The Three Most Common Types of Hypotheses
In this post, I discuss three of the most common hypotheses in psychology research, and what statistics are often used to test them.
- Post author By sean
- Post date September 28, 2013
- 37 Comments on The Three Most Common Types of Hypotheses

Simple main effects (i.e., X leads to Y) are usually not going to get you published. Main effects can be exciting in the early stages of research to show the existence of a new effect, but as a field matures the types of questions that scientists are trying to answer tend to become more nuanced and specific. In this post, I’ll briefly describe the three most common kinds of hypotheses that expand upon simple main effects – at least, the most common ones I’ve seen in my research career in psychology – as well as providing some resources to help you learn about how to test these hypotheses using statistics.
Incremental Validity
“Can X predict Y over and above other important predictors?”

This is probably the simplest of the three hypotheses I propose. Basically, you attempt to rule out potential confounding variables by controlling for them in your analysis. We do this because (in many cases) our predictor variables are correlated with each other. This is undesirable from a statistical perspective, but is common with real data. The idea is that we want to see if X can predict unique variance in Y over and above the other variables you include.
In terms of analysis, you are probably going to use some variation of multiple regression or partial correlations. For example, in my own work I’ve shown in the past that friendship intimacy as coded from autobiographical narratives can predict concern for the next generation over and above numerous other variables, such as optimism, depression, and relationship status ( Mackinnon et al., 2011 ).
“Under what conditions does X lead to Y?”
Of the three techniques I describe, moderation is probably the most tricky to understand. Essentially, it proposes that the size of a relationship between two variables changes depending upon the value of a third variable, known as a “moderator.” For example, in the diagram below you might find a simple main effect that is moderated by sex. That is, the relationship is stronger for women than for men:
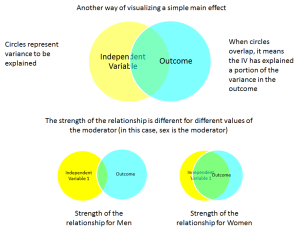
With moderation, it is important to note that the moderating variable can be a category (e.g., sex) or it can be a continuous variable (e.g., scores on a personality questionnaire). When a moderator is continuous, usually you’re making statements like: “As the value of the moderator increases, the relationship between X and Y also increases.”
“Does X predict M, which in turn predicts Y?”
We might know that X leads to Y, but a mediation hypothesis proposes a mediating, or intervening variable. That is, X leads to M, which in turn leads to Y. In the diagram below I use a different way of visually representing things consistent with how people typically report things when using path analysis.

I use mediation a lot in my own research. For example, I’ve published data suggesting the relationship between perfectionism and depression is mediated by relationship conflict ( Mackinnon et al., 2012 ). That is, perfectionism leads to increased conflict, which in turn leads to heightened depression. Another way of saying this is that perfectionism has an indirect effect on depression through conflict.
Helpful links to get you started testing these hypotheses
Depending on the nature of your data, there are multiple ways to address each of these hypotheses using statistics. They can also be combined together (e.g., mediated moderation). Nonetheless, a core understanding of these three hypotheses and how to analyze them using statistics is essential for any researcher in the social or health sciences. Below are a few links that might help you get started:
Are you a little rusty with multiple regression? The basics of this technique are required for most common tests of these hypotheses. You might check out this guide as a helpful resource:
https://statistics.laerd.com/spss-tutorials/multiple-regression-using-spss-statistics.php
David Kenny’s Mediation Website provides an excellent overview of mediation and moderation for the beginner.
http://davidakenny.net/cm/mediate.htm
http://davidakenny.net/cm/moderation.htm
Preacher and Haye’s INDIRECT Macro is a great, easy way to implement mediation in SPSS software, and their MODPROBE macro is a useful tool for testing moderation.
http://afhayes.com/spss-sas-and-mplus-macros-and-code.html
If you want to graph the results of your moderation analyses, the excel calculators provided on Jeremy Dawson’s webpage are fantastic, easy-to-use tools:
http://www.jeremydawson.co.uk/slopes.htm
- Tags mediation , moderation , regression , tutorial
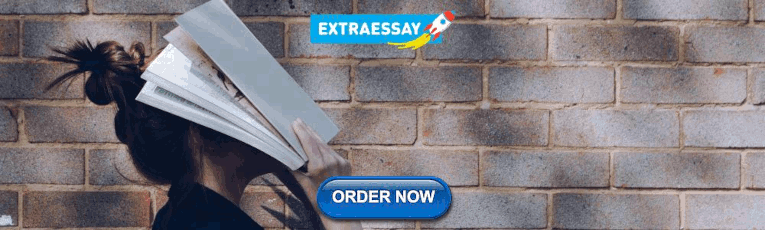
37 replies on “The Three Most Common Types of Hypotheses”
I want to see clearly the three types of hypothesis
Thanks for your information. I really like this
Thank you so much, writing up my masters project now and wasn’t sure whether one of my variables was mediating or moderating….Much clearer now.
Thank you for simplified presentation. It is clearer to me now than ever before.
Thank you. Concise and clear
hello there
I would like to ask about mediation relationship: If I have three variables( X-M-Y)how many hypotheses should I write down? Should I have 2 or 3? In other words, should I have hypotheses for the mediating relationship? What about questions and objectives? Should be 3? Thank you.
Hi Osama. It’s really a stylistic thing. You could write it out as 3 separate hypotheses (X -> Y; X -> M; M -> Y) or you could just write out one mediation hypotheses “X will have an indirect effect on Y through M.” Usually, I’d write just the 1 because it conserves space, but either would be appropriate.
Hi Sean, according to the three steps model (Dudley, Benuzillo and Carrico, 2004; Pardo and Román, 2013)., we can test hypothesis of mediator variable in three steps: (X -> Y; X -> M; X and M -> Y). Then, we must use the Sobel test to make sure that the effect is significant after using the mediator variable.
Yes, but this is older advice. Best practice now is to calculate an indirect effect and use bootstrapping, rather than the causal steps approach and the more out-dated Sobel test. I’d recommend reading Hayes (2018) book for more info:
Hayes, A. F. (2018). Introduction to mediation, moderation, and conditional process analysis: A regression-based approach (2nd ed). Guilford Publications.
Hi! It’s been really helpful but I still don’t know how to formulate the hypothesis with my mediating variable.
I have one dependent variable DV which is formed by DV1 and DV2, then I have MV (mediating variable), and then 2 independent variables IV1, and IV2.
How many hypothesis should I write? I hope you can help me 🙂
Thank you so much!!
If I’m understanding you correctly, I guess 2 mediation hypotheses:
IV1 –> Med –> DV1&2 IV2 –> Med –> DV1&2
Thank you so much for your quick answer! ^^
Could you help me formulate my research question? English is not my mother language and I have trouble choosing the right words. My x = psychopathy y = aggression m = deficis in emotion recognition
thank you in advance
I have mediator and moderator how should I make my hypothesis
Can you have a negative partial effect? IV – M – DV. That is my M will have negative effect on the DV – e.g Social media usage (M) will partial negative mediate the relationship between father status (IV) and social connectedness (DV)?
Thanks in advance
Hi Ashley. Yes, this is possible, but often it means you have a condition known as “inconsistent mediation” which isn’t usually desirable. See this entry on David Kenny’s page:
Or look up “inconsistent mediation” in this reference:
MacKinnon, D. P., Fairchild, A. J., & Fritz, M. S. (2007). Mediation analysis. Annual Review of Psychology, 58, 593-614.
This is very interesting presentation. i love it.
This is very interesting and educative. I love it.
Hello, you mentioned that for the moderator, it changes the relationship between iv and dv depending on its strength. How would one describe a situation where if the iv is high iv and dv relationship is opposite from when iv is low. And then a 3rd variable maybe the moderator increases dv when iv is low and decreases dv when iv is high.
This isn’t problematic for moderation. Moderation just proposes that the magnitude of the relationship changes as levels of the moderator changes. If the sign flips, probably the original relationship was small. Sometimes people call this a “cross-over” effect, but really, it’s nothing special and can happen in any moderation analysis.
i want to use an independent variable as moderator after this i will have 3 independent variable and 1 dependent variable…. my confusion is do i need to have some past evidence of the X variable moderate the relationship of Y independent variable and Z dependent variable.
