have documented the correlations between myriad deleterious psychological variables (depression, anxiety, etc.) and cyberbullying perpetration [ 5 ]. It is imperative, therefore, that cyberbullying perpetration be reduced. One method to potentially decrease the frequency of cyberbullying perpetration is to better understand the variables and processes that predict its frequency to better inform intervention efforts. Results from several meta-analytic reviews suggest that cyberbullying perpetration-focused interventions are successful [ 6 , 7 , 8 , 9 , 10 , 11 , 12 ]. Although these meta-analyses differ in their scope, articles retrieved, publication date, effect size used, and other differences, the results all converge to suggest that cyberbullying perpetration interventions are effective.
The primary literature that was sampled and synthesized in cyberbullying intervention meta-analyses differ greatly in their theoretical perspective. For example, Media Heroes [ 13 ], iZ Hero [ 14 ], and a video intervention to reduce cyberbullying [ 15 ] all employ the theory of reasoned action/planned behavior in their lessons. Cyber-Friendly Schools [ 16 ] and Tabby [ 17 ] apply social ecological theory to cyberbullying prevention. Finally, ConRED [ 18 ] uses the theory of normalized social behavior to reduce cyberbullying perpetration. Overall, the application of existing theories to prevent cyberbullying is welcomed, and a recent burgeoning of empirical research that purports to predict cyberbullying perpetration and/or validate a cyberbullying intervention has adopted a wealth of theoretical perspectives. We contend here that many of the theoretical strides made in this literature and the research on intervention efficacy can be explained more holistically with learning theory. There are too few studies that have directly applied learning theory to understand cyberbullying perpetration, which negates using systematic literature review techniques to summarize the literature. Instead, our aim is to summarize the relevant literature that applies learning theory to cyberbullying perpetration. First, we will elucidate the learning theories relevant to cyberbullying perpetration. Second, we will apply learning theory to understand cyberbullying perpetration and extend those findings to interventions. Finally, we conclude with suggestions for future work. Our thesis is that cyberbullying is a learned behavior that becomes automated over time via experience and reinforcement. If our central claim is valid then, logically, it should follow that cyberbullying perpetration frequency can be reduced using similar learning postulates within an intervention context.
2. Learning Theory
Before delving into the evidence that cyberbullying can be explained from a learning perspective, it is imperative to understand learning theory. That is, what processes and variables are important for social behavior to be learned? By learning, we mean, “…changes in the behavior of an organism that result from regularities in the environment of the organism” ([ 19 ]; p. 631). Historical reviews have thoroughly documented the evolution of learning research—from theory focusing on a very few specific processes to more general theories that integrate multiple processes [ 20 ]. Although we will not present an exhaustive list of all learning theories, we will focus on the theories that have application to cyberbullying.
3. Domain-Specific Learning Theories Related to Cyberbullying Perpetration
Domain-specific theories refer to a set of theoretical orientations that utilize a narrow set of concepts and processes while omitting others [ 21 ]. As outlined by Gentile and Gentile [ 22 ], there are myriad domain-specific theories that each emphasize learning differently, such as classical conditioning, discriminant learning, emotional learning, and others. We believe that all these theories have relevance to explain cyberbullying; however, data applying some of these domain-specific theories to cyberbullying are scarce due to the inability to measure cyberbullying (or cyber-aggression) at the state level. Indeed, most cyberbullying researchers use self-report questionnaires to assess how frequently cyberbullying behaviors have occurred across a given time-frame [ 23 ]—a type of trait measure that limits the application of certain learning theories in this domain. For example, emotional learning posits how state-based emotions can moderate the encoding and retrieval of information in memory, attentional processes, transfer of knowledge, and other cognitive processes [ 22 ]. Emotions—due to their reliance on affective episodes that foster current bodily changes, arousal, and appraisals [ 24 ]—are conceptualized as state (not trait) processes. Therefore, any application of emotional learning to cyberbullying is difficult due to mismatching measurement issues (cyberbullying as a trait and emotions as a state). We will focus our review on the learning theories that have empirical support.
3.1. Operant Conditioning
As reviewed by Gentile and Gentile [ 22 ], the premise of operant conditioning is that behavior is shaped (learned) in response to reinforcement, punishment, and extinction processes. Reinforcement is focused on shaping behavior by either rewarding positive behaviors with some internal or external reward (e.g., giving a child money for good grades: positive reinforcement) or the removal of negative stimuli (e.g., allowing a child to not go to a boring movie for being nice to their siblings: negative reinforcement). In both cases, the goal is to increase a desired behavior. Punishment is focused on providing aversive consequences with the goal of stopping future negative behaviors, such as the removal of something positive after an unwanted behavior is enacted (e.g., giving a child a time out for hitting another child: negative punishment) or doing something negative as a consequence for a behavior (e.g., spanking a child for lying to their teacher: positive punishment). Finally, extinction occurs when the reinforcement or punishment no longer has an impact on shaping behavior (desired or unwanted). For example, taking away a toy after an unwanted behavior (negative punishment) is not likely effective for a 17-year-old teenager as it is for a 5-year-old child, but taking away a cellular phone is likely effective.
Empirical evidence suggests that cyberbullying perpetration can be explained via operant conditioning tenets. In a cross-sectional study of emerging adults, Barlett and Gentile [ 25 ] found that positive reinforcement by peers and family for harming others online positively correlated with self-reported cyberbullying perpetration—a finding also observed in a sample of Iranian adults [ 26 ]. In other words, if peers and family agree with and support cyberbullying actions, cyberbullying perpetration is likely. Conversely, research has shown that punishing youth for their cyberbullying others should curtail subsequent behavior. For instance, Legate et al. [ 27 ] sampled child–parent dyads and had youth complete measures of their cyberbullying behavior while the parents read a hypothetical vignette that described cyberbullying and responded to how they would address the cyberbullying in that vignette. Results showed that child cyberbullying behavior was negatively related to parent punishment in the hypothetical vignettes—as punishment increased, cyberbullying decreased. Moreover, research has shown that adolescent perceptions of informal sanctions (the likelihood that someone would be punished for cyberbullying others) negatively predicts cyberbullying perpetration [ 28 ].
Research focused on cyberbullying bystanders offers additional insight into the operant conditioning underpinnings of cyberbullying. Bystanders are those who are aware of cyberbullying and either do not intervene (termed a passive outsider), defend the victim (either online or offline), or reinforce the cyberbully (either online or offline) [ 29 ]. Longitudinal findings suggest that if a cyber-bystander is aggressive, then they are likely to engage in cyberbullying perpetration 8 months later [ 30 ], which is mediated by moral disengagement [ 31 ]. Several variables have been shown to predict one’s bystander role. Zhao et al. [ 32 ] showed that youth who have a history of cyberbullying others are more likely to be cyberbullying reinforcers or be uninvolved when witnessing other cyberbullying actions, whereas Van Cleemput et al. [ 33 ] showed that bystander youth who are empathic are likely to help and less likely to join the cyberbully.
3.2. Social Learning
In the infamous Bobo Doll Study [ 34 ] researchers showed that children were more likely to punch, kick, and sit on an inflated Bobo doll after they viewed a model (real or filmed) acting aggressively. In short, individuals learn by witnessing how others behave. Additional empirical studies showed that social learning was observed when the model was rewarded for their aggressive actions (compared to punished [ 35 ]). Parents, peers, the media, and other entities model myriad positive and negative behaviors; a paucity of research has examined these influences on youth cyberbullying in a single study, but each individual source of learning has been tested.
Focusing on peers, research has shown that social norms related to cyberbullying positively predict cyberbullying perpetration [ 36 , 37 ] and the willingness to join in cyberbullying others in a bystander role [ 38 ]. Indeed, meta-analytic findings confirm that social norms associated with cyberbullying correlate with cyberbullying perpetration [ 39 ]. However, much of this research assesses participant’s cyberbullying perpetration frequency and perception of social norms. One study showed a positive relationship between the participant’s and peer’s cyberbullying frequency [ 28 ]. These findings suggest that peer behavior and the perception of the acceptability of cyberbullying norms positively relate to cyberbullying perpetration.
There has been an abundance of literature focused on the relationship between myriad parenting variables and cyberbullying. First, parenting styles have been shown to predict cyberbullying. Katz et al. [ 40 ] showed that cyberbullying was the highest for youth whose parents were perceived as controlling and inconsistent. Moreover, cyberbullying is higher when the parents are classified as neglectful or authoritarian [ 41 ]. Unfortunately, these results should be interpreted with caution due to the correlational of the data; however, longitudinal research confirms these findings—Wave 2 participant alienation mediated the relationship between Wave 1 parental flexibility and Wave 3 cyberbullying perpetration [ 42 ]. Second, research is mixed regarding the role that positive parental communication has on youth cyberbullying perpetration. Some studies have shown that open and positive communication between parents and youth negatively correlates with cyberbullying perpetration [ 43 ], whereas other studies have not shown this relationship [ 44 ]. Negative or problematic communication between parents and youth has been consistently shown to positively predict cyberbullying, which is mediated by psychological distress, social norms, and problematic social networking time [ 45 ]. Finally, research is mixed regarding the parental monitoring of cyberbullying behaviors. When youth perceive their parents monitoring their Internet time too much (e.g., having set time parameters for Internet time, parents checking websites, applying online filters, etc.) cyberbullying perpetration increases [ 46 , 47 ]. However, when that monitoring is less extreme—simply asking about the things youth do online instead of checking websites—then cyberbullying perpetration significantly decreases [ 48 ]. We believe that these findings can be partially explained by parental trust—higher levels of trust likely foster better and less restrictive online monitoring to decrease cyberbullying. Research has suggested that trust is correlated with cyberbullying [ 49 ].
Finally, research has shown that media exposure predicts cyberbullying perpetration; however, the type of effect (direct vs. indirect) is mixed. Supporting the direct effect of media violence on cyberbullying perpetration, longitudinal data suggest that media violence exposure predicts later cyberbullying perpetration [ 50 , 51 ], which seems intuitive since (a) cyberbullying is highly correlated with bullying and aggression [ 5 ] and (b) media violence exposure predicts aggression [ 52 ]. However, from a social learning perspective, it is unclear whether media violence exposure is directly related to cyberbullying perpetration. Indeed, we are unaware of any media program, movie, game, etc., that depicts cyberbullying perpetration in a positive light, which suggests that it is more likely that media violence exposure predicts more general aggressive tendencies that may manifest online [ 53 ]. In support of this indirect effect prediction, Zhu et al. [ 54 ] showed that normative aggressive beliefs mediated the relationship between media violence exposure and cyberbullying. More work is needed to fully disentangle whether the media violence to cyberbullying relationship is direct, indirect, or both.
Overall, research from a social learning perspective has shown that cyberbullying is likely learned and reinforced from peers, parents, the media, and personal experiences. One caveat to this conclusion, however, is that there is a paucity of research investigating a direct social learning pathway from the source (parent, peer, media) to youth’s cyberbullying perpetration. For instance, a study in which parents and youth completed a measure of cyberbullying perpetration would allow for scholars to calculate the correlations between parent’s behavior (not the child’s perception of their behavior) and the child’s behavior. Future work is desperately needed in this area.
3.3. Cognitive Learning
Theory posits that cognitive schemas—mental representations of people, places, and objects—and scripts—mental representations of events [ 55 ]—are developed via experiences (actual and vicarious), which are believed to reside in long-term memory. The creation, potential modification, and development of associations between myriad scripts and schemas is a result of cognitive learning. Script theory, for example, posits that once schemas are primed via myriad methods (reading words, viewing or listening to media, thinking of an object), they cause spreading activation that creates a network of related schemas to be active, and if a threshold of schemas in that network are active then numerous scripts will be activated and evaluated to guide subsequent behavior [ 56 ]. According to this theory [ 22 ], learning occurs when knowledge structures—scripts, schemas, cognitive biases, attitudes—are automatized and readily available for use. We contend that cognitive learning—because of the vast number of array of variables encompassed—has high applicability to cyberbullying research. This theory contends that cognitive learning via schema, scripts, and attitudes ultimately develops one’s personality [ 22 ], and an extensive amount of research in the cyberbullying domain has shown that myriad personality variables correlate with cyberbullying perpetration. Indeed, research has shown that cyberbullying perpetration is correlated with several learned aggressive knowledge structures, including normative aggressive beliefs, trait anger, moral disengagement, and others [ 5 ].
The integrative cognitive model of trait anger [ 57 ] posits that the interpretation of a hostile situation will ultimately predict anger and subsequent aggression due to (a) an increase in ruminative attention or (b) the inability to suppress anger via lack of effortful control. Li et al. [ 58 ] applied this theory to explain the cognitive basis for why childhood maltreatment predicts cyberbullying perpetration and showed that hostile attribution biases and anger rumination mediated these effects. Subsequent studies have shown the mediating role of other cognitive biases and variables that explain cyberbullying. For example, Li et al. [ 59 ] showed that belief in a just world mediated the relationship between parent–child attachment and cyberbullying perpetration, and Fan et al. [ 60 ] showed that self-esteem mediated the relationship between narcissism and cyberbullying perpetration.
4. Integrative Learning Models Related to Cyberbullying Perpetration
Each individual domain-specific type of learning has its own merit and offers an interesting perspective on how social behaviors are learned. Furthermore, these, and other, learning theories are not in competition with each other, but, rather, may occur simultaneously in parallel with each other to compound the speed of acquisition and stability of the ensuing social behavior [ 22 ]. However, one criticism is that each offers a very narrow interpretation of how behavior is learned—each focusing on one specific process or mechanism while, at the very least, downplaying other mechanisms. Such limitations were one impetus for the development of a learning theory that integrated the myriad specific learning theories into a single comprehensive theory—the general learning model (GLM).
The general learning model is segmented into proximate (short-term) and distal (long-term) learning tenets [ 22 ]. The proximate GLM starts with personality (e.g., traits, genetics) and situational (e.g., the current environment) variables that either additively or interactively influence the sensation and then perception of a given stimulus. Sensation and subsequent perception are integral to the mental processing of elements in one’s social world, and can result in the discrimination of stimuli, classical conditioning processes, observational learning, and/or emotional encoding of stimuli. Therefore, a single initial exposure to a stimulus can instigate several specific learning processes that affect, and are affected by, one’s present internal state, which consists of inter-correlated affect and cognitions and physiological arousal. At this point in the model, multiple feedback loops exist to posit that the cognitive, arousal, and/or emotional experiences from stimuli reinforce the initial learning after stimulus exposure. Moreover, the internal state leads to higher-order appraisal and decision-making processes that eventually predict behavior. These processes start with an initial attribution about the social situation, and if one does not have the resources (time, motivation, cognitive energy) to devote to the environment, then impulsive behavior is likely, which may be highlighted via learned priming and heuristic processes. If one does have the resources to devote to an unsatisfactory appraisal, then re-appraisal processes are engaged to possibly change the initial attribution. Independent of whether re-appraisal is successful, thoughtful behavior is likely, which may be highlighted by cognitive learning processes.
The proximate general learning model incorporates myriad learning processes into a single theory, and research has shown that the GLM validly predicts myriad learned behaviors in the moment, such as helping behavior after prosocial media exposure [ 61 ]. Unfortunately, there is no research available to test the viability of proximate GLM processes extended to cyberbullying perpetration, due to measurement issues in cyberbullying discussed earlier. However, one can speculate that if an individual attacks another online for the first time, then the perpetrator will attend to and perceive selected elements regarding the cyber-attack (e.g., the content of what was typed, how many likes one received, etc.). This will likely cause changes to the internal state specific to cyberbullying, which may include: positive emotional outcomes from harming another and not being identified, positive reinforcement from others who share your opinions and sanction the cyber-attack germane to operant conditioning, the physiological arousal response of doing something harmful that maps onto classical conditioning, and others. Following this, GLM explicates that subsequent cyber-aggressive behaviors are likely in the short-term.
The general learning model also posits distal, or long-term, learning processes that predict the development of personality. Here, the GLM argues that continued exposure to social behaviors and the subsequent short-term learning postulates previously described will lead to changes to one’s personality through the development of several knowledge structures consistent with behavior. Specifically, continued exposure and practice with stimuli predict the development of cognitive-emotional constructs (i.e., attitudes and stereotypes), emotional constructs (i.e., affective traits, affective habituation), and cognitive constructs (i.e., beliefs and scripts), which yield the development of one’s personality. In other words, continued cyberbullying experiences are one possible impetus for the development and automatization of myriad cyberbullying and aggression-related scripts, schemas, biases, attitudes, etc., and the accumulation of these, and other, knowledge structures defines cyberbullying personality.
5. Cyberbullying Perpetration as a Learned Social Behavior
Again, our central thesis is that cyberbullying perpetration is a learned social behavior. However, before delving into the evidence to support this thesis, an important question must be answered: What is the importance of understanding cyberbullying through a learning lens? We have noted elsewhere [ 62 ] that the best way to prevent antisocial behavior is to understand the psychological processes and variables germane to that behavior so that intervention efforts can be armed with the best possible information in order to be most successful. While multiple theoretical perspectives offer insights into cyberbullying that can accomplish the goal of understanding cyberbullying perpetration, a learning perspective offers important theoretical insights. For example, if social learning and operant conditioning postulates can be extended to cyberbullying, then interventions that focus on stopping positive reinforcement of cyberbullying actions should undermine the learning of cyberbullying attitudes, beliefs, and cognitions that likely predict its frequency. Moreover, a learning perspective highlights the need for interventions focused on parental, peer, and school entities in addition to the child’s education to prevent future cyberbullying perpetration. These and other examples highlight how learning theory as applied to cyberbullying perpetration can offer a unique application to prevention.
Can a learning theory, such as GLM, explain cyberbullying perpetration? We believe that it can. Figure 1 displays how we believe that cyberbullying can be explained by the distal GLM [ 63 ]. Three important points are noteworthy regarding Figure 1 . First, GLM postulates can explain the development of cyberbullying through several learning processes and constructs. In Figure 1 we replaced the theoretical constructs of GLM depicted on the left with the labels of variables found in the literature on cyberbullying perpetration on the right (we denoted these additions in yellow for ease of readability). Second, there are still many questions remaining that have received little to no empirical attention. For instance, we are unaware of any research investigating the “chunking/encoding” learning that GLM classifies as a cognitive-behavioral construct, which we will elaborate on later. Third, it is likely that some of the learning processes elucidated in the GLM do not transfer onto cyberbullying well. For instance, GLM notes physical skill as a learning process, which dictates how continued practice with a behavior automatizes a behavior. Physical skill as a cognitive-behavioral construct is clearly applicable to myriad trained behaviors, such as driving a car or shooting a free throw in basketball; however, it is less clear how this transitions to online bullying.
