Click through the PLOS taxonomy to find articles in your field.
For more information about PLOS Subject Areas, click here .
Loading metrics
Open Access
Peer-reviewed
Research Article
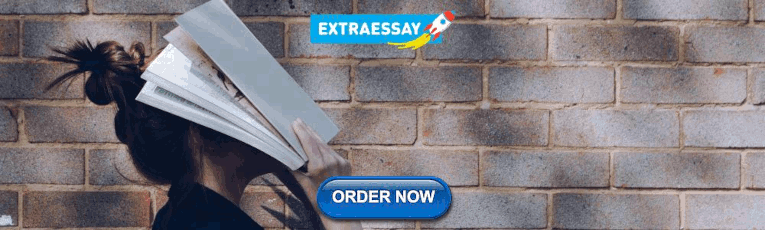
Assessment of publication bias and outcome reporting bias in systematic reviews of health services and delivery research: A meta-epidemiological study
Roles Data curation, Formal analysis, Investigation, Methodology, Project administration, Validation, Writing – original draft, Writing – review & editing
* E-mail: [email protected]
Affiliation Warwick Centre for Applied Health Research and Delivery, University of Warwick, Coventry, England, United Kingdom

Roles Funding acquisition, Investigation, Methodology, Writing – review & editing
Affiliation Health Services Management Centre, University of Birmingham, Birmingham, England, United Kingdom
Affiliation Department of Population Health and Primary Care, University of East Anglia, Norwich, England, United Kingdom
Roles Investigation, Methodology, Writing – review & editing
Affiliation Institute of Applied Health Research, University of Birmingham, Birmingham, England, United Kingdom
Roles Conceptualization, Funding acquisition, Investigation, Methodology, Writing – review & editing
Roles Conceptualization, Funding acquisition, Investigation, Methodology, Project administration, Supervision, Validation, Writing – review & editing
- Abimbola A. Ayorinde,
- Iestyn Williams,
- Russell Mannion,
- Fujian Song,
- Magdalena Skrybant,
- Richard J. Lilford,
- Yen-Fu Chen
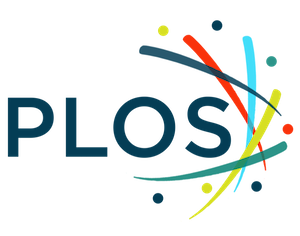
- Published: January 30, 2020
- https://doi.org/10.1371/journal.pone.0227580
- Peer Review
- Reader Comments
Strategies to identify and mitigate publication bias and outcome reporting bias are frequently adopted in systematic reviews of clinical interventions but it is not clear how often these are applied in systematic reviews relating to quantitative health services and delivery research (HSDR). We examined whether these biases are mentioned and/or otherwise assessed in HSDR systematic reviews, and evaluated associating factors to inform future practice. We randomly selected 200 quantitative HSDR systematic reviews published in the English language from 2007–2017 from the Health Systems Evidence database ( www.healthsystemsevidence.org ). We extracted data on factors that may influence whether or not authors mention and/or assess publication bias or outcome reporting bias. We found that 43% (n = 85) of the reviews mentioned publication bias and 10% (n = 19) formally assessed it. Outcome reporting bias was mentioned and assessed in 17% (n = 34) of all the systematic reviews. Insufficient number of studies, heterogeneity and lack of pre-registered protocols were the most commonly reported impediments to assessing the biases. In multivariable logistic regression models, both mentioning and formal assessment of publication bias were associated with: inclusion of a meta-analysis; being a review of intervention rather than association studies; higher journal impact factor, and; reporting the use of systematic review guidelines. Assessment of outcome reporting bias was associated with: being an intervention review; authors reporting the use of Grading of Recommendations, Assessment, Development and Evaluations (GRADE), and; inclusion of only controlled trials. Publication bias and outcome reporting bias are infrequently assessed in HSDR systematic reviews. This may reflect the inherent heterogeneity of HSDR evidence and different methodological approaches to synthesising the evidence, lack of awareness of such biases, limits of current tools and lack of pre-registered study protocols for assessing such biases. Strategies to help raise awareness of the biases, and methods to minimise their occurrence and mitigate their impacts on HSDR systematic reviews, are needed.
Citation: Ayorinde AA, Williams I, Mannion R, Song F, Skrybant M, Lilford RJ, et al. (2020) Assessment of publication bias and outcome reporting bias in systematic reviews of health services and delivery research: A meta-epidemiological study. PLoS ONE 15(1): e0227580. https://doi.org/10.1371/journal.pone.0227580
Editor: Tim Mathes, Universitat Witten/Herdecke, GERMANY
Received: September 13, 2019; Accepted: December 20, 2019; Published: January 30, 2020
Copyright: © 2020 Ayorinde et al. This is an open access article distributed under the terms of the Creative Commons Attribution License , which permits unrestricted use, distribution, and reproduction in any medium, provided the original author and source are credited.
Data Availability: We have archived the dataset in Warwick Research Archive Portal and have been given this link http://wrap.warwick.ac.uk/131604 .
Funding: This project is funded by the UK National Institute for Health Research (NIHR) Health Services and Delivery Research Programme (project grant number 15/71/06). https://www.nihr.ac.uk/ . AA, MS, RJL and YFC are also supported by the NIHR Collaboration for Leadership in Applied Health Research and Care West Midlands (NIHR CLAHRC WM), now recommissioned as NIHR Applied Research Collaboration West Midlands. The views expressed in this publication are those of the authors and not necessarily those of the NIHR or the Department of Health and Social Care. The funders had no role in study design, data collection and analysis, decision to publish, or preparation of the manuscript.
Competing interests: The authors have declared that no competing interests exist.
Introduction
Health services and delivery research (HSDR) can be defined as “research that is used to produce evidence on the quality, accessibility and organisation of health services including evaluation of how healthcare organisations might improve the delivery of services” [ 1 ]. Whilst clinical research into understanding biochemical mechanisms of diseases and their treatments has to some extent dominated health research, the importance of HSDR is increasingly being recognised [ 2 ]. For example, a study examining research grants that could impact upon childhood mortality in low-income countries found that 97% of grants were allocated to developing new health technologies, leading to a potential reduction in child death of about 22%, compared to a potential reduction of 63% from research aimed at improving the delivery and utilization of existing technologies [ 3 ]. Such finding suggests that while there is a need for research on effective treatments, there is arguably an even greater need for research on the delivery systems that support front line care [ 4 ]. With increasing recognition of the importance of HSDR has come increased scrutiny [ 5 ]. As with many other fields of research, systematic reviews have proven to be an important tool for summarising and synthesising the rapidly expanding evidence base. The validity of systematic reviews, however, can be undermined by publication bias, which occurs when the publication or non-publication of research findings is determined by the direction or strength of the evidence [ 6 ], and by outcome reporting bias whereby only a subset of outcomes, typically those most favourable, are reported [ 7 ]. Consequently, the findings that are published (and therefore more likely to be included in systematic reviews) may differ systematically from those that remain unpublished. This results in a biased summary of the evidence which in turn can impair decision making. In HSDR, this could have substantial implications for population health and resource allocation.
To minimise the potential for such biases, mitigating strategies are often included in the process of systematic reviewing. These include: comprehensive literature searching including attempts to locate grey literature or unpublished studies; assessment of outcome reporting bias of included studies; and assessment of potential publication bias using funnel plots, related regression methods and/or other techniques [ 8 ]. The level of adoption of such strategies in systematic reviews has been shown to vary by subject area. For example, a study from 2010 which assessed four categories of systematic review from MEDLINE showed that publication bias was assessed in 21% of treatment intervention reviews, 24% of diagnostic test accuracy reviews, 31% of reviews focusing on association between risk factors and health outcomes, and 54% of genetic reviews assessing association between genes and disease [ 6 ]. Another study which examined a random sample of 300 systematic reviews of biomedical research indexed in MEDLINE in February 2014 found that 31% had formally assessed publication bias [ 9 ]. However, a study examining the reporting characteristics and methodological quality of 99 systematic reviews of health policy research generated by the Cochrane Effective Practice and Organisation of Care Review Group prior to 2014 reported that only 9% of the reviews explicitly assessed publication bias [ 10 ]. These findings suggest that the assessment of publication bias is generally low in systematic reviews of clinical research and may be even lower in HSDR and policy research. More detailed information from a broader range of reviews is required to better understand current practice relating to the assessment of publication bias and outcome reporting bias in HSDR systematic reviews. Against this background, the objectives of this study are to examine whether publication bias and outcome reporting bias are mentioned and/or assessed in a representative sample of HSDR systematic reviews, and to summarise the methods adopted as well as findings reported or reasons stated for not formally assessing the biases.
We focus on systematic reviews of quantitative HSDR studies that involve evaluation of strength and direction of effects, which can be subject to hypothesis testing. Within this broad category, we sampled two review types:
- Intervention reviews, which aim to evaluate the effectiveness of service delivery interventions. These reviews often include randomised controlled trials (RCTs), other quasi-experimental studies and sometimes uncontrolled before-and-after studies, and
- Association reviews, which evaluate associations between different variables (such as nurse-patient ratio, frequency of patient monitoring and in-hospital mortality) along the service delivery causal chain [ 4 ]. Association reviews tend to include mostly observational studies.
While intervention reviews usually set out to examine pre-specified causal relationships between an intervention and designated outcomes, association reviews tend to be exploratory. Consequently, the characteristics (such as inclusion of meta-analysis, number and design of included studies, and the use of systematic review guidelines) of these two types of reviews may differ. We hypothesised that association studies may be more susceptible to publication and outcome reporting biases than intervention studies due to the exploratory nature of most association studies. We therefore investigate whether the practice of assessing these biases and the findings of these assessments differ between HSDR systematic reviews focusing on these two types of studies. In addition, we examine whether awareness and/or assessment of publication and outcome reporting biases is associated with factors other than the nature of the review, such as author’s use and journal’s endorsement of methodological guidelines for the conduct and reporting of systematic reviews, and journal impact factor [ 11 ].
We carried out a meta-epidemiological study [ 12 ] to estimate the frequency with which publication and outcome reporting bias were considered in systematic reviews and to explore factors associated with consideration of these forms of potential bias. The review was pre-registered in the PROSPERO International prospective register of systematic reviews (2016: CRD42016052366 www.crd.york.ac.uk/prospero/display_record.php?ID=CRD42016052366 ).
Sampling strategy
Our initial plan for identifying a sample of HSDR systematic reviews specified in the PROSPERO registration record was to conduct a literature search by using a combination of different information sources and searching methods [ 13 ]. Retrieved records would subsequently be screened for eligibility and classified as intervention or association reviews before a random sample was selected. However, the proposed sampling strategy was subsequently deemed not feasible given the large number of systematic reviews that would have to be checked for eligibility before sampling. This is due to the methodological diversity of HSDR-related research and the absence of universally accepted terms through which to search for HSDR systematic reviews. We therefore adopted the alternative method of selecting systematic reviews from the Health Systems Evidence (HSE) database ( www.healthsystemsevidence.org ) [ 14 ]. The HSE is a continuously updated repository of syntheses of research evidence about the governance, financial and delivery arrangements within health systems, and the implementation strategies that can support change in health systems [ 14 ]. It covers several databases including Medline and Cochrane Database of Systematic Reviews. With the help of the owner of the database, we downloaded all the available citations of systematic reviews indexed in the HSE as of August 2017 into a Microsoft Excel spreadsheet. The HSE classifies each of the systematic reviews into two groups based on the type of question the reviews address; ‘effectiveness’ for the systematic reviews concerned with effects and ‘other questions’. The reviews classed as effectiveness (n = 4416) served as the sampling frame for the intervention reviews while those classed as ‘others’ (n = 1505) were used for the association reviews. In order to facilitate random selection of reviews, we assigned a random number to each record using the RAND() function in Excel, sorted the random numbers in ascending order before screening the records for eligibility using pre-specified criteria, as described below, until the desired number of reviews was identified.
Sample size
We aimed to include 200 systematic reviews in total; 100 reviews of intervention studies and 100 reviews of association studies. This sample size has a statistical power of 80% to detect a 20% difference in the characteristics and findings between the two types of review, assuming a baseline rate of 32%, based on the proportion of Cochrane EPOC reviews in which publication bias was formally assessed or for which partial information was given [ 10 ].
Eligibility criteria
In this study, a systematic review was defined as any literature review which presents explicit statements with regard to research question(s), search strategy and criteria for study selection. We also defined HSDR as research that produces evidence on the quality, accessibility and organisation of health services based on the definition adopted by the United Kingdom’s National Institute for Health Research (NIHR) Health Services & Delivery Research Programme [ 1 ]. Systematic reviews examining quantitative data and relating to any aspects of HSDR were selected irrespective of whether they included a meta-analysis. To be eligible, the systematic review had to report at least one quantitative effect estimate or a statistical test which could be derived from the studies included in the review. Since contemporary literature are of more relevance to current practice, we included reviews from the last ten years (between2007 to 2017). We excluded records which were: not systematic reviews; not related to HSDR; not concerned with interventions or associations; not examining quantitative data; not published in English language; or were published before the year 2007. We also excluded systematic reviews that are usually classified as health technology assessment (such as those investigating effectiveness and cost effectiveness of clinical interventions) and those classified as clinical or genetic epidemiology (that is, those examining association between risk factors and disease conditions). Where more than one review within the initially selected samples cover overlapping interventions or associations, we included the latest review. This helped to maintain the independence of observations and also capture the contemporary practice.
Sample selection was conducted by one author (AAA) and checked by a second author (YFC). Discrepancies were resolved by discussion and members of the research project management team (in the first instance) and steering committee were consulted when the two authors could not reach an agreement or when generic issues concerning study eligibility criteria were identified.
Data extraction and classification of review characteristics
Data extraction focused on general systematic review characteristics and components that may influence whether or not authors refer to and/or assess publication bias or outcome reporting bias. Thus, data extracted from each eligible review included:
- key study question(s)
- databases searched
- whether an attempt was made to search grey literature and unpublished reports or whether reasons for not doing this were provided
- design of included studies (whether or not these are confined to controlled trials)
- number of included studies (categorised into <10 and ≥10 based on the minimum number of studies recommended for statistical approaches to assessment of publication bias[ 15 ])
- Whether meta-analyses were performed
- whether the use of systematic review related guidelines was reported (we assumed that all Cochrane reviews adhered to the Methodological Expectations of Cochrane Intervention Reviews (MECIR) standards [ 16 ] even if not reported by authors)
- whether the use of Grading of Recommendations, Assessment, Development and Evaluations (GRADE) was reported
- any mentioning of publication bias and/or outcome reporting bias
- methods (if used at all) for assessing potential publication bias and/or outcome reporting bias
- findings of assessment of publication bias and/or outcome reporting bias or reasons for not formally assessing these
We planned to categorise the types of journals in which the systematic reviews were published based on subject categories of the Journal Citation Reports (ISI Web of Knowledge, Thomson Reuters) as medical journals, health services research and health policy journals, management and social science journals or others(including grey literature), but discovered substantial overlap in the features between journal types which hindered reliable classification of some journals and in turn would cause difficulty in the interpretation of observations made based on the classification. We discussed this issue with the study steering committee members, who suggested us to use journal endorsement of systematic review guidelines and journal impact factors to characterise the journals instead.
Some journals/media require submitted systematic reviews to follow specific systematic review guidelines, for example, PRISMA statement [ 17 ], MOOSE checklist [ 18 ], MECIR standards (for Cochrane reviews) [ 16 ]. Such guidelines includes items on publication bias and may prompt reviewers to consider publication bias, particularly at the manuscript preparation stage. Based on the information available on journal websites, we categorised the journal/media in which the systematic reviews were published into those which formally endorse specific systematic review guidelines and those that do not (as of year 2018). Targeting prestigious journals for publication may also prompt reviewers to be more rigorous and so we identified the five year impact factors (as of year 2016) for the journal each review was published in from ISI Web of Knowledge, Thomson Reuters. When impact factor was not available on the Web of Knowledge website, it was obtained from other sources such as directly from the journal website. We imputed an impact factor of zero for journals with no impact factors and grey literature (such as theses). One author carried out all the data extraction and the data was independently checked by another author. Any discrepancies were resolved by discussion.
Quality assessment of included systematic reviews
Each systematic review included in the HSE was assessed independently by two reviewers using the Assessing the Methodological Quality of Systematic Reviews (AMSTAR) and the score was provided within the record for each review [ 19 ]. However, five of the selected systematic reviews had missing AMSTAR scores so two authors independently carried out the quality assessment for them using the same version of the AMSTAR tool as the remaining 195 systematic reviews. Discrepancies were resolved by discussion. Percentage AMSTAR scores were computed for each review taking into account the number of items (the denominator) that was applicable to individual reviews.
Statistical analysis
Descriptive statistics were used to summarise the characteristics of the selected HSDR systematic reviews, the practice of assessing publication bias and outcome reporting bias among the reviews, and their findings. Differences between association reviews and intervention reviews were explored. We presented confidence intervals to indicate the levels of uncertainty but avoided quoting p values and inferring to statistical significance given the descriptive nature of the study and the large number of exploratory comparisons made.
Three measures related to the awareness and actual practice of assessing publication and outcome reporting biases were evaluated:
- “mentioned publication bias”, that is, authors included statements related to publication bias in their report regardless of whether or not this was accompanied by formal assessment (with explicitly stated methods, e.g. use of funnel plots or comparison with findings from search of study registries known to capture all related studies that have been conducted; the latter is unlikely to be feasible in HSDR);
- “assessed publication bias”, which includes only those reviews where publication bias was formally assessed, and
- “assessed outcome reporting bias” where authors have assessed outcome reporting bias.
Univariable and multivariable logistic regressions were used to explore review and journal characteristics associated with mentioning/assessment of publication bias and outcome reporting bias in the reviews. The strength of association between these variables and practice of bias assessment was presented as an odds ratio (OR) with 95% confidence intervals.
Sampling of HSDR systematic reviews from HSE
We screened 220 of the 4416 systematic reviews classified as ‘systematic reviews of effects’ in the HSE to obtain 100 eligible systematic reviews of intervention for this study. Reviews were excluded mainly because their topics fell outside our definition of HSDR, such as those considered as public health research and health technology assessments. We screen all 1505 systematic reviews classified as ‘systematic reviews addressing other questions’ to identify 100 eligible systematic reviews of association for this study. Reviews were excluded because the topics under review fell outside our definitions of HSDR and association studies, and/or because their designs did not include a quantitative component, such as reviews adopting narrative and qualitative synthesis approaches and scoping reviews.
Characteristics of included intervention and association reviews
The characteristics of the included systematic reviews (100 intervention reviews and 100 association reviews) are shown in Table 1 . The majority of the 200 systematic reviews (79%) included at least ten studies but less than a quarter (22%) included a meta-analysis. Ninety of the reviews that did not include meta-analysis provided reasons for this–mainly small number of comparable studies and high heterogeneity between studies. Searches of grey/unpublished literature were conducted in 52% of the systematic reviews. Quality assessment of individual studies was performed in 79% of the systematic reviews but only 12% reported using GRADE for assessing the overall quality of evidence. The systematic reviews were of moderate quality with median AMSTAR score of 60% (IQR 44% to 73%). Many of the systematic reviews (70%) were published in journals which endorse PRISMA, although the use of such guidelines were only reported in 37% of them.
- PPT PowerPoint slide
- PNG larger image
- TIFF original image
https://doi.org/10.1371/journal.pone.0227580.t001
We observed notable differences between intervention and association reviews in many of the characteristics assessed. For example, intervention reviews were more likely to: include meta-analysis, inclusion of only controlled trials, carry out quality assessment of included studies, report the use of systematic review reporting guidelines and GRADE, have higher AMSTAR ratings and be published in journals with higher impact factors ( Table 1 ). Conversely, association reviews were more likely to include ten or more studies compared with intervention reviews (86% vs 71%). Only the search of grey literature and being published in journal which endorse systematic review guideline were similar in both intervention and association reviews.
Publication bias
Eighty-five (43%) of the systematic reviews mentioned publication bias and these included a higher proportion of intervention reviews than association reviews (54% vs 31%). Only about 10% (n = 19/200) formally assessed publication bias through statistical analysis, mostly using funnel plots and related methods. Again, intervention reviews assessed publication bias more frequently compared to association reviews (14% vs 5%; Table 1 ). Some evidence of publication bias (strictly speaking, evidence of small study effects in most instances) was reported in five (26%) of the reviews which assessed publication bias. The remaining reviews mostly reported low/no risk of publication bias. One review, which included four studies, constructed a funnel plot but reported that it was not very informative due to small numbers [ 20 ]. In five of the systematic reviews, authors reported planning statistical assessment of publication bias but did not carry out the assessment due to the conditions of using funnel plots not being met, especially insufficient number of studies and/or heterogeneity between included studies.[ 21 – 25 ]
Factors associated with mentioning (including assessing) publication bias.
In the univariable analysis, publication bias was more likely to be mentioned in intervention reviews when compared to association reviews (OR 2.61, 95% CI 1.47–4.66). Reviews which included meta-analysis were more than five times more likely to mention publication bias compared to those with no meta-analysis (OR 5.71, 95% CI 2.67–12.21). Mentioning publication bias appeared to be associated with quality assessment of individual studies, authors reporting the use of GRADE, journal impact factor, and authors reporting the use of systematic review guideline ( Table 2 ). Most of the apparent associations attenuated in the multivariable analysis, indicating some levels of interaction between these factors. Inclusion of meta-analysis remained strongly associated with mentioning publication bias ( Table 2 ).
https://doi.org/10.1371/journal.pone.0227580.t002
Factors associated with assessing publication bias.
Intervention reviews were again more likely to include an assessment of publication bias than association reviews (OR 3.09, 95% CI 1.07–8.95). Of all factors assessed, inclusion of meta-analysis was the factor most strongly associated with assessment of publication bias (OR 112.32, 95% CI 14.35–879.03) in the univariable analysis. Only one of the 19 systematic reviews which assessed publication bias did not carry out a meta-analysis. Assessment of publication bias also appeared to be associated with the inclusion of only RCTs and controlled trials, journal impact factor and authors reporting the use of systematic review guidelines ( Table 3 ). Other factors including number of included studies, search of grey/unpublished literature, quality assessment of individual studies and journal endorsement of systematic review guidelines were not significantly associated with assessment of publication bias. In the multivariable analysis, the pattern of apparent associations largely remained the same, although the relationship between assessment of publication bias and two of the factors (types of review and journal impact factors) diminished after adjusting for other factors ( Table 3 ).
https://doi.org/10.1371/journal.pone.0227580.t003
Outcome reporting bias
Thirty-four (17%) of all the systematic reviews mentioned and assessed outcome reporting bias as part of quality assessment of included studies. None of the systematic reviews mentioned outcome reporting bias without assessing it. Again this was more frequent in intervention reviews than in association reviews (30% vs 4%). The majority of the reviews which assessed outcome reporting bias used the Cochrane risk of bias tool (n = 28/34) [ 26 ]. Two reviews used the Agency for Healthcare Research and Quality’s (AHRQ’s) Methods Guide for Effectiveness and Comparative Effectiveness Reviews [ 27 ], one used the Amsterdam-Maastricht Consensus List for Quality Assessment, while the remaining three reviews used unspecified or bespoke tools. Of the 34 reviews which assessed outcome reporting bias, 31 reported the findings, while the remaining three did not report the findings despite having reported assessing the bias in the methods section. Of the 31 reviews which reported the findings, 35% (n = 11/31) identified at least one study with high risk of selective outcome reporting, 32% (n = 10/31) judged all included studies to be low risk while the remaining 10 reviews (32%) had at least one study where the authors were unable to judge the risk of bias and were classed as ‘unclear’. In three reviews, lack of pre-registered protocols was reported as the reason for judging articles as ‘unclear’.[ 20 , 22 , 28 ] In a review in which the review authors explicitly stated that they did not search for study protocols, 13 out of the 19 studies included in the review was judged as ‘unclear’ with regard to selective outcome reporting.[ 29 ]
Factors associated with assessing outcome reporting bias.
Intervention reviews were about ten times as likely to include an assessment of outcome reporting bias compared to association reviews (OR 10.29, 95% CI 3.47–30.53). Assessment of outcome reporting bias was also strongly associated with authors reporting the use of GRADE (OR 9.66, 95% CI 3.77–24.77) and inclusion of RCTs or controlled trials only (OR 7.74, 95% CI 3.39–17.75). Number of included studies, inclusion of meta-analysis, journal impact factor, journal endorsement of systematic review reporting guidelines and authors reporting the use of systematic review guidelines also appeared to be associated with the assessment of outcome reporting bias ( Table 4 ). The variable relating to quality assessment of individual studies was not included in the regression analysis because all studies which assessed outcome reporting bias performed quality assessment of individual studies. Two variables remained strongly associated with assessing outcome reporting bias in the multivariable analysis: author reporting the use of GRADE and being an intervention review ( Table 4 ).
https://doi.org/10.1371/journal.pone.0227580.t004
We obtained a random sample of 200 quantitative systematic reviews in HSDR and examined their characteristics in relation to assessment of publication bias and outcome reporting bias. Only 10% of the systematic reviews formally assessed publication bias even though 43% mentioned publication bias. The majority of the systematic reviews (83%) neither mentioned nor assessed outcome reporting bias. A higher proportion of the intervention reviews mentioned and assessed both biases compared to the association reviews.
Strengths and limitations
One of the strengths of the current study is that a broad range of quantitative HSDR systematic reviews was examined. The HSE database, from which the systematic reviews were selected, covers multiple sources of literature, and our selection was neither limited to a single source of literature nor restricted to highly ranked journals as was the case in previous studies.[ 30 – 32 ] Also, study selection and data extraction were carried out by one person and checked by another in order to ensure accuracy and completeness.
We targeted intervention and association reviews with a quantitative component in HSDR as defined earlier in this paper. The concept of intervention reviews matched well with the category of ‘systematic reviews of effects’ in the HSE database where we drew our sample. However, clearly delineating association reviews and identifying those incorporating some quantitative components have proven challenging. We had to screening more than a thousand records classified as ‘systematic reviews addressing other questions’ in the HSE to obtain our required sample, as the majority of reviews in this category either adopted descriptive, narrative or qualitative approaches, or did not match our definition of an HSDR association review.
We ensured that we only include the latest systematic review whenever we identified more than one which covers overlapping topics. There may be some overlap in the studies included within different systematic reviews, but we do not believe this would have significant impact on our findings as our study focuses on the overall features and methodology of the sampled systematic reviews rather than on individual studies included within them. We not only examined the proportion of systematic reviews which mentioned/assessed publication bias but also explored a number of factors which may influence these. Although the sample size of 200 reviews is still relatively small, as evident by the large confidence intervals for the ORs obtained from the multivariable logistic regression analyses, we were able to identify a few factors that may influence assessment of publication and outcome reporting bias in HSDR systematic reviews. We are aware that the variables which we examined may interact in various ways, as indicated by the changes in the estimated ORs between univariable and multivariable analyses for some of the variables examined. The relationships between the factors that could impact upon assessment of publication and outcome reporting bias in HSDR systematic reviews are intricate and will require further research to clarify.
