Type II restriction endonucleases--a historical perspective and more
Affiliations.
- 1 Institute of Biochemistry, Justus-Liebig-University Giessen, Heinrich-Buff-Ring 58, D-35392 Giessen, Germany [email protected].
- 2 New England Biolabs Inc., 240 County Road, Ipswich, MA 01938-2723, USA.
- 3 Institute of Biochemistry, Justus-Liebig-University Giessen, Heinrich-Buff-Ring 58, D-35392 Giessen, Germany.
- PMID: 24878924
- PMCID: PMC4081073
- DOI: 10.1093/nar/gku447
This article continues the series of Surveys and Summaries on restriction endonucleases (REases) begun this year in Nucleic Acids Research. Here we discuss 'Type II' REases, the kind used for DNA analysis and cloning. We focus on their biochemistry: what they are, what they do, and how they do it. Type II REases are produced by prokaryotes to combat bacteriophages. With extreme accuracy, each recognizes a particular sequence in double-stranded DNA and cleaves at a fixed position within or nearby. The discoveries of these enzymes in the 1970s, and of the uses to which they could be put, have since impacted every corner of the life sciences. They became the enabling tools of molecular biology, genetics and biotechnology, and made analysis at the most fundamental levels routine. Hundreds of different REases have been discovered and are available commercially. Their genes have been cloned, sequenced and overexpressed. Most have been characterized to some extent, but few have been studied in depth. Here, we describe the original discoveries in this field, and the properties of the first Type II REases investigated. We discuss the mechanisms of sequence recognition and catalysis, and the varied oligomeric modes in which Type II REases act. We describe the surprising heterogeneity revealed by comparisons of their sequences and structures.
© The Author(s) 2014. Published by Oxford University Press on behalf of Nucleic Acids Research.
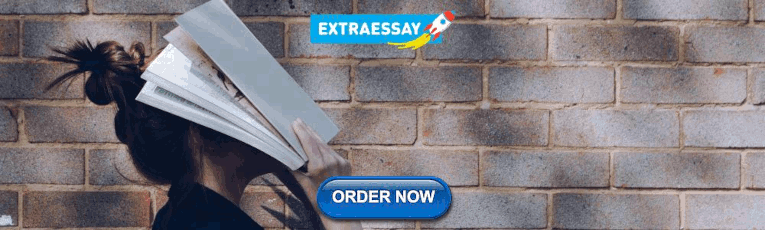
Publication types
- Historical Article
- Research Support, Non-U.S. Gov't
- DNA / chemistry
- DNA / metabolism
- Deoxyribonucleases, Type II Site-Specific / chemistry*
- Deoxyribonucleases, Type II Site-Specific / genetics
- Deoxyribonucleases, Type II Site-Specific / history
- Deoxyribonucleases, Type II Site-Specific / metabolism*
- Evolution, Molecular
- History, 20th Century
- History, 21st Century
- Protein Engineering
- Restriction Mapping
- Deoxyribonucleases, Type II Site-Specific

An official website of the United States government
The .gov means it’s official. Federal government websites often end in .gov or .mil. Before sharing sensitive information, make sure you’re on a federal government site.
The site is secure. The https:// ensures that you are connecting to the official website and that any information you provide is encrypted and transmitted securely.
- Publications
- Account settings
Preview improvements coming to the PMC website in October 2024. Learn More or Try it out now .
- Advanced Search
- Journal List
- Nucleic Acids Res
- v.46(Database issue); 2018 Jan 4
The 2018 Nucleic Acids Research database issue and the online molecular biology database collection
Daniel j rigden.
Institute of Integrative Biology, University of Liverpool, Crown Street, Liverpool L69 7ZB, UK
Xosé M Fernández
Institut Curie, 25 rue d’Ulm, 75005 Paris, France
The 2018 Nucleic Acids Research Database Issue contains 181 papers spanning molecular biology. Among them, 82 are new and 84 are updates describing resources that appeared in the Issue previously. The remaining 15 cover databases most recently published elsewhere. Databases in the area of nucleic acids include 3DIV for visualisation of data on genome 3D structure and RNArchitecture, a hierarchical classification of RNA families. Protein databases include the established SMART, ELM and MEROPS while GPCRdb and the newcomer STCRDab cover families of biomedical interest. In the area of metabolism, HMDB and Reactome both report new features while PULDB appears in NAR for the first time. This issue also contains reports on genomics resources including Ensembl, the UCSC Genome Browser and ENCODE. Update papers from the IUPHAR/BPS Guide to Pharmacology and DrugBank are highlights of the drug and drug target section while a number of proteomics databases including proteomicsDB are also covered. The entire Database Issue is freely available online on the Nucleic Acids Research website ( https://academic.oup.com/nar ). The NAR online Molecular Biology Database Collection has been updated, reviewing 138 entries, adding 88 new resources and eliminating 47 discontinued URLs, bringing the current total to 1737 databases. It is available at http://www.oxfordjournals.org/nar/database/c/ .
NEW AND UPDATED DATABASES
This 2018 Nucleic Acids Research Database Issue is the 25th annual collection of bioinformatic databases. The quarter century arrives with 181 papers which, as ever, span all areas of molecular biology research. The total includes 82 new databases (Table (Table1) 1 ) and 84 updates of resources that have previously appeared in the Database Issue. There are also 15 updates on databases previously described elsewhere (Table (Table2 2 ).
a For full references to the databases featured in this issue, please see the Table of Contents.
As in previous years, databases are grouped into eight broad subject categories. These cover (i) nucleic acid sequence and structure, transcriptional regulation; (ii) protein sequence and structure; (iii) metabolic and signalling pathways, enzymes and networks; (iv) genomics of viruses, bacteria, protozoa and fungi; (v) genomics of human and model organisms plus comparative genomics; (vi) human genomic variation, diseases and drugs; (vii) plants and (viii) other topics, such as proteomics databases. In an era of increasingly interdisciplinary research, it is no surprise that the content of many databases spans multiple categories so that resources often do not sit comfortably in a single category. Readers are again urged to browse the whole issue, rather than confining themselves to the most obviously relevant sections. The Nucleic Acids Research online Molecular Biology Database Collection, which is available at http://www.oxfordjournals.org/nar/database/c/ , retains its more finely grained organisation, encompassing 15 categories and 41 subcategories.
The issue begins with broad surveys of resources at major global centres, including the U.S. National Center for Biotechnology Information (NCBI), the European Bioinformatics Institute (EBI) and the BIG Data Center at the Beijing Institute of Genomics, Chinese Academy of Sciences. The NCBI Resources paper ( 1 ) presents an interesting analysis illustrating the extent of the cross-talk between different databases within the site, exemplifying the value to the user of the extensive data integration implemented at these centres. The EBI paper ( 2 ) describes new data types including image data, biobanks and biosamples, as well as charting the continued exponential growth in the volume of many kinds of data. The newest of the three, the BIG Data Center ( 3 ), focuses on genomic information, but also hosts facilities for samples, program code, and wikis. Many of the wikis are very active and have previously featured in NAR eg lncRNAwiki ( 4 ).
The ‘Nucleic acid databases’ section begins with updates from the International Nucleotide Sequence Database Collaboration ( 5 ) and its three contributors, GenBank, ENA and DDBJ ( 6 – 8 ) which together face the challenge of continued exponential growth in nucleic acid sequence data. Transcription factors (TF) and transcriptional regulation are represented by a number of databases. The popular returning database of TF binding profiles, JASPAR ( 9 ), is published back to back with the ReMAP database ( 10 ) of TF ChIP-seq data: data from ReMAP contributed directly to JASPAR’s improved coverage. With recent intense interest in the role of 3D chromatin structure in gene regulation, the 3DIV resource ( 11 ) for 3D genome interaction visualisation is timely. The key RNA database Rfam ( 12 ) contributes an update describing a move to content based on a set of reference genomes. Mirroring changes made in Pfam ( 13 ), this eliminates much unhelpful redundancy and allows for clearer taxonomic comparisons.
miRNA biology is strongly represented by updates from established databases such as DIANA-TarBase ( 14 ) and mirDIP ( 15 ), as well as new databases such as miRCarta ( 16 ). The new MSDD ( 17 ) links miRNA SNPs to diseases while EVLncRNAs ( 18 ) and MNDR ( 19 ) also major on disease links for non-coding RNAs. The well-established MODOMICS database of RNA modifications ( 20 ) is the subject of an update paper which, among other developments, reports on the availability of liquid chromatography/mass spectrometry data for modified nucleosides, facilitating profiling of such modifications by these methods. RNA structure is covered by the returning RMDB database ( 21 ), containing chemical mapping information that can be used to predict RNA secondary and tertiary structure, and the new RNArchitecture ( 22 ) which introduces a hierarchical organisation of RNA families with a focus on 3D structures, in the manner popularised by protein databases like SCOP.
In the section on protein sequence and structure databases, the venerable SMART database celebrates 20 years with an update paper ( 23 ). It describes a particularly valuable new visualisation option, whereby domain architecture information can be added to phylogenetic trees with the Interactive Tree of Life (iTOL) tool ( 24 ). Another update from PDBe ( 25 ) includes mention of a newly developed library of freely available web components for interactive data visualizations. One of these, the LiteMol 3D viewer, notably allows convenient display of electron density in the browser window. An update on the popular ELM database of protein sequence motifs ( 26 ) reports, among other developments, on how fascinating examples of bacterial pathogen mimicry of eukaryotic motifs are now included in the database. A new arrival, ChannelsDB ( 27 ) contributes our cover image and describes the channels, tunnels and pores in protein structures that allow substrate access to buried catalytic sites, for example, or molecular passage through a transmembrane protein. Certain protein classes or families justify their own bespoke databases through medical or biological importance. T-cell receptors are served in this issue by both VDJdb ( 28 ), focussing on receptor sequences of known specificity, and STCRDab ( 29 ) which collects and curates structural information, linking to and allowing searches against a wide variety of structural, sequence and functional data. The returning database GPCRdb ( 30 ), for G protein-coupled receptors, majors on carefully made homology models and mapping receptors to ligands.
Important updates in the metabolic and signalling section include the human metabolomics database HMDB ( 31 ). Release 4.0 brings huge increases in content, an improved interface and new kinds of information—predicted mass spectra and pharmacometabolomics. This issue also reports on a new metabolomics database, PAMDB ( 32 ), devoted to the bacterial pathogen Pseudomonas aeruginosa , justified not only by the biomedical importance of the organism but also by the novel metabolites that it contains. Metabolic pathways are covered by the well-known returning databases Reactome ( 33 ) and WikiPathways ( 34 ). The former update is notable for its Enhanced High Level Diagrams which superbly contextualise low-level pathways using images of cells, tissues and organs. Among enzyme-oriented databases MEROPS ( 35 ), devoted to proteases and their inhibitors, makes a welcome return with a near-doubling of sequences and cross-references to the PANTHER database ( 36 ). PANTHER full-length sequence based clustering is shown to be complementary to MEROP’s domain-based structure. Carbohydrate-active enzymes are covered by the arrival in NAR of PULDB ( 37 ), covering polysaccharide utilization loci in the prominent gut bacteria of the phylum Bacteriodetes, and dbCAN-seq ( 38 ), which usefully extrapolates information from the well-known CAZy database ( 39 ) to a genome scale. At the enzyme mechanism level, this issue sees the merger of two databases, MACiE and CSA, each veterans of multiple Database Issues, into a single new resource M-CSA (Mechanism and Catalytic Site Atlas) ( 40 ).
In the microbial genomics section, there is an update paper from the yeast-focused SGD ( 41 ) which now includes curated lists of yeast genes that can replace the functions of human counterparts or vice versa. The popular TADB, covering toxins and antitoxins, also presents an update ( 42 ), as does Subti Wiki ( 43 ), devoted to the biology of Bacillus subtilis . Two new databases address viruses. The Virus Taxonomy ( 44 ) appears in NAR for the first time, despite the International Committee behind it dating back to the 1960s. The second, MVP ( 45 ) describes the complex interactions between microbes and the phage clusters that can infect one or more of them.
Human and model organism genomics are strongly represented. The core resources Ensembl ( 46 ) and the UCSC Genome Browser ( 47 ) present their usual updates. The former is supplemented by an Ensembl Genomes paper ( 48 ) covering non-vertebrates which reports ∼20 000 new genomes covered. Other well-known returning databases include ENCODE ( 49 ), RefSeq ( 50 ) and Genomicus ( 51 ), the last showcasing new karyotype evolutionary trees. Among new databases, current trends in cell and molecular biology are reflected in StemMapper ( 52 ) that focusses specifically on stem cell gene expression, and SCPortalen ( 53 ) which stores transcriptomics data, metadata and cell images at the single cell level. Another notable new arrival is PICKLES ( 54 ) which collects information on human gene essentiality from the results of genome scale CRISPR knockout and shRNA knockdown experiments in cancer and other cell lines.
As ever, databases devoted to human genomic variation and biomedical research are very well represented. Important returning databases include the IUPHAR/BPS Guide to Pharmacology ( 55 ) which covers properties of existing and potential drug targets. The authors of the update also describe a major new sister resource, the Guide to Immunopharmacology. An interesting evolutionary perspective on drug targets is provided by ECOdrug ( 56 ) which maps the presence or absence of drug target orthologues across species. This will help in efforts to address ecotoxicology concerns over binding of drugs to non-target wild species and assist with appropriate species choices for ecological risk assessments. The popular DrugBank ( 57 ) also returns, now in release 5.0 and bringing huge increases in data volume, new data types such as pharmacotranscriptomics and content reporting on the status of clinical trials. A major new resource is the Genome Variation Map ( 58 ) from the BIG Data Center covering 19 species. Its arrival is particularly timely with the announcement that comparable NCBI resources dbSNP and dbVar are to stop accepting non-human submissions ( https://ncbiinsights.ncbi.nlm.nih.gov/2017/05/09/phasing-out-support-for-non-human-genome-organism-data-in-dbsnp-and-dbvar/ ).The well-used ClinVar resource ( 59 ) also contributes an update and is joined in interpreting human genome variation and its implications for disease by the newcomer VarCards ( 60 ). Two interesting new databases, PGG .Population ( 61 ) and PopHuman ( 62 ) present a population genomics perspective of human genome variation, each containing thousands of human genomes from across the world and allowing interactive exploration of and comparison between populations.
Plant databases represented here include the comparative genomics resources PLAZA ( 63 ) and Gramene ( 64 ). A major new Arabidopsis resource arrives in the form of the AraGWAS catalog ( 65 ) which contains hundreds of thousands of links between SNPs and curated phenotypes. In the last section proteomics databases are well-represented. An update is presented on the major quantitative proteomics resource proteomicsDB ( 66 ). Its protein-centric view links to an impressive variety of visualisations and to different kinds of omics data. Future plans include an extension from its current human focus to model organisms. An intuitive user interface is also a strong point of the new EPD database ( 67 ), while PIT-DB ( 68 ) explicitly works at the intersection of RNA-seq transcriptomics and proteomics mass spectrometry. After covering such a variety of biological areas, it seems appropriate to finish with mention of the BioStudies database ( 69 ) that collates data of any and all kinds relating to a single study.
NAR ONLINE MOLECULAR BIOLOGY DATABASE COLLECTION
We reach this year the 25th update of the NAR online Molecular Biology Database Collection (which is freely available at http://www.oxfordjournals.org/nar/database/c/ ), featuring 88 new databases (Table (Table1) 1 ) and 15 databases not described previously in the NAR Database Issue (Table (Table2). 2 ). Within our ongoing verification processes to make sure information is still relevant, we have removed 47 obsolete or discontinued databases. After contacting their authors, 138 database entries have been updated with respect to new URLs, new descriptions, and/or other metadata.