Dear Sean It is really helpful as my research model will use mediation. Because I still face difficulty in developing hyphothesis, can you give examples ? Thank you
Hi! is it possible to have all three pathways negative? My regression analysis showed significant negative relationships between x to y, x to m and m to y.
Hi, I have 1 independent variable, 1 dependent variable and 4 mediating variable May I know how many hypothesis should I develop?
Hello I have 4 IV , 1 mediating Variable and 1 DV
My model says that 4 IVs when mediated by 1MV leads to 1 Dv
Pls tell me how to set the hypothesis for mediation
Hi I have 4 IVs ,2 Mediating Variables , 1DV and 3 Outcomes (criterion variables).
Pls can u tell me how many hypotheses to set.
Thankyou in advance
I am in fact happy to read this webpage posts which carries tons of useful information, thanks for providing such data.
I see you don’t monetize savvystatistics.com, don’t waste your traffic, you can earn additional bucks every month with new monetization method. This is the best adsense alternative for any type of website (they approve all websites), for more info simply search in gooogle: murgrabia’s tools
what if the hypothesis and moderator significant in regrestion and insgificant in moderation?
Thank you so much!! Your slide on the mediator variable let me understand!
Very informative material. The author has used very clear language and I would recommend this for any student of research/
Hi Sean, thanks for the nice material. I have a question: for the second type of hypothesis, you state “That is, the relationship is stronger for men than for women”. Based on the illustration, wouldn’t the opposite be true?
Yes, your right! I updated the post to fix the typo, thank you!
I have 3 independent variable one mediator and 2 dependant variable how many hypothesis I have 2 write?
Sounds like 6 mediation hypotheses total:
X1 -> M -> Y1 X2 -> M -> Y1 X3 -> M -> Y1 X1 -> M -> Y2 X2 -> M -> Y2 X3 -> M -> Y2
Clear explanation! Thanks!
Leave a Reply Cancel reply
Your email address will not be published. Required fields are marked *
Save my name, email, and website in this browser for the next time I comment.
Have a language expert improve your writing
Run a free plagiarism check in 10 minutes, generate accurate citations for free.
- Knowledge Base
Methodology
- Mediator vs. Moderator Variables | Differences & Examples
Mediator vs. Moderator Variables | Differences & Examples
Published on March 1, 2021 by Pritha Bhandari . Revised on June 22, 2023.
A mediating variable (or mediator ) explains the process through which two variables are related, while a moderating variable (or moderator ) affects the strength and direction of that relationship.
Including mediators and moderators in your research helps you go beyond studying a simple relationship between two variables for a fuller picture of the real world. These variables are important to consider when studying complex correlational or causal relationships between variables.
Including these variables can also help you avoid or mitigate several research biases , like observer bias , survivorship bias , undercoverage bias , or omitted variable bias
Table of contents
What’s the difference, mediating variables, moderating variables, other interesting articles, frequently asked questions about mediators and moderators.
You can think of a mediator as a go-between for two variables. For example, sleep quality (an independent variable ) can affect academic achievement (a dependent variable) through the mediator of alertness. In a mediation relationship, you can draw an arrow from an independent variable to a mediator and then from the mediator to the dependent variable.
In contrast, a moderator is something that acts upon the relationship between two variables and changes its direction or strength. For example, mental health status may moderate the relationship between sleep quality and academic achievement: the relationship might be stronger for people without diagnosed mental health conditions than for people with them.
In a moderation relationship, you can draw an arrow from the moderator to the relationship between an independent and dependent variable.
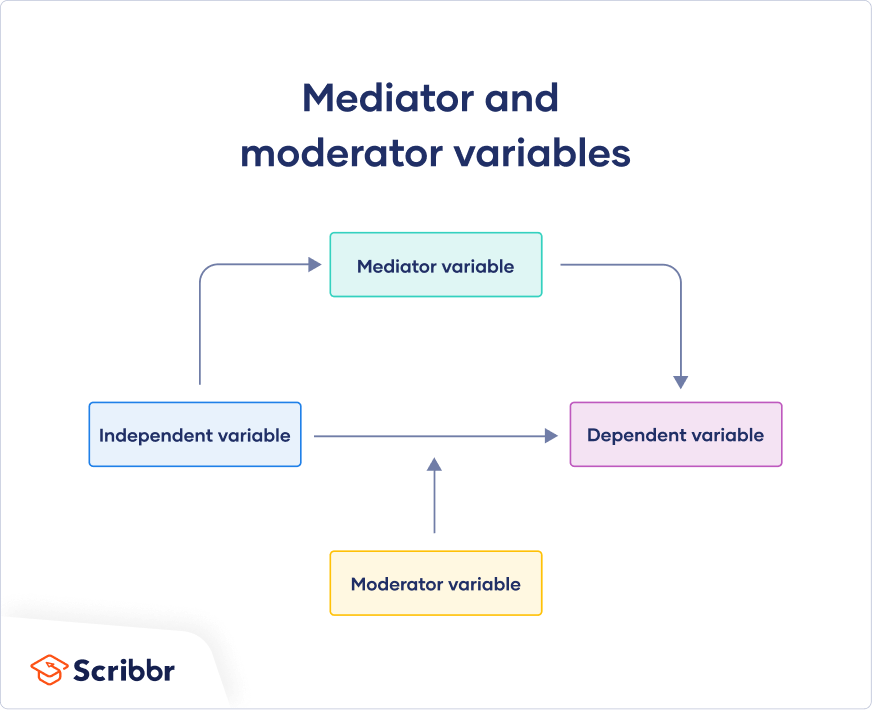
Here's why students love Scribbr's proofreading services
Discover proofreading & editing
A mediator is a way in which an independent variable impacts a dependent variable. It’s part of the causal pathway of an effect, and it tells you how or why an effect takes place.
If something is a mediator:
- It’s caused by the independent variable.
- It influences the dependent variable
- When it’s taken into account, the statistical correlation between the independent and dependent variables is higher than when it isn’t considered.
Mediation analysis is a way of statistically testing whether a variable is a mediator using linear regression analyses or ANOVAs .
In full mediation , a mediator fully explains the relationship between the independent and dependent variable: without the mediator in the model, there is no relationship.
In partial mediation , there is still a statistical relationship between the independent and dependent variable even when the mediator is taken out of a model: the mediator only partially explains the relationship.
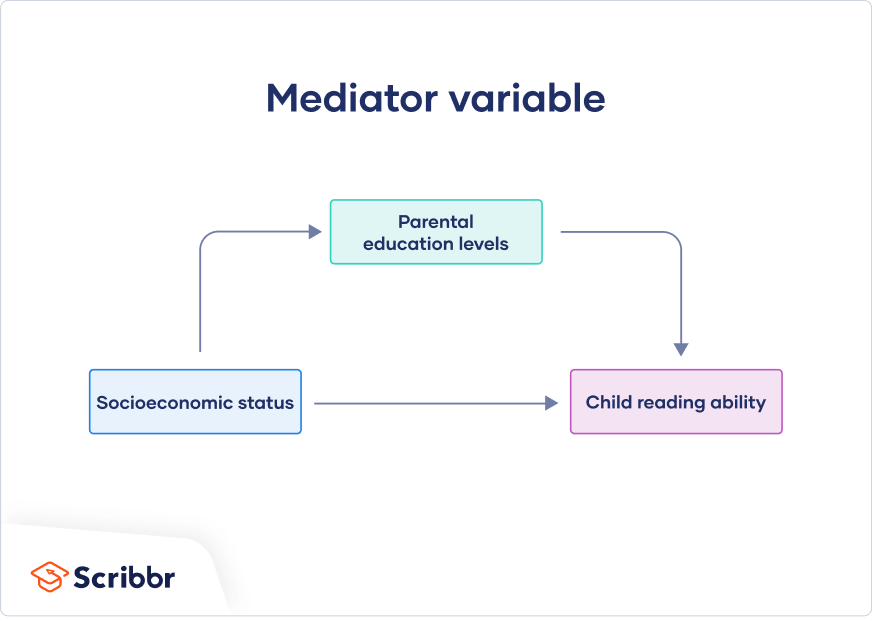
You use a descriptive research design for this study. After collecting data on each of these variables, you perform statistical analysis to check whether:
- Socioeconomic status predicts parental education levels,
- Parental education levels predicts child reading ability,
- The correlation between socioeconomic status and child reading ability is greater when parental education levels are taken into account in your model.