The association between journal’s endorsement and authors’ use of reporting guidelines and assessment of publication bias may not have been characterised very accurately in our study. We classified journals based on endorsement of reporting guidelines as of 2018 but we were not able to determine if this has been the case as at the time the systematic review authors prepared/published their manuscripts. Notwithstanding, journal endorsement of such guidelines may be an indication of the journal’s generic requirement of higher standard of reporting. Also, available reporting guidelines are mostly aimed at systematic reviews of intervention studies and authors of systematic reviews of association studies might not have considered that it was necessary to follow such guidelines, even if it was endorsed by the journal they published in. Alternatively, some authors might have used reporting guidelines during the preparation of their reviews without explicitly stating it.
HSE used AMSTAR to assess the quality of included systematic reviews. We also used the same tool to assess the quality of the five systematic reviews with missing AMSTAR scores in order to maintain consistency. However, AMSTAR was designed for quality assessment of systematic reviews of RCTs of interventions and therefore some of the items were not relevant for many of the systematic reviews in this study. An updated version of the tool, AMSTAR 2, was published in 2017 which includes items relevant to non-randomised studies and would have been more appropriate for assessing the qualities of the systematic reviews included in this study [ 33 ]. Another potential limitation of this study is that we only included systematic reviews of quantitative studies although HSDR involves a wide range of study design, including qualitative studies. However, we believe issues relating to publication bias and outcome reporting bias in qualitative research warrants separate investigation as the mechanisms and manifestation of such biases are likely to be different in qualitative research.
Explanation of results and implications
Overall, the awareness of publication bias in quantitative HSDR reviews seems comparable to those reported for reviews in some other fields, although formal assessment of publication bias is less common especially in association reviews. Table 5 shows that the level of documenting awareness of publication bias by at least mentioning it was generally low in systematic reviews examined in previous studies in various fields of biomedical research, with a notable exception among systematic reviews of genetic association studies in which 70% mentioned publication bias. Unlike publication bias where many authors did discuss the potential implications even when they were not able to assess it, outcome reporting bias was only mentioned when it was assessed. However, mentioning of outcome reporting bias was lower than 30% across the board (17% in the current study), with very low rates observed in reviews of HSDR association studies (4% in the current study) and reviews of epidemiological risk factors (3% [ 6 ]).
https://doi.org/10.1371/journal.pone.0227580.t005
A number of inter-related issues warrant further consideration when interpreting these findings and making recommendations. First, the research traditions and nature of evidence varies between different subject disciplines and may influence the perceived importance and relevance of considering publication and outcome reporting biases in the review process. These variations might have contributed to the apparently low prevalence of assessing and documenting these biases in HSDR reviews and wide variations observed in different disciplines. For example, we found that meta-analysis was conducted in only 33% of the HSDR intervention reviews. This is similar to 39% reported in a previous study of Cochrane reviews focusing on HSDR (health policy) interventions [ 10 ]. We found an even lower prevalence (10%) of including meta-analysis in HSDR association reviews. These figures are in contrast with at least 60% observed among both intervention and association reviews in clinical research ( Table 5 ). There is a general recognition that HSDR requires consideration of multiple factors in complex health systems,[ 4 ] and that evidence generated from HSDR tends to be context-specific.[ 35 – 37 ] It is therefore possible that HSDR systematic reviews which evaluate intervention effects and associations, and particularly the latter which examine associations between the myriads of structure, process, outcome measures and contextual factors, may tend to adopt a more configurative, descriptive approach (as opposed to the more aggregative, meta-analytical approach in reviews of various types of clinical research).[ 38 ] Since generating an overall estimate of a “true effect” is not the main focus, the issue of publication and outcome reporting biases may be perceived as unimportant or irrelevant in reviews adopting configurative approaches.
Furthermore, the diverse and context-specific nature of evidence in HSDR may have further impeded formal assessment of publication bias. Funnel plots and related techniques, the most commonly used methods, require that at least 10 studies of varied sample sizes that are addressing sufficiently similar questions and that have used compatible outcome measures to enable appropriate analyses [ 15 ]. In HSDR systematic reviews, the level of heterogeneity among included studies are often high and so reviewers are often not able to use these formal statistical techniques. Irrespective of the technical requirements, such statistical methods could only detect small study effects, which could be suggestive of publication bias but do not prove it, as several potential causes other than publication bias, such as issues related to study design, statistical artefact and chance, could also lead to the presence of small study effects [ 15 ].
With the inherent limitations of statistical tools, the most reliable way to directly assess publication and outcome reporting biases is by following up studies from protocol registration to see if the outcomes were subsequently published, as well as comparing the outcomes reported in protocols to those eventually reported in output publications. Mandatory registration of research protocols has been enforced among clinical studies on human subjects but not in other fields. The lack of prospective registration of study protocols has been a major barrier for evaluating publication and outcome reporting bias in HSDR as evidenced by the low prevalence of assessing these biases particularly among reviews of observational studies, e.g. 4% among HSDR association reviews in our study and 7% among epidemiological risk factor reviews examined by Song et al.[ 6 ]. Availability of pre-registered study protocols will potentially safeguard against publication and outcome reporting biases and also enable reviewers to assess those biases.
While pre-registration of study protocols is good research practice that should be encouraged irrespective of scientific disciplines, mandatory pre-registration of studies and their protocols in HSDR of different types of studies beyond clinical trials would require careful deliberation and assessment with regard to feasibility and practical value, weighing potential benefits against costs and potential harms. In the meantime, it is important to continue raising awareness of these biases and improving the levels of documenting the awareness when evidence from quantitative HSDR is synthesised. Our findings show that systematic reviews that report the use of a systematic review guideline are five times more likely than those that don’t to include an assessment of publication bias. Another study which evaluated the impact of the PRISMA Statement on reporting in systematic reviews published in high-impact surgical journals reported that the proportion of systematic reviews which assessed publication bias was significantly higher after the publication of PRISMA (53%) compared to before PRISMA (39%) [ 32 ]. Methodological standards such as Cochrane Collaboration’s Methodological Expectations of Cochrane Intervention Reviews (MECIR) and systematic reviews reporting guidelines such as PRISMA and MOOSE [ 18 ] are therefore likely to play an important role. Nevertheless, the sub-optimal level of documenting awareness found in this and other studies highlight that additional mechanisms may be required to enforce them. For example, although 70% of the systematic reviews in this study are published in journals which endorse systematic review guidelines, the use of such guidelines was only reported in 37% of the systematic reviews. Journal editors and peer reviewers can help ensure that review authors adhere to recommended guidelines which will in turn promote the consideration of publication bias.
All the reviews which assessed outcome reporting bas in the current study did so as part of quality assessment of individual studies, especially those that used the Cochrane risk of bias tool [ 26 ]. Outcome reporting bias is a standard item in the current Cochrane risk of bias tool [ 26 ], which is most widely used in intervention reviews. However, this item is not included in tools commonly used for assessing observational studies such as the Newcastle-Ottawa scale [ 39 ]. This may contribute, in part, to the much higher proportion of intervention reviews that assessed outcome reporting bias compared to association reviews. Given that the risk of outcome reporting bias is substantially higher for observational studies, this is an important deficit which developers of quality assessment tools for observational studies need to address in the future.
Finally, the search and inclusion of grey/unpublished literature remain a potentially important strategy in minimising the potential effect of publication bias. In this study, 52% of the selected systematic reviews reported searching at least one source of grey literature. This is comparable to that which was reported (64%) in a recent audit of systematic reviews published in high ranking journals such as Journal of the American Medical Association, The British Medical Journal, Lancet, Annals of Internal Medicine and the Cochrane Database of Systematic Reviews [ 30 ]. The slightly higher value reported in the audit may be attributable to inclusion of only high impact journals. Our study further showed that reviewers who searched for grey literature do not necessarily assess/discuss the potential effect of publication bias. This suggests some review authors might have followed the good practice of searching the grey/unpublished literature to ensure comprehensiveness without considering minimising publication bias as a rationale behind this. Alternatively, these authors may consider a comprehensive search as an ultimate strategy to mitigate potential publication bias and therefore deemed it unnecessary to assess and/or discuss the potential impact of publication bias. However, reviewers need to be aware that the search of grey literature alone is not enough to completely alleviate publication bias and it is often impractical to search all possible sources of grey literature. There is limited evidence suggesting that the quality and nature of data included in published HSDR studies differ from that included in grey literature [ 40 ]. Therefore, more empirical evidence is needed to guide future practice regarding search of grey/unpublished literature, taking into account the trade-off between biases averted and additional resources required.
Publication and outcome reporting biases are not consistently considered/assessed in HSDR systematic reviews. Formal assessment of publication bias and outcome reporting biases may not always be possible until a comprehensive registration of HSDR studies and their protocols becomes available. Notwithstanding this, review authors could still consider and acknowledge the potential implications of these biases on the findings. Adherence to existing systematic review guidelines may improve the consistency in assessment of these biases. Including items for outcome reporting bias in future quality assessment tools of observational studies would also be beneficial. The findings of this study would enhance awareness of publication and outcome reporting biases in HSDR systematic reviews and inform future systematic review methodologies and reporting.
Acknowledgments
We would like to thank the Health Systems Evidence for giving us access to the list of systematic reviews and Dr Kaelan Moat for facilitating it, and Alice Davis for her help in data checking. We also thank members of our Study Steering Committee for their helpful support and guidance through the project.
- 1. National Institute for Health Research. Health Services and Delivery Research [cited 2018 21 September]. https://www.nihr.ac.uk/funding-and-support/funding-for-research-studies/funding-programmes/health-services-and-delivery-research/ .
- View Article
- PubMed/NCBI
- Google Scholar
- 13. Chen Y-F, Lilford R, Mannion R, Williams I, Song F. An overview of current practice and findings related to publication bias in systematic reviews of intervention and association studies in health services and delivery research PROSPERO: International prospective register of systematic reviews2016 [updated 29 November 2016].
- 16. Cochrane Methods. The Methodological Expectations of Cochrane Intervention Reviews (MECIR) 2018 [9 May 2019]. https://methods.cochrane.org/mecir .
- 27. Agency for Healthcare Research and Quality. Methods Guide for Effectiveness and Comparative Effectiveness Reviews Rockville (MD): Agency for Healthcare Research and Quality (US); 2008. https://www.ncbi.nlm.nih.gov/books/NBK47095/ .
- 39. Wells GA, Shea B, O’Connell D, Peterson J, Welch V, Losos M, et al. The Newcastle-Ottawa Scale (NOS) for assessing the quality of nonrandomised studies in meta-analyses [13 February 2014]. http://www.ohri.ca/programs/clinical_epidemiology/oxford.asp .
- Research article
- Open access
- Published: 01 March 2017
Implicit bias in healthcare professionals: a systematic review
- Chloë FitzGerald 1 &
- Samia Hurst 1
BMC Medical Ethics volume 18 , Article number: 19 ( 2017 ) Cite this article
230k Accesses
1239 Citations
622 Altmetric
Metrics details
Implicit biases involve associations outside conscious awareness that lead to a negative evaluation of a person on the basis of irrelevant characteristics such as race or gender. This review examines the evidence that healthcare professionals display implicit biases towards patients.
PubMed, PsychINFO, PsychARTICLE and CINAHL were searched for peer-reviewed articles published between 1st March 2003 and 31st March 2013. Two reviewers assessed the eligibility of the identified papers based on precise content and quality criteria. The references of eligible papers were examined to identify further eligible studies.
Forty two articles were identified as eligible. Seventeen used an implicit measure (Implicit Association Test in fifteen and subliminal priming in two), to test the biases of healthcare professionals. Twenty five articles employed a between-subjects design, using vignettes to examine the influence of patient characteristics on healthcare professionals’ attitudes, diagnoses, and treatment decisions. The second method was included although it does not isolate implicit attitudes because it is recognised by psychologists who specialise in implicit cognition as a way of detecting the possible presence of implicit bias. Twenty seven studies examined racial/ethnic biases; ten other biases were investigated, including gender, age and weight. Thirty five articles found evidence of implicit bias in healthcare professionals; all the studies that investigated correlations found a significant positive relationship between level of implicit bias and lower quality of care.
The evidence indicates that healthcare professionals exhibit the same levels of implicit bias as the wider population. The interactions between multiple patient characteristics and between healthcare professional and patient characteristics reveal the complexity of the phenomenon of implicit bias and its influence on clinician-patient interaction. The most convincing studies from our review are those that combine the IAT and a method measuring the quality of treatment in the actual world. Correlational evidence indicates that biases are likely to influence diagnosis and treatment decisions and levels of care in some circumstances and need to be further investigated. Our review also indicates that there may sometimes be a gap between the norm of impartiality and the extent to which it is embraced by healthcare professionals for some of the tested characteristics.
Conclusions
Our findings highlight the need for the healthcare profession to address the role of implicit biases in disparities in healthcare. More research in actual care settings and a greater homogeneity in methods employed to test implicit biases in healthcare is needed.
Peer Review reports
A patient should not expect to receive a lower standard of care because of her race, age or any other irrelevant characteristic. However, implicit associations (unconscious, uncontrollable, or arational processes) may influence our judgements resulting in bias. Implicit biases occur between a group or category attribute, such as being black, and a negative evaluation (implicit prejudice) or another category attribute, such as being violent (implicit stereotype) [ 1 ]. Footnote 1 In addition to affecting judgements, implicit biases manifest in our non-verbal behaviour towards others, such as frequency of eye contact and physical proximity. Implicit biases explain a potential dissociation between what a person explicitly believes and wants to do (e.g. treat everyone equally) and the hidden influence of negative implicit associations on her thoughts and action (e.g. perceiving a black patient as less competent and thus deciding not to prescribe the patient a medication).
The term ‘bias’ is typically used to refer to both implicit stereotypes and prejudices and raises serious concerns in healthcare. Psychologists often define bias broadly; such as ‘the negative evaluation of one group and its members relative to another’ [ 2 ]. Another way to define bias is to stipulate that an implicit association represents a bias only when likely to have a negative impact on an already disadvantaged group; e.g. if someone associates young girls with dolls, this would count as a bias. It is not itself a negative evaluation, but it supports an image of femininity that may prevent girls from excelling in areas traditionally considered ‘masculine’ such as mathematics [ 3 ]. Another option is to stipulate that biases are not inherently bad, but only to be avoided when they incline us away from the truth [ 4 ].
In healthcare, we need to think carefully about exactly what is meant by bias. To fulfil the goal of delivering impartial care, healthcare professionals should be wary of any kind of negative evaluation they make that is linked to membership of a group or to a particular characteristic. The psychologists’ definition of bias thus may be adequate for the case of implicit prejudice; there are unlikely, in the context of healthcare, to be any justified reasons for negative evaluations related to group membership. The case of implicit stereotypes differs slightly because stereotypes can be damaging even when they are not negative per se. At least at a theoretical level, there is a difference between an implicit stereotype that leads to a distorted judgement and a legitimate association that correctly tracks real world statistical information. Here, the other definitions of bias presented above may prove more useful.
The majority of people tested from all over the world and within a wide range of demographics show responses to the most widely used test of implicit attitudes, the Implicit Association Test (IAT), that indicate a level of implicit anti-black bias [ 5 ]. Other biases tested include gender, ethnicity, nationality and sexual orientation; there is evidence that these implicit attitudes are widespread among the population worldwide and influence behaviour outside the laboratory [ 6 , 7 ]. For instance, one widely cited study found that simply changing names from white-sounding ones to black-sounding ones on CVs in the US had a negative effect on callbacks [ 8 ]. Implicit bias was suspected to be the culprit, and a replication of the study in Sweden, using Arab-sounding names instead of Swedish-sounding names, did in fact find a correlation between the HR professionals who preferred the CVs with Swedish-sounding names and a higher level of implicit bias towards Arabs [ 9 ].
We may consciously reject negative images and ideas associated with disadvantaged groups (and may belong to these groups ourselves), but we have all been immersed in cultures where these groups are constantly depicted in stereotyped and pejorative ways. Hence the description of ‘aversive racists’: those who explicitly reject racist ideas, but who are found to have implicit race bias when they take a race IAT [ 10 ]. Although there is currently a lack of understanding of the exact mechanism by which cultural immersion translates into implicit stereotypes and prejudices, the widespread presence of these biases in egalitarian-minded individuals suggests that culture has more influence than many previously thought.
The implicit biases of concern to health care professionals are those that operate to the disadvantage of those who are already vulnerable. Examples include minority ethnic populations, immigrants, the poor, low health-literacy individuals, sexual minorities, children, women, the elderly, the mentally ill, the overweight and the disabled, but anyone may be rendered vulnerable given a certain context [ 11 ]. The vulnerable in health-care are typically members of groups who are already disadvantaged on many levels. Work in political philosophy, such as the De-Shalit and Wolff concept of ‘corrosive disadvantage’, a disadvantage that is likely to lead to further disadvantages, is relevant here [ 12 ]. For instance, if a person is poor and constantly worried about making ends meet, this is a disadvantage in itself, but can be corrosive when it leads to further disadvantages. In a country such as Switzerland, where private health insurance is mandatory and yearly premiums can be lowered by increasing the deductible, a high deductible may lead such a person to refrain from visiting a physician because of the potential cost incurred. This, in turn, could mean that the diagnosis of a serious illness is delayed leading to poorer health. In this case, being poor is a corrosive disadvantage because it leads to a further disadvantage of poor health.
The presence of implicit biases among healthcare professionals and the effect on quality of clinical care is a cause for concern [ 13 , 14 , 15 ]. In the US, racial healthcare disparities are widely documented and implicit race bias is one possible cause. Two excellent literature reviews on the issue of implicit bias in healthcare have recently been published [ 16 , 17 ]. One is a narrative review that selects the most significant recent studies to provide a helpful overall picture of the current state of the research in healthcare on implicit bias [ 16 ]. The other is a systematic review that focusses solely on racial bias and thus captures only studies conducted in the US, where race is the most prominent issue [ 17 ]. Our review differs from the first because it poses a specific question, is systematic in its collection of studies, and includes an examination of studies solely employing the vignette method. Its systematic method lends weight to the evidence it provides and its inclusion of the vignette method enables it to compare two different literatures on bias in healthcare. It differs from the second because it includes all types of bias, not only racial; partly as a consequence, it captures many studies conducted outside the US. It is important to include studies conducted in non-US countries because race understood as white/black is not the source of the most potentially harmful stereotypes and disparities in all cultural contexts. For example, a recent vignette study in Switzerland found that in the German-speaking part of the country, physicians displayed negative bias in treatment decisions towards fictional Serbian patients (skin colour was unspecified, but it would typically be assumed to be white), but no significant negative bias towards fictional patients from Ghana (skin colour would be assumed to be black) [ 18 ]. In the Swiss German context, the issue of skin colour may thus be less significant for potential bias than that of country of origin. Footnote 2
Data sources and search strategy
Our research question was: do trained healthcare professionals display implicit biases towards certain types of patient? PubMed (Medline), PsychINFO, PsychARTICLE and CINAHL were searched for peer-reviewed articles published between 1st March 2003 and 31st March 2013. When we performed exploratory searches on PubMed before conducting the final search, we noticed that in 2003 there was a sharp increase in the number of articles on implicit bias and so we decided to begin from this year. The final searches were conducted on the 31st March 2013. We used a combination of subject headings and free text terms that related to the attitudes of healthcare professionals (e.g. “physician-patient relations”, “attitude of health personnel”), implicit biases (e.g. “prejudice”, “stereotyping”, “unconscious bias”), particular kinds of discrimination (e.g. “aversive racism”, anti-fat bias”, “women’s health”), and healthcare disparities (e.g. “health status disparities”, “delivery of health care”) which were combined with the Boolean operators “AND” and “OR”.
Study selection
3767 titles were retrieved and independently screened by the two reviewers (SH and CF). The titles that were agreed by both after discussion to be ineligible according to our inclusion criteria were discarded (3498) and the abstracts of the remaining articles (269) were independently screened by both reviewers. Abstracts that were agreed by both reviewers to be ineligible according to our inclusion criteria were discarded (241). When the ineligible abstracts were discarded, the remaining 28 articles were read and independently rated by us both. Out of these, 27 articles were agreed after discussion to merit inclusion in the final selection. One article was excluded at this stage because it did not fit our inclusion criteria (it did not employ the assumption method or an implicit measure). Additionally, the reference lists of these 27 articles were manually scanned by CF, and the full text articles resulting from this were independently read by both reviewers, resulting in the inclusion of a further 11 articles that both reviewers agreed fitted the inclusion criteria. After a repeat process of scanning the reference lists of the 11 articles from the second round, the final number of eligible articles was 42. All disagreements were resolved through discussion.
The inclusion criteria were:
Empirical study.
A method identifying implicit rather than explicit biases.
Participants were physicians or nurses who had completed their studies.
Written in English or another language spoken by CF or SH (CF: French, Italian, Spanish, Catalan; SH: French, Italian, German).
There is no clear consensus on the meaning of the term ‘implicit’. The term is used in psychology to refer to a feature or features of a mental process. We chose a wide negative definition of implicit processes, assuming that implicit social cognition is involved in the absence of any of the four features that characterise explicit cognition: intention, conscious availability, controllability, and the need for mental resources. This absence does not rule out the involvement of explicit processes, but indicates the presence of implicit processes. While most institutional policies against bias focus on explicit cognition, research on implicit bias shows that this is mistaken [ 6 ].
There is broad agreement in psychology that methods known as ‘implicit measures’, including the affective priming task, the IAT and the affective Simon task, reveal implicit attitudes [ 19 ]. We included articles using these measures. We also included studies that employed a method popular in bioethics literature that we label ‘the assumption method’. It involves measuring differences across participants in response to clinical vignettes, identical except for one feature, such as the race, of the character in the vignette. There is no direct measure of the implicitness or non-explicitness of the processes at work in participants; instead, there is an assumption that the majority are explicitly motivated to disregard factors such as race. If there is a statistically significant difference in the diagnosis or treatment prescribed correlated with –for example- the race of the patient, the researchers infer that it is partly a result of implicit processes in the physicians’ decision-making. The assumption method of measuring implicit bias has been used in a variety of naturalistic contexts where it is harder to bring subjects into the laboratory. It is recognised by psychologists who specialise in implicit cognition as a way of detecting the possible presence of implicit bias, if not as an implicit measure in itself [ 6 ].
Studies that used self-report questionnaires were not included because, although they can use subtle methods to estimate a subject’s attitudes, they are typically used in psychology as a measure of explicit mental processes. There are potential problems with the implicit/explicit distinction as applied to psychological measures and it may be preferable in future research to speak of ‘direct’ and ‘indirect’ measures, but for the purposes of the review we followed this convention in psychology. The original idea behind implicit measures was that they attempted to measure something other than explicit mental processes, whereas self-report questionnaires ask a subject direct questions and thus prompt a chain of explicit conscious reasoning in the subject.
Data extraction
Data were extracted by CF and reviewed by SH for accuracy and completeness. All disagreements with the information extracted were resolved through discussion. We contacted the corresponding author of an article to obtain information that was not available in the published manuscript that related to the nature of the presentation given to recruit participants, but received no response.
Identified studies
The eligible studies are described in Table 1 and their main characteristics are outlined in Table 2 . The most frequently examined biases were racial/ethnic and gender, but ten other biases were investigated (Table 2 ). Four of the assumption studies compared results from two or more countries to explore effects of differences in healthcare systems.
The 14 assumption method studies examining multiple biases investigated interactions between biases. They recorded the socio-demographic characteristics of the participants to reveal complex interactions between physician characteristics and the characteristics of the imaginary ‘patient’ in the vignette.
All IAT studies measured implicit prejudice; five also measured implicit stereotypes. When implicit prejudice is measured, words or images from one category are matched with positive or negative words (e.g., black faces with ‘pleasant’). When implicit stereotypes are measured, words or images from one category are matched with words from a conceptual category (e.g. female faces and ‘home’).
Nine IAT studies combined the IAT with a measure of physician behaviour or treatment decision to see if there were correlations between these and levels of implicit bias.
The subliminal priming studies were dissimilar: one was an exploratory study to see if certain diseases were stereotypically associated with African Americans, using faces as primes and reaction times to the names of diseases as the measure of implicit association; the other study used race words as primes and tested the effect of time pressure on responses to a clinical vignette.
A variety of media were used for the clinical vignette and the method of questioning participants within the assumption method. One unusual study used simulations of actual encounters with patients, hiring actors and using a set for the physicians to role-play. Physicians’ treatment decisions were recorded by observers, and the physician recorded his own diagnosis, prognosis and perceptions after the encounter.
Limitations
Of specific studies.
Limitations are detailed in Table 3 . Some studies failed to report response rates, or to provide full information on statistical methods or participant characteristics. Some had very small sample sizes and the majority did not mention calculating the power of their sample. Some authors explicitly informed participants of the purpose of the study, or gave participants questionnaires or other tests that indicated the subject of the study before presenting them with the vignette. For optimal results, participants should not be alerted to the particular patient characteristic(s) under study, particularly in an assumption study where knowing the characteristic(s) may influence the interpretation of the vignette. In IAT studies, this is less worrying because IAT effects are to some extent uncontrollable.
Of the field
Implicit bias in healthcare is an emerging field of research with no established methodology. This is to be expected and is not a problem in itself, but it does present an obstacle when conducting a review of this kind. The range of methods used and the variety of journals with differing standards and protocols for describing experiments made it difficult to compare the results. In addition, authors focusing on a particular bias (e.g. gender), often in combination with a particular health issue (e.g. heart disease), frequently did not appear to be familiar with one another’s research. This lack of familiarity meant that often used different terms to describe the same phenomenon, which also made conducting the review more difficult.
Few of the existing results can be described as ‘real world’ treatment outcomes. The two priming studies involved very small samples and were more exploratory than result-seeking [ 20 , 21 ]. The IAT and assumption studies were conducted under laboratory conditions. The only three studies conducted in naturalistic settings combined the IAT with measures of physician-patient interaction [ 22 , 23 , 24 ]. However, many of the assumption studies attempted to make their vignettes as realistic as possible by having them validated by clinicians [ 25 , 26 , 27 , 28 , 29 , 30 , 31 , 32 , 33 , 34 , 35 , 36 , 37 , 38 , 39 , 40 , 41 ] and also by having participants view/read the vignettes as part of a normal day at work [ 27 , 28 , 29 , 30 , 31 , 32 , 33 , 34 , 35 , 36 , 39 , 41 ].
Because the studies of interest used psychological techniques, but were mainly to be found in a medical database (PubMed), the classification of the studies was not always optimal. There is no heading in Medline for ‘implicit bias’ and studies using similar methods were sometimes categorized under different subject headings, some of which were introduced during the last ten years, which increased the risk of missing eligible studies.