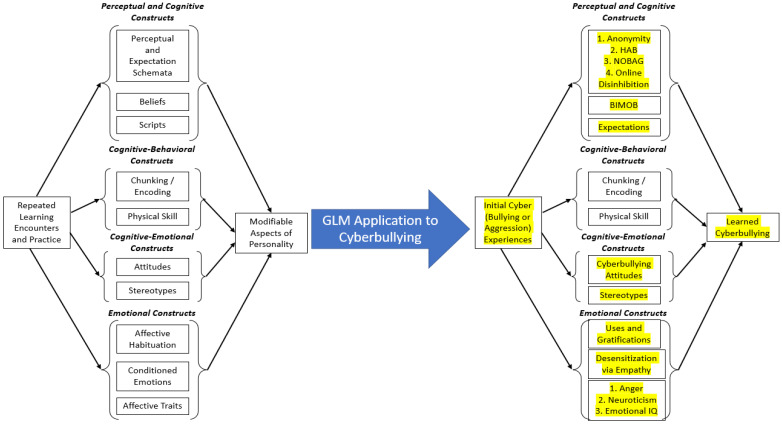
GLM applications to cyberbullying perpetration. Adapted with permission from Gentile et al. (2009). Copyright 2009 Sage Publications.
Evidence from empirical studies support the application of GLM to understand cyberbullying perpetration. We will elaborate on such transitions below:
5.1. Perceptual and Cognitive Constructs
There are three constructs within this section of GLM. The first is perceptual and expectation schemata, which describe cognitive knowledge structures regarding an individual’s behavior or behavior of others, and research has shown that cyberbullying is correlates with—and predicts—several of these knowledge structures. Perceptions of anonymity represent an individualized perceptual schema, in which individuals believe themselves to be less likely to be identified in online environments. Correlational [ 64 ] and longitudinal [ 65 ] findings show that cyberbullying is related to these perceptions. In an interesting study, Sticca and Perren [ 66 ] had youth read several hypothetical scenarios that described a peer who was excluded from a party, and the scenarios differed on whether the communication was delivered online or in person and if the message was anonymous or not. Participants then rated the scenario on aggression severity and humiliation, and results showed that the more humiliating and threatening scenario was when the rejection was online and anonymous. These findings can be best explained by online disinhibition theory [ 67 ], which posits that individuals are likely disinhibited in online (versus face-to-face) environments, which change the likelihood of aggressive behaviors (amongst other outcomes). For instance, the perceived anonymity afforded an online aggressor, juxtaposed with asynchronicity (the lack of real time interactions online), the minimization of status (absence of cues indicative of status or authority), and other constructs, likely increase cyberbullying [ 68 ]. Moreover, research has shown that cyberbullying perpetration correlates positively [ 69 , 70 , 71 ] with normative aggressive beliefs (NOBAG; the cognitive belief that aggression is acceptable after a perceived provocation [ 72 ]), and with cognitive interpretation of ambiguous situations as hostile [ 73 ], termed hostile attribution biases (HAB [ 74 ]). Moreover, GLM posits that several beliefs and scripts are important perceptual and cognitive constructs. One belief that has been shown to predict cyberbullying is the belief in the irrelevance of muscularity for online bullying (BIMOB). BIMOB is a belief theorized to be the consequence of cyber-aggression which emphasizes the common belief that anybody—no matter how physically small or weak—can harm others due to the online nature of cyberbullying [ 25 ]. Finally, research has shown that cyberbullying expectations (a behavioral script for the likelihood of future cyberbullying) is predicted by myriad variables that share variance with cyberbullying perpetration, such as moral disengagement, positive cyberbullying norms, and self-efficacy [ 75 ].
5.2. Cognitive-Behavioral Constructs
Gentile and Gentile [ 22 ] explicated several types of learned outcomes that are classified as cognitive-behavioral that form a function of continued practice. Evidence for learned cognitive-behavioral constructs can be seen by comparing experts to novices on some tasks. The translation of these GLM tenets to cyberbullying is less clear. While it is true that differences in conduct problems, prosocial behavior, and hyperactivity-inattention emerge between cyberbullies, cyber-victims, cyberbully-victims, and uninvolved youth [ 76 ], the conceptualization of an “expert cyberbully” is difficult. Moreover, the exact encoding and mental processing of cyberbullying-related information in the moment remains unclear. Therefore, we contend that the cognitive-behavioral portion of the distal GLM is in need of empirical attention and study.
5.3. Cognitive-Emotional Constructs
Attitudes and stereotypes are the two constructs that are labeled as cognitive-emotional according to GLM [ 22 ]. There is a rich social psychological literature showing that attitudes predict behavior [ 77 ], and research has shown that positive cyberbullying attitudes—evaluating cyberbullying as positive and/or justified—positively correlates with cyberbullying behavior [ 78 ]. For instance, using a correlational design with US adults, Doane et al. [ 79 ] showed that cyberbullying attitudes significantly predicted three types of cyberbullying (deception, malice, and public humiliation) indirectly through cyberbullying intentions. Moreover, several correlational studies show that cyberbullying attitudes directly predict cyberbullying perpetration [ 80 ], whereas other research findings suggest an indirect effect of cyberbullying attitudes to cyberbullying perpetration through behavioral intentions [ 81 ]. Finally, there is a paucity of research examining the relationship between stereotypes and cyberbullying; however, scholars have theorized about such a link. Indeed, Keum and Miller [ 82 ] described a model of online racism, which posits that online anonymity perceptions predict online disinhibition (described earlier) to predict in-group biases and stereotype formation. We are unaware of any primary research validating this model, and future work is needed.
5.4. Emotional Constructs
The final organizational section of the distal GLM consists of emotional constructs, which consist of several processes germane to changing personality as a function of repeated learning [ 22 ]. Affective habituation refers to the learned association between the behavior and emotional constructs (e.g., cyberbullying others is associated with excitement). Research employing the uses and gratifications framework [ 83 ] has shown that one possible motive for cyberbullying others is the entertainment and revenge that participants classified as cyberbully-victims (individuals who are both victimized and perpetrate online bullying) experience in harming others online [ 84 ]. Next, GLM includes conditioned emotions, which includes desensitization—the decreased emotional, physiological, and cognitive response to a stimulus [ 85 ], which can be operationalized via empathy [ 86 ]. Results from meta-analyses show that empathy is related to cyberbullying perpetration [ 5 ]. Finally, Gentile and Gentile [ 22 ] noted that affective traits—personality dimensions related to emotional expression—are conceptualized as an emotional construct within GLM. Research has shown that cyberbullying perpetration is correlated with several affective traits, including trait anger [ 87 ], neuroticism [ 88 ], and emotional intelligence [ 89 ].
6. Uniquely Predicting Cyberbullying
Despite the growing research support for GLM applications to cyberbullying, one caveat is that most learning theories are not specific to the online world—GLM included. Instead, both domain-specific and integrated learning theories can predict myriad antisocial behaviors, including cyberbullying, traditional bullying, and aggression. Indeed, one strength of GLM is its ability to describe and predict a plethora of behaviors, not just cyberbullying. Theoretical specification to online behavior is greatly needed in the cyberbullying domain because: (a) cyberbullying is specific to online mediums, making it theoretically distinct from traditional forms of bullying and aggression; and (b) cyberbullying interventions can be better informed with theory devoted specifically to online harm. Cyberbullying is unique because of the increased anonymity afforded to the online aggressor, the irrelevance of one’s physical stature, the non-physical nature of cyberbullying, the ability to have others see the online harm across the world at instantaneous speed, and other factors [ 90 ].
The theoretical gap in uniquely predicting cyberbullying incrementally from more traditional forms of bullying using learning-based underpinnings was filled with the validation of the Barlett Gentile Cyberbullying Model (BGCM [ 25 ]). Derived from the theoretical postulates of the general learning model, the BGCM posits that after youth cyber-attack another for the first time, they likely learn to perceive themselves as more anonymous online and learn that their physical stature is moot due to the online environment necessary for cyberbullying to occur (BIMOB). Continued cyber-attacks further develop and eventually automatize these perceptions and beliefs to form positive cyberbullying attitudes, which eventually become automatized to yield subsequent cyberbullying behavior. The importance of automatization and development highlight the learning emphasis of the BGCM. Figure 2 depicts the BGCM and highlights the learning stages that link initial cyber-aggressive actions to eventual development of cyberbullying propensities.
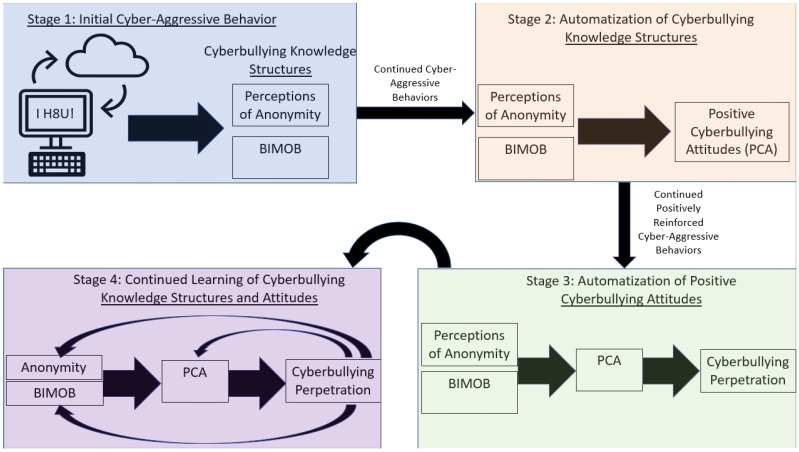
Barlett Gentile Cyberbullying Model.
The BGCM has received much empirical support. Indeed, research has shown that Wave 2 cyberbullying attitudes mediate the longitudinal relationships between Wave 1 anonymity and BIMOB with Wave 3 cyberbullying perpetration [ 91 ]. Importantly, BGCM postulates remain significant while controlling for traditional bullying perpetration [ 92 ]. This is important to show the incremental validity of the evidence that the BGCM predicts cyberbullying above and beyond traditional bullying, despite the high correlation between both forms of bullying perpetration [ 5 ]. Finally, research has shown that BGCM relationships are observed in countries across the world [ 93 ].
The core learning postulate of the BGCM is that each cyber-attack perpetrated is a learning trial, and longitudinal research has shown support for the learning tenets of BGCM—and by extension the GLM. For example, Barlett et al. [ 94 ] used a three-wave longitudinal design sampling Singaporean youth and found (a) strong stability over time for cyberbullying attitudes and cyberbullying perpetration, (b) early cyberbullying attitudes predicted later cyberbullying perpetration (consistent with BGCM), and (c) early cyberbullying perpetration predicted later cyberbullying attitudes (consistent with learning). In other words, cyberbullying attitudes reinforced cyberbullying perpetration, and, in turn, cyberbullying perpetration further reinforced cyberbullying attitudes. Moreover, Barlett and Kowalewski [ 95 ] used a four-wave longitudinal design with an emerging adult sample and showed that (a) Wave 1 anonymity perceptions and BIMOB predicted Wave 2 cyberbullying attitudes to predict Wave 3 cyberbullying behavior, consistent with BGCM, and (b) Wave 3 cyberbullying perpetration predicted Wave 4 anonymity perceptions and BIMOB, consistent with the learning theory. This is an important finding because this shows that continued cyberbullying perpetration—which was derived from BGCM tenets—further reinforces cyberbullying-related knowledge structures, supporting the feedback loop germane to BGCM processes.
7. Moderators in the Learning of Cyberbullying
The wealth of support for the BGCM validates the theoretical postulates and applied implications of this theory to understand and ultimately reduce cyberbullying. However, we do not believe that the current version of the BGCM depicted in Figure 1 is ubiquitous. Individual differences likely influence each observed variable in the BGCM framework and the underlying learning underpinnings of BGCM. Each will be briefly discussed.
First, research has shown substantial individual differences in cyberbullying perpetration. We have already elucidated several of these variables (in the cognitive learning section), but several other individual difference variables predict cyberbullying. For instance, meta-analytic results show sex differences in cyberbullying are moderated by age—males are more likely to cyberbully than females at older ages (emerging adult and above), but females are more likely to cyberbully than males at young ages (approximately 9–11 years old) [ 96 ]. Moreover, Barlett et al. [ 91 ] showed that emerging adult males had higher levels of anonymity perceptions and cyberbullying attitudes compared to emerging adult females. In addition to age and participant sex, other personality variables have been shown to moderate key relationships. Barlett et al. [ 97 ] used a cross-sectional study of US adults and showed that cyberbullying attitudes mediated the relationship between anonymity perceptions and cyberbullying perpetration (supporting BGCM); however, the relationship between anonymity and cyberbullying attitudes was moderated by dispositional fear of retaliation—a personality variable that measures the extent to which one is afraid of another’s retaliation and changes their behavior. Closer examination of this moderated effect suggests that people who perceive themselves as anonymous online are likely to endorse cyberbullying attitudes when they are fearful of another’s retaliation.
Second, technological moderators likely influence BGCM processes. Technological moderators are those individual differences that are specific to technology, such as time spent online and technology access. Indeed, research using a cross-sectional design showed that time spent online predicted BIMOB and anonymity perceptions, and that perceptions of technology access correlated with cyberbullying attitudes [ 98 ]. Moreover, self-efficacy related to the ability to cyberbully others (e.g., having the ability to create and send a computer virus) correlated with cyberbullying perpetration [ 99 ].
We want to explicate that there is no published research testing the moderating influence of any of these variables in BGCM processes. Future work is needed to uncover what variables moderate the learning explicated by BGCM; however, the general learning model theorizing posits that biological (e.g., genes, sex) and environmental modifiers (e.g., internet access, SES, living conditions) influence the learning of social behaviors [ 22 ]. This theorizing gives precedent to study myriad moderating variables within the context of BGCM learning—despite the paucity of work in this domain. Figure 3 displays a conceptual model of the role that various moderators may have on BGCM.
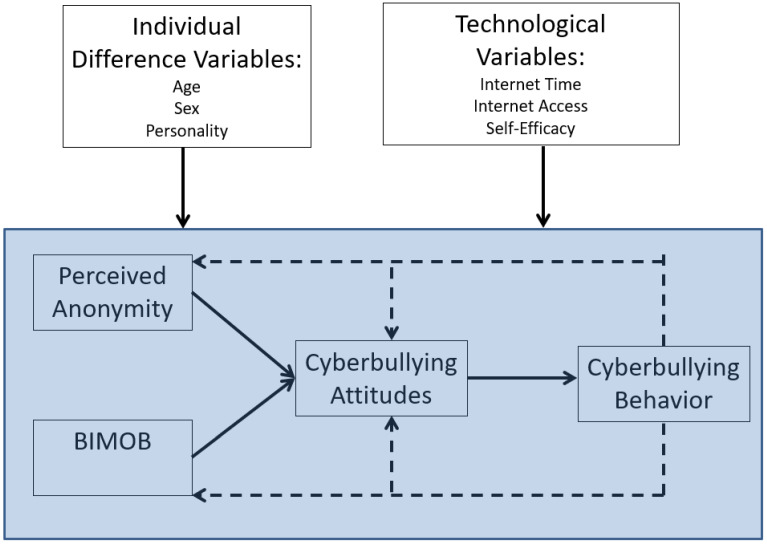
Conceptual Moderating Influence on BGCM Processes.
The moderating influence of myriad variables in the cyberbullying process has implications for intervention efforts. Within the context of cognitive learning, research has shown that school staff’s self-efficacy beliefs in intervening in a cyberbullying incident are moderated by school status—when self-efficacy beliefs are low, low-status staff are less likely to intervene compared to high-status staff [ 100 ]. In theory, if staff (or anyone) intervenes in a cyberbullying incident, then the perpetrator will likely not be positively reinforced for their online behavior to mitigate BGCM learning.
8. Intervention Applications
Results from several meta-analyses showed that interventions derived to reduce cyberbullying perpetration are successful [ 8 ]. These interventions vary widely in their focus, populations that the intervention is normed around, the degree of cyberbullying reduction, the study design, and others. Cioppa et al. [ 101 ] reviewed several cyberbullying interventions and rated each on ease of implementation (e.g., manual and training is available, instrumentation for evaluation is available) and scientific merit (e.g., having multiple sites, employing a control group, tailoring the program to a population based on pre-screening), and results showed great variability across the 12 evaluated interventions. Interestingly, the grading rubric for the scientific merit of these interventions omitted the application and use of theory. As we already articulated, many of the published interventions are derived—in part—based on theoretical underpinnings that vary greatly in their focus. Perhaps the reason for this omission is that most of the interventions do indeed utilize theory; however, meta-analytic work focused on parent-related interventions to reduce cyberbullying in youth found that the effect sizes are stronger (more effective interventions) when theory was used compared to effect sizes from studies that do not utilize theory [ 12 ].
Myriad theories have been applied to intervention lessons; however, very few utilize tenets specific to learning theory. We want to explicate that we are not implying intervention participants are not learning valuable skills that have ramifications for behavior, nor are we implying that intervention lessons are not using pedagogy consistent with learning. However, our perspective is that most cyberbullying interventions that use theory do not adequately utilize all learning theory postulates. Using the GLM as a guide ( Figure 1 ), we will discuss interventions that have utilized a portion of learning theory in their lessons—to great success.