A moderator influences the level, direction, or presence of a relationship between variables. It shows you for whom, when, or under what circumstances a relationship will hold.
Moderators usually help you judge the external validity of your study by identifying the limitations of when the relationship between variables holds. For example, while social media use can predict levels of loneliness, this relationship may be stronger for adolescents than for older adults. Age is a moderator here.
Moderators can be:
- Categorical variables such as ethnicity, race, religion, favorite colors, health status, or stimulus type,
- Quantitative variables such as age, weight, height, income, or visual stimulus size.
- years of work experience predicts salary, when controlling for relevant variables,
- gender identity moderates the relationship between work experience and salary.
This means that the relationship between years of experience and salary would differ between men, women, and those who do not identify as men or women.
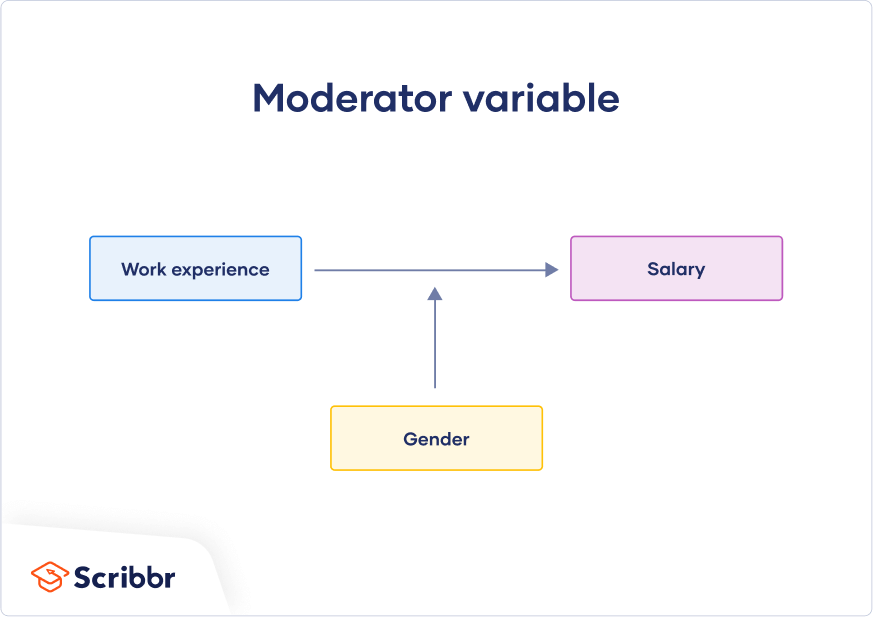
If you want to know more about statistics , methodology , or research bias , make sure to check out some of our other articles with explanations and examples.
- Normal distribution
- Degrees of freedom
- Null hypothesis
- Discourse analysis
- Control groups
- Mixed methods research
- Non-probability sampling
- Quantitative research
- Ecological validity
Research bias
- Rosenthal effect
- Implicit bias
- Cognitive bias
- Selection bias
- Negativity bias
- Status quo bias
Prevent plagiarism. Run a free check.
A mediator variable explains the process through which two variables are related, while a moderator variable affects the strength and direction of that relationship.
A confounder is a third variable that affects variables of interest and makes them seem related when they are not. In contrast, a mediator is the mechanism of a relationship between two variables: it explains the process by which they are related.
Including mediators and moderators in your research helps you go beyond studying a simple relationship between two variables for a fuller picture of the real world. They are important to consider when studying complex correlational or causal relationships.
Mediators are part of the causal pathway of an effect, and they tell you how or why an effect takes place. Moderators usually help you judge the external validity of your study by identifying the limitations of when the relationship between variables holds.
If something is a mediating variable :
- It’s caused by the independent variable .
Cite this Scribbr article
If you want to cite this source, you can copy and paste the citation or click the “Cite this Scribbr article” button to automatically add the citation to our free Citation Generator.
Bhandari, P. (2023, June 22). Mediator vs. Moderator Variables | Differences & Examples. Scribbr. Retrieved April 15, 2024, from https://www.scribbr.com/methodology/mediator-vs-moderator/
Is this article helpful?
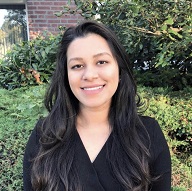
Pritha Bhandari
Other students also liked, independent vs. dependent variables | definition & examples, control variables | what are they & why do they matter, confounding variables | definition, examples & controls, unlimited academic ai-proofreading.
✔ Document error-free in 5minutes ✔ Unlimited document corrections ✔ Specialized in correcting academic texts
- cognitive sophistication
- tolerance of diversity
- exposure to higher levels of math or science
- age (which is currently related to educational level in many countries)
- social class and other variables.
- For example, suppose you designed a treatment to help people stop smoking. Because you are really dedicated, you assigned the same individuals simultaneously to (1) a "stop smoking" nicotine patch; (2) a "quit buddy"; and (3) a discussion support group. Compared with a group in which no intervention at all occurred, your experimental group now smokes 10 fewer cigarettes per day.
- There is no relationship among two or more variables (EXAMPLE: the correlation between educational level and income is zero)
- Or that two or more populations or subpopulations are essentially the same (EXAMPLE: women and men have the same average science knowledge scores.)
- the difference between two and three children = one child.
- the difference between eight and nine children also = one child.
- the difference between completing ninth grade and tenth grade is one year of school
- the difference between completing junior and senior year of college is one year of school
- In addition to all the properties of nominal, ordinal, and interval variables, ratio variables also have a fixed/non-arbitrary zero point. Non arbitrary means that it is impossible to go below a score of zero for that variable. For example, any bottom score on IQ or aptitude tests is created by human beings and not nature. On the other hand, scientists believe they have isolated an "absolute zero." You can't get colder than that.
- Chapter 1: Introduction
- Chapter 2: Indexing
- Chapter 3: Loops & Logicals
- Chapter 4: Apply Family
- Chapter 5: Plyr Package
- Chapter 6: Vectorizing
- Chapter 7: Sample & Replicate
- Chapter 8: Melting & Casting
- Chapter 9: Tidyr Package
- Chapter 10: GGPlot1: Basics
- Chapter 11: GGPlot2: Bars & Boxes
- Chapter 12: Linear & Multiple
- Chapter 13: Ploting Interactions
- Chapter 14: Moderation/Mediation
- Chapter 15: Moderated-Mediation
- Chapter 16: MultiLevel Models
- Chapter 17: Mixed Models
- Chapter 18: Mixed Assumptions Testing
- Chapter 19: Logistic & Poisson
- Chapter 20: Between-Subjects
- Chapter 21: Within- & Mixed-Subjects
- Chapter 22: Correlations
- Chapter 23: ARIMA
- Chapter 24: Decision Trees
- Chapter 25: Signal Detection
- Chapter 26: Intro to Shiny
- Chapter 27: ANOVA Variance
- Download Rmd
Chapter 14: Mediation and Moderation
Alyssa blair, 1 what are mediation and moderation.
Mediation analysis tests a hypothetical causal chain where one variable X affects a second variable M and, in turn, that variable affects a third variable Y. Mediators describe the how or why of a (typically well-established) relationship between two other variables and are sometimes called intermediary variables since they often describe the process through which an effect occurs. This is also sometimes called an indirect effect. For instance, people with higher incomes tend to live longer but this effect is explained by the mediating influence of having access to better health care.
In R, this kind of analysis may be conducted in two ways: Baron & Kenny’s (1986) 4-step indirect effect method and the more recent mediation package (Tingley, Yamamoto, Hirose, Keele, & Imai, 2014). The Baron & Kelly method is among the original methods for testing for mediation but tends to have low statistical power. It is covered in this chapter because it provides a very clear approach to establishing relationships between variables and is still occassionally requested by reviewers. However, the mediation package method is highly recommended as a more flexible and statistically powerful approach.