Existence of implicit biases/stereotypes in healthcare professionals and influence on quality of care
Healthcare professionals have implicit biases.
Almost all studies found evidence for implicit biases among physicians and nurses. Based on the available evidence, physicians and nurses manifest implicit biases to a similar degree as the general population. The following characteristics are at issue: race/ethnicity, gender, socio-economic status (SES), age, mental illness, weight, having AIDS, brain injured patients perceived to have contributed to their injury, Footnote 3 intravenous drug users, disability, and social circumstances.
Of the seven studies that did not find evidence of bias, one compared the mentally ill with another potentially unfavourable category, welfare recipients; this study did find a positive correlation between levels of implicit bias and over-diagnosis of the mentally ill patient in the vignette [ 42 ]. Another used simulated interactions with actors, which may result in participants being on ‘best behaviour’ in the role-play [ 41 ]. The two studies that reported no evidence of bias in diagnosis of depression found that physicians’ estimates of SES were influenced by race (lower SES estimated for black patients); [ 37 , 38 ] one reported that estimates of SES in turn were significantly related to estimates of patient demeanour (lower SES associated with hostile patient demeanour) [ 37 ]. A further study failed to find differences due to patient race in the prescription of opioids, but found an interaction whereby black patients who exhibited ‘challenging’ behaviour (such as belligerence and asking for a specific opioid) were more likely to be prescribed opioids than those who did not, an effect possibly due to a racial stereotype [ 43 ]. Another study that failed to find implicit race bias suggested that this was due to the setting of the study in an inner-city clinic with high levels of black patients and the fact that many physicians were born outside the US [ 24 ]. Finally, one study that found no evidence of racial bias in prescription of opioid analgesics presented each participant with three vignettes depicting patients of three different ethnicities, thus probably alerting them to the objective of the study [ 40 ].
The interaction effects between different patient characteristics in assumption studies are varied and a few are surprising. The authors of one study expected that physicians would be less likely to prescribe a higher dose of opioids to black patients who exhibited challenging behaviours; in fact, physicians were more likely to prescribe higher doses of opioids to challenging black patients, yet slightly less likely to do so to white patients exhibiting the same behaviour. Sometimes significant effects on the responses to the vignette of a patient characteristic, e.g. race, are only found when the interaction between gender and race or SES and race is examined. For example, physicians in one study were less certain of the diagnosis of coronary heart disease for middle-aged women, who were thus twice as likely to receive a mental health diagnosis than their male counterparts [ 34 ]. In another, low SES Latinas and blacks were more likely to have intrauterine contraception recommended than low SES whites, but there was no effect of race for high SES patients [ 39 ].
Implicit bias affects clinical judgement and behaviour
Three studies found a significant correlation between high levels of physicians’ implicit bias against blacks on IAT scores and interaction that was negatively rated by black patients [ 23 , 24 , 44 ] and, in one study, also negatively rated by external observers [ 23 ]. Four studies examining the correlation between IAT scores and responses to clinical vignettes found a significant correlation between high levels of pro-white implicit bias and treatment responses that favoured patients specified as white [ 42 , 45 , 46 , 47 ]. In one study, implicit prejudice of nurses towards injecting drug users significantly mediated the relationship between job stress and their intention to change jobs [ 48 ].
Twenty out of 25 assumption studies found that some kind of bias was evident either in the diagnosis, the treatment recommendations, the number of questions asked of the patient, the number of tests ordered, or other responses indicating bias against the characteristic of the patient under examination.
Determinants of bias
Socio-demographic characteristics of physicians and nurses (e.g. gender, race, type of healthcare setting, years of experience, country where medical training received) are correlated with level of bias. In one study, male staff were significantly less sympathetic and more frustrated than female staff with self-harming patients presenting in A&E [ 26 ]. Black patients in the US –but not the UK- were significantly more likely to be questioned about smoking than white [ 28 ]. In another study, international medical graduates rated the African-American male patient in the vignette as being of significantly lower SES than did US graduates [ 38 ]. One study found that paediatricians held less implicit race bias compared with other MDs [ 47 ].
Correlations between explicit and implicit attitudes varied depending on the type of bias and on the kind of explicit questions asked. For instance, implicit anti-fat bias tends to correlate more with an explicit anti-fat bias than racial bias, where explicit and implicit attitudes often diverge significantly. Because physicians’ and nurses’ implicit attitudes diverged frequently from their explicit attitudes, explicit measures cannot be used alone to measure the presence of bias among healthcare professionals.
A variety of studies, conducted in various countries, using different methods, and testing different patient characteristics, found evidence of implicit biases among healthcare professionals and a negative correlation exists between level of implicit bias and indicators of quality of care. The two most common methods employed were the assumption method and the IAT, the latter sometimes combined with another measure to test for correlations with the behaviour of healthcare professionals.
Our study has several limitations. Four studies included participants who were not trained physicians or nurses and failed to report separate results for these categories of participants [ 42 , 44 , 49 , 50 ]. Since either the majority of participants were qualified physicians and nurses, or were other health care professionals involved in patient care, we included these studies despite this limitation. Excluding them would not have changed the conclusions of this paper. In addition, we initially centred our research on studies employing implicit measures recognised in psychology, but the majority of the included studies in the final review used the assumption method. However, the limitations imposed by the lack of consistency in keywords and categorization of articles actually worked in our favour here, enabling us to capture a variety of methods and thus to consider including the assumption method. Scanning the references of the articles that were initially retained and repeating this process until there were no new articles helped us to capture further pertinent articles. From the degree of cross-referencing we are confident that we succeeded in identifying most of the relevant articles using the assumption method.
Publication bias could limit the availability of results that reveal little or no implicit bias among healthcare professionals. Moreover, eight articles appeared to refer to the same data collected in a single cross-country comparison study [ 27 , 28 , 29 , 30 , 31 , 32 , 34 , 35 ] and a further two articles analysed the same data [ 45 , 47 ]. The sum of 42 articles thus can give the impression that more research has been carried out on more participants than is the case. The solidity of data revealing high levels of implicit bias among the general population suggest that this is unlikely to have invalidated the conclusion that implicit bias is present in healthcare professionals [ 6 , 7 ].
However, our decision to exclude studies that involved students rather than fully-trained healthcare professionals meant that we did not include a study conducted on medical students that showed no significant association between implicit bias and clinical assessments [ 51 ]. Several studies post 2013 (thus after our cut-off date) have also indicated a null relationship between levels of implicit bias and clinical decision-making [ 52 , 53 , 54 ]. The scientific community working in this area agrees that the relationship between levels of implicit bias in healthcare professionals and clinical decision-making is complex and that there is currently a lack of good evidence for a direct negative influence of biases [ 16 , 17 ]. As our review shows, there is clearer evidence for a relationship between implicit bias and negative effects on clinical interaction [ 23 , 24 , 44 ]. While this may not always translate into negative treatment outcomes, the relationship between a healthcare professional and her patient is essential to providing good treatment, thus it seems likely that the more negative the clinical interaction, the worse the eventual treatment outcome (not to mention the likelihood that the patient will consult healthcare services for future worries or problems). This is where the bulk of future research should be concentrated.
The interactions between multiple patient characteristics and between healthcare professional and patient characteristics reveal the complexity of the phenomenon of implicit bias and its influence on clinician-patient interaction. They also highlight the pertinence of work in feminist theory on ‘intersectionality’, a term for the distinctive issues that arise when a person belongs to multiple identity categories that bring disadvantage, such as being both black and female [ 55 ]. For instance, one study only found evidence of bias against low SES Latina patients, not against high SES Latinas, illustrating how belonging to more than one category (here, both low SES and Latina) can have negative effects that are not present if membership of one category is eliminated (here, low SES) [ 39 ]. Class may trump race in some circumstances so that being high SES is more salient than being non-white. One criticism of mainstream feminism by theorists who work on intersectionality is that pertinent issues are unexplored because of the dominance of high SES white women in feminist theory. Using our example from the review, high SES Latina women may not experience the same prejudice as low SES Latina women and thus may falsely assume that there is no prejudice against Latina women tout court in this context. This could be frustrating for low SES Latina women who have unrecognized lived experiences of prejudice in a clinical setting.
In some studies, the attitudes of patients towards healthcare professionals were recorded and used to evaluate clinical interaction [ 23 , 24 , 44 ]. It is important to remember that patients also may come to a clinical interaction with biases. In these cases, the biases of one participant may trigger the biases of the other, magnifying the first participant’s biased responses and leading to a snowball effects [ 56 ]. Past experience of discrimination may mean that a patient may come to an interaction with negative expectations [ 57 ].
Our findings in the review suggest that the relationship between training and experience and levels of implicit bias is mixed. In one study, increased contact with patients with Hepatitis C virus was associated with more favourable explicit attitudes, yet more negative implicit attitudes towards intravenous drug users [ 49 ]. Another study demonstrated that nursing students were less prejudiced, more willing to help and desired more social interaction with patients with brain injury, when compared with qualified nurses [ 58 ]. Exposure to communication skills training was not associated with lower race-IAT scores for physicians [ 23 ]. However, individuals with mental health training demonstrated more positive implicit and explicit evaluations of people with mental illness than those without training [ 42 ]. Yet in the same study, graduate students had more positive implicit attitudes towards the mentally ill than mental health professionals.
We included all types of implicit bias in our review, not only race bias, partly in an effort to capture non-US studies, hypothesising that the focus on race in the US leaves fewer resources for investigation into other biases. It is possibly the case that a wider range of biases were investigated in non-US countries, but there is not enough evidence to deduce this from our review alone. For instance, two British studies examine bias against brain-injured patients who are perceived as having contributed to their injury [ 58 , 59 ], and two Australian studies looked at bias against intravenous drug users [ 48 , 49 ], but the sample size of studies is too small to warrant drawing any conclusions from this.
Is it possible that there are implicit associations that are justified because they are based on prevalence data for diseases? One study in our review aimed to test the statistical discrimination hypothesis by asking physicians to estimate the prevalence data among males and females for coronary heart disease in addition to presenting them with vignettes of a female or male coronary heart disease patient. It found that 48% of physicians were inconsistent in their population-level and individual level assessments and that the physicians’ gender-based population prevalence assessments were not associated with the certainty of their diagnosis of coronary heart disease. There was no evidence to support the theory of statistical discrimination as an explanation for why physicians were less certain of their diagnoses of CHD in women [ 36 ]. Another exploratory study looked at the diseases that were stereotypically associated with African-Americans and found that many diseases were associated with African-Americans that did not match prevalence data, such as drug abuse [ 20 ]. The danger in these cases is that a physician may apply a group-level stereotype to an individual and fail to follow-up with a search for individuating information.
Impartial treatment of patients by healthcare professionals is an uncontroversial norm of healthcare. Implicit biases have been identified as one possible factor in healthcare disparities and our review reveals that they are likely to have a negative impact on patients from stigmatized groups. Our review also indicates that there may sometimes be a gap between the norm of impartiality and the extent to which it is embraced by healthcare professionals for some of the tested characteristics. For instance, explicit anti-fat bias was found to be prevalent among healthcare professionals [ 60 ]. Since weight can be relevant to diagnosis and treatment, it is understandable that it is salient. It is nonetheless disturbing that healthcare professionals exhibit the same explicit anti-fat attitudes prevalent in the general population.
The most convincing studies from our review are those that combine the IAT and a method measuring the quality of treatment in the actual world. These studies provide some evidence for a relationship between bias as measured by the IAT and behaviour by clinicians that may contribute to healthcare disparities. More studies using real-world interaction measures would be helpful because studies using vignettes remain open to the criticism that they do not reveal the true behaviour of healthcare professionals. In this respect, the three studies using measures of physician-patient interaction are exemplary [ 22 , 23 , 24 ], in particular when using independent evaluators of the interactions [ 23 ]. Overall, our review reveals the need for discussion of methodology and for more interaction between different literatures that focus on different biases.
Our findings highlight the need for the healthcare profession to address the role of implicit biases in disparities in healthcare. In addition to addressing implicit biases, measures need to be taken to raise awareness of the potential conflict between holding negative explicit attitudes towards some patient characteristics, such as obesity, and committing to a norm to treat all patients equally.
Our review reveals that this is an area in need of more uniform methods of research to enable better comparison and communication between researchers interested in different forms of bias. Important avenues for further research include examination of the interactions between patient characteristics, and between healthcare professional and patient characteristics, and of possible ways in which to tackle the presence of implicit biases in healthcare.
There are conceptual problems with this distinction as used in psychology that have been pointed out by philosophers, but we will ignore these for the purposes of this review.
Interestingly, physicians were also asked for how they expected their colleagues to rate the vignette, and in these ratings there was a negative bias towards both patients from Ghana and from Serbia.
Bias against patients who are seen as contributing to their injury initially seems to be an odd category compared to the more familiar ones of race and gender. Clinicians may treat brain injured patients differently if they are somehow seen as ‘responsible’ for their injury, for instance, if they were engaging in risk-taking behaviour such as drug taking. Our review was intended to capture studies such as these that identify biases that are specific to clinical contexts and thus of particular interest to clinicians.
Holroyd J, Sweetman J. The Heterogeneity of Implicit Bias. In Brownstein, Michael, Saul, Jennifer, editors. Implicit Bias and Philosophy, Volume 1: Metaphysics and Epistemology 1. Oxford: Oxford University Press; 2016. p. 80–103.
Blair IV, Steiner JF, Havranek EP. Unconscious (implicit) bias and health disparities: where do we go from here? Perm J. 2011;15:71.
Google Scholar
Ambady N, Shih M, Kim A, Pittinsky TL. Stereotype susceptibility in children: effects of identity activation on quantitative performance. Psychol Sci. 2001;12:385–90.
Article Google Scholar
Louise A. Bias: Friend or Foe? Reflections on Saulish Skepticism. In Brownstein, Michael, Saul, Jennifer, editors. Implicit Bias and Philosophy, Volume 1: Metaphysics and Epistemology 1. Oxford: Oxford University Press; 2016. p. 157–190.
Nosek BA, et al. Pervasiveness and correlates of implicit attitudes and stereotypes. Eur Rev Soc Psychol. 2007;18:36–88.
Nosek BA, Riskind RG. Policy implications of implicit social cognition. Soc Issues Policy Rev. 2012;6:113–47.
Jost JT, et al. The existence of implicit bias is beyond reasonable doubt: a refutation of ideological and methodological objections and executive summary of ten studies that no manager should ignore. Res Organ Behav. 2009;29:39–69.
Bertrand M, Mullainathan S. Are Emily and Greg more employable than lakisha and Jamal? a field experiment on labor market discrimination. Am Econ Rev. 2004;94:991–1013.
Rooth D-O. Automatic associations and discrimination in hiring: real world evidence. Labour Econ. 2010;17:523–34.
Dovidio JF, Gaertner SL. Aversive racism. Adv Exp Soc Psychol. 2004;36:1–52.
Martin AK, Tavaglione N, Hurst S. Resolving the conflict: Clarifying’ Vulnerability’ in health care ethics. Kennedy Inst Ethics J. 2014;24:51–72.
De-Shalit A, Wolff J. Disadvantage. Oxford: Oxford University Press; 2007.
Burgess D, van Ryn M, Dovidio J, Saha S. Reducing racial bias among health care providers: lessons from social-cognitive psychology. J Gen Intern Med. 2007;22:882–7.
Stone J, Moskowitz GB. Non-conscious bias in medical decision making: what can be done to reduce it? Med Educ. 2011;45:768–76.
Shavers VL, et al. The state of research on racial/ethnic discrimination in the receipt of health care. Am J Public Health. 2012;102:953–66.
Zestcott, C. A., Blair, I. V. & Stone, J. Examining the presence, consequences, and reduction of implicit bias in health care: A narrative review. Group Process Intergroup Relat. 2016. doi: 10.1177/1368430216642029 .
Hall WJ, et al. Implicit racial/ethnic bias among health care professionals and its influence on health care outcomes: a systematic review. Am J Public Health. 2015;105:e60–76.
Drewniak D, Krones T, Sauer C, Wild V. The influence of patients’ immigration background and residence permit status on treatment decisions in health care. Results of a factorial survey among general practitioners in Switzerland. Soc Sci Med. 2016;161:64–73.
De Houwer J, Moors A. How to define and examine the implicitness of implicit measures. In Wittenbrink B, Schwartz N. (eds.). Implicit measures of attitudes: Procedures and controversies. New York: Guilford Press; 2007. pp. 179–194.
Moskowitz GB, Stone J, Childs A. Implicit stereotyping and medical decisions: unconscious stereotype activation in practitioners’ thoughts about African americans. Am J Public Health. 2012;102:996–1001.
Stepanikova I. Racial-ethnic biases, time pressure, and medical decisions. J Health Soc Behav. 2012;53:329–43.
Blair IV, et al. Assessment of biases against Latinos and African americans among primary care providers and community members. Am J Public Health. 2013;103:92–8.
Cooper LA, et al. The associations of clinicians’ implicit attitudes about race with medical visit communication and patient ratings of interpersonal care. Am J Public Health. 2012;102:979–87.
Penner LA, et al. Aversive racism and medical interactions with black patients: a field study. J Exp Soc Psychol. 2010;46:436–40.
Protière C, Viens P, Rousseau F, Moatti JP. Prescribers’ attitudes toward elderly breast cancer patients. Discrimination or empathy? Crit Rev Oncol Hematol. 2010;75:138–50.
Mackay N, Barrowclough C. Accident and emergency staff’s perceptions of deliberate self-harm: attributions, emotions and willingness to help. Br J Clin Psychol. 2005;44:255–67.
Arber S, et al. Influence of patient characteristics on doctors’ questioning and lifestyle advice for coronary heart disease: a UK/US video experiment. Br J Gen Pract. 2004;54:673–8.
McKinlay J, et al. How do doctors in different countries manage the same patient? results of a factorial experiment. Health Serv Res. 2006;41:2182–200.
Lutfey KE, et al. Diagnostic certainty as a source of medical practice variation in coronary heart disease: results from a cross-national experiment of clinical decision making. Med Decis Making. 2009;29(5):606–18.
McKinlay JB, et al. Sources of variation in physician adherence with clinical guidelines: results from a factorial experiment. J Gen Intern Med. 2007;22:289–96.
Bönte M, et al. Women and men with coronary heart disease in three countries: are they treated differently? Womens Health Issues. 2008;18:191–8.
Arber S, et al. Patient characteristics and inequalities in doctors’ diagnostic and management strategies relating to CHD: a video-simulation experiment. Soc Sci Med. 2006;62:103–15.
Lutfey KE, Eva KW, Gerstenberger E, Link CL, McKinlay JB. Physician cognitive processing as a source of diagnostic and treatment disparities in coronary heart disease results of a factorial priming experiment. J Health Soc Behav. 2010;51:16–29.
Maserejian NN, Link CL, Lutfey KL, Marceau LD, McKinlay JB. Disparities in physicians’ interpretations of heart disease symptoms by patient gender: results of a video vignette factorial experiment. J Womens Health. 2009;18:1661–7.
Lutfey KE, Link CL, Grant RW, Marceau LD, McKinlay JB. Is certainty more important than diagnosis for understanding race and gender disparities?: An experiment using coronary heart disease and depression case vignettes. Health Policy. 2009;89:279–87.
Maserejian NN, Lutfey KE, McKinlay JB. Do physicians attend to base rates? prevalence data and statistical discrimination in the diagnosis of coronary heart disease. Health Serv Res. 2009;44:1933–49.
Kales HC, et al. Race, gender, and psychiatrists’ diagnosis and treatment of major depression among elderly patients. Psychiatr Serv. 2005;56:721–8.
Kales HC, et al. Effect of race and Sex on primary care Physicians’ diagnosis and treatment of late-life depression. J Am Geriatr Soc. 2005;53:777–84.
Dehlendorf C, et al. Recommendations for intrauterine contraception: a randomized trial of the effects of patients’ race/ethnicity and socioeconomic status. Am J Obstet Gynecol. 2010;203:319–e1.
Tamayo-Sarver JH, et al. The effect of race/ethnicity and desirable social characteristics on physicians’ decisions to prescribe opioid analgesics. Acad Emerg Med. 2003;10:1239–48.
Barnato AE, et al. A randomized trial of the effect of patient race on physician ICU and life-sustaining treatment decisions for an acutely unstable elder with end-stage cancer. Crit Care Med. 2011;39:1663.
Peris TS, Teachman BA, Nosek BA. Implicit and explicit stigma of mental illness: Links to clinical care. J Nerv Ment Dis. 2008;196:752–60.
Burgess DJ, et al. Patient race and physicians’ decisions to prescribe opioids for chronic low back pain. Soc Sci Med. 2008;67:1852–60.
Blair IV, et al. Clinicians’ implicit ethnic/racial bias and perceptions of care among black and Latino patients. Ann Fam Med. 2013;11:43–52.
Sabin JA, Greenwald AG. The influence of implicit bias on treatment recommendations for four common pediatric conditions: pain, urinary tract infection, attention deficit hyperactivity disorder, and asthma. Am J Public Health. 2012;102:988–95.
Green AR, et al. Implicit bias among physicians and its prediction of thrombolysis decisions for black and white patients. J Gen Intern Med. 2007;22:1231–8.
Sabin JA, Rivara FP, Greenwald AG. Physician implicit attitudes and stereotypes about race and quality of medical care. Med Care. 2008;46:678–85.
von Hippel W, Brener L, von Hippel C. Implicit prejudice toward injecting drug users predicts intentions to change jobs among drug and alcohol nurses. Psychol Sci. 2008;19:7–11.
Brener L, von Hippel W, Kippax S. Prejudice among health care workers toward injecting drug users with hepatitis C: does greater contact lead to less prejudice? Int J Drug Policy. 2007;18:381–7.
Schwartz MB, Chambliss HO, Brownell KD, Blair SN, Billington C. Weight bias among health professionals specializing in obesity. Obes Res. 2003;11:1033–9.
Haider AH, et al. Association of unconscious race and social class bias with vignette-based clinical assessments by medical students. JAMA. 2011;306:942–51.
Oliver MN, Wells KM, Joy-Gaba JA, Hawkins CB, Nosek BA. Do physicians’ implicit views of African americans affect clinical decision making? J Am Board Fam Med. 2014;27:177–88.
Blair IV, et al. An investigation of associations between clinicians’ ethnic or racial bias and hypertension treatment, medication adherence and blood pressure control. J Gen Intern Med. 2014;29:987–95.
Hirsh AT, Hollingshead NA, Ashburn-Nardo L, Kroenke K. The interaction of patient race, provider bias, and clinical ambiguity on pain management decisions. J Pain. 2015;16:558–68.
Cole ER. Intersectionality and research in psychology. Am Psychol. 2009;64:170.
Burgess DJ, Fu SS, Van Ryn M. Why do providers contribute to disparities and what can be done about it? J Gen Intern Med. 2004;19:1154–9.
Dominicé Dao M. Vulnerability in the clinic: case study of a transcultural consultation. J Med Ethics. 2016. doi: 10.1136/medethics-2015-103337 .
Linden MA, Redpath SJ. A comparative study of nursing attitudes towards young male survivors of brain injury: a questionnaire survey. Int J Nurs Stud. 2011;48:62–9.
Redpath SJ, et al. Healthcare professionals’ attitudes towards traumatic brain injury (TBI): the influence of profession, experience, aetiology and blame on prejudice towards survivors of brain injury. Brain Inj. 2010;24:802–11.
Sabin JA, Marini M, Nosek BA. Implicit and explicit anti-fat bias among a large sample of medical doctors by BMI, race/ethnicity and gender. PLoS One. 2012;7:e48448.
Li L, et al. Using case vignettes to measure HIV-related stigma among health professionals in China. Int J Epidemiol. 2007;36:178–84.
Aaberg VA. A path to greater inclusivity through understanding implicit attitudes toward disability. J Nurs Educ. 2012;51:505–10.
Abuful A, Gidron Y, Henkin Y. Physicians’ attitudes toward preventive therapy for coronary artery disease: is there a gender bias? Clin Cardiol. 2005;28:389–93.
Chow LY, Kam WK, Leung CM. Attitudes of healthcare professionals towards psychiatric patients in a general hospital in Hong Kong. J Psychiatry. 2007;17(1):3–9.
Neauport A, et al. Effects of a psychiatric label on medical residents’ attitudes. Int J Soc Psychiatry. 2012;58:485–7.
Barnhart JM, Wassertheil-Smoller S. The effect of race/ethnicity, sex, and social circumstances on coronary revascularization preferences: a vignette comparison. Cardiol Rev. 2006;14:215–22.
Sabin DJA, Nosek DBA, Greenwald DAG, Rivara DFP. Physicians’ implicit and explicit attitudes about race by MD race, ethnicity, and gender. J Health Care Poor Underserved. 2009;20:896.
Michael Vallis T, Currie B, Lawlor D, Ransom T. Healthcare professional bias against the obese: how do we know if we have a problem? Can J Diabetes. 2007;31:365–70.
Download references
Acknowledgements
Not applicable. Only the two authors were implicated in the review.
This work was carried out with the support of grants from the Swiss National Science Foundation under grants numbers: PP00P3_123340 and 32003B_149407.
Availability of data and materials
The search strategy is available in the Appendix to the paper.
Authors’ contributions
Both authors discussed to select the databases and decide on the research question, based on CF’s knowledge of the field of implicit bias and SH’s knowledge of systematic reviews and bioethics literature. CF compiled the key words for the search strategy with constant advice and input from SH. CF drafted the inclusion criteria and received constant input on this from SH: CF carried out the search and downloaded the relevant articles to be scrutinised. CF and SH both independently read all the initial titles to select which were relevant, then the abstracts, and then the final included articles and discussed at each stage to resolve any disagreements. CF drafted the initial tables including the information from the studies and this was revised by SH. SH particularly revised the statistical methods used by the studies and both reviewed their methodology. CF drafted the manuscript and it was revised with comments by SH a number of times until both authors were satisfied with the manuscript. Both authors read and approved the final manuscript.
Competing interest
The authors declare that they have no competing interests.
Ethics approval and consent to participate
Not applicable.
Author information
Authors and affiliations.
Institute for Ethics, History, and the Humanities, Faculty of Medicine University of Geneva, Genève, Switzerland
Chloë FitzGerald & Samia Hurst
You can also search for this author in PubMed Google Scholar
Corresponding author
Correspondence to Chloë FitzGerald .