We welcome suggestions for inclusion in the Collection of additional databases that have been published in other journals. Such suggestions should be addressed to XMF at [email protected] and should include database summaries in plain text, organized in accordance with the http://www.oxfordjournals.org/nar/database/summary/1 template.
ACKNOWLEDGEMENTS
We thank Dr Martine Bernardes-Silva, especially, and the rest of the Oxford University Press team led by Joanna Ventikos and Elisabeth Waelkens for their help in compiling this issue.
Funding for open access charge: Oxford University Press.
Conflict of interest statement . The authors' opinions do not necessarily reflect the views of their respective institutions.
Thank you for visiting nature.com. You are using a browser version with limited support for CSS. To obtain the best experience, we recommend you use a more up to date browser (or turn off compatibility mode in Internet Explorer). In the meantime, to ensure continued support, we are displaying the site without styles and JavaScript.
- View all journals
- Explore content
- About the journal
- Publish with us
- Sign up for alerts
- Review Article
- Published: 08 April 2024
Nucleic acid-based drugs for patients with solid tumours
- Sebastian G. Huayamares ORCID: orcid.org/0000-0003-2859-3074 1 , 2 ,
- David Loughrey 1 , 2 ,
- Hyejin Kim ORCID: orcid.org/0000-0002-7937-2733 1 , 2 ,
- James E. Dahlman ORCID: orcid.org/0000-0001-7580-436X 1 , 2 &
- Eric J. Sorscher ORCID: orcid.org/0000-0001-9341-3354 2 , 3 , 4
Nature Reviews Clinical Oncology ( 2024 ) Cite this article
3 Altmetric
Metrics details
- Drug development
- Gene delivery
- Nanoparticles
- Targeted gene repair
- Tumour vaccines
The treatment of patients with advanced-stage solid tumours typically involves a multimodality approach (including surgery, chemotherapy, radiotherapy, targeted therapy and/or immunotherapy), which is often ultimately ineffective. Nucleic acid-based drugs, either as monotherapies or in combination with standard-of-care therapies, are rapidly emerging as novel treatments capable of generating responses in otherwise refractory tumours. These therapies include those using viral vectors (also referred to as gene therapies), several of which have now been approved by regulatory agencies, and nanoparticles containing mRNAs and a range of other nucleotides. In this Review, we describe the development and clinical activity of viral and non-viral nucleic acid-based treatments, including their mechanisms of action, tolerability and available efficacy data from patients with solid tumours. We also describe the effects of the tumour microenvironment on drug delivery for both systemically administered and locally administered agents. Finally, we discuss important trends resulting from ongoing clinical trials and preclinical testing, and manufacturing and/or stability considerations that are expected to underpin the next generation of nucleic acid agents for patients with solid tumours.
Nucleic acid drugs being developed for the treatment of patients with solid tumours can be subdivided into either viral vector-mediated or non-viral nanocarrier-type approaches, with distinct safety and efficacy profiles.
New technologies designed to advance drug development, improve tissue tropism and optimize immune responses are rapidly emerging.
The tumour microenvironment poses several barriers to both intratumoural and systemically administered nucleic acid-based therapies.
When developing nucleic acid treatments, various translational aspects including route of administration, optimal preclinical testing, manufacturing-related aspects and scalability are all important considerations.
Clinical trials investigating anticancer nucleic acid-based agents typically involve repeat dosing and are often tested in combination with standard-of-care therapies.
This is a preview of subscription content, access via your institution
Access options
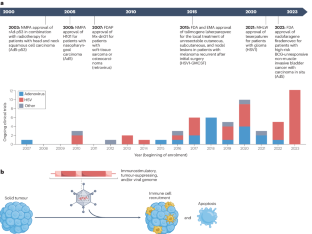
Similar content being viewed by others
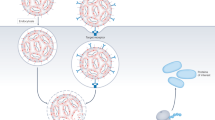
Targeting cancer with mRNA–lipid nanoparticles: key considerations and future prospects
Edo Kon, Nitay Ad-El, … Dan Peer
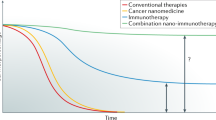
Cancer nanomedicine for combination cancer immunotherapy
Jutaek Nam, Sejin Son, … James J. Moon
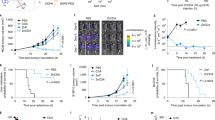
Zinc cyclic di-AMP nanoparticles target and suppress tumours via endothelial STING activation and tumour-associated macrophage reinvigoration
Kaiting Yang, Wenbo Han, … Ralph R. Weichselbaum
Siegel, R. L., Miller, K. D., Wagle, N. S. & Jemal, A. Cancer statistics, 2023. CA Cancer J. Clin. 73 , 17–48 (2023).
Article PubMed Google Scholar
National Cancer Institute. SEER Training modules: Cancer classification. NIH training.seer.cancer.gov/disease/categories/classification.html (2023).
Sung, H. et al. Global Cancer Statistics 2020: GLOBOCAN estimates of incidence and mortality worldwide for 36 cancers in 185 countries. CA Cancer J. Clin. 71 , 209–249 (2021).
Hager, S., Fittler, F. J., Wagner, E. & Bros, M. Nucleic acid-based approaches for tumor therapy. Cells 9 , 2061 (2020).
Article CAS PubMed PubMed Central Google Scholar
Kulkarni, J. A. et al. The current landscape of nucleic acid therapeutics. Nat. Nanotechnol. 16 , 630–643 (2021).
Article CAS PubMed Google Scholar
Paunovska, K., Loughrey, D. & Dahlman, J. E. Drug delivery systems for RNA therapeutics. Nat. Rev. Genet. 23 , 265–280 (2022).
Hou, X., Zaks, T., Langer, R. & Dong, Y. Lipid nanoparticles for mRNA delivery. Nat. Rev. Mater. 6 , 1078–1094 (2021).
Loughrey, D. & Dahlman, J. E. Non-liver mRNA delivery. Acc. Chem. Res. 55 , 13–23 (2022).
Wu, Q., Qian, W., Sun, X. & Jiang, S. Small-molecule inhibitors, immune checkpoint inhibitors, and more: FDA-approved novel therapeutic drugs for solid tumors from 1991 to 2021. J. Hematol. Oncol. 15 , 143 (2022).
Kahvejian, A., Quackenbush, J. & Thompson, J. F. What would you do if you could sequence everything? Nat. Biotechnol. 26 , 1125–1133 (2008).
Qi, F., Qian, S., Zhang, S. & Zhang, Z. Single cell RNA sequencing of 13 human tissues identify cell types and receptors of human coronaviruses. Biochem. Biophys. Res. Commun. 526 , 135–140 (2020).
Van de Sande, B. et al. Applications of single-cell RNA sequencing in drug discovery and development. Nat. Rev. Drug Discov. 22 , 496–520 (2023).
Article PubMed PubMed Central Google Scholar
Paunovska, K., Loughrey, D., Sago, C. D., Langer, R. & Dahlman, J. E. Using large datasets to understand nanotechnology. Adv. Mater. 31 , e1902798 (2019).
Radmand, A. et al. The transcriptional response to lung-targeting lipid nanoparticles in vivo. Nano Lett. 23 , 993–1002 (2023).
Zhao, Z., Anselmo, A. C. & Mitragotri, S. Viral vector-based gene therapies in the clinic. Bioeng. Transl. Med. 7 , e10258 (2022).
Curreri, A., Sankholkar, D., Mitragotri, S. & Zhao, Z. RNA therapeutics in the clinic. Bioeng. Transl. Med. 8 , e10374 (2023).
Baden, L. R. et al. Efficacy and safety of the mRNA-1273 SARS-CoV-2 vaccine. N. Engl. J. Med. 384 , 403–416 (2021).
Polack, F. P. et al. Safety and efficacy of the BNT162b2 mRNA Covid-19 vaccine. N. Engl. J. Med. 383 , 2603–2615 (2020).
Dooling, K. et al. The Advisory Committee on Immunization Practices’ updated interim recommendation for allocation of COVID-19 vaccine – United States, December 2020. MMWR Morb. Mortal. Wkly. Rep. 69 , 1657–1660 (2021).
Adams, D. et al. Patisiran, an RNAi therapeutic, for hereditary transthyretin amyloidosis. N. Engl. J. Med. 379 , 11–21 (2018).
Balwani, M. et al. Phase 3 trial of RNAi therapeutic givosiran for acute intermittent porphyria. N. Engl. J. Med. 382 , 2289–2301 (2020).
Lorentzen, C. L., Haanen, J. B., Met, Ö. & Svane, I. M. Clinical advances and ongoing trials on mRNA vaccines for cancer treatment. Lancet Oncol. 23 , e450–e458 (2022).
Mullard, A. 2020 FDA drug approvals. Nat. Rev. Drug. Discov. 20 , 85–90 (2021).
Mullard, A. Cancer drug approvals and setbacks in 2021. Nat. Cancer 2 , 1246–1247 (2021).
Huayamares, S. G., Lokugamage, M. P., Da Silva Sanchez, A. J. & Dahlman, J. E. A systematic analysis of biotech startups that went public in the first half of 2021. Curr. Res. Biotechnol. 4 , 392–401 (2022).
Article CAS Google Scholar
Barbier, A. J., Jiang, A. Y., Zhang, P., Wooster, R. & Anderson, D. G. The clinical progress of mRNA vaccines and immunotherapies. Nat. Biotechnol. 40 , 840–854 (2022).
Food and Drug Administration. Cellular & gene therapy guidances. FDA https://www.fda.gov/vaccines-blood-biologics/biologics-guidances/cellular-gene-therapy-guidances (2024).
Gene therapy needs a long-term approach. Nat. Med. 27 , 563 (2021).
Food and Drug Administration. Establishment of the Office of Therapeutic Products. FDA https://www.fda.gov/vaccines-blood-biologics/cellular-gene-therapy-products/establishment-office-therapeutic-products (2023).
Wang, L. L. et al. Cell therapies in the clinic. Bioeng. Transl. Med. 6 , e10214 (2021).
Bashor, C. J., Hilton, I. B., Bandukwala, H., Smith, D. M. & Veiseh, O. Engineering the next generation of cell-based therapeutics. Nat. Rev. Drug. Discov. 21 , 655–675 (2022).
Cappell, K. M. & Kochenderfer, J. N. Long-term outcomes following CAR T cell therapy: what we know so far. Nat. Rev. Clin. Oncol. 20 , 359–675 (2023).
Zhang, W. W. et al. The first approved gene therapy product for cancer Ad-p53 (Gendicine): 12 years in the clinic. Hum. Gene Ther. 29 , 160–179 (2018).
Daley, J. Gene therapy arrives. Nature 576 , S12–S13 (2019).
Cattaneo, R., Miest, T., Shashkova, E. V. & Barry, M. A. Reprogrammed viruses as cancer therapeutics: targeted, armed and shielded. Nat. Rev. Microbiol. 6 , 529–540 (2008).
Gordon, E. M. & Hall, F. L. Noteworthy clinical case studies in cancer gene therapy: tumor-targeted Rexin-G advances as an efficacious anti-cancer agent. Int. J. Oncol. 36 , 1341–1353 (2010).
Rehman, H., Silk, A. W., Kane, M. P. & Kaufman, H. L. Into the clinic: talimogene laherparepvec (T-VEC), a first-in-class intratumoral oncolytic viral therapy. J. Immunother. Cancer 4 , 53 (2016).
Maruyama, Y. et al. Regulatory issues: PMDA – review of Sakigake designation products: oncolytic virus therapy with delytact injection (Teserpaturev) for malignant glioma. Oncologist 28 , 664–670 (2023).
Food and Drug Administration. Highlights of prescribing information: ADSTILADRIN® (nadofaragene firadenovec-vncg). FDA www.fda.gov/media/164029/download (2022).
Sibbald, B. Death but one unintended consequence of gene-therapy trial. CMAJ 164 , 1612 (2001).
CAS PubMed PubMed Central Google Scholar
Hacein-Bey-Abina, S. et al. Insertional oncogenesis in 4 patients after retrovirus-mediated gene therapy of SCID-X1. J. Clin. Invest. 118 , 3132–3142 (2008).
Hacein-Bey-Abina, S. et al. LMO2-associated clonal T cell proliferation in two patients after gene therapy for SCID-X1. Science 302 , 415–419 (2003).
Maetzig, T., Galla, M., Baum, C. & Schambach, A. Gammaretroviral vectors: biology, technology and application. Viruses 3 , 677–713 (2011).
Hayward, A. Origin of the retroviruses: when, where, and how? Curr. Opin. Virol. 25 , 23–27 (2017).
Yi, Y., Noh, M. J. & Lee, K. H. Current advances in retroviral gene therapy. Curr. Gene Ther. 11 , 218–228 (2011).
Morse, M. A. et al. Tumor protein p53 mutation in archived tumor samples from a 12-year survivor of stage 4 pancreatic ductal adenocarcinoma may predict long-term survival with DeltaRex-G: a case report and literature review. Mol. Clin. Oncol. 15 , 186 (2021).
Pellinen, R. et al. Cancer cells as targets for lentivirus-mediated gene transfer and gene therapy. Int. J. Oncol. 25 , 1753–1762 (2004).
CAS PubMed Google Scholar
Yi, Y., Hahm, S. H. & Lee, K. H. Retroviral gene therapy: safety issues and possible solutions. Curr. Gene Ther. 5 , 25–35 (2005).
Benihoud, K., Yeh, P. & Perricaudet, M. Adenovirus vectors for gene delivery. Curr. Opin. Biotechnol. 10 , 440–447 (1999).
Crystal, R. G. Adenovirus: the first effective in vivo gene delivery vector. Hum. Gene Ther. 25 , 3–11 (2014).
Lynch, J. P. 3rd & Kajon, A. E. Adenovirus: epidemiology, global spread of novel serotypes, and advances in treatment and prevention. Semin. Respir. Crit. Care Med. 37 , 586–602 (2016).
Sakhuja, K. et al. Optimization of the generation and propagation of gutless adenoviral vectors. Hum. Gene Ther. 14 , 243–254 (2003).
Li, C. & Samulski, R. J. Engineering adeno-associated virus vectors for gene therapy. Nat. Rev. Genet. 21 , 255–272 (2020).
Hudry, E. & Vandenberghe, L. H. Therapeutic AAV gene transfer to the nervous system: a clinical reality. Neuron 101 , 839–862 (2019).
Goswami, R. et al. Gene therapy leaves a vicious cycle. Front. Oncol. 9 , 297 (2019).
Ail, D., Malki, H., Zin, E. A. & Dalkara, D. Adeno-associated virus (AAV)-based gene therapies for retinal diseases: where are we? Appl. Clin. Genet. 16 , 111–130 (2023).
Kuzmin, D. A. et al. The clinical landscape for AAV gene therapies. Nat. Rev. Drug. Discov. 20 , 173–174 (2021).
Frampton, A. R. Jr., Goins, W. F., Nakano, K., Burton, E. A. & Glorioso, J. C. HSV trafficking and development of gene therapy vectors with applications in the nervous system. Gene Ther. 12 , 891–901 (2005).
Miyagawa, Y. et al. Herpes simplex viral-vector design for efficient transduction of nonneuronal cells without cytotoxicity. Proc. Natl Acad. Sci. USA 112 , E1632–E1641 (2015).
Manservigi, R., Argnani, R. & Marconi, P. HSV recombinant vectors for gene therapy. Open. Virol. J. 4 , 123–156 (2010).