Moderation analysis also allows you to test for the influence of a third variable, Z, on the relationship between variables X and Y. Rather than testing a causal link between these other variables, moderation tests for when or under what conditions an effect occurs. Moderators can stength, weaken, or reverse the nature of a relationship. For example, academic self-efficacy (confidence in own’s ability to do well in school) moderates the relationship between task importance and the amount of test anxiety a student feels (Nie, Lau, & Liau, 2011). Specifically, students with high self-efficacy experience less anxiety on important tests than students with low self-efficacy while all students feel relatively low anxiety for less important tests. Self-efficacy is considered a moderator in this case because it interacts with task importance, creating a different effect on test anxiety at different levels of task importance.
In general (and thus in R), moderation can be tested by interacting variables of interest (moderator with IV) and plotting the simple slopes of the interaction, if present. A variety of packages also include functions for testing moderation but as the underlying statistical approaches are the same, only the “by hand” approach is covered in detail in here.
Finally, this chapter will cover these basic mediation and moderation techniques only. For more complicated techniques, such as multiple mediation, moderated mediation, or mediated moderation please see the mediation package’s full documentation.
1.1 Getting Started
If necessary, review the Chapter on regression. Regression test assumptions may be tested with gvlma . You may load all the libraries below or load them as you go along. Review the help section of any packages you may be unfamiliar with ?(packagename).
2 Mediation Analyses
Mediation tests whether the effects of X (the independent variable) on Y (the dependent variable) operate through a third variable, M (the mediator). In this way, mediators explain the causal relationship between two variables or “how” the relationship works, making it a very popular method in psychological research.
Both mediation and moderation assume that there is little to no measurement error in the mediator/moderator variable and that the DV did not CAUSE the mediator/moderator. If mediator error is likely to be high, researchers should collect multiple indicators of the construct and use SEM to estimate latent variables. The safest ways to make sure your mediator is not caused by your DV are to experimentally manipulate the variable or collect the measurement of your mediator before you introduce your IV.

Total Effect Model.

Basic Mediation Model.
c = the total effect of X on Y c = c’ + ab c’= the direct effect of X on Y after controlling for M; c’=c-ab ab= indirect effect of X on Y
The above shows the standard mediation model. Perfect mediation occurs when the effect of X on Y decreases to 0 with M in the model. Partial mediation occurs when the effect of X on Y decreases by a nontrivial amount (the actual amount is up for debate) with M in the model.
2.1 Example Mediation Data
Set an appropriate working directory and generate the following data set.
In this example we’ll say we are interested in whether the number of hours since dawn (X) affect the subjective ratings of wakefulness (Y) 100 graduate students through the consumption of coffee (M).
Note that we are intentionally creating a mediation effect here (because statistics is always more fun if we have something to find) and we do so below by creating M so that it is related to X and Y so that it is related to M. This creates the causal chain for our analysis to parse.
2.2 Method 1: Baron & Kenny
This is the original 4-step method used to describe a mediation effect. Steps 1 and 2 use basic linear regression while steps 3 and 4 use multiple regression. For help with regression, see Chapter 10.
The Steps: 1. Estimate the relationship between X on Y (hours since dawn on degree of wakefulness) -Path “c” must be significantly different from 0; must have a total effect between the IV & DV
Estimate the relationship between X on M (hours since dawn on coffee consumption) -Path “a” must be significantly different from 0; IV and mediator must be related.
Estimate the relationship between M on Y controlling for X (coffee consumption on wakefulness, controlling for hours since dawn) -Path “b” must be significantly different from 0; mediator and DV must be related. -The effect of X on Y decreases with the inclusion of M in the model
Estimate the relationship between Y on X controlling for M (wakefulness on hours since dawn, controlling for coffee consumption) -Should be non-significant and nearly 0.
2.3 Interpreting Barron & Kenny Results
Here we find that our total effect model shows a significant positive relationship between hours since dawn (X) and wakefulness (Y). Our Path A model shows that hours since down (X) is also positively related to coffee consumption (M). Our Path B model then shows that coffee consumption (M) positively predicts wakefulness (Y) when controlling for hours since dawn (X). Finally, wakefulness (Y) does not predict hours since dawn (X) when controlling for coffee consumption (M).
Since the relationship between hours since dawn and wakefulness is no longer significant when controlling for coffee consumption, this suggests that coffee consumption does in fact mediate this relationship. However, this method alone does not allow for a formal test of the indirect effect so we don’t know if the change in this relationship is truly meaningful.
There are two primary methods for formally testing the significance of the indirect test: the Sobel test & bootstrapping (covered under the mediatation method).
The Sobel Test uses a specialized t-test to determine if there is a significant reduction in the effect of X on Y when M is present. Using the sobel function of the multilevel package will show provide you with three of the basic models we ran before (Mod1 = Total Effect; Mod2 = Path B; and Mod3 = Path A) as well as an estimate of the indirect effect, the standard error of that effect, and the z-value for that effect. You can either use this value to calculate your p-value or run the mediation.test function from the bda package to receive a p-value for this estimate.
In this case, we can now confirm that the relationship between hours since dawn and feelings of wakefulness are significantly mediated by the consumption of coffee (z’ = 3.84, p < .001).
However, the Sobel Test is largely considered an outdated method since it assumes that the indirect effect (ab) is normally distributed and tends to only have adequate power with large sample sizes. Thus, again, it is highly recommended to use the mediation bootstrapping method instead.
2.4 Method 2: The Mediation Pacakge Method
This package uses the more recent bootstrapping method of Preacher & Hayes (2004) to address the power limitations of the Sobel Test. This method computes the point estimate of the indirect effect (ab) over a large number of random sample (typically 1000) so it does not assume that the data are normally distributed and is especially more suitable for small sample sizes than the Barron & Kenny method.
To run the mediate function, we will again need a model of our IV (hours since dawn), predicting our mediator (coffee consumption) like our Path A model above. We will also need a model of the direct effect of our IV (hours since dawn) on our DV (wakefulness), when controlling for our mediator (coffee consumption). When can then use mediate to repeatedly simulate a comparsion between these models and to test the signifcance of the indirect effect of coffee consumption.

2.5 Interpreting Mediation Results
The mediate function gives us our Average Causal Mediation Effects (ACME), our Average Direct Effects (ADE), our combined indirect and direct effects (Total Effect), and the ratio of these estimates (Prop. Mediated). The ACME here is the indirect effect of M (total effect - direct effect) and thus this value tells us if our mediation effect is significant.
In this case, our fitMed model again shows a signifcant affect of coffee consumption on the relationship between hours since dawn and feelings of wakefulness, (ACME = .28, p < .001) with no direct effect of hours since dawn (ADE = -0.11, p = .27) and significant total effect ( p < .05).
We can then bootstrap this comparison to verify this result in fitMedBoot and again find a significant mediation effect (ACME = .28, p < .001) and no direct effect of hours since dawn (ADE = -0.11, p = .27). However, with increased power, this analysis no longer shows a significant total effect ( p = .08).
3 Moderation Analyses
Moderation tests whether a variable (Z) affects the direction and/or strength of the relation between an IV (X) and a DV (Y). In other words, moderation tests for interactions that affect WHEN relationships between variables occur. Moderators are conceptually different from mediators (when versus how/why) but some variables may be a moderator or a mediator depending on your question. See the mediation package documentation for ways of testing more complicated mediated moderation/moderated mediation relationships.
Like mediation, moderation assumes that there is little to no measurement error in the moderator variable and that the DV did not CAUSE the moderator. If moderator error is likely to be high, researchers should collect multiple indicators of the construct and use SEM to estimate latent variables. The safest ways to make sure your moderator is not caused by your DV are to experimentally manipulate the variable or collect the measurement of your moderator before you introduce your IV.

Basic Moderation Model.
3.1 Example Moderation Data
In this example we’ll say we are interested in whether the relationship between the number of hours of sleep (X) a graduate student receives and the attention that they pay to this tutorial (Y) is influenced by their consumption of coffee (Z). Here we create the moderation effect by making our DV (Y) the product of levels of the IV (X) and our moderator (Z).