Search Strategy
The following combination of subject headings and free text terms was used:
(“Prejudice” [MAJR] AND “Attitude of health personnel” [MAJR]) OR (“Attitude of health personnel/ethnology” [MH] AND “Prejudice”[MH]) OR (“Stereotyping”[MH] AND “Attitude of health personnel”) OR (“Prejudice”[MH] AND “Healthcare disparities” [MH]) OR (“Prejudice”[MH] AND “Cultural Competency” [MH]) OR (“Social Class” [MH] AND “Attitude of health personnel” [MH]) OR (“Prejudice”[MH] AND “Physicians” [MH]) OR (“Prejudice”[MAJR] AND “Delivery of Health Care”[MAJR] AND “stereotyping”[MAJR]) OR (“Physician-Patient Relations” [MH] AND “health status disparities”[MH]) OR (“Prejudice”[MH] AND “Obesity”[MH]) OR (“African Americans/psychology” [MH] AND “Healthcare disparities” [MH]) OR (“Prejudice”[MH] AND “Mentally Ill Persons”[MH]) OR (“Prejudice”[MH] AND “Women’s Health”[MH]) OR “aversive racism” OR “anti-fat bias” OR “racial-ethnic bias” OR “racial-ethnic biases” OR “ethnic/racial bias” OR “ethnic/racial biases” OR (“disabled persons”[MAJR] AND “prejudice”[MAJR])
Dates: 1st March 2003 to 31st March 2013
Final number of retrieved articles: 2510
PsychINFO and PsychARTICLE
The following combination of subject headings and free text terms was used was used:
Health personnel AND (prejudice OR bias)
Other filters: Scholarly journals
Final number of retrieved articles: 377
Final result when duplicates removed: 360.
Prejudice [MM Exact Major Subject Heading] OR stereotyping [MM Exact Major Subject Heading] OR Discrimination [MM Exact Major Subject Heading] OR implicit bias OR unconscious bias
Other filters:
Exclude Medline records
Peer reviewed
Final number of retrieved articles: 897
Rights and permissions
Open Access This article is distributed under the terms of the Creative Commons Attribution 4.0 International License ( http://creativecommons.org/licenses/by/4.0/ ), which permits unrestricted use, distribution, and reproduction in any medium, provided you give appropriate credit to the original author(s) and the source, provide a link to the Creative Commons license, and indicate if changes were made. The Creative Commons Public Domain Dedication waiver ( http://creativecommons.org/publicdomain/zero/1.0/ ) applies to the data made available in this article, unless otherwise stated.
Reprints and permissions
About this article
Cite this article.
FitzGerald, C., Hurst, S. Implicit bias in healthcare professionals: a systematic review. BMC Med Ethics 18 , 19 (2017). https://doi.org/10.1186/s12910-017-0179-8
Download citation
Received : 19 October 2016
Accepted : 14 February 2017
Published : 01 March 2017
DOI : https://doi.org/10.1186/s12910-017-0179-8
Share this article
Anyone you share the following link with will be able to read this content:
Sorry, a shareable link is not currently available for this article.
Provided by the Springer Nature SharedIt content-sharing initiative
- Implicit bias
- Stereotyping
- Attitudes of health personnel
- Healthcare disparities
BMC Medical Ethics
ISSN: 1472-6939
- General enquiries: [email protected]
- Open access
- Published: 05 December 2023
A scoping review to identify and organize literature trends of bias research within medical student and resident education
- Brianne E. Lewis 1 &
- Akshata R. Naik 2
BMC Medical Education volume 23 , Article number: 919 ( 2023 ) Cite this article
802 Accesses
1 Citations
2 Altmetric
Metrics details
Physician bias refers to the unconscious negative perceptions that physicians have of patients or their conditions. Medical schools and residency programs often incorporate training to reduce biases among their trainees. In order to assess trends and organize available literature, we conducted a scoping review with a goal to categorize different biases that are studied within medical student (MS), resident (Res) and mixed populations (MS and Res). We also characterized these studies based on their research goal as either documenting evidence of bias (EOB), bias intervention (BI) or both. These findings will provide data which can be used to identify gaps and inform future work across these criteria.
Online databases (PubMed, PsycINFO, WebofScience) were searched for articles published between 1980 and 2021. All references were imported into Covidence for independent screening against inclusion criteria. Conflicts were resolved by deliberation. Studies were sorted by goal: ‘evidence of bias’ and/or ‘bias intervention’, and by population (MS or Res or mixed) andinto descriptive categories of bias.
Of the initial 806 unique papers identified, a total of 139 articles fit the inclusion criteria for data extraction. The included studies were sorted into 11 categories of bias and showed that bias against race/ethnicity, specific diseases/conditions, and weight were the most researched topics. Of the studies included, there was a higher ratio of EOB:BI studies at the MS level. While at the Res level, a lower ratio of EOB:BI was found.
Conclusions
This study will be of interest to institutions, program directors and medical educators who wish to specifically address a category of bias and identify where there is a dearth of research. This study also underscores the need to introduce bias interventions at the MS level.
Peer Review reports
Physician bias ultimately impacts patient care by eroding the physician–patient relationship [ 1 , 2 , 3 , 4 ]. To overcome this issue, certain states require physicians to report a varying number of hours of implicit bias training as part of their recurring licensing requirement [ 5 , 6 ]. Research efforts on the influence of implicit bias on clinical decision-making gained traction after the “Unequal Treatment: Confronting Racial and Ethnic Disparities in Health Care” report published in 2003 [ 7 ]. This report sparked a conversation about the impact of bias against women, people of color, and other marginalized groups within healthcare. Bias from a healthcare provider has been shown to affect provider-patient communication and may also influence treatment decisions [ 8 , 9 ]. Nevertheless, opportunities within medical education curriculum are created to evaluate biases at an earlier stage of physician-training and provide instruction to intervene them [ 10 , 11 , 12 ]. We aimed to identify trends and organize literature on bias training provided during medical school and residency programs since the meaning of ‘bias’ is broad and encompasses several types of attitudes and predispositions [ 13 ].
Several reviews, narrative or systematic in nature, have been published in the field of bias research in medicine and healthcare [ 14 , 15 , 16 ]. Many of these reviews have a broad focus on implicit bias and they often fail to define the patient’s specific attributes- such as age, weight, disease, or condition against which physicians hold their biases. However, two recently published reviews categorized implicit biases into various descriptive characteristics albeit with research goals different than this study [ 17 , 18 ]. The study by Fitzgerald et al. reviewed literature focused on bias among physicians and nurses to highlight its role in healthcare disparities [ 17 ]. While the study by Gonzalez et al. focused on bias curricular interventions across professions related to social determinants of health such as education, law, medicine and social work [ 18 ]. Our research goal was to identify the various bias characteristics that are studied within medical student and/or resident populations and categorize them. Further, we were interested in whether biases were merely identified or if they were intervened. To address these deficits in the field and provide clarity, we utilized a scoping review approach to categorize the literature based on a) the bias addressed and b) the study goal within medical students (MS), residents (Res) and a mixed population (MS and Res).
To date no literature review has organized bias research by specific categories held solely by medical trainees (medical students and/or residents) and quantified intervention studies. We did not perform a quality assessment or outcome evaluation of the bias intervention strategies, as it was not the goal of this work and is standard with a scoping review methodology [ 19 , 20 ]. By generating a comprehensive list of bias categories researched among medical trainee population, we highlight areas of opportunity for future implicit bias research specifically within the undergraduate and graduate medical education curriculum. We anticipate that the results from this scoping review will be useful for educators, administrators, and stakeholders seeking to implement active programs or workshops that intervene specific biases in pre-clinical medical education and prepare physicians-in-training for patient encounters. Additionally, behavioral scientists who seek to support clinicians, and develop debiasing theories [ 21 ] and models may also find our results informative.
We conducted an exhaustive and focused scoping review and followed the methodological framework for scoping reviews as previously described in the literature [ 20 , 22 ]. This study aligned with the four goals of a scoping review [ 20 ]. We followed the first five out of the six steps outlined by Arksey and O’Malley’s to ensure our review’s validity 1) identifying the research question 2) identifying relevant studies 3) selecting the studies 4) charting the data and 5) collating, summarizing and reporting the results [ 22 ]. We did not follow the optional sixth step of undertaking consultation with key stakeholders as it was not needed to address our research question it [ 23 ]. Furthermore, we used Covidence systematic review software (Veritas Health Innovation, Melbourne, Australia) that aided in managing steps 2–5 presented above.
Research question, search strategy and inclusion criteria
The purpose of this study was to identify trends in bias research at the medical school and residency level. Prior to conducting our literature search we developed our research question and detailed the inclusion criteria, and generated the search syntax with the assistance from a medical librarian. Search syntax was adjusted to the requirements of the database. We searched PubMed, Web of Science, and PsycINFO using MeSH terms shown below.
Bias* [ti] OR prejudice*[ti] OR racism[ti] OR homophobia[ti] OR mistreatment[ti] OR sexism[ti] OR ageism[ti]) AND (prejudice [mh] OR "Bias"[Mesh:NoExp]) AND (Education, Medical [mh] OR Schools, Medical [mh] OR students, medical [mh] OR Internship and Residency [mh] OR “undergraduate medical education” OR “graduate medical education” OR “medical resident” OR “medical residents” OR “medical residency” OR “medical residencies” OR “medical schools” OR “medical school” OR “medical students” OR “medical student”) AND (curriculum [mh] OR program evaluation [mh] OR program development [mh] OR language* OR teaching OR material* OR instruction* OR train* OR program* OR curricul* OR workshop*
Our inclusion criteria incorporated studies which were either original research articles, or review articles that synthesized new data. We excluded publications that were not peer-reviewed or supported with data such as narrative reviews, opinion pieces, editorials, perspectives and commentaries. We included studies outside of the U.S. since the purpose of this work was to generate a comprehensive list of biases. Physicians, regardless of their country of origin, can hold biases against specific patient attributes [ 17 ]. Furthermore, physicians may practice in a different country than where they trained [ 24 ]. Manuscripts were included if they were published in the English language for which full-texts were available. Since the goal of this scoping review was to assess trends, we accepted studies published from 1980–2021.
Our inclusion criteria also considered the goal and the population of the study. We defined the study goal as either that documented evidence of bias or a program directed bias intervention. Evidence of bias (EOB) had to originate from the medical trainee regarding a patient attribute. Bias intervention (BI) studies involved strategies to counter biases such as activities, workshops, seminars or curricular innovations. The population studied had to include medical students (MS) or residents (Res) or mixed. We defined the study population as ‘mixed’ when it consisted of both MS and Res. Studies conducted on other healthcare professionals were included if MS or Res were also studied. Our search criteria excluded studies that documented bias against medical professionals (students, residents and clinicians) either by patients, medical schools, healthcare administrators or others, and was focused on studies where the biases were solely held by medical trainees (MS and Res).
Data extraction and analysis
Following the initial database search, references were downloaded and bulk uploaded into Covidence and duplicates were removed. After the initial screening of title and abstracts, full-texts were reviewed. Authors independently completed title and abstract screening, and full text reviews. Any conflicts at the stage of abstract screening were moved to full-text screening. Conflicts during full-text screening were resolved by deliberation and referring to the inclusion and exclusion criteria detailed in the research protocol. The level of agreement between the two authors for full text reviews as measured by inter-rater reliability was 0.72 (Cohen’s Kappa).
A data extraction template was created in Covidence to extract data from included full texts. Data extraction template included the following variables; country in which the study was conducted, year of publication, goal of the study (EOB, BI or both), population of the study (MS, Res or mixed) and the type of bias studied. Final data was exported to Microsoft Excel for quantification. For charting our data and categorizing the included studies, we followed the Preferred Reporting Items for Systematic Reviews and Meta-Analyses extension for Scoping Reviews(PRISMA-ScR) guidelines [ 25 ]. Results from this scoping review study are meant to provide a visual synthesis of existing bias research and identify gaps in knowledge.
Study selection
Our search strategy yielded a total of 892 unique abstracts which were imported into ‘Covidence’ for screening. A total of 86 duplicate references were removed. Then, 806 titles and abstracts were screened for relevance independently by the authors and 519 studies were excluded at this stage. Any conflicts among the reviewers at this stage were resolved by discussion and referring to the inclusion and exclusion criteria. Then a full text review of the remaining 287 papers was completed by the authors against the inclusion criteria for eligibility. Full text review was also conducted independently by the authors and any conflicts were resolved upon discussion. Finally, we included 139 studies which were used for data extraction (Fig. 1 ).
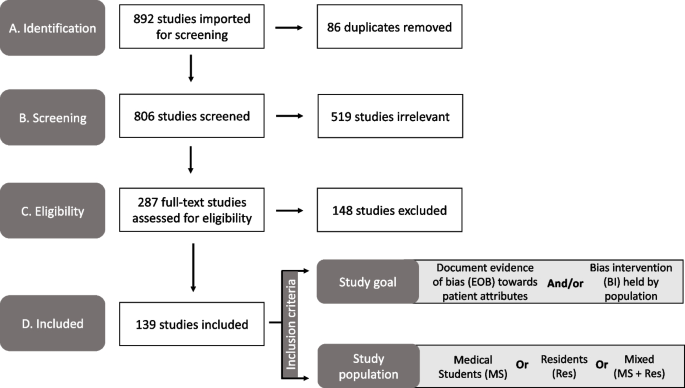
PRISMA diagram of the study selection process used in our scoping review to identify the bias categories that have been reported within medical education literature. Study took place from 2021–2022. Abbreviation: PRISMA, Preferred Reporting Items for Systematic Reviews and Meta-Analyses
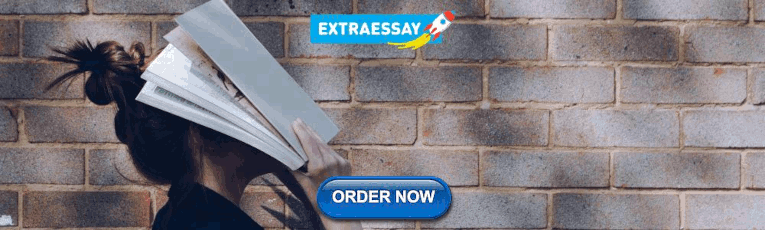
Publication trends in bias research
First, we charted the studies to demonstrate the timeline of research focused on bias within the study population of our interest (MS or Res or mixed). Our analysis revealed an increase in publications with respect to time (Fig. 2 ). Of the 139 included studies, fewer studies were published prior to 2001, with a total of only eight papers being published from the years 1985–2000. A substantial increase in publications occurred after 2004, with 2019 being the peak year where most of the studies pertaining to bias were published (Fig. 2 ).
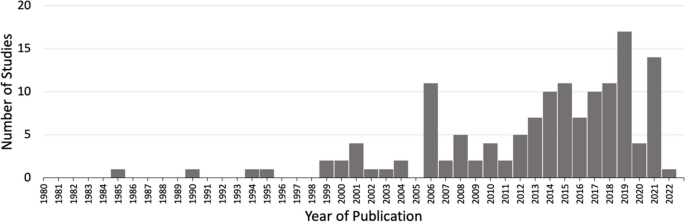
Studies matching inclusion criteria mapped by year of publication. Search criteria included studies addressing bias from 1980–2021 within medical students (MS) or residents (Res) or mixed (MS + Res) populations. * Publication in 2022 was published online ahead of print
Overview of included studies
We present a descriptive analysis of the 139 included studies in Table 1 based on the following parameters: study location, goal of the study, population of the study and the category of bias studied. All of the above parameters except the category of bias included a denominator of 139 studies. Several studies addressed more than one bias characteristic; therefore, we documented 163 biases sorted in 11 categories over the 139 papers. The bias categories that we generated and their respective occurrences are listed in Table 1 . Of the 139 studies that were included, most studies originated in the United States ( n = 89/139, 64%) and Europe ( n = 20/139, 20%).
Sorting of included research by bias category
We grouped the 139 included studies depending on the patient attribute or the descriptive characteristic against which the bias was studied (Table 1 ). By sorting the studies into different bias categories, we aimed to not only quantitate the amount of research addressing a particular topic of bias, but also reveal the biases that are understudied.
Through our analysis, we generated 11 descriptive categories against which bias was studied: Age, physical disability, education level, biological sex, disease or condition, LGBTQ + , non-specified, race/ethnicity, rural/urban, socio-economic status, and weight (Table 1 ). “Age” and “weight” categories included papers that studied bias against older population and higher weight individuals, respectively. The categories “education level” and “socio-economic status” included papers that studied bias against individuals with low education level and individuals belonging to low socioeconomic status, respectively. Within the bias category named ‘biological sex’, we included papers that studied bias against individuals perceived as women/females. Papers that studied bias against gender-identity or sexual orientation were included in its own category named, ‘LGBTQ + ’. The bias category, ‘disease or condition’ was broad and included research on bias against any patient with a specific disease, condition or lifestyle. Studies included in this category researched bias against any physical illnesses, mental illnesses, or sexually transmitted infections. It also included studies that addressed bias against a treatment such as transplant or pain management. It was not significant to report these as individual categories but rather as a whole with a common underlying theme. Rural/urban bias referred to bias that was held against a person based on their place of residence. Studies grouped together in the ‘non-specified bias’ category explored bias without specifying any descriptive characteristic in their methods. These studies did not address any specific bias characteristic in particular but consisted of a study population of our interest (MS or Res or mixed). Based on our analysis, the top five most studied bias categories in our included population within medical education literature were: racial or ethnic bias ( n = 39/163, 24%), disease or condition bias ( n = 29/163, 18%), weight bias ( n = 22/163, 13%), LGBTQ + bias ( n = 21/163, 13%), and age bias ( n = 16/163, 10%) which are presented in Table 1 .
Sorting of included research by population
In order to understand the distribution of bias research based on their populations examined, we sorted the included studies in one of the following: medical students (MS), residents (Res) or mixed (Table 1 ). The following distributions were observed: medical students only ( n = 105/139, 76%), residents only ( n = 19/139, 14%) or mixed which consisted of both medical students and residents ( n = 15/139, 11%). In combination, these results demonstrate that medical educators have focused bias research efforts primarily on medical student populations.
Sorting of included research by goal
A critical component of this scoping review was to quantify the research goal of the included studies within each of the bias categories. We defined the research goal as either to document evidence of bias (EOB) or to evaluate a bias intervention (BI) (see Fig. 1 for inclusion criteria). Some of the included studies focused on both, documenting evidence in addition to intervening biases and those studies were grouped separately. The analysis revealed that 69/139 (50%) of the included studies focused exclusively on documenting evidence of bias (EOB). There were fewer studies ( n = 51/139, 37%) which solely focused on bias interventions such as programs, seminars or curricular innovations. A small minority of the included studies were more comprehensive in that they documented EOB followed by an intervention strategy ( n = 19/139, 11%). These results demonstrate that most bias research is dedicated to documenting evidence of bias among these groups rather than evaluating a bias intervention strategy.
Research goal distribution
Our next objective was to calculate the distribution of studies with respect to the study goal (EOB, BI or both), within the 163 biases studied across the 139 papers as calculated in Table 1 . In general, the goal of the studies favors documenting evidence of bias with the exception of race/ethnic bias which is more focused on bias intervention (Fig. 3 ). Fewer studies were aimed at both, documenting evidence then providing an intervention, across all bias categories.
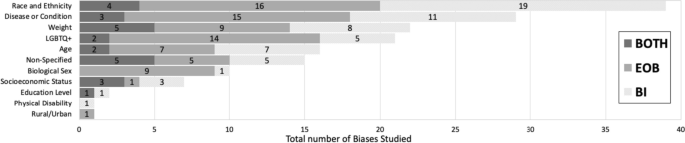
Sorting of total biases ( n = 163) within medical students or residents or a mixed population based on the bias category . Dark grey indicates studies with a dual goal, to document evidence of bias and to intervene bias. Medium grey bars indicate studies which focused on documenting evidence of bias. Light grey bars indicate studies focused on bias intervention within these populations. Numbers inside the bars indicate the total number of biases for the respective study goal. * Non-specified bias includes studies which focused on implicit bias but did not mention the type of bias investigated
Furthermore, we also calculated the ratio of EOB, BI and both (EOB + BI) within each of our population of interest (MS; n = 122, Res; n = 26 and mixed; n = 15) for the 163 biases observed in our included studies. Over half ( n = 64/122, 52%) of the total bias occurrences in MS were focused on documenting EOB (Fig. 4 ). Contrastingly, a shift was observed within resident populations where most biases addressed were aimed at intervention ( n = 12/26, 41%) rather than EOB ( n = 4/26, 14%) (Fig. 4 ). Studies which included both MS and Res (mixed) were primarily focused on documenting EOB ( n = 9/15, 60%), with 33% ( n = 5/15) aimed at bias intervention and 7% ( n = 1/15) which did both (Fig. 4 ). Although far fewer studies were documented in the Res population it is important to highlight that most of these studies were focused on bias intervention when compared to MS population where we documented a majority of studies focused on evidence of bias.
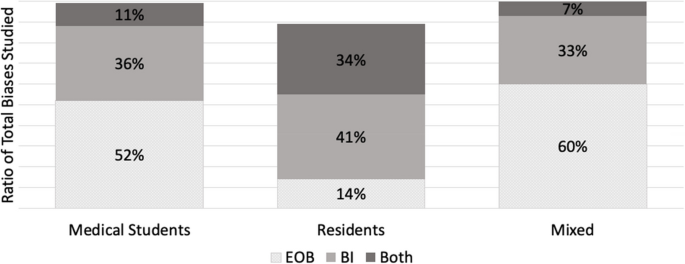
A ratio of the study goal for the total biases ( n = 163) mapped within each of the study population (MS, Res and Mixed). A study goal with a) documenting evidence of bias (EOB) is depicted in dotted grey, b) bias intervention (BI) in medium grey, and c) a dual focus (EOB + BI) is depicted in dark grey. * N = 122 for medical student studies. b N = 26 for residents. c N = 15 for mixed
Addressing biases at an earlier stage of medical career is critical for future physicians engaging with diverse patients, since it is established that bias negatively influences provider-patient interactions [ 171 ], clinical decision-making [ 172 ] and reduces favorable treatment outcomes [ 2 ]. We set out with an intention to explore how bias is addressed within the medical curriculum. Our research question was: how has the trend in bias research changed over time, more specifically a) what is the timeline of papers published? b) what bias characteristics have been studied in the physician-trainee population and c) how are these biases addressed? With the introduction of ‘standards of diversity’ by the Liaison Committee on Medical Education, along with the Association of American Medical Colleges (AAMC) and the American Medical Association (AMA) [ 173 , 174 ], we certainly expected and observed a sustained uptick in research pertaining to bias. As shown here, research addressing bias in the target population (MS and Res) is on the rise, however only 139 papers fit our inclusion criteria. Of these studies, nearly 90% have been published since 2005 after the “Unequal Treatment: Confronting Racial and Ethnic Disparities in Health Care” report was published in 2003 [ 7 ]. However, given the well documented effects of physician held bias, we anticipated significantly more number of studies focused on bias at the medical student or resident level.
A key component from this study was that we generated descriptive categories of biases. Sorting the biases into descriptive categories helps to identify a more targeted approach for a specific bias intervention, rather than to broadly intervene bias as a whole. In fact, our analysis found a number of publications (labeled “non-specified bias” in Table 1 ) which studied implicit bias without specifying the patient attribute or the characteristic that the bias was against. In total, we generated 11 descriptive categories of bias from our scoping review which are shown in Table 1 and Fig. 3 . Furthermore, our bias descriptors grouped similar kinds of biases within a single category. For example, the category, “disease or condition” included papers that studied bias against any type of disease (Mental illness, HIV stigma, diabetes), condition (Pain management), or lifestyle. We neither performed a qualitative assessment of the studies nor did we test the efficacy of the bias intervention studies and consider it a future direction of this work.
Evidence suggests that medical educators and healthcare professionals are struggling to find the appropriate approach to intervene biases [ 175 , 176 , 177 ] So far, bias reduction, bias reflection and bias management approaches have been proposed [ 26 , 27 , 178 ]. Previous implicit bias intervention strategies have been shown to be ineffective when biased attitudes of participants were assessed after a lag [ 179 ]. Understanding the descriptive categories of bias and previous existing research efforts, as we present here is only a fraction of the challenge. The theory of “cognitive bias” [ 180 ] and related branches of research [ 13 , 181 , 182 , 183 , 184 ] have been studied in the field of psychology for over three decades. It is only recently that cognitive bias theory has been applied to the field of medical education medicine, to explain its negative influence on clinical decision-making pertaining only to racial minorities [ 1 , 2 , 15 , 16 , 17 , 185 ]. In order to elicit meaningful changes with respect to targeted bias intervention, it is necessary to understand the psychological underpinnings (attitudes) leading to a certain descriptive category of bias (behaviors). The questions which medical educators need to ask are: a) Can these descriptive biases be identified under certain type/s of cognitive errors that elicits the bias and vice versa b) Are we working towards an attitude change which can elicit a sustained positive behavior change among healthcare professionals? And most importantly, c) are we creating a culture where participants voluntarily enroll themselves in bias interventions as opposed to being mandated to participate? Cognitive psychologists and behavioral scientists are well-positioned to help us find answers to these questions as they understand human behavior. Therefore, an interdisciplinary approach, a marriage between cognitive psychologists and medical educators, is key in targeting biases held by medical students, residents, and ultimately future physicians. This review may also be of interest to behavioral psychologists, keen on providing targeted intervening strategies to clinicians depending on the characteristics (age, weight, sex or race) the portrayed bias is against. Further, instead of an individualized approach, we need to strive for systemic changes and evidence-based strategies to intervene biases.
The next element in change is directing intervention strategies at the right stage in clinical education. Our study demonstrated that most of the research collected at the medical student level was focused on documenting evidence of bias. Although the overall number of studies at the resident level were fewer than at the medical student level, the ratio of research in favor of bias intervention was higher at the resident level (see Fig. 3 ). However, it could be helpful to focus on bias intervention earlier in learning, rather than at a later stage [ 186 ]. Additionally, educational resources such as textbooks, preparatory materials, and educators themselves are potential sources of propagating biases and therefore need constant evaluation against best practices [ 187 , 188 ].
This study has limitations. First, the list of the descriptive bias categories that we generated was not grounded in any particular theory so assigning a category was subjective. Additionally, there were studies that were categorized as “nonspecified” bias as the studies themselves did not mention the specific type of bias that they were addressing. Moreover, we had to exclude numerous publications solely because they were not evidence-based and were either perspectives, commentaries or opinion pieces. Finally, there were overall fewer studies focused on the resident population, so the calculated ratio of MS:Res studies did not compare similar sample sizes.
Future directions of our study include working with behavioral scientists to categorize these bias characteristics (Table 1 ) into cognitive error types [ 189 ]. Additionally, we aim to assess the effectiveness of the intervention strategies and categorize the approach of the intervention strategies.
The primary goal of our review was to organize, compare and quantify literature pertaining to bias within medical school curricula and residency programs. We neither performed a qualitative assessment of the studies nor did we test the efficacy of studies that were sorted into “bias intervention” as is typical of scoping reviews [ 22 ]. In summary, our research identified 11 descriptive categories of biases studied within medical students and resident populations with “race and ethnicity”, “disease or condition”, “weight”, “LGBTQ + ” and “age” being the top five most studied biases. Additionally, we found a greater number of studies conducted in medical students (105/139) when compared to residents (19/139). However, most of the studies in the resident population focused on bias intervention. The results from our review highlight the following gaps: a) bias categories where more research is needed, b) biases that are studied within medical school versus in residency programs and c) study focus in terms of demonstrating the presence of bias or working towards bias intervention.
