Thank you for visiting nature.com. You are using a browser version with limited support for CSS. To obtain the best experience, we recommend you use a more up to date browser (or turn off compatibility mode in Internet Explorer). In the meantime, to ensure continued support, we are displaying the site without styles and JavaScript.
- View all journals
Collection
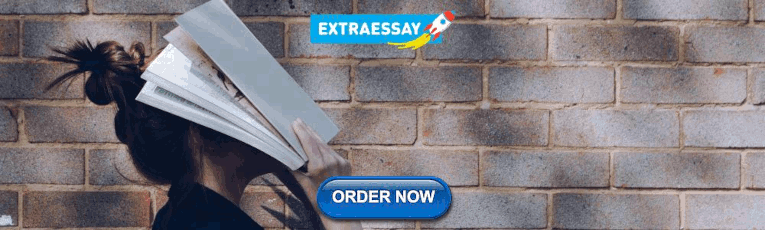
Clinical research in neurological disorders
Our knowledge of the pathological mechanisms driving neurodegeneration in disorders like Alzheimer’s disease and Parkinson’s disease is ever increasing. However, advances in diagnostics and disease modifying therapeutics are lagging.
The editors at Nature Communications , Communications Medicine , npj Parkinson’s Disease and Scientific Reports invite original research articles on the clinical aspects of neurological disorders and neurodegenerative diseases. This call for papers includes topics such as: biomarker discovery; approaches for more accurate diagnostics; assessment of clinical heterogeneity and in more diverse cohorts; clinical trials, both observational and interventional, as well as case studies. Preclinical work would not be within scope for this collection.
This is a joint Collection across Nature Communications, Communications Medicine , npj Parkinson’s Diseas e and Scientific Reports . Please see the relevant journal webpages to check which article types the journals consider. Please note, Nature Communications and Scientific Reports will only consider original research articles, npj Parkinson’s Disease welcomes original articles, reviews, perspectives and comments with a Parkinson’s disease focus, and Communications Medicine welcomes original articles, reviews, perspectives and comments across the whole scope of the collection.
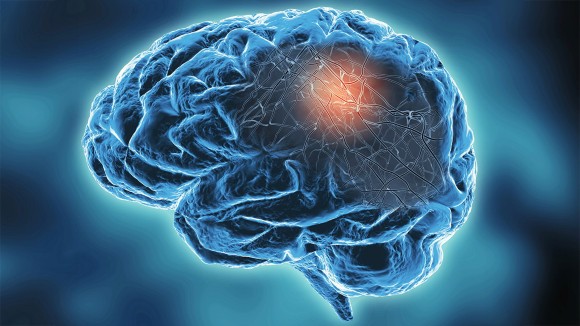
Nature Communications
Editorial Team
npj Parkinson's Disease
Associate Editors
Communications Medicine
Hideki mochizuki.
Osaka University in Osaka, Japan
Antonio Suppa
Sapienza University of Rome, Italy
- Collection content
- Participating journals
- About the Editors
- About this Collection
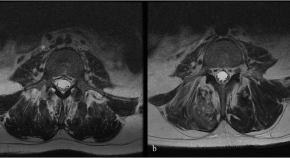
Intrathecal delivery of adipose-derived mesenchymal stem cells in traumatic spinal cord injury: Phase I trial
In the CELLTOP Phase I trial, stem cells were harvested from patients with spinal cord injury and injected into their central nervous system after processing. The procedure was safe, with no reported serious adverse events during the 2-year follow-up period.
- Mohamad Bydon
- Allan B. Dietz
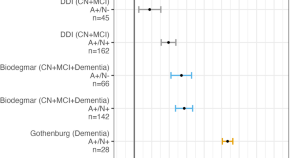
Plasma brain-derived tau is an amyloid-associated neurodegeneration biomarker in Alzheimer’s disease
The authors investigated associations of brain-derived-tau (BD-tau) with Aβ pathology, changes in cognition and MRI signatures. Staging Aβ-pathology according to neurodegeneration, using BD-tau, identifies individuals at risk of near-term cognitive decline and atrophy.
- Fernando Gonzalez-Ortiz
- Bjørn-Eivind Kirsebom
- Kaj Blennow
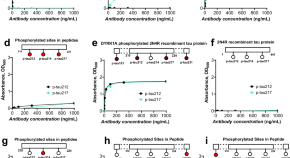
Plasma p-tau212 antemortem diagnostic performance and prediction of autopsy verification of Alzheimer’s disease neuropathology
A range of blood-based biomarkers have shown high specificity for Alzheimer’s disease (AD) pathophysiology with phosphorylated-tau (p-tau) being the most promising test. Here, the authors show the utility of plasma p-tau212 in autopsy-confirmed AD and memory clinic patient cohorts.
- Przemysław R. Kac
- Fernando González-Ortiz
- Thomas K. Karikari
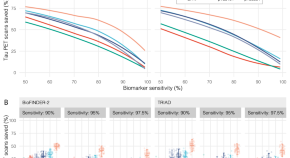
A blood-based biomarker workflow for optimal tau-PET referral in memory clinic settings
A screening strategy with plasma p-tau217, evaluated in two independent cohorts from Sweden and Canada, showed that this biomarker may effectively streamline tau-PET referrals in memory clinic settings, optimizing the prognostic work-up of Alzheimer’s disease.
- Wagner S. Brum
- Nicholas C. Cullen
- Oskar Hansson
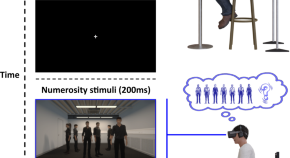
Numerosity estimation of virtual humans as a digital-robotic marker for hallucinations in Parkinson’s disease
Virtual reality, robotics and digital online technologies reveal heightened visual overestimation when estimating the number of humans, indexing presence hallucinations in healthy participants and patients with Parkinson’s disease.
- Louis Albert
- Jevita Potheegadoo
- Olaf Blanke
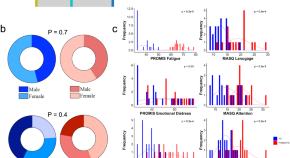
Deep phenotyping of post-infectious myalgic encephalomyelitis/chronic fatigue syndrome
Post-infectious myalgic encephalomyelitis/chronic fatigue syndrome (PI-ME/CFS) is a disabling disorder, yet the clinical phenotype is poorly defined and the pathophysiology unknown. Here, the authors conduct deep phenotyping of a cohort of PI-ME/CFS patients.
- Brian Walitt
- Komudi Singh
- Avindra Nath
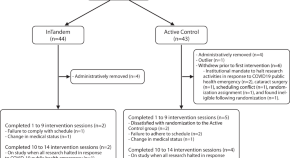
Efficacy and safety of using auditory-motor entrainment to improve walking after stroke: a multi-site randomized controlled trial of InTandem TM
Post-stroke walking impairment is a significant public health concern. Here, the authors perform an interventional, randomized controlled trial evaluating the efficacy and safety of InTandem™, an autonomous neurorehabilitation system utilizing auditory-motor entrainment to improve walking after stroke.
- Louis N. Awad
- Arun Jayaraman
- Sabrina R. Taylor
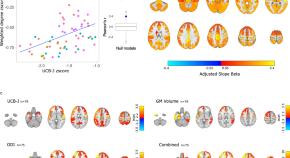
Synaptic density affects clinical severity via network dysfunction in syndromes associated with frontotemporal lobar degeneration
Translational neurodegeneration needs characterisation of the downstream consequences of synaptic loss. A multimodal imaging approach reveals that synaptic loss affects clinical severity via reduced connectivity in frontotemporal lobar degeneration.
- David J. Whiteside
- Negin Holland
- James B. Rowe
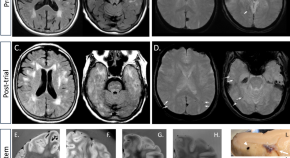
Fatal iatrogenic cerebral β-amyloid-related arteritis in a woman treated with lecanemab for Alzheimer’s disease
A 79-year-old woman received three doses of lecanemab, an experimental drug for Alzheimer’s disease, and suffered a seizure and cerebral edema. Neuropathological evaluation showed severe cerebral amyloid angiopathy, arteritis and microhemorrhages.
- Elena Solopova
- Wilber Romero-Fernandez
- Matthew Schrag
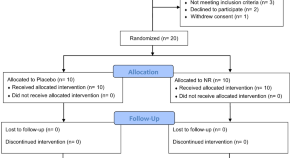
NR-SAFE: a randomized, double-blind safety trial of high dose nicotinamide riboside in Parkinson’s disease
Oral nicotinamide riboside (NR) at a dose of 3000 mg daily for 30 days is safe and associated with a pronounced systemic augmentation of the NAD metabolome, but no methyl donor depletion.
- Haakon Berven
- Simon Kverneng
- Charalampos Tzoulis
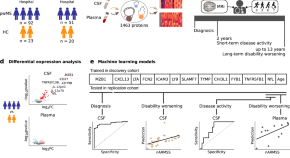
Proteomics reveal biomarkers for diagnosis, disease activity and long-term disability outcomes in multiple sclerosis
Precise biomarkers for multiple sclerosis prognosis are vital for treatment decisions. Here, the authors identify specific proteins in cerebrospinal fluid that can predict short-term disease activity and long-term disability outcomes in persons with multiple sclerosis.
- Julia Åkesson
- Sara Hojjati
- Mika Gustafsson
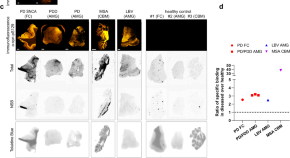
The α-synuclein PET tracer [18F] ACI-12589 distinguishes multiple system atrophy from other neurodegenerative diseases
A PET tracer for α-synuclein would help diagnosis and treatment of α-syn-related diseases. Here the authors show that ACI-12589 shows an uptake in the cerebellar white matter in patients with multiple-system atrophy.
- Ruben Smith
- Francesca Capotosti
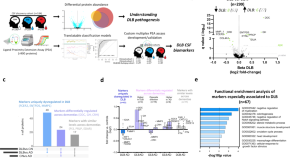
CSF proteome profiling reveals biomarkers to discriminate dementia with Lewy bodies from Alzheimer´s disease
This study characterizes the CSF proteome changes underlying Dementia with Lewy Bodies (DLB) and identifies pathophysiological and diagnostic leads associated to this cause of dementia. Findings have been translated into a biomarker panel that could identify DLB patients with high accuracy across different cohorts.
- Marta del Campo
- Lisa Vermunt
- Charlotte E. Teunissen
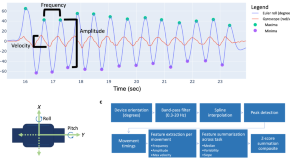
Improved measurement of disease progression in people living with early Parkinson’s disease using digital health technologies
Czech et al. develop and clinically validate a sensor-based approach to measure upper and lower body bradykinesia in an early Parkinson’s disease population. Results demonstrate enhanced sensitivity of sensor-based digital measurements to disease progression over one year relative to current clinical measurement standards.
- Matthew D. Czech
- Darryl Badley
- Josh D. Cosman
npj Parkinson’s disease
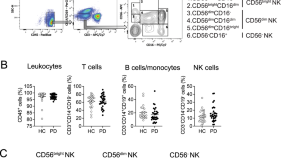
Distinctive CD56 dim NK subset profiles and increased NKG2D expression in blood NK cells of Parkinson’s disease patients
- Stephen Weber
- Kelly B. Menees
- Jae-Kyung Lee
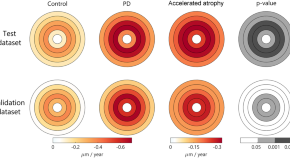
Association of retinal neurodegeneration with the progression of cognitive decline in Parkinson’s disease
- Ane Murueta-Goyena
- David Romero-Bascones
- Iñigo Gabilondo
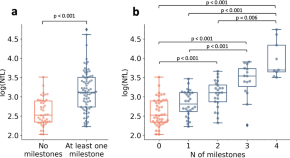
Serum neurofilament indicates accelerated neurodegeneration and predicts mortality in late-stage Parkinson’s disease
- Anika Frank
- Jonas Bendig
- Björn H. Falkenburger
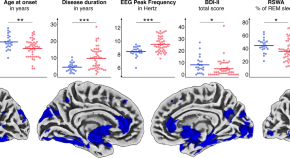
Clinical subtypes in patients with isolated REM sleep behaviour disorder
- Aline Seger
- Michael Sommerauer
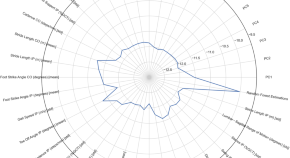
Identification of motor progression in Parkinson’s disease using wearable sensors and machine learning
- Charalampos Sotirakis
- Chrystalina A. Antoniades
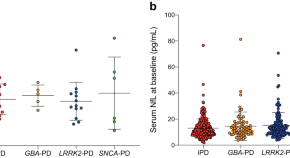
Neurofilament light chain as a mediator between LRRK2 mutation and dementia in Parkinson’s disease
- Guangyong Chen
Scientific Reports
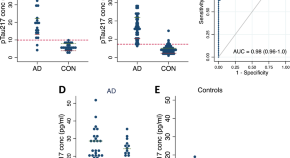
Clinical evaluation of a novel plasma pTau217 electrochemiluminescence immunoassay in Alzheimer’s disease
- Pia Kivisäkk
- Hadia A. Fatima
- Steven E. Arnold
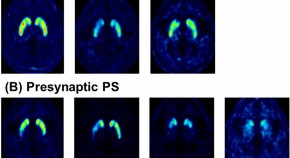
Diagnostic accuracy of 18 F-FP-CIT PET for clinically uncertain Parkinsonian syndrome
- Minyoung Oh
- Seung Jun Oh
- Jae Seung Kim
Quick links
- Explore articles by subject
- Guide to authors
- Editorial policies

- Search by keyword
- Search by citation
Page 1 of 7
Characteristics associated with occurrence of stroke in patients with infective endocarditis – a retrospective cohort study
Stroke is a severe complication of infective endocarditis (IE), associated with high rates of mortality. Data on how IE patients with and without stroke differ may help to improve understanding contributing me...
- View Full Text
Guillain-barré syndrome (GBS) with antecedent chikungunya infection: a case report and literature review
Guillain-Barré Syndrome (GBS) is an autoimmune neuropathy. Antecedent infections have been seen to be significant triggering factors for developing GBS. Among them, arboviral infections are rapidly gaining imp...
Status epilepticus in patients with brain tumors and metastases: A multicenter cohort study of 208 patients and literature review
Brain tumors and metastases account for approximately 10% of all status epilepticus (SE) cases. This study described the clinical characteristics, treatment, and short- and long-term outcomes of this population.
First seizure in elderly patients: Need to treat? Evidence from a retrospective study
The risk of seizure recurrence after a first unprovoked epileptic seizure is reported to be approximately 40%. Little is known about the recurrence risk after a first seizure in elderly patients, who may be at...
Brivaracetam and topiramate serum levels during pregnancy and delivery: a case report and a review of literature
An increasing use of newer antiseizure medication (ASM) such as SV2A ligand brivaracetam is observed. However, data on newer antiseizure medication and therapeutic drug monitoring during pregnancy is scarce.
Randomized controlled double-blind trial of methylprednisolone versus placebo in patients with post-COVID-19 syndrome and cognitive deficits: study protocol of the post-corona-virus immune treatment (PoCoVIT) trial
Post-COVID-19 Syndrome (PCS) includes neurological manifestations, especially fatigue and cognitive deficits. Immune dysregulation, autoimmunity, endothelial dysfunction, viral persistence, and viral reactivat...
Cognitive disorders in advanced Parkinson’s disease: challenges in the diagnosis of delirium
Parkinson’s disease (PD) is a neurodegenerative condition that is frequently associated with cognitive disorders. These can arise directly from the primary disease, or be triggered by external factors in susce...
Trends in stroke severity at hospital admission and rehabilitation discharge before and during the COVID-19 pandemic in Hesse, Germany: a register-based study
The COVID-19 pandemic has affected acute stroke care, resulting in a decrease in stroke admissions worldwide. We examined trends in stroke severity at hospital admission, including (1) probable need for rehabi...
Prospective study validating a multidimensional treatment decision score predicting the 24-month outcome in untreated patients with clinically isolated syndrome and early relapsing–remitting multiple sclerosis, the ProVal-MS study
In Multiple Sclerosis (MS), patients´ characteristics and (bio)markers that reliably predict the individual disease prognosis at disease onset are lacking. Cohort studies allow a close follow-up of MS historie...
Repetitive head injuries in German American football players do not change blood-based biomarker candidates for CTE during a single season
Repetitive traumatic brain injuries in American football players (AFPs) can lead to the neurodegenerative disease chronic traumatic encephalopathy (CTE). Clinical symptoms of CTE range from mood and behavioral...
Macrophage inclusions in cerebrospinal fluid following treatment initiation with antisense oligonucleotide therapies in motor neuron diseases
5q-associated spinal muscular atrophy (SMA) and amyotrophic lateral sclerosis (ALS) are two distinct neurological disorders leading to degeneration of lower motor neurons. The antisense oligonucleotides (ASOs)...
Fenfluramine for the treatment of status epilepticus: use in an adult with Lennox–Gastaut syndrome and literature review
Novel treatments are needed to control refractory status epilepticus (SE). This study aimed to assess the potential effectiveness of fenfluramine (FFA) as an acute treatment option for SE. We present a summary...
Creative thinking and cognitive estimation in Parkinson’s disease
Patients with Parkinson’s disease (PD) have been reported to exhibit unusual bouts of creativity (e.g., painting, writing), in particular in the context of treatment with dopaminergic agents. Here we investiga...
Preoperative motor deficits and depressive symptoms predict quality of life in patients with Parkinson’s disease at different time points after surgery for subthalamic stimulation: a retrospective study
While subthalamic nucleus deep brain stimulation (STN-DBS) improves the quality of life (QoL) of patients with Parkinson’s disease (PD), the clinical parameters that predict this improvement remain debated. Th...
Effects of transsectoral long-term neurorehabilitation
Acquired brain injuries are among the most common causes of disability in adulthood. An intensive rehabilitation phase is crucial for recovery. However, there is a lack of concepts to further expand the therap...
Functional long-term outcome following endovascular thrombectomy in patients with acute ischemic stroke
Endovascular thrombectomy (EVT) is the most effective treatment for acute ischemic stroke caused by large vessel occlusion (LVO). Yet, long-term outcome (LTO) and health-related quality of life (HRQoL) in thes...
Reevaluating the relevance of 18 F-FDG PET findings for diagnosis of neurosarcoidosis: a case series
The diagnosis of neurosarcoidosis (NS) remains challenging due to the difficulty to obtain central nervous system (CNS) biopsies. Various diagnostic parameters are considered for the definition of possible, pr...
Determination of brain death using 99m Tc-HMPAO scintigraphy and transcranial duplex sonography in a patient on veno-arterial ECMO
Management of status epilepticus in pregnancy: a clinician survey.
Status epilepticus in pregnancy (SEP) is rare and life-threatening for both mother and fetus. There are well-established guidelines for the management of women with epilepsy during pregnancy; however, there is...
Interdisciplinary network care collaboration in Parkinson’s disease: a baseline evaluation in Germany
The strengthening of interdisciplinary care collaboration in Parkinson's disease is taking on increasing importance in daily medical routine. Therefore, care providers worldwide are organizing themselves in di...
The evolution of acute stroke care in Germany from 2019 to 2021: analysis of nation-wide administrative datasets
The treatment of ischemic stroke (IS) has changed considerably in recent years. Particularly the advent of mechanical thrombectomy (MTE) has revolutionized the available treatment options. Most patients in dev...
Neuropathological hints from CSF and serum biomarkers in corticobasal syndrome (CBS): a systematic review
Corticobasal syndrome (CBS) is a clinical syndrome determined by various underlying neurodegenerative disorders requiring a pathological assessment for a definitive diagnosis. A literature review was performed...
Temporary and highly variable recovery of neuromuscular dysfunction by electrical stimulation in the follow-up of acute critical illness neuromyopathy: a pilot study
In sepsis-associated critical illness neuromyopathy (CIPNM) serial electrical stimulation of motor nerves induces a short-lived temporary recovery of compound muscle action potentials (CMAPs) termed facilitati...
Outcome of endovascular stroke therapy in a large mandatory stroke-registry
Endovascular stroke treatment (EST) has become the standard treatment for patients with stroke due to large vessel occlusion, especially in earlier time windows. Only few data from population-based registries ...
The impact of referring patients with drug-resistant focal epilepsy to an epilepsy center for presurgical diagnosis
Epilepsy surgery is an established treatment for drug-resistant focal epilepsy (DRFE) that results in seizure freedom in about 60% of patients. Correctly identifying an epileptogenic lesion in magnetic resonan...
Rendezvous intervention using combined surgical carotid endarterectomy followed by endovascular thrombectomy in patients with acute tandem occlusions: a proof-of-concept experience at a tertiary care center
Endovascular thrombectomy (EVT) is highly effective in acute stroke patients with intracranial large vessel occlusion (LVO), however, presence of concomitant cervical occlusion of the internal carotid artery (...
Validation of a German-language modified Rankin Scale structured telephone interview at 3 months in a real-life stroke cohort
The modified Rankin scale (mRS) at 3 months is established as the primary outcome measure in clinical stroke trials. Traditionally, the mRS is assessed through an unstructured face-to-face interview. This appr...
Differential diagnosis of chorea (guidelines of the German Neurological Society)
Choreiform movement disorders are characterized by involuntary, rapid, irregular, and unpredictable movements of the limbs, face, neck, and trunk. These movements often initially go unnoticed by the affected i...
Symptomatic treatment options for Huntington’s disease (guidelines of the German Neurological Society)
Ameliorating symptoms and signs of Huntington’s disease (HD) is essential to care but can be challenging and hard to achieve. The pharmacological treatment of motor signs (e.g. chorea) may favorably or unfavor...
Long-term functional outcome and quality of life 2.5 years after thrombolysis in acute ischemic stroke
Evaluation of outcome after stroke is largely based on assessment of gross function 3 months after stroke onset using scales such as mRS. Cognitive or social functions, level of symptom burden or emotional hea...
Correction: Guideline “Transient Global Amnesia (TGA)” of the German Society of Neurology (Deutsche Gesellschaft für Neurologie): S1-guideline
The original article was published in Neurological Research and Practice 2023 5 :15
Outcome analysis for patients with subarachnoid hemorrhage and vasospasm including endovascular treatment
As a complication of subarachnoid hemorrhage (SAH), vasospasm substantially contributes to its morbidity and mortality. We aimed at analyzing predictors of outcome for these patients including the role of endo...

Maternal immunoglobulin treatment can reduce severity of fetal acetylcholine receptor antibody-associated disorders (FARAD)
Fetal acetylcholine receptor antibody-associated disorders (FARAD), caused by in utero exposure to maternal antibodies directed against the fetal acetylcholine receptor (AChR), is a rare condition occurring in...
INTERCEPT H3: a multicenter phase I peptide vaccine trial for the treatment of H3-mutated diffuse midline gliomas
Diffuse midline gliomas (DMG) are universally lethal central nervous system tumors that carry almost unanimously the clonal driver mutation histone-3 K27M (H3K27M). The single amino acid substitution of lysine...
The role of creatine kinase in distinguishing generalized tonic–clonic seizures from psychogenic non-epileptic seizures (PNES) and syncope: a retrospective study and meta-analysis of 1300 patients
As the clinical differentiation between epileptic seizures, psychogenic non-epileptic seizures (PNES), and syncope depends mainly on a detailed report of the event, which may not be available, an objective ass...
Frequency and satisfaction of conventional and complementary or alternative therapies for neuromuscular disorders
Causal therapies are not yet available for most neuromuscular diseases. Additionally, data on the use of complementary or alternative therapies (CAM) in patients groups with a variety of different neuromuscula...
Independent external validation of a stroke recurrence score in patients with embolic stroke of undetermined source
Embolic stroke of undetermined source (ESUS) accounts for a substantial proportion of ischaemic strokes. A stroke recurrence score has been shown to predict the risk of recurrent stroke in patients with ESUS b...
No evidence for neuronal damage or astrocytic activation in cerebrospinal fluid of Neuro-COVID-19 patients with long-term persistent headache
Headache is one of the most common neurological manifestations of COVID-19, but it is unclear whether chronic headache as a symptom of Post-COVID-19 is associated with ongoing CNS damage. We compared cerebrosp...
Video-EEG monitoring as a valuable tool for antiseizure medication withdrawal in patients with epilepsy: implications for clinical practice and public health policies
This letter to the editor discusses “the use of video-EEG monitoring to guide antiseizure medication (ASM) withdrawal in patients with epilepsy” [ 1 ]. The author highlights the potential benefits of this approach,...
The original article was published in Neurological Research and Practice 2023 5 :20
Reply to: Camptocormia due to myotinilopathy, Parkinson’s disease, or both?
The original article was published in Neurological Research and Practice 2023 5 :45
Facial nerve neurographies in intensive care unit-acquired weakness
Patients with an intensive care unit-acquired weakness (ICU-AW) often present clinically with severe paresis of the limb and trunk muscles while facial muscles appear less affected. To investigate whether the ...
Resting state EEG as biomarker of cognitive training and physical activity’s joint effect in Parkinson’s patients with mild cognitive impairment
Cognitive decline is a major factor for the deterioration of the quality of life in patients suffering from Parkinson’s disease (PD). Recently, it was reported that cognitive training (CT) in PD patients with ...
Camptocormia due to myotinilopathy, Parkinson’s disease, or both?
The original article was published in Neurological Research and Practice 2023 5 :26
The Comment to this article has been published in Neurological Research and Practice 2023 5 :54
Evolution of neurodegeneration in patients with normal pressure hydrocephalus: a monocentric follow up study
The aim of this study was to examine in patients with idiopathic and neurodegenerative normal pressure hydrocephalus (NPH) if motor and cognitive performance as well as changes in biomarkers in cerebrospinal f...
Can ChatGPT explain it? Use of artificial intelligence in multiple sclerosis communication
German guidelines on community-acquired acute bacterial meningitis in adults.
The incidence of community-acquired acute bacterial meningitis has decreased during the last decades. However, outcome remains poor with a significant proportion of patients not surviving and up to 50% of surv...
Introducing electronic monitoring of disease activity in patients with chronic inflammatory demyelinating polyneuropathy (EMDA CIDP): trial protocol of a proof of concept study
Chronic inflammatory demyelinating polyneuropathy (CIDP) is one of the most common immune neuropathies leading to severe impairments in daily life. Current treatment options include intravenous immunoglobulins...
Differential effects of gender and age on dynamic subjective visual vertical
In a retrospective study, the data of direction-dependent deviations in dynamic subjective visual vertical (SVV) testing were analysed in 1811 dizzy patients (174 benign paroxysmal positional vertigo, 99 unilater...
Effects of body mass index on the immune response within the first days after major stroke in humans
Immunological alterations associated with increased susceptibility to infection are an essential aspect of stroke pathophysiology. Several immunological functions of adipose tissue are altered by obesity and a...
A familial missense ACTA2 variant p.Arg198Cys leading to Moyamoya-like arteriopathy with straight course of the intracranial arteries, aortic aneurysm and lethal aortic dissection
Cerebral vasculopathies frequently lead to severe medical conditions such as stroke or intracranial hemorrhage and have a broad range of possible etiologies that require different therapeutic regimens. However...