3.2 Moderation Analysis
Moderation can be tested by looking for significant interactions between the moderating variable (Z) and the IV (X). Notably, it is important to mean center both your moderator and your IV to reduce multicolinearity and make interpretation easier. Centering can be done using the scale function, which subtracts the mean of a variable from each value in that variable. For more information on the use of centering, see ?scale and any number of statistical textbooks that cover regression (we recommend Cohen, 2008).
A number of packages in R can also be used to conduct and plot moderation analyses, including the moderate.lm function of the QuantPsyc package and the pequod package. However, it is simple to do this “by hand” using traditional multiple regression, as shown here, and the underlying analysis (interacting the moderator and the IV) in these packages is identical to this approach. The rockchalk package used here is one of many graphing and plotting packages available in R and was chosen because it was especially designed for use with regression analyses (unlike the more general graphing options described in Chapters 8 & 9).

3.3 Interpreting Moderation Results
Results are presented similar to regular multiple regression results (see Chapter 10). Since we have significant interactions in this model, there is no need to interpret the separate main effects of either our IV or our moderator.
Our by hand model shows a significant interaction between hours slept and coffee consumption on attention paid to this tutorial (b = .23, SE = .04, p < .001). However, we’ll need to unpack this interaction visually to get a better idea of what this means.
The rockchalk function will automatically plot the simple slopes (1 SD above and 1 SD below the mean) of the moderating effect. This figure shows that those who drank less coffee (the black line) paid more attention with the more sleep that they got last night but paid less attention overall that average (the red line). Those who drank more coffee (the green line) paid more when they slept more as well and paid more attention than average. The difference in the slopes for those who drank more or less coffee shows that coffee consumption moderates the relationship between hours of sleep and attention paid.
4 References and Further Reading
Baron, R., & Kenny, D. (1986). The moderator-mediator variable distinction in social psychological research: Conceptual, strategic, and statistical considerations. Journal of Personality and Social Psychology, 51, 1173-1182.
Cohen, B. H. (2008). Explaining psychological statistics. John Wiley & Sons.
Imai, K., Keele, L., & Tingley, D. (2010). A general approach to causal mediation analysis. Psychological methods, 15(4), 309.
MacKinnon, D. P., Lockwood, C. M., Hoffman, J. M., West, S. G., & Sheets, V. (2002). A comparison of methods to test mediation and other intervening variable effects. Psychological methods, 7(1), 83.
Nie, Y., Lau, S., & Liau, A. K. (2011). Role of academic self-efficacy in moderating the relation between task importance and test anxiety. Learning and Individual Differences, 21(6), 736-741.
Tingley, D., Yamamoto, T., Hirose, K., Keele, L., & Imai, K. (2014). Mediation: R package for causal mediation analysis.
ORIGINAL RESEARCH article
The relationship between attachment and depression among college students: the mediating role of perfectionism.
- 1 School of Humanities and Social Sciences, Anhui Agricultural University, Hefei, China
- 2 College of Arts, Humanities and Social Sciences, Edinburgh College of Arts, University of Edinburgh, Edinburgh, United Kingdom
Objectives: Explore the relationship between adult attachment and depression among college students, and the mechanism of perfectionism.
Study design: Questionnaire survey.
Methods: A questionnaire survey was conducted nationwide, totally 313 valid questionnaires were received. The survey collected information such as adult attachment (Adult Attachment Scale, AAS), depression (The Center for Epidemiologic Studies Depression Scale, CESD), and perfectionism (The Frost Multidimensional Perfectionism Scale, FMPS). Then used SPSS26.0 to analyze the collected data.
Results: There were significant differences in perfectionism and depression between secure attachment and insecure attachment in college students. Perfectionism plays a partial mediating role in the relationship between the close-depend composite dimension, anxiety dimension of attachment and depression.
Conclusion: The closeness-dependence dimension of attachment significantly and negatively predicted perfectionism and depression; the anxiety dimension of attachment significantly and positively predicted perfectionism and depression; and perfectionism partially mediated the effect of both the closeness-dependence dimension of attachment on depression and the anxiety dimension of attachment on depression. Attachment can directly affect college students’ depression, but also indirectly through perfectionism.
Introduction
With the popularization of psychological knowledge and attention to psychological issues from all sectors of society, more and more people see the importance of mental health. Especially to young people who are at a critical stage of their development. The transition from academic to social life at university means that university students face a variety of challenges from the outside world as well as from themselves, such as establishing new interpersonal relationships, solving problems in life alone, and learning more specialized knowledge, making them more prone to psychological problems than other people. This is especially true in the context of the epidemic, which has put university students under even more pressure, not least because of depression, a psychological disorder that has become a common sight in recent years. The study of the mechanisms that lead to depression among university students is of great relevance to address and prevent depression among university students.
Attachment characteristics will also be the subject of this thesis as a necessary factor influencing interpersonal interaction patterns, intimate relationship development and other life issues that important university students need to address. Attachment is the initial connection between children and the world, and through various stages of growth and development, the attachment characteristics of adults can differ from those of children. Individuals with a tendency toward perfectionism are chronically unhappy and therefore also depressed. This study will therefore examine the correlated and internal mechanisms of attachment characteristics, perfectionism, and depression in university students.
Literature review
Depression is an affective disorder, a psychological condition characterized by low mood, hopelessness, and helplessness ( Su et al., 2011 ). Some studies have shown that the incidence of depression among university students is much higher than that of people in other stages ( Ibrahim et al., 2013 ). College students from rural areas who come to the city for study have a higher risk of depression, which may be related to the inadaptability to the learning or living environment and excessive mental or economic pressure ( He et al., 2018 ). In recent years, some scholars have also compared the detection rate of depression among university students in Guangdong before and after the epidemic, and the data showed that the detection rate of major depression was higher in 2020 than in 2022, with little difference in the detection rate of moderate depression and a higher detection rate of mild depression in 2022 ( Li et al., 2022 ).
People who are depressed often feel sad, depressed, lack interest in activities or things they used to enjoy, fail to experience pleasure in life, have reduced self-confidence, blame themselves, often feel fatigued, and are mentally inactive ( Su et al., 2011 ). Maladjustment can lead to depressive symptoms, depressive episodes, and even life-threatening behaviors such as suicide and homicide ( Li, 2018 ). Depression can also seriously affect the normal life of studying and working of university students. At present, depression is an important global public health problem, and its prevention and control are of great significance ( Wang et al., 2020 ). Therefore, we should pay attention to the study of depression among university students and explore its mechanisms in depth to obtain more effective coping strategies.
Adult attachment refers to an adult’s recollection and reproduction of their early childhood attachment experiences and current evaluation of childhood attachment experiences ( Shi and Wei, 2007 ). Attachment theory suggests that attachment enables individuals to form strong and secure affectional ties with significant others and is one of the main sources of security and a sense of control ( Liu et al., 2022 ). In contrast to infants and children who have a single object of attachment, adults have a much wider range of attachments, including parents, relatives, friends, and lovers. Attachment is a relatively stable structure that manipulates external consciousness, guides relationships with parents, and influences strategies and behaviors in subsequent relationships ( Wang et al., 2005 ). Also, different attachment characteristics and types of attachment are reflected in the individual’s unique interpersonal and intimate relationship management. Establishing and maintaining secure and satisfying social relationships is a basic human need, but the deprivation of this need affects interpersonal relationships in adulthood and may create insecure adult attachment patterns ( Mikulincer and Shaver, 2019 ).
The current available research suggests that people with insecure attachment types are at higher risk of chronic major depression ( Li et al., 2019 ), where the types of insecure attachment are preoccupied attachment and fearful attachment. Conradi and Jonge (2009) have shown that insecure attachment has a very important impact on the generation, development, and maintenance of depressed mood. A study found that individuals with insecure attachment were 3.9 times more likely to suffer from depression than individuals with secure attachment ( Shi et al., 2013 ). Studies of university students have also found that depressive status scores are significantly and positively correlated with attachment avoidance and attachment anxiety scores ( Ma et al., 2023 ).