GLM delineates that after repeated learning and practice, one class of learned constructs consists of perceptual and cognitive constructs, which include perceptual and expectation schemata, beliefs, and scripts. We argued that there is a rich literature in the cyberbullying domain that maps onto these learning constructs, such as anonymity, online disinhibition, hostile attribution biases, and normative aggressive beliefs. For instance, Media Heroes [ 13 ] and ConRED [ 18 ] include lessons that outline the legal and social consequences to perpetrating online harm—consequences that many youths may not be aware of. If a student in these, and other, programs learn of the consequences of cyberbullying others, then they may learn to expect those ramifications, which fits into GLM theorizing. Focused on anonymity and online disinhibition, emerging adult participants in the You’re Not Anonymous cyberbullying intervention group received training on how their online behaviors are not as anonymous as one would believe and had a significant decrease in self-reported anonymity perceptions compared to participants in the control condition, and anonymity perceptions predicted cyberbullying perpetration several months later [ 102 ]. Finally, although we are unaware of cyberbullying interventions that specifically target normative aggressive beliefs or hostile attribution biases directly, interventions that target aggression (more broadly) by helping youth identify and tackle aggression in their classroom and fostering a safe school environment through social skills training and group work have been shown to effectively curb cyberbullying perpetration for youth in the intervention program compared to youth not in the program (ViSC [ 103 ]).
Next, the cognitive-emotional constructs outlined in GLM include attitudes and stereotypes, which map onto cyberbullying attitudes and stereotypes. The video intervention to reduce cyberbullying created by Doane and colleagues [ 15 ] has implications for these GLM tenets. Emerging adults were presented with intervention materials that included (a) news stories of youth who committed suicide after being cyber-victimized, (b) information about what cyberbullying is and various risk and outcome factors to increase cyberbullying knowledge, and (c) acted vignettes of common cyberbullying events. Results showed that cyberbullying attitudes significantly decreased for participants in the intervention group compared to participants in the control group.
Finally, GLM posits that affective habituation, conditioned emotions, and affective traits are emotional constructs learned after repeated learning. We then argued that empathy, desensitization, and various other personality and motivational variables (e.g., revenge motivation, anger, neuroticism) can be organized within this class of variables. Many interventions (e.g., Media Heroes [ 13 ]; the KiVA program [ 104 ]) utilize empathy training as one, of many, key component to reduce cyberbullying.
Overall, several meta-analyses show the efficacy of cyberbullying interventions at reducing participant cyberbullying behavior, and the specific lessons across these interventions target processes delineated by GLM [ 22 ] as applicable to cyberbullying perpetration. However, much more work is needed. Here we offer some important recommendations that necessitate future work:
First, the BGCM and GLM both emphasize positively reinforced learning as an important mechanism in cyberbullying development. Therefore, it is prudent that interventions incorporate the entities that can reinforce or punish cyberbullying actions. Indeed, Barlett (2019) noted that cyberbullying prevention necessitates a multi-entity approach—parents, peers, the school, and the individual are all necessary to stop cyberbullying perpetration. Fortunately, many intervention programs include parents [ 18 ] and peers [ 105 ] in their approach. However, most of the lessons given to the larger community focus on cyberbullying knowledge (definitions, effects, motivations, etc.) without focusing on the reinforcement. We argue that interventions that target many interested groups are wonderful and cyberbullying knowledge is important. Perhaps additional information regarding how to appropriate reinforce or punish cyberbullying actions can help make these interventions more effective. However, this is an empirical question that requires future work.
Second, the evidence we presented to support the contention that cyberbullying is a learned social behavior suggests that cyberbullying interventions need to be administered to participants who are in a developmentally appropriate age range—old enough to understand the content of the intervention but young enough to begin to have access to online technologies and start using social media independent of their parents/guardians. For instance, Englander [ 106 ] showed that youth aged 8–11 started cyberbullying others, especially when they owned a cellular phone. Much thought will be needed to address the issue of participant age if such a cyberbullying curriculum can be tailored to elementary-school-aged youth.
Third, theories used to predict cyberbullying argue for myriad potential mediating variables that describe why cyberbullying perpetration occurs. Cyberbullying perpetration interventions target several of these, such as attitudes [ 15 ], empathy [ 13 ], aggressive behaviors [ 103 ], and others. However, several other potential mediators are left understudied. For example, we are unaware of any interventions that target emotional intelligence, BIMOB, stereotypes, or revenge or fun-seeking motives—all explicated by GLM ( Figure 1 ). Perhaps several of these variables change as a function of intervention lessons. For example, showing participants stories about youth who committed suicide after cyber-victimization incidents [ 15 ] may decrease fun-seeking motivations for cyberbullying others; however, this—and other variables—have not been tested. We understand that intervention specialists cannot measure every possible mediator, and we are not advocating for any one intervention or study to do that. Our position, though, is that other mediating variables that predict cyberbullying perpetration and can be the target of intervention lessons need to be studied in future work.
9. Future Work
While the notion of using learning theory and research to understand and ultimately reduce cyberbullying perpetration is not novel, there is surprisingly a few studies that have tested and validated such claims. There is a much room for future work, and we will elucidate some of these ideas here. First, we are unaware of any studies that have tested hypotheses important for exemplifying cyberbullying learning, such as: (a) the number of proximate GLM trials needed to develop cyberbullying knowledge structures in the distal GLM, (b) whether reinforcement/punishment moderates these learning tenets, and (c) the temporal ordering of variables within GLM to cause cyberbullying behavior to be learned. To answer these important questions, researchers would need to conduct a longitudinal study that samples youth participants who have never cyberbullied another nor been cyber-victimized at Wave 1 and then assess their cyberbullying-related knowledge structures and behavior in subsequent waves. Such a study design would identify youth who have (versus have not) engaged in cyberbullying behaviors and examine the longitudinal predictors of such learning. Such a study is procedurally questionable; however, if such a study can be conducted then many questions can be answered.
Second, we believe that the importance of reinforcement in the BGCM is an understated and understudied postulate that is desperately in need of future work. A paucity of research has examined the peer/family reinforcement positive correlation with cyberbullying [ 25 ], and much more longitudinal work is needed. Conversely, operant conditioning (and GLM/BGCM) posits that punishment may be helpful to reduce the likelihood of subsequent cyberbullying; however, we few studies have tested these predictions. We perceive punishment from parents/guardians as one method; however, punishment from peers, the victim’s retaliation, and oneself are all possible. For instance, the Media Heroes cyberbullying curriculum includes lessons specific to teaching youth about the legal consequences of cyberbullying [ 13 ]; however, while Media Heroes is effective at reducing cyberbullying, we are unaware of any research specifically delving into that component of the curriculum. Future work is needed to test whether informing participants about the legal ramifications is deterrent enough to reduce cyberbullying via operant conditioning tenets.
Finally, a state-based paradigm for measuring cyberbullying (or cyber-aggression) is desperately needed. To date, scientists have relied on vignettes to experimentally manipulate some aspect of a hypothetical cyberbullying event, which should not be interpreted as behavior. The challenge for scholars is to attempt to quantify online statements as hostile when those same statements are difficult to interpret given the lack of tone, sarcasm, emphasis, etc., that is common across online communication [ 107 ]. Research tools are getting closer to accomplishing this goal. For instance, Rezvani and Beheshti [ 108 ] validated computer software to detect cyberbullying, and future work should attempt to utilize these innovative programs to measure cyberbullying behavior in the moment.
10. Overall Conclusions
Scholars, school administrators, teachers, doctors, parents, and school pupils all recognize that cyberbullying perpetration and victimization are important topics. The purpose of the current manuscript was to argue that cyberbullying can be explained using learning theory—the general learning model and Barlett Gentile cyberbullying model. However, we also aimed to highlight the need for continued research that applies these, and other, theories to cyberbullying. Overall, we hope that a better understanding of the processes germane to cyberbullying can help guide intervention efforts that successfully reduce cyberbullying perpetration.
Conflicts of Interest
The author declares no conflict of interest.
Funding Statement
This research received no external funding.
Disclaimer/Publisher’s Note: The statements, opinions and data contained in all publications are solely those of the individual author(s) and contributor(s) and not of MDPI and/or the editor(s). MDPI and/or the editor(s) disclaim responsibility for any injury to people or property resulting from any ideas, methods, instructions or products referred to in the content.
- 1. Vogels E.A., Gelles-Watnick R., Massarat N. Teens, Social Media and Technology. 2022. [(accessed on 3 January 2023)]. Available online: https://www.pewresearch.org/internet/2022/08/10/teens-social-media-and-technology-2022/
- 2. Pew Research Center Internet/Broadband Fact Sheet. 2021. [(accessed on 3 January 2023)]. Available online: https://www.pewresearch.org/internet/fact-sheet/internet-broadband/
- 3. Smith P.K., Mahdavi J., Cavalho M., Fisher S., Russell S., Tippett N. Cyberbullying: Its nature and impact in secondary school pupils. J. Child Psychol. Psychiatry. 2008;49:376–385. doi: 10.1111/j.1469-7610.2007.01846.x. [ DOI ] [ PubMed ] [ Google Scholar ]
- 4. Zhu C., Huang S., Evans R., Zhang W. Cyberbullying among adolescents and children: A comprehensive review of the global situation, risk factors, and preventive measures. Front. Public Health. 2021;9:634909. doi: 10.3389/fpubh.2021.634909. [ DOI ] [ PMC free article ] [ PubMed ] [ Google Scholar ]
- 5. Kowalski R.M., Giumetti G.W., Schroeder A.N., Lattanner M.R. Bullying in the digital age: A critical review and meta-analysis of cyberbullying research among youth. Psychol. Bull. 2014;140:1073–1137. doi: 10.1037/a0035618. [ DOI ] [ PubMed ] [ Google Scholar ]
- 6. Chen Q., Chan K.L., Guo S., Chen M., Lo C.K., Ip P. Effectiveness of digital health interventions in reducing bullying and cyberbullying: A meta-analysis. Trauma Violence Abus. 2022 doi: 10.1177/15248380221082090. in press . [ DOI ] [ PubMed ] [ Google Scholar ]
- 7. Fraguas D., Diaz-Caneja C.M., Ayora M., Duran-Cutilla M., Abregu-Crespo R., Ezquiaga-Bravo I., Martin-Babarro J., Arango C. Assessment of school anti-bullying interventions: A meta-analysis of randomized clinical trials. JAMA Pediatr. 2021;175:44–55. doi: 10.1001/jamapediatrics.2020.3541. [ DOI ] [ PMC free article ] [ PubMed ] [ Google Scholar ]
- 8. Gaffney H., Farrington D.P., Espelage D.L., Ttofi M.M. Are cyberbullying intervention and prevention programs effective? A systematic and meta-analytical review. Aggress. Violent Behav. 2019;45:134–153. doi: 10.1016/j.avb.2018.07.002. [ DOI ] [ Google Scholar ]
- 9. Lan M., Law N., Pan Q. Effectiveness of anti-cyberbullying educational programs: A socio-ecologically grounded systematic review and meta-analysis. Comput. Hum. Behav. 2022;130:107200. doi: 10.1016/j.chb.2022.107200. [ DOI ] [ Google Scholar ]
- 10. Ng E.D., Chua J.Y.X., Shorey S. The effectiveness of educational interventions on traditional bullying and cyberbullying among adolescents: A systematic review and meta-analysis. Trauma Violence Abus. 2022;23:132–151. doi: 10.1177/1524838020933867. [ DOI ] [ PubMed ] [ Google Scholar ]
- 11. Polanin J.R., Espelage D.L., Grotpeter J.K., Ingram K., Michaelson L., Spinney E., Valido A., Torgal C., Robinson L. A systematic review and meta-analysis of interventions to decrease cyberbullying perpetration and victimization. Prev. Sci. 2022;23:439–454. doi: 10.1007/s11121-021-01259-y. [ DOI ] [ PMC free article ] [ PubMed ] [ Google Scholar ]
- 12. Wang L., Jiang S. Effectiveness of parent-related interventions on cyberbullying among adolescents: A systematic review and meta-analysis. Trauma Violence Abus. 2022 doi: 10.1177/15248380221137065. in press . [ DOI ] [ PubMed ] [ Google Scholar ]
- 13. Schultze-Krumbholz A., Schultze M., Zagorscak P., Wölfer R., Scheithauer H. Feeling cybervictims’ pain—The effect of empathy training on cyberbullying. Aggress. Behav. 2016;42:147–156. doi: 10.1002/ab.21613. [ DOI ] [ PubMed ] [ Google Scholar ]
- 14. Liau A.K., Park Y., Gentile D.A., Katna D.P., Tan C.H.A., Khoo A. iZ HERO adventure: Evaluating the effectiveness of a peer-mentoring and transmedia cyberwellness program for children. Psychol. Pop. Media Cult. 2017;6:326–337. doi: 10.1037/ppm0000094. [ DOI ] [ Google Scholar ]
- 15. Doane A.N., Kelley M.L., Pearson M.R. Reducing cyberbullying: A theory of reasoned action-based video prevention program for college students. Aggress. Behav. 2016;42:136–146. doi: 10.1002/ab.21610. [ DOI ] [ PubMed ] [ Google Scholar ]
- 16. Cross D., Shaw T., Hadwen K., Cardoso P., Slee P., Roberts C., Thomas L., Barnes A. Longitudinal impact of the Cyber Friendly Schools Program on adolescents’ cyberbullying behavior. Aggress. Behav. 2016;42:166–180. doi: 10.1002/ab.21609. [ DOI ] [ PubMed ] [ Google Scholar ]
- 17. Sorrentino A., Baldry A.C., Farrington D.P. The efficacy of the Tabby improved prevention and intervention program in reducing cyberbullying and cybervictimization among students. Int. J. Environ. Res. Public Health. 2018;15:2536. doi: 10.3390/ijerph15112536. [ DOI ] [ PMC free article ] [ PubMed ] [ Google Scholar ]
- 18. Ortega-Ruiz R., Del Rey R., Casas J.A. Knowing, building and living together on Internet and social networks: The ConRED cyberbullying prevention program. Int. J. Confl. Violence. 2012;6:302–312. [ Google Scholar ]
- 19. De Houwer J., Barnes-Holmes D., Moors A. What is learning? On the nature and merits of a functional definition of learning. Psychon. Bull. Rev. 2013;20:631–642. doi: 10.3758/s13423-013-0386-3. [ DOI ] [ PubMed ] [ Google Scholar ]
- 20. Illeris K. An overview of the history of learning theory. Eur. J. Educ. 2018;53:86–101. doi: 10.1111/ejed.12265. [ DOI ] [ Google Scholar ]
- 21. Carnagey N.L., Anderson C.A. Theory in the study of media violence: The General Aggression Model. In: Gentile D.A., editor. Media Violence and Children. Praeger Publishers; Westport, CT, USA: 2003. pp. 87–105. [ Google Scholar ]
- 22. Gentile D.A., Gentile J.R. Learning from Video Games (and Everything Else): The General Learning Model. Cambridge University Press; Cambridge, UK: 2022. [ Google Scholar ]
- 23. Chun J., Lee J., Kim J., Lee S. An international systemic review of cyberbullying measurements. Comput. Hum. Behav. 2020;113:106485. doi: 10.1016/j.chb.2020.106485. [ DOI ] [ Google Scholar ]
- 24. Mulligan K., Scherer K.R. Toward a working definition of emotion. Emot. Rev. 2012;4:345–357. doi: 10.1177/1754073912445818. [ DOI ] [ Google Scholar ]
- 25. Barlett C.P., Gentile D.A. Attacking others online: The formation of cyber-bullying in late adolescence. Psychol. Pop. Media Cult. 2012;1:130–135. doi: 10.1037/a0028113. [ DOI ] [ Google Scholar ]
- 26. Shadmanfaat S.M., Howell C.J., Muniz C.N., Cochran J.K., Kabiri S., Fontaine E.M. Cyberbullying perpetration: An empirical test of social learning theory in Iran. Deviant Behav. 2020;41:278–293. doi: 10.1080/01639625.2019.1565513. [ DOI ] [ Google Scholar ]
- 27. Legate N., Weinstein N., Przybylski A.K. Parenting strategies and adolescents’ cyberbullying behaviors: Evidence from a preregistered study of parent-child dyads. J. Youth Adolesc. 2019;48:399–409. doi: 10.1007/s10964-018-0962-y. [ DOI ] [ PubMed ] [ Google Scholar ]
- 28. Hinduja S., Patchin J.W. Social influences on cyberbullying behaviors among middle and high school students. J. Youth Adolesc. 2013;42:711–722. doi: 10.1007/s10964-012-9902-4. [ DOI ] [ PubMed ] [ Google Scholar ]
- 29. Sarmiento A., Herrera-López M., Zych I. Is cyberbullying a group process? Online and offline bystanders of cyberbullying act as defenders, reinforcers and outsiders. Comput. Hum. Behav. 2019;99:328–334. doi: 10.1016/j.chb.2019.05.037. [ DOI ] [ Google Scholar ]
- 30. Orue I., Fernández-González L., Machimbarrena J.M., González-Cabrera J., Calvete E. Bidirectional relationships between cyberbystanders’ roles, cyberbullying perpetration, and justification of violence. Youth Soc. 2021 doi: 10.1177/0044118X211053356. [ DOI ] [ Google Scholar ]
- 31. Luo A., Bussey K. Mediating role of moral disengagement in the perpetration of cyberbullying by victims and bystanders. J. Adolesc. 2022;94:1142–1149. doi: 10.1002/jad.12092. [ DOI ] [ PubMed ] [ Google Scholar ]
- 32. Zhao Y., Chu X., Rong K. Cyberbullying experience and bystander behavior in cyberbullying incidents: The serial mediating roles of perceived incident severity and empathy. Comput. Hum. Behav. 2023;138:107484. doi: 10.1016/j.chb.2022.107484. [ DOI ] [ Google Scholar ]