- Editorial Board
- Instructions for Editors
- Sign up for article alerts and news from this journal
Annual Journal Metrics
2022 Citation Impact 1.397 - SNIP (Source Normalized Impact per Paper) 2023 Speed 9 days submission to first editorial decision for all manuscripts (Median) 61 days submission to accept (Median) 2023 Usage 781,593 downloads 260 Altmetric mentions
Affiliated with
Neurological Research and Practice is the official journal of the German Neurological Society ( Deutsche Gesellschaft für Neurologie e.V. ). To learn more, please visit the DGN landing page .
- Follow us on Twitter
Neurological Research and Practice
ISSN: 2524-3489
- Submission enquiries: Access here and click Contact Us
- General enquiries: [email protected]
- Open access
- Published: 12 June 2023
Neuroinflammation and Brain Disease
- A. Bersano 1 ,
- J. Engele 2 &
- M.K.E. Schäfer 3
BMC Neurology volume 23 , Article number: 227 ( 2023 ) Cite this article
1966 Accesses
3 Citations
1 Altmetric
Metrics details
Starting from the perspective of an immune-privileged site, our knowledge of the inflammatory processes within the central nervous system has increased rapidly over the last 30 years, leading to a rather puzzling picture today. Of particular interest is the emergence of disease- and injury-specific inflammatory responses within the brain, which may form the basis for future therapeutic approaches. To advance this important topic, we invite authors to contribute research and clinical papers to the Collection “Neuroinflammation and Brain Disease”.
Inflammation is a biological process that dynamically alters the surrounding microenvironment, including participating immune cells [ 1 ]. Surrounded by specialized barriers and with immune-specific properties, the central nervous system (CNS) tightly regulates immune responses [ 2 ]. In ‘neuroinflammatory’ conditions, pathogenic immunity can disrupt CNS structure and function [ 3 ]. Neuroinflammation has been observed as a key pathway in the onset and/or progression of several neurological disorders defined as inflammatory (e.g., multiple sclerosis, vasculitis, etc.), but also in neurological conditions not usually categorized as inflammatory, such as Alzheimer’s disease (AD), Parkinson’s disease, amyotrophic lateral sclerosis, stroke and traumatic brain injuries (TBI) [ 4 , 5 , 6 , 7 , 8 ].
The activation of glial cells and complement-mediated pathways, the synthesis of inflammation mediators, and the recruitment of leukocytes, are key elements of brain inflammation. Under the influence of exogenous and endogenous factors (e.g., trauma, stroke, chronic infections, disease-related proteins like amyloid-β (Aβ), tau/p-tau or α-synuclein), the activation of microglia triggers several signal transduction pathways, including phosphoinositide 3-kinase/protein kinase B (PI3K/AKT), mitogen-activated protein kinase (MAPK) and mammalian target of rapamycin (mTOR), leading to transcription factor nuclear factor kappa-light-chain-enhancer of activated B cells (NF-κB) activation ( 9 – 10 ). The subsequent production of pro-inflammatory cytokines, chemokines, inducible enzymes (e.g., inducible nitric oxide synthase -iNOS) and cyclooxygenase 2 (COX-2) drive neuroinflammation. Numerous studies have indeed documented the increased production of different cytokines, including interleukin-1β (IL-1β), IL-6, IL-18, IL-12, IL-23, IL-33 and tumor necrosis factor-α (TNF-α), in various neurological and neuropsychiatric disorders [ 11 ]. For example, high expression of IL-1β in microglia cells surrounding Aβ plaques was observed in AD patients [ 12 ]. Moreover, the neuroinflammation observed in neurological disorders has a pivotal role in exacerbating Aβ burden and tau hyperphosphorylation, suggesting that stimulating cytokines in response to an undesirable external response could be a checkpoint for treating neurological disorders.
It has become clear that inflammation also contributes to pathological, clinical and functional outcomes in the context of acquired brain injuries such as TBI and stroke [ 7 ]. It is noteworthy that acquired brain injuries represent a risk factor for the chronic neurodegenerative diseases mentioned above. Much research has focused on the role of brain-resident microglia, the primary immune cells in the CNS, and astrocytes, and how they either exacerbate inflammatory damage or help to maintain a healthy environment in the CNS. However, the duality of inflammatory reactions, often referred to as the “double-edged sword”, is still challenging and complicates the development of therapeutic options [ 13 , 14 ]. The underlying mechanisms of neuroinflammation are likely to involve multiple cell types and knowledge about their in vivo interactions remains elusive. This not only applies to brain-resident cells such as neurons, astrocytes, microglia, oligodendrocytes and neural progenitor cells, but also to the role of early infiltrating and possibly persisting peripheral immune cells, such as monocytes, macrophages, neutrophils, and T cells. Therefore, it is necessary to decipher the crosstalk between various cell types, identify differences and commonalities in molecular signaling pathways, and modulate critical signaling pathways, in order to gain a more complete knowledge to develop therapeutic strategies for treatment. This could become possible through the integration of network modeling approaches for multi-omics at the tissue and single-cell level ( 15 – 16 ).
Another level of complexity arises from crosstalk between the brain and other organs. Several studies have reported on reciprocal interactions between the injured or diseased brain with the gut microbiome and how therapeutic drugs may influence these interactions [ 17 , 18 , 19 , 20 ]. Moreover, organ dysfunction has been recognized to be bidirectional, meaning that dysfunction in one organ potentiates injury to others. Scientists are just beginning to understand how these processes trigger neuroinflammation. For example, TBI can negatively impact various organs, including the pulmonary, gastrointestinal, cardiovascular, renal, and immune systems [ 21 ]. Furthermore, it should also be considered that sex, age and comorbidities can strongly influence inflammatory responses in acute and chronic neurodegeneration ( 22 – 23 ). Finally, to translate results from bench to bedside, consistent improvement and application of diagnostic and prognostic tools, including functional neuroimaging, advanced magnetic resonance imaging processing and meaningful biomarkers [ 24 ] to characterize the timing, localization, extent, and duration of inflammation are clearly important. The identification of suitable biomarkers could be promoted, for example, by unified classification schemes to assess their clinical utility [ 25 – 26 ]. A better understanding of the role that inflammatory processes play in the natural history of diseases is essential to identify potential therapeutic targets and develop integrated pharmacological approaches acting at different levels and stages of disease. We hope that this collection will provide a useful platform for articles that address focused research questions on molecular and cellular mechanisms in the area of neuroinflammation and brain diseases, and also provide ideas for integrative organism-level approaches and perspectives on therapeutic options.
Data Availability
Abbreviations.
Central nervous system
Traumatic brain injury
Phosphoinositide 3-kinase/protein kinase B
Mitogen-activated protein kinase
Mammalian target of rapamycin
Nuclear factor kappa-light-chain-enhancer of activated B cells
Inducible nitric oxide synthase
Cyclooxygenase 2
Interleukin-1β
Interleukin-6
Interleukin-18
Interleukin-12
Interleukin-23
Interleukin-33
Tumor necrosis factor-α
Alzheimer's disease
Carson MJ, Thrash JC, Walter B. The cellular response in neuroinflammation: the role of leukocytes, microglia and astrocytes in neuronal death and survival. Clin Neurosci Res. 2009;6(5):237–45.
Article Google Scholar
Kanegawa N, Collste K, Forsberg A, Schain M, Arakawa R, Jucaite A, Lekander M, Olgart Höglund C, Kosek E, Lampa J, Halldin C, Farde L, Varrone A, Cervenka S. In vivo evidence of a functional association between immune cells in blood and brain in healthy human subjects. Brain Behav Immun. 2016;54:149–57. https://doi.org/10.1016/j.bbi.2016.01.019 .
Article CAS PubMed Google Scholar
Shabab T, Khanabdali R, Moghadamtousi SZ, Kadir HA, Mohan G. Neuroinflammation pathways: a general review. Int J Neurosci. 2017;127(7):624–33. https://doi.org/10.1080/00207454.2016.1212854 .
Heneka MT, Carson MJ, El Khoury J, Landreth GE, Brosseron F, Feinstein DL, Jacobs AH, Wyss-Coray T, Vitorica J, Ransohoff RM, Herrup K, Frautschy SA, Finsen B, Brown GC, Verkhratsky A, Yamanaka K, Koistinaho J, Latz E, Halle A, Petzold GC, Town T, Morgan D, Shinohara ML, Perry VH, Holmes C, Bazan NG, Brooks DJ, Hunot S, Joseph B, Deigendesch N, Garaschuk O, Boddeke E, Dinarello CA, Breitner JC, Cole GM, Golenbock DT, Kummer MP. Neuroinflammation in Alzheimer’s disease. Lancet Neurol. 2015;14(4):388–405.
Article CAS PubMed PubMed Central Google Scholar
Ransohoff RM. How neuroinflammation contributes to neurodegeneration. Science. 2016;353(6301):777–83.
Schain M, Kreisl WC. Neuroinflammation in neurodegenerative Disorders—a review. Curr Neurol Neurosci Rep. 2017;17:25. https://doi.org/10.1007/s11910-017-0733-2 .
Jayaraj RL, Azimullah S, Beiram R, Jalal FY, Rosenberg GA. Neuroinflammation: friend and foe for ischemic stroke. J Neuroinflamm. 2019;16(1):142. https://doi.org/10.1186/s12974-019-1516-2 .
Lim S, Chun Y, Lee JS, Lee SJ. Neuroinflammation in synucleinopathies. Brain Pathol. 2016;26(3):404–9.
Article PubMed PubMed Central Google Scholar
Cianciulli A, Porro C, Calvello R, Trotta T, Lofrumento DD, Panaro MA. Microglia mediated neuroinflammation: focus on PI3K modulation. Biomolecules. 2020;10(1):137.
Jadhav SP, Kamath SP, Choolani M, Lu J, Dheen ST. microRNA-200b modulates microglia‐mediated neuroinflammation via the cJun/MAPK pathway. J Neurochem. 2014;130(3):388–401.
Becher B, Spath S, Goverman J. Cytokine networks in neuroinflammation. Nat Rev Immunol. 2017;17(1):49–59.
Rivera-Escalera F, Pinney JJ, Owlett L, Ahmed H, Thakar J, Olschowka JA, Elliott MR, O’Banion MK. IL-1β-driven amyloid plaque clearance is associated with an expansion of transcriptionally reprogrammed microglia. J Neuroinflamm. 2019;16(1):261. https://doi.org/10.1186/s12974-019-1645-7 .
Article CAS Google Scholar
Jadhav P, Karande M, Sarkar A, Sahu S, Sarmah D, Datta A, Chaudhary A, Kalia K, Sharma A, Wang X, Bhattacharya P. Glial cells response in stroke. Cell Mol Neurobiol. 2023;43:99–113.
Blencowe M, Arneson D, Ding J, Chen YW, Saleem Z, Yang X. Network modeling of single-cell omics data: challenges, opportunities, and progresses. Emerg Top Life Sci. 2019;3:379–98.
Tuohy MC, Hillman EMC, Marshall R, Agalliu D. The age-dependent immune response to ischemic stroke. Curr Opin Neurobiol. 2023;78:102670.
Benakis C, Liesz A. The gut-brain axis in ischemic stroke: its relevance in pathology and as a therapeutic target. Neurol Res Pract. 2022;4:57.
Hanscom M, Loane DJ, Shea-Donohue T. Brain-gut axis dysfunction in the pathogenesis of traumatic brain injury. J Clin Invest. 2021;131(12):e143777.
Li X, Wang Q, Wu D, Zhang D-w, Li S-c, Zhang S-w, Chen X, Li W. The effect of a novel anticonvulsant chemical Q808 on gut microbiota and hippocampus neurotransmitters in pentylenetetrazole-induced seizures in rats. BMC Neurosci. 2022;23:7.
Yang C, Feng Z, Deng H, Dai L, He L, Yin L, Zhao J. CXCL1/CXCR2 is involved in white matter injury in neonatal rats via the gut–brain axis. BMC Neurosci. 2022;23:67.
Faden AI, Barrett JP, Stoica BA, Henry RJ. Bidirectional brain-systemic interactions and outcomes after TBI. Trends in Neuroscience. 2021;44:406–18.
Ramiro L, Faura J, Simats A, García-Rodríguez P, Ma F, Martín L, Canals F, Rosell A, Montaner J. Influence of sex, age and diabetes on brain transcriptome and proteome modifications following cerebral ischemia. BMC Neurosci. 2023;24:7.
Tariq MB, Lee J, McCullough LD. Sex differences in the inflammatory response to stroke. Semin Immunopathol. 2022. https://doi.org/10.1007/s00281-022-00969-x .
Irimia A, Wang B, Aylward SR, Prastawa MW, Pace DF, Gerig G, Hovda DA, Kikinis R, Vespa PM, Van Horn JD. Neuroimaging of structural pathology and connectomics in traumatic brain injury: toward personalized outcome prediction. Neuroimage: Clin. 2012;1:1–17.
Article PubMed Google Scholar
Bernhardt AM, Tiedt S, Teupser D, Dichgans M, Meyer B, Gempt J, Kuhn PH, Simons M, Palleis C, Weidinger E, Nübling G, Holdt L, Hönikl L, Gasperi C, Giesbertz P, Müller SA, Breimann S, Lichtenthaler SF, Kuster B, Mann M, Imhof A, Barth T, Hauck SM, Zetterberg H, Otto M, Weichert W, Hemmer B, Levin J. A unified classification approach rating clinical utility of protein biomarkers across neurologic diseases. EBioMedicine . 2023;89:104456 .
Download references
Acknowledgements
Author information, authors and affiliations.
Cerebrovascular Unit, Fondazione IRCCS Istituto Neurologico Carlo Besta Milan, Via Celoria 11, Milan, 20133, Italy
Institute of Anatomy, Leipzig University, Leipzig, Germany
Department of Anesthesiology, University Medical Center of the Johannes Gutenberg- University, Mainz, Germany
M.K.E. Schäfer
You can also search for this author in PubMed Google Scholar
Contributions
AB and MKES drafted the manuscript and revised it critically. JE critically revised the text.
Corresponding author
Correspondence to A. Bersano .
Ethics declarations
Ethics approval and consent to participate, consent for publication, competing interests.
The authors declare no competing interests.
Additional information
Publisher’s note.
Springer Nature remains neutral with regard to jurisdictional claims in published maps and institutional affiliations.
Rights and permissions
Open Access This article is licensed under a Creative Commons Attribution 4.0 International License, which permits use, sharing, adaptation, distribution and reproduction in any medium or format, as long as you give appropriate credit to the original author(s) and the source, provide a link to the Creative Commons licence, and indicate if changes were made. The images or other third party material in this article are included in the article’s Creative Commons licence, unless indicated otherwise in a credit line to the material. If material is not included in the article’s Creative Commons licence and your intended use is not permitted by statutory regulation or exceeds the permitted use, you will need to obtain permission directly from the copyright holder. To view a copy of this licence, visit http://creativecommons.org/licenses/by/4.0/ . The Creative Commons Public Domain Dedication waiver ( http://creativecommons.org/publicdomain/zero/1.0/ ) applies to the data made available in this article, unless otherwise stated in a credit line to the data.
Reprints and permissions
About this article
Cite this article.
Bersano, A., Engele, J. & Schäfer, M. Neuroinflammation and Brain Disease. BMC Neurol 23 , 227 (2023). https://doi.org/10.1186/s12883-023-03252-0
Download citation
Received : 23 April 2023
Accepted : 18 May 2023
Published : 12 June 2023
DOI : https://doi.org/10.1186/s12883-023-03252-0
Share this article
Anyone you share the following link with will be able to read this content:
Sorry, a shareable link is not currently available for this article.
Provided by the Springer Nature SharedIt content-sharing initiative
BMC Neurology
ISSN: 1471-2377
- General enquiries: [email protected]
Neurological Disorders
Book editors.
- PMID: 27227247
- Bookshelf ID: NBK361950
- DOI: 10.1596/978-1-4648-0426-7_ch5
Neurological disorders pose a large burden on worldwide health. The most recent estimates show that the neurological disorders included in the Global Burden of Disease (GBD) Study–Alzheimer’s and other dementias, Parkinson’s disease, multiple sclerosis, epilepsy, and headache disorders (migraine, tension-type headache [TTH], and medication-overuse headache [MOH])–represent 3 percent of the worldwide burden of disease. Although this is a seemingly small overall percentage, dementia, epilepsy, migraine, and stroke rank in the top 50 causes of disability-adjusted life years (DALYs) (Murray and others 2012).
Migraine and epilepsy represent one-third and one-fourth of this neurological burden, respectively (Murray and others 2012), and dementia and Parkinson’s disease are among the top 15 conditions with the most substantial increase in burden in the past decade. In 2010, neurological disorders constituted 5.5 percent of years lived with disability (YLDs), or 42.9 million YLDs; migraine, epilepsy, and dementia were among the top 25 causes of YLDs. Migraine leads the list of neurological disorders, representing more than 50 percent of neurological YLDs or 2.9 percent of global YLDs; epilepsy represents 1.1 percent of global YLDs (Vos and others 2012).
The neurological burden of disease is expected to grow exponentially in low- and middle-income countries (LMICs) in the next decade (Murray and others 2012). Despite the significant impact of neurological disorders on patients and societies, knowledge of their epidemiology, including variation in disease frequency across place and time and understanding of associated risk factors and outcomes, remains limited, particularly in LMICs. Patients with neurological disorders often require significant social and economic support because of physical, cognitive, and psychosocial limitations (WHO 2006). Despite the high prevalence of disability, there is increasing recognition that services and resources are disproportionately scarce, especially in LMICs (WHO 2004). In addition, knowledge of the cost-effectiveness of interventions to improve neurological care in these settings remains limited.
This chapter addresses three neurological disorders: epilepsy, dementia, and headache disorders. The chapter reviews current knowledge of the epidemiology, risk factors, and cost-effective interventions for these conditions. The focus is on interventions that provide meaningful reduction in the burden to the global population, with particular emphasis on applicability to LMICs. Neurological disorders are an emerging challenge to health care systems globally, requiring further study, government and social engagement, and improvements in health care infrastructure.
This chapter uses the World Health Organization (WHO) regions—African, the Americas, Eastern Mediterranean, European, South-East Asia, and Western Pacific—to describe the global burden of the highlighted neurological disorders.
© 2016 International Bank for Reconstruction and Development / The World Bank.
- Introduction
- Headache Disorders
- Conclusions and Recommendations
Publication types
Publish with Us
Mathematics & physics group.
International Journal of Physics Research and Applications
Chemistry Group
Annals of Advances in Chemistry
Pharma Group
Archives of Pharmacy and Pharmaceutical Sciences
Engineering Group
Annals of Biomedical Science and Engineering
Annals of Civil and Environmental Engineering
Biology Group
Annals of Proteomics and Bioinformatics
Archives of Biotechnology and Biomedicine
Insights in Biology and Medicine
Journal of Forensic Science and Research
Journal of Plant Science and Phytopathology
Clinical Group
Archives of Food and Nutritional Science
Archives of Asthma, Allergy and Immunology
Archives of Clinical and Experimental Orthopaedics
Annals of Dermatological Research
Journal of child, adult vaccines and immunology.
International Journal of Bone Marrow Research
International Journal of Clinical Microbiology and Biochemical Technology
Archives of Cancer Science and Therapy
International journal of clinical virology, journal of advanced pediatrics and child health.
Clinical Journal of Nursing Care and Practice
New Insights in Obesity: Genetics and Beyond
Journal of Pulmonology and Respiratory Research
Annals of Clinical Gastroenterology and Hepatology
Advanced Treatments in ENT Disorders
Insights in Clinical and Cellular Immunology
Journal of Hematology and Clinical Research
Journal of Clinical Advances in Dentistry
International Journal of Clinical Anesthesia and Research
Archives of Pathology and Clinical Research
Insights on the Depression and Anxiety
Journal of Clinical Intensive Care and Medicine
Annals of Clinical Hypertension
Journal of Stem Cell Therapy and Transplantation
Heighpubs Otolaryngology and Rhinology
Journal of Clinical, Medical and Experimental Images
Journal of Oral Health and Craniofacial Science
Clinical Journal of Obstetrics and Gynecology
Journal of Neuroscience and Neurological Disorders
International Journal of Clinical and Experimental Ophthalmology
Archives of Surgery and Clinical Research
Journal of Clinical Nephrology
Insights in veterinary science.
Journal of Radiology and Oncology
Medical Group
Journal of Community Medicine and Health Solutions
Archives of Vascular Medicine
Journal of Genetic Medicine and Gene Therapy
Archives of Psychiatry and Mental Health
Journal of Addiction Therapy and Research
Annals of Clinical Endocrinology and Metabolism
Archives of Case Reports
Journal of Novel Physiotherapy and Rehabilitation
Journal of Cardiology and Cardiovascular Medicine
Journal of Sports Medicine and Therapy
Share by HSPI

- Hybridizing intra and extra perspectives in infectious disease modeling -Ruffin-Benoît M Ngoie
- Status of hemodialysis patients using complementary and alternative medicine practices during the COVID-19 pandemic... -Sevil Güler
- Obesity may contribute to Japanese COVID-19 death rate -Tetsuo Fukawa
- Interferons as a Potential Therapeutic Drug for COVID-19: A Literature Review of Mechanisms, Current Clinical Trials, and Challenges... -Pramod Yadav*
- Fatal acute necrotizing pancreatitis in a 15 years old boy, is it multisystem inflammatory syndrome in children associated with COVID-19; MIS-C?... -Masoumeh Asgarshirazi
Laws of Pathophysiology of Migraine in the Third Millennium
- Review Article
- 20th March 2024
Chemotherapy-induced Peripheral Neuropathy: A Mini-review of Current & Developmental Treatments
- Mini Review
- 22nd February 2024
A Water-soluble Form of Dihydroquercetin Reduces LPS-induced Astrogliosis, Vascular Remodeling, and mRNA VEGF-A Levels in the Substantia Nigra of Aged Rats
- Research Article
- 16th February 2024
Biomarkers for High Metabolic Burden in Neurologic Disease
- Short Communication
- 15th February 2024
A Strength-based Approach to Achieving Academic Success for Individuals with Autism Spectrum Disorder (ASD)
- 14th February 2024
Autoimmune Diseases Associated with Iron Deficiency Anemia; Coexistence of Demyelinating Disease and Inflammatory Bowel Disease
- Letter to Editor
- 24th January 2024
Most Comparative Study of Cerebral Volumetric Variations in Patients with Schizophrenia with their Unaffected First-degree Relatives, using Magnetic Resonance Imaging Technique, a Case-control Study
- 3rd January 2024
Bimatoprost Ophthalmic Solution (BOS) 0.3 mg w/v for 1 Open Trial of Long-term Preventive Therapy of Migraine in 3 patients with Pathophysiologic Shift from Brain to Eye
- 9th November 2023
Application of Nonlinear Dynamic Models of the Oculo-Motor System in Diagnostic Studies in Neurosciences
- 30th October 2023
The Neuroprotective Role of TERT Influences the Expression of SOD1 in Motor Neurons and Mouse Brain: Implications for fALS
- 14th October 2023
Effect of Balance Exercises with Smart Phone Based Virtual Reality Programme on Balance in Stroke Patients
- 28th September 2023
Stroke Mimics: Insights from a Retrospective Neuroimaging Study
- Retrospective Study
- 27th September 2023
Interconnection and Communication between Bone Marrow - The Central Immune System - And the Central Nervous System
- 25th September 2023
The Dynamics of Eating Behavior Disorders and the Level of General Psychological Adaptation in Bodybuilding and Fitness Athletes
- 30th August 2023
Update in the Understanding, Diagnosis, and Management of Sturge Weber Syndrome: Case Report
- Case Report
- 24th August 2023
Synergistic Approach: Photobiomodulation, Neuromodulation, and Drug Therapy in Neuropsychiatry. A Promising Strategy to Enhance PBM Adoption and Neurotherapy Efficacy
- 18th August 2023
Psychosis in Parkinson’s Disease and Current Management Trends- an Updated Review of Literature
- 26th July 2023
Sleep quality and Laboratory Findings in Patients with Varicose Vein Leg Pain
- 24th June 2023
Cost Variation Analysis of Various Brands of Anti-Depressants Agents Currently Available in Indian Markets
- 21st June 2023
Considering antioxidant supplements as a means to prevent diseases
- 27th April 2023
Mercury toxicity and amyotrophic lateral sclerosis
- 23rd March 2023
Case study (A and B): a patient with Parkinson’s disease
- 15th March 2023
Case report: contrast imaging in the setting of venous thrombosis
- 28th February 2023
Pseudomonas stutzeri meningitis in a patient with post-laminectomy CSF leak and infected pseudomeningocele: a case report
- 11th November 2022
Nutritional interventions for the prevention and treatment of neurological disorders such as anxiety, bipolar disorder, depression, epilepsy, multiple sclerosis, and schizophrenia
State of the science in diabetic foot: subjective screening vs. objective diabetic neuropathy examination in primary care.
- 14th October 2022
Idiopathic progressive polyneuropathy presented with frequently acute pulmonary oedema: a case report
- 29th September 2022
Sural nerve conduction study: Reference values in the Algerian population
- 20th September 2022
Knowledge, attitude and behaviour of undergraduate medical students towards mentally Ill patients-Suez Canal University, Egypt
- 10th September 2022
Circadian temperature rhythms of the healthy and damaged brain
- 25th August 2022
Role of perioperative plasma D-dimer in intracerebral hemorrhage after brain tumor surgery: A prospective study
- 2nd August 2022
A case of hemiplegia with a cerebrovascular accident in which motor imagery of finger extension on the affected side with finger extension on the unaffected side was effective - A study using F-waves
- 1st July 2022
Idiopathic parkinson’s disease and fatigue
- 10th May 2022
Intrasellar psammomatous meningioma: a case report and review of the literature
- 18th January 2022
Neurotoxicity related exposure to ambient nanoparticles
Pediatric brainstem glioma.
- 3rd January 2022
Neurosciences and dynamical system theory: some short remarks
- 24th September 2021
Differential roles of trithorax protein MLL-1 in regulating neuronal Ion channels
- 8th September 2021
Pituitary adenoma and meningioma simulating a single selar and paraseal injury
- 24th August 2021
Five-year follow up on the single level corpectomy
- 27th July 2021
Multimodal treatment strategies in Huntington’s disease
- 15th July 2021
Facial-onset sensory-motor neuronopathy, a rare variant of Huntington’s disease or chance association?
Role of neuron specific enolase as a biomarker in parkinson’s disease.
- 6th July 2021
Endovascular management of tandem occlusions in stroke: Treatment strategies in a real-world scenario
- 29th June 2021
Differential diagnosis of POLG related disorders: What to keep in mind when multiorgan system is involved?
- 9th June 2021
Endocannabinoidome and its role in neurological disorders-A comprehensive update of existing literature
- 3rd June 2021
Factors associated with mortality after decompressive craniectomy in large basal ganglia bleeds
- 11th May 2021
Endovascular treatment experience in acute ischemic stroke
- 26th April 2021
Characterization of the immune response in neuroimmune disorders in children
- 20th April 2021
Cortical spreading depolarizations in the context of subarachnoid hemorrhage and the role of ketamine
- 23rd March 2021
Cerebral arterial air embolism with anterior spinal cord syndrome after CT-guided hook-wire localization of Lung mass and pulmonary nodule
- 19th March 2021
After human: The world of brain-net on earth
- 25th January 2021
Acute urinary retention and hyponatremia from central hypothyroidism
- 8th January 2021
Impact of mandibular advancement device in quantitative electroencephalogram and sleep quality in mild to severe obstructive sleep apnea
- 30th December 2020
High suspicion of paradoxical embolism due to atrial septal Defect: A rare cause of ischemic stroke
Primary intracranial hodgkin’s lymphoma after a blunt trauma: a case report.