Perfectionism has been conceptualized as a personality variable that underlies a variety of psychological difficulties. Recently, however, theorists and researchers have begun to distinguish between two distinct types of perfectionism, one a maladaptive form that results in emotional distress, and a second form that is relatively benign, perhaps even adaptive ( Bieling et al., 2004 ). Some studies have found that the development of depression are associated with negative perfectionists’ tendency to focus excessively on mistakes, self-doubt, and expectation criticism ( Zhang et al., 2013 ). It has been suggested that insecure attachment and negative perfectionism are risk factors for the formation of an obsessive-compulsive personality ( Xu et al., 2021 ).
Rice and Mirzadeh (2000) examined the relationship between attachment, perfectionism and depression and showed that subjects with insecure attachment were more likely to be maladaptive perfectionists and to experience more significant depression than those with secure attachment. Research on perfectionism and attachment has also found a significant positive correlation between attachment anxiety dimensions and negative perfectionism in adults ( Ma et al., 2010 ). A study explored the mediating role of perfectionism between attachment and depression, and found that attachment anxiety and attachment avoidance both promote the formation of negative dimensions of perfectionism, which in turn leads to depression ( Xin-Chun et al., 2013 ). A study on the relationship between attachment styles and positive/negative perfectionism, anger, and emotional regulation among college students showed that unsafe attachment styles (avoidance and conflict) can predict negative perfectionism, safe attachment types (avoidance and conflict) can predict emotional regulation, and attachment patterns can determine many behaviors, emotions, and adult relationships, which are necessary for personality growth and mental health foundations ( Nader et al., 2017 ).
Purpose and hypothesis of the study
The purpose of this study is to explore the mechanism of the relationship between attachment characteristics, depression, and perfectionism among college students.
There are three hypotheses in this study: (1) Attachment characteristics significantly predict depression and perfectionism, (2) Perfectionism significantly predicts depression, and (3) Perfectionism mediates the relationship between attachment characteristics and depression in college students.
Materials and methods
Participants.
This study used the university student population in different regions as the subjects and used the online distribution method to collect a total of 313 valid questionnaires. There were 130 male students (41.5%) and 183 female students (58.5%); 104 only children (33.2%) and 209 non-only children (66.8%); 32 first year university students; 52 s year students, 54 third year students, 140 fourth year students and 35 postgraduate students.
Adult attachment
The revised Adult Attachment Scale (AAS) ( Wu et al., 2004 ) was used to measure attachment characters. The scale was revised and developed by Wili Wu et al. and divided into three subscales of closeness, dependence, and anxiety, each with 6 questions each, for a total of 18 questions. A 5-point scale was used, in which the closeness and dependence subscales were combined into a composite closeness-dependence dimension, which was juxtaposed with the anxiety dimension, with higher scores indicating a stronger characteristic dimension. Based on the mean scores of the two dimensions there are four further types of attachment: secure, preoccupied, rejective and fearful, the latter three being insecure attachments. In the present study, the Cronbach’s alpha coefficients for the closeness-dependence dimension and the anxiety dimension of the scale were 0.774 and 0.866, respectively.
The Center for Epidemiologic Studies Depression Scale (CES-D) ( Wang et al., 1999 ) was used to measure depression, it contains 20 items on a 4-point scale, scored on a scale of 0, 1, 2 and 3, with the higher the score the more depressed they are. Unlike the SDS, the CES-D is not used to clinically judge depressed patients and is therefore more appropriate for the group of university students in this study, focusing on measuring depressed state of mind. In this study, the Cronbach’s alpha coefficient for the scale was 0.902.
Perfectionism
The Frost Multidimensional Perfectionism Scale (FMPS) ( Zi and Zhou, 2006 ) was used to measure perfectionism, developed and revised by Zi Fei et al., is divided into 27 entries and five dimensions. The dimensions are organization, concern over mistakes, parental expectations, personal standards, and doubts about action. The organization dimension is positive perfectionism, and the remaining four dimensions represent negative perfectionism. The scale is rated on a 5-point scale, with higher scores indicating a higher tendency toward perfectionism. In this study the Cronbach’s alpha coefficient for the total scale was 0.916 and the Cronbach’s alpha coefficients for each subscale were 0.834, 0.901, 0.842, 0.824 and 0.730, respectively.
Data analysis
The raw data from the returned questionnaires were processed using SPSS 26.0 software and firstly, the Harman single factor test was used to test for common method bias. The results of the test showed that the variance explained by the first common factor was 22.206%, which was below the threshold of 40%, and therefore there was no serious common method bias in this study. The mediating role of perfectionism in the relationship between attachment characteristics and depression was also tested using Model 4 in the SPSS plug-in Process v3.5.
Analysis of the characteristics of attachment, depression and perfectionism among university students
The AAS scores were transformed by combining the closeness-dependence subscale and the dependence subscale into a composite closeness-dependence dimension and calculating the mean closeness-dependence score; the anxiety subscale was used as a separate anxiety dimension and the mean anxiety score was calculated. The mean scores of the two dimensions were compared and categorized. Those with mean scores of closeness-dependence greater than 3 and mean scores of anxiety less than 3 were classified as secure attachment, while the rest were classified as insecure attachment. Insecure attachment was further classified into three different types of attachment: those with a mean score of closeness-dependence greater than 3 and a mean score of anxiety greater than 3 were preoccupied attachment, those with a mean score of closeness-dependence less than 3 and a mean score of anxiety less than 3 were rejective attachment, and those with a mean score of closeness-dependence less than 3 and a mean score of anxiety greater than 3 were fearful attachment. In this study, only 154 (49.2%) were secure attachment, while 54 (17.3%) were preoccupied attachment, 40 (12.8%) were rejective attachment and 65 (20.8%) were fearful attachment.
Of all subjects, 141 (45.05%) experienced some degree of depression.
In the current study, college students’ attachment characteristics, depression and perfectionism did not differ significantly ( p > 0.05) in the demographic variables of gender, whether they were an only child, and grade level.
The AAS scale scores were transformed to classify the subjects into secure attachment and insecure attachment, 154 in total for the former and 159 in total for the latter, and independent samples t -tests were conducted. The results yielded significant differences ( p < 0.001) between secure attachment subjects and insecure attachment subjects on both depression and perfectionism. As shown in Table 1 , subjects with insecure attachment scored significantly higher on depression and perfectionism than those with secure attachment.
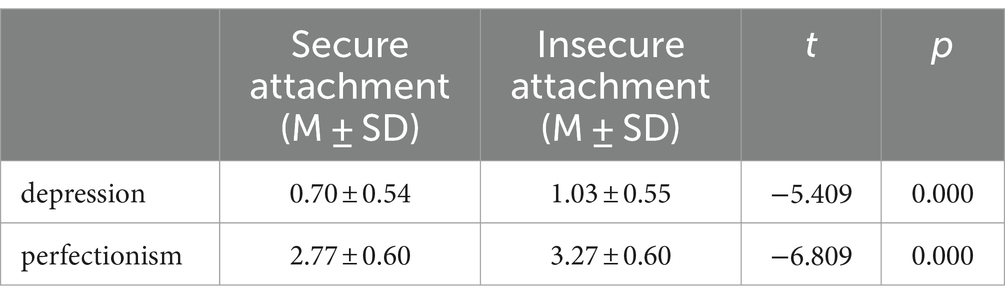
Table 1 . Analysis of differences in depression and perfectionism among college students with different attachment types ( N = 313).
Correlation analysis of attachment characteristics, depression, and perfectionism among university students
Pearson correlation analysis was conducted using SPSS 26.0 on the three variables of attachment characteristics, depression and perfectionism, and the results indicated that all three variables were correlated, which was consistent with the premise of the mediating effect test. The results of the specific correlation analysis are shown in Table 2 .