Kremer, L. P. M. et al. High throughput screening of novel AAV capsids identifies variants for transduction of adult NSCs within the subventricular zone. Mol. Ther. Methods Clin. Dev. 23 , 33–50 (2021).
Westhaus, A. et al. High-throughput in vitro, ex vivo, and in vivo screen of adeno-associated virus vectors based on physical and functional transduction. Hum. Gene Ther. 31 , 575–589 (2020).
Jang, M. J. et al. Spatial transcriptomics for profiling the tropism of viral vectors in tissues. Nat. Biotechnol. 41 , 1272–1286 (2023).
Lawler, S. E., Speranza, M.-C., Cho, C.-F. & Chiocca, E. A. Oncolytic viruses in cancer treatment: a review. JAMA Oncol. 3 , 841–849 (2017).
Shalhout, S. Z., Miller, D. M., Emerick, K. S. & Kaufman, H. L. Therapy with oncolytic viruses: progress and challenges. Nat. Rev. Clin. Oncol. 20 , 160–177 (2023).
Andtbacka, R. H. I. et al. Final analyses of OPTiM: a randomized phase III trial of talimogene laherparepvec versus granulocyte-macrophage colony-stimulating factor in unresectable stage III-IV melanoma. J. Immunother. Cancer 7 , 145 (2019).
Food and Drug Administration. Highlights of prescribing information: IMLYGIC® (talimogene laherparepvec). FDA . www.fda.gov/media/94129/download (2015).
Chesney, J. A. et al. Randomized, double-blind, placebo-controlled, global phase III trial of talimogene laherparepvec combined with pembrolizumab for advanced melanoma. J. Clin. Oncol. 41 , 528–540 (2023).
Ferrucci, P. F., Pala, L., Conforti, F. & Cocorocchio, E. Talimogene laherparepvec (T-VEC): an intralesional cancer immunotherapy for advanced melanoma. Cancers 13 , 1386 (2021).
Article Google Scholar
Georgina, L. et al. 429 long-term analysis of MASTERKEY-265 phase 1b trial of talimogene laherparepvec (T-VEC) plus pembrolizumab in patients with unresectable stage IIIB-IVM1c melanoma. J. Immunother. Cancer 8 , A261 (2020).
Google Scholar
Sobol, R. E. et al. Analysis of adenoviral p53 gene therapy clinical trials in recurrent head and neck squamous cell carcinoma. Front. Oncol. 11 , 645745 (2021).
Yao, M. et al. Prognostic comparison between cTACE and H101-TACE in unresectable hepatocellular carcinoma (HCC): a propensity-score matching analysis. Appl. Bionics Biomech. 2022 , 9084852 (2022).
GeoVax. Gedeptin Technology Overview. GeoVax www.geovax.com/our-technology/gedeptin-technology-overview (2024).
Rosenthal, E. L. et al. Phase I dose-escalating trial of Escherichia coli purine nucleoside phosphorylase and fludarabine gene therapy for advanced solid tumors. Ann. Oncol. 26 , 1481–1487 (2015).
Xie, Y. et al. Alpha-herpesvirus thymidine kinase genes mediate viral virulence and are potential therapeutic targets. Front. Microbiol. 10 , 941 (2019).
Deswal, P. Hookipa debuts phase I/II data. ClinicalTrials Arena www.clinicaltrialsarena.com/news/hookipa-debuts-phase-1-2-data/?cf-view (2023).
Palmer, C. D. et al. Individualized, heterologous chimpanzee adenovirus and self-amplifying mRNA neoantigen vaccine for advanced metastatic solid tumors: phase 1 trial interim results. Nat. Med. 28 , 1619–1629 (2022).
Shigdar, S., Schrand, B., Giangrande, P. H. & de Franciscis, V. Aptamers: cutting edge of cancer therapies. Mol. Ther. 29 , 2396–2411 (2021).
Agnello, L. et al. Aptamer-based strategies to boost immunotherapy in TNBC. Cancers 15 , 2010 (2023).
Sorscher, E. J., Hong, J. S., Allan, P. W., Waud, W. R. & Parker, W. B. In vivo antitumor activity of intratumoral fludarabine phosphate in refractory tumors expressing E. coli purine nucleoside phosphorylase. Cancer Chemother. Pharmacol. 70 , 321–329 (2012).
Nakamura, T. et al. The effect of size and charge of lipid nanoparticles prepared by microfluidic mixing on their lymph node transitivity and distribution. Mol. Pharm. 17 , 944–953 (2020).
Dilliard, S. A., Cheng, Q. & Siegwart, D. J. On the mechanism of tissue-specific mRNA delivery by selective organ targeting nanoparticles. Proc. Natl Acad. Sci. USA 118 , e2109256118 (2021).
Hobbs, S. K. et al. Regulation of transport pathways in tumor vessels: role of tumor type and microenvironment. Proc. Natl Acad. Sci. USA 95 , 4607–4612 (1998).
Dvorak, H. F., Nagy, J. A., Dvorak, J. T. & Dvorak, A. M. Identification and characterization of the blood vessels of solid tumors that are leaky to circulating macromolecules. Am. J. Pathol. 133 , 95–109 (1988).
Iyer, A. K., Khaled, G., Fang, J. & Maeda, H. Exploiting the enhanced permeability and retention effect for tumor targeting. Drug. Discov. Today 11 , 812–818 (2006).
Zhen, Z. et al. Tumor vasculature targeted photodynamic therapy for enhanced delivery of nanoparticles. ACS Nano 8 , 6004–6013 (2014).
Lazarovits, J., Chen, Y. Y., Sykes, E. A. & Chan, W. C. Nanoparticle-blood interactions: the implications on solid tumour targeting. Chem. Commun. 51 , 2756–2767 (2015).
Chan, W. C. W. Principles of nanoparticle delivery to solid tumors. BME Front. 4 , 0016 (2023).
Ruoslahti, E., Bhatia, S. N. & Sailor, M. J. Targeting of drugs and nanoparticles to tumors. J. Cell Biol. 188 , 759–768 (2010).
Matsumura, Y. & Maeda, H. A new concept for macromolecular therapeutics in cancer chemotherapy: mechanism of tumoritropic accumulation of proteins and the antitumor agent smancs. Cancer Res. 46 , 6387–6392 (1986).
Huayamares, S. G. et al. High-throughput screens identify a lipid nanoparticle that preferentially delivers mRNA to human tumors in vivo. J. Control. Rel. 357 , 394–403 (2023).
Kon, E., Ad-El, N., Hazan-Halevy, I., Stotsky-Oterin, L. & Peer, D. Targeting cancer with mRNA–lipid nanoparticles: key considerations and future prospects. Nat. Rev. Clin. Oncol. 20 , 739–754 (2023).
Lammers, T., Kiessling, F., Hennink, W. E. & Storm, G. Drug targeting to tumors: principles, pitfalls and (pre-)clinical progress. J. Control. Rel. 161 , 175–187 (2012).
Schlich, M. et al. Cytosolic delivery of nucleic acids: the case of ionizable lipid nanoparticles. Bioeng. Transl. Med. 6 , e10213 (2021).
Gilleron, J. et al. Image-based analysis of lipid nanoparticle-mediated siRNA delivery, intracellular trafficking and endosomal escape. Nat. Biotechnol. 31 , 638–646 (2013).
Sheth, V., Wang, L., Bhattacharya, R., Mukherjee, P. & Wilhelm, S. Strategies for delivering nanoparticles across tumor blood vessels. Adv. Funct. Mater. 31 , 2007363 (2021).
Sabnis, S. et al. A novel amino lipid series for mRNA delivery: improved endosomal escape and sustained pharmacology and safety in non-human primates. Mol. Ther. 26 , 1509–1519 (2018).
Guimaraes, P. P. G. et al. Ionizable lipid nanoparticles encapsulating barcoded mRNA for accelerated in vivo delivery screening. J. Control. Rel. 316 , 404–417 (2019).
Hatit, M. Z. C. et al. Species-dependent in vivo mRNA delivery and cellular responses to nanoparticles. Nat. Nanotechnol. 17 , 310–318 (2022).
Santinha, A. J. et al. Transcriptional linkage analysis with in vivo AAV-Perturb-seq. Nature 622 , 367–375 (2023).
Huayamares, S. G. et al. Nanoparticle delivery of a prodrug-activating bacterial enzyme leads to anti-tumor responses. Nat Commun . (in the press, 2024).
Noble, R. et al. Spatial structure governs the mode of tumour evolution. Nat. Ecol. Evol. 6 , 207–217 (2022).
Lomakin, A. et al. Spatial genomics maps the structure, nature and evolution of cancer clones. Nature 611 , 594–602 (2022).
Ota, Y. et al. A practical spatial analysis method for elucidating the biological mechanisms of cancers with abdominal dissemination in vivo. Sci. Rep. 12 , 20303 (2022).
Hsieh, W.-C. et al. Spatial multi-omics analyses of the tumor immune microenvironment. J. Biomed. Sci. 29 , 96 (2022).
Kranz, L. M. et al. Systemic RNA delivery to dendritic cells exploits antiviral defence for cancer immunotherapy. Nature 534 , 396–401 (2016).
Beck, J. D. et al. mRNA therapeutics in cancer immunotherapy. Mol. Cancer 20 , 69 (2021).
Mullard, A. COVID-19 vaccine success enables a bolder vision for mRNA cancer vaccines, says BioNTech CEO. Nat. Rev. Drug Discov. 20 , 500–501 (2021).
Chen, J. et al. Targeting CLDN18.2 in cancers of the gastrointestinal tract: new drugs and new indications. Front. Oncol. 13 , 1132319 (2023).
Mackensen, A. et al. CLDN6-specific CAR-T cells plus amplifying RNA vaccine in relapsed or refractory solid tumors: the phase 1 BNT211-01 trial. Nat. Med. 29 , 2844–2853 (2023).
Qu, H., Jin, Q. & Quan, C. CLDN6: from traditional barrier function to emerging roles in cancers. Int. J. Mol. Sci. 22 , 13416 (2021).
Doherty, K. CLDN6 CAR T-cell therapy shows encouraging efficacy in relapsed/refractory advanced solid tumours. OncLive www.onclive.com/view/cldn6-car-t-cell-therapy-shows-encouraging-efficacy-in-relapsed-refractory-advanced-solid-tumors (2022).
Qian Wei, Z.-Y. F., Zhang, Z.-M. & Zhang, T.-F. Therapeutic tumor vaccines – a rising star to benefit cancer patients. Artif. Intell. Cancer 2 , 25–41 (2021).
BioNTech. BioNTech expands clinical oncolocgy portfolio with first patient dosed in phase 2 trial of mRNA-based individualized immunotherapy BNT122 in colorectal cancer patients. BioNTech investors.biontech.de/news-releases/news-release-details/biontech-expands-clinical-oncology-portfolio-first-patient-dosed (2021).
Lopez, J. S. et al. A phase Ib study to evaluate RO7198457, an individualized neoantigen specific immunotherapy (iNeST), in combination with atezolizumab in patients with locally advanced or metastatic solid tumors [abstract]. Cancer Res. 80 (Suppl. 16), CT301 (2020).
Rojas, L. A. et al. Personalized RNA neoantigen vaccines stimulate T cells in pancreatic cancer. Nature 618 , 144–150 (2023).
Dolgin, E. Personalized cancer vaccines pass first major clinical test. Nat. Rev. Drug Discov. 22 , 607–609 (2023).
Li, S. et al. Payload distribution and capacity of mRNA lipid nanoparticles. Nat. Commun. 13 , 5561 (2022).
Burris, H. A. et al. A phase I multicenter study to assess the safety, tolerability, and immunogenicity of mRNA-4157 alone in patients with resected solid tumors and in combination with pembrolizumab in patients with unresectable solid tumors [abstract]. J. Clin. Oncol. 37 (Suppl. 15), 2523 (2019).
Bauman, J. et al. Safety, tolerability, and immunogenicity of mRNA-4157 in combination with pembrolizumab in subjects with unresectable solid tumors (KEYNOTE-603): an update [abstract 798]. J. Immunother. Cancer 8 (Suppl. 3), A477 (2020).
Moderna. Moderna and Merck announce mRNA-4157/V940, an investigational personalized mRNA cancer vaccine, in combination with KEYTRUDA(R) (pembrolizumab), met primary efficacy endpoint in phase 2b KEYNOTE-942 trial. moderna investors.modernatx.com/news/news-details/2022/Moderna-and-Merck-Announce-mRNA-4157V940-an-Investigational-Personalized-mRNA-Cancer-Vaccine-in-Combination-with-KEYTRUDAR-pembrolizumab-Met-Primary-Efficacy-Endpoint-in-Phase-2b-KEYNOTE-942-Trial/default.aspx (2022).
Khattak, A. et al. A personalized cancer vaccine, mRNA-4157, combined with pembrolizumab versus pembrolizumab in patients with resected high-risk melanoma: efficacy and safety results from the randomized, open-label Phase 2 mRNA-4157-P201/Keynote-942 trial [abstract]. Cancer Res. 83 (Suppl. 8), CT001–CT001 (2023).
Ryan, C. FDA grants breakthrough therapy designation to mRNA-4157/V940 plus pembrolizumab in high-risk melanoma. OncLive www.onclive.com/view/fda-grants-breakthrough-therapy-designation-to-mrna-4157-v940-plus-pembrolizumab-in-high-risk-melanoma (2023).
Patel, M. et al. Phase 1 study of mRNA-2752, a lipid nanoparticle encapsulating mRNAs encoding human OX40L/IL-23/IL-36γ, for intratumoral (ITu) injection +/- durvalumab in advanced solid tumors and lymphoma [abstract 539]. J. Immunother. Cancer 9 (Suppl. 2), A569 (2021).
Hamid, O. et al. Preliminary safety, antitumor activity and pharmacodynamics results of HIT-IT MEDI1191 (mRNA IL-12) in patients with advanced solid tumours and superficial lesions [abstract 19O]. Ann. Oncol. 32 (Suppl. 1), S9 (2021).
Carneiro, B. A. et al. First-in-human study of MEDI1191 (mRNA encoding IL-12) plus durvalumab in patients (pts) with advanced solid tumors [abstract]. Cancer Res. 82 (Suppl. 12), CT183 (2022).
Taylor, N. P. AstraZeneca discards Moderna-partnered solid tumor prospect, kidney disease asset in pipeline clear-out. Fierce Biotech www.fiercebiotech.com/biotech/astrazeneca-discards-moderna-partnered-solid-tumor-prospect-kidney-disease-asset-pipeline (2022).
Chen, H., Liu, H. & Qing, G. Targeting oncogenic Myc as a strategy for cancer treatment. Signal. Transduct. Target. Ther. 3 , 5 (2018).
Biospace. Omega Therapeutics announces promising preliminary clinical data for OTX-2002 from ongoing MYCHELANGELO™ I trial. BioSpace www.biospace.com/article/releases/omega-therapeutics-announces-promising-preliminary-clinical-data-for-otx-2002-from-ongoing-mychelangelo-i-trial/ (2023).
Omega Therapeutics. MYCHELANGELO™ I: preliminary phase 1 clinical update. Omega Therapeutics ir.omegatherapeutics.com/static-files/19eca20b-260d-4f30-8a13-16b05a5cb26b (2023).
Besin, G. et al. Accelerated blood clearance of lipid nanoparticles entails a biphasic humoral response of B-1 followed by B-2 lymphocytes to distinct antigenic moieties. Immunohorizons 3 , 282–293 (2019).