- 15th December 2020
Post-stroke dizziness of visual-vestibular cortices origin
- 27th November 2020
Atlantoaxial subluxation in the pediatric patient: Case series and literature review
- 26th November 2020
Epilepsy due to Neurocysticercosis: Analysis of a Hospital Cohort
- 24th September 2020
Central nervous system diseases associated with blood brain barrier breakdown - A Comprehensive update of existing literature
- 25th August 2020
Visual evoked potentials: Normative values from healthy Senegalese adults
- 11th August 2020
Role of yoga in Parkinson’s disease-A comprehensive update of the literature
- 26th May 2020
PISA Syndrome-Orthopedic manifestation of a neurological disease?
Comparison of resting-state functional and effective connectivity between default mode network and memory encoding related areas.
- 24th April 2020
Obesity may increase the prevalence of Parkinson’s Disease (PD) while PD may reduce obesity index in patients
- 6th April 2020
Do genes matter in sleep?-A comprehensive update
- 5th March 2020
Brain washing systems and other circulating factors in some neurological condition like Parkinson (Pd) and vascular and diabetic dementia: How dynamics- saturation of clearance can act on toxic molecule?
- 24th January 2020
Immunohistochemical expression of Nestin as Cancer Stem Cell Marker in gliomas
- 11th November 2019
Protection from the Pathogenesis of Neurodegenerative Disorders, including Alzheimer’s Disease, Amyotrophic Lateral Sclerosis, Huntington’s Disease, and Parkinson’s Diseases, through the Mitigation of Reactive Oxygen Species
- 4th November 2019
Tamsulosin and Dementia in old age: Is there any relationship?
- 1st October 2019
Carbonic Anhydrase I modifies SOD1-induced motor neuron toxicity in Drosophila via ER stress pathway
- 1st August 2019
The turing machine theory for some spinal cord and brain condition, A toxicological - antidotic depurative approach
- 31st July 2019
Comparative study of carboxylate and amide forms of HLDF-6 peptide: Neuroprotective and nootropic effects in animal models of ischemic stroke
- 24th July 2019
Mimicking multiple sclerosis - Ghost tumor that comes and goes in different parts of the brain without any treatment
- 9th July 2019
Role of plants, environmental toxins and physical neurotoxicological factors in Amyotrophic lateral sclerosis, Alzheimer Disease and other Neurodegenerative Diseases
- 4th March 2019
A Three approach at one stage to surgical management of Cervical Spondyloptosis after Failed Primary anterior fusion
- 31st December 2018
Endogenous archeological sciences: Physiology, Neuroscience, Biochemistry, Immunology, Pharmacology, Oncology and Genetics as instrument for a new field of investigation? Modern global aspects for a new discipline
- 14th December 2018
Cranioplasty with preoperatively customized Polymethyl-methacrylate by using 3-Dimensional Printed Polyethylene Terephthalate Glycol Mold
- 30th November 2018
Neuroscience, Rehabilitation and New Technologies: Perspectives and critical points for a synergistic development
- 28th November 2018
Vigour of CRISPR/Cas9 Gene Editing in Alzheimer’s Disease
- 5th October 2018
Neurobiology of Common Sleep Disorders
- 14th August 2018
Herpes simplex virus (HSV)-1 encephalitis can induce chronic anti-N-methyl-D-aspartate receptor (NMDAR) encephalitis
- 27th July 2018
Discrepancy in Microglia and Peripheral Monocytic Cells - A scope in the Pathophysiology of Psychiatric maladies
- 29th June 2018
Brain and immune system: KURU disease a toxicological process?
- 3rd May 2018
Spinal muscular atrophy counteracted by Agrin biological NT-1654
- 20th April 2018
Comorbidity of alcohol dependence with attention-deficit/hyperactivity disorder and the role of executive dysfunctions
- 30th January 2018
Focal Ab-amyloid deposition precedes cerebral microbleeds and Superficial siderosis: a case report
- 13th October 2017
Experimental ‘hindbrain related’ syringomyelia: some mechanisms of spinal cord damage
- 6th October 2017
Lateralized Cerebral Amyloid Angiopathy presenting with recurrent Lacunar Ischemic Stroke
- 30th August 2017
Direct Carotid Puncture for Flow Diverter Stent Insertion
- 30th June 2017
Protective functions of AEURA in Cell Based Model of Stroke and Alzheimer disease
- 6th June 2017
The “Calcium Paradox” Due To Ca2+/Camp Interaction: New Insights for the Neuroscience Field
- 21st February 2017
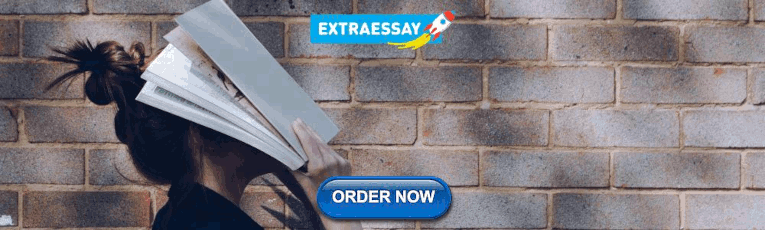
Analysis of early Versus Delayed Carotid Surgery after Acute Ischemic Stroke
- 3rd January 2017
Indexing / Archiving
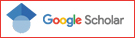
Author's satisfaction with
- Friendly and hassle-free publication process
- Less production time of articles
- Constructive peer-review
- Enhancing journal reputation
- Regular feedback system
- Quick response to authors' queries
Recently Viewed
Most viewed, open journals.
- All Journals 54
- Mathematics & Physics Group 1
- Chemistry Group 1
- Pharma Group 1
- Engineering Group 2
- Biology Group 5
- Clinical Group 34
- Medical Group 10
Clinical Images
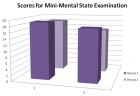
Journal of Neuroscience and Neurological Disorders | ISSN: 2639-3220
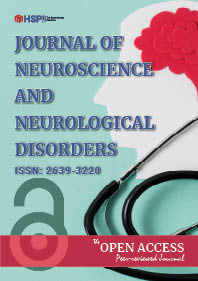
- Pubmed NLM Abbr: J Neurosci Neurol Disord
- Website: www.neuroscijournal.com
- E-ISSN: 2639-3220
- Current Issue: Volume-7 | Issue-2
- Category: Clinical Group
- Issue Regularity: Semi Annual
- Review Process type: Double Blind
- Publication fee: Visit here
- Plagiarism checker: iThenticate
- Managing entity: USA
- Editor-in-Chief: Zuoxin Wang , USA Jang-Yen Wu, USA
- Language: English
- Ability to collect research papers: Worldwide
- IndexCopernicus ICV: 87.42
- Archiving: Portico
- Organized by: Heighten Science Publications Inc.
Current Issue Make a Submission Guidelines Archive RSS

Explore Journal
paper prints policy.
Journal of Neuroscience and Neurological Disorders facilitates reprinting or republishing of the manuscripts with an objective to encourage and promot
Peer Review Policy
Journal of Neuroscience and Neurological Disorders uses Double-Blind Peer Review policy for all the journals. Under this policy both the reviewers and
For Editors
Journal of Neuroscience and Neurological Disorders trail a critical evaluation prior to selection of Editorial Board members. In order to streamline o
Digital Archiving Policy
Journal of Neuroscience and Neurological Disorders is committed to protect the reliability of the scholarly manuscript in all aspects of research and
Explore Universities
- Editor-in-Chief
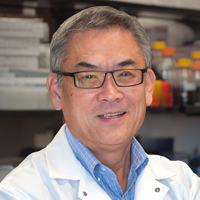
Zuoxin Wang
Professor, Department of Psychology & Program in Neuroscience, Florida State University, USA.
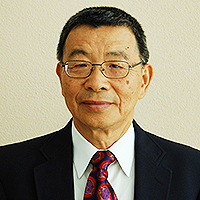
Jang-Yen Wu
Professor, Department of Biomedical Science, Florida Atlantic University, USA.
Editorial Board
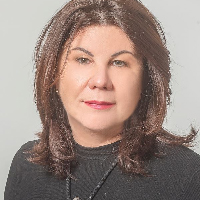
Caterina Pistarini
- University of Pavia, Italy
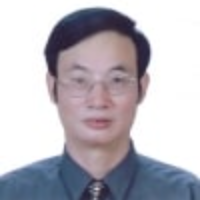
Shan-Quan Sun
- Chongqing Medical University, China
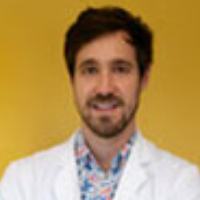
Mikel Vicente-Pascual
- Universitat Rovira i Virgili, Spain
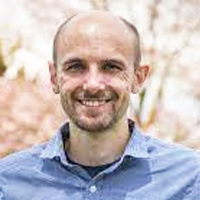
Brandon Lucke-Wold
- University of Florida, USA
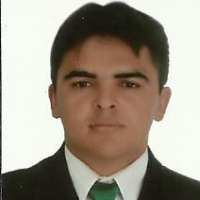
Jalles Dantas de Lucena
- College Santa Maria, Brazil
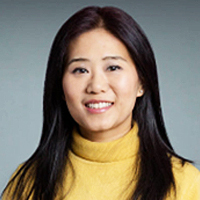
- New York University School of Medicine, USA
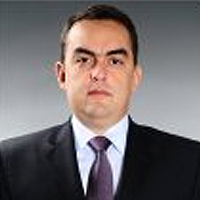
Jean Gonçalves de Oliveira
- Santa Casa de São Paulo School of Medical Sciences, Brazil
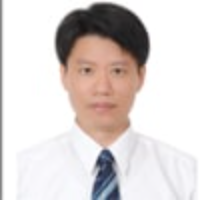
Tsair-Fwu Lee
Most viewed keywords.
- Forensic science
- Abnormal uterine bleeding
- System and synthetic biology
- Pancreatic fistula
- Iliac Aneurysm
- Penile cancer
- Case report
- ACA: Anterior Cerebral Artery
- Peripheral neuropathy
- Agranulocytosis
- Cervical cancer
- Erythrocyte
- Blood hypertension
- Ethnic and race
- Affordability
Latest Articles
Editorial Office Emails:
Name : #echo $editorial_name; ?>
Email : # echo $submission_emails; ?>
Contact: "># echo _COMPANY_EMAIL ?>
Journal Mail: journal_array['email']; ?>">#echo $this->journal_array['email']; ?>
Journal E-Mail : journal_array['secondary_email']; ?>"># echo $this->journal_array['secondary_email']; ?>
Select by Volume & Issue
University/institution.
Select and search by University/Institution.
Articles by Country
Select and search by country to get related articles.
All Issues in 2024
All issues in 2023, all issues in 2022.
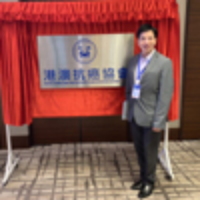
William Cho Queen Elizabeth Hospital, China
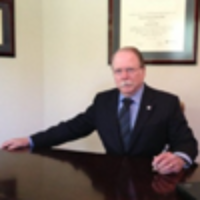
Roger V. Lebo Northeast Ohio Medical University, USA
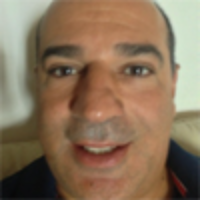
Francesco Loria University of Messina, Italy
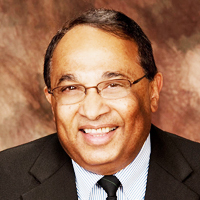
Narayan S Hosmane Northern Illinois University, USA
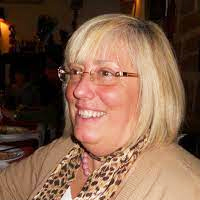
Carmela Saturnino University of Basilicata, Italy
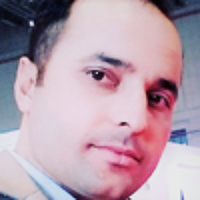
Zul Kamal Shanghai Jiao Tong University, China
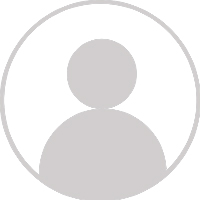
Jihan Saber Raja Mahmoud Stevenson University, USA
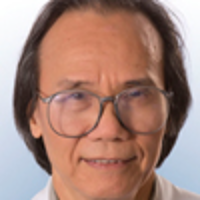
Luan Dinh Truong Cornell University, USA
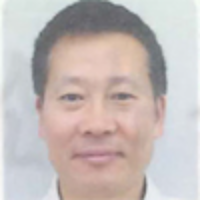
Wenyi (David) Gu University of Queensland (UQ), Australia
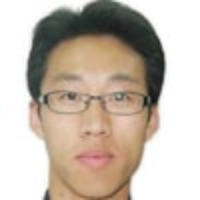
Yun-Feng Zhang University of Pennsylvania, USA
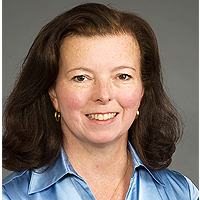
Jane Gibert Boggs Wake Forest School of Medicine, USA
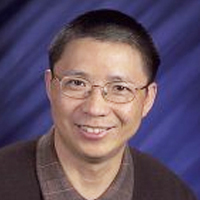
Jian Zhi Hu Pacific Northwest National Laboratories (PNNL), USA
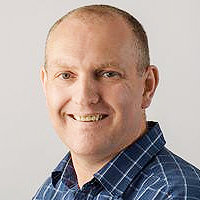
Jonathan Mathers University of Birmingham, United Kingdom
Testmonials.

I would like to thank JPRA for taking this decision. I understand the effort it represents for you. I'm truly happy to have the paper published in JPRA. And I'll certainly consider JPRA for my next publications as I was satisfied of the service provided, the efficiency and promptness of the interactions we had.
Emmanuel BUSATO
Publishing with the International Journal of Clinical and Experimental Ophthalmology was a rewarding experience as review process was thorough and brisk. Their visibility online is second to none as their published articles appear in all search engines. I will encourage researchers to publish with them.
Elizabeth Awoyesuku
“The choice to submit the forensic case study to the Journal of Addiction Therapy and Research was dictated by the match between the content and the potential readership. The publication process proved to be expedient and we were provided with constructive feedback from reviewers. The final article layout is attractive and conforms to standards. All-in-all, it has been a rewarding process.”
Elisabeth H Wiig
Archives of Vascular Medicine is one of the top class journal for vascular medicine with highly interesting topics. You did a professional and great Job!
Elias Noory
Thank you very much. I think the review process and all of what concerns the administration of the publication concerning our paper has been excellent. The nice and quick answers have been very good I think.
Doris Nilsson
Journal of Pulmonary and Respiratory Research is good journal for respiratory research purposes. It takes 2-3 weeks maximum for review of the manuscript to get published and any corrections to be made in the manuscript. It needs good articles and studies to get publish in the respiratory medicine. I am really glad that this journal editors helped me to get my case report published.
Divya Khanduja
Thanks you and your colleague for the great help for our publication. You always provide prompt responses and high quality of service. I am so happy to have you working with me. Thanks again!
Diana (Ding) Dai
Service and process were excellent as was the “look” of the article when published.
Deane Waldman
Great, thank you! It was very efficient working w/ your group. Very thorough reviews (i.e., plagiarism, peer, etc.). Would certainly recommend that future authors consider working w/ your group.
David W Brett
Your services are very good
Chukwuka Ireju Onyinye
I very much appreciate the humanitarian services provided in my stead by this journal/publisher. It exhibits total absence of editorial impertinence. As an Author, I have been guided to have a fruitful experience. The editorial care is highly commendable.
Chrysanthus Chukwuma
"An amazing experience with the Journal of Advanced Pediatrics and Child Health. Very fast blind review with pertinent corrections and suggestions. I highly recommand both the journal and the editor."
Chaimae Khairoun
The submission is very easy and the time from submission to response from the reviewers is short. Correspondence with the journal is nice and rapid.
Catrin Henriksson
The Clinical Journal of Obstetrics and Gynecology is an open access journal focused on scientific knowledge publication with emphasis laid on the fields of Gynecology and Obstetrics. Their services toward us have been encouraging through their kindness and respect. Great consideration has been given to us as young budding researchers and we are very grateful for this.
Carole Assontsa
During the process your positive communication, prompt feedback and professional approach is very highly appreciated. We would like to thank you very much for your support.
I do appreciate for your service including submission, analysis, review, editorial and publishing process. I believe these esteemed journal enlighten the science with its high-quality personel.
I am very much pleased with the fast track publication by your reputed journal's editorial team. It is really helpful for researchers like me from developing nations. I strongly recommend your journal for publication.
Badri Kumar Gupta
It has been a fabulous journey writing articles for your journal because of the encouragement you people provide for writers from developing nations like India. Kindly continue the same. Looking forward for a long term association.
Badareesh Lakshminarayana
Many thanks for publishing my article in your great journal and the friendly and hassle-free publication process, the constructive peer-review, the regular feedback system, and the Quick response to any queries.
Azab Elsayed Azab
I would like to thank this journal for publishing my Research Article. Something I really appreciate about this journal is, they did not take much time from the day of Submission to the publishing date. Looking forward to have more publications in future.
Ayush Chandra
Submission of paper was smooth, the review process was fast. I had excellent communication and on time response from the editor.
Ayokunle Dada
Your service is very good and fast reply, also your service understand our situation and support us to publication our articles.
Ayman M Abu Mustafa
Really good service with prompt response. Looking forward to having long lasting relationship with your journal
Avishek Bagchi
Your service is excellent. Processing and editing were very fast. I hope to publish more of my works in your journal.
Ausraful Islam
I wanna to thank Clinical Journal of Nursing Care and Practice for its effort to review and publish my manuscript. This is reputable journal. Thank you!
Atsedemariam Andualem
“It was a delightful experience publishing my manuscript with the Clinical Journal of Obstetrics and Gynecology. They offered me lots of opportunities I never had from most publishing houses and their prompt services are greatly appreciated.”
Asafo Jones
I hope to ability to make some new investigation and publish in Your Company in future.
Artur Stopyra
I like the quality of the print & overall service. The paper looks quite impressive. Hope this will attract interested readers. All of you have our best wishes for continued success.
Arshad Khan
Your big support from researchers around the world is the best appreciation from your scientific teams. We believe that there should be no barrier in science and you make it real and this motto come true.
Arefhosseinir Rafi
Your journal co-operation is very appreciable and motivational. I am really thankful to your journal and team members for the motivation and collaboration to publish my work.
Archna Dhasmana
I am glad to submit the article to Heighten Science Publications as it has a very smooth and fast peer-review process, which enables the researchers to communicate their work on time.
This is to specify that I have had an extensive and detailed interaction with the Editorial team of Annals of Clinical Gastroenterology and Hepatology, USA, lasting over a significant period of time. My interaction has been extremely pleasant, especially with Ms Allie Smith, as I find the communication quite inspiring and crystal clear. The attitude of aforesaid individuals is quite helpful and guiding in pertinent instances. It has been a commemorative journey so far working with the Journal and I hope that the symbiosis will continue, evolve and flourish in the forthcoming years. I wish the journal, related personnel and aforementioned individuals a fruitful, successful run.
Anubha Bajaj
We appreciate the fact that you decided to give us full waiver for the applicable charges and approve the final version. You did an excellent job preparing the PDF version. Of course we will consider your magazine for our future submissions and we will pay the applicable fees then.
Anna Dionysopoulou
''Co-operation of Archives of Surgery and Clinical Research journal is appreciable. I'm impressed at the promptness of the publishing staff and the professionalism displayed. Thank you very much for your support, help and encouragement.''
Congratulations for the excellence of your journal and high quality of its publications.
Angel MARTIN CASTELLANOS
The service from the journal staff has been excellent.
I was very pleased with the quick editorial process. We are sure that our paper will have great visibility, among other things due to its open access. We believe in science accessible to all.
Anderson Fernando de Souza
It was a great experience publishing through JCICM. The article has reached out to several institutions. Appreciate your professional work. Hope to work with you again
Anas Wardeh
Publishing an article is a long process, but working with your publication department made things go smoothly, even though the process took exactly 5 months from the time of submitting the article till the time I have favourable response, the missing part is the peer review details, which is essential in self auditing and future improvement, overall experience was excellent giving your understanding of the situation of lack of financial institution support.
I think that Heighpubs very good. You are very helpful. Thank you for everything.
Ana Ribeiro
Regarding to be services, we note that are work with high standards of professionalism translated into quick response, efficiency which makes communication accessible. Furthermore, I believe to be much inviting for the submission of future works for publication purposes.
Amélia João Alice Nkutxi
I would like to mention that I had a wonderful experience working with HSPI. The whole process right from manuscript submission to peer review till the publication of the article was very prompt & efficient. I wish you good luck for the future.
Amarjeet Gambhir
Once I submitted the manuscript, the response time of the reviewers was very fast. The fine-tuning of the galley proof was likewise prompt. I believe the journal provide a valuable outlet to disseminate physical rehabilitation scientific knowledge to the clinical community. Respectfully. Dr. Alon
We really appreciate and thanks the full waiver you provide for our article. We happy to publish our paper in your journal. Thank you very much for your good support and services.
Ali Abusafia
It was a real pleasure working with your team. The review was done fast, and it was very clear, the editing was flawless, the article was published quickly compared to other journals, and everyone was understanding and helpful. I will gladly recommend this journal to my acquaintances in academia.
Alexandra Cozma
To the editorial team at HSPI and the Journal of Clinical Nephrology: Thank you so much for your hard work and collaboration in bringing our article to life. Your staff was responsive, flexible, and communicative and made the process smooth and easy. Thank you!
Alejandro Munoz
Dear colleagues! I am satisfied with our cooperation with you. Your service is at a high level. I hope for a future relationship. Let me know if I can get a paper version of the magazine with my articles from you. I see them on the Internet.
Aksenov V.V
"This is my first time publishing with the journal/publisher. I am impressed at the promptness of the publishing staff and the professionalism displayed. Thank you for encouraging young researchers like me!"
Ajite Kayode
I want to thank you for our collaboration. You were fast and effective with a positive spirit of teamwork. I am truly excited from our collaboration. You were like always fast, efficient and accurate. I hope that in the near future we will collaborate again.
Aikaterini Solomou
In my opinion, you provide a very fast and practical service.
Ahmet Eroglu
Great, We are too comfortable with the process including the peer review process and quality. But, the journal should be indexed in different databases such scopus.
Afework Edmealem
We really appreciate your efforts towards our article, the professional way you handle our request for exemption from charges. It was a great honor for us to publish in your magazine.
Achraf elbakkaly
I really liked the ease of submitting my manuscript in the HSPI journal. Further, the peer review was timely completed and I was communicated the final decision on my manuscript within 10 days of submission which is really appreciable. I strongly recommend all the scientists and researchers to submit their work in this journal”
My candid opinion is that the service you render is second to none. My favourite part is the prompt response to issue, really i value that.
Abiodun Akanbi Adeogun
Thank you very much for accepting our manuscript in your journal “International Journal of Clinical Virology”. We are very thankful to the esteemed team for timely response and quick review process. The editorial team of International Journal of Clinical Virology is too cooperative and well-mannered during the publication process. We are hopeful to publish many quality papers in your journal and I suggest the International Journal of Clinical Virology to all of my colleagues, researchers and friends to publish their research here.
Abdul Baset
I, Muhammad Sarwar Khan, am serving as Editor on Archives of Biotechnology and Biomedicine (ABB). I submitted an editorial titled, 'Edible vaccines to combat Infectious Bursal Disease of poultry' for publication in ABB. After submitting the manuscript; the services rendered by the management and technical personnel to handle and process the manuscript were marvelous. Plagiarism report was shared with me with complements before reviewers' comments, All steps including article processing and service charges were well taken care of keeping in view the author's interest/preference. All together, it was an encouraging and wonderful experience working with ABB personnel.
Muhammad Sarwar Khan
Your journal has accomplished its intended mission of providing very effective and efficient goals in dealing with submissions, conducting the reviewing process and in publishing accepted manuscripts in a timely manner. Keep up the great work and services that you provide.
John St. Cyr
I am to express my view that Heighten Science Publications are reliable quick even after peer review process. I hope and wish the publications will go a long way in disseminating science to many interested in scientific research.
Ajit Kumar Roy
The Journal Clinical Nephrology provides a good opportunity for readers to stay updated in the field of clinical nephrology. Additionally - it provides a good opportunity for authors to publish their work. 1. Publication of the accepted manuscripts is sufficiently rapid. 2. The trust factor between the journal and me, as an author, is very important and well preserved. 3. Peer review process very rapid and effective.
Leonid Feldman
In 2017, I submitted a manuscript to the journal Archives of Biotechnology and Biomedicine belonging to Heighten Science Publications Corporation. Within one week I already received the response from the editor. All processing steps were really fast so in terms of a speedy publication I can particularly recommend the journal Archives of Biotechnology and Biomedicine. The responsible contact person of the journal was always available, which gives a trustworthy impression to the author. Also the peer review process was clear and constructive. So from my experience with Heighten Science Publications Corporation I can recommend publishing there.
Yvonne Mast
We thank to the heighten science family, who speed up the publication of our article and provide every support.
Mehmet Besir
The services of the journal were excellent. The most important thing for an author is the speed of the peer review which was really fast here. They returned in a few days and immediately replied all of my questions. I want to refer this platform to all scholars. Many thanks.
Zehra Guchan TOPCU
Thank you for your attitude and support. I am sincerely grateful to you and the entire staff of the magazine for the high professionalism and fast quality work. Thank you very much!
Igor Klepikov
Thank you and your company for effective support of authors which are very much dependable on the funds gambling for science in the different countries of our huge and unpredictable world. We are doing our work and should rely on a teams like Galley Proof-HSPC. Great success to all of you for the 2019th! Be well all the year long.
Victor V Apollonov
The editorial process was quickly done. The galley proof was sent within a week after being accepted for publication. The editorial team was very helpful and responded promptly.
Rohit Kulshrestha
Dr. elizabeth a awoyesuku.
"It was a pleasure to work with the editorial team of the journal on the submission of the manuscript. The team was professional, fast, and to the point".
Moran Sciamama-Saghiv
I am delighted and satisfied with. Heighten Science Publications as my manuscript was thoroughly assessed and published on time without delay. Keep up the good work.
Dr. Shuaib Kayode Aremu
Adebukola ajite.
I wanna to thank clinical journal of nursing care and practice for its effort to review and publish my manuscript. This is reputable journal. Thank you!
We appreciate your approach to scholars and will encourage you to collaborate with your organization, which includes interesting and different medical journals. With the best wishes of success, creativity and joy in life, prosperity in the medical field.
Nataliya Kitsera
Thank you very much for your support and encouragement. I am truly impressed by your tolerance and support. Thank you very much
Nasrulla Abutaleb
You are such a nice person. Your journal co-operation is very appreciable and motivational.
“Mobile apps and wearable technology are becoming ubiquitous in our environment. Their integration with healthcare delivery is just beginning to take shape. The early results are promising and the possibilities great."
Helen Figge
Elisabeth h. wiig.
The service is nice and the time of processing the application is fast.
Your service is very good and fast reply, Also your service understand our situation and support us to publication our articles.
Akowuah Jones Asafo
Related journals.
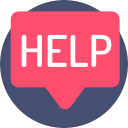
HSPI: We're glad you're here. Please click "create a new Query" if you are a new visitor to our website and need further information from us.
If you are already a member of our network and need to keep track of any developments regarding a question you have already submitted, click "take me to my Query."
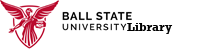
Biomarkers of neurological diseases
Guest Editors: Santiago Perez-Lloret: Pontifical Catholic University of Argentina, National Research Council of Argentina Wen-Jun Tu: Chinese Academy of Medical Sciences & Peking Union Medical College, China
Articles will undergo the journal’s standard peer-review process and are subject to all of the journal’s standard policies . Articles will be added to the Collection as they are published.