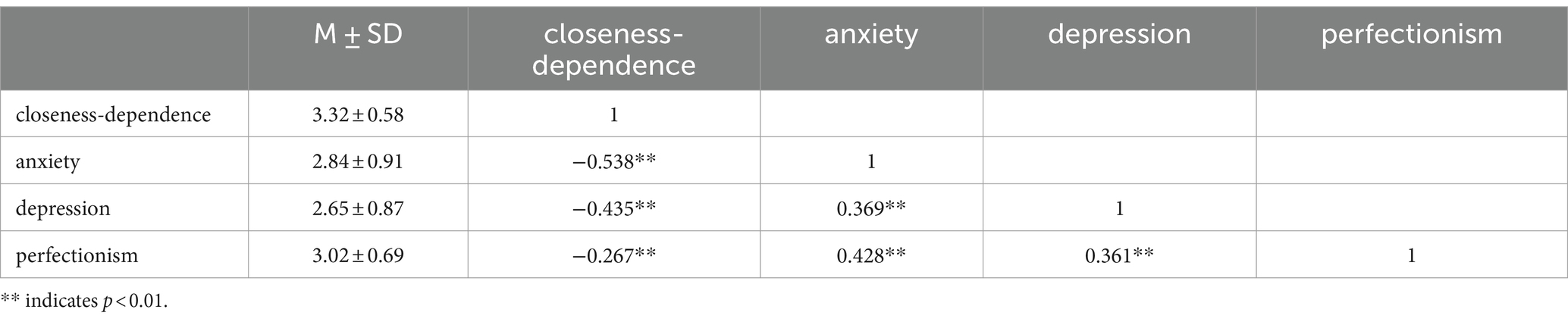
Table 2 . Correlation analysis of attachment characteristics, depression, and perfectionism among university students ( N = 313).
The closeness-dependence dimension of attachment was negatively associated with depression and perfectionism, while the anxiety dimension of attachment was positively associated with depression and perfectionism. This means that attachment closeness-dependence scores negatively predict depression and perfectionism, while attachment anxiety dimensions positively predict depression and perfectionism scores. Perfectionism also positively predicted depression.
A test of the mediating role of perfectionism between attachment characteristics and depression among university students
Model 4 in the SPSS plug-in Process prepared by Hayes ( Hayes, 2018 ) was used to test for the mediating effect of perfectionism and the results are shown in Table 3 . Before perfectionism was placed as a mediating variable, the closeness-dependence dimension of attachment was a significant negative predictor of depression ( β = −0.42, t = −8.25, p < 0.001), the anxiety dimension of attachment was a significant positive predictor of depression ( β = 0.37, t = 6.99, p < 0.001). The closeness- dependence dimension of attachment was a significant negative predictor of perfectionism ( β = −0.25, t = −4.88, p < 0.001) and the anxiety dimension of attachment was a significant positive predictor of perfectionism ( β = 0.43, t = 8.35, p < 0.001). When perfectionism was added as a mediating variable, the closeness-dependence dimension of attachment was still a significant negative predictor of depression ( β = −0.36, t = −6.98, p < 0.001) The perfectionism dimension of attachment was also a significant positive predictor of depression ( β = 0.27, t = 5.32, p < 0.001); after adding perfectionism as a mediating variable, the anxiety dimension of attachment was still a significant positive predictor of depression ( β = 0.26, t = 4.63, p < 0.001), and perfectionism was also a significant positive predictor of depression ( β = 0.25, t = 4.38, p < 0.001). The results of the analysis of the mediating effect can be represented in Figure 1 .
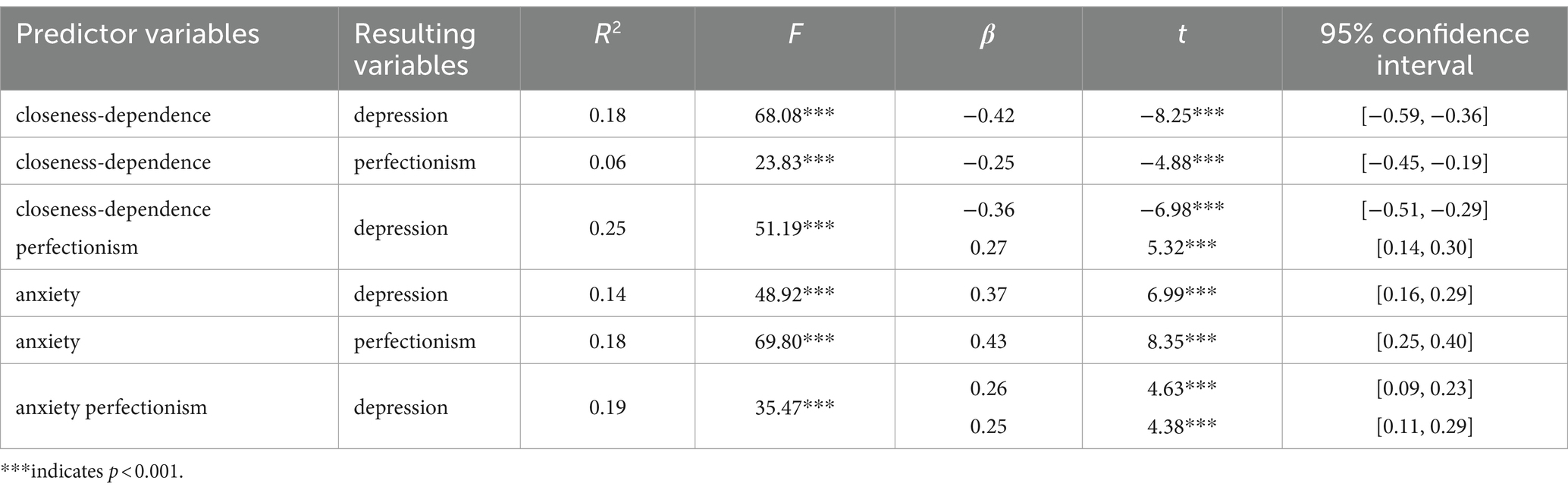
Table 3 . Mediating role of perfectionism between attachment and depression ( N = 313).
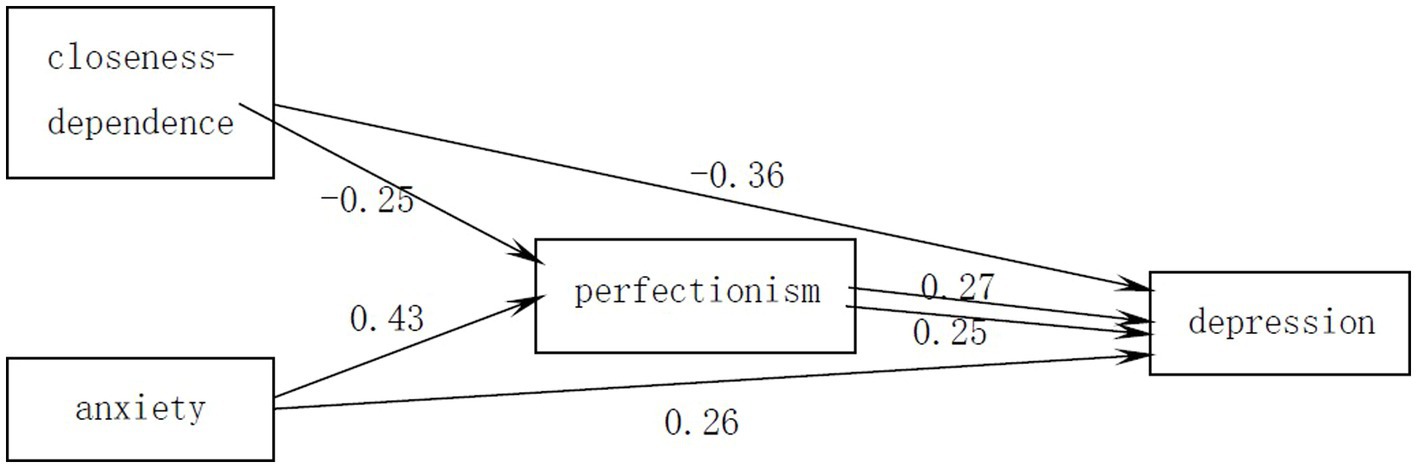
Figure 1 . Graph showing the results of the test for the mediating role of perfectionism.
The mediating effects of the attachment closeness-dependence dimension and the anxiety dimension with their respective effect sizes are shown in Table 4 .