Bavli, Y. et al. Anti-PEG antibodies before and after a first dose of Comirnaty® (mRNA-LNP-based SARS-CoV-2 vaccine). J. Control. Rel. 354 , 316–322 (2023).
Sanchez, A. J. D. S. et al. Substituting poly(ethylene glycol) lipids with poly(2-ethyl-2-oxazoline) lipids improves lipid nanoparticle repeat dosing. Adv. Healthc. Mater. https://doi.org/10.1002/adhm.202304033 (2024).
Hattab, D., Gazzali, A. M. & Bakhtiar, A. Clinical advances of siRNA-based nanotherapeutics for cancer treatment. Pharmaceutics 13 , 1009 (2021).
Molyneaux, M., Berman, B., Xu, J., Evans, D. M. & Lu, P. Y. Effect of TGF-B1/COX-2 small interfering RNA combination product (STP705) on cell viability and tumor growth in a human squamous carcinoma xenograft tumor model in nude mice [abstract 15580]. J. Am. Acad. Dermatol. 83 (Suppl. 6), AB156 (2020).
Sirnaomics. Sirnaomics achieves 100% complete response in phase II clinical trial of STP705 for treatment of cutaneous basal cell carcinoma. Sirnaomics sirnaomics.com/en/news-room/press-release/20220829sirnaomics-achieves-100-complete-response-in-phase-ii-clinical-trial-of-stp705-for-treatment-of-cutaneous-basal-cell-carcinoma/ (2022).
Sirnaomics. Pipeline. Sirnaomics sirnaomics.com/en/science-pipeline/pipeline/ (2023).
Sirnaomics. Sirnaomics launches phase I clinical trial of RNAi therapeutic STP707 delivered systemically for the treatment of solid tumors. Sirnaomics sirnaomics.com/en/news-room/press-release/20220209sirnaomics-launches-phase-i-clinical-trial-of-rnai-therapeutic-stp707-delivered-systemically-for-the-treatment-of-solid-tumors-1/ (2022).
Zhou, J. et al. Simultaneous silencing of TGF-β1 and COX-2 reduces human skin hypertrophic scar through activation of fibroblast apoptosis. Oncotarget 8 , 80651–80665 (2017).
Yan, Z. et al. Human rhomboid family-1 gene silencing causes apoptosis or autophagy to epithelial cancer cells and inhibits xenograft tumor growth. Mol. Cancer Ther. 7 , 1355–1364 (2008).
Leng, Q., Scaria, P., Lu, P., Woodle, M. C. & Mixson, A. J. Systemic delivery of HK Raf-1 siRNA polyplexes inhibits MDA-MB-435 xenografts. Cancer Gene Ther. 15 , 485–495 (2008).
Tandon, M., Vemula, S. V. & Mittal, S. K. Emerging strategies for EphA2 receptor targeting for cancer therapeutics. Expert. Opin. Ther. Targets 15 , 31–51 (2011).
Cina, C. et al. A novel glutathione S-transferase P (GSTP) siRNA (NDT-05-1040) for the treatment of KRAS-driven non-small cell lung cancer [abstract]. Cancer Res. 78 (Suppl. 13), 5918 (2018).
Jiao, L. et al. Glutathione S-transferase gene polymorphisms and risk and survival of pancreatic cancer. Cancer 109 , 840–848 (2007).
Bio-Path Holdings. Bio-Path Holdings presents data from ongoing phase 2 study of prexigebersen at 2021 American Society of Hematology Annual Meeting. Bio-Path Holdings www.sec.gov/Archives/edgar/data/1133818/000155837021016703/bpth-20211213xex99d1.htm (2021).
Anderluzzi, G. et al. The role of nanoparticle format and route of administration on self-amplifying mRNA vaccine potency. J. Control. Rel. 342 , 388–399 (2022).
Pardi, N. et al. Expression kinetics of nucleoside-modified mRNA delivered in lipid nanoparticles to mice by various routes. J. Control. Rel. 217 , 345–351 (2015).
De Lombaerde, E., De Wever, O. & De Geest, B. G. Delivery routes matter: safety and efficacy of intratumoral immunotherapy. Biochim. Biophys. Acta Rev. Cancer 1875 , 188526 (2021).
Wu, L. et al. Quantitative comparison of three widely-used pulmonary administration methods in vivo with radiolabeled inhalable nanoparticles. Eur. J. Pharm. Biopharm. 152 , 108–115 (2020).
Liu, J. Q. et al. Is AAV-delivered IL-27 a potential immunotherapeutic for cancer? Am. J. Cancer Res. 10 , 3565–3574 (2020).
Kedmi, R., Ben-Arie, N. & Peer, D. The systemic toxicity of positively charged lipid nanoparticles and the role of Toll-like receptor 4 in immune activation. Biomaterials 31 , 6867–6875 (2010).
Tousignant, J. D. et al. Comprehensive analysis of the acute toxicities induced by systemic administration of cationic lipid:plasmid DNA complexes in mice. Hum. Gene Ther. 11 , 2493–2513 (2000).
Liu, J. Q. et al. Intratumoral delivery of IL-12 and IL-27 mRNA using lipid nanoparticles for cancer immunotherapy. J. Control. Rel. 345 , 306–313 (2022).
Mitchell, M. J. et al. Engineering precision nanoparticles for drug delivery. Nat. Rev. Drug. Discov. 20 , 101–124 (2021).
Li, H.-J. et al. Stimuli-responsive clustered nanoparticles for improved tumor penetration and therapeutic efficacy. Proc. Natl Acad. Sci. USA 113 , 4164–4169 (2016).
Scheetz, L. et al. Engineering patient-specific cancer immunotherapies. Nat. Biomed. Eng. 3 , 768–782 (2019).
Sindhwani, S. et al. The entry of nanoparticles into solid tumours. Nat. Mater. 19 , 566–575 (2020).
Naumenko, V. A. et al. Extravasating neutrophils open vascular barrier and improve liposomes delivery to tumors. ACS Nano 13 , 12599–12612 (2019).
Lin, Z. P., Ngo, W., Mladjenovic, S. M., Wu, J. L. Y. & Chan, W. C. W. Nanoparticles bind to endothelial cells in injured blood vessels via a transient protein corona. Nano Lett. 23 , 1003–1009 (2023).
Sun, D., Zhou, S. & Gao, W. What went wrong with anticancer nanomedicine design and how to make it right. ACS Nano 14 , 12281–12290 (2020).
Wilhelm, S. et al. Analysis of nanoparticle delivery to tumours. Nat. Rev. Mater. 1 , 16014 (2016).
Prabhakar, U. et al. Challenges and key considerations of the enhanced permeability and retention effect for nanomedicine drug delivery in oncology. Cancer Res. 73 , 2412–2417 (2013).
Price, L. S. L., Stern, S. T., Deal, A. M., Kabanov, A. V. & Zamboni, W. C. A reanalysis of nanoparticle tumor delivery using classical pharmacokinetic metrics. Sci. Adv. 6 , eaay9249 (2020).
McNeil, S. E. Evaluation of nanomedicines: stick to the basics. Nat. Rev. Mater. 1 , 16073 (2016).
Ding, H. et al. Long distance from microvessel to cancer cell predicts poor prognosis in non-small cell lung cancer patients. Front. Oncol. 11 , 632352 (2021).
Miar, A. et al. Hypoxia induces transcriptional and translational downregulation of the type I IFN pathway in multiple cancer cell types. Cancer Res. 80 , 5245–5256 (2020).
Tang, Y., Weng, X., Liu, C., Li, X. & Chen, C. Hypoxia enhances activity and malignant behaviors of colorectal cancer cells through the STAT3/microRNA-19a/PTEN/PI3K/AKT axis. Anal. Cell Pathol. 2021 , 4132488 (2021).
Durymanov, M. O., Rosenkranz, A. A. & Sobolev, A. S. Current approaches for improving intratumoral accumulation and distribution of nanomedicines. Theranostics 5 , 1007–1020 (2015).
Gerweck, L. E., Kozin, S. V. & Stocks, S. J. The pH partition theory predicts the accumulation and toxicity of doxorubicin in normal and low-pH-adapted cells. Br. J. Cancer 79 , 838–842 (1999).
Huayamares, S. G. et al. Constructing a biomaterial to simulate extracellular drug transport in solid tumors. Macromol. Biosci. 20 , 2000251 (2020).
Cheng, Q. et al. Selective organ targeting (SORT) nanoparticles for tissue-specific mRNA delivery and CRISPR-Cas gene editing. Nat. Nanotechnol. 15 , 313–320 (2020).
Mohanty, R. P., Liu, X. & Ghosh, D. Electrostatic driven transport enhances penetration of positively charged peptide surfaces through tumor extracellular matrix. Acta Biomater. 113 , 240–251 (2020).
Pressnall, M. M. et al. Glatiramer acetate enhances tumor retention and innate activation of immunostimulants. Int. J. Pharmaceutics 605 , 120812 (2021).
Huang, A. et al. Human intratumoral therapy: linking drug properties and tumor transport of drugs in clinical trials. J. Control. Rel. 326 , 203–221 (2020).
Pressnall, M. M., Huayamares, S. G. & Berkland, C. J. Immunostimulant complexed with polylysine limits transport and maintains immune cell activation. J. Pharm. Sci. 109 , 2836–2846 (2020).
Henke, E., Nandigama, R. & Ergün, S. Extracellular matrix in the tumor microenvironment and its impact on cancer therapy. Front. Mol. Biosci. 6 , 160 (2019).
Hartmann, N. et al. Prevailing role of contact guidance in intrastromal T-cell trapping in human pancreatic cancer. Clin. Cancer Res. 20 , 3422–3433 (2014).
Gordon-Weeks, A. & Yuzhalin, A. E. Cancer extracellular matrix proteins regulate tumour immunity. Cancers 12 , 3331 (2020).
Tan, T. et al. Bioinspired lipoproteins-mediated photothermia remodels tumor stroma to improve cancer cell accessibility of second nanoparticles. Nat. Commun. 10 , 3322 (2019).
Miao, L., Lin, C. M. & Huang, L. Stromal barriers and strategies for the delivery of nanomedicine to desmoplastic tumors. J. Control. Rel. 219 , 192–204 (2015).
Hall, C., Lueshen, E., Mošat, A. & Linninger, A. A. Interspecies scaling in pharmacokinetics: a novel whole-body physiologically based modeling framework to discover drug biodistribution mechanisms in vivo. J. Pharm. Sci. 101 , 1221–1241 (2012).
Nair, A. B. & Jacob, S. A simple practice guide for dose conversion between animals and human. J. Basic. Clin. Pharm. 7 , 27–31 (2016).
Elmeliegy, M., Udata, C., Liao, K. & Yin, D. Considerations on the calculation of the human equivalent dose from toxicology studies for biologic anticancer agents. Clin. Pharmacokinetics 60 , 563–567 (2021).
Li, Y., Wang, J., Wientjes, M. G. & Au, J. L. Delivery of nanomedicines to extracellular and intracellular compartments of a solid tumor. Adv. Drug. Deliv. Rev. 64 , 29–39 (2012).
Heldin, C. H., Rubin, K., Pietras, K. & Ostman, A. High interstitial fluid pressure – an obstacle in cancer therapy. Nat. Rev. Cancer 4 , 806–813 (2004).
Danhier, F. To exploit the tumor microenvironment: since the EPR effect fails in the clinic, what is the future of nanomedicine? J. Control. Rel. 244 , 108–121 (2016).
Gualdrón-López, M. et al. Multiparameter flow cytometry analysis of the human spleen applied to studies of plasma-derived EVs from Plasmodium vivax patients. Front. Cell Infect. Microbiol. 11 , 596104 (2021).
Bjornson-Hooper, Z. B. et al. A comprehensive atlas of immunological differences between humans, mice, and non-human primates. Front. Immunol. 13 , 867015 (2022).
Europeam Medicines Agency. Assessment report: Imlygic. EMA www.ema.europa.eu/en/documents/assessment-report/imlygic-epar-public-assessment-report_en.pdf (2015).
Europeam Medicines Agency. Annex I: Summary of product characteristics. Imlygic. EMA www.ema.europa.eu/en/documents/product-information/imlygic-epar-product-information_en.pdf (2015).
Barrett, J. A. et al. Regulated intratumoral expression of IL-12 using a RheoSwitch Therapeutic System(®) (RTS(®)) gene switch as gene therapy for the treatment of glioma. Cancer Gene Ther. 25 , 106–116 (2018).
Hotz, C. et al. Local delivery of mRNA-encoded cytokines promotes antitumor immunity and tumor eradication across multiple preclinical tumor models. Sci. Transl. Med. 13 , eabc7804 (2021).
BioNTech. US Securities and Exchange Commission. Form F-1: Registration Statement. www.sec.gov/Archives/edgar/data/1776985/000119312520022991/d838504df1.htm US Securities and Exchange Commission (2020).
Madigan, V., Zhang, F. & Dahlman, J. E. Drug delivery systems for CRISPR-based genome editors. Nat. Rev. Drug Discov. 22 , 875–894 (2023).
Liu, Z. et al. Recent advances and applications of CRISPR-Cas9 in cancer immunotherapy. Mol. Cancer 22 , 35 (2023).
Fujii, E., Kato, A. & Suzuki, M. Patient-derived xenograft (PDX) models: characteristics and points to consider for the process of establishment. J. Toxicol. Pathol. 33 , 153–160 (2020).
Rab, R. et al. Evaluating antitumor activity of Escherichia coli purine nucleoside phosphorylase against head and neck patient-derived xenografts. Cancer Rep. 6 , e1708 (2023).
Parker, W. B. et al. The use of Trichomonas vaginalis purine nucleoside phosphorylase to activate fludarabine in the treatment of solid tumors. Cancer Chemother. Pharmacol. 85 , 573–583 (2020).
Chuprin, J. et al. Humanized mouse models for immuno-oncology research. Nat. Rev. Clin. Oncol. 20 , 192–206 (2023).
Dray, B. K. et al. Mismatch repair gene mutations lead to Lynch syndrome colorectal cancer in rhesus macaques. Genes. Cancer 9 , 142–152 (2018).
Simmons, H. A. & Mattison, J. A. The incidence of spontaneous neoplasia in two populations of captive rhesus macaques ( Macaca mulatta ). Antioxid. Redox Signal. 14 , 221–227 (2011).
Simon, D., Bruno, G., Jehad, C., Maurizio, C. & Cline, J. M. Spontaneous, naturally occurring cancers in non-human primates as a translational model for cancer immunotherapy. J. Immunother. Cancer 11 , e005514 (2023).
Shah, S. B. et al. Combinatorial treatment rescues tumour-microenvironment-mediated attenuation of MALT1 inhibitors in B-cell lymphomas. Nat. Mater. 22 , 511–523 (2023).
Barbolosi, D., Ciccolini, J., Lacarelle, B., Barlési, F. & André, N. Computational oncology – mathematical modelling of drug regimens for precision medicine. Nat. Rev. Clin. Oncol. 13 , 242–254 (2016).
Fotakis, G., Trajanoski, Z. & Rieder, D. Computational cancer neoantigen prediction: current status and recent advances. Immunooncol. Technol. 12 , 100052 (2021).
Food and Drug Administration. Focus Area: Novel technologies to improve predictivity of non-clinical studies and replace, reduce, and refine reliance on animal testing. FDA www.fda.gov/science-research/focus-areas-regulatory-science-report/focus-area-novel-technologies-improve-predictivity-non-clinical-studies-and-replace-reduce-and (2022).
Cheng, F. et al. Research advances on the stability of mRNA vaccines. Viruses 15 , 668 (2023).