The Guest Editors have no competing interests with the submissions which they handle through the peer review process. The peer review of any submissions for which the Guest Editors have competing interests is handled by another Editorial Board Member who has no competing interests.
Advertisement
Relationship of Sleep Disorder with Neurodegenerative and Psychiatric Diseases: An Updated Review
- Published: 18 December 2023
- Volume 49 , pages 568–582, ( 2024 )
Cite this article
- Xiao Xiao 1 , 2 na1 ,
- Yimin Rui 1 , 2 na1 ,
- Yu Jin 2 &
- Ming Chen 2
633 Accesses
2 Citations
10 Altmetric
Explore all metrics
Sleep disorders affect many people worldwide and can accompany neurodegenerative and psychiatric diseases. Sleep may be altered before the clinical manifestations of some of these diseases appear. Moreover, some sleep disorders affect the physiological organization and function of the brain by influencing gene expression, accelerating the accumulation of abnormal proteins, interfering with the clearance of abnormal proteins, or altering the levels of related hormones and neurotransmitters, which can cause or may be associated with the development of neurodegenerative and psychiatric diseases. However, the detailed mechanisms of these effects are unclear. This review mainly focuses on the relationship between and mechanisms of action of sleep in Alzheimer’s disease, depression, and anxiety, as well as the relationships between sleep and Parkinson’s disease, Huntington’s disease, and amyotrophic lateral sclerosis. This summary of current research hotspots may provide researchers with better clues and ideas to develop treatment solutions for neurodegenerative and psychiatric diseases associated with sleep disorders.
This is a preview of subscription content, log in via an institution to check access.
Access this article
Price includes VAT (Russian Federation)
Instant access to the full article PDF.
Rent this article via DeepDyve
Institutional subscriptions
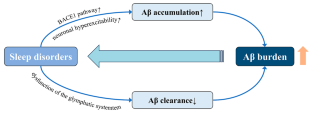
Similar content being viewed by others
The missing link between sleep disorders and age-related dementia: recent evidence and plausible mechanisms.
Feng Zhang, Rujia Zhong, … Weidong Le
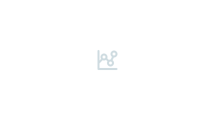
Sleep as a Therapeutic Target in the Aging Brain
Thierno M. Bah, James Goodman & Jeffrey J. Iliff
Sleep in Neurodegenerative Disorders
Roneil Malkani & Hrayr Attarian
Abbreviations
Amyloid β-protein
Alzheimer’s disease
Amyotrophic lateral sclerosis
Amyloid precursor protein
Aquaporin 4
Acute sleep deprivation
Β-site APP cleaving enzyme 1
Brain-derived neurotrophic factor
Brain and muscle arnt-like protein-1
Chronic sleep deprivation
Cerebrospinal fluid
Chronic sleep restriction
Huntington’s disease
Hypothalamic-pituitary-adrenal
Interleukin
Interstitial fluid
Kelch-like ECH-associated protein 1
Low-density lipoprotein receptor-related protein 1
Mild cognitive impairment
Normal cognition
Non-rapid eye movement
Nuclear factor erythroid 2-related factor
Obstructive sleep apnea
Parkinson’s disease
Receptor of advanced glycation end products
Rapid eye movement sleep-behavior disorder
Rapid eye movement
Sleep deprivation
Sleep-disordered breathing
Slow-wave activity
Slow wave sleep
43-kDa TAR DNA-binding protein
Total sleep deprivation
Grandner MA, Fernandez FX (2021) The translational neuroscience of sleep: a contextual framework. Science 374:568–573
Article CAS PubMed PubMed Central ADS Google Scholar
Voysey Z, Fazal SV, Lazar AS, Barker RA (2021) The sleep and circadian problems of Huntington’s Disease: when, why and their importance. J Neurol 268:2275–2283
Article CAS PubMed Google Scholar
Joiner WJ (2018) The neurobiological basis of Sleep and Sleep disorders. Physiol (Bethesda) 33:317–327
CAS Google Scholar
Léger D, Bayon V (2010) Societal costs of insomnia. Sleep Med Rev 14:379–389
Article PubMed Google Scholar
Hillman DR, Lack LC (2013) Public health implications of sleep loss: the community burden. Med J Aust 199:S7–10
Latreille MKP V (2019) Sleep disorders. Am J Med 132:292–299
Liew SC, Aung T (2021) Sleep deprivation and its association with diseases- a review. Sleep Med 77:192–204
Banks S, Dinges DF (2007) Behavioral and physiological consequences of sleep restriction. J Clin Sleep Med 3:519–528
Article PubMed PubMed Central Google Scholar
Umemura GS, Furtado F, Dos Santos FC, Gonçalves B, Forner-Cordero A (2022) Is Balance Control affected by Sleep Deprivation? A systematic review of the impact of Sleep on the control of balance. Front Neurosci 16:779086
Pires GN, Bezerra AG, Tufik S, Andersen ML (2016) Effects of acute sleep deprivation on state anxiety levels: a systematic review and meta-analysis. Sleep Med 24:109–118
Colwell CS (2021) Defining circadian disruption in neurodegenerative disorders. J Clin Invest 131
Fang H, Tu S, Sheng J, Shao A (2019) Depression in sleep disturbance: a review on a bidirectional relationship, mechanisms and treatment. J Cell Mol Med 23:2324–2332
Wang YQ, Li R, Zhang MQ, Zhang Z, Qu WM, Huang ZL (2015) The neurobiological mechanisms and treatments of REM sleep disturbances in Depression. Curr Neuropharmacol 13:543–553
Article CAS PubMed PubMed Central Google Scholar
Guo B, Zhang M, Hao W, Wang Y, Zhang T, Liu C (2023) Neuroinflammation mechanisms of neuromodulation therapies for anxiety and depression. Transl Psychiatry 13:5
Gauthier S, Webster C, Servaes S, Morais JA, Rosa-Neto P (2022) World Alzheimer Report 2022: Life after diagnosis: Navigating treatment, care and support. In. Alzheimer’s Disease International, London, England
Okuda S, Tetsuka J, Takahashi K, Toda Y, Kubo T, Tokita S (2019) Association between sleep disturbance in Alzheimer’s Disease patients and burden on and health status of their caregivers. J Neurol 266:1490–1500
Kastanenka KV, Hou SS, Shakerdge N, Logan R, Feng D, Wegmann S, Chopra V, Hawkes JM, Chen X, Bacskai BJ (2017) Optogenetic restoration of disrupted slow oscillations halts amyloid deposition and restores Calcium Homeostasis in an animal model of Alzheimer’s Disease. PLoS ONE 12:e0170275
Suh SW, Han JW, Lee JR, Byun S, Kwon SJ, Oh SH, Lee KH, Han G, Hong JW, Kwak KP, Kim BJ, Kim SG, Kim JL, Kim TH, Ryu SH, Moon SW, Park JH, Seo J, Youn JC, Lee DY, Lee DW, Lee SB, Lee JJ, Jhoo JH, Kim KW (2018) Sleep and cognitive decline: a prospective nondemented elderly cohort study. Ann Neurol 83:472–482
Lucey BP, Wisch J, Boerwinkle AH, Landsness EC, Toedebusch CD, McLeland JS, Butt OH, Hassenstab J, Morris JC, Ances BM, Holtzman DM (2021) Sleep and longitudinal cognitive performance in preclinical and early symptomatic Alzheimer’s Disease. Brain 144:2852–2862
Maestri M, Carnicelli L, Tognoni G, Di Coscio E, Giorgi FS, Volpi L, Economou NT, Ktonas P, Ferri R, Bonuccelli U, Bonanni E (2015) Non-rapid eye movement sleep instability in mild cognitive impairment: a pilot study. Sleep Med 16:1139–1145
Pase MP, Himali JJ, Grima NA, Beiser AS, Satizabal CL, Aparicio HJ, Thomas RJ, Gottlieb DJ, Auerbach SH, Seshadri S (2017) Sleep architecture and the risk of incident Dementia in the community. NEUROLOGY 89:1244–1250
Lucey BP, McCullough A, Landsness EC, Toedebusch CD, McLeland JS, Zaza AM, Fagan AM, McCue L, Xiong C, Morris JC, Benzinger TLS, Holtzman DM (2019) Reduced non-rapid eye movement sleep is associated with tau pathology in early Alzheimer’s disease. Sci Transl Med 11
Liu S, Meng Y, Wang N, Lei Q, He L, Hong X, Li Z (2023) Disturbance of REM sleep exacerbates microglial activation in APP/PS1 mice. Neurobiol Learn Mem 200:107737
Chylinski D, Van Egroo M, Narbutas J, Muto V, Bahri MA, Berthomier C, Salmon E, Bastin C, Phillips C, Collette F, Maquet P, Carrier J, Lina JM, Vandewalle G (2022) Timely coupling of sleep spindles and slow waves linked to early amyloid-β burden and predicts memory decline. eLife 11
Chylinski DO, Van Egroo M, Narbutas J, Grignard M, Koshmanova E, Berthomier C, Berthomier P, Brandewinder M, Salmon E, Bahri MA, Bastin C, Collette F, Phillips C, Maquet P, Muto V, Vandewalle G (2021) Heterogeneity in the links between sleep arousals, amyloid-β, and cognition. JCI Insight 6
Winer JR, Deters KD, Kennedy G, Jin M, Goldstein-Piekarski A, Poston KL, Mormino EC (2021) Association of short and long sleep duration with Amyloid-β Burden and Cognition in Aging. JAMA Neurol 78:1187–1196
Fan L, Xu W, Cai Y, Hu Y, Wu C (2019) Sleep duration and the risk of Dementia: a systematic review and Meta-analysis of prospective cohort studies. J Am Med Dir Assoc 20:1480–1487e1485
Leng Y, Ackley SF, Glymour MM, Yaffe K, Brenowitz WD (2021) Genetic risk of Alzheimer’s Disease and Sleep Duration in Non-demented elders. Ann Neurol 89:177–181
Xu W, Tan L, Su BJ, Yu H, Bi YL, Yue XF, Dong Q, Yu JT (2020) Sleep characteristics and cerebrospinal fluid biomarkers of Alzheimer’s Disease pathology in cognitively intact older adults: the CABLE study. Alzheimers Dement 16:1146–1152
Cavaillès C, Berr C, Helmer C, Gabelle A, Jaussent I, Dauvilliers Y (2022) Complaints of daytime sleepiness, insomnia, hypnotic use, and risk of Dementia: a prospective cohort study in the elderly. Alzheimers Res Ther 14:12
Anderson EL, Richmond RC, Jones SE, Hemani G, Wade KH, Dashti HS, Lane JM, Wang H, Saxena R, Brumpton B, Korologou-Linden R, Nielsen JB, Åsvold BO, Abecasis G, Coulthard E, Kyle SD, Beaumont RN, Tyrrell J, Frayling TM, Munafò MR, Wood AR, Ben-Shlomo Y, Howe LD, Lawlor DA, Weedon MN, Davey Smith G (2021) Is disrupted sleep a risk factor for Alzheimer’s Disease? Evidence from a two-sample mendelian randomization analysis. Int J Epidemiol 50:817–828
André C, Rehel S, Kuhn E, Landeau B, Moulinet I, Touron E, Ourry V, Le Du G, Mézenge F, Tomadesso C, de Flores R, Bejanin A, Sherif S, Delcroix N, Manrique A, Abbas A, Marchant NL, Lutz A, Klimecki OM, Collette F, Arenaza-Urquijo EM, Poisnel G, Vivien D, Bertran F, de la Sayette V, Chételat G, Rauchs G (2020) Association of Sleep-Disordered Breathing with Alzheimer Disease biomarkers in Community-Dwelling older adults: a secondary analysis of a Randomized Clinical Trial. JAMA Neurol 77:716–724
Lee JE, Yang SW, Ju YJ, Ki SK, Chun KH (2019) Sleep-disordered breathing and Alzheimer’s Disease: a nationwide cohort study. Psychiatry Res 273:624–630
Liguori C, Maestri M, Spanetta M, Placidi F, Bonanni E, Mercuri NB, Guarnieri B (2021) Sleep-disordered breathing and the risk of Alzheimer’s Disease. Sleep Med Rev 55:101375
Bubu OM, Andrade AG, Umasabor-Bubu OQ, Hogan MM, Turner AD, de Leon MJ, Ogedegbe G, Ayappa I, Jean-Louis GG, Jackson ML, Varga AW, Osorio RS (2020) Obstructive sleep apnea, cognition and Alzheimer’s Disease: a systematic review integrating three decades of multidisciplinary research. Sleep Med Rev 50:101250
Sharma RA, Varga AW, Bubu OM, Pirraglia E, Kam K, Parekh A, Wohlleber M, Miller MD, Andrade A, Lewis C, Tweardy S, Buj M, Yau PL, Sadda R, Mosconi L, Li Y, Butler T, Glodzik L, Fieremans E, Babb JS, Blennow K, Zetterberg H, Lu SE, Badia SG, Romero S, Rosenzweig I, Gosselin N, Jean-Louis G, Rapoport DM, de Leon MJ, Ayappa I, Osorio RS (2018) Obstructive sleep apnea severity affects amyloid Burden in Cognitively Normal Elderly. A longitudinal study. Am J Respir Crit Care Med 197:933–943
Punjabi NM (2008) The epidemiology of adult obstructive sleep apnea. Proc Am Thorac Soc 5:136–143
Ooms S, Overeem S, Besse K, Rikkert MO, Verbeek M, Claassen JA (2014) Effect of 1 night of total sleep deprivation on cerebrospinal fluid β-amyloid 42 in healthy middle-aged men: a randomized clinical trial. JAMA Neurol 71:971–977
Lucey BP, Hicks TJ, McLeland JS, Toedebusch CD, Boyd J, Elbert DL, Patterson BW, Baty J, Morris JC, Ovod V, Mawuenyega KG, Bateman RJ (2018) Effect of sleep on overnight cerebrospinal fluid amyloid β kinetics. Ann Neurol 83:197–204
Benedict C, Blennow K, Zetterberg H, Cedernaes J (2020) Effects of acute sleep loss on diurnal plasma dynamics of CNS health biomarkers in young men. NEUROLOGY 94:e1181–e1189
Wei M, Zhao B, Huo K, Deng Y, Shang S, Liu J, Li Y, Ma L, Jiang Y, Dang L, Chen C, Wei S, Zhang J, Yang H, Gao F, Qu Q (2017) Sleep Deprivation Induced plasma Amyloid-β transport disturbance in healthy young adults. J Alzheimers Dis 57:899–906
Ju Y-ES, Ooms SJ, Sutphen C, Macauley SL, Zangrilli MA, Jerome G, Fagan AM, Mignot E, Zempel JM, Claassen JAHR, Holtzman DM (2017) Slow wave sleep disruption increases cerebrospinal fluid amyloid-β levels. Brain 140:2104–2111
Qiu H, Zhong R, Liu H, Zhang F, Li S, Le W (2016) Chronic sleep deprivation exacerbates Learning-Memory disability and Alzheimer’s Disease-Like pathologies in AβPP(swe)/PS1(∆E9) mice. J Alzheimers Dis 50:669–685
Zhao HY, Wu HJ, He JL, Zhuang JH, Liu ZY, Huang LQ, Zhao ZX (2017) Chronic sleep restriction induces cognitive deficits and cortical Beta-amyloid deposition in mice via BACE1-Antisense activation. CNS Neurosci Ther 23:233–240
Zhao B, Liu P, Wei M, Li Y, Liu J, Ma L, Shang S, Jiang Y, Huo K, Wang J, Qu Q (2019) Chronic sleep restriction induces Aβ Accumulation by disrupting the balance of Aβ production and clearance in rats. Neurochem Res 44:859–873
Xie Y, Ba L, Wang M, Deng SY, Chen SM, Huang LF, Zhang M, Wang W, Ding FF (2020) Chronic sleep fragmentation shares similar pathogenesis with neurodegenerative Diseases: Endosome-autophagosome-lysosome pathway dysfunction and microglia-mediated neuroinflammation. CNS Neurosci Ther 26:215–227
Vasciaveo V, Iadarola A, Casile A, Dante D, Morello G, Minotta L, Tamagno E, Cicolin A, Guglielmotto M (2023) Sleep fragmentation affects glymphatic system through the different expression of AQP4 in wild type and 5xFAD mouse models. Acta Neuropathol Commun 11:16
Nick H, Fenik P, Zhu Y, Veasey S (2022) Hypocretin/orexin influences chronic sleep disruption injury in the hippocampus. Front Aging Neurosci 14:1025402
Kent BA, Feldman HH, Nygaard HB (2021) Sleep and its regulation: an emerging pathogenic and treatment frontier in Alzheimer’s Disease. Prog Neurobiol 197:101902
Tabuchi M, Lone SR, Liu S, Liu Q, Zhang J, Spira AP, Wu MN (2015) Sleep interacts with aβ to modulate intrinsic neuronal excitability. Curr Biol 25:702–712
Ba L, Huang L, He Z, Deng S, Xie Y, Zhang M, Jacob C, Antonecchia E, Liu Y, Xiao W, Xie Q, Huang Z, Yi C, D’Ascenzo N, Ding F (2021) Does chronic sleep fragmentation lead to Alzheimer’s Disease in Young Wild-Type mice? Front Aging Neurosci 13:759983
Owen JE, Zhu Y, Fenik P, Zhan G, Bell P, Liu C, Veasey S (2021) Late-in-life neurodegeneration after chronic sleep loss in young adult mice. Sleep 44
Zhang F, Niu L, Zhong R, Li S, Le W (2023) Chronic sleep disturbances alters Sleep structure and tau phosphorylation in AβPP/PS1 AD mice and their wild-type littermates. J Alzheimers Dis 92:1341–1355
Zhu Y, Zhan G, Fenik P, Brandes M, Bell P, Francois N, Shulman K, Veasey S (2018) Chronic sleep disruption advances the temporal progression of Tauopathy in P301S Mutant mice. J Neurosci 38:10255–10270
Niu L, Zhang F, Xu X, Yang Y, Li S, Liu H, Le W (2022) Chronic sleep deprivation altered the expression of circadian clock genes and aggravated Alzheimer’s Disease neuropathology. Brain Pathol 32:e13028
Lucey BP (2020) It’s complicated: the relationship between sleep and Alzheimer’s Disease in humans. Neurobiol Dis 144:105031
Sato C, Barthélemy NR, Mawuenyega KG, Patterson BW, Gordon BA, Jockel-Balsarotti J, Sullivan M, Crisp MJ, Kasten T, Kirmess KM, Kanaan NM, Yarasheski KE, Baker-Nigh A, Benzinger TLS, Miller TM, Karch CM, Bateman RJ (2018) Tau kinetics in neurons and the Human Central Nervous System. Neuron 98:861–864
Yanamandra K, Patel TK, Jiang H, Schindler S, Ulrich JD, Boxer AL, Miller BL, Kerwin DR, Gallardo G, Stewart F, Finn MB, Cairns NJ, Verghese PB, Fogelman I, West T, Braunstein J, Robinson G, Keyser J, Roh J, Knapik SS, Hu Y, Holtzman DM (2017) Anti-tau antibody administration increases plasma tau in transgenic mice and patients with tauopathy. Sci Transl Med 9
Yamada K, Holth JK, Liao F, Stewart FR, Mahan TE, Jiang H, Cirrito JR, Patel TK, Hochgräfe K, Mandelkow EM, Holtzman DM (2014) Neuronal activity regulates extracellular tau in vivo. J Exp Med 211:387–393
Holth JK, Fritschi SK, Wang C, Pedersen NP, Cirrito JR, Mahan TE, Finn MB, Manis M, Geerling JC, Fuller PM, Lucey BP, Holtzman DM (2019) The sleep-wake cycle regulates brain interstitial fluid tau in mice and CSF tau in humans. Sci (New York NY) 363:880–884
Article CAS ADS Google Scholar
Irwin MR, Vitiello MV (2019) Implications of sleep disturbance and inflammation for Alzheimer’s Disease Dementia. Lancet Neurol 18:296–306
Wu R, Tripathy S, Menon V, Yu L, Buchman AS, Bennett DA, De Jager PL, Lim ASP (2022) Fragmentation of rest periods, astrocyte activation, and cognitive decline in older adults with and without Alzheimer’s disease. Alzheimers Dement
Kaneshwaran K, Olah M, Tasaki S, Yu L, Bradshaw EM, Schneider JA, Buchman AS, Bennett DA, De Jager PL, Lim ASP (2019) Sleep fragmentation, microglial aging, and cognitive impairment in adults with and without Alzheimer’s Dementia. Sci Adv 5:eaax7331
Bellesi M, de Vivo L, Chini M, Gilli F, Tononi G, Cirelli C (2017) Sleep loss promotes astrocytic phagocytosis and microglial activation in mouse cerebral cortex. J Neurosci 37:5263–5273
Kincheski GC, Valentim IS, Clarke JR, Cozachenco D, Castelo-Branco MTL, Ramos-Lobo AM, Rumjanek V, Donato J Jr., De Felice FG, Ferreira ST (2017) Chronic sleep restriction promotes Brain Inflammation and synapse loss, and potentiates memory impairment induced by amyloid-β oligomers in mice. Brain Behav Immun 64:140–151
Liu P, Zhao B, Wei M, Li Y, Liu J, Ma L, Shang S, Huo K, Wang J, Li R, Qu Q (2020) Activation of inflammation is Associated with Amyloid-β Accumulation Induced by Chronic Sleep restriction in rats. J Alzheimers Dis 74:759–773
Berk M, Williams LJ, Jacka FN, O’Neil A, Pasco JA, Moylan S, Allen NB, Stuart AL, Hayley AC, Byrne ML, Maes M (2013) So depression is an inflammatory Disease, but where does the inflammation come from? BMC Med 11:200
Siegel JM (2005) Clues to the functions of mammalian sleep. Nature 437:1264–1271
Wulff K, Gatti S, Wettstein JG, Foster RG (2010) Sleep and circadian rhythm disruption in psychiatric and neurodegenerative Disease. Nat Rev Neurosci 11:589–599
Mendoza J (2019) Circadian insights into the biology of depression: symptoms, treatments and animal models. Behav Brain Res 376:112186
Chellappa SL, Aeschbach D (2022) Sleep and anxiety: from mechanisms to interventions. Sleep Med Rev 61:101583
Mirchandaney R, Barete R, Asarnow LD (2022) Moderators of cognitive behavioral treatment for Insomnia on Depression and anxiety outcomes. Curr Psychiatry Rep 24:121–128
Soehner AM, Kaplan KA, Harvey AG (2014) Prevalence and clinical correlates of co-occurring insomnia and hypersomnia symptoms in depression. J Affect Disord 167:93–97
Zhou F, Li S, Xu H (2022) Insomnia, sleep duration, and risk of anxiety: a two-sample mendelian randomization study. J Psychiatr Res 155:219–225
Bean CAL, Ciesla JA (2021) Naturalistic partial sleep deprivation leads to Greater Next-Day anxiety: the moderating role of baseline anxiety and depression. Behav Ther 52:861–873
Palmer CA, Alfano CA (2020) Anxiety modifies the emotional effects of sleep loss. Curr Opin Psychol 34:100–104
Zhou L, Zhang H, Luo Z, Liu X, Yang L, Hu H, Wang Y, Wang C, Wang F (2020) Abnormal night sleep duration and inappropriate sleep initiation time are associated with elevated anxiety symptoms in Chinese rural adults: the Henan Rural Cohort. Psychiatry Res 291:113232
Riemann D (2007) Insomnia and comorbid psychiatric disorders. Sleep Med 8(Suppl 4):S15–20
Lopez R, Barateau L, Evangelista E, Dauvilliers Y (2017) Depression and Hypersomnia: a Complex Association. Sleep Med Clin 12:395–405
Luik AI, Zuurbier LA, Direk N, Hofman A, Van Someren EJ, Tiemeier H (2015) 24-HOUR ACTIVITY RHYTHM AND SLEEP DISTURBANCES IN DEPRESSION AND ANXIETY: A POPULATION-BASED STUDY OF MIDDLE-AGED AND OLDER PERSONS. Depress Anxiety 32:684–692
Bouwmans ME, Conradi HJ, Bos EH, Oldehinkel AJ, de Jonge P (2017) Bidirectionality between sleep symptoms and core depressive symptoms and their long-term course in Major Depression. Psychosom Med 79:336–344
Casement MD, Keenan KE, Hipwell AE, Guyer AE, Forbes EE (2016) Neural reward Processing mediates the relationship between insomnia symptoms and Depression in Adolescence. Sleep 39:439–447
Coles ME, Schubert JR, Nota JA (2015) Sleep, circadian rhythms, and anxious traits. Curr Psychiatry Rep 17:73
Horváth A, Montana X, Lanquart JP, Hubain P, Szűcs A, Linkowski P, Loas G (2016) Effects of state and trait anxiety on sleep structure: a polysomnographic study in 1083 subjects. Psychiatry Res 244:279–283
Short MA, Louca M (2015) Sleep deprivation leads to mood deficits in healthy adolescents. Sleep Med 16:987–993
Taraku B, Zavaliangos-Petropulu A, Loureiro JR, Al-Sharif NB, Kubicki A, Joshi SH, Woods RP, Espinoza R, Narr KL, Sahib AK (2023) White matter microstructural perturbations after total sleep deprivation in depression. Front Psychiatry 14:1195763
Hu B, Liu C, Mou T, Luo F, Lv T, Qian C, Zhang J, Ye M, Liu Z (2021) Meta-analysis of Sleep Deprivation effects on patients with Depression. Front Psychiatry 12:783091
Dong L, Xie Y, Zou X (2022) Association between sleep duration and depression in US adults: a cross-sectional study. J Affect Disord 296:183–188
Tartar JL, Ward CP, Cordeira JW, Legare SL, Blanchette AJ, McCarley RW, Strecker RE (2009) Experimental sleep fragmentation and sleep deprivation in rats increases exploration in an open field test of anxiety while increasing plasma corticosterone levels. Behav Brain Res 197:450–453
Pires GN, Bezerra AG, Tufik S, Andersen ML (2016) Effects of experimental sleep deprivation on anxiety-like behavior in animal research: systematic review and meta-analysis. Neurosci Biobehav Rev 68:575–589
Finan PH, Quartana PJ, Smith MT (2015) The effects of Sleep Continuity disruption on positive Mood and Sleep Architecture in healthy adults. Sleep 38:1735–1742
Meerlo P, Mistlberger RE, Jacobs BL, Heller HC, McGinty D (2009) New neurons in the adult brain: the role of sleep and consequences of sleep loss. Sleep Med Rev 13:187–194
Clasadonte J, McIver SR, Schmitt LI, Halassa MM, Haydon PG (2014) Chronic sleep restriction disrupts sleep homeostasis and behavioral sensitivity to alcohol by reducing the extracellular accumulation of adenosine. J Neurosci 34:1879–1891
Arvin P, Ghafouri S, Bavarsad K, Hajipour S, Khoshnam SE, Sarkaki A, Farbood Y (2023) Administration of growth hormone ameliorates adverse effects of total sleep deprivation. Metab Brain Dis 38:1671–1681
Klumpers UM, Veltman DJ, van Tol MJ, Kloet RW, Boellaard R, Lammertsma AA, Hoogendijk WJ (2015) Neurophysiological effects of sleep deprivation in healthy adults, a pilot study. PLoS ONE 10:e0116906
Jordan W, Tumani H, Cohrs S, Eggert S, Rodenbeck A, Brunner E, Rüther E, Hajak G (2004) Prostaglandin D synthase (beta-trace) in healthy human sleep. Sleep 27:867–874
Zhang Y, Dai C, Shao Y, Peng J, Yang Y, Hou Y (2021) Decreased functional connectivity in the reward network and its relationship with negative emotional experience after total sleep deprivation. Front Neurol 12:641810
Bosch OG, Rihm JS, Scheidegger M, Landolt HP, Stämpfli P, Brakowski J, Esposito F, Rasch B, Seifritz E (2013) Sleep deprivation increases dorsal nexus connectivity to the dorsolateral prefrontal cortex in humans. Proc Natl Acad Sci U S A 110:19597–19602
Lopez-Rodriguez F, Wilson CL, Maidment NT, Poland RE, Engel J (2003) Total sleep deprivation increases extracellular serotonin in the rat hippocampus. Neuroscience 121:523–530
Wu JC, Gillin JC, Buchsbaum MS, Schachat C, Darnall LA, Keator DB, Fallon JH, Bunney WE (2008) Sleep deprivation PET correlations of Hamilton symptom improvement ratings with changes in relative glucose metabolism in patients with depression. J Affect Disord 107:181–186
Grassi Zucconi G, Cipriani S, Balgkouranidou I, Scattoni R (2006) One night’ sleep deprivation stimulates hippocampal neurogenesis. Brain Res Bull 69:375–381
Tartar JL, Ward CP, McKenna JT, Thakkar M, Arrigoni E, McCarley RW, Brown RE, Strecker RE (2006) Hippocampal synaptic plasticity and spatial learning are impaired in a rat model of sleep fragmentation. Eur J Neurosci 23:2739–2748
Grubac Z, Sutulovic N, Ademovic A, Velimirovic M, Rasic-Markovic A, Macut D, Petronijevic N, Stanojlovic O, Hrncic D (2019) Short-term sleep fragmentation enhances anxiety-related behavior: the role of hormonal alterations. PLoS ONE 14:e0218920
Nair D, Zhang SX, Ramesh V, Hakim F, Kaushal N, Wang Y, Gozal D (2011) Sleep fragmentation induces cognitive deficits via nicotinamide adenine dinucleotide phosphate oxidase-dependent pathways in mouse. Am J Respir Crit Care Med 184:1305–1312
Stepnowsky CJ, Palau JJ, Zamora T, Ancoli-Israel S, Loredo JS (2011) Fatigue in sleep apnea: the role of depressive symptoms and self-reported sleep quality. Sleep Med 12:832–837
Gong L, Liao T, Liu D, Luo Q, Xu R, Huang Q, Zhang B, Feng F, Zhang C (2019) Amygdala Changes in Chronic Insomnia and Their Association with sleep and anxiety symptoms: insight from shape analysis. Neural Plast 2019:8549237
Gong L, Shi M, Wang J, Xu R, Yu S, Liu D, Ding X, Zhang B, Zhang X, Xi C (2021) The abnormal functional connectivity in the Locus Coeruleus-Norepinephrine System Associated with anxiety Symptom in Chronic Insomnia Disorder. Front Neurosci 15:678465
Xu M, Wang Q, Li B, Qian S, Wang S, Wang Y, Chen C, Liu Z, Ji Y, Liu K, Xin K, Niu Y (2023) Cerebellum and hippocampus abnormalities in patients with insomnia comorbid depression: a study on cerebral blood perfusion and functional connectivity. Front Neurosci 17:1202514
Motomura Y, Katsunuma R, Ayabe N, Oba K, Terasawa Y, Kitamura S, Moriguchi Y, Hida A, Kamei Y, Mishima K (2021) Decreased activity in the reward network of chronic insomnia patients. Sci Rep 11:3600