This review provides a visual analysis of the known categories of bias addressed within the medical school curriculum and in residency programs in addition to providing a comparison of studies with respect to the study goal within medical education literature. The results from our review should be of interest to community organizations, institutions, program directors and medical educators interested in knowing and understanding the types of bias existing within healthcare populations. It might be of special interest to researchers who wish to explore other types of biases that have been understudied within medical school and resident populations, thus filling the gaps existing in bias research.
Despite the number of studies designed to provide bias intervention for MS and Res populations, and an overall cultural shift to be aware of one’s own biases, biases held by both medical students and residents still persist. Further, psychologists have recently demonstrated the ineffectiveness of some bias intervention efforts [ 179 , 190 ]. Therefore, it is perhaps unrealistic to expect these biases to be eliminated altogether. However, effective intervention strategies grounded in cognitive psychology should be implemented earlier on in medical training. Our focus should be on providing evidence-based approaches and safe spaces for an attitude and culture change, so as to induce actionable behavioral changes.
Availability of data and materials
The datasets used and/or analyzed during the current study are available from the corresponding author upon reasonable request.
Abbreviations
- Medical student
Evidence of bias
- Bias intervention
Hagiwara N, Mezuk B, Elston Lafata J, Vrana SR, Fetters MD. Study protocol for investigating physician communication behaviours that link physician implicit racial bias and patient outcomes in Black patients with type 2 diabetes using an exploratory sequential mixed methods design. BMJ Open. 2018;8(10):e022623.
Article Google Scholar
Haider AH, Schneider EB, Sriram N, Dossick DS, Scott VK, Swoboda SM, Losonczy L, Haut ER, Efron DT, Pronovost PJ, et al. Unconscious race and social class bias among acute care surgical clinicians and clinical treatment decisions. JAMA Surg. 2015;150(5):457–64.
Penner LA, Dovidio JF, Gonzalez R, Albrecht TL, Chapman R, Foster T, Harper FW, Hagiwara N, Hamel LM, Shields AF, et al. The effects of oncologist implicit racial bias in racially discordant oncology interactions. J Clin Oncol. 2016;34(24):2874–80.
Phelan SM, Burgess DJ, Yeazel MW, Hellerstedt WL, Griffin JM, van Ryn M. Impact of weight bias and stigma on quality of care and outcomes for patients with obesity. Obes Rev. 2015;16(4):319–26.
Garrett SB, Jones L, Montague A, Fa-Yusuf H, Harris-Taylor J, Powell B, Chan E, Zamarripa S, Hooper S, Chambers Butcher BD. Challenges and opportunities for clinician implicit bias training: insights from perinatal care stakeholders. Health Equity. 2023;7(1):506–19.
Shah HS, Bohlen J. Implicit bias. In: StatPearls. Treasure Island (FL): StatPearls Publishing; 2023. Copyright © 2023, StatPearls Publishing LLC.
Google Scholar
Institute of Medicine (US) Committee on Understanding and Eliminating Racial and Ethnic Disparities in Health Care. Unequal Treatment: Confronting Racial and Ethnic Disparities in Health Care. In: Smedley BD, Stith AY, Nelson AR, editors. Washington (DC): National Academies Press (US); 2003. PMID: 25032386.
Dehon E, Weiss N, Jones J, Faulconer W, Hinton E, Sterling S. A systematic review of the impact of physician implicit racial bias on clinical decision making. Acad Emerg Med. 2017;24(8):895–904.
Oliver MN, Wells KM, Joy-Gaba JA, Hawkins CB, Nosek BA. Do physicians’ implicit views of African Americans affect clinical decision making? J Am Board Fam Med. 2014;27(2):177–88.
Rincon-Subtirelu M. Education as a tool to modify anti-obesity bias among pediatric residents. Int J Med Educ. 2017;8:77–8.
Gustafsson Sendén M, Renström EA. Gender bias in assessment of future work ability among pain patients - an experimental vignette study of medical students’ assessment. Scand J Pain. 2019;19(2):407–14.
Hardeman RR, Burgess D, Phelan S, Yeazel M, Nelson D, van Ryn M. Medical student socio-demographic characteristics and attitudes toward patient centered care: do race, socioeconomic status and gender matter? A report from the medical student CHANGES study. Patient Educ Couns. 2015;98(3):350–5.
Greenwald AG, Banaji MR. Implicit social cognition: attitudes, self-esteem, and stereotypes. Psychol Rev. 1995;102(1):4–27.
Kruse JA, Collins JL, Vugrin M. Educational strategies used to improve the knowledge, skills, and attitudes of health care students and providers regarding implicit bias: an integrative review of the literature. Int J Nurs Stud Adv. 2022;4:100073.
Zestcott CA, Blair IV, Stone J. Examining the presence, consequences, and reduction of implicit bias in health care: a narrative review. Group Process Intergroup Relat. 2016;19(4):528–42.
Hall WJ, Chapman MV, Lee KM, Merino YM, Thomas TW, Payne BK, Eng E, Day SH, Coyne-Beasley T. Implicit racial/ethnic bias among health care professionals and its influence on health care outcomes: a systematic review. Am J Public Health. 2015;105(12):E60–76.
FitzGerald C, Hurst S. Implicit bias in healthcare professionals: a systematic review. BMC Med Ethics. 2017;18(1):19.
Gonzalez CM, Onumah CM, Walker SA, Karp E, Schwartz R, Lypson ML. Implicit bias instruction across disciplines related to the social determinants of health: a scoping review. Adv Health Sci Educ. 2023;28(2):541–87.
Pham MT, Rajić A, Greig JD, Sargeant JM, Papadopoulos A, McEwen SA. A scoping review of scoping reviews: advancing the approach and enhancing the consistency. Res Synth Methods. 2014;5(4):371–85.
Levac D, Colquhoun H, O’Brien KK. Scoping studies: advancing the methodology. Implement Sci. 2010;5:69.
Pat C, Geeta S, Sílvia M. Cognitive debiasing 1: origins of bias and theory of debiasing. BMJ Qual Saf. 2013;22(Suppl 2):ii58.
Arksey H, O’Malley L. Scoping studies: towards a methodological framework. Int J Soc Res Methodol. 2005;8(1):19–32.
Thomas A, Lubarsky S, Durning SJ, Young ME. Knowledge syntheses in medical education: demystifying scoping reviews. Acad Med. 2017;92(2):161–6.
Hagopian A, Thompson MJ, Fordyce M, Johnson KE, Hart LG. The migration of physicians from sub-Saharan Africa to the United States of America: measures of the African brain drain. Hum Resour Health. 2004;2(1):17.
Tricco AC, Lillie E, Zarin W, O’Brien KK, Colquhoun H, Levac D, Moher D, Peters MDJ, Horsley T, Weeks L, et al. PRISMA Extension for Scoping Reviews (PRISMA-ScR): checklist and explanation. Ann Intern Med. 2018;169(7):467–73.
Teal CR, Shada RE, Gill AC, Thompson BM, Frugé E, Villarreal GB, Haidet P. When best intentions aren’t enough: Helping medical students develop strategies for managing bias about patients. J Gen Intern Med. 2010;25(Suppl 2):S115–8.
Gonzalez CM, Walker SA, Rodriguez N, Noah YS, Marantz PR. Implicit bias recognition and management in interpersonal encounters and the learning environment: a skills-based curriculum for medical students. MedEdPORTAL. 2021;17:11168.
Hoffman KM, Trawalter S, Axt JR, Oliver MN. Racial bias in pain assessment and treatment recommendations, and false beliefs about biological differences between blacks and whites. Proc Natl Acad Sci U S A. 2016;113(16):4296–301.
Mayfield JJ, Ball EM, Tillery KA, Crandall C, Dexter J, Winer JM, Bosshardt ZM, Welch JH, Dolan E, Fancovic ER, et al. Beyond men, women, or both: a comprehensive, LGBTQ-inclusive, implicit-bias-aware, standardized-patient-based sexual history taking curriculum. MedEdPORTAL. 2017;13:10634.
Morris M, Cooper RL, Ramesh A, Tabatabai M, Arcury TA, Shinn M, Im W, Juarez P, Matthews-Juarez P. Training to reduce LGBTQ-related bias among medical, nursing, and dental students and providers: a systematic review. BMC Med Educ. 2019;19(1):325.
Perdomo J, Tolliver D, Hsu H, He Y, Nash KA, Donatelli S, Mateo C, Akagbosu C, Alizadeh F, Power-Hays A, et al. Health equity rounds: an interdisciplinary case conference to address implicit bias and structural racism for faculty and trainees. MedEdPORTAL. 2019;15:10858.
Sherman MD, Ricco J, Nelson SC, Nezhad SJ, Prasad S. Implicit bias training in a residency program: aiming for enduring effects. Fam Med. 2019;51(8):677–81.
van Ryn M, Hardeman R, Phelan SM, Burgess DJ, Dovidio JF, Herrin J, Burke SE, Nelson DB, Perry S, Yeazel M, et al. Medical school experiences associated with change in implicit racial bias among 3547 students: a medical student CHANGES study report. J Gen Intern Med. 2015;30(12):1748–56.
Chary AN, Molina MF, Dadabhoy FZ, Manchanda EC. Addressing racism in medicine through a resident-led health equity retreat. West J Emerg Med. 2020;22(1):41–4.
DallaPiazza M, Padilla-Register M, Dwarakanath M, Obamedo E, Hill J, Soto-Greene ML. Exploring racism and health: an intensive interactive session for medical students. MedEdPORTAL. 2018;14:10783.
Dennis SN, Gold RS, Wen FK. Learner reactions to activities exploring racism as a social determinant of health. Fam Med. 2019;51(1):41–7.
Gonzalez CM, Walker SA, Rodriguez N, Karp E, Marantz PR. It can be done! a skills-based elective in implicit bias recognition and management for preclinical medical students. Acad Med. 2020;95(12S Addressing Harmful Bias and Eliminating Discrimination in Health Professions Learning Environments):S150–5.
Motzkus C, Wells RJ, Wang X, Chimienti S, Plummer D, Sabin J, Allison J, Cashman S. Pre-clinical medical student reflections on implicit bias: Implications for learning and teaching. PLoS ONE. 2019;14(11):e0225058.
Phelan SM, Burke SE, Cunningham BA, Perry SP, Hardeman RR, Dovidio JF, Herrin J, Dyrbye LN, White RO, Yeazel MW, et al. The effects of racism in medical education on students’ decisions to practice in underserved or minority communities. Acad Med. 2019;94(8):1178–89.
Zeidan A, Tiballi A, Woodward M, Di Bartolo IM. Targeting implicit bias in medicine: lessons from art and archaeology. West J Emerg Med. 2019;21(1):1–3.
Baker TK, Smith GS, Jacobs NN, Houmanfar R, Tolles R, Kuhls D, Piasecki M. A deeper look at implicit weight bias in medical students. Adv Health Sci Educ Theory Pract. 2017;22(4):889–900.
Eymard AS, Douglas DH. Ageism among health care providers and interventions to improve their attitudes toward older adults: an integrative review. J Gerontol Nurs. 2012;38(5):26–35.
Garrison CB, McKinney-Whitson V, Johnston B, Munroe A. Race matters: addressing racism as a health issue. Int J Psychiatry Med. 2018;53(5–6):436–44.
Geller G, Watkins PA. Addressing medical students’ negative bias toward patients with obesity through ethics education. AMA J Ethics. 2018;20(10):E948-959.
Onyeador IN, Wittlin NM, Burke SE, Dovidio JF, Perry SP, Hardeman RR, Dyrbye LN, Herrin J, Phelan SM, van Ryn M. The value of interracial contact for reducing anti-black bias among non-black physicians: a Cognitive Habits and Growth Evaluation (CHANGE) study report. Psychol Sci. 2020;31(1):18–30.
Poustchi Y, Saks NS, Piasecki AK, Hahn KA, Ferrante JM. Brief intervention effective in reducing weight bias in medical students. Fam Med. 2013;45(5):345–8.
Ruiz JG, Andrade AD, Anam R, Taldone S, Karanam C, Hogue C, Mintzer MJ. Group-based differences in anti-aging bias among medical students. Gerontol Geriatr Educ. 2015;36(1):58–78.
Simpson T, Evans J, Goepfert A, Elopre L. Implementing a graduate medical education anti-racism workshop at an academic university in the Southern USA. Med Educ Online. 2022;27(1):1981803.
Wittlin NM, Dovidio JF, Burke SE, Przedworski JM, Herrin J, Dyrbye L, Onyeador IN, Phelan SM, van Ryn M. Contact and role modeling predict bias against lesbian and gay individuals among early-career physicians: a longitudinal study. Soc Sci Med. 2019;238:112422.
Miller DP Jr, Spangler JG, Vitolins MZ, Davis SW, Ip EH, Marion GS, Crandall SJ. Are medical students aware of their anti-obesity bias? Acad Med. 2013;88(7):978–82.
Gonzalez CM, Deno ML, Kintzer E, Marantz PR, Lypson ML, McKee MD. A qualitative study of New York medical student views on implicit bias instruction: implications for curriculum development. J Gen Intern Med. 2019;34(5):692–8.
Gonzalez CM, Kim MY, Marantz PR. Implicit bias and its relation to health disparities: a teaching program and survey of medical students. Teach Learn Med. 2014;26(1):64–71.
Gonzalez CM, Nava S, List J, Liguori A, Marantz PR. How assumptions and preferences can affect patient care: an introduction to implicit bias for first-year medical students. MedEdPORTAL. 2021;17:11162.
Hernandez RA, Haidet P, Gill AC, Teal CR. Fostering students’ reflection about bias in healthcare: cognitive dissonance and the role of personal and normative standards. Med Teach. 2013;35(4):e1082-1089.
Kushner RF, Zeiss DM, Feinglass JM, Yelen M. An obesity educational intervention for medical students addressing weight bias and communication skills using standardized patients. BMC Med Educ. 2014;14:53.
Nazione S, Silk KJ. Patient race and perceived illness responsibility: effects on provider helping and bias. Med Educ. 2013;47(8):780–9.
Ogunyemi D. Defeating unconscious bias: the role of a structured, reflective, and interactive workshop. J Grad Med Educ. 2021;13(2):189–94.
Phelan SM, Burke SE, Hardeman RR, White RO, Przedworski J, Dovidio JF, Perry SP, Plankey M, A Cunningham B, Finstad D, et al. Medical school factors associated with changes in implicit and explicit bias against gay and lesbian people among 3492 graduating medical students. J Gen Intern Med. 2017;32(11):1193–201.
Phelan SM, Puhl RM, Burke SE, Hardeman R, Dovidio JF, Nelson DB, Przedworski J, Burgess DJ, Perry S, Yeazel MW, et al. The mixed impact of medical school on medical students’ implicit and explicit weight bias. Med Educ. 2015;49(10):983–92.
Barber Doucet H, Ward VL, Johnson TJ, Lee LK. Implicit bias and caring for diverse populations: pediatric trainee attitudes and gaps in training. Clin Pediatr (Phila). 2021;60(9–10):408–17.
Burke SE, Dovidio JF, Przedworski JM, Hardeman RR, Perry SP, Phelan SM, Nelson DB, Burgess DJ, Yeazel MW, van Ryn M. Do contact and empathy mitigate bias against gay and lesbian people among heterosexual first-year medical students? A report from the medical student CHANGE study. Acad Med. 2015;90(5):645–51.
Johnston B, McKinney-Whitson V, Garrison V. Race matters: addressing racism as a health issue. WMJ. 2021;120(S1):S74–7.
Kost A, Akande T, Jones R, Gabert R, Isaac M, Dettmar NS. Use of patient identifiers at the University of Washington School of Medicine: building institutional consensus to reduce bias and stigma. Fam Med. 2021;53(5):366–71.
Madan AK, Aliabadi-Wahle S, Beech DJ. Ageism in medical students’ treatment recommendations: the example of breast-conserving procedures. Acad Med. 2001;76(3):282–4.
Marbin J, Lewis L, Kuo AK, Schudel C, Gutierrez JR. The power of place: travel to explore structural racism and health disparities. Acad Med. 2021;96(11):1569–73.
Phelan SM, Dovidio JF, Puhl RM, Burgess DJ, Nelson DB, Yeazel MW, Hardeman R, Perry S, van Ryn M. Implicit and explicit weight bias in a national sample of 4,732 medical students: the medical student CHANGES study. Obesity (Silver Spring). 2014;22(4):1201–8.
Van J, Aloman C, Reau N. Potential bias and misconceptions in liver transplantation for alcohol- and obesity-related liver disease. Am J Gastroenterol. 2021;116(10):2089–97.
White-Means S, Zhiyong D, Hufstader M, Brown LT. Cultural competency, race, and skin tone bias among pharmacy, nursing, and medical students: implications for addressing health disparities. Med Care Res Rev. 2009;66(4):436–55.
Williams RL, Vasquez CE, Getrich CM, Kano M, Boursaw B, Krabbenhoft C, Sussman AL. Racial/gender biases in student clinical decision-making: a mixed-method study of medical school attributes associated with lower incidence of biases. J Gen Intern Med. 2018;33(12):2056–64.
Cohen RW, Persky S. Influence of weight etiology information and trainee characteristics on physician-trainees’ clinical and interpersonal communication. Patient Educ Couns. 2019;102(9):1644–9.
Haider AH, Sexton J, Sriram N, Cooper LA, Efron DT, Swoboda S, Villegas CV, Haut ER, Bonds M, Pronovost PJ, et al. Association of unconscious race and social class bias with vignette-based clinical assessments by medical students. JAMA. 2011;306(9):942–51.
Lewis R, Lamdan RM, Wald D, Curtis M. Gender bias in the diagnosis of a geriatric standardized patient: a potential confounding variable. Acad Psychiatry. 2006;30(5):392–6.
Matharu K, Shapiro JF, Hammer RR, Kravitz RL, Wilson MD, Fitzgerald FT. Reducing obesity prejudice in medical education. Educ Health. 2014;27(3):231–7.
McLean ME, McLean LE, McLean-Holden AC, Campbell LF, Horner AM, Kulkarni ML, Melville LD, Fernandez EA. Interphysician weight bias: a cross-sectional observational survey study to guide implicit bias training in the medical workplace. Acad Emerg Med. 2021;28(9):1024–34.
Meadows A, Higgs S, Burke SE, Dovidio JF, van Ryn M, Phelan SM. Social dominance orientation, dispositional empathy, and need for cognitive closure moderate the impact of empathy-skills training, but not patient contact, on medical students’ negative attitudes toward higher-weight patients. Front Psychol. 2017;8:15.
Stone J, Moskowitz GB, Zestcott CA, Wolsiefer KJ. Testing active learning workshops for reducing implicit stereotyping of Hispanics by majority and minority group medical students. Stigma Health. 2020;5(1):94–103.
Symons AB, Morley CP, McGuigan D, Akl EA. A curriculum on care for people with disabilities: effects on medical student self-reported attitudes and comfort level. Disabil Health J. 2014;7(1):88–95.
Ufomata E, Eckstrand KL, Hasley P, Jeong K, Rubio D, Spagnoletti C. Comprehensive internal medicine residency curriculum on primary care of patients who identify as LGBT. LGBT Health. 2018;5(6):375–80.
Aultman JM, Borges NJ. A clinical and ethical investigation of pre-medical and medical students’ attitudes, knowledge, and understanding of HIV. Med Educ Online. 2006;11:1–12.
Bates T, Cohan M, Bragg DS, Bedinghaus J. The Medical College of Wisconsin senior mentor program: experience of a lifetime. Gerontol Geriatr Educ. 2006;27(2):93–103.
Chiaramonte GR, Friend R. Medical students’ and residents’ gender bias in the diagnosis, treatment, and interpretation of coronary heart disease symptoms. Health Psychol. 2006;25(3):255–66.
Friedberg F, Sohl SJ, Halperin PJ. Teaching medical students about medically unexplained illnesses: a preliminary study. Med Teach. 2008;30(6):618–21.
Gonzales E, Morrow-Howell N, Gilbert P. Changing medical students’ attitudes toward older adults. Gerontol Geriatr Educ. 2010;31(3):220–34.
Hinners CK, Potter JF. A partnership between the University of Nebraska College of Medicine and the community: fostering positive attitudes towards the aged. Gerontol Geriatr Educ. 2006;27(2):83–91.
Lee M, Coulehan JL. Medical students’ perceptions of racial diversity and gender equality. Med Educ. 2006;40(7):691–6.
Schmetzer AD, Lafuze JE. Overcoming stigma: involving families in medical student and psychiatric residency education. Acad Psychiatry. 2008;32(2):127–31.
Willen SS, Bullon A, Good MJD. Opening up a huge can of worms: reflections on a “cultural sensitivity” course for psychiatry residents. Harv Rev Psychiatry. 2010;18(4):247–53.
Dogra N, Karnik N. First-year medical students’ attitudes toward diversity and its teaching: an investigation at one U.S. medical school. Acad Med. 2003;78(11):1191–200.
Fitzpatrick C, Musser A, Mosqueda L, Boker J, Prislin M. Student senior partnership program: University of California Irvine School of Medicine. Gerontol Geriatr Educ. 2006;27(2):25–35.
Hoffman KG, Gray P, Hosokawa MC, Zweig SC. Evaluating the effectiveness of a senior mentor program: the University of Missouri-Columbia School of Medicine. Gerontol Geriatr Educ. 2006;27(2):37–47.
Kantor BS, Myers MR. From aging…to saging-the Ohio State Senior Partners Program: longitudinal and experiential geriatrics education. Gerontol Geriatr Educ. 2006;27(2):69–74.
Klamen DL, Grossman LS, Kopacz DR. Medical student homophobia. J Homosex. 1999;37(1):53–63.
Kopacz DR, Grossman LS, Klamen DL. Medical students and AIDS: knowledge, attitudes and implications for education. Health Educ Res. 1999;14(1):1–6.
Leiblum SR. An established medical school human sexuality curriculum: description and evaluation. Sex Relatsh Ther. 2001;16(1):59–70.
Rastegar DA, Fingerhood MI, Jasinski DR. A resident clerkship that combines inpatient and outpatient training in substance abuse and HIV care. Subst Abuse. 2004;25(4):11–5.
Roberts E, Richeson NA, Thornhill JTIV, Corwin SJ, Eleazer GP. The senior mentor program at the University of South Carolina School of Medicine: an innovative geriatric longitudinal curriculum. Gerontol Geriatr Educ. 2006;27(2):11–23.
Burgess DJ, Burke SE, Cunningham BA, Dovidio JF, Hardeman RR, Hou YF, Nelson DB, Perry SP, Phelan SM, Yeazel MW, et al. Medical students’ learning orientation regarding interracial interactions affects preparedness to care for minority patients: a report from medical student CHANGES. BMC Med Educ. 2016;16:254.
Burgess DJ, Hardeman RR, Burke SE, Cunningham BA, Dovidio JF, Nelson DB, Perry SP, Phelan SM, Yeazel MW, Herrin J, et al. Incoming medical students’ political orientation affects outcomes related to care of marginalized groups: results from the medical student CHANGES study. J Health Pol Policy Law. 2019;44(1):113–46.
Kurtz ME, Johnson SM, Tomlinson T, Fiel NJ. Teaching medical students the effects of values and stereotyping on the doctor/patient relationship. Soc Sci Med. 1985;21(9):1043–7.
Matharu K, Kravitz RL, McMahon GT, Wilson MD, Fitzgerald FT. Medical students’ attitudes toward gay men. BMC Med Educ. 2012;12:71.
Pearl RL, Argueso D, Wadden TA. Effects of medical trainees’ weight-loss history on perceptions of patients with obesity. Med Educ. 2017;51(8):802–11.
Perry SP, Dovidio JF, Murphy MC, van Ryn M. The joint effect of bias awareness and self-reported prejudice on intergroup anxiety and intentions for intergroup contact. Cultur Divers Ethnic Minor Psychol. 2015;21(1):89–96.
Phelan SM, Burgess DJ, Burke SE, Przedworski JM, Dovidio JF, Hardeman R, Morris M, van Ryn M. Beliefs about the causes of obesity in a national sample of 4th year medical students. Patient Educ Couns. 2015;98(11):1446–9.
Phelan SM, Puhl RM, Burgess DJ, Natt N, Mundi M, Miller NE, Saha S, Fischer K, van Ryn M. The role of weight bias and role-modeling in medical students’ patient-centered communication with higher weight standardized patients. Patient Educ Couns. 2021;104(8):1962–9.
Polan HJ, Auerbach MI, Viederman M. AIDS as a paradigm of human behavior in disease: impact and implications of a course. Acad Psychiatry. 1990;14(4):197–203.
Reuben DB, Fullerton JT, Tschann JM, Croughan-Minihane M. Attitudes of beginning medical students toward older persons: a five-campus study. J Am Geriatr Soc. 1995;43(12):1430–6.
Tsai J. Building structural empathy to marshal critical education into compassionate practice: evaluation of a medical school critical race theory course. J Law Med Ethics. 2021;49(2):211–21.
Weyant RJ, Bennett ME, Simon M, Palaisa J. Desire to treat HIV-infected patients: similarities and differences across health-care professions. AIDS. 1994;8(1):117–21.
Ross PT, Lypson ML. Using artistic-narrative to stimulate reflection on physician bias. Teach Learn Med. 2014;26(4):344–9.
Calabrese SK, Earnshaw VA, Krakower DS, Underhill K, Vincent W, Magnus M, Hansen NB, Kershaw TS, Mayer KH, Betancourt JR, et al. A closer look at racism and heterosexism in medical students’ clinical decision-making related to HIV Pre-Exposure Prophylaxis (PrEP): implications for PrEP education. AIDS Behav. 2018;22(4):1122–38.
Fitterman-Harris HF, Vander Wal JS. Weight bias reduction among first-year medical students: a quasi-randomized, controlled trial. Clin Obes. 2021;11(6):e12479.
Madan AK, Cooper L, Gratzer A, Beech DJ. Ageism in breast cancer surgical options by medical students. Tenn Med. 2006;99(5):37–8, 41.
Bikmukhametov DA, Anokhin VA, Vinogradova AN, Triner WR, McNutt LA. Bias in medicine: a survey of medical student attitudes towards HIV-positive and marginalized patients in Russia, 2010. J Int AIDS Soc. 2012;15(2):17372.
Dijkstra AF, Verdonk P, Lagro-Janssen AL. Gender bias in medical textbooks: examples from coronary heart disease, depression, alcohol abuse and pharmacology. Med Educ. 2008;42(10):1021–8.
Dobrowolska B, Jędrzejkiewicz B, Pilewska-Kozak A, Zarzycka D, Ślusarska B, Deluga A, Kościołek A, Palese A. Age discrimination in healthcare institutions perceived by seniors and students. Nurs Ethics. 2019;26(2):443–59.