Polaka, S. et al. in Pharmacokinetics and Toxicokinetic Considerations Vol. 2 (ed. Tekade, R. K.) 543–567 (Academic Press, 2022).
Hemmrich, E. & McNeil, S. Active ingredient vs excipient debate for nanomedicines. Nat. Nanotechnol. 18 , 692–695 (2023).
Marden, E., Ntai, I., Bass, S. & Flühmann, B. Correction to: Reflections on FDA draft guidance for products containing nanomaterials: is the abbreviated new drug application (ANDA) a suitable pathway for nanomedicines? AAPS J. 20 , 104 (2018).
Kiaie, S. H. et al. Recent advances in mRNA-LNP therapeutics: immunological and pharmacological aspects. J. Nanobiotechnol. 20 , 276 (2022).
Sayedahmed, E. E., Kumari, R. & Mittal, S. K. Current use of adenovirus vectors and their production methods. Methods Mol. Biol. 1937 , 155–175 (2019).
Kim, J. W. et al. in: Gene Therapy for Neurological Disorders: Methods and Protocols (ed. Manfredsson, F. P.) 115–130 (Springer, 2016).
Tombácz, I. et al. Highly efficient CD4+ T cell targeting and genetic recombination using engineered CD4+ cell-homing mRNA-LNPs. Mol. Ther. 29 , 3293–3304 (2021).
Rurik, J. G. et al. CAR T cells produced in vivo to treat cardiac injury. Science 375 , 91–96 (2022).
Breda, L. et al. In vivo hematopoietic stem cell modification by mRNA delivery. Science 381 , 436–443 (2023).
Shi, D., Toyonaga, S. & Anderson, D. G. In vivo RNA delivery to hematopoietic stem and progenitor cells via targeted lipid nanoparticles. Nano Lett. 23 , 2938–2944 (2023).
Barbieri, I. & Kouzarides, T. Role of RNA modifications in cancer. Nat. Rev. Cancer 20 , 303–322 (2020).
Qiu, L., Jing, Q., Li, Y. & Han, J. RNA modification: mechanisms and therapeutic targets. Mol. Biomed. 4 , 25 (2023).
Morse, M. A. et al. Clinical trials of self-replicating RNA-based cancer vaccines. Cancer Gene Ther. 30 , 803–811 (2023).
Delgado, A. & Guddati, A. K. Clinical endpoints in oncology – a primer. Am. J. Cancer Res. 11 , 1121–1131 (2021).
PubMed PubMed Central Google Scholar
Food and Drug Administration. Diversity plans to improve enrollment of participants from underrepresented racial and ethnic populations in clinical trials; availability: draft guidance for industry. FDA www.fda.gov/regulatory-information/search-fda-guidance-documents/diversity-plans-improve-enrollment-participants-underrepresented-racial-and-ethnic-populations (2022).
Lek, A. et al. Death after high-dose rAAV9 gene therapy in a patient with Duchenne’s muscular dystrophy. N. Engl. J. Med. 389 , 1203–1210 (2023).
Ilham, S. et al. Cancer incidence in immunocompromised patients: a single-center cohort study. BMC Cancer 23 , 33 (2023).
Fourie Zirkelbach, J. et al. Improving dose-optimization processes used in oncology drug development to minimize toxicity and maximize benefit to patients. J. Clin. Oncol. 40 , 3489–3500 (2022).
Shah, M., Rahman, A., Theoret, M. R. & Pazdur, R. The drug-dosing conundrum in oncology – when less is more. N. Engl. J. Med. 385 , 1445–1447 (2021).
Khattak, A. et al. Distant metastasis-free survival results from the randomized, phase 2 mRNA-4157-P201/KEYNOTE-942 trial [abstract]. J. Clin. Oncol. 41 (Suppl. 17), LBA9503 (2023).
Ruzzi, F. et al. Virus-like particle (VLP) vaccines for cancer immunotherapy. Int J. Mol. Sci. 24 , 12963 (2023).
Tornesello, A. L., Tagliamonte, M., Buonaguro, F. M., Tornesello, M. L. & Buonaguro, L. Virus-like particles as preventive and therapeutic cancer vaccines. Vaccines 10 , 227 (2022).
Li, Y. et al. mRNA vaccine in cancer therapy: current advance and future outlook. Clin. Transl. Med. 13 , e1384 (2023).
Reichmuth, A. M., Oberli, M. A., Jaklenec, A., Langer, R. & Blankschtein, D. mRNA vaccine delivery using lipid nanoparticles. Ther. Deliv. 7 , 319–334 (2016).
Perche, F. et al. Enhancement of dendritic cells transfection in vivo and of vaccination against B16F10 melanoma with mannosylated histidylated lipopolyplexes loaded with tumor antigen messenger RNA. Nanomed. Nanotechnol. Biol. Med. 7 , 445–453 (2011).
Raimondo, T. M., Reed, K., Shi, D., Langer, R. & Anderson, D. G. Delivering the next generation of cancer immunotherapies with RNA. Cell 186 , 1535–1540 (2023).
Food and Drug Administration. Highlights of prescribing information: ONPATTRO® (patisiran). FDA www.accessdata.fda.gov/drugsatfda_docs/label/2018/210922s000lbl.pdf (2018).
Bratman, S. V. et al. Personalized circulating tumor DNA analysis as a predictive biomarker in solid tumor patients treated with pembrolizumab. Nat. Cancer 1 , 873–881 (2020).
Pessoa, L. S., Heringer, M. & Ferrer, V. P. ctDNA as a cancer biomarker: a broad overview. Crit. Rev. Oncol. Hematol. 155 , 103109 (2020).
Wen, X., Pu, H., Liu, Q., Guo, Z. & Luo, D. Circulating tumor DNA – a novel biomarker of tumor progression and its favorable detection techniques. Cancers 14 , 6025 (2022).
Nassiri, F. et al. Oncolytic DNX-2401 virotherapy plus pembrolizumab in recurrent glioblastoma: a phase 1/2 trial. Nat. Med. 29 , 1370–1378 (2023).
Download references
Acknowledgements
The authors thank J. Tindall (Emory University, Atlanta, GA) for copyediting the manuscript. The authors also thank B. Kinkead (University of Utah, Salt Lake City, UT) for critical review. The authors gratefully acknowledge funding support from the FDA Office of Orphan Products Division and R01 DE026941.
Author information
Authors and affiliations.
Wallace H. Coulter Department of Biomedical Engineering, Georgia Institute of Technology, Atlanta, GA, USA
Sebastian G. Huayamares, David Loughrey, Hyejin Kim & James E. Dahlman
Emory University School of Medicine, Atlanta, GA, USA
Sebastian G. Huayamares, David Loughrey, Hyejin Kim, James E. Dahlman & Eric J. Sorscher
Department of Pediatrics, Emory University, Atlanta, GA, USA
Eric J. Sorscher
Winship Cancer Institute, Emory University, Atlanta, GA, USA
You can also search for this author in PubMed Google Scholar
Contributions
S.G.H., D.L., E.J.S., and H.K. researched data for the article, all authors contributed substantially to discussions of content, S.G.H., D.L. and H.K. wrote the article, and all authors reviewed and/or edited the manuscript before submission.
Corresponding authors
Correspondence to James E. Dahlman or Eric J. Sorscher .
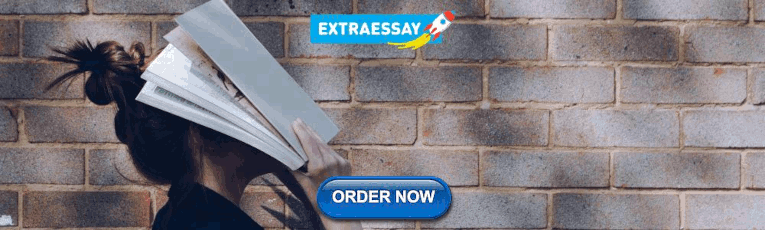
Ethics declarations
Competing interests.
J.E.D. has acted as an adviser for GV, Nava Therapeutics and Edge Animal Health. E.J.S. has ownership interests in and serves on the Board of Directors for PNP Therapeutics, and is listed as a co-inventor on patents related to some of the technologies used in the research described in this Review (including Patent IDs 17/908,465, 08/122,321 and 08/702,181). The terms of this arrangement for E.J.S. have been evaluated and approved by Emory University in accordance with its conflict-of-interest policies. The other authors declare no competing interests.
Peer review
Peer review information.
Nature Reviews Clinical Oncology thanks D. Peer, H. Kaufman and the other, anonymous, reviewers for their contribution to the peer review of this work.
Additional information
Publisher’s note Springer Nature remains neutral with regard to jurisdictional claims in published maps and institutional affiliations.
Supplementary information
Rights and permissions.
Springer Nature or its licensor (e.g. a society or other partner) holds exclusive rights to this article under a publishing agreement with the author(s) or other rightsholder(s); author self-archiving of the accepted manuscript version of this article is solely governed by the terms of such publishing agreement and applicable law.
Reprints and permissions
About this article
Cite this article.
Huayamares, S.G., Loughrey, D., Kim, H. et al. Nucleic acid-based drugs for patients with solid tumours. Nat Rev Clin Oncol (2024). https://doi.org/10.1038/s41571-024-00883-1
Download citation
Accepted : 18 March 2024
Published : 08 April 2024
DOI : https://doi.org/10.1038/s41571-024-00883-1
Share this article
Anyone you share the following link with will be able to read this content:
Sorry, a shareable link is not currently available for this article.
Provided by the Springer Nature SharedIt content-sharing initiative
Quick links
- Explore articles by subject
- Guide to authors
- Editorial policies
Sign up for the Nature Briefing: Translational Research newsletter — top stories in biotechnology, drug discovery and pharma.

- Search Menu
- Chemical Biology and Nucleic Acid Chemistry
- Computational Biology
- Critical Reviews and Perspectives
- Data Resources and Analyses
- Gene Regulation, Chromatin and Epigenetics
- Genome Integrity, Repair and Replication
- Methods Online
- Molecular Biology
- Nucleic Acid Enzymes
- RNA and RNA-protein complexes
- Structural Biology
- Synthetic Biology and Bioengineering
- Advance Articles
- Breakthrough Articles
- Special Collections
- Scope and Criteria for Consideration
- Author Guidelines
- Data Deposition Policy
- Database Issue Guidelines
- Web Server Issue Guidelines
- Submission Site
- About Nucleic Acids Research
- Editors & Editorial Board
- Information of Referees
- Self-Archiving Policy
- Dispatch Dates
- Advertising and Corporate Services
- Journals Career Network
- Journals on Oxford Academic
- Books on Oxford Academic
Article Contents
Introduction, overall design and workflow of metaboanalyst 6.0, supporting asari and ms2 spectra in lc–ms spectra processing workflow, ms2 peak annotation, causal analysis via two-sample mendelian randomization, dose–response analysis, updated compound database and knowledge libraries, other features, comparison with other tools, data availability, acknowledgements, metaboanalyst 6.0: towards a unified platform for metabolomics data processing, analysis and interpretation.

- Article contents
- Figures & tables
- Supplementary Data
Zhiqiang Pang, Yao Lu, Guangyan Zhou, Fiona Hui, Lei Xu, Charles Viau, Aliya F Spigelman, Patrick E MacDonald, David S Wishart, Shuzhao Li, Jianguo Xia, MetaboAnalyst 6.0: towards a unified platform for metabolomics data processing, analysis and interpretation, Nucleic Acids Research , 2024;, gkae253, https://doi.org/10.1093/nar/gkae253
- Permissions Icon Permissions
We introduce MetaboAnalyst version 6.0 as a unified platform for processing, analyzing, and interpreting data from targeted as well as untargeted metabolomics studies using liquid chromatography - mass spectrometry (LC–MS). The two main objectives in developing version 6.0 are to support tandem MS (MS2) data processing and annotation, as well as to support the analysis of data from exposomics studies and related experiments. Key features of MetaboAnalyst 6.0 include: (i) a significantly enhanced Spectra Processing module with support for MS2 data and the asari algorithm; (ii) a MS2 Peak Annotation module based on comprehensive MS2 reference databases with fragment-level annotation; (iii) a new Statistical Analysis module dedicated for handling complex study design with multiple factors or phenotypic descriptors; (iv) a Causal Analysis module for estimating metabolite - phenotype causal relations based on two-sample Mendelian randomization, and (v) a Dose-Response Analysis module for benchmark dose calculations. In addition, we have also improved MetaboAnalyst's visualization functions, updated its compound database and metabolite sets, and significantly expanded its pathway analysis support to around 130 species. MetaboAnalyst 6.0 is freely available at https://www.metaboanalyst.ca .

Metabolomics involves the comprehensive study of all small molecules in a biological system. It has diverse applications ranging from basic biochemical research to clinical investigation of diseases, food safety assessment, environmental monitoring, etc. ( 1–5 ). User-friendly and easily accessible bioinformatics tools are essential to deal with the complex data produced from metabolomics studies. MetaboAnalyst is a user-friendly, web-based platform developed to provide comprehensive support for metabolomics data analysis ( 6–10 ). The early versions (1.0–3.0) focused primarily on supporting statistical and functional analysis of targeted metabolomics data. Increasing support for untargeted metabolomics data from liquid chromatography–mass spectrometry (LC–MS) experiments have been gradually introduced in more recent versions of MetaboAnalyst. For instance, version 4.0 implemented a new module to support functional analysis directly from LC–MS peaks, while version 5.0 added an auto-optimized LC–MS spectral processing module that works seamlessly with the functional analysis module. A detailed protocol on how to use different modules for comprehensive analysis of untargeted metabolomics data was published in 2022 ( 11 ). According to Google Analytics, the MetaboAnalyst web server has processed over 2 million jobs, including 33 000 spectral processing jobs over the past 12 months. Many of these jobs are associated with untargeted metabolomics and exposomics studies.
Untargeted metabolomics data generated from high-resolution LC–MS instruments are typically characterized by thousands of peaks with unknown chemical identities. To assist with compound identification, tandem MS (called MS/MS or MS2) spectra are often collected from pooled QC samples during the experiments ( 12 ). The two commonly used MS2 methods are data-dependent acquisition (DDA) and data-independent acquisition (DIA), with sequential window acquisition of all theoretical mass spectra (SWATH) being a promising special case of the latter. DDA data usually have clear associations between the precursor ions and the corresponding MS2 spectra, while DIA data generally require deconvolution of the MS2 data to reconstruct associations with their precursor ions ( 13 ). Incorporating MS2 processing and annotation into untargeted metabolomics workflows can greatly improve compound annotations and functional interpretation.
Exposomics is an emerging field centered on profiling the complete set of exposures individuals encounter across their lifespan, which often involves MS analysis of chemical mixtures traditionally rooted in toxicology and public health ( 4 ). Untargeted LC–MS based metabolomics is increasingly applied to exposomics and toxicology studies. Exposomics data from human cohorts is often associated with complex phenotypic data due to their observational nature. This requires more sophisticated data analysis and visualization methods that can take into consideration of multiple factors or covariates. Exposomics studies typically produce long lists of potential biomarkers that are significantly associated with phenotypes of interest. Identification of causal links from this large number of metabolite-phenotype relations is a natural next step. It has become possible recently with the availability of many metabolomic genome-wide association studies (mGWAS) that link metabolites and genotypes ( 14–16 ). By integrating mGWAS data with comparable GWAS data that associate genotypes with various phenotypes ( 17 ), we can now estimate causal relationships between a metabolite and a phenotype of interest through Mendelian randomization (MR) ( 18 ). Dose-response experiments are often performed to further quantify cause-and-effect relationships. The experiments are often conducted at multiple dose levels using in vitro assays or animal models to calculate dose-response curves for risk assessment of chemical exposures ( 19–21 ).