Gong L, Yu S, Xu R, Liu D, Dai X, Wang Z, Hu Y, Yan B, Kui Y, Zhang B, Feng F (2021) The abnormal reward network associated with insomnia severity and depression in chronic insomnia disorder. Brain Imaging Behav 15:1033–1042
Rahmani M, Rahmani F, Rezaei N (2020) The brain-derived neurotrophic factor: missing Link between Sleep Deprivation, Insomnia, and Depression. Neurochem Res 45:221–231
Schmitt K, Holsboer-Trachsler E, Eckert A (2016) BDNF in sleep, insomnia, and sleep deprivation. Ann Med 48:42–51
Konakanchi S, Raavi V, Ml HK, Shankar Ms V (2022) Effect of chronic sleep deprivation and sleep recovery on hippocampal CA3 neurons, spatial memory and anxiety-like behavior in rats. Neurobiol Learn Mem 187:107559
Xia M, Li Z, Li S, Liang S, Li X, Chen B, Zhang M, Dong C, Verkhratsky A, Guan D, Li B (2020) Sleep Deprivation Selectively Down-Regulates Astrocytic 5-HT(2B) Receptors and Triggers Depressive-Like Behaviors via Stimulating P2X(7) Receptors in Mice. Neurosci Bull 36:1259–1270
Manchanda S, Singh H, Kaur T, Kaur G (2018) Low-grade neuroinflammation due to chronic sleep deprivation results in anxiety and learning and memory impairments. Mol Cell Biochem 449:63–72
Kiecolt-Glaser JK, Derry HM, Fagundes CP (2015) Inflammation: depression fans the flames and feasts on the heat. Am J Psychiatry 172:1075–1091
Liu H, Huang X, Li Y, Xi K, Han Y, Mao H, Ren K, Wang W, Wu Z (2022) TNF signaling pathway-mediated microglial activation in the PFC underlies acute paradoxical sleep deprivation-induced anxiety-like behaviors in mice. Brain Behav Immun 100:254–266
Merikanto I, Partonen T (2021) Eveningness increases risks for depressive and anxiety symptoms and hospital treatments mediated by insufficient sleep in a population-based study of 18,039 adults. Depress Anxiety 38:1066–1077
Stahl ST, Smagula SF, Rodakowski J, Dew MA, Karp JF, Albert SM, Butters M, Gildengers A, Reynolds CF 3rd (2021) Subjective Sleep Quality and trajectories of Interleukin-6 in older adults. Am J Geriatr Psychiatry 29:204–208
Selvi Y, Boysan M, Kandeger A, Uygur OF, Sayin AA, Akbaba N, Koc B (2018) Heterogeneity of sleep quality in relation to circadian preferences and depressive symptomatology among major depressive patients. J Affect Disord 235:242–249
Taylor BJ, Hasler BP (2018) Chronotype and Mental Health: recent advances. Curr Psychiatry Rep 20:59
Merikanto I, Kronholm E, Peltonen M, Laatikainen T, Vartiainen E, Partonen T (2015) Circadian preference links to depression in general adult population. J Affect Disord 188:143–148
Schapira AHV, Chaudhuri KR, Jenner P (2017) Non-motor features of Parkinson Disease. Nat Rev Neurosci 18:435–450
Stefani A, Högl B (2020) Sleep in Parkinson’s Disease. NEUROPSYCHOPHARMACOLOGY 45:121–128
Morawska MM, Moreira CG, Ginde VR, Valko PO, Weiss T, Büchele F, Imbach LL, Masneuf S, Kollarik S, Prymaczok N, Gerez JA, Riek R, Baumann CR, Noain D (2021) Slow-wave sleep affects synucleinopathy and regulates proteostatic processes in mouse models of Parkinson’s Disease. Sci Transl Med 13:eabe7099
Zuzuárregui JRP, During EH (2020) Sleep issues in Parkinson’s Disease and their management. Neurotherapeutics 17:1480–1494
Hermanowicz N, Jones SA, Hauser RA (2019) Impact of non-motor symptoms in Parkinson’s Disease: a PMDAlliance survey. Neuropsychiatr Dis Treat 15:2205–2212
Kay DB, Tanner JJ, Bowers D (2018) Sleep disturbances and depression severity in patients with Parkinson’s Disease. Brain Behav 8:e00967
Latif S, Jahangeer M, Maknoon Razia D, Ashiq M, Ghaffar A, Akram M, El Allam A, Bouyahya A, Garipova L, Ali Shariati M, Thiruvengadam M, Azam Ansari M (2021) Dopamine in Parkinson’s Disease. Clin Chim Acta 522:114–126
Dauvilliers Y, Schenck CH, Postuma RB, Iranzo A, Luppi PH, Plazzi G, Montplaisir J, Boeve B (2018) REM sleep behaviour disorder. Nat Rev Dis Primers 4:19
Miglis MG, Adler CH, Antelmi E, Arnaldi D, Baldelli L, Boeve BF, Cesari M, Dall’Antonia I, Diederich NJ, Doppler K, Dušek P, Ferri R, Gagnon JF, Gan-Or Z, Hermann W, Högl B, Hu MT, Iranzo A, Janzen A, Kuzkina A, Lee JY, Leenders KL, Lewis SJG, Liguori C, Liu J, Lo C, Ehgoetz Martens KA, Nepozitek J, Plazzi G, Provini F, Puligheddu M, Rolinski M, Rusz J, Stefani A, Summers RLS, Yoo D, Zitser J, Oertel WH (2021) Biomarkers of conversion to α-synucleinopathy in isolated rapid-eye-movement sleep behaviour disorder. Lancet Neurol 20:671–684
Si XL, Gu LY, Song Z, Zhou C, Fang Y, Jin CY, Wu JJ, Gao T, Guo T, Guan XJ, Xu XJ, Yin XZ, Yan YP, Zhang MM, Pu JL (2020) Different Perivascular Space burdens in Idiopathic Rapid Eye Movement Sleep Behavior Disorder and Parkinson’s Disease. Front Aging Neurosci 12:580853
Maggi G, Trojano L, Barone P, Santangelo G (2021) Sleep disorders and cognitive dysfunctions in Parkinson’s Disease: a Meta-Analytic Study. Neuropsychol Rev 31:643–682
Lin YQ, Chen SD (2018) RBD: a red flag for cognitive impairment in Parkinson’s Disease? Sleep Med 44:38–44
Lazar AS, Panin F, Goodman AO, Lazic SE, Lazar ZI, Mason SL, Rogers L, Murgatroyd PR, Watson LP, Singh P, Borowsky B, Shneerson JM, Barker RA (2015) Sleep deficits but no metabolic deficits in Premanifest Huntington’s Disease. Ann Neurol 78:630–648
Vas S, Nicol AU, Kalmar L, Miles J, Morton AJ (2021) Abnormal patterns of sleep and EEG power distribution during non-rapid eye movement sleep in the sheep model of Huntington’s Disease. Neurobiol Dis 155:105367
Cabanas M, Pistono C, Puygrenier L, Rakesh D, Jeantet Y, Garret M, Cho YH (2019) Neurophysiological and behavioral effects of anti-orexinergic treatments in a mouse model of Huntington’s Disease. Neurotherapeutics: The Journal of the American Society for Experimental NeuroTherapeutics 16:784–796
Hulisz D (2018) Amyotrophic Lateral Sclerosis: Disease state overview. Am J Manag Care 24:S320–s326
PubMed Google Scholar
Liu S, Ren Q, Gong G, Sun Y, Zhao B, Ma X, Zhang N, Zhong S, Lin Y, Wang W, Zheng R, Yu X, Yun Y, Zhang D, Shao K, Lin P, Yuan Y, Dai T, Zhang Y, Li L, Li W, Zhao Y, Shan P, Meng X, Yan C (2022) Hypothalamic subregion abnormalities are related to body mass index in patients with sporadic Amyotrophic Lateral Sclerosis. J Neurol 269:2980–2988
Gnoni V, Zoccolella S, Giugno A, Urso D, Tamburrino L, Filardi M, Logroscino G (2023) Hypothalamus and Amyotrophic Lateral Sclerosis: potential implications in sleep disorders. Front Aging Neurosci 15:1193483
Lo Martire V, Caruso D, Palagini L, Zoccoli G, Bastianini S (2020) Stress & sleep: a relationship lasting a lifetime. Neurosci Biobehav Rev 117:65–77
Basta M, Vgontzas AN, Fernandez-Mendoza J, Antypa D, Li Y, Zaganas I, Panagiotakis S, Karagkouni E, Simos P (2022) Basal cortisol levels are increased in patients with mild cognitive impairment: role of Insomnia and short sleep duration. J Alzheimers Dis 87:933–944
Gabery S, Ahmed RM, Caga J, Kiernan MC, Halliday GM, Petersén Å (2021) Loss of the metabolism and sleep regulating neuronal populations expressing orexin and oxytocin in the hypothalamus in Amyotrophic Lateral Sclerosis. Neuropathol Appl Neurobiol 47:979–989
Boentert M (2019) Sleep disturbances in patients with Amyotrophic Lateral Sclerosis: current perspectives. Nat Sci Sleep 11:97–111
Download references
Acknowledgements
We would like to thank Editage ( www.editage.cn ) for English language editing.
This study was supported by grants from the National Natural Science Foundation of China (No. 82371541), the project for the improvement of research skill in Anhui Medical University (No.2021xkjT003), Talent Training Program from the School of Basic Medical Sciences of Anhui Medical University (No. 2022YPJH201), Research Fund of Anhui Institute of translational medicine (No. 2022zhyx-C11) and National Training Project of Innovation and Entrepreneurship for Undergraduates of China (No. 202310366060 & No. 202310366037).
Author information
Xiao Xiao and Yimin Rui contributed equally to this work.
Authors and Affiliations
School of Mental Health and Psychological Sciences, Anhui Medical University, Hefei, Anhui, China
Xiao Xiao & Yimin Rui
Department of Pharmacology, School of Basic Medical Sciences, Anhui Medical University, Hefei, Anhui, China
Xiao Xiao, Yimin Rui, Yu Jin & Ming Chen
You can also search for this author in PubMed Google Scholar
Contributions
All authors contributed to the manuscript. X.X. had the idea for the article. X.X., Y.M.R. and Y. J. performed the literature search, drafted the manuscript and created the figures. M.C. revised critically the manuscript. All authors read and approved the manuscript.
Corresponding author
Correspondence to Ming Chen .
Ethics declarations
Competing interests.
The authors have no relevant financial or non-financial interests to disclose.
Additional information
Publisher’s note.
Springer Nature remains neutral with regard to jurisdictional claims in published maps and institutional affiliations.
Rights and permissions
Springer Nature or its licensor (e.g. a society or other partner) holds exclusive rights to this article under a publishing agreement with the author(s) or other rightsholder(s); author self-archiving of the accepted manuscript version of this article is solely governed by the terms of such publishing agreement and applicable law.
Reprints and permissions
About this article
Xiao, X., Rui, Y., Jin, Y. et al. Relationship of Sleep Disorder with Neurodegenerative and Psychiatric Diseases: An Updated Review. Neurochem Res 49 , 568–582 (2024). https://doi.org/10.1007/s11064-023-04086-5
Download citation
Received : 22 September 2023
Revised : 08 December 2023
Accepted : 09 December 2023
Published : 18 December 2023
Issue Date : March 2024
DOI : https://doi.org/10.1007/s11064-023-04086-5
Share this article
Anyone you share the following link with will be able to read this content:
Sorry, a shareable link is not currently available for this article.
Provided by the Springer Nature SharedIt content-sharing initiative
- Sleep Disorder
- Alzheimer’s Disease
- Parkinson’s Disease
- Find a journal
- Publish with us
- Track your research
Skip to content
Explore CUIMC
Diversity, equity, and inclusion.
At CUIMC, we are committed to continuous improvement in providing culturally inclusive medical education and clinical care.
Columbia Vagelos College of Physicians and Surgeons is dedicated to developing the next generation of leaders in medicine
Patient Care
- Find a Doctor
Search for a provider by specialty, expertise, location and insurance. Schedule an appointment online.
Read the latest news stories about CUIMC faculty, research, and events
Newly Found Genetic Variant Defends Against Alzheimer’s Disease
Discovery could launch new types of drugs to prevent, treat the disease, share this page.
- Share on Facebook
- Share on X (formerly Twitter)
- Share on LinkedIn
- Share by email
Columbia researchers have discovered a genetic variant that reduces the odds of developing Alzheimer’s disease by up to 70% and may be protecting thousands of people in the United States from the disease.
The discovery of the protective variant, which appears to allow toxic forms of amyloid out of the brain and through the blood-brain barrier, supports emerging evidence that the brain’s blood vessels play a large role in Alzheimer's disease and could herald a new direction in therapeutic development.
“Alzheimer’s disease may get started with amyloid deposits in the brain, but the disease manifestations are the result of changes that happen after the deposits appear,” says Caghan Kizil, PhD , a co-leader of the study that identified the variant and associate professor of neurological sciences (in neurology and in the Taub Institute) at Columbia University Vagelos College of Physicians and Surgeons.
“Our findings suggest that some of these changes occur in the brain’s vasculature and that we may be able to develop new types of therapies that mimic the gene’s protective effect to prevent or treat the disease.”
An attractive drug target?
The protective variant identified by the study occurs in a gene that makes fibronectin, a component of the blood-brain barrier, a lining surrounding the brain’s blood vessels that controls the movement of substances in and out of the brain.
Fibronectin is usually present in the blood-brain barrier in very minute amounts, but it is increased in large amounts in people with Alzheimer’s disease. The variant identified in the fibronectin gene seems to protect against Alzheimer’s disease by preventing the buildup of excess fibronectin at the blood-brain barrier.
"It’s a classic case of too much of a good thing,” Kizil says. “It made us think that excess fibronectin could be preventing the clearance of amyloid deposits from the brain.”
The researchers confirmed that hypothesis in a zebrafish model of Alzheimer’s disease and have additional studies in mice underway. They also found that reducing fibronectin in the animals increased amyloid clearance and improved other damage caused by Alzheimer’s disease.
“These results gave us the idea that a therapy targeting fibronectin and mimicking the protective variant could provide a strong defense against the disease in people,” says study co-leader Richard Mayeux, MD , chair of neurology and the Gertrude H. Sergievsky Professor of Neurology, Psychiatry, and Epidemiology.
The newest treatments for Alzheimer’s disease target the amyloid deposits directly and are very efficient at removing the deposits via the immune system. However, simply removing the deposits this way doesn’t improve symptoms or repair other damage.
“We may need to start clearing amyloid much earlier and we think that can be done through the bloodstream,” Mayeux adds. “That's why we are excited about the discovery of this variant in fibronectin, which may be a good target for drug development.”
Protective gene was found in people resilient to Alzheimer’s disease
The researchers discovered the protective variant in people who never developed symptoms but who had inherited the e4 form of the APOE gene, which significantly increases the risk of developing Alzheimer’s disease.
“These resilient people can tell us a lot about the disease and what genetic and non-genetic factors might provide protection,” says study co-leader Badri N. Vardarajan, PhD , assistant professor of neurological science (in neurology, the Gertrude H. Sergievsky Center, and the Taub Institute), who is an expert in using computational approaches to discover Alzheimer’s disease genes.
"We hypothesized that these resilient people may have genetic variants that protect them from APOEe4.”
To find protective mutations, the Columbia researchers sequenced the genomes of several hundred APOEe4 carriers over age 70 of various ethnic backgrounds, including those with and without Alzheimer's disease. Many participants were residents of Northern Manhattan who were enrolled in the Washington Heights/Inwood Columbia Aging Project, an ongoing study that has been conducted by Columbia University’s Department of Neurology for more than 30 years.
The study identified the fibronectin variant, and the Columbia team publicized their results in a preprint for other researchers to view. Based on the Columbia team’s observations, another group from Stanford and Washington universities replicated the study in an independent cohort of APOEe4 carriers, mostly of European origin.
“They found the same fibronectin variant, which confirmed our finding and gave us even more confidence in our result,” Vardarajan says.
The two groups combined the data on their 11,000 participants, which allowed them to calculate that the mutation reduces the odds of developing Alzheimer’s in APOE4 carriers by 71% and forestalls the disease by roughly four years in those who eventually develop the disease.
The researchers estimate that 1% to 3% of APOEe4 carriers in the United States—roughly 200,000 to 620,000 people—may also carry the protective fibronectin mutation.
Wide therapeutic potential
The fibronectin variant, though discovered in APOEe4 carriers, could protect against Alzheimer’s disease in people with other forms of APOE.
“There’s a significant difference in fibronectin levels in the blood-brain barrier between cognitively healthy individuals and those with Alzheimer's disease, independent of their APOEe4 status,” Kizil says.
“Anything that reduces excess fibronectin should provide some protection, and a drug that does this could be a significant step forward in the fight against this debilitating condition.”
More information
The study, “ Rare genetic variation in Fibronectin 1 (FN1) protects against APOEε4 in Alzheimer’s disease ,” was published online April 10 in the journal Acta Neuropathologica.
The research and researchers were supported by the Carol and Gene Ludwig Family Foundation, the Agouron Institute, the U.S. National Institutes of Health (grants R01AG067501, RF1AG066107, R01AG072474, R35GM148348, R01AG061796, U19AG074879, U01AG066752, U01AG046139, R01AG060747, P50AG047366, and R00AG075238), a Schaefer Research Scholars Award, Taub Institute Grant for Emerging Research (TIGER), the Thompson Family Foundation Program for Accelerated Medicine Exploration in Alzheimer’s Disease and Related Disorders of the Nervous System, and an Alzheimer’s Association Zenith Fellows Award.
Richard Mayeux, MD, is also director of the Gertrude H. Sergievsky Center and co-director of the Taub Institute for Research on Alzheimer's Disease and the Aging Brain at Columbia University Vagelos College of Physicians and Surgeons and neurologist-in-chief at NewYork-Presbyterian/Columbia University Irving Medical Center.
Other contributors and funding sources are listed in the paper.
- U.S. Department of Health & Human Services

- Virtual Tour
- Staff Directory
- En Español
You are here
News releases.
Media Advisory
Thursday, April 11, 2024
Study reveals no causal link between neurodevelopmental disorders and acetaminophen exposure before birth
NIH-funded research in siblings finds previously reported connection is likely due to other underlying factors.
Acetaminophen exposure during pregnancy is not linked to the risk of developing autism, ADHD, or intellectual disability, according to a new study of data from more than 2 million children in Sweden. The collaborative research effort by Swedish and American investigators, which appears in JAMA , is the largest of its kind and was funded by the National Institute of Neurological Disorders and Stroke (NINDS), part of the National Institutes of Health (NIH).
Scientists compared siblings — who share genetics and other variables such as parental health, environmental exposures, and socioeconomic factors — and were able to limit the influence of other potential risk factors. This allowed them to focus specifically on, and eliminate, the risk associated with acetaminophen. The study design was unique due to the size of the population captured in the Swedish Medical Birth Register and the Swedish Prescribed Drug Register. Before siblings were considered, there appeared to be a small increase in risk for neurodevelopmental disorders in children exposed to acetaminophen, which was noted in previous studies.
Acetaminophen is commonly used as a pain reliever and fever reducer and is found in a variety of medicines available over the counter and via prescription. It is often taken during pregnancy instead of nonsteroidal anti-inflammatory drugs, known as NSAIDs, which can cause low levels of amniotic fluid, according to the Food and Drug Administration . The reasons pregnant people might take acetaminophen, including fever, or conditions such as chronic migraine, could be, and in some cases are, associated with an increased risk for later neurodevelopmental disorders following pregnancy.
One limitation of this study is that it relies on data from prescribed acetaminophen and from self-reporting from pregnant people during prenatal care. It may not capture all use or dosage in all people, particularly over-the-counter medicines. However, the number of patients included in the study sample and the ability to control for many other confounding factors support the conclusion that acetaminophen is not directly linked to an increase link of autism, ADHD, or intellectual disability.
To inform best preventative strategies, additional research is required to fully understand the genetic and non-genetic factors that increase the risk of autism, ADHD, and intellectual disability.
This study was supported by NINDS (NS107607).
Vicky Whittemore, Ph.D., program director, NINDS
Ahlqvist VH, et al. “Acetaminophen use during pregnancy and children’s risk of autism, ADHD, and intellectual disability.” JAMA. April 9, 2024. DOI: 10.1001/jama.2024.3172
NINDS is the nation’s leading funder of research on the brain and nervous system. The mission of NINDS is to seek fundamental knowledge about the brain and nervous system and to use that knowledge to reduce the burden of neurological disease.
About the National Institutes of Health (NIH): NIH, the nation's medical research agency, includes 27 Institutes and Centers and is a component of the U.S. Department of Health and Human Services. NIH is the primary federal agency conducting and supporting basic, clinical, and translational medical research, and is investigating the causes, treatments, and cures for both common and rare diseases. For more information about NIH and its programs, visit www.nih.gov .
NIH…Turning Discovery Into Health ®
Connect with Us
- More Social Media from NIH

An official website of the United States government
The .gov means it’s official. Federal government websites often end in .gov or .mil. Before sharing sensitive information, make sure you’re on a federal government site.
The site is secure. The https:// ensures that you are connecting to the official website and that any information you provide is encrypted and transmitted securely.
- Publications
- Account settings
Preview improvements coming to the PMC website in October 2024. Learn More or Try it out now .
- Advanced Search
- Journal List
- Diagnostics (Basel)

Modern Methods of Diagnostics and Treatment of Neurodegenerative Diseases and Depression
Natalia shusharina.
1 Baltic Center for Neurotechnologies and Artificial Intelligence, Immanuel Kant Baltic Federal University, 236041 Kaliningrad, Russia
Denis Yukhnenko
2 Department of Social Security and Humanitarian Technologies, N. I. Lobachevsky State University of Nizhny Novgorod, 603022 Nizhny Novgorod, Russia
Stepan Botman
Viktor sapunov, vladimir savinov, gleb kamyshov, dmitry sayapin, igor voznyuk.
3 Department of Neurology, Pavlov First Saint Petersburg State Medical University, 197022 Saint Petersburg, Russia
Associated Data
No new data were created or analyzed in this study. Data sharing is not applicable to this article.
This paper discusses the promising areas of research into machine learning applications for the prevention and correction of neurodegenerative and depressive disorders. These two groups of disorders are among the leading causes of decline in the quality of life in the world when estimated using disability-adjusted years. Despite decades of research, the development of new approaches for the assessment (especially pre-clinical) and correction of neurodegenerative diseases and depressive disorders remains among the priority areas of research in neurophysiology, psychology, genetics, and interdisciplinary medicine. Contemporary machine learning technologies and medical data infrastructure create new research opportunities. However, reaching a consensus on the application of new machine learning methods and their integration with the existing standards of care and assessment is still a challenge to overcome before the innovations could be widely introduced to clinics. The research on the development of clinical predictions and classification algorithms contributes towards creating a unified approach to the use of growing clinical data. This unified approach should integrate the requirements of medical professionals, researchers, and governmental regulators. In the current paper, the current state of research into neurodegenerative and depressive disorders is presented.
1. Neurodegenerative Disorders
Neurodegenerative disorders are a group of slowly progressing irreversible conditions characterized by neuronal death and subsequent atrophy of certain areas of the brain. These disorders predominantly manifest in old age and include Alzheimer’s disease, Parkinson’s disease, Huntington’s disease, Pick’s disease, amyotrophic lateral sclerosis, dementia with Lewy bodies, and other similar other associated conditions [ 1 ]. All of them are characterized by a decrease in cognitive abilities, severe motor impairment, impaired social functioning, and significant difficulties in one’s day-to-day tasks. Currently, neurodegenerative disorders are not curable, and their treatments are aimed at slowing down the disease’s progression, improving the quality of life of patients, and addressing comorbid disorders. The number of people with known neurodegenerative disorders has increased over the past decades. This increase has been predominantly associated with an increase in life expectancy (so, individuals are more likely to develop a disorder during their lifetime) and an increase in the effectiveness of early diagnostics (more cases became known to healthcare systems) [ 2 ]. According to recent data, up to 30% of all people over 85 suffer from Alzheimer’s disease, and 5% of people over 65 suffer from Parkinson’s disease [ 3 ]. This increase in the number of patients as well as the need to utilize a comprehensive approach necessitates the high standards for medical data systems and machine learning algorithms deployed on these systems [ 4 ].