Hamberg K, Risberg G, Johansson EE, Westman G. Gender bias in physicians’ management of neck pain: a study of the answers in a Swedish national examination. J Womens Health Gend Based Med. 2002;11(7):653–66.
Magliano L, Read J, Sagliocchi A, Oliviero N, D’Ambrosio A, Campitiello F, Zaccaro A, Guizzaro L, Patalano M. “Social dangerousness and incurability in schizophrenia”: results of an educational intervention for medical and psychology students. Psychiatry Res. 2014;219(3):457–63.
Reis SP, Wald HS. Contemplating medicine during the Third Reich: scaffolding professional identity formation for medical students. Acad Med. 2015;90(6):770–3.
Schroyen S, Adam S, Marquet M, Jerusalem G, Thiel S, Giraudet AL, Missotten P. Communication of healthcare professionals: Is there ageism? Eur J Cancer Care (Engl). 2018;27(1):e12780.
Swift JA, Hanlon S, El-Redy L, Puhl RM, Glazebrook C. Weight bias among UK trainee dietitians, doctors, nurses and nutritionists. J Hum Nutr Diet. 2013;26(4):395–402.
Swift JA, Tischler V, Markham S, Gunning I, Glazebrook C, Beer C, Puhl R. Are anti-stigma films a useful strategy for reducing weight bias among trainee healthcare professionals? Results of a pilot randomized control trial. Obes Facts. 2013;6(1):91–102.
Yertutanol FDK, Candansayar S, Seydaoğlu G. Homophobia in health professionals in Ankara, Turkey: developing a scale. Transcult Psychiatry. 2019;56(6):1191–217.
Arnold O, Voracek M, Musalek M, Springer-Kremser M. Austrian medical students’ attitudes towards male and female homosexuality: a comparative survey. Wien Klin Wochenschr. 2004;116(21–22):730–6.
Arvaniti A, Samakouri M, Kalamara E, Bochtsou V, Bikos C, Livaditis M. Health service staff’s attitudes towards patients with mental illness. Soc Psychiatry Psychiatr Epidemiol. 2009;44(8):658–65.
Lopes L, Gato J, Esteves M. Portuguese medical students’ knowledge and attitudes towards homosexuality. Acta Med Port. 2016;29(11):684–93.
Papadaki V, Plotnikof K, Gioumidou M, Zisimou V, Papadaki E. A comparison of attitudes toward lesbians and gay men among students of helping professions in Crete, Greece: the cases of social work, psychology, medicine, and nursing. J Homosex. 2015;62(6):735–62.
Papaharitou S, Nakopoulou E, Moraitou M, Tsimtsiou Z, Konstantinidou E, Hatzichristou D. Exploring sexual attitudes of students in health professions. J Sex Med. 2008;5(6):1308–16.
Roberts JH, Sanders T, Mann K, Wass V. Institutional marginalisation and student resistance: barriers to learning about culture, race and ethnicity. Adv Health Sci Educ. 2010;15(4):559–71.
Wilhelmi L, Ingendae F, Steinhaeuser J. What leads to the subjective perception of a ‘rural area’? A qualitative study with undergraduate students and postgraduate trainees in Germany to tailor strategies against physician’s shortage. Rural Remote Health. 2018;18(4):4694.
Herrmann-Werner A, Loda T, Wiesner LM, Erschens RS, Junne F, Zipfel S. Is an obesity simulation suit in an undergraduate medical communication class a valuable teaching tool? A cross-sectional proof of concept study. BMJ Open. 2019;9(8):e029738.
Ahadinezhad B, Khosravizadeh O, Maleki A, Hashtroodi A. Implicit racial bias among medical graduates and students by an IAT measure: a systematic review and meta-analysis. Ir J Med Sci. 2022;191(4):1941–9. https://doi.org/10.1007/s11845-021-02756-3 .
Hsieh JG, Hsu M, Wang YW. An anthropological approach to teach and evaluate cultural competence in medical students - the application of mini-ethnography in medical history taking. Med Educ Online. 2016;21:32561.
Poreddi V, Thimmaiah R, Math SB. Attitudes toward people with mental illness among medical students. J Neurosci Rural Pract. 2015;6(3):349–54.
Mino Y, Yasuda N, Tsuda T, Shimodera S. Effects of a one-hour educational program on medical students’ attitudes to mental illness. Psychiatry Clin Neurosci. 2001;55(5):501–7.
Omori A, Tateno A, Ideno T, Takahashi H, Kawashima Y, Takemura K, Okubo Y. Influence of contact with schizophrenia on implicit attitudes towards schizophrenia patients held by clinical residents. BMC Psychiatry. 2012;12:8.
Banwari G, Mistry K, Soni A, Parikh N, Gandhi H. Medical students and interns’ knowledge about and attitude towards homosexuality. J Postgrad Med. 2015;61(2):95–100.
Lee SY. Obesity education in medical school curricula in Korea. J Obes Metab Syndr. 2018;27(1):35–8.
Aruna G, Mittal S, Yadiyal MB, Acharya C, Acharya S, Uppulari C. Perception, knowledge, and attitude toward mental disorders and psychiatry among medical undergraduates in Karnataka: a cross-sectional study. Indian J Psychiatry. 2016;58(1):70–6.
Wong YL. Review paper: gender competencies in the medical curriculum: addressing gender bias in medicine. Asia Pac J Public Health. 2009;21(4):359–76.
Earnshaw VA, Jin H, Wickersham JA, Kamarulzaman A, John J, Lim SH, Altice FL. Stigma toward men who have sex with men among future healthcare providers in Malaysia: would more interpersonal contact reduce prejudice? AIDS Behav. 2016;20(1):98–106.
Larson B, Herx L, Williamson T, Crowshoe L. Beyond the barriers: family medicine residents’ attitudes towards providing Aboriginal health care. Med Educ. 2011;45(4):400–6.
Wagner AC, Girard T, McShane KE, Margolese S, Hart TA. HIV-related stigma and overlapping stigmas towards people living with HIV among health care trainees in Canada. AIDS Educ Prev. 2017;29(4):364–76.
Tellier P-P, Bélanger E, Rodríguez C, Ware MA, Posel N. Improving undergraduate medical education about pain assessment and management: a qualitative descriptive study of stakeholders’ perceptions. Pain Res Manage. 2013;18(5):259–65.
Loignon C, Boudreault-Fournier A, Truchon K, Labrousse Y, Fortin B. Medical residents reflect on their prejudices toward poverty: a photovoice training project. BMC Med Educ. 2014;14:1050.
Phillips SP, Clarke M. More than an education: the hidden curriculum, professional attitudes and career choice. Med Educ. 2012;46(9):887–93.
Jaworsky D, Gardner S, Thorne JG, Sharma M, McNaughton N, Paddock S, Chew D, Lees R, Makuwaza T, Wagner A, et al. The role of people living with HIV as patient instructors—Reducing stigma and improving interest around HIV care among medical students. AIDS Care. 2017;29(4):524–31.
Sukhera J, Wodzinski M, Teunissen PW, Lingard L, Watling C. Striving while accepting: exploring the relationship between identity and implicit bias recognition and management. Acad Med. 2018;93(11S Association of American Medical Colleges Learn Serve Lead: Proceedings of the 57th Annual Research in Medical Education Sessions):S82-s88.
Harris R, Cormack D, Curtis E, Jones R, Stanley J, Lacey C. Development and testing of study tools and methods to examine ethnic bias and clinical decision-making among medical students in New Zealand: the Bias and Decision-Making in Medicine (BDMM) study. BMC Med Educ. 2016;16:173.
Cormack D, Harris R, Stanley J, Lacey C, Jones R, Curtis E. Ethnic bias amongst medical students in Aotearoa/New Zealand: findings from the Bias and Decision Making in Medicine (BDMM) study. PLoS ONE. 2018;13(8):e0201168.
Harris R, Cormack D, Stanley J, Curtis E, Jones R, Lacey C. Ethnic bias and clinical decision-making among New Zealand medical students: an observational study. BMC Med Educ. 2018;18(1):18.
Robinson EL, Ball LE, Leveritt MD. Obesity bias among health and non-health students attending an Australian university and their perceived obesity education. J Nutr Educ Behav. 2014;46(5):390–5.
Sopoaga F, Zaharic T, Kokaua J, Covello S. Training a medical workforce to meet the needs of diverse minority communities. BMC Med Educ. 2017;17:19.
Parker R, Larkin T, Cockburn J. A visual analysis of gender bias in contemporary anatomy textbooks. Soc Sci Med. 2017;180:106–13.
Gomes MdM. Doctors’ perspectives and practices regarding epilepsy. Arq Neuropsiquiatr. 2000;58(2):221–6.
Caixeta J, Fernandes PT, Bell GS, Sander JW, Li LM. Epilepsy perception amongst university students - A survey. Arq Neuropsiquiatr. 2007;65:43–8.
Tedrus GMAS, Fonseca LC, da Câmara Vieira AL. Knowledge and attitudes toward epilepsy amongst students in the health area: intervention aimed at enlightenment. Arq Neuropsiquiatr. 2007;65(4-B):1181–5.
Gomez-Moreno C, Verduzco-Aguirre H, Contreras-Garduño S, Perez-de-Acha A, Alcalde-Castro J, Chavarri-Guerra Y, García-Lara JMA, Navarrete-Reyes AP, Avila-Funes JA, Soto-Perez-de-Celis E. Perceptions of aging and ageism among Mexican physicians-in-training. Clin Transl Oncol. 2019;21(12):1730–5.
Campbell MH, Gromer J, Emmanuel MK, Harvey A. Attitudes Toward Transgender People Among Future Caribbean Doctors. Arch Sex Behav. 2022;51(4):1903-11. https://doi.org/10.1007/s10508-021-02205-3 .
Hatala R, Case SM. Examining the influence of gender on medical students’ decision making. J Womens Health Gend Based Med. 2000;9(6):617–23.
Deb T, Lempp H, Bakolis I, et al. Responding to experienced and anticipated discrimination (READ): anti -stigma training for medical students towards patients with mental illness – study protocol for an international multisite non-randomised controlled study. BMC Med Educ. 2019;19:41. https://doi.org/10.1186/s12909-019-1472-7 .
Morgan S, Plaisant O, Lignier B, Moxham BJ. Sexism and anatomy, as discerned in textbooks and as perceived by medical students at Cardiff University and University of Paris Descartes. J Anat. 2014;224(3):352–65.
Alford CL, Miles T, Palmer R, Espino D. An introduction to geriatrics for first-year medical students. J Am Geriatr Soc. 2001;49(6):782–7.
Stone J, Moskowitz GB. Non-conscious bias in medical decision making: what can be done to reduce it? Med Educ. 2011;45(8):768–76.
Nazione S. Slimming down medical provider weight bias in an obese nation. Med Educ. 2015;49(10):954–5.
Dogra N, Connin S, Gill P, Spencer J, Turner M. Teaching of cultural diversity in medical schools in the United Kingdom and Republic of Ireland: cross sectional questionnaire survey. BMJ. 2005;330(7488):403–4.
Aultman JM, Borges NJ. A clinical and ethical investigation of pre-medical and medical students’ attitudes, knowledge, and understanding of HIV. Med Educ Online. 2006;11(1):4596.
Deb T, Lempp H, Bakolis I, Vince T, Waugh W, Henderson C, Thornicroft G, Ando S, Yamaguchi S, Matsunaga A, et al. Responding to experienced and anticipated discrimination (READ): anti -stigma training for medical students towards patients with mental illness – study protocol for an international multisite non-randomised controlled study. BMC Med Educ. 2019;19(1):41.
Gonzalez CM, Grochowalski JH, Garba RJ, Bonner S, Marantz PR. Validity evidence for a novel instrument assessing medical student attitudes toward instruction in implicit bias recognition and management. BMC Med Educ. 2021;21(1):205.
Ogunyemi D. A practical approach to implicit bias training. J Grad Med Educ. 2021;13(4):583–4.
Dennis GC. Racism in medicine: planning for the future. J Natl Med Assoc. 2001;93(3 Suppl):1S-5S.
Maina IW, Belton TD, Ginzberg S, Singh A, Johnson TJ. A decade of studying implicit racial/ethnic bias in healthcare providers using the implicit association test. Soc Sci Med. 2018;199:219–29.
Blair IV, Steiner JF, Hanratty R, Price DW, Fairclough DL, Daugherty SL, Bronsert M, Magid DJ, Havranek EP. An investigation of associations between clinicians’ ethnic or racial bias and hypertension treatment, medication adherence and blood pressure control. J Gen Intern Med. 2014;29(7):987–95.
Stanford FC. The importance of diversity and inclusion in the healthcare workforce. J Natl Med Assoc. 2020;112(3):247–9.
Education LCoM. Standards on diversity. 2009. https://health.usf.edu/~/media/Files/Medicine/MD%20Program/Diversity/LCMEStandardsonDiversity1.ashx?la=en .
Onyeador IN, Hudson STJ, Lewis NA. Moving beyond implicit bias training: policy insights for increasing organizational diversity. Policy Insights Behav Brain Sci. 2021;8(1):19–26.
Forscher PS, Mitamura C, Dix EL, Cox WTL, Devine PG. Breaking the prejudice habit: mechanisms, timecourse, and longevity. J Exp Soc Psychol. 2017;72:133–46.
Lai CK, Skinner AL, Cooley E, Murrar S, Brauer M, Devos T, Calanchini J, Xiao YJ, Pedram C, Marshburn CK, et al. Reducing implicit racial preferences: II. Intervention effectiveness across time. J Exp Psychol Gen. 2016;145(8):1001–16.
Sukhera J, Watling CJ, Gonzalez CM. Implicit bias in health professions: from recognition to transformation. Acad Med. 2020;95(5):717–23.
Vuletich HA, Payne BK. Stability and change in implicit bias. Psychol Sci. 2019;30(6):854–62.
Tversky A, Kahneman D. Judgment under uncertainty: Heuristics and biases. Science. 1974;185(4157):1124–31.
Miller DT, Ross M. Self-serving biases in the attribution of causality: fact or fiction? Psychol Bull. 1975;82(2):213–25.
Nickerson RS. Confirmation bias: a ubiquitous phenomenon in many guises. Rev Gen Psychol. 1998;2(2):175–220.
Suveren Y. Unconscious bias: definition and significance. Psikiyatride Guncel Yaklasimlar. 2022;14(3):414–26.
Dietrich D, Olson M. A demonstration of hindsight bias using the Thomas confirmation vote. Psychol Rep. 1993;72(2):377–8.
Green AR, Carney DR, Pallin DJ, Ngo LH, Raymond KL, Iezzoni LI, Banaji MR. Implicit bias among physicians and its prediction of thrombolysis decisions for black and white patients. J Gen Intern Med. 2007;22(9):1231–8.
Rushmer R, Davies HT. Unlearning in health care. Qual Saf Health Care. 2004;13 Suppl 2(Suppl 2):ii10-15.
Vu MT, Pham TTT. Gender, critical pedagogy, and textbooks: Understanding teachers’ (lack of) mediation of the hidden curriculum in the EFL classroom. Lang Teach Res. 2022;0(0). https://doi.org/10.1177/13621688221136937 .
Kalantari A, Alvarez A, Battaglioli N, Chung A, Cooney R, Boehmer SJ, Nwabueze A, Gottlieb M. Sex and race visual representation in emergency medicine textbooks and the hidden curriculum. AEM Educ Train. 2022;6(3):e10743.
Satya-Murti S, Lockhart J. Recognizing and reducing cognitive bias in clinical and forensic neurology. Neurol Clin Pract. 2015;5(5):389–96.
Chang EH, Milkman KL, Gromet DM, Rebele RW, Massey C, Duckworth AL, Grant AM. The mixed effects of online diversity training. Proc Natl Acad Sci U S A. 2019;116(16):7778–83.
Download references
Acknowledgements
The authors would like to thank Dr. Misa Mi, Professor and Medical Librarian at the Oakland University William Beaumont School of Medicine (OWUB) for her assistance with selection of databases and construction of literature search strategies for the scoping review. The authors also wish to thank Dr. Changiz Mohiyeddini, Professor in Behavioral Medicine and Psychopathology at Oakland University William Beaumont School of Medicine (OUWB) for his expertise and constructive feedback on our manuscript.
Author information
Authors and affiliations.
Department of Foundational Sciences, Central Michigan University College of Medicine, Mt. Pleasant, MI, 48859, USA
Brianne E. Lewis
Department of Foundational Medical Studies, Oakland University William Beaumont School of Medicine, 586 Pioneer Dr, Rochester, MI, 48309, USA
Akshata R. Naik
You can also search for this author in PubMed Google Scholar
Contributions
A.R.N and B.E.L were equally involved in study conception, design, collecting data and analyzing the data. B.E.L and A.R.N both contributed towards writing the manuscript. A.R.N and B.E.L are both senior authors on this paper. All authors reviewed the manuscript.
Corresponding author
Correspondence to Akshata R. Naik .
Ethics declarations
Ethics approval and consent to participate.
Not applicable.
Consent for publication
Competing interests.
The authors declare no competing interests.
Additional information
Publisher’s note.
Springer Nature remains neutral with regard to jurisdictional claims in published maps and institutional affiliations.
Rights and permissions
Open Access This article is licensed under a Creative Commons Attribution 4.0 International License, which permits use, sharing, adaptation, distribution and reproduction in any medium or format, as long as you give appropriate credit to the original author(s) and the source, provide a link to the Creative Commons licence, and indicate if changes were made. The images or other third party material in this article are included in the article's Creative Commons licence, unless indicated otherwise in a credit line to the material. If material is not included in the article's Creative Commons licence and your intended use is not permitted by statutory regulation or exceeds the permitted use, you will need to obtain permission directly from the copyright holder. To view a copy of this licence, visit http://creativecommons.org/licenses/by/4.0/ . The Creative Commons Public Domain Dedication waiver ( http://creativecommons.org/publicdomain/zero/1.0/ ) applies to the data made available in this article, unless otherwise stated in a credit line to the data.
Reprints and permissions
About this article
Cite this article.
Lewis, B.E., Naik, A.R. A scoping review to identify and organize literature trends of bias research within medical student and resident education. BMC Med Educ 23 , 919 (2023). https://doi.org/10.1186/s12909-023-04829-6
Download citation
Received : 14 March 2023
Accepted : 01 November 2023
Published : 05 December 2023
DOI : https://doi.org/10.1186/s12909-023-04829-6
Share this article
Anyone you share the following link with will be able to read this content:
Sorry, a shareable link is not currently available for this article.
Provided by the Springer Nature SharedIt content-sharing initiative
- Preclinical curriculum
- Evidence of bis
BMC Medical Education
ISSN: 1472-6920
- Submission enquiries: [email protected]
- General enquiries: [email protected]
Measurement and Mitigation of Bias in Artificial Intelligence: A Narrative Literature Review for Regulatory Science
Affiliations.
- 1 Division of Bioinformatics & Biostatistics, National Center for Toxicological Research, US Food and Drug Administration, Jefferson, Arkansas, USA.
- 2 Division of Imaging, Diagnostics, and Software Reliability, Office of Science and Engineering Laboratories, US Food and Drug Administration Center for Devices and Radiological Health, Silver Spring, Maryland, USA.
- 3 Office of Clinical Pharmacology, Office of Translational Sciences, Center for Drug Evaluation and Research, US Food and Drug Administration, Silver Spring, Maryland, USA.
- 4 Office of Management, National Center for Toxicological Research, US Food and Drug Administration, Jefferson, Arkansas, USA.
- PMID: 38018360
- DOI: 10.1002/cpt.3117
Artificial intelligence (AI) is increasingly being used in decision making across various industries, including the public health arena. Bias in any decision-making process can significantly skew outcomes, and AI systems have been shown to exhibit biases at times. The potential for AI systems to perpetuate and even amplify biases is a growing concern. Bias, as used in this paper, refers to the tendency toward a particular characteristic or behavior, and thus, a biased AI system is one that shows biased associations entities. In this literature review, we examine the current state of research on AI bias, including its sources, as well as the methods for measuring, benchmarking, and mitigating it. We also examine the biases and methods of mitigation specifically relevant to the healthcare field and offer a perspective on bias measurement and mitigation in regulatory science decision making.
Published 2023. This article is a U.S. Government work and is in the public domain in the USA. Clinical Pharmacology & Therapeutics published by Wiley Periodicals LLC on behalf of American Society for Clinical Pharmacology and Therapeutics.
Publication types
- Artificial Intelligence*
- Benchmarking*
- Public Health
Grants and funding
- E0782201/FDA Chief Scientist's Intramural Challenge

An official website of the United States government
The .gov means it’s official. Federal government websites often end in .gov or .mil. Before sharing sensitive information, make sure you’re on a federal government site.
The site is secure. The https:// ensures that you are connecting to the official website and that any information you provide is encrypted and transmitted securely.
- Publications
- Account settings
Preview improvements coming to the PMC website in October 2024. Learn More or Try it out now .
- Advanced Search
- Journal List

Weak evidence of country- and institution-related status bias in the peer review of abstracts
Mathias wullum nielsen.
1 Department of Sociology, University of Copenhagen, Copenhagen, Denmark
Christine Friis Baker
2 Danish Centre for Studies in Research and Research Policy, Department of Political Science, Aarhus University, Aarhus, Denmark
Michael Bang Petersen
3 Department of Political Science, Aarhus University, Aarhus University, Denmark
Jens Peter Andersen
Associated data.
Nielsen MW. 2020. Weak evidence of institutional and country-related status bias. Open Science Framework. x4rj8
All data and code needed to evaluate the conclusions are available here: https://osf.io/x4rj8/ .
The following dataset was generated:
Research suggests that scientists based at prestigious institutions receive more credit for their work than scientists based at less prestigious institutions, as do scientists working in certain countries. We examined the extent to which country- and institution-related status signals drive such differences in scientific recognition. In a preregistered survey experiment, we asked 4,147 scientists from six disciplines (astronomy, cardiology, materials science, political science, psychology and public health) to rate abstracts that varied on two factors: (i) author country (high status vs lower status in science); (ii) author institution (high status vs lower status university). We found only weak evidence of country- or institution-related status bias, and mixed regression models with discipline as random-effect parameter indicated that any plausible bias not detected by our study must be small in size.
Introduction
The growth in scientific publishing ( Larsen and von Ins, 2010 ) makes it increasingly difficult for researchers to keep up-to-date with the newest trends in their fields ( Medoff, 2006 ; Collins, 1998 ). Already in the 1960s, sociologists of science suggested that researchers, in the midst of this information overload, would search for cues as to what literature to read ( Merton, 1968 ; Crane, 1965 ). One important cue was the author’s location. Academic affiliations would cast a “halo-effect” ( Crane, 1967 ) on scholarly work that would amplify the recognition of researchers based at prestigious institutions at the expense of authors from institutions and nations of lower status. This halo effect ties closely to the idea of the “Matthew effect” in science, i.e. “the accumulation of differential advantages for certain segments of the population [of researchers] that are not necessarily bound up with demonstrated differences in capacity” ( Merton, 1988 ).
From a social psychological perspective, country- and institution-related halo effects may arise from stereotypic beliefs about the relative performance capacity of scientists working at more or less prestigious institutions ( Jost et al., 2009 ; Greenwald and Banaji, 1995 ; Ridgeway, 2001 ). According to status characteristics theory, a scientist’s nationality or institutional affiliation can be considered “status signals” that, when salient, implicitly influence peer-evaluations ( Berger et al., 1977 ; Correll and Bernard, 2015 ). Moreover, system justification theory asserts that members of a social hierarchy, such as the scientific community, regardless of their position in this hierarchy, will feel motivated to see existing social arrangements as fair and justifiable to preserve a sense of predictability and certainty around their own position ( Jost et al., 2004 ; Jost et al., 2002 ; Magee and Galinsky, 2008 ; Son Hing et al., 2011 ). As a result, both higher and lower status groups may internalize implicit behaviours and attitudes that favour higher-status groups ( Jost, 2013 ).
Several observational studies have examined the influence of country- and institution-related halo effects in peer-reviewing ( Crane, 1967 ; Link, 1998 ; Murray et al., 2018 ). Most of them indicate slight advantages in favor of researchers from high status countries (such as the US or UK) and universities (such as Harvard University or Oxford University). However, a key limitation of this literature concerns the unobserved heterogeneity attributable to differences in quality. Non-experimental designs do not allow us to determine the advantages enjoyed by scientists at high-status locations independent of their capabilities as scientists, or the content and character of their work.
Here we examine the impact of academic affiliations on scientific judgment, independent of manuscript quality. Specifically, we consider how information about the geographical location and institutional affiliation of authors influence how scientific abstracts are evaluated by their peers. In a preregistered survey experiment, we asked 4147 scientists from six disciplines (astronomy, cardiology, materials science, political science, psychology and public health) to rate abstracts that vary on two factors: (i) author country (high status vs. lower status scientific nation); (ii) institutional affiliation (high status vs. lower status university; see Table 1 ). All other content of the discipline-specific abstracts was held constant.
Number of observations, N, by manipulation (rows) and disciplines (columns).
A few pioneering studies have already attempted to discern the influence of national and institutional location on scholarly peer-assessments, independent of manuscript quality. One study ( Blank, 1991 ) used a randomized field experiment to examine the effects of double blind vs. single blind peer reviewing on acceptance rates in the American Economic Review . The study found no evidence that a switch from single blind to double blind peer reviewing influenced the relative ratings of papers from high-ranked and lower-ranked universities. Another study ( Ross et al., 2006 ) examined the effect of single blind vs. double blind peer reviewing on the assessment of 13,000 abstracts submitted to the American Heart Association’s annual Scientific Sessions between 2000 and 2004. The study found that when abstracts underwent single blind compared to double blind reviewing the relative increase in acceptance rates was higher for US authored abstracts compared to non-US authored abstracts, and for abstracts from highly prestigious US institutions compared to abstracts from non-prestigious US institutions.
A recent survey experiment also found that professors at schools of public health in the US (N: 899) rated one abstract higher on likelihood of referral to a peer, when the authors’ affiliation was changed from a low-income to a high-income country ( Harris et al., 2015 ). However, each participant was asked to rate four abstracts and the results for the remaining three abstracts were inconclusive. Likewise, the study found no evidence of country-related bias in the ratings of the strength of the evidence presented in the abstracts. In another randomized, blinded cross-over study (N: 347), the same authors found that changing the source of an abstract from a low-income to a high-income country slightly improved English clinicians’ ratings of relevance and recommendation to a peer ( Harris et al., 2017 ). Finally, a controlled field experiment recently examined the “within subject” effect of peer-review model (single blind vs. double blind) on the acceptance rates of full-length submissions for a prestigious computer-science conference ( Tomkins et al., 2017 ). The study allocated 974 double blind and 983 single blind reviewers to 500 papers. Two single blind and two double blind reviewers assessed each paper. The study found that single blind reviewers were more likely than double blind reviewers to accept papers from top-ranked universities compared to papers from lower-ranked universities.