To address these emerging needs from both the metabolomics and exposomics communities, we have developed MetaboAnalyst version 6.0. This version includes many key features:
A significantly enhanced spectra processing workflow with the addition of asari algorithm for LC–MS spectra processing ( 22 ), as well as support for MS2 (DDA or SWATH-DIA) data processing.
A new module for MS2 spectral database searching for compound identification and results visualization.
A new module for causal analysis between metabolites and phenotypes of interest based on two-sample MR (2SMR).
A new module for dose-response analysis including dose-response curve fitting and benchmark dose (BMD) calculation.
A new module for statistical analysis with complex metadata;
A number of other important updates including: improved functional analysis of untargeted metabolomics data by integrating MS2-based compound identification; updated compound database, pathways and metabolite sets; as well as improved data visualization support across multiple modules.
MetaboAnalyst 6.0 is feely accessible at https://www.metaboanalyst.ca , with comprehensive documentations and updated tutorials. To better engage with our users, a dedicated user forum ( https://omicsforum.ca ) has been operational since May 2022. To dates, this forum contains >4000 posts on ∼700 topics related to different aspects of using MetaboAnalyst.
MetaboAnalyst 6.0 accepts a total of five different data types across various modules encompassing spectra processing, statistical analysis, functional analysis, meta-analysis, and integration with other omics data. Once the data are uploaded, all analysis steps are conducted within a consistent framework including data integrity checks, parameter customization, and results visualization (Figure 1 ). Some of the key features in MetaboAnalyst 6.0 are described below.

MetaboAnalyst 6.0 workflow for targeted and untargeted metabolomics data. Multiple data input types are accepted. Untargeted metabolomics inputs require extra steps for spectra processing and peak annotation. The result table can be used for statistical and functional analysis within a consistent workflow in the same manner as for targeted metabolomics data.
LC–MS spectra processing remains an active research topic in the field of untargeted metabolomics. Many powerful tools have been developed over time, including XCMS ( 23 ), MZmine ( 24 ), MS-DIAL ( 13 ) and asari ( 22 ). In addition to using different peak detection algorithms, most tools require manual parameter tuning to ensure good results. Such practice often leads to results that vary significantly ( 25 ). To mitigate this issue, MetaboAnalyst 5.0 introduced an auto-optimized LC–MS processing pipeline to minimize the parameter-related effects ( 10 , 26 ). The asari software has introduced a set of quality metrics, concepts of mass tracks and composite mass tracks and new algorithmic design to minimize errors in feature correspondence. It requires minimal parameter tuning while achieving much faster computational performance ( 22 ). The asari algorithm is now available in the LC–MS spectra processing options, alongside the traditional approaches.
MS2 spectra processing and metabolite identification are important components of untargeted metabolomics. It is now recognized that MS2 spectral deconvolution is necessary to achieve high-quality compound identification results for both DDA and SWATH-DIA data ( 27–29 ). MetaboAnalyst 6.0 offers an efficient, auto-optimized pipeline for MS2 spectral deconvolution. The DDA data deconvolution method is derived from the DecoID algorithm ( 28 ), which employs a database-dependent regression model to deconvolve contaminated spectra. The SWATH-DIA data deconvolution algorithm is based on the DecoMetDIA method ( 29 ), with the core algorithm re-implemented using a Rcpp/C++ framework to achieve high performance. When MS2 spectra replicates are provided, an extra step will be performed to generate consensus spectra across replicates. The consensus spectra are searched against MetaboAnalyst's curated MS2 reference databases for compound identification based on dot product ( 28 ) or spectral entropy ( 30 ) similarity scores. The complete pipelines for DDA and SWATH-DIA are available from the Spectra Processing [LC–MS w/wo MS2] module.
Raw spectra must be saved in common open formats and uploaded individually as separate zip files. LC–MS spectra data is mandatory, while MS2 is optional. Upon data uploading, MetaboAnalyst 6.0 first validates the status of the MS files. For SWATH-DIA data, the SWATH window design is automatically extracted from the spectra. If the related information is missing, users will be prompted to manually enter the window design. On the parameters setting page, users can choose the auto-optimized centWave algorithm ( 26 ) or the asari algorithm for LC–MS data processing. If MS2 data is included, spectra deconvolution, consensus, and database searching will be performed using the identified MS features as target list. Once the spectra processing is complete, users can explore both MS and MS2 data processing results (Figure 2A - B ) and download the files or directly go to the Functional Analysis module.

Example outputs from MetaboAnalyst 6.0. ( A ) Integrated 3D PCA score and loading plots summarizing the raw spectra processing results. ( B ) An interactive mirror plot showing the MS2 matching result. Matched fragments are marked with a red diamond. ( C ) Functional analysis results with the top four significant pathways labelled. ( D ) A forest plot comparing the effect sizes calculated based on individual SNPs (black) or using all SNPs by different MR methods (red). ( E ) Bar plots of the dose response curve fitting results showing how many times each model type was identified as the best fit. ( F ) A dose-response curve fitting result showing each of the concentration values (black points), the fitted curve (solid blue line), and the estimated benchmark dose (solid red line) with its lower and upper 95% confidence intervals (dashed red lines), respectively.
MS2 data could be acquired independently from MS data acquisition. To accommodate this scenario and offer compatibility with MS2 spectra results from other popular tools such as MS-DIAL, we have added a Peak Annotation [MS2-DDA/DIA] module to allow users to directly upload MS2 spectra for database searching. Users can enter a single MS2 spectrum or upload an MSP or MGF file containing multiple MS2 spectra. For single spectrum searching, users must specify the m/z value of the precursor ion. However, for batch searching based on an MSP file, users do not need to specify the precursors’ m/z values. To ensure timely completion of database searching, the public server processes only 20 spectra for each submission (the first 20 spectra by default). Users can manually specify spectra for searching. After conducting this pilot analysis with 20 spectra, users can download the R command history and use our MetaboAnalystR package to annotate all MS2 spectra ( 26 ).
Multiple databases are available for compound identification. Database searching can be performed based on regular reference MS2 spectra and/or their corresponding neutral loss spectra. The results are visually summarized as mirror plots based on the matching scores (Figure 2B). Users can interactively explore the MS2 database matching results. The molecular formulas for the MS2 peaks in the reference database spectra are predicted using the BUDDY program ( 31 ). Users can download the complete compound identification table together with the mirror plots.
Understanding the causal relationships between metabolites and phenotypes is of great interest in both metabolomics and exposomics. GWAS have established links between genetic variants (e.g. single nucleotide polymorphism, or SNPs) and various phenotypes ( 32 ), while recent mGWAS provide connections between genotypes with metabolites or metabolite concentration changes. It becomes possible to estimate causal relationships between metabolites and a phenotype of interest. If a metabolite is causal for a given disease, genetic variants which influence the levels of that metabolite, either directly through affecting related enzymes or indirectly through influencing lifestyle choices (such as dietary habits), should result in a higher risk of the disease. These causal effects can be estimated through Mendelian randomization (MR) analysis ( 18 ). MR relies on the principle that genetic variants are randomly distributed across populations, similar to how treatments are randomly assigned in clinical trials. By leveraging this random allocation, MR can evaluate whether a relationship between a metabolite and a phenotype is causal, while reducing the impact of confounding factors and reverse causality that often plague observational studies.
MR analysis in MetaboAnalyst is based on the 2SMR approach (using the TwoSampleMR and MRInstruments R packages) which enables application of MR methods using summary statistics from non-overlapping individuals ( 17 , 33 ). Users should first select an exposure (i.e. a metabolite) and an outcome (i.e. a disease) of interest. Based on the selections, the program searches for potential instrumental variables (i.e. SNPs) that are associated with both the metabolite from our large collections of the recent mGWAS studies ( 14 ) and the disease from the OpenGWAS database ( 17 ). The next step is to perform SNP filtering and harmonization to identify independent SNPs through linkage disequilibrium (LD) clumping ( 34 ). When SNPs are absent in the GWAS database, proxy SNPs are identified using LD. In addition, it is critical to harmonize SNPs to make sure effect sizes for the SNPs on both exposures and the outcomes are for the same reference alleles. The last step before conducting MR analysis is to exclude SNPs affecting multiple metabolites to reduce horizontal pleiotropy which occurs when a genetic variant influences the outcome through pathways other than the exposure of interest ( 35 ). MetaboAnalyst's MR analysis page provides diverse statistical methods (currently 12), each of which has its own strengths and limitations. For instance, the weighted median method is robust to the violation of MR assumptions by some of the genetic variants, while Egger regression method is more robust to horizontal pleiotropy. Users can point their mouse over the corresponding question marks beside each method to learn more details.
Dose–response analysis is commonly used in toxicology and pharmacology for understanding how varying concentrations of a chemical can impact a biological system. It plays a pivotal role in risk assessment of chemical exposures ( 36 ). A key output of dose-response analysis is the benchmark dose (BMD), the minimum dose of a substance that produces a clear, low level health risk relative to the control group ( 37 ). Chemicals identified from exposomics are often followed up by dose–response studies to understand their mechanism of action or adverse outcome pathways ( 21 , 38 , 39 ).
Dose–response experiment design includes a control group (dose = 0) and at least three different dose groups, typically with the same number of replicates in each group. The data should be formatted as a csv file with their dose information included as the second row or column. The analysis workflow consists of four main steps: (i) data upload, integrity checking, processing and normalization; (ii) differential analysis to select features that vary with dose levels; (iii) curve fitting on the intensity or concentration values of those selected features against a suite of linear and non-linear models, and (iv) computing BMD values for each feature. The algorithm for dose–response analysis was adapted from the algorithm we developed for transcriptomics BMD analysis ( 40 , 41 ).
Compound database
The compound database has been updated based on HMDB 5.0 ( 42 ), with particular efforts made to synchronize with the IDs of other databases such as KEGG ( 43 ) and PubChem ( 44 ) to improve cross-references during compound mapping and pathway analysis. The compound database was expanded by ∼4000 compounds (after removing ∼10 000 deprecated HMDB entries and adding ∼14 000 new entries).
MS2 reference spectra database.
A total of 12 MS2 reference databases were collected and curated from public resources, including the HMDB experimental MS2 database ( 42 ), the HMDB predicted MS2 database ( 42 ), Global Natural Product Social Molecular Networking (GNPS) database ( 45 ), MoNA ( 46 ), MassBank ( 46 ), MINEs ( 47 ), LipidBlast ( 48 ), RIKEN ( 49 ), ReSpect ( 50 ), BMDMS ( 51 ), VaniyaNP ( 46 ) and the MS-DIAL database (v4.90) ( 52 ). The complete MS2 reference database currently comprises 10 420 215 MS2 records from 1 551 012 unique compounds. We also created a neutral loss spectra database calculated based on the algorithm implemented by the METLIN neutral loss database ( 53 ). The molecular formula of all MS2 fragments were pre-calculated using BUDDY ( 31 ).
Pathway and metabolite set libraries
The KEGG pathway libraries have been updated to their recent version (12/20/2023) via KEGG API. Based on user feedback, the pathway analysis for both targeted and untargeted metabolomics data now supports ∼130 species (up from 28 species in version 5.0), including many new mammals, plants, insects, fungi, and bacteria, etc. We also updated the metabolite set libraries based on HMDB 5.0, MarkerDB ( 54 ), as well as manual curation. For instance, a total of 62 metabolite sets associated with dietary and chemical exposures were added during this process. The metabolite set library also incorporated ∼3700 pathways downloaded from the RaMP-DB ( 55 ).
Statistical analysis with complex metadata
The Statistical Analysis [metadata table] module in MetaboAnalyst 6.0 now provides a comprehensive suite of methods for analyzing and visualizing metabolomics data in relation to various metadata, be it discrete or continuous. Users can quickly assess the correlation patterns among different experimental factors using the metadata overview heatmaps or interactive PCA visualization. The interactive heatmap visualization coupled with hierarchical clustering allows users to easily explore feature abundance variations across different samples and metadata variables. The statistical methods in this module include both univariate linear models with covariate adjustment as well as multivariate methods such as ANOVA Simultaneous Component Analysis ( 56 , 57 ). Random forest is offered for classification with consideration of different metadata variables of interest. More details about this module can be found in our recently published protocol ( 11 ).
Enhanced functional analysis for untargeted metabolomics
Functional analysis of untargeted metabolomics was initially established based on mummichog and Gene Set Enrichment Analysis (GSEA) since MetaboAnalyst 4.0 ( 58 ). It was further enhanced in MetaboAnalyst 5.0 by incorporating retention time into calculating empirical compounds. MetaboAnalyst 6.0 now allows users to upload an LC–MS peak list along with a corresponding MS2-based compound list to filter out unrealistic empirical compounds to further improve the accuracy in functional analysis ( 59 ).
Enhanced data visualization support
We have enhanced the quality of the interactive and synchronized 3D plots across the dimensionality reduction methods (PCA, PLS-DA, sPLS-DA) used in MetaboAnalyst based on the powerful three.js library ( https://threejs.org/ ). New features include customizable backgrounds, data point annotations and confidence ellipsoids (Figure 2A ). We have also implemented interactive plots for clustering heatmaps in the Statistical Analysis modules to better support visual exploration of large data matrices typical in untargeted metabolomics. Both mouse-over and zoom-in functionalities are supported to allow users to examine specific features or patterns of interest. In addition to these enhancements, we also updated the visualization for KEGG’s global metabolic network ( 43 ).
To illustrate the utility of the new features of MetaboAnalyst 6.0, we used a metabolomics dataset collected in-house that aimed at studying glucose-induced insulin secretion in isolated human islets. The dataset contains five samples of high-glucose (16.7 mM) exposures, five samples of low-glucose (2.8 mM) exposures, both for 30 min, and five quality control (QC) samples. The LC-MS spectra were collected using our Q-Exactive Orbitrap platform (Thermo Scientific, Waltham, MA USA), together with three SWATH-DIA acquisitions from the pooled QC. The spectra were first centroided and converted into mzML format using ProteoWizard ( 60 , 61 ) and uploaded to MetaboAnalyst 6.0. LC–MS spectra processing was performed using the asari algorithm. All detected MS1 features were used as a target list for MS2 deconvolution and database searching. A total of 27 209 MS1 features were detected, with 4959 of them identified with at least one potential named chemical identity. Functional analysis using the mummichog algorithm indicated compounds showing significant changes between the high-glucose and low-glucose groups were involved in the C arnitine shuttle , C affeine, Tryptophan , and C oenzyme A metabolism pathways (Figure 2C ). These pathways have been consistently identified in previous studies ( 62–65 ). Finally, we performed a causal analysis on the associations between one of the significant metabolites identified, L-Cystathionine and type 2 diabetes (GWAS ID: finn-b-E4_DM2). The default parameters were used for both SNP filtering and harmonization, as well as MR analysis. Based on these results, a significantly altered cystathionine level was found to have a causal effect on type 2 diabetes (Figure 2D ), which aligns well with a study published recently ( 66 ). This case study highlights how MetaboAnalyst 6.0 allows users to investigate the chemical identities of MS peaks, elucidate associations between metabolites and phenotypes to unveil previously unknown functional insights. To showcase the dose-response analysis module, we utilized a published data collected from BT549 breast cancer cells treated with four different doses of etomoxir ( 21 ). Figure 2E summarizes the results from dose-response modeling. Figure 2F shows an example feature-level BMD calculated based on the fitted curve. The workflow is included as a series of tutorials on our website.