1.1. The Assessment of Neurodegenerative Disorders
The effectiveness of a given therapy in slowing down the progression of neurodegenerative disorders depends on their timely detection. Early detection through screening will allow initiation of treatment before the onset of severe clinical symptoms and significantly delay their onset. In recent decades, pre-clinical assessment has become a major focus of research into neurodegenerative disorders [ 5 , 6 ]. The assessment might take into account behavioral symptoms, biomarkers from blood and cerebrospinal fluid, neuroimaging data (PET and MRI), conductivity and electrical activity of the brain (TMS and EEG), and the results of neuropsychological tests. Often, differential diagnosis is required for depression and conditions that could cause a potentially reversible cognitive decline [ 7 ]. Thus, the number of instrumentally determined markers and parameters is quite large. A feature of most neurodegenerative disorders is the low specificity of individual assessment methods and markers. This often leads to the inability to establish the correct diagnosis without multifaceted and often costly examinations [ 8 ]. Below, we will describe the main groups of diagnostic methods used both to detect the disease and to monitor its progression.
1.2. Biomarkers in Blood and Cerebrospinal Fluid
The main biomarkers used in the differential diagnostics of neurodegenerative disorders, in particular Alzheimer’s disease, are the levels of beta-amyloids and tau proteins in the cerebrospinal fluid of patients [ 9 ]. Using the ratio of the amount of beta-amyloid 1-42 to the total tau index makes it possible to identify patients with neurodegenerative disorders with high accuracy as well as to distinguish patients with Alzheimer’s disease from patients with other forms of dementia with moderate accuracy [ 10 ]. The level of tau protein phosphorylated at position P217 (P217 tau) in blood plasma also demonstrated the potential to be used in differential diagnostics of neurodegenerative disorders, primarily Alzheimer’s disease [ 11 ]. Tau 217 values outperformed other blood biomarkers, as well as MRI markers, in a study of 1402 patients from 3 independent cohorts that included patients with dementia, a cognitive decline of a different nature, and healthy subjects [ 12 ]. The accuracy of the assessment using blood biomarkers in this study was comparable to the accuracy of diagnosis using PET markers. Other studies have also demonstrated that tRNAs could serve as potential biomarkers to detect various neurodegenerative disorders at an early stage [ 13 ].
1.3. Psychological and Neuropsychological Assessment
Psychological (including experimental pathopsychological assessment as practiced in post-USSR countries) and neuropsychological methods for assessing the cognitive deficit present in neurodegenerative disorders are also used to monitor the progression of the disorder and the effectiveness of treatment. The most sensitive to specific cognitive deficits caused by neurodegenerative disorders are short-term memory assessments (e.g., the Visual Short-Term Memory Binding Test) and executive functions assessments. A meta-analysis of 142 trials of sorting tests evaluating executive functions (Wisconsin Card Sorting Test [WCST] and Delis-Kaplan Executive Function System [DKEFS-ST]) demonstrated their high diagnostic validity in detecting neurodegenerative disorders and vascular dementias [ 14 ]. The results of memory and executive function tests, conducted in isolation or as part of standardized neuropsychological batteries, correlate with pathological changes in the medulla as well as with the patient’s overall level of maladjustment. Their use, therefore, represents a cost-effective and non-invasive way to track the progression of the disease. Neuropsychological testing has also demonstrated the potential for the pre-clinical diagnosis of neurodegenerative disorders, especially Alzheimer’s disease. Composite score from the ADCS-PACC scale, which is derived from several neuropsychological tests of memory and executive functions, showed a correlation with the level of beta-amyloids in the cerebrospinal fluid of patients in two studies conducted in the USA and Australia [ 15 ]. These preliminary research results demonstrate that sufficiently sensitive neuropsychological batteries might be used as a non-invasive method for the early detection of neurodegenerative disorders.
1.4. Neuroimaging
The regularity of the spread of the degenerative process in the brain is one of the main criteria for the differential diagnosis of various neurodegenerative disorders. The formation of tau links, as well as a decrease in the volume of certain areas of the brain, correlates with the prognosis and clinical symptoms. These changes are determined using MRI and PET methods. MRI can detect damage to the white matter and a decrease in the local volume of various parts of the brain [ 16 ]. PET allows for assessing changes in glucose metabolism and the presence of neurofibrillary tangles in the brain [ 17 ]. Machine learning methods are currently actively applied to neuroimaging data to minimize diagnostic errors and automate the process. Currently, MRI and PET methods are quite expensive and their use as methods for pre-clinical diagnosis of neurodegenerative diseases is severely limited.
1.5. Connectivity
Transcranial magnetic stimulation (TMS) demonstrated some potential for differential diagnosis in patients with neurodegenerative disorders of various etiologies. In a blinded study of 273 patients (with 421 controls) with Alzheimer’s disease, Lewy body dementia, and frontotemporal dementia, the assessment of cortical connectivity with TMS was conducted [ 18 ]. The researchers then trained a prediction model in the form of an ensemble of the binary decision three classifiers. The model showed extremely high classification accuracy when discriminating between disorders. This study demonstrates the potential of combining TMS and machine learning methods in the non-invasive differential diagnosis of neurodegenerative disorders.
1.6. Electrical Activity of the Brain
Abnormal patterns of electrophysiological activity of the brain in neurodegenerative disorders result from several factors including disturbed functional connections between cortical zones, axon pathology, cholinergic deficiency, and others [ 19 ]. Typical EEG patterns used in screening have been described in the scientific and practical literature [ 20 ]. However, at the moment, the described EEG patterns have not demonstrated high diagnostic accuracy. Potentially, their diagnostic accuracy can be significantly improved through the application of deep learning methods to the analysis of neurophysiological data. Such studies are already being actively carried out [ 21 ].
Thus, the development of algorithms for pre-clinical non-invasive assessment of neurodegenerative disorders and their differential diagnosis remains a promising area of research. The main challenge is to ensure the generalizability and universality of developed algorithms, so they can by deployed using various EEG devices. The other promising direction of research is the application of machine learning methods to the differential diagnosis between neurodegenerative disorders and mild cognitive impairments of different etiology [ 22 ].
1.7. Application of Machine Learning
The process of establishing a diagnosis varies depending on the data about a patient available to a medical practitioner at a particular moment. The results of psychosocial and behavioral assessments are often presented as a small set of tabular data and/or several composite indicators. In this case, the practitioner makes a decision based often on his subjective clinical experience. The evaluation of clinical tests (biomarkers of blood, cerebrospinal fluid, etc.) is often also carried out by the medical practitioner who is provided with the generally accepted reference values. That is, for each set of parameters, there are specific ranges that are the “norm” for a healthy person and numerical indicators of the level of deviations associated with potential health problems. This form of data presentation is easy to interpret and convenient for the practitioner.
However, when interpreting the results of neuroimaging and the analysis of the electrical activity of the brain, not only the amount of data is increased substantially, but the format of the data presentation changes. Instead of concise tabular values, a medical practitioner sees images of nerve tissues or a graphical representation of the electrical signals of the brain. In this form, the data are hard to interpret by a person directly, and to establish a diagnosis, preliminary decoding based on complex groups of instrumental parameters is necessary. The situation complicates further if the assessments are performed periodically and there is a need to track the progression of the disease. In this case, the complexity of a patient’s data increases significantly along with the probability of a medical error, simply because of the large number of parameters examined. Therefore, although the use of neuroimaging (PET and SPECT in particular) is one of the most effective and accurate methods for detecting neurodegenerative disorders at the pre-clinical stage, these methods are rarely employed as part of routine medical screenings [ 23 ]. The problem of analysis of patient data that are too large in volume and too complex for human perception has a potential solution: automation of processing using mathematical pre-processing and machine learning technologies. Modern algorithms can already analyze large volumes of data and produce summary reports with a list of detected signs of a patient’s health problems. Of course, this method has disadvantages: difficulties in creating a training sample of medical data of sufficient size and quality, “opacity” of algorithm predictions (a medical practitioner cannot know why the machine made this or that decision), technological challenges (deployment of such systems in the infrastructure of a medical institution could be a difficult task), social perception (distrust of automatic “assistants” by medical practitioners and patients), and the presence of controversial ethical aspects regarding the use of such technologies in healthcare [ 24 ].
To date, diagnostic systems based on machine learning are rarely used in clinical practice, since the listed disadvantages outweigh their potential advantages. However, a large body of research and development in this area demonstrates that such advanced systems will play an important role in the delivery of palliative care for patients with neurodegenerative disorders. This statement is supported by a significant increase in the number of relevant publications: almost an order of magnitude over the period 2014–2019. It should be noted that at the same time, the proportion of research devoted to deep learning algorithms has noticeably increased, which is illustrated by Figure 1 [ 4 ].

The number of publications on the applications of machine learning ( right ) and deep learning ( left ) to diagnostics of Parkinson’s disease (yellow graph) and Alzheimer’s disease (purple graph) by year (reproduced from [ 4 ]).
In support of this argument, we will further report on some of the applications of machine learning to the assessment and correction of the disorders under consideration. Assistive technologies and methodological approaches will be discussed separately in the relevant sections below.
The use of machine learning for the analysis of blood and cerebrospinal fluid biomarkers is relatively uncommon, and the algorithms used, in most cases, are quite simple from a mathematical point of view. For example, the method of linear discriminant analysis (LDA) has been used to stratify patients by their blood work [ 25 ]. In total, 377 people participated in the study: individuals without symptoms of neurodegenerative diseases (97 individuals), with signs of Alzheimer’s disease (35 + 41 with mild cognitive impairment), with parkinsonism (57 + 29 with cognitive impairment in addition to the usual damage to motor functions), with dementia due to Parkinson’s disease (87), and with frontotemporal dementia (31). Five blood markers were assessed: the levels of beta-amyloids 42 and 40, the total level of tau protein (t-Tau) phosphorylated at position 181 (p-Tau181), and alpha-synucleins. LDA was used to build 2D and 3D linear models from blood work data, and subsequent classification was performed using a random forest (RF) algorithm. The average accuracy of differentiation by categories (Alzheimer’s disease, parkinsonism, and frontotemporal dementia) was 76%, and by subgroups: 83% in the spectrum of Alzheimer’s disease and 63% in the spectrum of Parkinson’s disease. The researchers in another study used cerebrospinal fluid to differentiate patients with Alzheimer’s disease from healthy controls [ 26 ]. They examined the levels of beta-amyloid 42, t-Tau, and p-Tau. The inflammatory proteins were determined by immunoassay. The authors used the LASSO algorithm (linear model with L1 regularization) for feature selection and logistic regression for classification, which reached 0.91 AUC (quantitative interpretation of receiver operating characteristic curve [ROC]). Another study used an alternative set of indicators from cerebrospinal fluid: the presence of elements in the fluid (As, Fe, Mg, Ni, Se, Sr, etc.). Their presence was determined with optical emission spectrometry systems with inductively coupled plasma and sector mass spectrometry. The data were then processed by a support vector machine (SVM) algorithm with a radial kernel [ 27 ]. As a result, the researchers were able to achieve an AUC of 0.76 for the diagnosis of Parkinson’s disease (binary classification versus healthy people) in a sample of 187 people (82 patients with parkinsonism). However, the out-of-sample prediction performance of the resulting model turned out to be quite unusual. At first, the model showed high accuracy when fitted on local patients and extremely low accuracy in people living in geographically remote areas. This was due to the characteristics of the training sample: SVM was trained on the sample of individuals living in the same area. After the model was re-trained using cross-validation with different territories serving as references, the average accuracy of the algorithm reached AUC 0.78, regardless of where the patients came from. The results of the latter work highlight the importance of applying proper training procedures and the need to use a sufficient data sample, even for relatively straightforward algorithms.
The diversity of changes in brain structures in neurodegenerative disorders, including those linked to genetic factors, makes creating computational models a challenge. The performance of the model can sharply decrease if the preliminary classification of pathological changes used to train additional models was performed erroneously. The possibility of automating pre-classification has been explored in detail by A. Young et al. [ 28 ]. The researchers made an attempt to combine algorithms that identify subtypes and the progression of neurodegenerative disorders into a single ensemble of learning models. In addition to a very detailed pre-classification of images by the type of brain changes, the authors created a model that estimated the stage of disease progression. To do this, they introduced time granulation related to the severity of a particular observed parameter within each pre-classified type of brain change. The model was also supplemented with data on genetically caused symptoms (Genetic Frontotemporal Dementia Initiative [GENFI]) that significantly affect the course of the disease. The mathematical model itself was an ensemble of simple linear z-score-based models that performed clustering tasks. For genetically determined frontotemporal dementia, the SuStaIn model was able not only to identify the genotype based on MRI images alone but also to resolve further heterogeneity within specific genotypes. For Alzheimer’s disease, the model was able to identify three subtypes with unique progressions over time. A more detailed specification of the structure of each subtype of disease according to physiological characteristics (e.g., localization and severity of atrophic changes) could be considered a fairly promising approach and, in addition to the work noted above, is covered in [ 29 ].
Peran and Barbagallo [ 30 ] compared MRI image classification algorithms for distinguishing cases of multiple system atrophy and Parkinson’s syndrome in a mixed dataset (29 patients with MSA and 26 with Parkinson’s syndrome) using multimodal MRI. Comparing the algorithms of discriminant analysis, voxel-based morphometry (supervised learning), and Kohonen networks (unsupervised learning), the authors concluded that the higher number of MRI modalities leads to better classification performance regardless of the processing method used.
For Alzheimer’s disease, the published studies [ 31 , 32 ] demonstrated the advantage of analyzing morphological and physiological changes in the brain with deep learning methods using MRI data. For example, Xinyang Feng et al. [ 31 ] compared the performance of a convolutional neural network to the classification based on morphometry markers, cognitive tests, and PET scans obtained from a multimodal dataset containing 975 images of patients with Alzheimer’s syndrome and 1943 images of healthy controls. The accuracy of the model based on the convolutional neural network turned out to be very high (AUC = 0.973). In addition, the developers were able to accurately determine specific anatomical features that turned out to be significant for the classification. It was due to the visually illustrative way of how information is represented in convolutional networks. This feature of convolutional networks was implemented in [ 32 ] as an independent diagnostic tool directly used by a medical professional. The researchers proposed not only to classify MRI data, but also to visually display the detected significant “abnormal” anatomical zones. Their relationship maps with morphological signs of atrophy correlated well with the results from earlier studies. This approach makes the application of the algorithm much more transparent for medical practitioners and increases confidence in its estimates.
To improve the speed of machine learning processing, Leonie Henschela et al. [ 33 ] proposed an algorithm for rapid segmentation of the entire brain into 95 classes using a convolutional neural network. The FastSurfer algorithm proposed by the authors has been commonly used as a means of preliminary data markup. This machine learning pipeline can be used as a standalone classifier and can also be integrated into more complex architectures.
There is an apparent lack of studies into the application of deep learning methods (when the model classifies “raw” data without access to human-designed features) to the analysis of blood and cerebrospinal fluid biomarkers. This can be explained both by the comparative simplicity of this data modality (a limited set of numerical values that is easily interpreted by a person), which eliminates the need to use “complex” solutions, and by insufficient research on this type of biomarker in the context of neurodegenerative disorder diagnostics in general. For example, in [ 34 ], a simple method for analyzing the concentration of p-tau181 in a patient’s blood is proposed, which provides accuracy and specificity sufficient for the preliminary screening of patients with suspected Alzheimer’s disease. However, the specificity of such a single marker is insufficient for a reliable final diagnosis, which makes it necessary to obtain additional tests. At some point, the amount of diverse data necessitates the use of more powerful machine learning methods.
It is important to note that the results of comparative studies show the advantages of deep learning models compared to simpler models in the diagnostics of various symptoms. The accuracy and specificity of deep learning models are higher than those of classical models [ 35 ].
An extensive comparative study of the accuracy and specificity of different algorithms for the detection of Parkinson’s disease was carried out by Mei et al. [ 36 ]. The authors concluded that the classification accuracy of machine learning algorithms was, on average, about ~94% for SPECT, ~86% for PET, and ~87% for MRI (including fMRI). The most used when working with neuroimaging data and the most accurate classifiers were SVM algorithms and artificial neural networks. SVM was used more frequently (50–70% for SPECT or PET and ~60% for MRI) compared to neural networks (22–53% for SPECT or PET and ~23% for MRI). Figure 2 demonstrates different data types and ML models described in the literature.

Data type, machine learning models applied, and accuracy. ( A ) Accuracy achieved in individual studies and average accuracy for each data type. Error bar: standard deviation. ( B ) Distribution of machine learning models applied per data type. MRI, magnetic resonance imaging; SPECT, single-photon emission computed tomography; PET, positron emission tomography; CSF, cerebrospinal fluid; SVM, support vector machine; NN, neural network; EL, ensemble learning; k-NN, nearest neighbor; regr, regression; DT, decision tree; NB, naïve Bayes; DA, discriminant analysis; other: data/models that do not belong to any of the given categories (reproduced from [ 36 ]).
Machine learning methods were also successfully employed in the studies into genetic markers of neurodegenerative disorders. For example, when studying miRNAs and non-coding RNAs, Ángela García-Fonseca et al. [ 37 ] noted the complex relationships of identified sites with specific disorders and outlined the possibility of modelling these relationships. The most effective algorithms were shown to be SVM and various types of decision trees. An example combining manual feature selection and deep learning was provided by Yuan Sh et al. [ 38 ]. As in the previous study, the authors confirmed, using statistical methods of data analysis, the presence of a strong correlation between miRNA expression and ratios of various blood cells. The authors selected the most informative features for their machine learning model. The selected features were associated with the presence of Alzheimer’s disease, mild cognitive disorders, and were also predictive of the disease’s progression. The final model was based on a non-linear neural network and demonstrated the out-of-sample classification accuracy of 91.59%. The area under the curve (AUC) for the control group, the mild cognitive impairment group, and the Alzheimer’s group was 0.97, 0.95, and 0.98, respectively.
Similarly, Jianhu Zhang et al. [ 39 ] suggested working with blood biomarkers. As in the studies described above, feature selection was performed manually based on statistical data. The neural network-based classifier demonstrated an accuracy of about 98%, which is roughly in line with the results shown in previous work.
The general mechanisms linking neurodegenerative and other mental disorders are important to consider for theories that aim to describe the relation between genetic markers and the progression of pathological changes in the brain [ 40 ]. The discovery of common mechanisms for neurodegenerative and mental changes might lead to new research avenues in omics technologies for diagnostics.
The growing popularity of computational approaches in bioinformatics, the relative simplicity of analyzing biological fluids statistically, and the good interpretability of the obtained results will likely lead to a steady increase in the number of studies on the assessment of neurodegenerative disorders using machine learning applied to genetic data. In addition, the possibility of using genetic data with instrumental and sociodemographic variables is attracting more and more attention [ 41 ].
The nature of the data obtained during the neuropsychological examination does not encourage the use of deep learning, so we will focus on classical algorithms. In one study, the use of 9 standardized psychological tests (including the assessment of memory, speech, daily activities, and general cognition) in combination with a test of spatial attention (also to evaluate cognitive abilities) in a sample of 28 patients with Alzheimer’s disease and 50 healthy controls made it possible to achieve a classification accuracy of 91.08% for the SVM algorithm [ 42 ]. The authors also evaluated several other algorithms: RF, gradient boosting (GB), and its variation adaptive boosting (AB). However, the performance of these algorithms was noticeably lower: 81.75%, 85.92%, and 80.75%, respectively. A slightly more sophisticated approach was implemented in another study, where the authors classified the patients into three groups (healthy controls, possible Alzheimer’s disease, and confirmed Alzheimer’s disease) with a two-stage algorithm based on additional modality data [ 43 ]. Initially, the authors identified patients at risk based on biomarkers: levels of cholesterol, blood glucose, high-density lipoprotein, glycosylated hemoglobin, and blood pressure, which was performed using SVM. In the second step, the results of a standardized cognitive ability test (CAT) of the selected individuals were classified by a polynomial logistic regression (LR) algorithm. The accuracy of the SVM classifier was 0.86 AUC, and the addition of LR increased it to 0.89 AUC; these results were achieved on a sample of 2361 patients.
Unfortunately, these and other similar studies are not of particular interest with regard to the objective diagnosis of neurodegenerative disorders. The data on the cognitive and psychological state of a person underlying their predictions have little specificity: similar symptoms (decreased cognitive abilities, lowered mood, etc.) are observed in various disorders with different etiologies. The more promising approach might be to combine data from neuropsychological assessments with data on pathophysiological changes in the brain (e.g., recorded using neuroimaging methods). This would allow not only to maintain the high sensitivity of neuropsychological data but also to significantly increase the specificity of diagnosis. For example, combining the results from the Addenbrooke’s Cognitive Examination, INECO Frontal Screening, and several parameters calculated from MRI data (in particular, volumes of gray matter in different areas of the brain) made it possible to carry out differential diagnosis of Alzheimer’s disease and frontotemporal dementia with an average accuracy of more than 0.91 AUC [ 44 ]. Moreover, despite the use of classic machine learning algorithms (the k-means method for primary feature clustering with LR for the final classification), this approach also demonstrated an outstanding generalizability: the model trained on patient data from one country (Argentina) retained an average accuracy above 0.9 AUC when validated on patients from other countries (Colombia and Australia). In another study, the authors examined different combinations of cognitive and neurophysiological markers to estimate which would perform the best for the differential diagnosis of Alzheimer’s disease (171 patients) and frontotemporal dementia (72 patients). They used a genetic machine learning algorithm for selecting optimal features for subsequent classification using SVM [ 45 ]. The final values of the f-measure (similar to AUC, but more reliable for data with a strong asymmetry in the distribution of class labels) were as follows: only for cognitive factors (memory, speech, attention, etc.), 0.882; only neurophysiological (hypermetabolism foci in various areas of the brain), 0.921; and the optimal combination of traits, 0.949.
The implementation of machine learning methods will likely reduce costs by enabling faster diagnostics as well as reducing the likelihood of medical errors. There are three main areas of application of modern methods of data analysis in the diagnosis of neurodegenerative disorders:
- 1. Increasing the accuracy and coverage of pre-clinical diagnostics. Identification of the pathological process before the onset of clinically observable symptoms will allow treatment to be started in advance, slowing down the progression of the disease and improving the quality of life of the patient.
- 2. Differential diagnosis of various neurodegenerative disorders and comorbidities. Increasing the accuracy of diagnosis will improve the selection of treatment and correction of symptoms.
- 3. Increasing the accuracy of forecasts for the progression of the disease after its onset. The application of machine learning methods to the analysis of longitudinal data will make it possible to build dynamic models for symptom monitoring.
1.8. The Treatment of Neurodegenerative Disorders
Due to the irreversible nature of the degeneration of the nervous tissue, the therapy of neurodegenerative disorders is aimed at slowing down the progression of degeneration and improving patients’ quality of life. Pharmacotherapy slows down degenerative processes through changes in the metabolism of neurons and glial cells. In addition, pharmacological interventions can be aimed at compensating for the functions of destroyed neuron populations. Non-pharmacological interventions are aimed at improving adaptation to the environment and improving the quality of life of the patient as well as helping patients to perform their household tasks. The main approach to non-pharmacological interventions is comprehensive rehabilitation that combines aerobic physical activity, cognitive training, and occupational therapy [ 46 ]. It is also possible to additionally use methods of brain stimulation.
1.9. Pharmacological Therapy
The main drugs used in the pharmacotherapy of neurodegenerative disorders are cholinesterase inhibitors (donepezil, rivastigmine), NMDA receptor antagonists (memantine), and their combinations [ 47 ]. Dopamine receptor agonists (apomorphine), dopamine precursors (levodopa), and monamine oxidase inhibitors (MAO-B) are used to reduce the intensity of motor disorders in parkinsonism (clinical syndrome) [ 48 ]. At the moment of publication, studies into potential neuroprotective drugs are being conducted, but there is no consensus on the use of such drugs in practice.
1.10. Cognitive Training
Exercises aimed at training memory, attention, and thinking have been extensively used as a therapy for individuals with neurodegenerative disorders [ 46 ]. Examples of such training include episodic memory capacity training with mnemonic techniques and visual information processing speed training [ 49 ]. The meta-analysis of 32 studies on the effectiveness of cognitive training in individuals with neurodegenerative disorders showed moderate effectiveness of training for improving cognitive functions [ 50 ]. However, many of the studies included in the meta-analysis were of low quality and had small sample sizes, so the authors cautioned against drawing definitive conclusions. At the moment, the effectiveness of cognitive training for the therapy of neurodegenerative disorders has not been sufficiently studied.
1.11. Physical Exercises
Decreased levels of neurotrophic factors—mainly brain-derived neurotrophic factor (BDNF) and its receptors—are one of the most common physiological consequences of various neurodegenerative disorders. The meta-analysis of 18 randomized trials showed an association of exercise with neurotrophic factor levels in patients with neurodegenerative disorders. Physical exercise increases the level of a neurotrophic factor in blood plasma [ 51 ]. High concentrations of neurotrophic factors reduce the toxic effect of nerve cell death [ 52 , 53 ]. In the studies, an increase in the levels of neurotrophic factors in plasma occurred regardless of the type of exercise: aerobic, strength, or combined exercise programs had similar effects.
1.12. Ergotherapy
Lifestyle counselling, socialization assistance, and re-training of everyday skills are critical for slowing down the progression of neurodegenerative disorders and improving patients’ general quality of life [ 54 ]. In some European countries and the US, occupational therapy is often provided by specialized employees of medical and social institutions (occupational therapists). In Russia, occupational therapy is usually provided by clinical psychologists.
1.13. Brain Stimulation
Methods of TMS and deep brain stimulation (DMS) have not demonstrated significant effects on clinical outcomes in neurodegenerative disorders [ 55 ]. One exception is the use of the DMS method for the correction of motor symptoms in Parkinson’s disease. The DMS stimulation led to a clinically significant decrease in tremor and muscle rigidity in patients.
1.14. Prevention of the Associated Psychological Problems
Affective symptoms (including anxiety and depression) can occur in patients with neurodegenerative disorders. The symptoms could occur as a direct result of dementia, and independently as a reaction to one’s disease or social situation (e.g., as a reaction to impaired communication with relatives) [ 56 ]. Psychosocial interventions could be aimed at facilitating social support and managing the symptoms of anxiety and depression.
Early pre-clinical diagnostics of neurodegenerative disorders will enable the early start of pharmacological therapy, which would slow down the degeneration of the brain matter, as well as cognitive training, which would slow down the development of cognitive deficits. Currently, pre-clinical diagnostics of neurodegenerative disorders using neuroimaging, brain stimulation, and analysis of brain electrical activity is the most promising area for the application of machine learning and data analysis methods.
1.15. Application of Machine Learning
Since the changes in brain matter that occur in neurodegenerative disorders are often irreversible, their therapy is aimed primarily at maintaining the quality of life of patients. Machine learning might be employed to assist with the therapy in several ways: the evaluation of the effectiveness of therapeutic interventions, dynamic monitoring of the disease progression, and the optimization of brain stimulation methods.
When conventional DBS therapy is conducted, continuous pulsation is delivered without adjustment for the patient’s current condition. This approach could lead to sub-optimal results. One possible way to optimize the delivery of pulsation would be to use real-time data on the patient’s condition to select the right moment for stimulation. These data can include information obtained from chemical sensors (e.g., dopamine levels), accelerometers, and ECoG and EMG data [ 57 ]. For example, the occurrence of tremors can be detected in real time by applying a pre-trained classifier to the EMG signal [ 58 ]. In the case of DBS, this approach is called adaptive DBS.