- 33. Van Cleemput K., Vandebosch H., Pabian S. Personal characteristics and contextual factors that determine “helping,” “joining in”, and “doing nothing” when witnessing cyberbullying. Aggress. Behav. 2014;40:383–396. doi: 10.1002/ab.21534. [ DOI ] [ PubMed ] [ Google Scholar ]
- 34. Bandura A., Ross D., Ross S.A. Imitation of film-mediated aggressive models. J. Abnorm. Soc. Psychol. 1963;66:3–11. doi: 10.1037/h0048687. [ DOI ] [ PubMed ] [ Google Scholar ]
- 35. Bandura A., Ross D., Ross S.A. Vicarious reinforcement and imitative learning. J. Abnorm. Soc. Psychol. 1963;67:601–607. doi: 10.1037/h0045550. [ DOI ] [ PubMed ] [ Google Scholar ]
- 36. Dang J., Liu L. Me and others around: The roles of personal and social norms in chinese adolescent bystanders’ responses toward cyberbullying. J. Interpers. Violence. 2022;37:NP6329–NP6354. doi: 10.1177/0886260520967128. [ DOI ] [ PubMed ] [ Google Scholar ]
- 37. Festl R., Scharkow M., Quandt T. The individual or the group: A multilevel analysis of cyberbullying in school classes. Hum. Commun. Res. 2015;41:535–556. doi: 10.1111/hcre.12056. [ DOI ] [ Google Scholar ]
- 38. Bastiaensens S., Pabian S., Vandebosch H., Poels K., Van Cleemput K., DeSmet A., De Bourdeaudhuij I. From normative influence to social pressure: How relevant others affect whether bystanders join in cyberbullying. Soc. Dev. 2016;25:193–211. doi: 10.1111/sode.12134. [ DOI ] [ Google Scholar ]
- 39. Chen L., Ho S.S., Lwin M.O. A meta-analysis of factors predicting cyberbullying perpetration and victimization: From the social cognitive and media effects approach. New Media Soc. 2017;19:1194–1213. doi: 10.1177/1461444816634037. [ DOI ] [ Google Scholar ]
- 40. Katz I., Lemish D., Cohen R., Arden A. When parents are inconsistent: Parenting style and adolescents’ involvement in cyberbullying. J. Adolesc. 2019;74:1–12. doi: 10.1016/j.adolescence.2019.04.006. [ DOI ] [ PubMed ] [ Google Scholar ]
- 41. Moreno–Ruiz D., Martínez–Ferrer B., García–Bacete F. Parenting styles, cyberaggression, and cybervictimization among adolescents. Comput. Hum. Behav. 2019;93:252–259. doi: 10.1016/j.chb.2018.12.031. [ DOI ] [ Google Scholar ]
- 42. Charalampous K., Demetriou C., Tricha L., Ioannou M., Georgiou S., Nikiforou M., Stavrinides P. The effect of parental style on bullying and cyber bullying behaviors and the mediating role of peer attachment relationships: A longitudinal study. J. Adolesc. 2018;64:109–123. doi: 10.1016/j.adolescence.2018.02.003. [ DOI ] [ PubMed ] [ Google Scholar ]
- 43. Barón J.O., Postigo J., Iranzo B., Buelga S., Carrascosa L. Parental communication and feelings of affiliation in adolescent aggressors and victims of cyberbullying. Soc. Sci. 2018;8:3. doi: 10.3390/socsci8010003. [ DOI ] [ Google Scholar ]
- 44. Larrañaga E., Yubero S., Ovejero A., Navarro R. Loneliness, parent-child communication and cyberbullying victimization among Spanish youths. Comput. Hum. Behav. 2016;65:1–8. doi: 10.1016/j.chb.2016.08.015. [ DOI ] [ Google Scholar ]
- 45. Romero-Abrio A., Martínez-Ferrer B., Musitu-Ferrer D., León-Moreno C., Villarreal-González M.E., Callejas-Jerónimo J.E. Family communication problems, psychosocial adjustment and cyberbullying. Int. J. Environ. Res. Public Health. 2019;16:2417. doi: 10.3390/ijerph16132417. [ DOI ] [ PMC free article ] [ PubMed ] [ Google Scholar ]
- 46. Baldry A.C., Sorrentino A., Farrington D.P. Cyberbullying and cybervictimization versus parental supervision, monitoring and control of adolescents’ online activities. Child. Youth Serv. Rev. 2019;96:302–307. doi: 10.1016/j.childyouth.2018.11.058. [ DOI ] [ Google Scholar ]
- 47. Meter D.J., Bauman S. Moral disengagement about cyberbullying and parental monitoring: Effects on traditional bullying and victimization via cyberbullying involvement. J. Early Adolesc. 2018;38:303–326. doi: 10.1177/0272431616670752. [ DOI ] [ Google Scholar ]
- 48. Doty J.L., Gower A.L., Sieving R.E., Plowman S.L., McMorris B.J. Cyberbullying victimization and perpetration, connectedness, and monitoring of online activities: Protection from parental figures. Soc. Sci. 2018;7:265. doi: 10.3390/socsci7120265. [ DOI ] [ Google Scholar ]
- 49. Pieschl S., Porsch T. The complex relationship between cyberbullying and trust. Int. J. Dev. Sci. 2017;11:9–17. doi: 10.3233/DEV-160208. [ DOI ] [ Google Scholar ]
- 50. den Hamer A.H., Konijn E.A. Adolescents’ media exposure may increase their cyberbullying behavior: A longitudinal study. J. Adolesc. Health. 2015;56:203–208. doi: 10.1016/j.jadohealth.2014.09.016. [ DOI ] [ PubMed ] [ Google Scholar ]
- 51. Fanti K.A., Demetriou A.G., Hawa V.V. A longitudinal study of cyberbullying: Examining risk and protective factors. Eur. J. Dev. Psychol. 2012;9:168–181. doi: 10.1080/17405629.2011.643169. [ DOI ] [ Google Scholar ]
- 52. Bushman B.J., Huesmann L.R. Short-term and long-term effects of violent media on aggression in children and adults. Arch. Pediatr. Adolesc. Med. 2006;160:348–352. doi: 10.1001/archpedi.160.4.348. [ DOI ] [ PubMed ] [ Google Scholar ]
- 53. Barlett C.P., Kowalewski D.A., Kramer S.S., Helmstetter K.M. Testing the relationship between media violence exposure and cyberbullying perpetration. Psychol. Pop. Media Cult. 2019;8:280–286. doi: 10.1037/ppm0000179. [ DOI ] [ Google Scholar ]
- 54. Zhu X.W., Chu X.W., Zhang Y.H., Li Z.H. Exposure to online game violence and cyberbullying among Chinese adolescents: Normative beliefs about aggression as a mediator and trait aggressiveness as a moderator. J. Aggress. Maltreatment Trauma. 2020;29:148–166. doi: 10.1080/10926771.2018.1550830. [ DOI ] [ Google Scholar ]
- 55. James I.A., Southam L., Blackburn I.M. Schemas revisited. Clin. Psychol. Psychother. Int. J. Theory Pract. 2004;11:369–377. doi: 10.1002/cpp.423. [ DOI ] [ Google Scholar ]
- 56. Huesmann R.L. An information processing model for the development of aggression. Aggress. Behav. 1988;14:13–24. doi: 10.1002/1098-2337(1988)14:1<13::AID-AB2480140104>3.0.CO;2-J. [ DOI ] [ Google Scholar ]
- 57. Wilkowski B.M., Robinson M.D. The cognitive basis of trait anger and reactive aggression: An integrative analysis. Personal. Soc. Psychol. Rev. 2008;12:3–21. doi: 10.1177/1088868307309874. [ DOI ] [ PubMed ] [ Google Scholar ]
- 58. Li M., He Q., Zhao J., Xu Z., Yang H. The effects of childhood maltreatment on cyberbullying in college students: The roles of cognitive processes. Acta Psychol. 2022;226:103588. doi: 10.1016/j.actpsy.2022.103588. [ DOI ] [ PubMed ] [ Google Scholar ]
- 59. Li S., Wang X., Nie Y. The relationship between parent-child attachment, belief in a just world, school climate and cyberbullying: A moderated mediation. Int. J. Environ. Res. Public Health. 2022;19:6207. doi: 10.3390/ijerph19106207. [ DOI ] [ PMC free article ] [ PubMed ] [ Google Scholar ]
- 60. Fan C., Chu X., Zhang M., Zhou Z. Are narcissists more likely to be involved in cyberbullying? Examining the mediating role of self-esteem. J. Interpers. Violence. 2019;34:3127–3150. doi: 10.1177/0886260516666531. [ DOI ] [ PubMed ] [ Google Scholar ]
- 61. Greitemeyer T. Effects of prosocial media on social behavior: When and why does media exposure affect helping and aggression? Curr. Dir. Psychol. Sci. 2011;20:251–255. doi: 10.1177/0963721411415229. [ DOI ] [ Google Scholar ]
- 62. Barlett C.P. Predicting Cyberbullying: Research, Theory, and Interventions. Academic Press; San Diego, CA, USA: 2019. [ Google Scholar ]
- 63. Gentile D.A., Anderson C.A., Yukawa S., Ihori N., Saleem M., Ming L.K., Shibuya A., Liau A.K., Khoo A., Bushman B.J., et al. The effects of prosocial video games on prosocial behaviors: International evidence from correlational, longitudinal, and experimental studies. Pers. Soc. Psychol. Bull. 2009;35:752–763. doi: 10.1089/cyber.2013.0457. [ DOI ] [ PMC free article ] [ PubMed ] [ Google Scholar ]
- 64. Wright M.F. Predictors of anonymous cyber aggression: The role of adolescents’ beliefs about anonymity, aggression, and the permanency of digital content. Cyberpsychol. Behav. Soc. Netw. 2014;17:431–438. doi: 10.1089/cyber.2013.0457. [ DOI ] [ PubMed ] [ Google Scholar ]
- 65. Wright M.F. The relationship between young adults’ beliefs about anonymity and subsequent cyber aggression. Cyberpsychol. Behav. Soc. Netw. 2013;16:858–862. doi: 10.1089/cyber.2013.0009. [ DOI ] [ PubMed ] [ Google Scholar ]
- 66. Sticca F., Perren S. Is cyberbullying worse than traditional bullying? Examining the differential roles of medium, publicity, and anonymity for the perceived severity of bullying. J. Youth Adolesc. 2013;42:739–750. doi: 10.1007/s10964-012-9867-3. [ DOI ] [ PubMed ] [ Google Scholar ]
- 67. Suler J. The online disinhibition effect. Cyberpsychol. Behav. 2004;7:321–326. doi: 10.1089/1094931041291295. [ DOI ] [ PubMed ] [ Google Scholar ]
- 68. Udris R. Cyberbullying among high school students in Japan: Development and validation of the Online Disinhibition Scale. Comput. Hum. Behav. 2014;41:253–261. doi: 10.1016/j.chb.2014.09.036. [ DOI ] [ Google Scholar ]
- 69. Ang R.P., Tan K., Mansor A.T. Normative beliefs about aggression as a mediator of narcissistic exploitativeness and cyberbullying. J. Interpers. Violence. 2011;26:2619–2634. doi: 10.1177/0886260510388286. [ DOI ] [ PubMed ] [ Google Scholar ]
- 70. Ang R.P., Li X., Seah S.L. The role of normative beliefs about aggression in the relationship between empathy and cyberbullying. J. Cross-Cult. Psychol. 2017;48:1138–1152. doi: 10.1177/0022022116678928. [ DOI ] [ Google Scholar ]
- 71. Burton K.A., Florell D., Wygant D.B. The role of peer attachment and normative beliefs about aggression on traditional bullying and cyberbullying. Psychol. Sch. 2013;50:103–115. doi: 10.1002/pits.21663. [ DOI ] [ Google Scholar ]
- 72. Huesmann R.L., Guerra N.G. Children’s normative beliefs about aggression and aggressive behavior. J. Personal. Soc. Psychol. 1997;72:408–419. doi: 10.1037/0022-3514.72.2.408. [ DOI ] [ PubMed ] [ Google Scholar ]
- 73. Wei H., Liu M. Loving your parents and treating others well: The effect of filial piety on cyberbullying perpetration and its functional mechanism among Chinese graduate students. J. Interpers. Violence. 2022;37:NP8670–NP8695. doi: 10.1177/0886260520978182. [ DOI ] [ PubMed ] [ Google Scholar ]
- 74. Dodge K.A., Coie J.D. Social-information-processing factors in reactive and proactive aggression in children’s peer groups. J. Personal. Soc. Psychol. 1987;53:1146–1158. doi: 10.1037/0022-3514.53.6.1146. [ DOI ] [ PubMed ] [ Google Scholar ]
- 75. Lazuras L., Barkoukis V., Ourda D., Tsorbatzoudis H. A process model of cyberbullying in adolescence. Comput. Hum. Behav. 2013;29:881–887. doi: 10.1016/j.chb.2012.12.015. [ DOI ] [ Google Scholar ]
- 76. Kaiser S., Kyrrestad H., Fossum S. Cyberbullying status and mental health in Norwegian adolescents. Scand. J. Psychol. 2020;61:707–713. doi: 10.1111/sjop.12656. [ DOI ] [ PubMed ] [ Google Scholar ]
- 77. Glasman L.R., Albarricin D. Forming attitudes that predict future behavior: A meta-analysis of the attitude-behavior relation. Psychol. Bull. 2006;132:778–822. doi: 10.1037/0033-2909.132.5.778. [ DOI ] [ PMC free article ] [ PubMed ] [ Google Scholar ]
- 78. Wang L., Ngai S.S. Cyberbullying perpetration among Chinese adolescents: The rol of power imbalance, fun-seeking tendency, and attitude toward cyberbullying. J. Interpers. Violence. 2022;37:NP21647. doi: 10.1177/08862605211062988. [ DOI ] [ PubMed ] [ Google Scholar ]
- 79. Doane A.N., Pearson M.R., Kelley M.L. Predictors of cyberbullying perpetration among college students: An application of the Theory of Reasoned Action. Comput. Hum. Behav. 2014;36:154–162. doi: 10.1016/j.chb.2014.03.051. [ DOI ] [ Google Scholar ]
- 80. Cavalcanti J.G., Pinto A.V., Pimentel C.E., Fernandes de Melo L.M. Psychometric parameters of the cyberbullying attitdue scale in Brazil. Curr. Psychol. 2021;40:3897–3907. doi: 10.1007/s12144-019-00326-6. [ DOI ] [ Google Scholar ]
- 81. Heriman W., Walrave M. Predicting adolescent perpetration in cyberbullying: An application of the theory of planned behavior. Psicothema. 2012;24:614–620. [ PubMed ] [ Google Scholar ]
- 82. Keum B.T., Miller M.J. Racism on the Internet: Conceptualization and recommendations for research. Psychol. Violence. 2018;8:782–791. doi: 10.1037/vio0000201. [ DOI ] [ Google Scholar ]
- 83. Ruggiero T.E. Uses and gratifications theory in the 21st century. Mass Commun. Soc. 2000;3:3–37. doi: 10.1207/S15327825MCS0301_02. [ DOI ] [ Google Scholar ]
- 84. Tanrikulu I., Erdur-Baker O. Motives behind cyberbullying perpetration: A test of Uses and Gratifications Theory. J. Interpers. Violence. 2021;36:NP6699–NP6724. doi: 10.1177/0886260518819882. [ DOI ] [ PubMed ] [ Google Scholar ]
- 85. Rule B.G., Ferguson T.J. The effects of media violence on attitudes, emotions, and cognitions. J. Soc. Issues. 1986;42:29–50. doi: 10.1111/j.1540-4560.1986.tb00241.x. [ DOI ] [ Google Scholar ]
- 86. Pabian S., Vandebosch H., Poels K., Van Cleemput K., Bastiaensens S. Exposure to cyberbullying as a bystander: An investigation of desensitization effects among early adolescents. Comput. Hum. Behav. 2016;62:480–487. doi: 10.1016/j.chb.2016.04.022. [ DOI ] [ Google Scholar ]
- 87. Wang X., Yang L., Yang J., Wang P., Lei L. Trait anger and cyberbullying among young adults: A moderated mediation model of moral disengagement and moral identity. Comput. Hum. Behav. 2017;73:519–526. doi: 10.1016/j.chb.2017.03.073. [ DOI ] [ Google Scholar ]
- 88. Zhang D., Huebner E.S., Tian L. Longitudinal associations among neuroticism, depression, and cyberbullying in early adolescents. Comput. Hum. Behav. 2020;112:106475. doi: 10.1016/j.chb.2020.106475. [ DOI ] [ Google Scholar ]
- 89. Baroncelli A., Ciucci E. Unique effects of different components of trait emotional intelligence in traditional bullying and cyberbullying. J. Adolesc. 2014;37:807–815. doi: 10.1016/j.adolescence.2014.05.009. [ DOI ] [ PubMed ] [ Google Scholar ]
- 90. Vandebosch H., Van Cleemput K. Defining cyberbullying: A qualitative research into the perceptions of youngsters. CyberPsychology Behav. 2008;11:499–503. doi: 10.1089/cpb.2007.0042. [ DOI ] [ PubMed ] [ Google Scholar ]
- 91. Barlett C.P., Chamberlin K., Witkower Z. Predicting cyberbullying perpetration in emerging adults: A test of the Barlett Gentile Cyberbullying Model. Aggress. Behav. 2017;43:147–154. doi: 10.1002/ab.21670. [ DOI ] [ PubMed ] [ Google Scholar ]
- 92. Barlett C.P., Helmstetter K.M. Longitudinal relations between early online disinhibition and anonymity perceptions on later cyberbullying perpetration: A theoretical test on youth. Psychol. Pop. Media Cult. 2018;7:561–571. doi: 10.1037/ppm0000149. [ DOI ] [ Google Scholar ]
- 93. Barlett C.P., Seyfert L.W., Simmers M.M., Chen V.H.H., Cavalcanit J.G., Krahe B., Suzuke K., Warburton W.A., Wong R.Y.M., Pimentel C.E., et al. Cross-cultural similarities and differences in the theoretical predictors of cyberbullying preparation: Results from a seven-country study. Aggress. Behav. 2021;47:111–119. doi: 10.1002/ab.21923. [ DOI ] [ PubMed ] [ Google Scholar ]
- 94. Barlett C.P., Gentile D.A., Li D., Khoo A. Predicting cyberbullying behavior from attitudes: A three-year cross-lagged analysis of Singaporean youth. J. Media Psychol. 2019;31:81–91. doi: 10.1027/1864-1105/a000231. [ DOI ] [ Google Scholar ]
- 95. Barlett C.P., Kowalewski D.A. Learning to cyberbully: An extension of the Barlett Gentile Cyberbullying Model. Psychol. Pop. Media Cult. 2019;8:437–443. doi: 10.1037/ppm0000183. [ DOI ] [ Google Scholar ]
- 96. Barlett C., Coyne S.M. A meta-analysis of sex differences in cyber-bullying behavior: The moderating role of age. Aggress. Behav. 2014;40:474–488. doi: 10.1002/ab.21555. [ DOI ] [ PubMed ] [ Google Scholar ]
- 97. Barlett C.P., Seyfert L.W., Rinker A.M., Roth B.R. The moderating role of dispositional fear of retaliation in cyberbullying perpetration processes. Personal. Individ. Differ. 2022;196:111731. doi: 10.1016/j.paid.2022.111731. [ DOI ] [ Google Scholar ]
- 98. Barlett C.P., Madison C.S., Heath J.B., DeWitt C.C. Please browse responsibly: A correlational examination of technology access and time spent online in the Barlett Gentile Cyberbullying Model. Comput. Hum. Behav. 2019;92:250–255. doi: 10.1016/j.chb.2018.11.013. [ DOI ] [ Google Scholar ]
- 99. Barlett C.P., Roth B.R., Rinker A.M. Muscles, popularity, social capital, and computer skills: Examining “power” in cyberbullying. Aggress. Behav. 2022;48:608–615. doi: 10.1002/ab.22047. [ DOI ] [ PubMed ] [ Google Scholar ]
- 100. Williford A., DePaolis K.J. Predictors of cyberbullying intervention among elementary school staff: The moderating effect of staff status. Psychol. Sch. 2016;53:1032–1044. doi: 10.1002/pits.21973. [ DOI ] [ Google Scholar ]
- 101. Cioppa V.D., O’Neil A., Craig W. Learning from traditional bullying interventions: A review of research on cyberbullying and best practices. Aggress. Violent Behav. 2015;23:61–68. doi: 10.1016/j.avb.2015.05.009. [ DOI ] [ Google Scholar ]
- 102. Barlett C.P., Heath J.B., Madison C.S., DeWitt C.C., Kirkpatrick S.M. You’re not anonymous online: The development and validation of a new cyberbullying intervention curriculum. Psychol. Pop. Media Cult. 2020;9:135–144. doi: 10.1037/ppm0000226. [ DOI ] [ Google Scholar ]
- 103. Gradinger P., Yanagida T., Strohmeier D., Spiel C. Prevention of cyberbullying and cybervictimization: Evaluation of the ViSC social competence program. J. Sch. Violence. 2015;14:87–110. doi: 10.1080/15388220.2014.963231. [ DOI ] [ Google Scholar ]
- 104. Williford A., Elledge L.C., Boulton A.J., DePaolis K.J., Little T.D., Salmivalli C. Effects of the KiVa antibullying program on cyberbullying and cybervictimization frequency among Finnish youth. J. Clin. Child Adolesc. Psychol. 2013;42:820–833. doi: 10.1080/15374416.2013.787623. [ DOI ] [ PubMed ] [ Google Scholar ]
- 105. DeSmet A., Bastiaensens S., Van Cleemput K., Poels K., Vandebosch H., Deboutte G., Herrewijn L., Malliet S., Pabian S., Van Broeckhoven F., et al. The efficacy of the Friendly Attac serious digital game to promote prosocial bystander behvaior in cyberbullying among young adolescents: A cluster-randomized controlled trial. Comput. Hum. Behav. 2018;78:336–347. doi: 10.1016/j.chb.2017.10.011. [ DOI ] [ Google Scholar ]
- 106. Englander E. Cell phone ownership and cyberbullying in 8–11 year olds: New research. Pediatrics. 2018;142:724. [ Google Scholar ]
- 107. Viney L.L. The assessment of psychological states through content analysis of verbal communications. Psychol. Bull. 1983;94:542–563. doi: 10.1037/0033-2909.94.3.542. [ DOI ] [ Google Scholar ]
- 108. Rezvani N., Beheshti A. Towards attention-based context-boosted cyberbullying detection in social media. J. Data Intell. 2021;2:418–433. doi: 10.26421/JDI2.4-2. [ DOI ] [ Google Scholar ]
- View on publisher site
- PDF (1.2 MB)
- Collections
Similar articles
Cited by other articles, links to ncbi databases.