Several web-based tools have been developed to address various aspects of metabolomics data processing, statistical analysis, functional interpretation, and results visualization. Table 1 compares the main features of MetaboAnalyst 6.0 with other popular tools including the previous version, XCMS online ( 23 ), GNPS ( 45 ), Workflow4Metabolomics (W4M) ( 67 ) and MetExplore ( 68 ). For raw data processing, MetaboAnalyst primarily focuses on supporting LC–MS data, whereas W4M also supports GC–MS and NMR raw data processing, and GNPS emphasizes MS2-based compound identification via molecular networks. In comparison, MetaboAnalyst provides an auto-optimized workflow along with an additional algorithm (asari) for efficient LC–MS spectra processing, together with more extensive MS2 spectra libraries for compound identification. In terms of statistical analysis, MetaboAnalyst 6.0 has introduced new modules for dealing with complex metadata, causal analysis and dose–response analysis, while maintaining all other functionalities. MetaboAnalyst contains unique features for enrichment and pathway analysis, and these strengths were further improved in version 6.0, with the addition of unique functions and supports for more species. For network analysis and integration, MetExplore specializes in metabolic network visualization and integration with other omics. These features are addressed by our companion tool, OmicsNet ( 69 ). Overall, MetaboAnalyst 6.0 continues to be the most comprehensive tool for metabolomics data processing, analysis and interpretation.
Comparison of MetaboAnalyst 6.0 with its previous version and other common web-based metabolomics tools. Symbols used for feature evaluations with ‘√’ for present, ‘-’ for absent, and ‘+’ for a more quantitative assessment (more ‘+’ indicate better support)
• XCMS online: https://xcmsonline.scripps.edu/ .
• GNPS: https://gnps.ucsd.edu/ .
• Workflow4Metabolomics (W4M): https://workflow4metabolomics.org/ .
• MetExplore: https://metexplore.toulouse.inra.fr/metexplore2/
By incorporating a new MS2 data processing workflow, MetaboAnalyst 6.0 now offers a web-based, end-to-end platform for metabolomics data analysis. The workflow spans from raw MS spectra processing to compound identification to functional analysis. A key motivation in developing version 6.0 was to support the data analysis needs emerging from exposomics and follow-up validation studies. The new statistical analysis module specifically takes into account of complex metadata to better identify robust associations. From these associations, users can perform causal analysis based on 2SMR to narrow down candidate compounds. The remaining compounds can be validated through dose-response studies based on in vitro or animal models. Our case study highlights the streamlined analysis workflow from raw spectra processing to compound annotation, to functional interpretation, and finally to causal insights. In conclusion, MetaboAnalyst 6.0 is a user-friendly platform for comprehensive analysis of metabolomics data and help address emerging needs from recent exposomics research. For future directions, we will continue to improve metabolome annotations, better integrate with other omics data, and explore new ways to interact with users via generative artificial intelligence technologies ( 70–73 ).
MetaboAnalyst 6.0 is freely available at https://www.metaboanalyst.ca . No log in required.
Human islets for research were provided by the Alberta Diabetes Institute IsletCore at the University of Alberta in Edmonton with the assistance of the Human Organ Procurement and Exchange (HOPE) program, Trillium Gift of Life Network (TGLN), and other Canadian organ procurement organizations. Islet isolation was approved by the Human Research Ethics Board at the University of Alberta (Pro00013094). All donors’ families gave informed consent for the use of pancreatic tissue in research.
This research was funded by Genome Canada, Canadian Foundation for Innovation (CFI), US National Institutes of Health (U01 CA235493), Canadian Institutes of Health Research (CIHR), Juvenile Diabetes Research Foundation (JDRF), Natural Sciences and Engineering Research Council of Canada (NSERC), and Diabetes Canada. Funding for open access charge: NSERC.
Conflict of interest statement . J. Xia is the founder of XiaLab Analytics.
Lloyd-Price J. , Arze C. , Ananthakrishnan A.N. , Schirmer M. , Avila-Pacheco J. , Poon T.W. , Andrews E. , Ajami N.J. , Bonham K.S. , Brislawn C.J. et al. . Multi-omics of the gut microbial ecosystem in inflammatory bowel diseases . Nature . 2019 ; 569 : 655 – 662 .
Google Scholar
Utpott M. , Rodrigues E. , Rios A.O. , Mercali G.D. , Flores S.H. Metabolomics: an analytical technique for food processing evaluation . Food Chem. 2022 ; 366 : 130685 .
Wishart D.S. Metabolomics for investigating physiological and pathophysiological processes . Physiol. Rev. 2019 ; 99 : 1819 – 1875 .
Vermeulen R. , Schymanski E.L. , Barabasi A.L. , Miller G.W. The exposome and health: where chemistry meets biology . Science . 2020 ; 367 : 392 – 396 .
Danzi F. , Pacchiana R. , Mafficini A. , Scupoli M.T. , Scarpa A. , Donadelli M. , Fiore A. To metabolomics and beyond: a technological portfolio to investigate cancer metabolism . Signal. Transduct. Target. Ther. 2023 ; 8 : 137 .
Xia J. , Psychogios N. , Young N. , Wishart D.S. MetaboAnalyst: a web server for metabolomic data analysis and interpretation . Nucleic Acids Res. 2009 ; 37 : W652 – W660 .
Xia J. , Mandal R. , Sinelnikov I.V. , Broadhurst D. , Wishart D.S. MetaboAnalyst 2.0—A comprehensive server for metabolomic data analysis . Nucleic Acids Res. 2012 ; 40 : W127 – W133 .
Xia J. , Sinelnikov I.V. , Han B. , Wishart D.S. MetaboAnalyst 3.0—Making metabolomics more meaningful . Nucleic Acids Res. 2015 ; 43 : W251 – W257 .
Chong J. , Soufan O. , Li C. , Caraus I. , Li S. , Bourque G. , Wishart D.S. , Xia J. MetaboAnalyst 4.0: towards more transparent and integrative metabolomics analysis . Nucleic Acids Res. 2018 ; 46 : W486 – W494 .
Pang Z. , Chong J. , Zhou G. , de Lima Morais D.A. , Chang L. , Barrette M. , Gauthier C. , Jacques P.-É. , Li S. , Xia J. MetaboAnalyst 5.0: narrowing the gap between raw spectra and functional insights . Nucleic Acids Res. 2021 ; 49 : W388 – W396 .
Pang Z. , Zhou G. , Ewald J. , Chang L. , Hacariz O. , Basu N. , Xia J. Using MetaboAnalyst 5.0 for LC–HRMS spectra processing, multi-omics integration and covariate adjustment of global metabolomics data . Nat. Protoc. 2022 ; 17 : 1735 – 1761 .
Frigerio G. , Moruzzi C. , Mercadante R. , Schymanski E.L. , Fustinoni S. Development and application of an LC–MS/MS untargeted exposomics method with a separated pooled quality control strategy . Molecules . 2022 ; 27 : 2580 .
Tsugawa H. , Cajka T. , Kind T. , Ma Y. , Higgins B. , Ikeda K. , Kanazawa M. , VanderGheynst J. , Fiehn O. , Arita M. MS-DIAL: data-independent MS/MS deconvolution for comprehensive metabolome analysis . Nat. Methods . 2015 ; 12 : 523 – 526 .
Chang L. , Zhou G. , Xia J. mGWAS-Explorer 2.0: causal analysis and interpretation of metabolite-phenotype associations . Metabolites . 2023 ; 13 : 826 .
Shin S.Y. , Fauman E.B. , Petersen A.K. , Krumsiek J. , Santos R. , Huang J. , Arnold M. , Erte I. , Forgetta V. , Yang T.P. et al. . An atlas of genetic influences on human blood metabolites . Nat. Genet. 2014 ; 46 : 543 – 550 .
Chen Y. , Lu T. , Pettersson-Kymmer U. , Stewart I.D. , Butler-Laporte G. , Nakanishi T. , Cerani A. , Liang K.Y.H. , Yoshiji S. , Willett J.D.S. et al. . Genomic atlas of the plasma metabolome prioritizes metabolites implicated in human diseases . Nat. Genet. 2023 ; 55 : 44 – 53 .
Hemani G. , Zheng J. , Elsworth B. , Wade K.H. , Haberland V. , Baird D. , Laurin C. , Burgess S. , Bowden J. , Langdon R. et al. . The MR-Base platform supports systematic causal inference across the human phenome . eLife . 2018 ; 7 : e34408 .
Sanderson E. , Glymour M.M. , Holmes M.V. , Kang H. , Morrison J. , Munafò M.R. , Palmer T. , Schooling C.M. , Wallace C. , Zhao Q. et al. . Mendelian randomization . Nat. Rev. Methods Primers . 2022 ; 2 : 6 .
Zhao H. , Liu M. , Lv Y. , Fang M. Dose-response metabolomics and pathway sensitivity to map molecular cartography of bisphenol A exposure . Environ. Int. 2022 ; 158 : 106893 .
Thomas R.S. , Wesselkamper S.C. , Wang N.C.Y. , Zhao Q.J. , Petersen D.D. , Lambert J.C. , Cote I. , Yang L. , Healy E. , Black M.B. et al. . Temporal concordance between apical and transcriptional points of departure for chemical risk assessment . Toxicol. Sci. 2013 ; 134 : 180 – 194 .
Yao C.-H. , Wang L. , Stancliffe E. , Sindelar M. , Cho K. , Yin W. , Wang Y. , Patti G.J. Dose-response metabolomics to understand biochemical mechanisms and off-target drug effects with the TOXcms software . Anal. Chem. 2020 ; 92 : 1856 – 1864 .
Li S. , Siddiqa A. , Thapa M. , Chi Y. , Zheng S. Trackable and scalable LC–MS metabolomics data processing using asari . Nat. Commun. 2023 ; 14 : 4113 .
Tautenhahn R. , Patti G.J. , Rinehart D. , Siuzdak G. XCMS Online: a web-based platform to process untargeted metabolomic data . Anal. Chem. 2012 ; 84 : 5035 – 5039 .
Schmid R. , Heuckeroth S. , Korf A. , Smirnov A. , Myers O. , Dyrlund T.S. , Bushuiev R. , Murray K.J. , Hoffmann N. , Lu M. et al. . Integrative analysis of multimodal mass spectrometry data in MZmine 3 . Nat. Biotechnol. 2023 ; 41 : 447 – 449 .
Myers O.D. , Sumner S.J. , Li S. , Barnes S. , Du X. Detailed investigation and comparison of the XCMS and MZmine 2 chromatogram construction and chromatographic peak detection methods for preprocessing mass spectrometry metabolomics data . Anal. Chem. 2017 ; 89 : 8689 – 8695 .
Pang Z. , Chong J. , Li S. , Xia J. MetaboAnalystR 3.0: toward an optimized workflow for global metabolomics . Metabolites . 2020 ; 10 : 186 .
Xing S. , Yu H. , Liu M. , Jia Q. , Sun Z. , Fang M. , Huan T. Recognizing contamination fragment ions in liquid chromatography–Tandem mass spectrometry data . J. Am. Soc. Mass. Spectrom. 2021 ; 32 : 2296 – 2305 .
Stancliffe E. , Schwaiger-Haber M. , Sindelar M. , Patti G.J. DecoID improves identification rates in metabolomics through database-assisted MS/MS deconvolution . Nat. Methods . 2021 ; 18 : 779 – 787 .
Yin Y. , Wang R. , Cai Y. , Wang Z. , Zhu Z.-J. DecoMetDIA: deconvolution of multiplexed MS/MS spectra for metabolite identification in SWATH-MS-based untargeted metabolomics . Anal. Chem. 2019 ; 91 : 11897 – 11904 .
Li Y. , Kind T. , Folz J. , Vaniya A. , Mehta S.S. , Fiehn O. Spectral entropy outperforms MS/MS dot product similarity for small-molecule compound identification . Nat. Methods . 2021 ; 18 : 1524 – 1531 .
Xing S. , Shen S. , Xu B. , Li X. , Huan T. BUDDY: molecular formula discovery via bottom-up MS/MS interrogation . Nat. Methods . 2023 ; 20 : 881 – 890 .
Sollis E. , Mosaku A. , Abid A. , Buniello A. , Cerezo M. , Gil L. , Groza T. , Gunes O. , Hall P. , Hayhurst J. et al. . The NHGRI-EBI GWAS Catalog: knowledgebase and deposition resource . Nucleic Acids Res. 2023 ; 51 : D977 – D985 .
Hemani G. , Tilling K. , Davey Smith G. Orienting the causal relationship between imprecisely measured traits using GWAS summary data . PLos Genet. 2017 ; 13 : e1007081 .
Marees A.T. , de Kluiver H. , Stringer S. , Vorspan F. , Curis E. , Marie-Claire C. , Derks E.M. A tutorial on conducting genome-wide association studies: quality control and statistical analysis . Int. J. Methods Psychiatr. Res. 2018 ; 27 : e1608 .
de Leeuw C. , Savage J. , Bucur I.G. , Heskes T. , Posthuma D Understanding the assumptions underlying mendelian randomization . Eur. J. Hum. Genet. 2022 ; 30 : 653 – 660 .
Altshuler B. Modeling of dose-response relationships . Environ. Health Perspect. 1981 ; 42 : 23 – 27 .
Thomas R.S. , Wesselkamper S.C. , Wang N.C. , Zhao Q.J. , Petersen D.D. , Lambert J.C. , Cote I. , Yang L. , Healy E. , Black M.B. et al. . Temporal concordance between apical and transcriptional points of departure for chemical risk assessment . Toxicol. Sci. 2013 ; 134 : 180 – 194 .
Kleensang A. , Maertens A. , Rosenberg M. , Fitzpatrick S. , Lamb J. , Auerbach S. , Brennan R. , Crofton K.M. , Gordon B. , Fornace A.J. Jr et al. . Pathways of toxicity . ALTEX . 2014 ; 31 : 53 – 61 .
Ewald J. , Soufan O. , Xia J. , Basu N. FastBMD: an online tool for rapid benchmark dose–response analysis of transcriptomics data . Bioinformatics . 2020 ; 37 : 1035 – 1036 .
Ewald J. , Zhou G. , Lu Y. , Xia J. Using ExpressAnalyst for comprehensive gene expression analysis in model and non-model organisms . Curr Protoc . 2023 ; 3 : e922 .
Wishart D.S. , Guo A. , Oler E. , Wang F. , Anjum A. , Peters H. , Dizon R. , Sayeeda Z. , Tian S. , Lee B.L. et al. . HMDB 5.0: the Human Metabolome Database for 2022 . Nucleic Acids Res. 2021 ; 50 : D622 – D631 .
Kanehisa M. , Furumichi M. , Sato Y. , Kawashima M. , Ishiguro-Watanabe M. KEGG for taxonomy-based analysis of pathways and genomes . Nucleic Acids Res. 2022 ; 51 : D587 – D592 .
Kim S. Exploring chemical information in PubChem . Curr. Protoc. 2021 ; 1 : e217 .
Aron A.T. , Gentry E.C. , McPhail K.L. , Nothias L.-F. , Nothias-Esposito M. , Bouslimani A. , Petras D. , Gauglitz J.M. , Sikora N. , Vargas F. et al. . Reproducible molecular networking of untargeted mass spectrometry data using GNPS . Nat. Protoc. 2020 ; 15 : 1954 – 1991 .
Horai H. , Arita M. , Kanaya S. , Nihei Y. , Ikeda T. , Suwa K. , Ojima Y. , Tanaka K. , Tanaka S. , Aoshima K. et al. . MassBank: a public repository for sharing mass spectral data for life sciences . J. Mass Spectrom. 2010 ; 45 : 703 – 714 .