Our study contributes to this pioneering work by targeting a broader range of disciplines in the social sciences, health sciences and natural sciences. This allows us to examine possible between-discipline variation in the prevalence of country- or institution-related rater bias.
Our six discipline-specific experiments show weak and inconsistent evidence of country- or institution-related status bias in abstract ratings, and mixed regression models indicate that any plausible effect must be small in size.
In accordance with our preregistered plan (see https://osf.io/4gjwa ), the analysis took place in two steps. First, we used ANOVAs and logit models to conduct discipline-specific analyses of how country- and institution-related status signals influence scholarly judgments made by peer-evaluators. Second, we employed mixed regression models with disciplines as random effect parameter to estimate the direct effect and moderation effects of the presumed association between status signals and evaluative outcomes at an aggregate, cross-disciplinary level.
We used three measures to gauge the assessments of abstracts by peer-evaluators. Abstract score is our main outcome variable. It consists of four items recorded on a five-point scale (1=“very poor”, 5 = “excellent”) that ask the peer-evaluators to assess (i) the originality of the presented research, (ii) the credibility of the results, (iii) the significance for future research, and (iv) the clarity and comprehensiveness of the abstract. We computed a composite measure that specifies each participant’s total-item score for these four items (Cronbach’s α = 0.778). Open full-text is a dichotomous outcome measure that asks whether the peer-evaluator would choose to open the full text and continue reading, if s/he came across the abstract online. Include in conference is a dichotomous outcome measure that asks whether the peer-evaluator would choose to include the abstract for an oral presentation, if s/he was reviewing it as a committee member of a selective international scientific conference (the full questionnaire is included in the Supplementary file 1 ).
As specified in the registered plan, we used G*Power to calculate the required sample size per discipline (N = 429) for detecting a Cohen’s f = 0.15 (corresponding to a Cohen’s d = 0.30) or larger with α = 0.05 and a power of 0.80 in the cross-group comparisons with abstract score as outcome. Table 1 presents the final sample distributions for the three-way factorial design across the six disciplines.
To test for statistical equivalence in the discipline-specific comparisons of our main outcome (abstract score) across manipulations, we performed the two-one-sided tests procedure [TOST] for differences between independent means ( Lakens, 2017a ). We used the pre-registered ‘minimum detectable effect size’ of Cohen’s D= ±0.30 for the discipline-specific equivalence tests.
The boxplots in Figure 1 display the abstracts ratings for respondents assigned to each manipulation across the six disciplines. The discipline-specific ANOVAs with the manipulations as the between-subject factor did not indicate any country- or institution-related status bias in abstract ratings (astronomy: F = 0.71, p=0.491, N = 592; cardiology: F = 1.50, p=0.225, N = 609; materials science: F = 0.73, p=0.482, N = 546; political science: F = 0.53, p=0.587, N = 1008; psychology: F = 0.19, p=0.827, N = 624; public health: F = 0.34, p=0.715, N = 732). The η 2 coefficients were 0.002 in astronomy, 0.005 in cardiology, 0.003 in materials science, and 0.001 in political science, psychology and public health.

Each panel reports results for a specific discipline. The red box plots specify results for respondents exposed to an abstract authored at a high-status institution in the US. The blue box plots specify results for respondents exposed to an abstract authored at a lower-status institution in the US. The green box plots specify results for respondents exposed to an abstract authored at a lower-status institution outside the US. Whiskers show the 1.5 interquartile range. The red, blue and green dots represent outliers, and the grey dots display data points. The boxplots do not indicate any notable variations in abstract scores across manipulations.
Figure 1—figure supplement 1.

Average abstract ratings and 95% CIs across the three manipulations and six disciplines for the question “Please rate the abstract on the following dimension: Originality of the presented research” (Response categories: Very poor = 1, Excellent = 5). Error bars represent 95% confidence intervals based on 1000 bootstrap samples.
Figure 1—figure supplement 2.

Average abstract ratings and 95% CIs across the three manipulations and six disciplines for the question “Please rate the abstract on the following dimension: Credibility of the results” (Response categories: Very poor = 1, Excellent = 5). Error bars represent 95% confidence intervals based on 1000 bootstrap samples.
Figure 1—figure supplement 3.

Average abstract ratings and 95% CIs across the three manipulations and six disciplines for the question “Please rate the abstract on the following dimension: Significance for future research” (Response categories: Very poor = 1, Excellent = 5). Error bars represent 95% confidence intervals based on 1000 bootstrap samples.
Figure 1—figure supplement 4.

Average abstract ratings and 95% CIs across the three manipulations and six disciplines for the question “Please rate the abstract on the following dimension: Clarity of the abstract” (Response categories: Very poor = 1, Excellent = 5). Error bars represent 95% confidence intervals based on 1000 bootstrap samples.
Figure 1—figure supplement 5.

Abstracts could receive a score of between 4 (when the originality, credibility, significance and clarity were all deemed to be very poor) and 20 (when these four factors were all deemed to be excellent). This plot shows the distribution of abstract scores for the six abstracts as assessed by the 4147 reviewers.
A TOST procedure with an α level of 0.05 indicated that the observed effect sizes were within the equivalence bound of d = −0.3 and d = 0.3 for 11 of the 12 between-subject comparisons at the disciplinary level (see Supplementary file 2 , Tables S1–S12). In raw scores, this equivalence bound corresponds to a span from −0.8 to 0.8 abstract rating points on a scale from 4 to 20. In cardiology, the TOST test failed to reject the null-hypothesis of non-equivalence in the evaluative ratings of subjects exposed to abstracts from higher-status US universities and lower-status US universities.
A closer inspection suggests that these findings are robust across the four individual items that make up our composite abstract-score. None of these items show notable variations in abstract ratings across manipulations ( Figure 1—figure supplement 1 – 4 ).
Figures 2 and and3 3 report the outcomes of the discipline-specific logit models with Open full-text and Include in conference as outcomes. The uncertainty of the estimates is notably larger in these models than for the one-way ANOVAs reported above, indicating wider variability in the peer-evaluators’ dichotomous assessments.

Panel A displays the odds ratios for respondents exposed to manipulation 1 (high-status university, US) or manipulation 3 (lower-status university, non-US). Manipulation 2 (lower-status university, US) is the reference group. Panel B plots the adjusted means for manipulation 1, manipulation 2 and manipulation 3. Error bars represent 95% CIs. As shown in Panel A, peer-evaluators in public health were slightly less likely to show interest in opening the full text when the author affiliation was changed from a lower-status university in the US to a lower-status university elsewhere. The results for the remaining eleven comparisons are inclusive. For model specifications, see Supplementary file 2 , Tables S13–S18.

Panel A displays the odds ratios for respondents exposed to manipulation 1 (high-status university, US) or manipulation 3 (lower-status university, non-US). Manipulation 2 (lower-status university, US) is the reference group. Panel B plots the adjusted means for manipulation 1, manipulation 2 and manipulation 3. Error bars represent 95% CIs. As shown in Panel A, peer-evaluators in political science were slightly less likely to show interest in opening the full text when the author affiliation was changed from a lower-status university in the US to a high-status university in the US. The results for the remaining eleven comparisons are inclusive. For model specifications, see Supplementary file 2 , Tables S19–S24.
As displayed in Figure 2 , peer-evaluators in Public Health were between 7.5% and 56.5% less likely to show interest in opening the full-text, when the author affiliation was changed from a lower-status university in the US to a lower-status university elsewhere (Odds ratio:. 634, CI: 0.435–0.925). The odds ratios for the remaining 11 comparisons across the six disciplines all had 95% confidence intervals spanning one. Moreover, in five of the 12 between-subject comparisons, the direction of the observed effects was inconsistent with the a-priori expectation that abstracts from higher-status US universities would be favoured over abstracts from lower-status US universities, and that abstracts from lower-status US universities would be favoured over abstracts from lower-status universities elsewhere.
As displayed in Figure 3 , peer-evaluators in political science were between 7.0% and 59.4% less likely to consider an abstract relevant for a selective, international conference program, when the abstract’s author was affiliated with a higher-status US university compared to a lower-status US university (Odds ratio:. 613, CI:. 406-.930). This result goes against a-priori expectations concerning the influence of status signals on evaluative outcomes. The remaining five disciplines had 95% confidence intervals spanning one, and in six of the 12 comparisons, the direction of the observed effects was inconsistent with a-priori expectations. As indicated in panel b, we observe notable variation in the participants’ average responses to the Include in conference item across disciplines.
Figure 4 plots the fixed coefficients (panel a) and adjusted means (panel b) from the mixed linear regression with Abstract rating as outcome. In accordance with the pre-registered analysis plan, we report the mixed regression models with 99% confidence intervals. The fixed coefficients for the two between-group comparisons tested in this model are close to zero, and the 99% confidence intervals suggest that any plausible difference would fall within the bounds of −0.26 to 0.28 points on the 16-point abstract-rating scale. These confidence bounds can be converted into standardized effects of Cohen’s d = −0.10 to 0.11.

Panel A plots the fixed coefficients for manipulation 1 (high-status university, US) and manipulation 3 (lower-status university, non-US). Manipulation 2 (lower-status university, US) is the reference group. Panel B plots the adjusted means for manipulation 1, manipulation 2 and manipulation 3. Error bars represent 99% CIs. The figure shows that status cues in the form of institutional affiliation or national affiliation have no tangible effects on the respondents’ assessments of abstracts. For model specifications, see Supplementary file 2 , Table S25.
Figure 5 displays odds ratios and 99% confidence intervals for the mixed logit regressions with Open full-text (panel a, upper display) and Include in conference (panel a, lower display) as outcomes. The odds ratios for the experimental manipulations used as predictors in these models range from 0.86 to 1.05 and have 99% confidence intervals spanning the line of no difference. The 99% confidence intervals (panel a) indicate that any plausible effect would fall within the bounds of odds ratio = 0.68 and 1.35, which corresponds to a standardized confidence bound of Cohen’s d = −0.21 to 0.17. The wide confidence bounds for the estimated proportion for Include in conference (panel b) reflect the large variations in average assessments of abstracts across disciplines.

Panel A displays the odds ratios for respondents exposed to manipulation 1 (high-status university, US) or manipulation 3 (lower-status university, non-US). Manipulation 2 (lower-status university, US) is the reference group. Panel B plots the adjusted means for manipulation 1, manipulation 2 and manipulation 3. Error bars represent 99% CIs. As shown in Panel A, the results for both regression models are inconclusive, and the effect sizes are small. For model specifications, see Supplementary file 2 , Tables S26–27.
Robustness checks based on mixed linear and logit models were carried out to examine the effects of the experimental manipulations on the three outcome measures, while restricting the samples to (i) participants that responded correctly to a manipulation-check item, and (ii) participants that saw their own research as being ‘extremely close’, ‘very close’ or ‘somewhat close’ to the subject addressed in the abstract. All of these models yielded qualitatively similar results, with small residual effects and confidence intervals spanning 0 in the linear regressions and one in the logistic regressions (see Supplementary file 2 , Tables S28–S33). Moreover, a pre-registered interaction analysis was conducted to examine whether the influence of country- and institution-related status signals was moderated by any of the following characteristics of the peer evaluators: (i) their descriptive beliefs in the objectivity and fairness of peer-evaluation; (ii) their structural location in the science system (in terms of institutional affiliation and scientific rank); (iii) their research accomplishments; (iv) their self-perceived scientific status. All of these two-way interactions had 99% CI intervals spanning 0 in the linear regressions and one in the logistic regressions, indicating no discernible two-way interactions (see Supplementary file 2 , Tables S34–S39).
Contrary to the idea of halo effects, our study shows weak and inconsistent evidence of country- or institution-related status bias in abstract ratings. In the discipline-specific analyses, we observed statistically significant differences in two of 36 pairwise comparisons (i.e. 5.6%) and, of these, one was inconsistent with our a-priori expectation of a bias in favour of high-status sources. Moreover, the estimated confidence bounds derived from the multilevel regressions were either very small or small according to Cohen’s classification of effect sizes ( Cohen, 2013 ). These results align with the outcomes of three out of five existing experiments, which also show small and inconsistent effects of country- and institution-related status bias in peer-evaluation ( Blank, 1991 ; Harris et al., 2015 ; Harris et al., 2017 ). However, it should be noted that even small effects may produce large population-level variations if they accumulate over the course of scientific careers. Moreover, one could argue that small effects would be the expected outcome for a lightweight manipulation like ours in an online survey context, where the participants are asked to make decisions without real-world implications. Indeed, it is possible that the effects of country- and institution level status signals would be larger in real-world evaluation processes, where reviewers have more at stake.
Certain scope conditions also delimit the situations to which our conclusions may apply. First, our findings leave open the possibility that peer-evaluators discard research from less reputable institutions and science nations without even reading their abstracts. In other words, our study solely tests whether scientists, after being asked to carefully read an assigned abstract, on average will rate contributions from prestigious locations more favourably.
Second, even when peer-evaluators identify and read papers from “lower-status” sources, they may still omit to cite them, and instead frame their contributions in the context of more high-status sources. In the future, researchers might add to this work by examining if these more subtle processes contribute to shape the reception and uptake of scientific knowledge. Third, our conclusion of little or no bias in abstract review does not necessarily imply that biases are absent in other types of peer assessment, such as peer reviewing of journal and grant submissions, where evaluations usually follow formalized criteria and focus on full-text manuscripts and proposals. Likewise, our study does not capture how country- and institution-related status signals may influence the decisions of journal editors. Indeed, journal editors play an important role in establishing scientific merits and reputations and future experimental work should examine how halo effects may shape editorial decisions. Fourth, although we cover a broad span of research areas, it is possible that our experiment would have produced different results for other disciplines. Fifth, it should be noted that our two dichotomous outcome items (open full text and include in conference) refer to two rather different evaluative situations that may be difficult to compare. For instance, a researcher may wish to read a paper (based on its abstract) while finding it inappropriate for a conference presentation and vice versa. Moreover, the competitive aspect of selecting an abstract for a conference makes this evaluative situation quite different from the decision to open a full text.
A key limitation is that our experiment was conducted in non-probability samples with low response rates, which raises questions about selection effects. One could speculate that the scientists that are most susceptible to status bias would be less likely to participate in a study conducted by researchers from two public universities in Denmark. Moreover, we decided to include internationally outstanding universities in our high-status category (California Institute of Technology, Columbia University, Harvard University, Yale University, Johns Hopkins University, Massachusetts Institute of Technology, Princeton University, Stanford University, and University of California, Berkeley). By so doing, we aimed to ensure a relatively strong status signal in the abstract’s byline. There is a risk that this focus on outstanding institutions may have led some survey participants to discern the purpose of the experiment and censor their responses to appear unbiased. However, all participants were blinded to the study objectives (the invitation email is included in Supplementary file 1 ), and to reduce the salience of the manipulation, we asked each participant to review only one abstract. Moreover, in the minds of the reviewers, many other factors than the scholarly affiliations might have been manipulated in the abstracts, including the gender of the authors ( Knobloch-Westerwick et al., 2013 ; Forscher et al., 2019 ), the style of writing, the source of the empirical data presented in the abstract, the clarity of the statistical reporting, the reporting of positive vs. negative and mixed results ( Mahoney, 1977 ), the reporting of intuitive vs. counter-intuitive findings ( Mahoney, 1977 ; Hergovich et al., 2010 ), and the reporting of journal information and visibility metrics (e.g. citation indicators or Altmetric scores) ( Teplitskiy et al., 2020 ). A final limitation concerns the varying assessments of abstract quality across disciplines. This cross-disciplinary variation was particularly salient for the Include in conference item ( Figure 3 , panel b), which may indicate differences in the relative difficulty of getting an oral presentation at conferences in the six disciplines. In the mixed-regression models based on data from all six disciplines, we attempt to account for this variation by including discipline as random-effect parameter. In summary, this paper presents a large-scale, cross-disciplinary examination of how country- and institution-related status signals influence the reception of scientific research. In a controlled experiment that spanned six disciplines, we found no systematic evidence of status bias against abstracts from lower status universities and countries. Future research should add to this work by examining if other processes related to the social, material and intellectual organization of science contribute to producing and reproducing country- and institution-related status hierarchies.
Our study complies with all ethical regulations. Aarhus University’s Institutional Review Board approved the study (case no. 2019-616-000014). We obtained informed consent from all participants. The sampling and analysis plan was preregistered at the Open Science Framework on September 18, 2019. We have followed all of the steps presented in the registered plan, with two minor deviations. First, we did not preregister the equivalence tests reported for the discipline-specific analyses presented in Figure 1 . Second, in the results section, we report the outcomes of the mixed regression models with abstract score as outcome based on linear models instead of the tobit models included in the registered plan. Tables S40–S44 in Supplementary file 2 report the outcomes of the mixed-tobit models, which are nearly identical to the results from the linear models reported in Tables S25, S28, S31, S36, S37 in Supplementary file 2 .
Participants
The target population consisted of research-active academics with at least three articles published between 2015 and 2018 in Clarivate’s Web of Science (WoS). To allow for the retrieval of contact information (specifically email addresses), we limited our focus to corresponding authors with a majority of publications falling into one of the following six disciplinary categories: astronomy, cardiology, materials science, political science, psychology and public health. These disciplines were chosen to represent the top-level domains; natural science, technical science, health science, and social science. We did not include the arts and humanities as the majority of fields in this domain have very different traditions of publishing and interpret scholarly quality in less comparable terms. While other fields could have been chosen as representative of those domains, practical aspects of access to field experts and coverage in Web of Science were deciding for the final delineation. We used the WoS subject categories and the Centre for Science and Technology Studies’ (CWTS) meso-level cluster classification system to identify eligible authors within each of these disciplines. For all fields, except for materials science, the WoS subject categories provided a useful field delineation. In materials science, the WoS subject categories were too broad. Hence, we used the article-based meso-level classification of CWTS to identify those papers most closely related to the topic of our abstract. In our sampling strategy, we made sure that no participants were asked to review abstracts written by (fictive) authors affiliated with their own research institutions.
We used G*Power to calculate the required sample size for detecting a Cohen’s f = 0.15 (corresponding to a Cohen’s d = 0.30) or larger with α = 0.05 and a power of 0.80 in the discipline-specific analyses with abstract rating as outcome. With these assumptions, a comparison of three groups would require at least 429 respondents per discipline. Response rates for email-based academic surveys are known to be low ( Myers et al., 2020 ). Based on the outcomes of a pilot study targeting neuroscientists, we expected a response rate around 5% and distributed the survey to approximately 72,000 researchers globally (i.e. approximately 12,000 researchers per field) (for specifications, see Supplementary file 2 , Table S45). All data were collected in October and November 2019. Due to low response rates in materials science and cardiology, we expanded the recruitment samples by an additional ~12,000 scientists in each of these disciplines. In total, our recruitment sample consisted 95,317 scientists. Each scientist was invited to participate in the survey by email, and we used the web-based Qualtrics software for data collection. We sent out five reminders and closed the survey two weeks after the final reminder. Eight percent (N = 7,401) of the invited participants opened the email survey link, and about six percent (N = 5,413) completed the questionnaire (for specifications on discipline-specific completion rates, see Supplementary file 2 , Table S45). For ethical reasons, our analysis solely relies on data from participants that reached the final page of the survey, where we debriefed about the study’s experimental manipulations. The actual response rate is difficult to estimate. Some scientists may have refrained from participating in the study because they did not see themselves as belonging to one of the targeted disciplines. Others may not have responded because they were on sabbatical, parental leave or sick leave. Moreover, approximately 16 percent (15,247) of the targeted email addresses were inactive or bounced for other reasons. A crude estimate of the response rate would thus be 5,413/(95,317–15,247)=0.07, or seven percent. The gender composition of the six respondent samples largely resembles that of the targeted WoS populations ( Supplementary file 2 , Table S46). However, the average publication age (i.e. years since first publication in WoS) is slightly higher in the respondent samples compared to the targeted WoS populations, which may be due to the study’s restricted focus on corresponding authors (the age distributions are largely similar across the recruitment and respondent samples). The distribution of respondents (per discipline) across countries in WoS, the recruitment sample, and the respondent sample is reported in Supplementary file 2 , Table S47.
Pretesting and pilot testing
Prior to launching, the survey was pretested with eight researchers in sociology, information science, political science, psychology, physics, biomedicine, clinical medicine, and public health. In the pretesting, we used verbal probing techniques and “think alouds” to identify questions that the participants found vague and unclear. Moreover, we elicited how the participants arrived at answers to the questions and whether the questions were easy or hard to answer, and why.
In addition, we pilot-tested the survey in a sample of 6,000 Neuroscientists to (i) estimate the expected response rate per discipline, (ii) check the feasibility of a priming instrument that we chose not to include in the final survey, (iii) detect potential errors in the online version of the questionnaire before launching, and (iv) verify the internal consistency of two of the composite measures used in the survey (i.e. abstract score and meritocracy beliefs).
In each of the six online-surveys (one per discipline), we randomly assigned participants to one of the three manipulations ( Table 1 ). All participants were blinded to the study objectives.
Manipulations
We manipulated information about the abstract’s institutional source (high status vs. lower status US research institution) and country source (lower status US research institution vs. lower-status research institution in a select group of European, Asian, Middle Eastern, African and South American countries). The following criteria guided our selection of universities for the manipulation of institutional affiliation: Candidates for the “high status” category all ranked high in the US National Research Council’s field-specific rankings of doctorate programs, and consistently ranked high (Top 20) in five subfield-specific university rankings (US News’ graduate school ranking, Shanghai ranking, Times Higher Education, Leiden Ranking and QS World University Ranking).
The universities assigned to the “lower status” category were selected based on the PP-top 10% citation indicator used in the Leiden University Ranking. For non-US universities, we limited our focus to less esteemed science nations in Southern Europe, Eastern Europe, Latin America, Asia, Africa and the Middle East. Institutions assigned to the “lower-status” category were matched (approximately) across countries with respect to PP-top 10% rank in the field-specific Leiden University ranking. We decided to restrict our focus to universities in the Leiden ranking to ensure that all fictive affiliations listed under the abstracts represented research active institutions in the biomedical and health sciences, the physical sciences and engineering, or the social sciences. By matching lower-status universities on their PP-top 10% rank, we ensured that the lower-status universities selected for each discipline had a comparable level of visibility in the scientific literature. Since a given university’s rank on the PP-top 10% indicator may not necessarily align with its general reputation, we also ensured that none of the lower-status universities were within the top-100 in the general Shanghai, Times Higher Education and QS World University rankings.
Given this approach, the specific institutions that fall into the “high status” and “lower status” categories vary by discipline. We carried out manual checks to ensure that all of the selected universities had active research environments within the relevant research disciplines. The universities assigned to each category (high-status [US], lower-status [US], lower-status [non-US]), and the average abstract scores per university, per discipline, are listed in Supplementary file 2 . Table S48, S49.
The abstracts were created or adapted for this study and are not published in their current form. The abstracts used in astronomy, materials science, political science and psychology were provided by relevant researchers in the respective disciplines and have been slightly edited for the purposes of this study. The abstracts used in cardiology and public health represent rewritten versions of published abstracts with numerous alterations to mask any resemblance with the published work (the six abstracts are available in Supplementary file 1 ). Author names were selected by searching university websites for each country and identifying researchers in disciplines unrelated to this study.
Variable specifications are reported in Supplementary file 2 , Table S50. The outcome variables used in this analysis are specified above. We used dichotomous variables to estimate the effect of the manipulations on the outcomes in all regression models. We used the following measures to compute the moderation variables included in the two-way interaction analyses. Our measure of the respondents’ descriptive beliefs in the objectivity and fairness of peer-evaluation in their own research field (i.e. meritocratic beliefs ) was adapted from ref ( Anderson et al., 2010 ). A sample item from this measure reads: “In my research field, scientists evaluate research primarily on its merit, i.e. according to accepted standards of the field”. We adapted the sample item from ref ( Anderson et al., 2010 ). The two other items were developed for this study. Ratings were based on a five-point scale ranging from (1) ‘Strongly agree’ to (5) ‘Strongly disagree’. Based on these items, we computed a composite measure that specifies each participant’s total-item score for these three items (i.e. meritocratic beliefs) (Cronbach’s α = 0.765). We used two pieces of information to measure the participants’ structural location in the science system (i.e. s tructural location ): (i) information about scientific rank collected through the survey, and (ii) information about scientific institution obtained from Web of Science. Our measure of structural location is dichotomous. Associate professors, full professors, chairs and deans at top ranked international research institutions are scored as 1; all other participants are scored as 0. Here, we define top-ranked research institutions as institutions that have consistently ranked among the top 100 universities with the highest proportion of top 10% most cited papers within the past 10 years, according to the Leiden Ranking. We used article metadata from WOS to construct an author-specific performance profile for each respondent (i.e. research accomplishments ). Specifically, we assigned researchers that were among the top-10% most cited in their fields (based on cumulative citation impact) to the “high status” group. All other participants were assigned to the “lower status” group. Our measure of self-perceived status was adapted from the MacArthur Scale of Subjective Social Status ( Adler and Stewart, 2007 ). We asked the respondents to locate themselves on a ladder with ten rungs representing the status hierarchy in their research area. Respondents that positioned themselves at the top of the ladder were scored as 9, and respondents positioning themselves at the bottom were scored as 0.
Manipulation and robustness checks
As a manipulation check presented at the end of the survey, we asked the participants to answer a question about the author’s institutional affiliation/country affiliation in the abstract that they just read. The question varied depending on manipulation and discipline. For robustness checks, we included an item to measure the perceived distance between the participant’s own research area and the topic addressed in the abstract. Responses were based on a five-point scale ranging from (1) ‘Extremely close’ to (5) ‘Not close at all’. In the statistical analysis, these response options were recoded into dichotomous categories (‘Not close at all’, ‘Not too close’=0, ‘Somewhat close’, ‘Very close’, ‘Extremely close’=1).
Data exclusion criteria
In accordance with our registered plan, respondents that demonstrated response bias (10 items of the same responses, e.g. all ones or fives) were removed from the analysis. Moreover, we removed all respondents that completed the survey in less than 2.5 min.
Statistical analysis
We used one-way ANOVAs and logit models to perform the discipline-specific, between-group comparisons. We estimated mixed linear regressions and tobit models (reported in Supplementary file 2 ) with disciplines as random effect parameter to measure the relationship between the experimental manipulations and abstract rating. The tobit models were specified with a left-censoring at four and a right-censoring at 20. Figure 1—figure supplement 5 displays the data distribution for the outcome measure abstract rating. The data distribution for this measure was assumed to be normal.