- Download .nbib .nbib
- Format: AMA APA MLA NLM
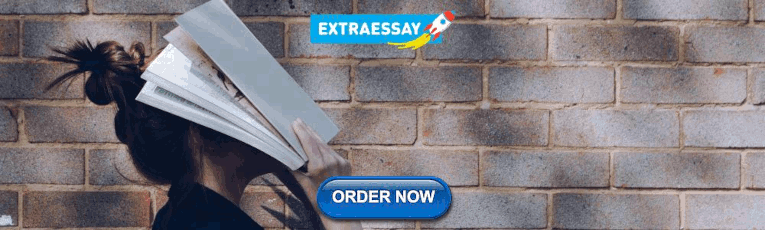
Add to Collections
SYSTEMATIC REVIEW article
Cyberbullying among adolescents and children: a comprehensive review of the global situation, risk factors, and preventive measures.

- 1 School of Political Science and Public Administration, Wuhan University, Wuhan, China
- 2 School of Medicine and Health Management, Tongji Medical College, Huazhong University of Science and Technology, Wuhan, China
- 3 College of Engineering, Design and Physical Sciences, Brunel University London, Uxbridge, United Kingdom
Background: Cyberbullying is well-recognized as a severe public health issue which affects both adolescents and children. Most extant studies have focused on national and regional effects of cyberbullying, with few examining the global perspective of cyberbullying. This systematic review comprehensively examines the global situation, risk factors, and preventive measures taken worldwide to fight cyberbullying among adolescents and children.
Methods: A systematic review of available literature was completed following PRISMA guidelines using the search themes “cyberbullying” and “adolescent or children”; the time frame was from January 1st, 2015 to December 31st, 2019. Eight academic databases pertaining to public health, and communication and psychology were consulted, namely: Web of Science, Science Direct, PubMed, Google Scholar, ProQuest, Communication & Mass Media Complete, CINAHL, and PsycArticles. Additional records identified through other sources included the references of reviews and two websites, Cyberbullying Research Center and United Nations Children's Fund. A total of 63 studies out of 2070 were included in our final review focusing on cyberbullying prevalence and risk factors.
Results: The prevalence rates of cyberbullying preparation ranged from 6.0 to 46.3%, while the rates of cyberbullying victimization ranged from 13.99 to 57.5%, based on 63 references. Verbal violence was the most common type of cyberbullying. Fourteen risk factors and three protective factors were revealed in this study. At the personal level, variables associated with cyberbullying including age, gender, online behavior, race, health condition, past experience of victimization, and impulsiveness were reviewed as risk factors. Likewise, at the situational level, parent-child relationship, interpersonal relationships, and geographical location were also reviewed in relation to cyberbullying. As for protective factors, empathy and emotional intelligence, parent-child relationship, and school climate were frequently mentioned.
Conclusion: The prevalence rate of cyberbullying has increased significantly in the observed 5-year period, and it is imperative that researchers from low and middle income countries focus sufficient attention on cyberbullying of children and adolescents. Despite a lack of scientific intervention research on cyberbullying, the review also identified several promising strategies for its prevention from the perspectives of youths, parents and schools. More research on cyberbullying is needed, especially on the issue of cross-national cyberbullying. International cooperation, multi-pronged and systematic approaches are highly encouraged to deal with cyberbullying.
Introduction
Childhood and adolescence are not only periods of growth, but also of emerging risk taking. Young people during these periods are particularly vulnerable and cannot fully understand the connection between behaviors and consequences ( 1 ). With peer pressures, the heat of passion, children and adolescents usually perform worse than adults when people are required to maintain self-discipline to achieve good results in unfamiliar situations. Impulsiveness, sensation seeking, thrill seeking, and other individual differences cause adolescents to risk rejecting standardized risk interventions ( 2 ).
About one-third of Internet users in the world are children and adolescents under the age of 18 ( 3 ). Digital technology provide a new form of interpersonal communication ( 4 ). However, surveys and news reports also show another picture in the Internet Age. The dark side of young people's internet usage is that they may bully or suffer from others' bullying in cyberspace. This behavior is also acknowledged as cyberbullying ( 5 ). Based on Olweus's definition, cyberbullying is usually regarded as bullying implemented through electronic media ( 6 , 7 ). Specifically, cyberbullying among children and adolescents can be summarized as the intentional and repeated harm from one or more peers that occurs in cyberspace caused by the use of computers, smartphones and other devices ( 4 , 8 – 12 ). In recent years, new forms of cyberbullying behaviors have emerged, such as cyberstalking and online dating abuse ( 13 – 15 ).
Although cyberbullying is still a relatively new field of research, cyberbullying among adolescents is considered to be a serious public health issue that is closely related to adolescents' behavior, mental health and development ( 16 , 17 ). The increasing rate of Internet adoption worldwide and the popularity of social media platforms among the young people have worsened this situation with most children and adolescents experiencing cyberbullying or online victimization during their lives. The confines of space and time are alleviated for bullies in virtual environments, creating new venues for cyberbullying with no geographical boundaries ( 6 ). Cyberbullying exerts negative effects on many aspects of young people's lives, including personal privacy invasion and psychological disorders. The influence of cyberbullying may be worse than traditional bullying as perpetrators can act anonymously and connect easily with children and adolescents at any time ( 18 ). In comparison with traditional victims, those bullied online show greater levels of depression, anxiety and loneliness ( 19 ). Self-esteem problems and school absenteeism have also proven to be related to cyberbullying ( 20 ).
Due to changes in use and behavioral patterns among the youth on social media, the manifestations and risk factors of cyberbullying have faced significant transformation. Further, as the boundaries of cyberbullying are not limited by geography, cyberbullying may not be a problem contained within a single country. In this sense, cyberbullying is a global problem and tackling it requires greater international collaboration. The adverse effects caused by cyberbullying, including reduced safety, lower educational attainment, poorer mental health and greater unhappiness, led UNICEF to state that “no child is absolutely safe in the digital world” ( 3 ).
Extant research has examined the prevalence and risk factors of cyberbullying to unravel the complexity of cyberbullying across different countries and their corresponding causes. However, due to variations in cyberbullying measurement and methodologies, no consistent conclusions have been drawn ( 21 ). Studies into inconsistencies in prevalence rates of cyberbullying, measured in the same country during the same time period, occur frequently. Selkie et al. systematically reviewed cyberbullying among American middle and high school students aged 10–19 years old in 2015, and revealed that the prevalence of cyberbullying victimization ranged from 3 to 72%, while perpetration ranged from 1 to 41% ( 22 ). Risk and protective factors have also been broadly studied, but confirmation is still needed of those factors which have more significant effects on cyberbullying among young people. Clarification of these issues would be useful to allow further research to recognize cyberbullying more accurately.
This review aims to extend prior contributions and provide a comprehensive review of cyberbullying of children and adolescents from a global perspective, with the focus being on prevalence, associated risk factors and protective factors across countries. It is necessary to provide a global panorama based on research syntheses to fill the gaps in knowledge on this topic.
Search Strategies
This study strictly employed Preferred Reporting Items for Systematic Reviews and Meta-Analyses (PRISMA) guidelines. We consulted eight academic databases pertaining to public health, and communication and psychology, namely: Web of Science, Science Direct, PubMed, Google Scholar, ProQuest, Communication & Mass Media Complete, CINAHL, and PsycArticles. Additional records identified through other sources included the references of reviews and two websites, Cyberbullying Research Center and United Nations Children's Fund. With regard to the duration of our review, since most studies on cyberbullying arose around 2015 ( 9 , 21 ), this study highlights the complementary aspects of the available information about cyberbullying during the recent 5 year period from January 1st, 2015 to December 31st, 2019.
One researcher extracted keywords and two researchers proposed modifications. We used two sets of subject terms to review articles, “cyberbullying” and “child OR adolescent.” Some keywords that refer to cyberbullying behaviors and young people are also included, such as threat, harass, intimidate, abuse, insult, humiliate, condemn, isolate, embarrass, forgery, slander, flame, stalk, manhunt, as well as teen, youth, young people and student. The search formula is (cyberbullying OR cyber-bullying OR cyber-aggression OR ((cyber OR online OR electronic OR Internet) AND (bully * OR aggres * OR violence OR perpetrat * OR victim * OR threat * OR harass * OR intimidat * OR * OR insult * OR humiliate * OR condemn * OR isolate * OR embarrass * OR forgery OR slander * OR flame OR stalk * OR manhunt))) AND (adolescen * OR child OR children OR teen? OR teenager? OR youth? OR “young people” OR “elementary school student * ” OR “middle school student * ” OR “high school student * ”). The main search approach is title search. Search strategies varied according to the database consulted, and we did not limit the type of literature for inclusion. Journals, conference papers and dissertations are all available.
Specifically, the inclusion criteria for our study were as follows: (a). reported or evaluated the prevalence and possible risk factors associated with cyberbullying, (b). respondents were students under the age of 18 or in primary, junior or senior high schools, and (c). studies were written in English. Exclusion criteria were: (a). respondents came from specific groups, such as clinical samples, children with disabilities, sexual minorities, specific ethnic groups, specific faith groups or samples with cross-national background, (b). review studies, qualitative studies, conceptual studies, book reviews, news reports or abstracts of meetings, and (c). studies focused solely on preventive measures that were usually meta-analytic and qualitative in nature. Figure 1 presents the details of the employed screening process, showing that a total of 63 studies out of 2070 were included in our final review.
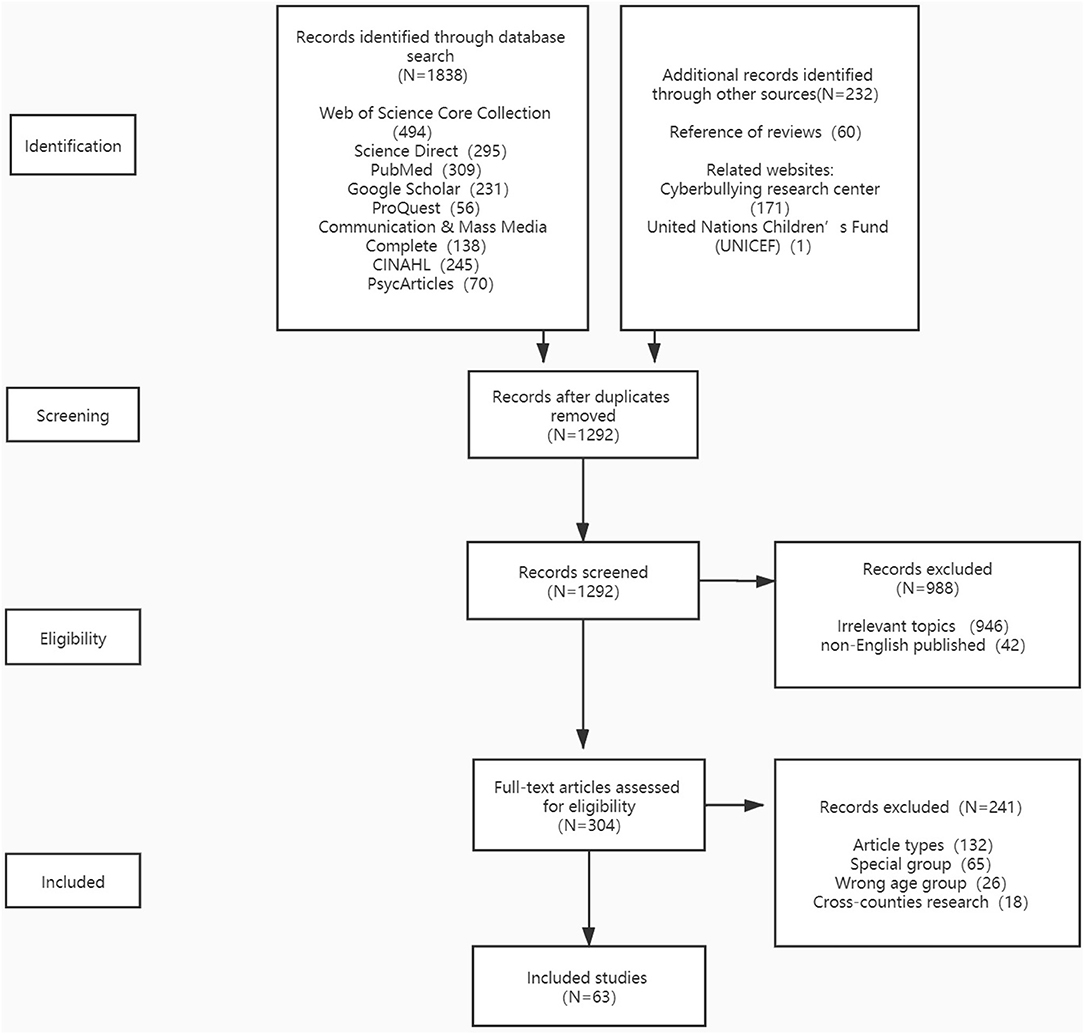
Figure 1 . PRISMA flow chart diagram showing the process of study selection for inclusion in the systematic review on children and adolescents cyberbullying.
Meta-analysis was not conducted as the limited research published within the 5 years revealed little research which reported odds ratio. On the other hand, due to the inconsistency of concepts, measuring instruments and recall periods, considerable variation could be found in research quality ( 23 ). Meta-analysis is not a preferred method.
Coding Scheme
For coding, we created a comprehensive code scheme to include the characteristics. For cyberbullying, we coded five types proposed by Willard ( 24 – 26 ), which included verbal violence, group violence, visual violence, impersonating and account forgery, and other behaviors. Among them, verbal violence is considered one of the most common types of cyberbullying and refers to the behavior of offensive responses, insults, mocking, threats, slander, and harassment. Group violence is associated with preventing others from joining certain groups or isolating others, forcing others to leave the group. Visual violence relates to the release and sharing of embarrassing photos and information without the owners' consent. Impersonating and account forgery refers to identity theft, stealing passwords, violating accounts and the creation of fake accounts to fraudulently present the behavior of others. Other behaviors include disclosure of privacy, sexual harassment, and cyberstalking. To comprehensively examine cyberbullying, we coded cyberbullying behaviors from both the perspectives of cyberbullying perpetrators and victims, if mentioned in the studies.