Jeffryes J.G. , Colastani R.L. , Elbadawi-Sidhu M. , Kind T. , Niehaus T.D. , Broadbelt L.J. , Hanson A.D. , Fiehn O. , Tyo K.E. , Henry C.S. MINEs: open access databases of computationally predicted enzyme promiscuity products for untargeted metabolomics . J Cheminform . 2015 ; 7 : 44 .
Kind T. , Liu K.-H. , Lee D.Y. , DeFelice B. , Meissen J.K. , Fiehn O. LipidBlast in silico tandem mass spectrometry database for lipid identification . Nat. Methods . 2013 ; 10 : 755 – 758 .
Tsugawa H. , Nakabayashi R. , Mori T. , Yamada Y. , Takahashi M. , Rai A. , Sugiyama R. , Yamamoto H. , Nakaya T. , Yamazaki M. et al. . A cheminformatics approach to characterize metabolomes in stable-isotope-labeled organisms . Nat. Methods . 2019 ; 16 : 295 – 298 .
Sawada Y. , Nakabayashi R. , Yamada Y. , Suzuki M. , Sato M. , Sakata A. , Akiyama K. , Sakurai T. , Matsuda F. , Aoki T. et al. . RIKEN tandem mass spectral database (ReSpect) for phytochemicals: a plant-specific MS/MS-based data resource and database . Phytochemistry . 2012 ; 82 : 38 – 45 .
Lee S. , Hwang S. , Seo M. , Shin K.B. , Kim K.H. , Park G.W. , Kim J.Y. , Yoo J.S. , No K.T. BMDMS-NP: a comprehensive ESI-MS/MS spectral library of natural compounds . Phytochemistry . 2020 ; 177 : 112427 .
Tsugawa H. , Ikeda K. , Takahashi M. , Satoh A. , Mori Y. , Uchino H. , Okahashi N. , Yamada Y. , Tada I. , Bonini P. et al. . A lipidome atlas in MS-DIAL 4 . Nat. Biotechnol. 2020 ; 38 : 1159 – 1163 .
Aisporna A. , Benton H.P. , Chen A. , Derks R.J.E. , Galano J.M. , Giera M. , Siuzdak G. Neutral loss mass spectral data enhances molecular similarity analysis in METLIN . J. Am. Soc. Mass. Spectrom. 2022 ; 33 : 530 – 534 .
Wishart D.S. , Bartok B. , Oler E. , Liang K.Y.H. , Budinski Z. , Berjanskii M. , Guo A. , Cao X. , Wilson M. MarkerDB: an online database of molecular biomarkers . Nucleic Acids Res. 2021 ; 49 : D1259 – D1267 .
Braisted J. , Patt A. , Tindall C. , Sheils T. , Neyra J. , Spencer K. , Eicher T. , Mathé E.A. RaMP-DB 2.0: a renovated knowledgebase for deriving biological and chemical insight from metabolites, proteins, and genes . Bioinformatics . 2023 ; 39 : btac726 .
Smilde A.K. , Jansen J.J. , Hoefsloot H.C. , Lamers R.J. , van der Greef J. , Timmerman M.E. ANOVA-simultaneous component analysis (ASCA): a new tool for analyzing designed metabolomics data . Bioinformatics . 2005 ; 21 : 3043 – 3048 .
Ritchie M.E. , Phipson B. , Wu D. , Hu Y. , Law C.W. , Shi W. , Smyth G.K. limma powers differential expression analyses for RNA-sequencing and microarray studies . Nucleic Acids Res. 2015 ; 43 : e47 .
Li S. , Park Y. , Duraisingham S. , Strobel F.H. , Khan N. , Soltow Q.A. , Jones D.P. , Pulendran B. Predicting network activity from high throughput metabolomics . PLoS Comput. Biol. 2013 ; 9 : e1003123 .
Lu Y. , Pang Z. , Xia J. Comprehensive investigation of pathway enrichment methods for functional interpretation of LC–MS global metabolomics data . Brief. Bioinform. 2023 ; 24 : bbac553 .
Chambers M.C. , Maclean B. , Burke R. , Amodei D. , Ruderman D.L. , Neumann S. , Gatto L. , Fischer B. , Pratt B. , Egertson J. et al. . A cross-platform toolkit for mass spectrometry and proteomics . Nat. Biotechnol. 2012 ; 30 : 918 – 920 .
Adusumilli R. , Mallick P. Data conversion with ProteoWizard msConvert . Methods Mol. Biol. 2017 ; 1550 : 339 – 368 .
Bene J. , Hadzsiev K. , Melegh B. Role of carnitine and its derivatives in the development and management of type 2 diabetes . Nutr Diabetes . 2018 ; 8 : 8 .
Lane J.D. , Barkauskas C.E. , Surwit R.S. , Feinglos M.N. Caffeine impairs glucose metabolism in type 2 diabetes . Diabetes Care. 2004 ; 27 : 2047 – 2048 .
Unluturk U. , Erbas T. Engin A. , Engin A.B. Tryptophan Metabolism: Implications for Biological Processes, Health and Disease . 2015 ; Cham Springer International Publishing 147 – 171 .
Google Preview
Jackowski S. , Leonardi R. Deregulated coenzyme A, loss of metabolic flexibility and diabetes . Biochem. Soc. Trans. 2014 ; 42 : 1118 – 1122 .
Cruciani-Guglielmacci C. , Meneyrol K. , Denom J. , Kassis N. , Rachdi L. , Makaci F. , Migrenne-Li S. , Daubigney F. , Georgiadou E. , Denis R.G. et al. . Homocysteine metabolism pathway is involved in the control of glucose homeostasis: a cystathionine beta synthase deficiency study in mouse . Cells . 2022 ; 11 : 1737 .
Giacomoni F. , Le Corguillé G. , Monsoor M. , Landi M. , Pericard P. , Pétéra M. , Duperier C. , Tremblay-Franco M. , Martin J.-F. , Jacob D. et al. . Workflow4Metabolomics: a collaborative research infrastructure for computational metabolomics . Bioinformatics . 2014 ; 31 : 1493 – 1495 .
Cottret L. , Frainay C. , Chazalviel M. , Cabanettes F. , Gloaguen Y. , Camenen E. , Merlet B. , Heux S. , Portais J.C. , Poupin N. et al. . MetExplore: collaborative edition and exploration of metabolic networks . Nucleic Acids Res. 2018 ; 46 : W495 – W502 .
Zhou G. , Pang Z. , Lu Y. , Ewald J. , Xia J. OmicsNet 2.0: a web-based platform for multi-omics integration and network visual analytics . Nucleic Acids Res. 2022 ; 50 : W527 – W533 .
Lu Y. , Zhou G. , Ewald J. , Pang Z. , Shiri T. , Xia J. MicrobiomeAnalyst 2.0: comprehensive statistical, functional and integrative analysis of microbiome data . Nucleic Acids Res. 2023 ; 51 : W310 – W318 .
Liu P. , Ewald J. , Pang Z. , Legrand E. , Jeon Y.S. , Sangiovanni J. , Hacariz O. , Zhou G. , Head J.A. , Basu N. et al. . ExpressAnalyst: a unified platform for RNA-sequencing analysis in non-model species . Nat. Commun. 2023 ; 14 : 2995 .
Zhou G. , Ewald J. , Xia J. OmicsAnalyst: a comprehensive web-based platform for visual analytics of multi-omics data . Nucleic Acids Res. 2021 ; 49 : W476 – W482 .
Moor M. , Banerjee O. , Abad Z.S.H. , Krumholz H.M. , Leskovec J. , Topol E.J. , Rajpurkar P. Foundation models for generalist medical artificial intelligence . Nature . 2023 ; 616 : 259 – 265 .
Email alerts
Citing articles via.
- Editorial Board
Affiliations
- Online ISSN 1362-4962
- Print ISSN 0305-1048
- Copyright © 2024 Oxford University Press
- About Oxford Academic
- Publish journals with us
- University press partners
- What we publish
- New features
- Open access
- Institutional account management
- Rights and permissions
- Get help with access
- Accessibility
- Advertising
- Media enquiries
- Oxford University Press
- Oxford Languages
- University of Oxford
Oxford University Press is a department of the University of Oxford. It furthers the University's objective of excellence in research, scholarship, and education by publishing worldwide
- Copyright © 2024 Oxford University Press
- Cookie settings
- Cookie policy
- Privacy policy
- Legal notice
This Feature Is Available To Subscribers Only
Sign In or Create an Account
This PDF is available to Subscribers Only
For full access to this pdf, sign in to an existing account, or purchase an annual subscription.
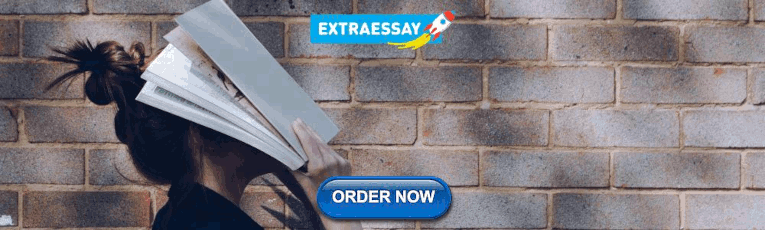
IMAGES
VIDEO
COMMENTS
Standard research articles are published on a variety of subject categories including chemical biology and nucleic acid chemistry, computational biology, data resources and analyses, gene regulation, chromatin and epigenetics, molecular biology, RNA and RNA-protein complexes, structural biology and more. Browse this article type
The structure of DNA. ( A) A nucleotide (guanosine triphosphate). The nitrogenous base (guanine in this example) is linked to the 1′ carbon of the deoxyribose and the phosphate groups are linked to the 5′ carbon. A nucleoside is a base linked to a sugar. A nucleotide is a nucleoside with one or more phosphate groups.
NEW AND UPDATED DATABASES. The 28th annual Nucleic Acids Research Database Issue contains 189 papers spanning, as usual, a wide range of biology. Unsurprisingly, COVID-19 casts a long shadow over the Issue. Seven new databases specifically address the pandemic and the SARS-CoV-2 virus responsible (Table (Table1) 1) but new and returning databases in all areas have rushed to support research ...
In recent years, nucleic acids have emerged as powerful biomaterials, revolutionizing the field of biomedicine. This review explores the multifaceted applications of nucleic acids, focusing on their pivotal role in various biomedical applications. Nucleic acids, including deoxyribonucleic acid (DNA) and ribonucleic acid (RNA), possess unique properties such as molecular recognition ability ...
Read the latest Research articles in Nucleic acids from Nature. ... Nucleic acids articles within Nature. Featured. Article | 21 June 2023. Intricate 3D architecture of a DNA mimic of GFP.
Nucleic acids articles from across Nature Portfolio. ... Research Highlights 20 Oct 2022 Nature Chemical Biology. ... Cas12f1 is a member of type V Cas12 family, which has a hypercompact size. ...
Here, Bou-Nader et al., define the nucleic acid-binding specificity of S9.6 and report its crystal structures free and bound to a hybrid, which reveal the asymmetric recognition of the RNA and DNA ...
Nucleic acids are the storage of genetic information. It is an example of biopolymer because of long-chain polymer of nucleotide. Deoxyribonucleic acid (DNA) and ribonucleic acid (RNA) are two types of nucleic acids. DNA and RNA have an important function in the biological system.
This article covers the chemistry of nucleic acids, describing the structures and properties that allow them to serve as the transmitters of genetic information. For a discussion of the genetic code, see heredity, and for a discussion of the role played by nucleic acids in protein synthesis, see metabolism. Nucleotides: building blocks of ...
Abstract. Nucleic acids are emerging as powerful and functional biomaterials due to their molecular recognition ability, programmability, and ease of synthesis and chemical modification. Various types of nucleic acids have been used as gene regulation tools or therapeutic agents for the treatment of human diseases with genetic disorders.
Abstract. The 2024 Nucleic Acids Research database issue contains 180 papers from across biology and neighbouring disciplines. There are 90 papers reporting on new databases and 83 updates from resources previously published in the Issue. Updates from databases most recently published elsewhere account for a further seven.
This article continues the series of Surveys and Summaries on restriction endonucleases (REases) begun this year in Nucleic Acids Research. Here we discuss 'Type II' REases, the kind used for DNA analysis and cloning. We focus on their biochemistry: what they are, what they do, and how they do it. T …
This timeline depicts key discoveries and events during the development of nucleic acid therapeutics, with a specific focus on the delivery platform technologies reviewed herein. Each timeline ...
Deoxyribose nucleic acid (DNA) is a linear or cyclic polymer composed of four deoxyribonucleotides linked by 3′-5′ phosphodiester bonds, which serve as the genetic material in cells. ... Types of DNA damage and research progress. Liu Nian a School of Chemistry and Life Sciences, Suzhou University of Science and Technology, Suzhou, China;b ...
The 'Nucleic acid databases' section begins with updates from the International Nucleotide Sequence Database Collaboration and its three contributors, GenBank, ENA and DDBJ (6-8) which together face the challenge of continued exponential growth in nucleic acid sequence data. Transcription factors (TF) and transcriptional regulation are ...
G-quadruplex (G4) nucleic acid structures have been reported to be involved in several human pathologies, including cancer, neurodegenerative disorders and infectious diseases; however, G4 targeting compounds still need implementation in terms of drug-like properties and selectivity in order to reach the clinical use.
Read the latest Research articles in Nucleic acids from Scientific Reports. ... Nucleic acids articles within Scientific Reports. Featured. Article 27 November 2023 | Open Access.
The CRISPR/Cas systems offer a programmable platform for nucleic acid detection, and CRISPR/Cas-based diagnostics (CRISPR-Dx) have demonstrated the ability to target nucleic acids with greater accuracy and flexibility. However, due to the configuration of the reporter and the underlying labeling mechanism, almost all reported CRISPR-Dx rely on a single-option readout, resulting in limitations ...
Nucleic Acids Research | 51 | 3 | February 2023. Cover: The phosphorothioate (PS) backbone is the most widely used modification in therapeutic antisense oligonucleotides (ASO). Introduction of PS linkages to ASO increases its interaction with cellular proteins, but the molecular mechanisms that underlie this effect are poorly understood.
A related nucleic acid-type strategy involves the use ... studies of both cell type and tissue targeting to optimize antitumour immune activity are key areas of ongoing nucleic acid drug research.
Materials and methods. The computational workflow of SingmiR consists of two main stages. First, the alignment and trimming pipeline, which removes the adapters specific to the library preparation method used, aligns the reads to the human genome and quantifies miRNA abundances. Second, an optional analysis pipeline computes overview plots and ...
Wild-type (WT) or ARID1A-KO AID-DIvA cells were treated with 300 nM 4-hydroxytamoxifen (4OHT) for 4 h to induce AsiSI introgression into the nucleus and generation of DSBs. DSB induction was terminated by Auxin supply as described previously . The type of repair pathway acting at the different DSB sites had been described previously .
Introduction. The biomedical literature is a primary resource to address information needs across the biological and clinical sciences (), however the requirements for literature search vary widely.Activities such as formulating a research hypothesis require an exploratory approach, whereas tasks like interpreting the clinical significance of genetic variants are more focused.
Introduction. Metabolomics involves the comprehensive study of all small molecules in a biological system. It has diverse applications ranging from basic biochemical research to clinical investigation of diseases, food safety assessment, environmental monitoring, etc. ().User-friendly and easily accessible bioinformatics tools are essential to deal with the complex data produced from ...