We estimated multilevel logistic regressions with disciplines as random effect parameter to examine the relationship between the manipulations and the outcome variables Open full-text and Include in conference . Consistent with our pre-registered analysis plan, we used 95% confidence intervals to make inferences based on the discipline specific ANOVAs and logistic regressions. To minimize Type I errors arising from multiple testing, we reported the results of all multilevel regression models with 99% confidence intervals.
We created two specific samples for the moderation analyses. The first of these samples only include respondents that had been exposed to abstracts from a high status US university or a lower status US university. The second sample was restricted to respondents that had been exposed to abstracts from a lower status US university or a lower status university outside the US. The Variance Inflation Factors for the predictors included in the moderation analyses (i.e. the manipulation variables, Meritocracy beliefs, Structural Location, Research Accomplishments and Self-perceived status) were all below two.
We conducted the statistical analyses in STATA 16 and R version 4.0.0. For the multilevel linear, tobit and logit regressions, we used the “mixed”, “metobit” and “melogit” routines in STATA. Examinations of between-group equivalence were performed with the R package ‘TOSTER’ ( Lakens, 2017b ). Standardized effects were calculated using the R package ‘esc’ ( Lüdecke, 2018 ).
Acknowledgements
The Centre for Science and Technology Studies (CWTS) at Leiden University generously provided bibliometric indices and article metadata. We thank Emil Bargmann Madsen, Jesper Wiborg Schneider and Friedolin Merhout for very useful comments on the manuscript.
Biographies
Mathias Wullum Nielsen is in the Department of Sociology, University of Copenhagen, Copenhagen, Denmark
Christine Friis Baker is in the Danish Centre for Studies in Research and Research Policy, Department of Political Science, Aarhus University, Aarhus, Denmark
Emer Brady is in the Danish Centre for Studies in Research and Research Policy, Department of Political Science, Aarhus University, Aarhus, Denmark
Michael Bang Petersen is in the Department of Political Science, Aarhus University, Aarhus, Denmark
Jens Peter Andersen is in the Danish Centre for Studies in Research and Research Policy, Department of Political Science, Aarhus University, Aarhus, Denmark
Funding Statement
The funders had no role in study design, data collection and interpretation, or the decision to submit the work for publication.
Contributor Information
Peter Rodgers, eLife, United Kingdom.
Funding Information
This paper was supported by the following grants:
- Carlsbergfondet CF19-0566 to Mathias Wullum Nielsen.
- Aarhus Universitets Forskningsfond AUFF-F-2018-7-5 to Christine Friis Baker, Emer Brady, Jens Peter Andersen.
Additional information
No competing interests declared.
Conceptualization, Resources, Data curation, Formal analysis, Supervision, Funding acquisition, Validation, Investigation, Visualization, Methodology, Writing - original draft, Project administration, Writing - review and editing.
Formal analysis, Investigation, Visualization, Methodology, Writing - review and editing.
Formal analysis, Validation, Investigation, Methodology, Writing - review and editing.
Conceptualization, Data curation, Formal analysis, Funding acquisition, Validation, Investigation, Visualization, Methodology, Project administration, Writing - review and editing.
Human subjects: Aarhus University's Institutional Review Board approved the study. We obtained informed consent from all participants (case no. 2019-616-000014).
Additional files
Supplementary file 1., supplementary file 2., transparent reporting form, data availability.
- Adler N, Stewart J. The MacArthur scale of subjective social status. [March 18, 2021]; 2007 https://macses.ucsf.edu/research/psychosocial/subjective.php
- Anderson MS, Ronning EA, Devries R, Martinson BC. Extending the Mertonian norms: scientists' subscription to norms of research. The Journal of Higher Education. 2010; 81 :366–393. doi: 10.1080/00221546.2010.11779057. [ PMC free article ] [ PubMed ] [ CrossRef ] [ Google Scholar ]
- Berger J, Fisek H, Norman R, Zelditch M. Status Characteristics and Social Interaction. New York: Elsevier; 1977. [ Google Scholar ]
- Blank RM. The effects of double-blind versus single-blind reviewing: experimental evidence from the american economic review. The American Economic Review. 1991; 81 :1041–1067. [ Google Scholar ]
- Cohen J. Statistical Power Analysis for the Behavioral Sciences. Cambridge, MA: Academic Press; 2013. [ CrossRef ] [ Google Scholar ]
- Collins R. The Sociology of Philosophies. Cambridge, MA: Harvard University Press; 1998. [ Google Scholar ]
- Correll S, Bernard S. Biased estimators? Comparing status and statistical theories of gender discrimination. In: Thye S. R, Lawler E. J, editors. Advances in Group Processes. Vol. 23. Bingley: Emerald Group Publishing Limited; 2015. pp. 89–116. [ CrossRef ] [ Google Scholar ]
- Crane D. Scientists at major and minor universities: a study of productivity and recognition. American Sociological Review. 1965; 30 :699–714. doi: 10.2307/2091138. [ PubMed ] [ CrossRef ] [ Google Scholar ]
- Crane D. The gatekeepers of science: some factors affecting the selection of articles for scientific journals. The American Sociologist. 1967; 2 :195–201. [ Google Scholar ]
- Forscher PS, Cox WTL, Brauer M, Devine PG. Little race or gender bias in an experiment of initial review of NIH R01 grant proposals. Nature Human Behaviour. 2019; 3 :257–264. doi: 10.1038/s41562-018-0517-y. [ PMC free article ] [ PubMed ] [ CrossRef ] [ Google Scholar ]
- Greenwald AG, Banaji MR. Implicit social cognition: attitudes, self-esteem, and stereotypes. Psychological Review. 1995; 102 :4–27. doi: 10.1037/0033-295X.102.1.4. [ PubMed ] [ CrossRef ] [ Google Scholar ]
- Harris M, Macinko J, Jimenez G, Mahfoud M, Anderson C. Does a research article's country of origin affect perception of its quality and relevance? A national trial of US public health researchers. BMJ Open. 2015; 5 :e008993. doi: 10.1136/bmjopen-2015-008993. [ PMC free article ] [ PubMed ] [ CrossRef ] [ Google Scholar ]
- Harris M, Marti J, Watt H, Bhatti Y, Macinko J, Darzi AW. Explicit bias toward high-income-country research: a randomized, blinded, crossover experiment of English clinicians. Health Affairs. 2017; 36 :1997–2004. doi: 10.1377/hlthaff.2017.0773. [ PubMed ] [ CrossRef ] [ Google Scholar ]
- Hergovich A, Schott R, Burger C. Biased evaluation of abstracts depending on topic and conclusion: further evidence of a confirmation bias within scientific psychology. Current Psychology. 2010; 29 :188–209. doi: 10.1007/s12144-010-9087-5. [ CrossRef ] [ Google Scholar ]
- Jost JT, Pelham BW, Carvallo MR. Non-conscious forms of system justification: implicit and behavioral preferences for higher status groups. Journal of Experimental Social Psychology. 2002; 38 :586–602. doi: 10.1016/S0022-1031(02)00505-X. [ CrossRef ] [ Google Scholar ]
- Jost JT, Banaji MR, Nosek BA. A decade of system justification theory: accumulated evidence of conscious and unconscious bolstering of the status quo. Political Psychology. 2004; 25 :881–919. doi: 10.1111/j.1467-9221.2004.00402.x. [ CrossRef ] [ Google Scholar ]
- Jost JT, Rudman LA, Blair IV, Carney DR, Dasgupta N, Glaser J, Hardin CD. The existence of implicit bias is beyond reasonable doubt: a refutation of ideological and methodological objections and executive summary of ten studies that no manager should ignore. Research in Organizational Behavior. 2009; 29 :39–69. doi: 10.1016/j.riob.2009.10.001. [ CrossRef ] [ Google Scholar ]
- Jost JT. Cognitive social psychology. In: Moskowitz G. B, editor. Social Cognition. Psychology Press; 2013. pp. 92–105. [ Google Scholar ]
- Knobloch-Westerwick S, Glynn CJ, Huge M. The Matilda effect in science communication: an experiment on gender bias in publication quality perceptions and collaboration interest. Science Communication. 2013; 35 :603–625. doi: 10.1177/1075547012472684. [ CrossRef ] [ Google Scholar ]
- Lakens D. Equivalence tests: a practical primer for t tests, correlations, and meta-analyses. Social Psychological and Personality Science. 2017a; 8 :355–362. doi: 10.1177/1948550617697177. [ PMC free article ] [ PubMed ] [ CrossRef ] [ Google Scholar ]
- Lakens D. R package version 0. 2-648 TOSTER: Two One-Sided Tests (TOST) Equivalence Testing. 2017b https://rdrr.io/cran/TOSTER/
- Larsen PO, von Ins M. The rate of growth in scientific publication and the decline in coverage provided by Science Citation Index. Scientometrics. 2010; 84 :575–603. doi: 10.1007/s11192-010-0202-z. [ PMC free article ] [ PubMed ] [ CrossRef ] [ Google Scholar ]
- Link AM. US and non-US submissions: an analysis of reviewer bias. JAMA. 1998; 280 :246–247. doi: 10.1001/jama.280.3.246. [ PubMed ] [ CrossRef ] [ Google Scholar ]
- Lüdecke D. R package version 0, 4(1)42 Esc: Effect Size Computation for Meta-Analysis. 2018 https://rdrr.io/cran/esc/
- Magee JC, Galinsky AD. Social hierarchy: The self‐reinforcing nature of power and status. Academy of Management Annals. 2008; 2 :351–398. doi: 10.5465/19416520802211628. [ CrossRef ] [ Google Scholar ]
- Mahoney MJ. Publication prejudices: an experimental study of confirmatory bias in the peer review system. Cognitive Therapy and Research. 1977; 1 :161–175. doi: 10.1007/BF01173636. [ CrossRef ] [ Google Scholar ]
- Medoff MH. Evidence of a Harvard and Chicago Matthew Effect. Journal of Economic Methodology. 2006; 13 :485–506. doi: 10.1080/13501780601049079. [ CrossRef ] [ Google Scholar ]
- Merton RK. The Matthew effect in science. Science. 1968; 159 :56–63. doi: 10.1126/science.159.3810.56. [ PubMed ] [ CrossRef ] [ Google Scholar ]
- Merton RK. The Matthew effect in science, II: Cumulative advantage and the symbolism of intellectual property. Isis. 1988; 79 :606–623. doi: 10.1086/354848. [ CrossRef ] [ Google Scholar ]
- Murray D, Siler K, Lariviére V, Chan WM, Collings AM, Raymond J, Sugimoto CR. Gender and international diversity improves equity in peer review. bioRxiv. 2018 doi: 10.1101/400515. [ CrossRef ]
- Myers KR, Tham WY, Yin Y, Cohodes N, Thursby JG, Thursby MC, Schiffer P, Walsh JT, Lakhani KR, Wang D. Unequal effects of the COVID-19 pandemic on scientists. Nature Human Behaviour. 2020; 4 :880–883. doi: 10.1038/s41562-020-0921-y. [ PubMed ] [ CrossRef ] [ Google Scholar ]
- Ridgeway CL. Blackwell Handbook of Social Psychology: Group Processes. Blackwell; 2001. [ CrossRef ] [ Google Scholar ]
- Ross JS, Gross CP, Desai MM, Hong Y, Grant AO, Daniels SR, Hachinski VC, Gibbons RJ, Gardner TJ, Krumholz HM. Effect of blinded peer review on abstract acceptance. JAMA. 2006; 295 :1675–1680. doi: 10.1001/jama.295.14.1675. [ PubMed ] [ CrossRef ] [ Google Scholar ]
- Son Hing LS, Bobocel DR, Zanna MP, Garcia DM, Gee SS, Orazietti K. The merit of meritocracy. Journal of Personality and Social Psychology. 2011; 101 :433–450. doi: 10.1037/a0024618. [ PubMed ] [ CrossRef ] [ Google Scholar ]
- Teplitskiy M, Duede E, Menietti M, Lakhani KR. Citations systematically misrepresent the quality and impact of research articles: survey and experimental evidence from thousands of citers. arXiv. 2020 https://arxiv.org/abs/2002.10033
- Tomkins A, Zhang M, Heavlin WD. Reviewer bias in single- versus double-blind peer review. PNAS. 2017; 114 :12708–12713. doi: 10.1073/pnas.1707323114. [ PMC free article ] [ PubMed ] [ CrossRef ] [ Google Scholar ]
- eLife. 2021; 10: e64561.
Decision letter
Bjorn hammarfelt.
University of Boras, Sweden
In the interests of transparency, eLife publishes the most substantive revision requests and the accompanying author responses.
Thank you for submitting your article "Weak evidence of institutional and country-related bias in experimental study of 4,000 scientists' research assessments" to eLife for consideration as a Feature Article. Your article has been reviewed by three peer reviewers, and the following individual involved in review of your submission has agreed to reveal their identity: Bjorn Hammarfelt (Reviewer #1).
In view of the reviewers comments, you are invited to prepare a revised submission that addresses their comments, please see below.
This is a carefully designed study and a well-written paper about bias in peer review based on authors' institutional and country location. The survey design is novel and the results seem robust. The main results show weak evidence for the claim that researchers are biased towards high-status countries and institutions. The presentation is logical and the paper is well-structured, and the main arguments are clearly articulated. However, there are also a number of points that need to be addressed to make the article suitable for publication.
Essential revisions:
1) Why choose the very top universities – like Harvard and MIT – rather than top universities that are less likely to be identified as the classic examples of “the best”. Is there a risk that participants of the survey would realize that this was part of the experiment, and thus acting accordingly in terms of “social desirability”. Perhaps a short discussion of these choices, maybe in the limitations section, would be useful.
2) Similarly, I wonder why there are no "Elite (non-US)" institutions (e.g. Oxford, ETH, etc.). Would not the inclusion of such universities make sense given that you include Elite (US) elite, and Non-elite (US) as well as Non-elite (non-US)? As it stands the selection might come off as a bit too focused on an American context, while the participants in the survey are from the entire world.
3) Please list somewhere in the article or supporting material the countries and institutions included in the survey that fall into each category and field.
4) The authors divide institutions into three categories. If I understood well, high US institutions are those in the top 20 of several discipline based rankings and in high positions in the US NRC doctorate programs ranking. Low status are those with low share of highly cited papers according to Leiden Ranking, and the same for non-US non-Elite from certain regions. While rankings may be a plausible way to go, I am not sure to what extent using the PP-top 10% score will help identifying low status institutions. In this case, the authors are looking for institutions with lower reputation (which not necessarily aligns with low citation performance), furthermore, the PP-top indicator is non-size independent, and therefore will work against larger institutions. Also, the Leiden Ranking ranks around 1000 institutions worldwide which fit certain publication threshold, probably including institutions that do not make it to the Leiden Ranking would give a better idea of low status.
5) I wonder to what extent the difference between the three categories is sufficiently detailed as to be able to show any differences. My concern is that you divide in so few groups that in the end differences may be hidden within those three large groups of institutions.
6) Effect sizes
The authors find that effect of manipulations on abstract quality ratings is likely not larger than the band -0.8 to 0.8 (16 pt scale) (Results). That does seem small, although in Cohen's d terms, d=-0.3 to 0.3 isn't all that small. Overall, we would expect effects of a light-weight manipulation in an online survey context to be tiny, but it's really hard to generalize such interventions to the field, where status effects may be large and subtle. So I'd like a little more language emphasizing low-ish (but informative) external validity here, and that the prior on light weight manipulation is very small effects.
7) You control for the reliability on the peers' judgment (how close is the abstract to their area of expertise). Why did you not do something similar with the institution as a means to control on the appropriateness of the category assignment of each institution? Could this be affecting also the results?
8) I understand that the six fields/disciplines were selected in order to cover a range of different disciplines, but a few paragraphs about why these particular fields were chosen would be useful.
9) Please discuss further the difference between choosing to read the full text and to include it in a conference. These are rather different evaluative situations: one may want to read a paper that one would not accept for a conference and vice versa. For example, a researcher might deem a paper as interesting, yet have doubts about the quality, or judge it as “worthy” of presentation while not being interested in reading it. You discuss that the disciplinary differences were higher when choosing to include in a conference or not (Discussion), and I would add that the competitive aspect of selecting for a conference makes this choice rather different than choosing to read a paper or not. This could be elaborated upon in the text and perhaps be something to study closer in future research.
10) In the framing of the paper the authors refer to biases on peer review but also discuss the Matthew Effect. While there is a relation between the halo effect that the authors are exploring and the Matthew Effect, the latter refers to the reception of publications (in terms of citations and prestige) while the former refers to biases in peer review. I think this difference is not sufficiently clear in the text, especially when reading the Appendix Text. I'd say that the fact that an halo effect may not be present in peer review would not necessarily reflect on a Matthew Effect or vice versa.
11) Situating this work in the existing literature.
I think the part of the literature review section that tries to distinguish this contribution from others isn't entirely fair. Here are the distinguishing points, followed by my comments on them:
a) Separating out location status vs. institution status
Comment: Why would this separating out really matter? Surely status of country is highly correlated with status of institution, and, presumably, the mechanism at work are inferences of quality from status. It seems fine to me to separate them out, but would be good to have an argument for why this is valuable to do
b) Previous RCTs in the peer review context may have been hampered by treatment leakage to the control group.
Comment: This surely happens ( https://arxiv.org/abs/1709.01609 ). But it just means the reported effects in those RCTs are lower bounds, as those papers I believe readily admit. So I don't think this has been a major methodological problem in dire need of fixing.
So I don't think what this experiment is doing is super novel and fixes glaring oversights in the literature. BUT, that isn't a bad thing, in my view. Simply adding observations, particularly those that are well executed, adequately powered, and cross-disciplinary, is important enough. It's an important topic, so we should have more than just one or two RCTs in it. Crucially, we know there are file-drawer effects out there, likely in this space too, so this study is useful for making sure the published record is unbiased.
Author response
Essential revisions: 1) Why choose the very top universities – like Harvard and MIT – rather than top universities that are less likely to be identified as the classic examples of “the best”. Is there a risk that participants of the survey would realize that this was part of the experiment, and thus acting accordingly in terms of “social desirability”. Perhaps a short discussion of these choices, maybe in the limitations section, would be useful.
This is an excellent point. We now reflect on why we made this choice, and the possible limitations of this choice, in the Discussion section.
2) Similarly, I wonder why there are no "Elite (non-US)" institutions (e.g. Oxford, ETH, etc). Would not the inclusion of such universities make sense given that you include Elite (U.S) elite, and Non-elite (US) as well as Non-elite (non-US)? As it stands the selection might come off as a bit too focused on an American context, while the participants in the survey are from the entire world.
This is a fair point. We decided to restrict the category of elite universities to the U.S. for the following reasons. (1) Our design allowed us to compare within-country variations in university status, and hereby tease apart the effect of country status and institution status. If we had included Oxford, Cambridge, ETH and University of Amsterdam, this would have required a more comprehensive set of lower-ranked universities in the UK, Switzerland and Netherlands as well, which in turn would have made our setup more complex. In other words, we wanted to keep the experiment simple. (2) 15 of the top 20 universities in the 2019 THE ranking, and 10 of the top 20 institutions in the 2019 US News ranking were located the U.S. Given this ratio, we found it justifiable to restrict our measure of institutional halo effects to elite universities in the U.S.
Thank you for this request. We have included information on countries and institutions in Supplementary file 2.
Thank you for raising this point. There is certainly a status differential between institutions included in the Leiden Ranking and those not included. However, there is also a concern that using institutions that are not internationally recognized, e.g. by being present on such a ranking, could create other sentiments, such as distrust. Our argument is that we measure a status differential because the institutions are “on the same scale”, since they can be found on these rankings, while if we had included those outside the rankings, we might end up measuring on two different scales. In this case, we decided to rely on the PP-top 10% indicator because it measures a university’s share of publications among the 10% most cited as opposed to the number of publications among the 10% most cited (i.e. P-top 10%). In the revised Materials and methods section, we reflect in more detail on these considerations.
Thank you for raising this important point. As an attempt to address this issue, we have computed the distribution of the average abstract ratings across universities, per discipline. As shown in Supplementary table 49 in Supplementary file 2, the within-group variations are quite modest. We chose to operate with the three categories to ensure reasonable statistical power in the analysis. As shown in Supplementary table 49 in Supplementary file 2 the Ns for individual universities are too small for any meaningful statistical comparison.
6) Effect sizes The authors find that effect of manipulations on abstract quality ratings is likely not larger than the band -0.8 to 0.8 (16 pt scale) (Results). That does seem small, although in Cohen's d terms, d=-0.3 to 0.3 isn't all that small. Overall, we would expect effects of a light-weight manipulation in an online survey context to be tiny, but it's really hard to generalize such interventions to the field, where status effects may be large and subtle. So I'd like a little more language emphasizing low-ish (but informative) external validity here, and that the prior on light weight manipulation is very small effects.
Thank you for pointing this out. We now reflect on this issue in the first paragraph of the Discussion section.
This is an interesting point. However, we are not entirely sure how such a control measure would be operationalized in practice? As we state in the Materials and methods section, “We carried out manual checks to ensure that all of the selected universities had active research environments within the relevant research disciplines.” Moreover, our selection of high-status institutions were all ranked highly in the U.S. National Research Council’s discipline-specific rankings of doctorate programs.
This is a great point. In the updated Materials and methods section, we outline our reasons for targeting these particular disciplines.
We completely agree. We designed the two dichotomous outcome items to capture additional types of evaluative situations. While the two dichotomous outcomes may not be comparable, we find both of them suitable for the purposes of our experiment. We have revised our discussion of these outcomes in accordance with your suggestions (please see the paragraph on scope conditions).
This is an excellent point. In the revised Introduction, we have limited our focus to studies that relate directly to the question of halo effects.
11) Situating this work in the existing literature. I think the part of the literature review section that tries to distinguish this contribution from others isn't entirely fair. Here are the distinguishing points, followed by my comments on them: a) Separating out location status vs. institution status Comment: Why would this separating out really matter? Surely status of country is highly correlated with status of institution, and, presumably, the mechanism at work are inferences of quality from status. It seems fine to me to separate them out, but would be good to have an argument for why this is valuable to do b) Previous RCTs in the peer review context may have been hampered by treatment leakage to the control group. Comment: This surely happens ( https://arxiv.org/abs/1709.01609 ). But it just means the reported effects in those RCTs are lower bounds, as those papers I believe readily admit. So I don't think this has been a major methodological problem in dire need of fixing. So I don't think what this experiment is doing is super novel and fixes glaring oversights in the literature. BUT, that isn't a bad thing, in my view. Simply adding observations, particularly those that are well executed, adequately powered, and cross-disciplinary, is important enough. It's an important topic, so we should have more than just one or two RCTs in it. Crucially, we know there are file-drawer effects out there, likely in this space too, so this study is useful for making sure the published record is unbiased.
Thank you for raising these important points. We have revised the last part of the Introduction in accordance with your suggestions.
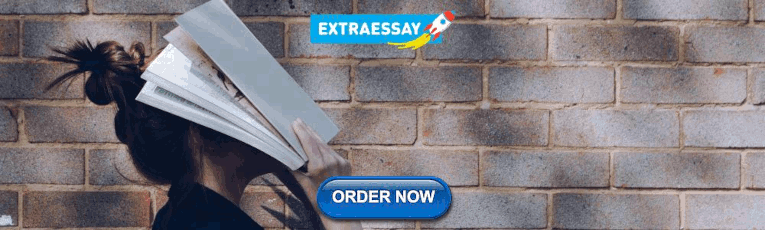
COMMENTS
Risk-of-bias assessment is a central component of systematic reviews but little conclusive empirical evidence exists on the validity of such assessments. In the context of such uncertainty, we present pragmatic recommendations that can be applied consistently across review topics, promote transparency and reproducibility in processes, and address methodological advances in the risk-of-bias ...
Channeling bias is a type of selection bias noted in observational studies. It occurs most frequently when patient characteristics, such as age or severity of illness, affect cohort assignment. This can occur, for example, in surgical studies where different interventions carry different levels of risk.
Writing a literature review requires a range of skills to gather, sort, evaluate and summarise peer-reviewed published data into a relevant and informative unbiased narrative. Digital access to research papers, academic texts, review articles, reference databases and public data sets are all sources of information that are available to enrich ...
Various types of bias and confounding have been described in the biomedical literature that can affect a study before, during, or after the intervention has been delivered. The peer review process can also introduce bias. A compelling ethical and moral rationale necessitates improving the peer review process. A double-blind peer review system is supported on equipoise and fair-play principles.
Strategies to identify and mitigate publication bias and outcome reporting bias are frequently adopted in systematic reviews of clinical interventions but it is not clear how often these are applied in systematic reviews relating to quantitative health services and delivery research (HSDR). We examined whether these biases are mentioned and/or otherwise assessed in HSDR systematic reviews, and ...
A P value, or the magnitude or direction of results can influence decisions about whether, when, and how research findings are disseminated. Regardless of whether an entire study or a particular study result is unavailable because investigators considered the results to be unfavorable, bias in a meta-analysis may occur when available results differ systematically from missing results.
Implicit biases involve associations outside conscious awareness that lead to a negative evaluation of a person on the basis of irrelevant characteristics such as race or gender. This review examines the evidence that healthcare professionals display implicit biases towards patients. PubMed, PsychINFO, PsychARTICLE and CINAHL were searched for peer-reviewed articles published between 1st March ...
The purpose of this review is to clarify the concepts of bias, precision and accuracy as they are commonly defined in the biostatistical literature, ... We finish with a literature review of promising new research related to species richness estimation, and summarize the results of 14 studies that compared estimator performance, which confirm ...
Introduction. Bias, perhaps best described as 'any process at any stage of inference which tends to produce results or conclusions that differ systematically from the truth,' can pollute the entire spectrum of research, including its design, analysis, interpretation and reporting. 1 It can taint entire bodies of research as much as it can ...
Physician bias refers to the unconscious negative perceptions that physicians have of patients or their conditions. Medical schools and residency programs often incorporate training to reduce biases among their trainees. In order to assess trends and organize available literature, we conducted a scoping review with a goal to categorize different biases that are studied within medical student ...
Given the heterogenous nature of the judgment and decision-making literature in nursing, and the lack of a clear body of work focused on how cognitive and implicit bias influences nurses' clinical judgment, a scoping review was selected as appropriate to map (Munn et al., 2018) the existing literature while not restricting included articles to ...
Bias, as used in this paper, refers to the tendency towards a particular characteristic or behavior, and thus, a biased AI system is one that shows biased associations entities. In this literature review, we examine the current state of research on AI bias, including its sources, as well as the methods for measuring, benchmarking, and ...
Again, to avoid publication bias, researchers should also attempt to obtain unpublished data and gray literature and conduct a quality assessment analysis. Assessment and reporting on the heterogeneity of the included studies, both clinical and methodological, and exploration of potential sources of variations are crucial for future researchers.
This is a carefully designed study and a well-written paper about bias in peer review based on authors' institutional and country location. The survey design is novel and the results seem robust. The main results show weak evidence for the claim that researchers are biased towards high-status countries and institutions.