In relation to risk factors, we drew insights from the general aggression model, which contributes to the understanding of personal and situational factors in the cyberbullying of children and adolescents. We chose the general aggression model because (a) it contains more situational factors than other models (e.g., social ecological models) - such as school climate ( 9 ), and (b) we believe that the general aggression model is more suitable for helping researchers conduct a systematic review of cyberbullying risk and protective factors. This model provides a comprehensive framework that integrates domain specific theories of aggression, and has been widely applied in cyberbullying research ( 27 ). For instance, Kowalski and colleagues proposed a cyberbullying encounter through the general aggression model to understand the formation and development process of youth cyberbullying related to both victimization and perpetration ( 9 ). Victims and perpetrators enter the cyberbullying encounter with various individual characteristics, experiences, attitudes, desires, personalities, and motives that intersect to determine the course of the interaction. Correspondingly, the antecedents pertaining to cyberbullying are divided into two broad categories, personal factors and situational factors. Personal factors refer to individual characteristics, such as gender, age, motivation, personality, psychological states, socioeconomic status and technology use, values and perceptions, and other maladaptive behaviors. Situational factors focus on the provocation/support, parental involvement, school climate, and perceived anonymity. Consequently, our coders related to risk factors consisting of personal factors and situational factors from the perspectives of both cyberbullying perpetrators and victims.
We extracted information relating to individual papers and sample characteristics, including authors, year of publication, country, article type, sampling procedures, sample characteristics, measures of cyberbullying, and prevalence and risk factors from both cyberbullying perpetration and victimization perspectives. The key words extraction and coding work were performed twice by two trained research assistants in health informatics. The consistency test results are as follows: the Kappa value with “personal factors” was 0.932, and the Kappa value with “situational factors” was 0.807. The result shows that the coding consistency was high enough and acceptable. Disagreements were resolved through discussion with other authors.
Quality Assessment of Studies
The quality assessment of the studies is based on the recommended tool for assessing risk of bias, Cochrane Collaboration. This quality assessment tool focused on seven items: random sequence generation, allocation concealment, blinding of participants and personnel, blinding of outcome assessment, incomplete outcome data, selective reporting, and other sources of bias ( 28 ). We assessed each item as “low risk,” “high risk,” and “unclear” for included studies. A study is considered of “high quality” when it meets three or more “low risk” requirements. When one or more main flaw of a study may affect the research results, the study is considered as “low quality.” When a lack of information leads to a difficult judgement, the quality is considered to be “unclear.” Please refer to Appendix 1 for more details.
This comprehensive systematic review comprised a total of 63 studies. Appendices 2 , 3 show the descriptive information of the studies included. Among them, 58 (92%) studies measured two or more cyberbullying behavior types. The sample sizes of the youths range from several hundred to tens of thousands, with one thousand to five thousand being the most common. As for study distribution, the United States of America, Spain and China were most frequently mentioned. Table 1 presents the detail.
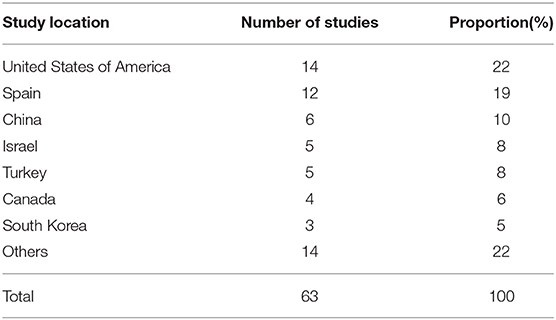
Table 1 . Descriptive information of studies included (2015–2019).
Prevalence of Global Cyberbullying
Prevalence across countries.
Among the 63 studies included, 22 studies reported on cyberbullying prevalence and 20 studies reported on prevalence from victimization and perpetration perspectives, respectively. Among the 20 studies, 11 national studies indicated that the prevalence of cyberbullying victimization and cyberbullying perpetration ranged from 14.6 to 52.2% and 6.3 to 32%, respectively. These studies were conducted in the United States of America ( N = 4) ( 29 – 32 ), South Korea ( N = 3) ( 33 – 35 ), Singapore ( N = 1) ( 36 ), Malaysia ( N = 1) ( 37 ), Israel ( N = 1) ( 38 ), and Canada ( N = 1) ( 39 ). Only one of these 11 national studies is from an upper middle income country, and the rest are from highincome countries identified by the World Bank ( 40 ). By combining regional and community-level studies, the prevalence of cyberbullying victimization and cyberbullying perpetration ranged from 13.99 to 57.5% and 6.0 to 46.3%, respectively. Spain reported the highest prevalence of cyberbullying victimization (57.5%) ( 41 ), followed by Malaysia (52.2%) ( 37 ), Israel (45%) ( 42 ), and China (44.5%) ( 43 ). The lowest reported victim rates were observed in Canada (13.99%) and South Korea (14.6%) ( 34 , 39 ). The reported prevalence of cyberbullying victimization in the United States of America ranged from 15.5 to 31.4% ( 29 , 44 ), while in Israel, rates ranged from 30 to 45% ( 26 , 42 ). In China, rates ranged from 6 to 46.3% with the country showing the highest prevalence of cyberbullying perpetration (46.30%) ( 15 , 43 , 45 , 46 ). Canadian and South Korean studies reported the lowest prevalence of cyberbullying perpetration at 7.99 and 6.3%, respectively ( 34 , 39 ).
A total of 10 studies were assessed as high quality studies. Among them, six studies came from high income countries, including Canada, Germany, Italy, Portugal, and South Korea ( 13 , 34 , 39 , 46 – 48 ). Three studies were from upper middle income countries, including Malaysia and China ( 37 , 43 ) and one from a lower middle income country, Nigeria ( 49 ). Figures 2 , 3 describe the prevalence of cyberbullying victimization and perpetration respectively among high quality studies.
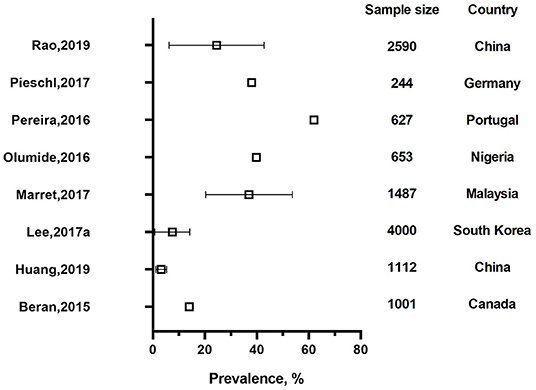
Figure 2 . The prevalence of cyberbullying victimization of high quality studies.
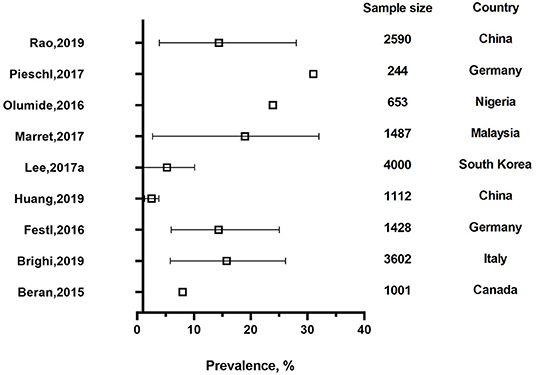
Figure 3 . The prevalence of cyberbullying perpetration of high quality studies.
Prevalence of Various Cyberbullying Behaviors
For the prevalence of cyberbullying victimization and perpetration, the data were reported in 18 and 14 studies, respectively. Figure 4 shows the distribution characteristics of the estimated value of prevalence of different cyberbullying behaviors with box plots. The longer the box, the greater the degree of variation of the numerical data and vice versa. The rate of victimization and crime of verbal violence, as well as the rate of victimization of other behaviors, such as cyberstalking and digital dating abuse, has a large degree of variation. Among the four specified types of cyberbullying behaviors, verbal violence was regarded as the most commonly reported behaviors in both perpetration and victimization rates, with a wide range of prevalence, ranging from 5 to 18%. Fewer studies reported the prevalence data for visual violence and group violence. Studies also showed that the prevalence of impersonation and account forgery were within a comparatively small scale. Specific results were as follows.
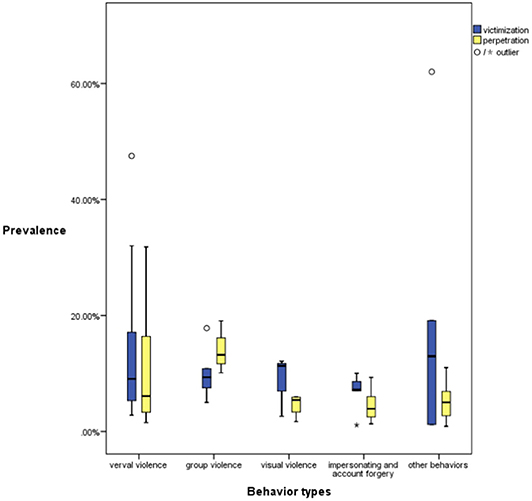
Figure 4 . Cyberbullying prevalence across types (2015–2019).
Verbal Violence
A total of 13 studies reported verbal violence prevalence data ( 15 , 26 , 34 , 37 – 39 , 42 , 43 , 47 , 48 , 50 , 51 ). Ten studies reported the prevalence of verbal violence victimization ranging from 2.8 to 47.5%, while seven studies claimed perpetration prevalence ranging from 1.5 to 31.8%. Malaysia reported the highest prevalence of verbal violence victimization (47.5%) ( 37 ), followed by China (32%) ( 43 ). China reported that the prevalence of verbal violence victimization ranged from 5.1 to 32% ( 15 , 43 ). Israel reported that the prevalence of verbal violence victimization ranged from 3.4 to 18% ( 26 , 38 , 42 ). For perpetration rate, Malaysia reported the highest level at 31.8% ( 37 ), while a study for Spain reported the lowest, ranging from 3.2 to 6.4% ( 51 ).
Group Violence
The prevalence of group violence victimization was explored within 4 studies and ranged from 5 to 17.8% ( 26 , 34 , 42 , 43 ), while perpetration prevalence was reported in three studies, ranging from 10.1 to 19.07% ( 34 , 43 , 47 ). An Israeli study suggested that 9.8% of respondents had been excluded from the Internet, while 8.9% had been refused entry to a group or team ( 26 ). A study in South Korea argued that the perpetration prevalence of group violence was 10.1% ( 34 ), while a study in Italy reported that the rate of online group violence against others was 19.07% ( 47 ).
Visual Violence
The prevalence of visual violence victimization was explored within three studies and ranged from 2.6 to 12.1% ( 26 , 34 , 43 ), while the perpetration prevalence reported in four studies ranged from 1.7 to 6% ( 34 , 43 , 47 , 48 ). For victimization prevalence, a South Korean study found that 12.1% of respondents reported that their personal information was leaked online ( 34 ). An Israel study reported that the prevalence of outing the picture was 2.6% ( 26 ). For perpetration prevalence, a South Korean study found that 1.7% of respondents had reported that they had disclosed someone's personal information online ( 34 ). A German study reported that 6% of respondents had written a message (e.g., an email) to somebody using a fake identity ( 48 ).
Impersonating and Account Forgery
Four studies reported on the victimization prevalence of impersonating and account forgery, ranging from 1.1 to 10% ( 15 , 42 , 43 ), while five studies reported on perpetration prevalence, with the range being from 1.3 to 9.31% ( 15 , 43 , 47 , 48 , 51 ). In a Spanish study, 10% of respondents reported that their accounts had been infringed by others or that they could not access their account due to stolen passwords. In contrast, 4.5% of respondents reported that they had infringed other people's accounts or stolen passwords, with 2.5% stating that they had forged other people's accounts ( 51 ). An Israeli study reported that the prevalence of being impersonated was 7% ( 42 ), while in China, a study reported this to be 8.6% ( 43 ). Another study from China found that 1.1% of respondents had been impersonated to send dating-for-money messages ( 15 ).
Other Behaviors
The prevalence of disclosure of privacy, sexual harassment, and cyberstalking were also explored by scholars. Six studies reported the victimization prevalence of other cyberbullying behaviors ( 13 , 15 , 34 , 37 , 42 , 43 ), and four studies reported on perpetration prevalence ( 34 , 37 , 43 , 48 ). A study in China found that 1.2% of respondents reported that their privacy had been compromised without permission due to disputes ( 15 ). A study from China reported the prevalence of cyberstalking victimization was 11.9% ( 43 ), while a Portuguese study reported that this was 62% ( 13 ). In terms of perpetration prevalence, a Malaysian study reported 2.7% for sexual harassment ( 37 ).
Risk and Protective Factors of Cyberbullying
In terms of the risk factors associated with cyberbullying among children and adolescents, this comprehensive review highlighted both personal and situational factors. Personal factors referred to age, gender, online behavior, race, health conditions, past experiences of victimization, and impulsiveness, while situational factors consisted of parent-child relationship, interpersonal relationships, and geographical location. In addition, protective factors against cyberbullying included: empathy and emotional intelligence, parent-child relationship, and school climate. Table 2 shows the risk and protective factors for child and adolescent cyberbullying.
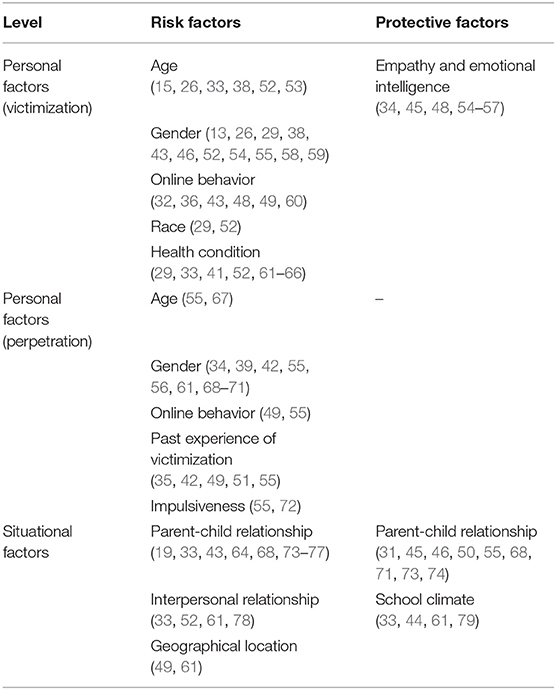
Table 2 . Risk and protective factors of cyberbullying among children and adolescents.
In terms of the risk factors associated with cyberbullying victimization at the personal level, many studies evidenced that females were more likely to be cyberbullied than males ( 13 , 26 , 29 , 38 , 43 , 52 , 54 , 55 , 58 ). Meanwhile, adolescents with mental health problems ( 61 ), such as depression ( 33 , 62 ), borderline personality disorder ( 63 ), eating disorders ( 41 ), sleep deprivation ( 56 ), and suicidal thoughts and suicide plans ( 64 ), were more likely to be associated with cyberbullying victimization. As for Internet usage, researchers agreed that youth victims were probably those that spent more time online than their counterparts ( 32 , 36 , 43 , 45 , 48 , 49 , 60 ). For situational risk factors, some studies have proven the relationship between cyberbullying victims and parental abuse, parental neglect, family dysfunction, inadequate monitoring, and parents' inconsistency in mediation, as well as communication issues ( 33 , 64 , 68 , 73 ). In terms of geographical location, some studies have reported that youths residing in city locations are more likely to be victims of cyberbullying than their peers from suburban areas ( 61 ).
Regarding the risk factors of cyberbullying perpetration at the personal level, it is generally believed that older teenagers, especially those aged over 15 years, are at greater risk of becoming cyberbullying perpetrators ( 55 , 67 ). When considering prior cyberbullying experiences, evidence showed that individuals who had experienced cyberbullying or face-to-face bullying tended to be aggressors in cyberbullying ( 35 , 42 , 49 , 51 , 55 ); in addition, the relationship between impulsiveness and cyberbullying perpetration was also explored by several pioneering scholars ( 55 , 72 , 80 ). The situational factors highlight the role of parents and teachers in cyberbullying experiences. For example, over-control and authoritarian parenting styles, as well as inharmonious teacher-student relationships ( 61 ) are perceived to lead to cyberbullying behaviors ( 74 , 75 ). In terms of differences in geographical locations, students residing in cities have a higher rate of online harassment than students living in more rural locations ( 49 ).
In terms of the protective factors in child and adolescent cyberbullying, scholars have focused on youths who have limited experiences of cyberbullying. At the personal level, high emotional intelligence, an ability for emotional self-control and empathy, such as cognitive empathy ability ( 44 , 55 ), were associated with lower rates of cyberbullying ( 57 ). At the situational level, a parent's role is seen as critical. For example, intimate parent-child relationships ( 46 ) and open active communication ( 19 ) were demonstrated to be related to lower experiences of cyberbullying and perpetration. Some scholars argued that parental supervision and monitoring of children's online activities can reduce their tendency to participate in some negative activities associated with cyberbullying ( 31 , 46 , 73 ). They further claimed that an authoritative parental style protects youths against cyberbullying ( 43 ). Conversely, another string of studies evidenced that parents' supervision of Internet usage was meaningless ( 45 ). In addition to conflicting roles of parental supervision, researchers have also looked into the role of schools, and posited that positive school climates contribute to less cyberbullying experiences ( 61 , 79 ).
Some risk factors may be protective factors under another condition. Some studies suggest that parental aggressive communication is related to severe cyberbullying victims, while open communication is a potential protective factor ( 19 ). Parental neglect, parental abuse, parental inconsistency in supervision of adolescents' online behavior, and family dysfunction are related to the direct or indirect harm of cyberbullying ( 33 , 68 ). Parental participation, a good parental-children relationship, communication and dialogue can enhance children's school adaptability and prevent cyberbullying behaviors ( 31 , 74 ). When parental monitoring reaches a balance between control and openness, it could become a protective factor against cyberbullying, and it could be a risk factor, if parental monitoring is too low or over-controlled ( 47 ).
Despite frequent discussion about the risk factors associated with cyberbullying among children and adolescents, some are still deemed controversial factors, such as age, race, gender, and the frequency of suffering on the internet. For cyberbullying victims, some studies claim that older teenagers are more vulnerable to cyberbullying ( 15 , 38 , 52 , 53 ), while other studies found conflicting results ( 26 , 33 ). As for student race, Alhajji et al. argued that non-white students were less likely to report cyberbullying ( 29 ), while Morin et al. observed no significant correlation between race and cyberbullying ( 52 ). For cyberbullying perpetration, Alvarez-Garcia found that gender differences may have indirect effects on cyberbullying perpetration ( 55 ), while others disagreed ( 42 , 61 , 68 – 70 ). Specifically, some studies revealed that males were more likely to become cyberbullying perpetrators ( 34 , 39 , 56 ), while Khurana et al. presented an opposite point of view, proposing that females were more likely to attack others ( 71 ). In terms of time spent on the Internet, some claimed that students who frequently surf the Internet had a higher chance of becoming perpetrators ( 49 ), while others stated that there was no clear and direct association between Internet usage and cyberbullying perpetration ( 55 ).