Other methods of pharmacological therapy and physiotherapy used in individuals with neurodegenerative disorders are less invasive compared to DBS. However, since these methods’ effects are not immediate, evaluating their effectiveness can be more challenging. This problem can be solved by adapting the approach used for diagnostics: automated gait analysis [ 59 ] using wearable devices or computer vision systems. A similar approach is used to prevent fall injuries by continuously monitoring and evaluating the probability of falling using a trained regression model [ 60 ]. The analogous solutions for the assessment of neurodegenerative disorders are discussed in the corresponding section.
In conclusion, it is important to note the partial intersection of the proposed methods both in the feature space and in the algorithms being used. There is an emerging approach to aggregate individual markers and even intermediate diagnostic models into higher-level hierarchical ensembles. This approach entails an end-to-end system for processing instrumental data, whose results can then be used in different processes including diagnostics and disease progression monitoring (as depicted in Figure 3 ).

A multilevel approach to working with data on neurodegenerative disorders [ 4 ].
Such an approach, however, is characterized by high computational, technological, and regulatory complexity. To develop such multichannel systems, it is also necessary to address the issues of collecting and pre-processing data, cross-checking the fit of models, and verifying the effectiveness of the analyses.
2. Depressive Disorders
Around 15% of the world population has been diagnosed with a depressive disorder at least once in their life [ 61 ]. The average annual prevalence of depressive disorders among the adult population, according to the World Health Organization, is 5% [ 62 ]. Depression is one of the most common mental disorders. Depression negatively impacts the ability to work, decreases the quality of life, and constitutes a major risk factor for suicide [ 63 ] and other adverse health outcomes [ 64 ]. Subclinical depression may also precede the onset of neurodegenerative disorders [ 65 ].
2.1. Diagnostics of Depressive Disorders
Depressive symptoms can have many etiologies, and their potential neurophysiological mechanisms have not been well understood yet. The current consensus is to consider depressive states as a consequence of poorly differentiated functional, neurotransmitter, and metabolic changes in the brain [ 64 ]. Since there is no specific localized pathological process in the nervous tissue in depressive disorders, neuroimaging methods for the diagnosis of depression have been rarely used in practice. Biomarker studies of blood and cerebrospinal fluid have been also rarely used. Diagnosis of major depressive disorder, minor depressive disorder, or bipolar depression is usually based on the results of clinical interviews and standardized questionnaires. The potential use of brain electrical activity data to diagnose depression and predict therapeutic responses to antidepressants is currently the subject of active research.
2.1.1. Use of Standardized Questionnaires
In addition to the clinical interview, standardized questionnaires are used to assess the severity of depressive symptoms. In Russia, among others, the following are used for this: the Beck Depression Inventory (BDI) [ 66 ], the Hamilton Scale (HAM-D) [ 67 ], and the Hospital Anxiety and Depression Scale (HADS) [ 68 ]. The diagnosis of a depressive disorder is not made solely based on questionnaires. Their results serve as a guide for a psychiatrist in determining the severity of symptoms and finalizing the diagnosis. The final diagnosis is based on the combination of identified symptoms that fit the diagnostic criteria of the International Classification of Diseases. However, the periodic completion of questionnaires by the patient can be used as a relatively objective way to track the dynamics of depressive symptoms to adjust the course of treatment.
2.1.2. Analysis of the Functional Activity of the Brain (EEG)
Methods for analyzing brain activity can be used both to diagnose depressive states (in particular, to assess the severity of depression) and to predict the response to pharmacological treatment. For example, a decrease in interhemispheric coherence of the frontal leads, observed regardless of the etiology of the depressive state, has been identified as a potential predictor of resistance to pharmacotherapy [ 69 ]. Identification of predictors of drug resistance and response to drugs is one of the directions of research on the use of EEG methods in the diagnosis of depression [ 70 ].
2.1.3. Application of Machine Learning
Attempts have been made to use neuroimaging data (MRI, functional MRI, and diffusion MRI) [ 71 ] and brain electrical activity (EEG) data [ 72 ] to diagnose depressive disorders. The recorded data were analyzed with classical machine learning algorithms (e.g., the support vector machine) and deep learning methods [ 73 ].
The main types of data sources and methods used in the assessment of various mental disorders, including depression, were described in the review by Chung and Teo [ 74 ]. The authors analyzed several works on the assessment of anxiety and depression based on written texts, voice recordings, data from MRI scans, survey methods, and their combinations. The paper noted that different algorithms were better suited for different types of data (they examined gradient boosting, random forest, and neural networks), but the average accuracy of the best-performing algorithms did not exceed 80%. The authors attribute the low results both to the small amount of data and to incomplete or imbalanced training datasets. Chen et al. [ 75 ] also noted the need to use additional data channels, including voice, activity, sleep, questionnaire, and instrumental methods (e.g., MRI) to potentially improve the accuracy of diagnosis. Additional data channels can also represent different aspects of everyday activity to add behavioral patterns into the data. The authors emphasized the promise of multimodal datasets, since various mental disorders are often accompanied by external signs progressing with time ( Table 1 ).
Multimodal data features and their uses in mental health [ 75 ].
The use of multimodal data potentially makes it possible not only to diagnose a wide range of mental disorders, but also to monitor the dynamics of the development of the disease, since the observed signs become more pronounced at later stages. However, the collection of multimodal data is often associated with a number of difficulties, in particular with the need to observe the patient for a long time, as well as to confirm the observed signs by cross-checking on historical data performed by an experienced doctor. Big data collected from mobile devices reflect the patient’s social interactions and fine motor skills features such as taps duration, typing speed and rhythm, reaction speed, etc. It is worth noting that in order to automatically collect such an amount of data, the mobile application installed on the patient’s device should have access to the correspondence and system software, which can become a security issue. However, such solutions can be easily implemented on the architecture of social interaction platforms such as social networks. The authors proposed the implementation of platform solutions for big data as the main processing unit. The units then are used as a basis for more complex models. In addition, the authors proposed a machine learning model that can be used to evaluate the effectiveness of treatment and to monitor the patient’s condition using biofeedback.
One of the features of mental disorders, when compared to other disorders, is that their assessment relies heavily on the subjective experience of a patient subjectively described by him or her. The quality of the assessment is highly dependent on the clinical experience of the medical professionals conducting the assessment. To systematize the data collection process of mental illness clinical signs, a general methodology for filling the database is needed. The same methodology, on the other hand, forces doctors to use standardized data collection forms, which positively affects the entire dataset. The fact is that the different experiences of doctors and the peculiarities of the method of presenting the picture of the disease cause certain markup anomalies in the database [ 76 ]. Often, this feature does not allow one to reasonably assert that the sample data are not biased. In addition, the amount of relevant data differs from channel to channel, which often requires the deployment of different cleaning and pre-processing algorithms.
In their review of machine learning methods for neuroimaging, Quaak et al. [ 77 ] pointed out the need for a more conservative approach to testing the quality of trained models, since their results on the test set often do not reflect the model’s real performance. In addition, the authors noted the popularity of the EEG as a source of data for the diagnosis of mental disorders. MRI/EEG databases for diagnostics of depression, which are used to train machine learning algorithms, can be recorded in different modes: when a patient is performing certain tasks (game), responding to external stimuli (video, images, and music), and in a resting state. The most common approach is to receive data from a person in a resting state (eyes open or closed).
The information about the patient’s depression is often considered protected private information, which leads to very few datasets being openly available. The information on openly available datasets for diagnostics of depression by EEG is presented in Table 2 .
Available open datasets for diagnostics of depression by EEG.
The common problem with openly available datasets is that they often include a limited number of participants (the average sample size of patients with depressive disorder is around 30 people). This problem calls into question the diagnostic value of studies conducted using the datasets. This problem can be mitigated either by combining existing datasets [ 82 ] or by the collection of more data. The latter option requires substantial resources.
Convolutional neural networks are the most common types of neural networks for classifying the presence or absence of a depressive disorder. They rely on a two-dimensional or one-dimensional convolution operation, or hybrid models of convolutional and recurrent neural networks [ 83 ].
The input of the neural network is either the raw EEG signal (after pre-processing in the form of filtering and denoising) or its converted version. Given the existence of well-known models of neural networks used for image classification, the transformation often consists of the formation of an “image” from the EEG signal, which is then fed to the input of a two-dimensional convolutional network. To form an “image”, the power values of brain rhythms can be used, which are spatially projected onto a plane, following the location of the electrodes [ 84 ].
Since deep learning algorithms are demanding towards an amount of training data, and the existing samples of training data are often of limited size, the augmentation method is applied. Augmentation is a method of artificially increasing the amount of data used for training [ 85 ]. The use of data augmentation in training deep neural networks can reduce the effect of overfitting and improve accuracy and stability. EEG data augmentation uses sliding window data sampling, data from generative models, noise addition (generally Gaussian noise is used), sampling, segment recombination, and Fourier transform [ 86 ]. The architectures of neural networks and the achieved accuracy of depression detection according to EEG data are presented in Table 3 .
EEG classification accuracy for depressive disorder.
It should be noted that the claimed high accuracy of depression classification, exceeding 90%, may be associated with testing models on a limited set of initial data and possible incorrect partitioning of training data by patients, which might have led to the implicit leakage of training data into the test subset. Leakage of this kind leads to overestimated accuracy and reduces the generalizability of the model due to the effect of overfitting [ 99 ].
The application of methods for automated assessment of depressive disorder severity involves the selection of relevant features of the received signal and the development of complex metrics of the depressive state based on them. Isolation of non-linear spectral characteristics of EEG signals in combination together with the support vector machine methods demonstrated high classification accuracy in several studies [ 100 ]. The combination of linear discriminant analysis and genetic algorithms also demonstrated high discrimination performance when classifying patients with the depressive disorder [ 101 ]. This line of research can be developed further by incorporating new diagnostic categories as comparison groups and the development of classifiers with higher ecological validity.
The development and validation of composite diagnostic indices, as well as ensembles of algorithms for solving specific diagnostic problems, are most often carried out on small samples in the absence of independent external validation. This creates a high risk of overfitting the algorithms and reduces the generalizability of the results. To increase the validity in the development of EEG-based diagnostic indices, attention should be paid to the composition of a training sample, its size, and its quality. The use of the combination of neurophysiological data labelled according to a single protocol from different clinical sites could be an optimal solution.
2.2. Treatment of Depressive Disorders
The main methods of treatment of depressive disorders include pharmacological therapy and psychosocial interventions (psychoeducation and group and individual psychotherapy) [ 102 ]. Biofeedback and TMS are less commonly used. In individuals with depression, relevant therapeutic targets include maladaptive thoughts and beliefs, lowered mood, quality of life, and others. Reducing the risk of suicide in severe depression is also an important therapeutic goal.
2.2.1. Pharmacological Therapy
The most common medication used for treating depression is serotonin reuptake inhibitors (e.g., sertraline and paroxetine), tricyclic antidepressants (citalopram and fluoxetine), and monoamine oxidase inhibitors (moclobemide and pirlindole). The mechanism of action of antidepressants is based on a change in the concentration of neurotransmitters available for binding in the brain, which leads to long-term potentiation or depression of synaptic connections [ 103 ]. The choice of medication is made based on their safety and the observed therapeutic effect in a particular patient. Doses are adjusted by the medical professional during treatment. Antipsychotics can also be used in depressive states that have arisen as part of psychotic disorders (e.g., bipolar disorder) [ 104 ]. Pharmacological therapy is usually only required for moderate to severe depressive disorders. The combination of pharmacological therapy and psychotherapy is optimal for the treatment of such forms of depression [ 105 ].
2.2.2. Psychosocial Interventions
The main psychosocial intervention for working with patients with mild depression is psychoeducation, providing information about the symptoms and the possible progression of the disorder, recommendations for self-help, and lifestyle changes (if necessary). Other suitable psychosocial interventions for patients with depressive disorders are group and individual psychotherapy. The most studied approaches for the treatment of depression are cognitive behavioral therapy and its variations, psychodynamic therapy, schema therapy, and decision-oriented therapy [ 106 , 107 ]. In general, psychotherapeutic approaches do not differ significantly in effectiveness, so the choice of a particular approach in each case depends on the availability of a specialist and the individual preferences of the patient. Besides psychotherapy, regular physical exercise could also be considered a part of a psychosocial intervention. Regular exercise has been shown to be effective in reducing depressive symptoms [ 108 ]. Positive changes may result from the metabolic and hormonal changes that accompany regular exercise.
2.2.3. Biofeedback
Biofeedback methods have also been shown to be effective in reducing depressive symptoms. A meta-analysis of 14 randomized controlled trials in 794 subjects showed significant improvements in symptoms in patients with depression [ 109 ]. Neurofeedback using EEG and fMRI demonstrated promising results in reducing depressive symptoms, but the number of published studies is still limited [ 110 , 111 ].
2.2.4. Brain Stimulation
Studies demonstrated that transcranial magnetic stimulation had a potentiating effect on antidepressant intake and was associated with improved clinical outcomes [ 112 ]. TMS is recommended by several national medical agencies for use in the treatment of depression as a procedure with potentially positive results and no side effects [ 113 ]. However, there is currently insufficient data to draw definitive conclusions about the effectiveness of TMS in reducing depressive symptoms.
2.2.5. Application of Machine Learning
Machine learning is also used to assess the treatment effectiveness in depressive disorders [ 114 ]. The effectiveness of depression treatment using antidepressants or TMS can be assessed by using and applying classical machine learning methods (support vector machine [ 115 ] and random forest [ 116 ]) or deep neural network [ 97 ] algorithms to EEG data [ 117 ].
The machine learning methods used and the accuracy achieved in predicting depression treatment based on EEG data are presented in Table 4 .
EEG predictive accuracy of treatment for depressive disorder.
Studies on the predictive treatment of depression, as well as studies on the diagnosis of depression, are characterized by a limited number of participants, which does not allow us to assert that the methods used have sufficient generalizability. At the moment, the most promising areas for the development of applied methods of machine learning for the therapy of depression are dynamic monitoring of the symptoms and treatment of pharmacoresistant depression.
3. The Use of Auxiliary Indicators
Along with well-established instrumental methods, such as MRI, fMRI, and EEG, it became possible to automatize the use of several auxiliary diagnostics indicators. These indicators had been used as visually observable markers of a disorder and were usually determined by a medical professional during direct contact with a patient. At the same time, the evaluation of the severity of these indicators and their association with the symptoms were always performed by medical professionals based on their subjective experience. Such auxiliary indicators include both observed disturbances in motor functions (gait, gross and fine motor skills, the ability to speak and swallow, etc.) as well as their various combinations with cognitive impairments. Automating the processing of such indicators is a promising direction of work for machine learning specialists since relevant training datasets are easy to collect and label, e.g., based on neuroimaging methods. For some types of motor disorders, researchers have already obtained promising results [ 120 , 121 , 122 , 123 ].
Instrumental methods for determining gait disorders, which are quite pronounced, in particular for Parkinson’s disease cases, are based on data from video cameras and sensors of wearable devices. Wearable devices are relatively easy to construct, and it is possible to use them outside the clinical setting to collect more data. On the other hand, small-sized three-axis accelerometers and inertial systems most often used for such solutions are subject to external interference because the device is loosely attached to the body and interacts with clothing and furniture. The daily activity data obtained from such devices are poorly interpreted outside the context of the situation. Nevertheless, the results of comparative experiments using such devices and machine learning methods look quite promising.
For example, Dante Trabassi et al. [ 122 ] provided a comparative analysis of various machine learning algorithms on a dataset from inertial sensors of a wearable device to detect gait anomalies which are common for Parkinson’s disease. The trials were conducted with 81 subjects with Parkinson’s disease and a control group of 80 subjects. The test protocol was the same for the test and control groups and included walking along a building corridor which was about 30 meters long with a wearable device in the form of a sensor on the belt. The collected data was pre-processed manually and then used to train several different machine learning models, such as decision trees, random forests, k-nearest neighbors, support vector machines, and artificial neural networks. The best result with an accuracy of 0.86 was achieved using an algorithm based on the support vector machine. At the same time, artificial neural networks showed a lower accuracy, comparable to a random forest.
In contrast, in an article by Robbin Romijnders et al. [ 123 ], the best result (classification accuracy of about 98% and classification completeness >95%) was shown using artificial neural networks. In this publication, a different approach to sensor placement was used, and gait features were reconstructed based on data from accelerometers and position sensors placed on the lower leg and ankle. The study used a larger dataset of 157 subjects with several disorders (Parkinson’s disease, multiple sclerosis, recent stroke, and chronic low back pain) and a larger test program that included the passage of a five-meter segment at a randomly defined slow, medium, and fast pace. Deep learning using temporal convolutional networks was chosen as the primary algorithm for this research. The high result shown allows us to conclude that this approach is suitable both for the preliminary screening of patients and for monitoring the dynamics of disease development.
Jinglin Sun et al. [ 120 ] proposed a similar approach for the diagnosis of Alzheimer’s disease, but eye movements were used as the primary source. A comparative study was conducted for classical machine learning methods (support vector machine and k-nearest neighbors) and well-established neural network architectures VGG-16 and Resnet-18, and for the architecture proposed by the authors based on a dual autoencoder module with a separate final classifier in the form of a fully connected three-layer network. Mean accuracy values of 0.87 ± 0.04 and recall values of 0.89 ± 0.04 were demonstrated, respectively.
Chonghua Xue [ 124 ] proposed to use and analyze patients’ voice recordings by convolutional and recurrent neural networks for the detection of Alzheimer’s disease. This architecture is well suited for time-distributed data; therefore, it is often used in voice analysis.
A number of works proposed an analysis of behavioral responses for the diagnosis of depression [ 125 , 126 ]. In such experiments, a patient is asked to determine the emotional coloring of various words and images that are demonstrated by a special program. The accuracy of the classifier based on bagged decision trees, trained on a sample with manual feature selection, reached 0.76, which does not allow us to speak of a stable result.
The issue of predicting and early diagnosis of cognitive dysfunctions in the elderly using machine learning methods on various data, including sociodemographic data, electronic medical records, clinical and psychometric studies, and neuroimaging data, is considered in a review paper [ 127 ]. Sarah Graham et al. highlighted the need to use multimodal datasets and maintain the transparency of the algorithms. They also noted the direct comparative analysis of the effectiveness of clinical methods for the early diagnosis of cognitive impairment and new methods based on machine learning have not yet been carried out.
A growing number of studies on the potential application of machine learning highlights the need of a general approach to evaluate such potential applications. Without determining the existing barriers to the introduction of technology, its features, strengths, and weaknesses, and understanding the methods for ensuring its safe use, the implementation of such methods in clinical practice will be difficult [ 128 ]. For most countries, the procedures of state regulators regarding the introduction of new medical instruments, as well as ethical issues related to the low transparency of the results of the model and the specific features of collecting and processing medical data, significantly slow down the introduction of systems based on machine learning. This was especially evident during the COVID-19 pandemic when many predictive, diagnostic, and clinical models were created, but only a few point developments reached successful practical use in medical practice. From a technical point of view, when developing solutions based on machine learning, it is necessary to carefully check all the main stages of data collection and preparation, justify the choice of a specific algorithm for solving the problem, and explain the results obtained [ 129 ].
Machine learning methods are gradually becoming an increasingly mature research tool in areas such as radiology, immunology, and the synthesis of new drugs [ 130 ]. There are studies on the application of machine learning methods in general, and in relation to the tasks of diagnosing neurodegenerative and mental diseases. In particular, there are a number of inter-related works that are being actively studied.
The issue of achieving consensus between several experts when labeling data and the features of training models on such a set is covered in [ 131 ]. The authors managed to get the convolutional neural network to reproduce the manual data markup features that are specific to each expert. Thus, the algorithm imitates the consensus of experts in the form of an ensemble of layers trained on separate markups.
The phenomenon of sustainable overestimation of the effectiveness of machine learning methods developed for use in psychiatry was studied in [ 132 ]. The authors noted that in the Predictive Analytics Competition (PAC-2018), in which participants were asked to identify depression from MRI scans, the results were between 60% and 65% on a large independent dataset, while on smaller datasets, the accuracy reached 80% or more. A more detailed study showed a steady overestimation of the accuracy of the model when testing on small sample sizes. For simple methods, in which the number of hyperparameters is small, with the correct tuning, the model is trained quite quickly, and with an increase in the size of the training sample, no further noticeable increase in accuracy and recall is observed. At the same time, many researchers split the dataset in such a way that as much of the data as possible is used in the training process. Given the relatively small number of publicly available datasets for training, the question of the realism of the declared high performance of the developed models when tested on a new larger independent dataset becomes increasingly relevant.
Based on the foregoing information, machine learning methods in no way can be considered a universal recipe for a diagnostician or therapist. A systematic approach has yet to be formulated and, at the moment, there are a number of shortcomings that are characteristic of not fully formed methodologies. Functionally, the first group can be attributed to “data and methods”, and the second to “the interpretability and trust”.
First, there is no generally accepted method for collecting information on the course of neurodegenerative diseases, on the basis of which it would be possible to collect a uniform and consistent dataset. As a result, available datasets described in the literature are often fragmentary and unbalanced or contain a limited number of respondents. The average sample size in terms of the number of respondents is about 500 people. The datasets themselves may contain more detailed information about each patient, but for a relatively small number of respondents. Thus, the dataset in [ 27 ] was collected from 277 patients. The largest dataset by the number of patients includes several thousand images but belonging to different patients [ 31 ]. It is worth noting the difficulty of tracking disease development because of data format limitations since they reflect the clinical situation only at a specific point in time.
To use the data in training models, it is required to clean them, balance by classes, and correctly split them into training and test sets. In addition, manual feature engineering based on the experience of the investigator and known patterns becomes important. Thus, in heterogeneous datasets, the features are difficult to validate and augment with data from other patients to increase the number of features. Augmentation with synthetic data is a promising and effective method, applicable for image [ 85 ] and time-series EEG data [ 86 ]. The selection of features for machine learning also depends on the characteristics of the dataset. A comparative study of some open datasets is given in [ 114 ]. Comparison of datasets, model’s accuracy, and participant-to-feature ratio is shown in Figure 4 .

Balanced accuracy and participant-to-feature ratio in published machine learning studies of outcome prediction in the treatment of MDD. The x-axis plots the ratio of participants to predictive features. The y-axis plots the mean balanced accuracy within each study. Studies predicting response, remission, and TRD are plotted as circles, diamonds, and triangles, respectively. Adequate quality studies are highlighted with large, filled symbols. The dark gray horizontal dashed line shows the mean balanced accuracy of the eight adequate quality studies. The pale gray horizontal dashed line shows the average balanced accuracy of the other 45 studies (reproduced from [ 114 ]).
After data preparation, the model learns on the samples in the training set. As data differ in structure and volume in different sets, selection of a machine learning model becomes a non-trivial task. In particular, it is difficult to control the quality of learning in small datasets. In addition, the generalization ability of models is often overestimated due to the small sample size and possible overfitting, which was described in detail above. As a result, researchers offered a large number of models, with different architecture and characteristics. Such a cardinal difference does not allow for an adequate quantitative comparison for ensembled models, despite the simplicity of models such as decision trees or k-nearest neighbors.
Su et al. in [ 41 ] confirmed the complex nature of data mining and processing task for genetic and transcriptomic data on PD. The authors summarize the remaining limitations and challenges, and accordingly discuss potential future directions which may lead to promising machine learning approaches to address the discovered issues. Table 5 demonstrates several common points about dealing with mixed datasets and additional methods, applied in sequence both for the data and the model. The separate point on the interdisciplinary issue highlights the need for better collaboration and better domain expertise, as an expert’s opinion is still often the best ‘ground truth’ source.
Summary points of challenges and potential future directions to address them [ 41 ].
The issues of interpretability and trust to algorithms, listed in Table 5 , can be broadened in several aspects. First, the regulatory framework for the use of machine learning models in clinical practice is rather incomplete. This is inherent not only in the diagnostics and treatment of neurodegenerative diseases, but rather in the clinical approach as a whole. As noted in [ 76 ], the methodology for processing mental disorders data for precision medicine approach is only being formed and it will take time to develop it. Second, whenever we use an artificial intelligence approach in healthcare, it is always related to making a decision. Disease detection, disease prediction, drug repurposing, precision medicine, medical resource allocation, and much more can be shown as evidence for it. In all of them, a machine learning algorithm either makes a decision or at least supports a decision. The system works as a complex visualization and analyzing tool to catch patterns in medical data [ 129 ]. The true reason why the recommendation system supports one decision or another is often hidden from the user. So, the questions of ethics and potential software issues arise and stay unresolved before medical systems become more mature and common in clinical practice. Quality and integrity checks of data, models, and protocols can help to speed up the development of ML-based medical systems and discover potential faults during development and trial stages.
In a study by Vinny et al. [ 133 ], the authors critically examined the main issues of quality assessment and the possibility of implementing solutions based on machine learning models. The authors noted the need for a consistent multistage analysis of each of the components of the solutions offered to physicians, starting with the dataset, its size, completeness, representativeness, method and protocol of collection, and ending with the re-checking of the demonstrated characteristics of the model and the method of their determination. Separately, the authors noted the importance of transparency, the interpretability of the applied model, and the “common sense” check. The authors formulated the main provisions in 14 questions as shown in Table 6 .
Summary of key questions in critical appraisal of a machine learning research paper [ 133 ].
4. Conclusions
The promising areas of research outlined in this review are currently being explored by various scientific groups around the world. The main factor limiting research in the identified areas is the lack of data of adequate quality and volume for training and validating machine learning models. The introduction of common standards for collecting, labeling, and storing data in shared databanks will allow the development of methods for the early diagnostics and correction of neurodegenerative and depressive disorders.
Funding Statement
This work was performed within the scope of the Agreement #FZWM-2020-0013.
Author Contributions
Conceptualization, N.S. and I.V.; methodology, D.Y. and D.S.; validation, S.B., D.S., N.S. and D.Y.; data curation, S.B., V.S. (Viktor Sapunov), D.Y.; writing—original draft preparation, D.Y., S.B., V.S. (Viktor Sapunov) and V.S. (Vladimir Savinov); writing—review and editing, S.B., D.Y., D.S., G.K., N.S. and I.V.; supervision, N.S. All authors have read and agreed to the published version of the manuscript.
Data Availability Statement
Conflicts of interest.
The authors declare no conflict of interest.
Disclaimer/Publisher’s Note: The statements, opinions and data contained in all publications are solely those of the individual author(s) and contributor(s) and not of MDPI and/or the editor(s). MDPI and/or the editor(s) disclaim responsibility for any injury to people or property resulting from any ideas, methods, instructions or products referred to in the content.