In addition to personal and situational factors, scholars have also explored other specific factors pertaining to cyberbullying risk and protection. For instance, mindfulness and depression were found to be significantly related to cyber perpetration ( 76 ), while eating disorder psychopathology in adolescents was associated with cyber victimization ( 41 ). For males who were familiar with their victims, such as family members, friends and acquaintances, they were more likely to be cyberstalking perpetrators than females or strangers, while pursuing desired closer relationships ( 13 ). In the school context, a lower social likability in class was identified as an indirect factor for cyberbullying ( 48 ).
This comprehensive review has established that the prevalence of global childhood and adolescent victimization from cyberbullying ranges from 13.99 to 57.5%, and that the perpetration prevalence ranges from 6.0 to 46.3%. Across the studies included in our research, verbal violence is observed as one of the most common acts of cyberbullying, including verbal offensive responses, insults, mocking, threats, slander, and harassment. The victimization prevalence of verbal violence is reported to be between 5 and 47.5%, and the perpetration prevalence is between 3.2 and 26.1%. Personal factors, such as gender, frequent use of social media platforms, depression, borderline personality disorder, eating disorders, sleep deprivation, and suicidal tendencies, were generally considered to be related to becoming a cyberbullying victim. Personal factors, such as high school students, past experiences, impulse, improperly controlled family education, poor teacher-student relationships, and the urban environment, were considered risk factors for cyberbullying perpetration. Situational factors, including parental abuse and neglect, improper monitoring, communication barriers between parents and children, as well as the urban environment, were also seen to potentially contribute to higher risks of both cyberbullying victimization and perpetration.
Increasing Prevalence of Global Cyberbullying With Changing Social Media Landscape and Measurement Alterations
This comprehensive review suggests that global cyberbullying rates, in terms of victimization and perpetration, were on the rise during the 5 year period, from 2015 to 2019. For example, in an earlier study conducted by Modecki et al. the average cyberbullying involvement rate was 15% ( 81 ). Similar observations were made by Hamm et al. who found that the median rates of youth having experienced bullying or who had bullied others online, was 23 and 15.2%, respectively ( 82 ). However, our systematic review summarized global children and adolescents cyberbullying in the last 5 years and revealed an average cyberbullying perpetration rate of 25.03%, ranging from 6.0 to 46.3%, while the average victimization was 33.08%, ranging from 13.99 to 57.5%. The underlying reason for increases may be attributed to the rapid changing landscape of social media and, in recent years, the drastic increase in Internet penetration rates. With the rise in Internet access, youths have greater opportunities to participate in online activities, provided by emerging social media platforms.
Although our review aims to provide a broader picture of cyberbullying, it is well-noted in extant research that difficulties exist in accurately estimating variations in prevalence in different countries ( 23 , 83 ). Many reasons exist to explain this. The first largely relates poor or unclear definition of the term cyberbullying; this hinders the determination of cyberbullying victimization and perpetration ( 84 ). Although traditional bullying behavior is well-defined, the definition cannot directly be applied to the virtual environment due to the complexity in changing online interactions. Without consensus on definitions, measurement and cyberbullying types may vary noticeably ( 83 , 85 ). Secondly, the estimation of prevalence of cyberbullying is heavily affected by research methods, such as recall period (lifetime, last year, last 6 months, last month, or last week etc.), demographic characteristics of the survey sample (age, gender, race, etc.), perspectives of cyberbullying experiences (victims, perpetrators, or both victim and perpetrator), and instruments (scales, study-specific questions) ( 23 , 84 , 86 ). The variety in research tools and instruments used to assess the prevalence of cyberbullying can cause confusion on this issue ( 84 ). Thirdly, variations in economic development, cultural backgrounds, human values, internet penetration rates, and frequency of using social media may lead to different conclusions across countries ( 87 ).
Acknowledging the Conflicting Role of the Identified Risk Factors With More Research Needed to Establish the Causality
Although this review has identified many personal and situational factors associated with cyberbullying, the majority of studies adopted a cross-sectional design and failed to reveal the causality ( 21 ). Nevertheless, knowledge on these correlational relationships provide valuable insights for understanding and preventing cyberbullying incidents. In terms of gender differences, females are believed to be at a higher risk of cyberbullying victimization compared to males. Two reasons may help to explain this. First, the preferred violence behaviors between two genders. females prefer indirect harassment, such as the spreading of rumors, while males tend toward direct bullying (e.g., assault) ( 29 ) and second, the cultural factors. From the traditional gender perspective, females tended to perceive a greater risk of communicating with others on the Internet, while males were more reluctant to express fear, vulnerability and insecurity when asked about their cyberbullying experiences ( 46 ). Females were more intolerant when experiencing cyberstalking and were more likely to report victimization experiences than males ( 13 ). Meanwhile, many researchers suggested that females are frequent users of emerging digital communication platforms, which increases their risk of unpleasant interpersonal contact and violence. From the perspective of cultural norms and masculinity, the reporting of cyberbullying is also widely acknowledged ( 37 ). For example, in addition, engaging in online activities is also regarded as a critical predictor for cyberbullying victimization. Enabled by the Internet, youths can easily find potential victims and start harassment at any time ( 49 ). Participating in online activities directly increases the chance of experiencing cyberbullying victimization and the possibility of becoming a victim ( 36 , 45 ). As for age, earlier involvement on social media and instant messaging tools may increase the chances of experiencing cyberbullying. For example, in Spain, these tools cannot be used without parental permission before the age of 14 ( 55 ). Besides, senior students were more likely to be more impulsive and less sympathetic. They may portray more aggressive and anti-social behaviors ( 55 , 72 ); hence senior students and students with higher impulsivity were usually more likely to become cyberbullying perpetrators.
Past experiences of victimization and family-related factors are another risk for cyberbullying crime. As for past experiences, one possible explanation is that young people who had experienced online or traditional school bullying may commit cyberbullying using e-mails, instant messages, and text messages for revenge, self-protection, or improving their social status ( 35 , 42 , 49 , 55 ). In becoming a cyberbullying perpetrator, the student may feel more powerful and superior, externalizing angry feelings and relieving the feelings of helplessness and sadness produced by past victimization experiences ( 51 ). As for family related factors, parenting styles are proven to be highly correlated to cyberbullying. In authoritative families, parents focus on rational behavioral control with clear rules and a high component of supervision and parental warmth, which have beneficial effects on children's lifestyles ( 43 ). Conversely, in indulgent families, children's behaviors are not heavily restricted and parents guide and encourage their children to adapt to society. The characteristics of this indulgent style, including parental support, positive communication, low imposition, and emotional expressiveness, possibly contribute to more parent-child trust and less misunderstanding ( 75 ). The protective role of warmth/affection and appropriate supervision, which are common features of authoritative or indulgent parenting styles, mitigate youth engagement in cyberbullying. On the contrary, authoritarian and neglectful styles, whether with excessive or insufficient control, are both proven to be risk factors for being a target of cyberbullying ( 33 , 76 ). In terms of geographical location, although several studies found that children residing in urban areas were more likely to be cyberbullying victims than those living in rural or suburban areas, we cannot draw a quick conclusion here, since whether this difference attributes to macro-level differences, such as community safety or socioeconomic status, or micro-level differences, such as teacher intervention in the classroom, courses provided, teacher-student ratio, is unclear across studies ( 61 ). An alternative explanation for this is the higher internet usage rate in urban areas ( 49 ).
Regarding health conditions, especially mental health, some scholars believe that young people with health problems are more likely to be identified as victims than people without health problems. They perceive health condition as a risk factor for cyberbullying ( 61 , 63 ). On the other hand, another group of scholars believe that cyberbullying has an important impact on the mental health of adolescents which can cause psychological distress consequences, such as post-traumatic stress mental disorder, depression, suicidal ideation, and drug abuse ( 70 , 87 ). It is highly possible that mental health could be risk factors, consequences of cyberbullying or both. Mental health cannot be used as standards, requirements, or decisive responses in cyberbullying research ( 13 ).
The Joint Effort Between Youth, Parents, Schools, and Communities to Form a Cyberbullying-Free Environment
This comprehensive review suggests that protecting children and adolescents from cyberbullying requires joint efforts between individuals, parents, schools, and communities, to form a cyberbullying-free environment. For individuals, young people are expected to improve their digital technology capabilities, especially in the use of social media platforms and instant messaging tools ( 55 ). To reduce the number of cyberbullying perpetrators, it is necessary to cultivate emotional self-regulation ability through appropriate emotional management training. Moreover, teachers, counselors, and parents are required to be armed with sufficient knowledge of emotional management and to develop emotional management capabilities and skills. In this way, they can be alert to the aggressive or angry emotions expressed by young people, and help them mediate any negative emotions ( 45 ), and avoid further anti-social behaviors ( 57 ).
For parents, styles of parenting involving a high level of parental involvement, care and support, are desirable in reducing the possibility of children's engagement in cyberbullying ( 74 , 75 ). If difficulties are encountered, open communication can contribute to enhancing the sense of security ( 73 ). In this vein, parents should be aware of the importance of caring, communicating and supervising their children, and participate actively in their children's lives ( 71 ). In order to keep a balance between control and openness ( 47 ), parents can engage in unbiased open communication with their children, and reach an agreement on the usage of computers and smart phones ( 34 , 35 , 55 ). Similarly, it is of vital importance to establish a positive communication channel with children ( 19 ).
For schools, a higher priority is needed to create a safe and positive campus environment, providing students with learning opportunities and ensuring that every student is treated equally. With a youth-friendly environment, students are able to focus more on their academic performance and develop a strong sense of belonging to the school ( 79 ). For countries recognizing collectivist cultural values, such as China and India, emphasizing peer attachment and a sense of collectivism can reduce the risk of cyberbullying perpetration and victimization ( 78 ). Besides, schools can cooperate with mental health agencies and neighboring communities to develop preventive programs, such as extracurricular activities and training ( 44 , 53 , 62 ). Specifically, school-based preventive measures against cyberbullying are expected to be sensitive to the characteristics of young people at different ages, and the intersection of race and school diversity ( 29 , 76 ). It is recommended that school policies that aim to embrace diversity and embody mutual respect among students are created ( 26 ). Considering the high prevalence of cyberbullying and a series of serious consequences, it is suggested that intervention against cyberbullying starts from an early stage, at about 10 years old ( 54 ). Schools can organize seminars to strengthen communication between teachers and students so that they can better understand the needs of students ( 61 ). In addition, schools should encourage cyberbullying victims to seek help and provide students with opportunities to report cyberbullying behaviors, such as creating online anonymous calls.
Conclusions and Limitations
The comprehensive study has reviewed related research on children and adolescents cyberbullying across different countries and regions, providing a positive understanding of the current situation of cyberbullying. The number of studies on cyberbullying has surged in the last 5 years, especially those related to risk factors and protective factors of cyberbullying. However, research on effective prevention is insufficient and evaluation of policy tools for cyberbullying intervention is a nascent research field. Our comprehensive review concludes with possible strategies for cyberbullying prevention, including personal emotion management, digital ability training, policy applicability, and interpersonal skills. We highlight the important role of parental control in cyberbullying prevention. As for the role of parental control, it depends on whether children believe their parents are capable of adequately supporting them, rather than simply interfering in their lives, restricting their online behavior, and controlling or removing their devices ( 50 ). In general, cyberbullying is on the rise, with the effectiveness of interventions to meet this problem still requiring further development and exploration ( 83 ).
Considering the overlaps between cyberbullying and traditional offline bullying, future research can explore the unique risk and protective factors that are distinguishable from traditional bullying ( 86 ). To further reveal the variations, researchers can compare the outcomes of interventions conducted in cyberbullying and traditional bullying preventions simultaneously, and the same interventions only targeting cyberbullying ( 88 ). In addition, cyberbullying also reflects a series of other social issues, such as personal privacy and security, public opinion monitoring, multinational perpetration and group crimes. To address this problem, efforts from multiple disciplines and novel analytical methods in the digital era are required. As the Internet provides enormous opportunities to connect young people from all over the world, cyberbullying perpetrators may come from transnational networks. Hence, cyberbullying of children and adolescents, involving multiple countries, is worth further attention.
Our study has several limitations. First, national representative studies are scarce, while few studies from middle and low income countries were included in our research due to language restrictions. Many of the studies included were conducted in schools, communities, provinces, and cities in high income countries. Meanwhile, our review only focused on victimization and perpetration. Future studies should consider more perspectives, such as bystanders and those with the dual identity of victim/perpetrator, to comprehensively analyze the risk and protective factors of cyberbullying.
Data Availability Statement
The original contributions presented in the study are included in the article/ Supplementary Material , further inquiries can be directed to the corresponding author/s.
Author Contributions
SH, CZ, RE, and WZ conceived the study and developed the design. WZ analyzed the result and supervised the study. CZ and SH wrote the first draft. All authors contributed to the article and approved the submitted version.
Conflict of Interest
The authors declare that the research was conducted in the absence of any commercial or financial relationships that could be construed as a potential conflict of interest.
Supplementary Material
The Supplementary Material for this article can be found online at: https://www.frontiersin.org/articles/10.3389/fpubh.2021.634909/full#supplementary-material
1. Ang RP. Adolescent cyberbullying: a review of characteristics, prevention and intervention strategies. Aggress Violent Behav. (2015) 25:35–42. doi: 10.1016/j.avb.2015.07.011
CrossRef Full Text | Google Scholar
2. Reyna VF, Farley F. Risk and rationality in adolescent decision making: implications for theory, practice, and public policy. Psychol Sci Public Interest. (2006) 7:1–44. doi: 10.1111/j.1529-1006.2006.00026.x
PubMed Abstract | CrossRef Full Text | Google Scholar
3. UNICEF ed. Children in a Digital World . New York, NY: UNICEF (2017).
Google Scholar
4. Thomas HJ, Connor JP, Scott JG. Integrating traditional bullying and cyberbullying: challenges of definition and measurement in adolescents - a review. Educ Psychol Rev. (2015) 27:135–52. doi: 10.1007/s10648-014-9261-7
5. Baldry AC, Farrington DP, Sorrentino A. “Am I at risk of cyberbullying”? A narrative review and conceptual framework for research on risk of cyberbullying and cybervictimization: the risk and needs assessment approach. Aggress Violent Behav. (2015) 23:36–51. doi: 10.1016/j.avb.2015.05.014
6. Olweus D. Bullying at School: What We Know and What We Can Do . Oxford; Cambridge, MA: Blackwell (1993).
PubMed Abstract | Google Scholar
7. Dooley JJ, Pyzalski J, Cross D. Cyberbullying versus face-to-face bullying: a theoretical and conceptual review. J Psychol. (2009) 217:182–8. doi: 10.1027/0044-3409.217.4.182
8. Smith PK, Mahdavi J, Carvalho M, Fisher S, Russell S, Tippett N. Cyberbullying: its nature and impact in secondary school pupils. J Child Psychol Psychiatry. (2008) 49:376–85. doi: 10.1111/j.1469-7610.2007.01846.x
9. Kowalski RM, Giumetti GW, Schroeder AN, Lattanner MR. Bullying in the digital age: a critical review and meta-analysis of cyberbullying research among youth. Psychol Bull. (2014) 140:1073–137. doi: 10.1037/a0035618
10. León Vicente I. Cybervictimization by cyberbullying: children at risk and children as risk (dissertation). University of the Basque Country, Leioa, Spain (2016).
11. Hinduja S, Patchin JW. Identification, Prevention, and Response. (2020).
12. Jadambaa A, Thomas HJ, Scott JG, Graves N, Brain D, Pacella R. Prevalence of traditional bullying and cyberbullying among children and adolescents in Australia: a systematic review and meta-analysis. Aust N Z J Psychiatry. (2019) 53:878–88. doi: 10.1177/0004867419846393
13. Pereira F, Matos M. Cyber-stalking victimization: what predicts fear among Portuguese adolescents? Eur J Crim Policy Res. (2016) 22:253–70. doi: 10.1007/s10610-015-9285-7
14. Reed LA, Ward LM, Tolman RM, Lippman JR, Seabrook RC. The association between stereotypical gender and dating beliefs and digital dating abuse perpetration in adolescent dating relationships. J Interpers Violence . (2018). doi: 10.1177/0886260518801933
15. Huang CL, Yang SC, Hsieh LS. The cyberbullying behavior of Taiwanese adolescents in an online gaming environment. Children Youth Serv Rev. (2019) 106:104461. doi: 10.1016/j.childyouth.2019.104461
16. Raskauskas J, Huynh A. The process of coping with cyberbullying: a systematic review. Aggress Violent Behav. (2015) 23:118–25. doi: 10.1016/j.avb.2015.05.019
17. Bradshaw J, Crous G, Rees G, Turner N. Comparing children's experiences of schools-based bullying across countries. Children Youth Serv Rev. (2017) 80:171–80. doi: 10.1016/j.childyouth.2017.06.060
18. Hutson E, Kelly S, Militello LK. Systematic review of cyberbullying interventions for youth and parents with implications for evidence-based practice. Worldviews Evid Based Nurs. (2018) 15:72–9. doi: 10.1111/wvn.12257
19. Larranaga E, Yubero S, Ovejero A, Navarro R. Loneliness, parent-child communication and cyberbullying victimization among Spanish youths. Comp Hum Behav. (2016) 65:1–8. doi: 10.1016/j.chb.2016.08.015
20. van Geel M, Vedder P, Tanilon J. Relationship between peer victimization, cyberbullying, and suicide in children and adolescents: a meta-analysis. JAMA Pediatr. (2014) 168:435–42. doi: 10.1001/jamapediatrics.2013.4143
21. Zych I, Ortega-Ruiz R, Del Rey R. Systematic review of theoretical studies on bullying and cyberbullying: facts, knowledge, prevention, and intervention. Aggress Violent Behav. (2015) 23:1–21. doi: 10.1016/j.avb.2015.10.001
22. Selkie EM, Kota R, Chan Y-F, Moreno M. Cyberbullying, depression, and problem alcohol use in female college students: a multisite study. Cyberpsychol Behav Soc Netw. (2015) 18:79–86. doi: 10.1089/cyber.2014.0371
23. Brochado S, Soares S, Fraga S. A scoping review on studies of cyberbullying prevalence among adolescents. Trauma Violence Abus. (2017) 18:523–31. doi: 10.1177/1524838016641668
24. Nocentini A, Calmaestra J, Schultze-Krumbholz A, Scheithauer H, Ortega R, Menesini E. Cyberbullying: labels, behaviours and definition in three European Countries. Aust J Guid Couns. (2010) 20:129–42. doi: 10.1375/ajgc.20.2.129
25. Willard NE. Cyberbullying and Cyberthreats: Responding to the Challenge of Online Social Aggression, Threats, and Distress . Champaign: Research Press (2007).
26. Aizenkot D, Kashy-Rosenbaum G. Cyberbullying victimization in whatsapp classmate groups among Israeli Elementary, Middle, and High School Students. J Interpers Violence . (2019). doi: 10.1177/0886260519842860
27. Anderson CA, Bushman BJ. Human aggression. Ann Rev Psychol. (2002) 53:27–51. doi: 10.1146/annurev.psych.53.100901.135231
28. Higgins JP, Green S. Cochrane Handbook for Systematic Reviews of Interventions . (2011). Available online at: http://handbook-5-1.cochrane.org/ (accessed January 17, 2021).
29. Alhajji M, Bass S, Dai T. Cyberbullying, mental health, and violence in adolescents and associations with sex and race: data from the 2015 youth risk behavior survey. Global Pediatr Health . (2019) 6:2333794X19868887. doi: 10.1177/2333794X19868887
30. Grinshteyn E, Yang YT. The association between electronic bullying and school absenteeism among high school students in the United States. J School Health. (2017) 87:142–9. doi: 10.1111/josh.12476
31. Mesch GS. Parent-child connections on social networking sites and cyberbullying. Youth Soc. (2018) 50:1145–62. doi: 10.1177/0044118X16659685
32. Sam J, Wisniewski P, Xu H, Rosson MB, Carroll JM. How are social capital and parental mediation associated with cyberbullying and cybervictimization among youth in the United States? In: Stephanidis C, editor. HCI International 2017 – Posters' Extended Abstracts Communications in Computer Information Science Cham: Springer International Publishing. p. 638–644.
33. Hong JS, Kim DH, Thornberg R, Kang JH, Morgan JT. Correlates of direct and indirect forms of cyberbullying victimization involving South Korean adolescents: an ecological perspective. Comput Hum Behav. (2018) 87:327–36. doi: 10.1016/j.chb.2018.06.010
34. Lee C, Shin N. Prevalence of cyberbullying and predictors of cyberbullying perpetration among Korean adolescents. Comp Hum Behav. (2017) 68:352–8. doi: 10.1016/j.chb.2016.11.047
35. You S, Lim SA. Longitudinal predictors of cyberbullying perpetration: evidence from Korean middle school students. Person Ind Differ. (2016) 89:172–6. doi: 10.1016/j.paid.2015.10.019
36. Holt TJ, Fitzgerald S, Bossler AM, Chee G, Ng E. Assessing the risk factors of cyber and mobile phone bullying victimization in a nationally representative sample of Singapore Youth. Int J Offend Ther Comp Criminol. (2016) 60:598–615. doi: 10.1177/0306624X14554852
37. Marret MJ, Choo WY. Factors associated with online victimisation among Malaysian adolescents who use social networking sites: a cross-sectional study. BMJ Open. (2017) 7:e014959. doi: 10.1136/bmjopen-2016-014959
38. Tesler R, Nissanholtz-Gannot R, Zigdon A, Harel-Fisch Y. The association of cyber-bullying and adolescents in religious and secular schools in Israel. J Relig Health. (2019) 58:2095–109. doi: 10.1007/s10943-019-00938-z
39. Beran T, Mishna F, McInroy LB, Shariff S. Children's experiences of cyberbullying: a Canadian National Study. Child School. (2015) 37:207–14. doi: 10.1093/cs/cdv024
40. World Bank Country and Lending Groups – World Bank Data Help Desk. Available online at: https://datahelpdesk.worldbank.org/knowledgebase/articles/906519-world-bank-country-and-lending-groups [accessed January 25, 2021).
41. Marco JH, Tormo-Irun P. Cyber victimization is associated with eating disorder psychopathology in adolescents. Front Psychol. (2018) 9:987. doi: 10.3389/fpsyg.2018.00987
42. Olenik-Shemesh D, Heiman T. Cyberbullying victimization in adolescents as related to body esteem, social support, and social self-efficacy. J Genet Psychol. (2017) 178:28–43. doi: 10.1080/00221325.2016.1195331
43. Rao J, Wang H, Pang M, Yang J, Zhang J, Ye Y, et al. Cyberbullying perpetration and victimisation among junior and senior high school students in Guangzhou, China. Inj Prev. (2019) 25:13–9. doi: 10.1136/injuryprev-2016-042210
44. Lee C. Weak Commitment to School, Deviant Peers, and Cyberbullying Victimization-Strain in Adolescent Cyberbullying . (2017). Available online at: https://search.proquest.com/pqdtglobal/docview/1937915933/abstract/9D85437600564444PQ/30 (accessed June 17, 2020).
45. Lin MT. Risk factors associated with cyberbullying victimization and perpetration among Taiwan children (dissertation). The University of Texas, Austin, TX, United States (2019).
46. Pieschl S, Porsch T. The complex relationship between cyberbullying and trust. Int J Dev Sci. (2017) 11:9–17. doi: 10.3233/DEV-160208
47. Brighi A, Menin D, Skrzypiec G, Guarini A. Young, bullying, and connected. Common pathways to cyberbullying and problematic internet use in adolescence. Front Psychol. (2019) 10:1467. doi: 10.3389/fpsyg.2019.01467
48. Festl R. Perpetrators on the internet: analyzing individual and structural explanation factors of cyberbullying in school context. Comp Hum Behav. (2016) 59:237–48. doi: 10.1016/j.chb.2016.02.017
49. Olumide AO, Adams P, Amodu OK. Prevalence and correlates of the perpetration of cyberbullying among in-school adolescents in Oyo State, Nigeria. Int J Adolesc Med Health. (2016) 28:183–91. doi: 10.1515/ijamh-2015-0009
50. Baldry AC, Sorrentino A, Farrington DP. Cyberbullying and cybervictimization versus parental supervision, monitoring and control of adolescents' online activities. Child Youth Serv Rev. (2019) 96:302–7. doi: 10.1016/j.childyouth.2018.11.058
51. Garaigordobil M. Cyberbullying in adolescents and youth in the Basque Country: prevalence of cybervictims, cyberaggressors, and cyberobservers. J Youth Stud. (2015) 18:569–82. doi: 10.1080/13676261.2014.992324
52. Morin HK, Bradshaw CP, Kush JM. Adjustment outcomes of victims of cyberbullying: the role of personal and contextual factors. J School Psychol. (2018) 70:74–88. doi: 10.1016/j.jsp.2018.07.002
53. Baraldsnes D. The prevalence of cyberbullying and the views of 5-12 grade pupils and teachers on cyberbullying prevention in Lithuanian Schools. Uinv J Educ Res. (2015) 3:949–59. doi: 10.13189/ujer.2015.031201
54. Razjouyan K, Mobarake AH, Sadr SS, Ardestani SMS, Yaseri M. The relationship between emotional intelligence and the different roles in cyberbullying among high school students in Tehran. Iran J Psychiatry Behav Sci. (2018) 12:UNSP e11560. doi: 10.5812/ijpbs.11560
55. Alvarez-Garcia D, Carlos Nunez J, Garcia T, Barreiro-Collazo A. Individual, family, and community predictors of cyber-aggression among adolescents. Eur J Psychol Appl Legal Context. (2018) 10:79–88. doi: 10.5093/ejpalc2018a8
56. Horzum MB, Ayas T, Randler C, Dusunceli B. The effects of empathy and circadian preference on cyberbullying of adolescents in Turkey. Biol Rhythm Res . (2019). doi: 10.1080/09291016.2019.1603839
57. Carmen Martinez-Monteagudo M, Delgado B, Manuel Garcia-Fernandez J, Rubio E. Cyberbullying, aggressiveness, and emotional intelligence in adolescence. Int J Environ Res Public Health. (2019) 16:5079. doi: 10.3390/ijerph16245079
58. Sasson H, Mesch G. The role of parental mediation and peer norms on the likelihood of cyberbullying. J Genet Psychol. (2017) 178:15–27. doi: 10.1080/00221325.2016.1195330
59. Wang X, Lei L, Liu D, Hu H. Moderating effects of moral reasoning and gender on the relation between moral disengagement and cyberbullying in adolescents. Person Ind Differ. (2016) 98:244–9. doi: 10.1016/j.paid.2016.04.056
60. Simsek N, Sahin D, Evli M. Internet addiction, cyberbullying, and victimization relationship in adolescents a sample from Turkey. J Addict Nurs. (2019) 30:201–10. doi: 10.1097/JAN.0000000000000296
61. McQuillan BE. Ecological Factors Associated with Middle School Students' Experiences of Cyberbullying . (2016). Available online at: https://search.proquest.com/pqdtglobal/docview/1794167537/abstract/9D85437600564444PQ/4 (accessed June 17, 2020).
62. Rose CA, Tynes BM. Longitudinal associations between cybervictimization and mental health among U.S. adolescents. J Adolesc Health. (2015) 57:305–12. doi: 10.1016/j.jadohealth.2015.05.002
63. Stockdale LA, Coyne SM, Nelson DA, Erickson DH. Borderline personality disorder features, jealousy, and cyberbullying in adolescence. Pers Individ Differ. (2015) 83:148–53. doi: 10.1016/j.paid.2015.04.003
64. Chen Q, Lo Camilla KM, Yuhong Z, Anne C, Ling CK, Patrick I. Family poly-victimization and cyberbullying among adolescents in a Chinese school sample. Child Abuse Negl. (2018) 77:180–7. doi: 10.1016/j.chiabu.2018.01.015
65. Landoll RR, La Greca AM, Lai BS, Chan SF, Herge WM. Cyber victimization by peers: prospective associations with adolescent social anxiety and depressive symptoms. J Adolesc. (2015) 42:77–86. doi: 10.1016/j.adolescence.2015.04.002
66. Iranzo B, Buelga S, Cava M-J, Ortega-Baron J. Cyberbullying, psychosocial adjustment, and suicidal ideation in adolescence. Psychosoc Interv. (2019) 28:75–81. doi: 10.5093/pi2019a5
67. Buelga S, Cava MJ, Musitu G, Torralba E. Cyberbullying aggressors among Spanish secondary education students: an exploratory study. Interact Tech Smart Ed. (2015) 12:100–15. doi: 10.1108/ITSE-08-2014-0025
68. Katz I, Lemish D, Cohen R, Arden A. When parents are inconsistent: parenting style and adolescents' involvement in cyberbullying. J Adolesc. (2019) 74:1–2. doi: 10.1016/j.adolescence.2019.04.006
69. Cénat JM, Blais M, Lavoie F, Caron P-O, Hébert M. Cyberbullying victimization and substance use among Quebec high schools students: the mediating role of psychological distress. Comp Hum Behav. (2018) 89:207–12. doi: 10.1016/j.chb.2018.08.014
70. Hoareau N, Bages C, Allaire M, Guerrien A. The role of psychopathic traits and moral disengagement in cyberbullying among adolescents. Crim Behav Ment Health. (2019) 29:321–31. doi: 10.1002/cbm.2135
71. Khurana A, Bleakley A, Jordan A, Romer D. The protective effects of parental monitoring and internet restriction on adolescents' risk of online harassment. J Youth Adolesc. (2015) 44:1039–47. doi: 10.1007/s10964-014-0242-4
72. Martínez I, Murgui S, Garcia OF, Garcia F. Parenting in the digital era: protective and risk parenting styles for traditional bullying and cyberbullying victimization. Comp Hum Behav. (2019) 90:84–92. doi: 10.1016/j.chb.2018.08.036
73. Yusuf S, Salleh H, Bahaman A, Shamsul M, Ramli N, Ramli AN, et al. Parental attachment and cyberbullying experiences among Malaysian children. Pertanika J Scholarly Res Rev . (2018) 4:67–80.
74. Martinez-Ferrer B, Leon-Moreno C, Musitu-Ferrer D, Romero-Abrio A, Callejas-Jeronimo JE, Musitu-Ochoa G. Parental socialization, school adjustment and cyber-aggression among adolescents. Int J Environ Res Public Health. (2019) 16:4005. doi: 10.3390/ijerph16204005
75. Moreno–Ruiz D, Martínez–Ferrer B, García–Bacete F. Parenting styles, cyberaggression, and cybervictimization among adolescents. Comp Hum Behav. (2019) 93:252–9. doi: 10.1016/j.chb.2018.12.031
76. Ho SS, Chen L, Ng APY. Comparing cyberbullying perpetration on social media between primary and secondary school students. Comp Educ. (2017) 109:74–84. doi: 10.1016/j.compedu.2017.02.004
77. Gómez-Ortiz O, Romera EM, Ortega-Ruiz R, Del Rey R. Parenting practices as risk or preventive factors for adolescent involvement in cyberbullying: contribution of children and parent gender. Int J Environ Res Public Health. (2018) 15:2664. doi: 10.3390/ijerph15122664
78. Wright MF, Kamble SV, Soudi SP. Indian adolescents' cyber aggression involvement and cultural values: the moderation of peer attachment. Sch Psychol Int. (2015) 36:410–27. doi: 10.1177/0143034315584696
79. Holfeld B, Leadbeater BJ. Concurrent and longitudinal associations between early adolescents' experiences of school climate and cyber victimization. Comput Hum Behav. (2017) 76:321–8. doi: 10.1016/j.chb.2017.07.037
80. Álvarez-García D, Núñez JC, González-Castro P, Rodríguez C, Cerezo R. The effect of parental control on cyber-victimization in adolescence: the mediating role of impulsivity and high-risk behaviors. Front Psychol. (2019) 10:1159. doi: 10.3389/fpsyg.2019.01159
81. Modecki KL, Minchin J, Harbaugh AG, Guerra NG, Runions KC. Bullying prevalence across contexts: a meta-analysis measuring cyber and traditional bullying. J Adolesc Health. (2014) 55:602–11. doi: 10.1016/j.jadohealth.2014.06.007
82. Hamm MP, Newton AS, Chisholm A, Shulhan J, Milne A, Sundar P, et al. Prevalence and effect of cyberbullying on children and young people: a scoping review of social media studies. JAMA Pediatr. (2015) 169:770. doi: 10.1001/jamapediatrics.2015.0944
83. Gaffney H, Farrington DP, Espelage DL, Ttofi MM. Are cyberbullying intervention and prevention programs effective? A systematic and meta-analytical review. Aggress Violent Behav. (2019) 45:134–53. doi: 10.1016/j.avb.2018.07.002
84. Selkie EM, Fales JL, Moreno MA. Cyberbullying prevalence among US middle and high school-aged adolescents: a systematic review and quality assessment. J Adolesc Health. (2016) 58:125–33. doi: 10.1016/j.jadohealth.2015.09.026
85. Ybarra ML, Boyd D, Korchmaros JD, Oppenheim J. Defining and measuring cyberbullying within the larger context of bullying victimization. J Adolesc Health. (2012) 51:53–8. doi: 10.1016/j.jadohealth.2011.12.031
86. Kowalski RM, Limber SP, McCord A. A developmental approach to cyberbullying: prevalence and protective factors. Aggress Violent Behav. (2019) 45:20–32. doi: 10.1016/j.avb.2018.02.009
87. Dilmac B, Yurt E, Aydin M, Kasarci I. Predictive relationship between humane values of adolescents cyberbullying and cyberbullying sensibility. Electron J Res Educ Psychol. (2016) 14:3–22. doi: 10.14204/ejrep.38.14123
88. Reed KP, Cooper RL, Nugent WR, Russell K. Cyberbullying: a literature review of its relationship to adolescent depression and current intervention strategies. J Hum Behav Soc Environ. (2016) 26:37–45. doi: 10.1080/10911359.2015.1059165
Keywords: cyberbullying, children, adolescents, globalization, risk factors, preventive measures
Citation: Zhu C, Huang S, Evans R and Zhang W (2021) Cyberbullying Among Adolescents and Children: A Comprehensive Review of the Global Situation, Risk Factors, and Preventive Measures. Front. Public Health 9:634909. doi: 10.3389/fpubh.2021.634909
Received: 29 November 2020; Accepted: 10 February 2021; Published: 11 March 2021.
Reviewed by:
Copyright © 2021 Zhu, Huang, Evans and Zhang. This is an open-access article distributed under the terms of the Creative Commons Attribution License (CC BY) . The use, distribution or reproduction in other forums is permitted, provided the original author(s) and the copyright owner(s) are credited and that the original publication in this journal is cited, in accordance with accepted academic practice. No use, distribution or reproduction is permitted which does not comply with these terms.
*Correspondence: Wei Zhang, weizhanghust@hust.edu.cn
† These authors have contributed equally to this work and share first authorship
Disclaimer: All claims expressed in this article are solely those of the authors and do not necessarily represent those of their affiliated organizations, or those of the publisher, the editors and the reviewers. Any product that may be evaluated in this article or claim that may be made by its manufacturer is not guaranteed or endorsed by the publisher.
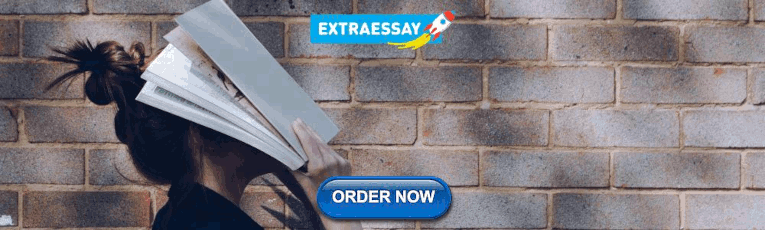
IMAGES
VIDEO