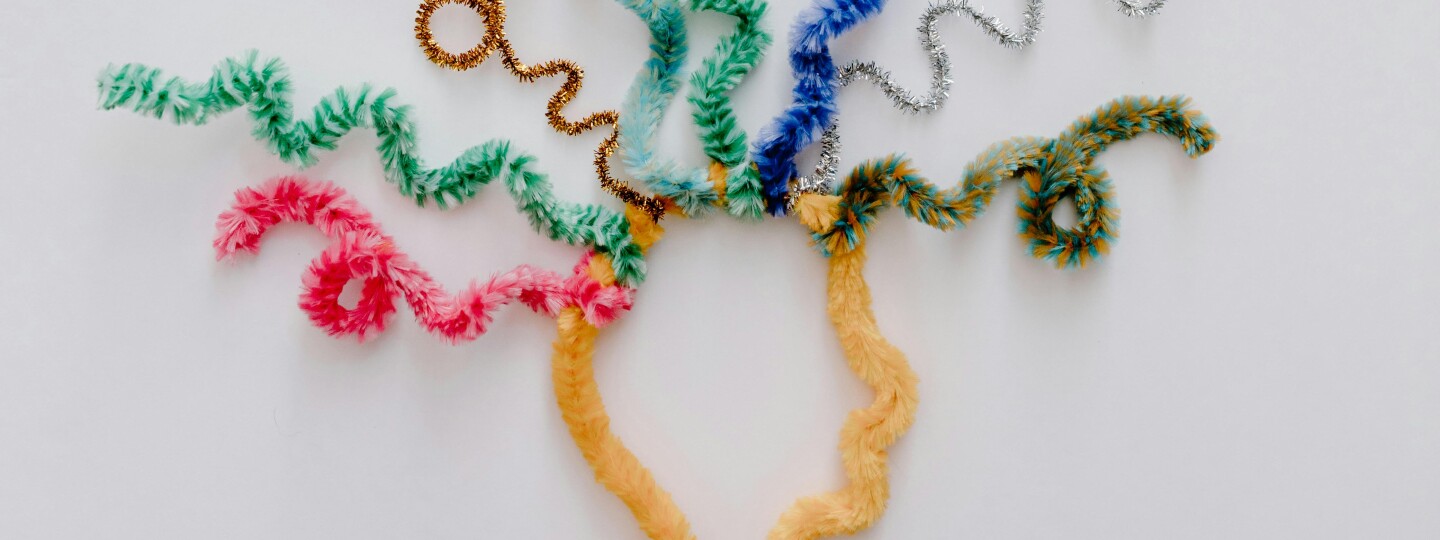
Stirring up emotions – Parkinson’s disease alters emotion-related bodily sensations
Researchers at the University of Turku and Turku University Hospital in Finland have shown that bodily sensations related to emotions are altered by the neurological disorder Parkinson's disease.
Emotions have a major impact on the way we act, and they regulate our hormones and many of the body’s vital functions. Emotions can also be associated with strong physical reactions and sensations, such as an increase in heart rate and blood pressure when a berry picker encounters a bear in the woods or butterflies in the stomach when going on a first date. Emotions are also reflected in the symptoms of many neurological and psychiatric disorders, with negative emotions in particular often increasing the symptoms of the disease.
Parkinson's disease is a neurological movement disorder that is characterised by motor symptoms, such as slowness, stiffness, and tremor. Parkinson's disease is also associated with a number of non-motor symptoms, such as depression, anxiety, and dysfunction of the autonomic nervous system. Dysfunction of the autonomic nervous system has an influence, for example, on the blood circulation and gastrointestinal tract function.
Doctoral Researcher and physician specialising in neurology, MD Kalle Niemi and his colleagues investigated the bodily sensations of basic emotions (anger, disgust, fear, happiness, sadness, surprise, and neutral) in Finnish Parkinson's disease patients.
The subjects were asked to identify their symptoms and bodily sensations associated with different emotions by drawing them on an electronic human body map using a computer mouse.
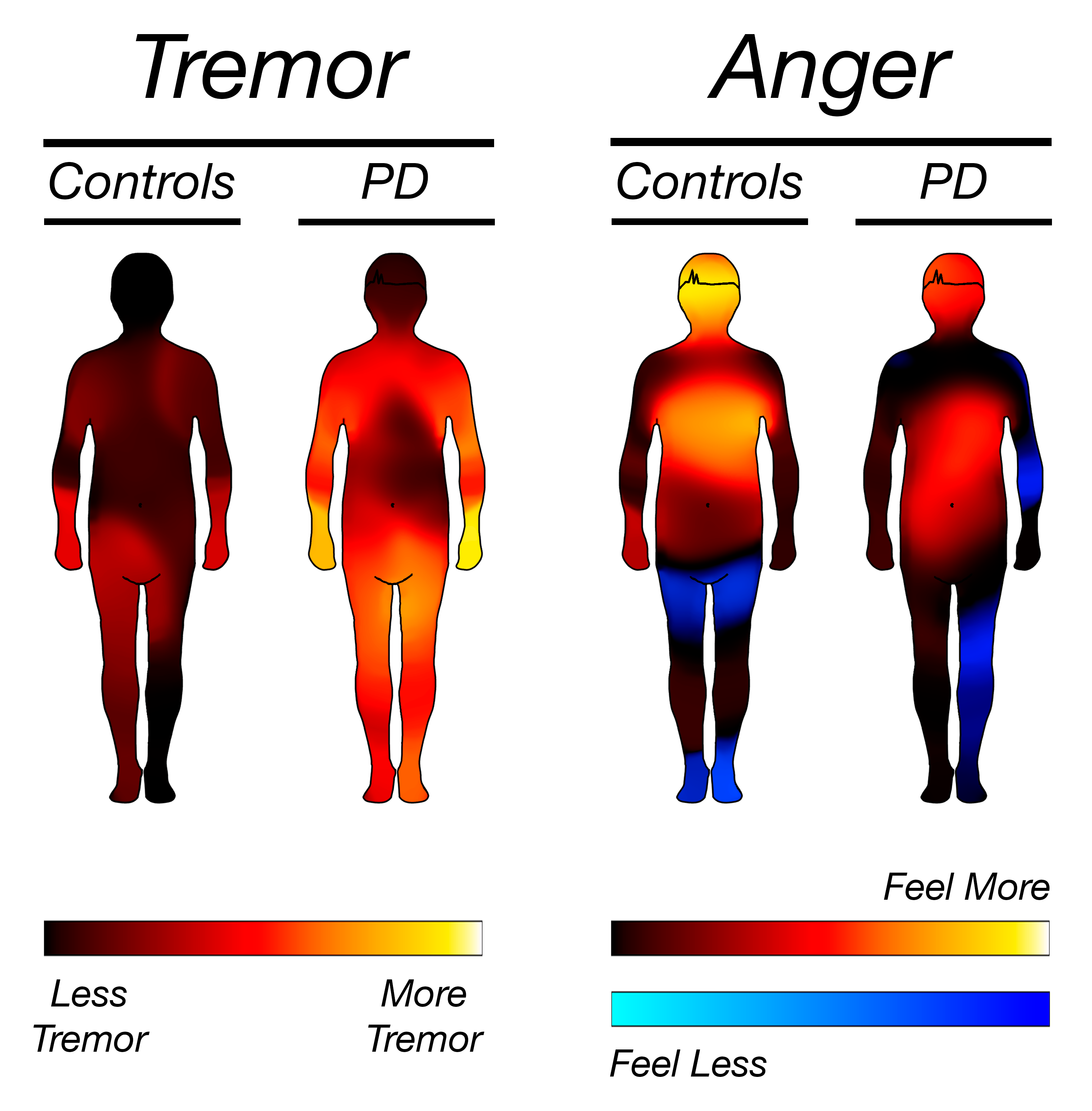
The descriptions of the Parkinson's disease patients on their emotion-related bodily sensations differed from those of the control subjects. Photo: Clinical Neurosciences, University of Turku
People with Parkinson's disease were found to have significant differences in all bodily sensations related to basic emotions when compared with the control subjects. The differences were most pronounced in the bodily sensations of anger, which in healthy people are focused in the chest area. In people with Parkinson's disease, the bodily sensation of anger in the chest was reduced and seemed to shift more to the abdominal region as the disease progressed, consistent with the dysfunction of the autonomic nervous system associated with Parkinson’s disease.
“In recent years, there has been a growing realisation that the non-motor symptoms of Parkinson's disease have a significant impact on the patients’ quality of life. The results of our study highlight yet another non-motor phenomenon," says Niemi.
Emotional abnormalities are common in psychiatric disorders, but this study is the first to show abnormalities in the emotion-related bodily sensations in a neurological disorder. The results may open up new perspectives into the symptoms and possibly even treatment of symptoms in neurological disorders.
“The results of our study raise many interesting questions about the role of emotions in the symptoms of Parkinson's disease. Extending our research method to other diseases offers new possibilities for neurology research," summarises Juho Joutsa , Professor of Neurology at the University of Turku and principal investigator of the study.
The research article Bodily maps of symptoms and emotions in Parkinson's disease has been published on 8 April 2024 in Movement Disorders , the most prestigious journal in movement disorder neurology.
More information:
Doctoral Researcher Kalle Niemi University of Turku [email protected]
Professor Juho Joutsa University of Turku [email protected]
Image for media:
The photos are free to use in news concerning the topic. Please credit the photographer or the publication when using the photos.
> The descriptions of the Parkinson's disease patients on their emotion-related bodily sensations differed from those of the control subjects . Photo: Clinical Neurosciences, University of Turku
Read the original research article
You might also be interested in.
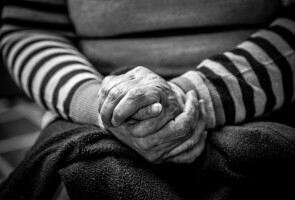
Increased Risk of Parkinson's Disease in Patients with Schizophrenia
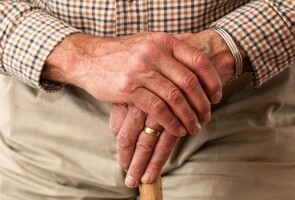
Parkinson’s Patient Skin Samples Provide Clues to Disease Mechanism and a Possible Clinical Test

Apr. 12, 2024
Rice team demonstrates miniature brain stimulator in humans, tiny skull implant places rice lab at the forefront of neurotech research.
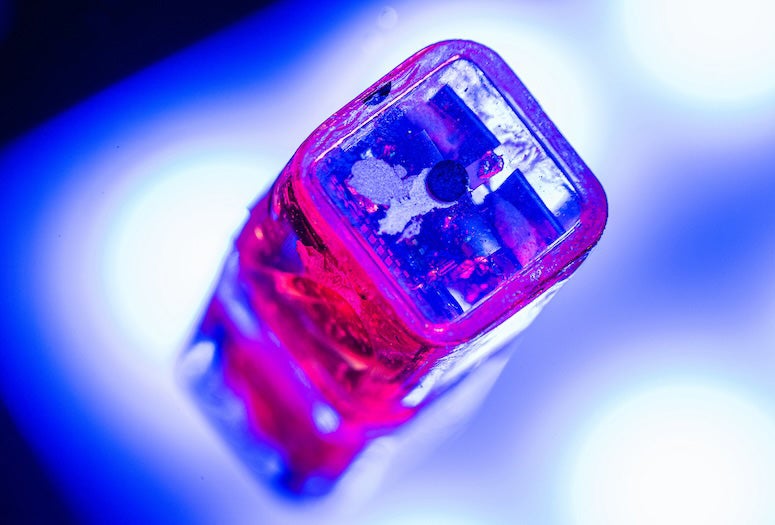
Rice University engineers have developed the smallest implantable brain stimulator demonstrated in a human patient. Thanks to pioneering magnetoelectric power transfer technology, the pea-sized device developed in the Rice lab of Jacob Robinson in collaboration with Motif Neurotech and clinicians Dr. Sameer Sheth and Dr. Sunil Sheth can be powered wirelessly via an external transmitter and used to stimulate the brain through the dura ⎯ the protective membrane attached to the bottom of the skull.
The device, known as the Digitally programmable Over-brain Therapeutic (DOT), could revolutionize treatment for drug-resistant depression and other psychiatric or neurological disorders by providing a therapeutic alternative that offers greater patient autonomy and accessibility than current neurostimulation-based therapies and is less invasive than other brain-computer interfaces (BCIs).
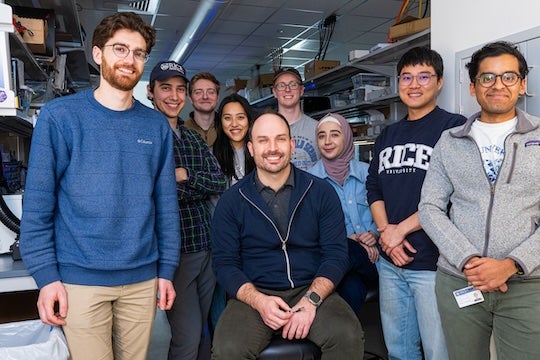
"In this paper we show that our device, the size of a pea, can activate the motor cortex, which results in the patient moving their hand,” said Robinson, a professor of electrical and computer engineering and of bioengineering at Rice. “In the future, we can place the implant above other parts of the brain, like the prefrontal cortex, where we expect to improve executive functioning in people with depression or other disorders.”
Existing implantable technologies for brain stimulation are powered by relatively large batteries that need to be placed under the skin elsewhere in the body and connected to the stimulating device via long wires. Such design limitations require more surgery and subject the individual to a greater burden of hardware implantation, risks of wire breakage or failure and the need for future battery replacement surgeries.
“We eliminated the need for a battery by wirelessly powering the device using an external transmitter,” explained Joshua Woods , an electrical engineering graduate student in the Robinson lab and lead author on the study published in Science Advances. Amanda Singer, a former graduate student in Rice’s applied physics program who is now at Motif Neurotech, is also a lead author.
The technology relies on a material that converts magnetic fields into electrical pulses. This conversion process is very efficient at small scales and has good misalignment tolerance, meaning it does not require complex or minute maneuvering to activate and control. The device has a width of 9 millimeters and can deliver 14.5 volts of stimulation.
“Our implant gets all of its energy through this magnetoelectric effect,” said Robinson, who is founder and CEO of Motif , a startup formed through the Rice Biotech Launch Pad that is working to bring the device to market. “The physics of that power transfer makes this much more efficient than any other wireless power transfer technologies under these conditions.”
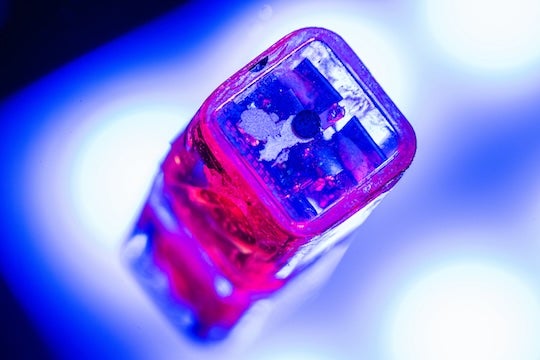
Motif is one of several neurotech companies that are probing the potential of BCIs to revolutionize treatments for neurological disorders.
“Neurostimulation is key to enabling therapies in the mental health space where drug side effects and a lack of efficacy leave many people without adequate treatment options,” Robinson said.
The researchers tested the device temporarily in a human patient, using it to stimulate the motor cortex ⎯ the part of the brain responsible for movement ⎯ and generating a hand movement response. They next showed the device interfaces with the brain stably for a 30-day duration in pigs.
“This has not been done before because the quality and strength of the signal needed to stimulate the brain through the dura were previously impossible with wireless power transfer for implants this small,” Woods said.
Robinson envisions the technology being used from the comfort of one’s home. A physician would prescribe the treatment and provide guidelines for using the device, but patients would retain complete control over how the treatment is administered. “Back home, the patient would put on their hat or wearable to power and communicate with the implant, push ‘go’ on their iPhone or their smartwatch and then the electrical stimulation from that implant would activate a neuronal network inside the brain,” Robinson said.
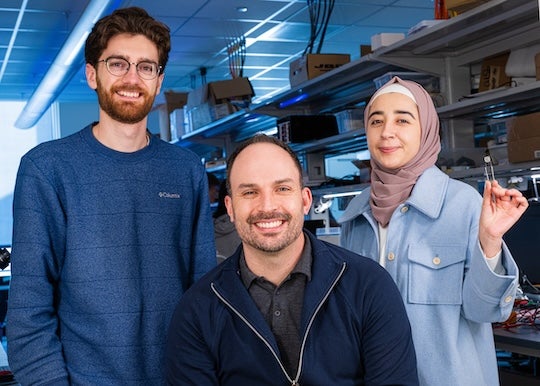
Implantation would require a minimally invasive 30-minute procedure that would place the device in the bone over the brain. Both the implant and the incision would be virtually invisible, and the patient would go home the same day. “When you think about a pacemaker, it’s a very routine part of cardiac care,” said Sheth, professor and vice-chair of research, McNair Scholar and Cullen Foundation Endowed Chair of Neurosurgery at the Baylor College of Medicine. “In neurological and psychiatric disorders, the equivalent is deep brain stimulation (DBS), which sounds scary and invasive. DBS is actually quite a safe procedure, but it’s still brain surgery, and its perceived risk will place a very low ceiling on the number of people who are willing to accept it and may benefit from it. Here’s where technologies like this come in. A 30-minute minor procedure that is little more than skin surgery, done in an outpatient surgery center, is much more likely to be tolerated than DBS. So if we can show that it is about as effective as more invasive alternatives, this therapy will likely make a much larger impact on mental health.”
For some conditions, epilepsy for example, the device may need to be on permanently or most of the time, but for disorders such as depression and OCD, a regimen of just a few minutes of stimulation per day could suffice to bring about the desired changes in the functioning of the targeted neuronal network.
In terms of next steps, Robinson said that on the research side he is “really interested in the idea of creating networks of implants and creating implants that can stimulate and record, so that they can provide adaptive personalized therapies based on your own brain signatures.” From the therapeutic development standpoint, Motif Neurotech is in the process of seeking FDA approval for a long-term clinical trial in humans. Patients and caregivers can sign up on the Motif Neurotech website to learn when and where these trials will begin. The work was supported in part by The Robert and Janice McNair Foundation, the McNair Medical Institute, DARPA and the National Science Foundation.
Miniature battery-free epidural cortical stimulators | Science Advances | DOI: 10.1126/sciadv.adn0858 Authors: Joshua Woods, Amanda Singer, Fatima Alrashdan, Wendy Tan, Chufeng Tan, Sunil Sheth, Sameer Sheth and Jacob Robinson https://doi.org/10.1126/sciadv.adn0858
https://youtu.be/jhAEpAJGKSE (Video by Brandon Martin/Rice University)
https://news-network.rice.edu/news/files/2024/04/240213_Implant_Fitlow_152-5b1ea18ebb9fdfc8.jpg CAPTION: Rice University’s Jacob Robinson and his team of researchers have developed the smallest implantable brain stimulator demonstrated in a human patient that could revolutionize treatment for drug-resistant depression and other psychiatric or neurological disorders. (Photo by Jeff Fitlow/Rice University) https://news-network.rice.edu/news/files/2024/04/JWF_8628-39b4feadc43c1c91.jpg CAPTION: Joshua Woods (from left), Jacob Robinson and Fatima Alrashdan (Photo by Jeff Fitlow/Rice University) https://news-network.rice.edu/news/files/2024/04/JWF_8429-88e28c285ed94f0d.jpg CAPTION: Rice University engineers have developed the first miniaturized brain stimulator shown to work in a human patient. (Photo by Jeff Fitlow/Rice University) Box Link: https://rice.box.com/s/il8b8lxzkekxxodzb1peqpjwcpv97a3c
Robinson lab: www.robinsonlab.com
Sheth lab: https://www.bcm.edu/research/faculty-labs/functional-and-cognitive-neurophysiology-laboratory
Motif Neurotech: www.motifneuro.tech
Rice Neuroengineering Initiative: neuroengineering.rice.edu
Rice Department of Electrical and Computer Engineering: eceweb.rice.edu Rice Department of Bioengineering: https://bioengineering.rice.edu/
George R. Brown School of Engineering: engineering.rice.edu
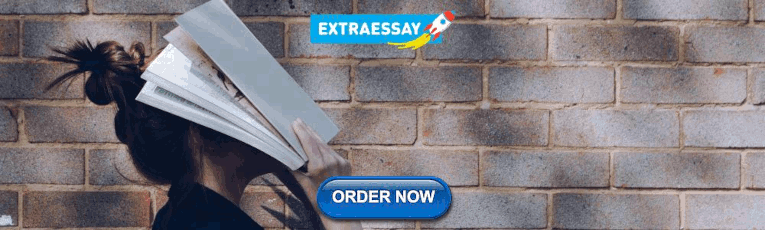
IMAGES
VIDEO
COMMENTS
Neurological disorders are disorders that affect the central nervous system or the peripheral nervous systems and can impair the brain, spinal cord, peripheral nerve or neuromuscular function ...
Neurological disorders pose a large burden on worldwide health. The most recent estimates show that the neurological disorders included in the Global Burden of Disease (GBD) Study-Alzheimer's and other dementias, Parkinson's disease, multiple sclerosis, epilepsy, and headache disorders (migraine, tension-type headache [TTH], and medication-overuse headache [MOH])-represent 3 percent of ...
Neurological disorders pose significant challenges to healthcare systems worldwide. These conditions can severely impact an individual's quality of life, leading to physical, emotional, and cognitive impairments [ 1 ]. Managing neurological disorders often requires specialized care, including access to medical experts, various diagnostic ...
2. Neurodegenerative Disorders (NDs) Neurons are central to the proper functioning of the human brain since they play a critical role in communication [7,8].Most neurons originate in the brain; however, neurons are present everywhere in the body [9,10].During childhood, neural stem cells produce the majority of neurons, the number of which is significantly reduced in adulthood [].
This research paper embarks on a comprehensive exploration of the multifaceted landscape of neurological disorder treatments, offering insights into the genetic and molecular underpinnings of ...
Clinical research in neurological disorders. Submission status. Open. Submission deadline. 30 June 2024. Our knowledge of the pathological mechanisms driving neurodegeneration in disorders like ...
Therapeutic Advances in Neurological Disorders (TAND) is a peer-reviewed open access journal which focuses on pioneering efforts and innovative studies across all areas of neurology. View full journal description. This journal is a member of the Committee on Publication Ethics (COPE).
Dirk Sander, Thorsten Bartsch, Florian Connolly, Christian Enzinger, Urs Fischer, Nils Nellessen, Holger Poppert, Kristina Szabo and Helge Topka. Neurological Research and Practice 2023 5 :64. Correction Published on: 8 November 2023. The original article was published in Neurological Research and Practice 2023 5 :15.
The global burden of neurological disorders is increasing [].The Global Burden of Disease neurology collaborators reported that there has been a 39% increase in deaths due to neurological disorders between 1990 and 2016 [].Alongside this increase in the burden of disease, there is a predicted future shortfall in the US neurology workforce [], and in the UK there is considerable concern ...
Neurological disorders pose significant challenges to healthcare systems worldwide. These conditions can severely impact an individual's quality of life, leading to physical, emotional, and cognitive impairments [ 1 ]. Managing neurological disorders often requires specialized care, including access to medical experts, various diagnostic ...
Neurological diseases represent a great social and economic burden that threatens public health. Neurodegeneration is associated with several transitions in brain including synaptic dysfunction ...
To advance this important topic, we invite authors to contribute research and clinical papers to the Collection "Neuroinflammation and Brain Disease". ... Moreover, the neuroinflammation observed in neurological disorders has a pivotal role in exacerbating Aβ burden and tau hyperphosphorylation, suggesting that stimulating cytokines in ...
Neurological disorders pose a large burden on worldwide health. The most recent estimates show that the neurological disorders included in the Global Burden of Disease (GBD) Study-Alzheimer's and other dementias, Parkinson's disease, multiple sclerosis, epilepsy, and headache disorders (migraine, tension-type headache [TTH], and medication-overuse headache [MOH])-represent 3 percent of ...
Ability to collect research papers: Worldwide; Indexing In: GOOGLE SCHOLAR; ... Journal of Neuroscience and Neurological Disorders has a rich history and track-record of delivering simpler and updated access to the research needs ... Boston University Department of Communication Sciences and Disorders and Knowledge Research Institute, Inc ...
Since its inception, there have been over 120 trainees on the editorial board, over 9,750 articles submitted, and over 2,200 articles published. In honor of our 20th anniversary, we surveyed past and current board members on their RFS experiences and the impact their tenure had on their careers. Read Blog Post.
Neurological disorders remain a significant health and economic burden worldwide. Addressing the challenges imposed by existing drugs, associated side- effects, and immune responses in neurodegenerative diseases is essential for developing better therapies. The immune activation in a diseased state has complex treatment protocols and results in hurdles for clinical translation. There is an ...
Feature papers represent the most advanced research with significant potential for high impact in the field. A Feature Paper should be a substantial original Article that involves several techniques or approaches, provides an outlook for future research directions and describes possible research applications. ... Neurological disorders are ...
Neurological Research is an international, peer-reviewed journal for reporting both basic and clinical research in the fields of neurosurgery, neurology, neuroengineering and neurosciences. It provides a medium for those who recognize the wider implications of their work and who wish to be informed of the relevant experience of others in related and more distant fields.
Importantly, the pathogenesis of many of these neurological disorders can occur long before the onset of clinical manifestations, and a diagnostic tool for early detection of the disease is a must. This Special Issue invites submissions of original research articles, communications, and comprehensive reviews, encompassing advanced research on ...
Biomarkers of neurological diseases. BMC Neurology announced a new Collection focused on biomarkers of neurological diseases. This Collection aims to explore how research into associated biomarkers can contribute to our understanding, diagnosis, and management of neurological conditions that affect millions of people worldwide.
Research into the neuroscience and genetics of SLD has benefited from modern technologies. ... As we have discussed extensively in a previous paper, ... multiply for all those children with organic issues also present in comorbidities. In particular, in the presence of a neurological disease, there is a tendency to consider this the main cause ...
Sleep disorders have many adverse consequences, affecting cardiovascular, neurological, endocrine, and immune health , and chronic sleep restriction (CSR) can lead to the long-term impairment of cognitive function . Research on sleep disorders usually involves a variety of sleep deprivation (SD) protocols.
A major new study released by The Lancet Neurology shows that, in 2021, more than 3 billion people worldwide were living with a neurological condition. The World Health Organization (WHO) contributed to the analysis of the Global Burden of Disease, Injuries, and Risk Factor Study (GBD) 2021 data. Neurological conditions are now the leading cause of ill health and disability worldwide.
A new hypothesis paper appearing in the Journal of Parkinson's Disease on World Parkinson's Day unites the brain- and body-first models with some of the likely causes of the disease-environmental ...
More information . The study, "Rare genetic variation in Fibronectin 1 (FN1) protects against APOEε4 in Alzheimer's disease," was published online April 10 in the journal Acta Neuropathologica. The research and researchers were supported by the Carol and Gene Ludwig Family Foundation, the Agouron Institute, the U.S. National Institutes of Health (grants R01AG067501, RF1AG066107 ...
NINDS is the nation's leading funder of research on the brain and nervous system. The mission of NINDS is to seek fundamental knowledge about the brain and nervous system and to use that knowledge to reduce the burden of neurological disease.
This paper discusses the promising areas of research into machine learning applications for the prevention and correction of neurodegenerative and depressive disorders. These two groups of disorders are among the leading causes of decline in the quality of life in the world when estimated using disability-adjusted years.
Parkinson's disease is a neurological movement disorder that is characterised by motor symptoms, such as slowness, stiffness, and tremor. Parkinson's disease is also associated with a number of non-motor symptoms, such as depression, anxiety, and dysfunction of the autonomic nervous system. ... Extending our research method to other diseases ...
Experts from NYU Langone's Department of Neurology present their latest clinical findings and research discoveries at the American Academy of Neurology (AAN) 2024 Annual Meeting, April 13 to 18, in Denver.. Among the topics presented: measuring effects of burnout and appreciation practices within a neurology department; using sophisticated magnetic resonance imaging (MRI) to better ...
Rice University engineers have developed the smallest implantable brain stimulator demonstrated in a human patient. Thanks to pioneering magnetoelectric power transfer technology, the pea-sized device developed in the Rice lab of Jacob Robinson in collaboration with Motif Neurotech and clinicians Dr. Sameer Sheth and Dr. Sunil Sheth can be powered wirelessly via an external transmitter and ...