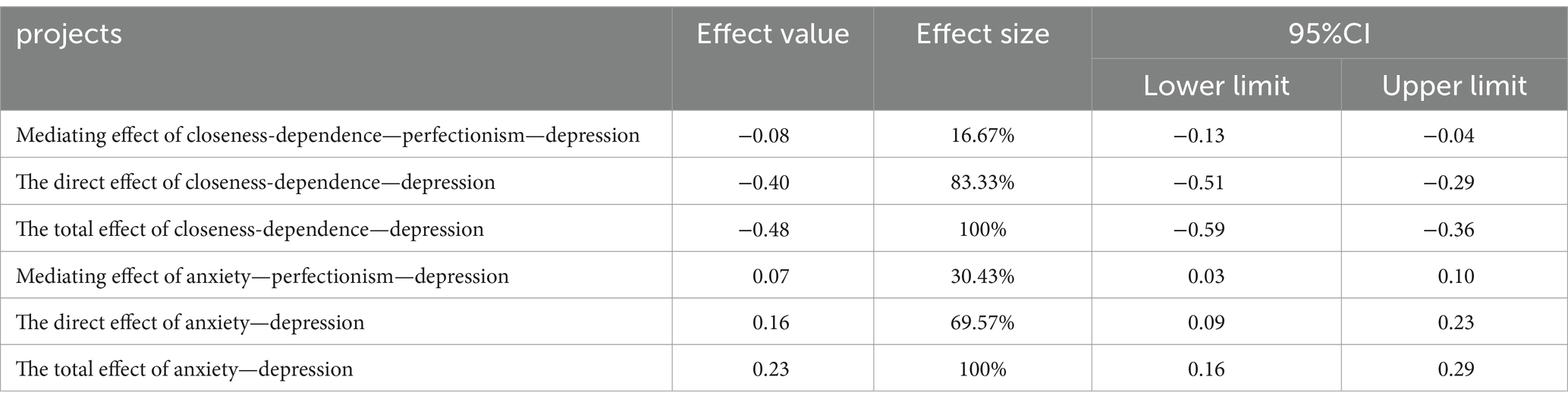
Table 4 . Attachment mediated effects and effect sizes.
The Bootstrap 95% confidence interval for the direct effect of the closeness-dependence dimension of attachment on depression was [−0.51, −0.29], excluding 0, indicating that its direct effect was significant; the Bootstrap 95% confidence interval for the mediating effect of perfectionism between the closeness-dependence dimension of attachment and depression was [−0.13, −0.04], excluding 0, indicating that the mediating effect of perfectionism was significant. The Bootstrap 95% confidence interval for the total effect of attachment closeness -dependence dimension on depression was [−0.59, −0.36], excluding 0, indicating a significant total effect and an effect value of −0.48. Thus, it can be suggested that perfectionism partially mediates the relationship between attachment closeness-dependence dimension and depression, with a mediating effect of 16.67%.
The Bootstrap 95% confidence interval for the direct effect of the anxiety dimension of attachment on depression was [0.09, 0.23], which did not contain 0, indicating a significant direct effect; the Bootstrap 95% confidence interval for the mediating effect of perfectionism between the anxiety dimension of attachment and depression was [0.03, 0.10], which did not contain 0, indicating a significant mediating effect of perfectionism between the anxiety dimension of attachment and depression. The Bootstrap 95% confidence interval for the total effect of attachment anxiety dimension on depression was [0.16, 0.29], excluding 0, indicating a significant total effect with an effect value of 0.23. It can therefore be shown that perfectionism partially mediates between attachment anxiety dimension and depression, with a mediating effect of 30.43%.
Status of attachment characteristics, perfectionism, and depression among university students
In this study, a total of 45.05% of the university students who participated in the questionnaire experienced some degree of depression, and there were no significant differences in the demographic variables of depression scores in gender, whether they were an only child, or grade level. This also suggests, to some extent, that depression is somewhat prevalent in the university population. In addition, subjects with insecure attachment types were more likely to experience depressive mood than those with secure attachment types. This is in line with the findings of some scholars on adolescent depression and parent–child attachment: secure parent–child attachment reduces the risk of adolescent depression ( Lai et al., 2022 ). The need for excessive attention and commitment is not always met, leading to severe feelings of loss, further doubts, and anxiety about the safety of the relationship ( Shaver et al., 2005 ). College students with insecure attachment types experience more abuse, neglect, separation, and loss in their early years of interaction with their primary caregivers, and such early adverse experiences are often associated with depression ( Whiffen et al., 1999 ). It also points to the important role that attachment plays in the development of adolescents and even university students.
The mechanism by which adult attachment affects depression lies in the internal negative self-model, i.e., the negative evaluation of the self is an important factor in forming depression ( Yang, 2005 ). Individuals with high scores on the anxiety dimension of attachment often fear that the attachment partner will leave them, and one of the reasons for this is a lack of self-confidence and doubt. Because of their negative perceptions of themselves, these groups do not see themselves as worthy of love and therefore feel insecure that they may be abandoned at any time, even if they have established an intimate relationship. Insecure attachment individuals are more likely to have problems in making emotional connections with external objects than secure attachment individuals, e.g., individuals with preoccupied attachment (high closeness-dependence, high anxiety) have a stronger desire to connect with others and a high level of insecurity about existing connections, so they often seek approval from others, are completely focused on others, are dependent on others, easily lose themselves and are in constant fear of relationship breakdown ( Zhang, 2021 ).
In this study, perfectionism was also scored significantly higher in insecure attachment individuals than in secure attachment individuals. Some scholars believe that doing things perfectly and not making mistakes is a means of survival for children who grow up in harsh, punishing, harsh or even abusive families to avoid unnecessary harm or enhance their control over uncertain environments ( Flett et al., 2012 ). Maladaptive perfectionism has overly idealistic expectations and ideas about real life, thinking that they can easily achieve success or achieve goals, often ignoring objective conditions, and often feeling lost and inadequate in the process ( Rice and Ashby, 2007 ). One of the characteristics of perfectionism is that they always set much higher goals for themselves and too difficult to achieve them, so perfectionistic individuals are chronically unfulfilled and dissatisfied with themselves. The insecure attachment individuals’ negative evaluation of self also contributes to the pursuit of high goals. At the same time, prolonged failure to achieve goals can lead to new negative evaluations of the self, which are likely to be accompanied by devaluation of the self, forming a pattern of internal evaluations that can lead to depression. Researcher found that perfectionists’ rigid core thinking produces low levels of unconditional self-acceptance, accompanied by low self-liking and a low sense of self-competence, which in turn leads to depression ( Scott, 2007 ). The Beck depression model suggests that negative notions of self and others increase an individual’s susceptibility to depression ( Kernis et al., 1991 ). It has been shown that maladaptive perfectionist individuals act with excessive consideration for the correctness of their behavior, hesitate to act and fear failure, and are more likely to adopt negative coping styles in stressful situations, leading to anxiety, depression, and other negative emotions ( Zhang et al., 2014 ).
The mediating role of perfectionism between attachment and depression
The results of the analysis of the mediating role of perfectionism found that the direct effects of the closeness-dependence dimension and the anxiety dimension on depression were significantly weakened after perfectionism was introduced between attachment characteristics and depression as the mediated variable, indicating that perfectionism partially mediates the relationship between both the closeness- dependence composite dimension of adult attachment and the anxiety dimension and depression, meaning that university students’ attachment can directly affect their depression levels, as well as indirectly affect depression levels by influencing perfectionist tendencies.
The closeness-dependence dimension of attachment reflects the degree to which individuals are comfortable in intimate relationships and assess whether they have someone to rely on when needed ( Zhang et al., 2014 ). Individuals with higher levels of comfort in intimate relationships are more likely to feel satisfied and therefore less likely to have negative evaluations of themselves. As shown in the study, scores on the closeness-dependence dimension of attachment were significantly and negatively correlated with scores on perfectionism and depression. It has been found that individuals with higher levels of attachment anxiety have lower levels of emotion expression ( Yao and Zhao, 2021 ), and that the prolonged lack of emotional expression can easily lead to depression. Insecure attachment individuals have negative attitudes toward interpersonal interactions and have less social support than other people, making it more difficult for them to get out of difficult situations when they have negative life events or encounter difficulties and setbacks.
The results of this study show that the closeness-dependence dimension of attachment is negatively related to perfectionism, while the anxiety dimension of attachment is positively related to perfectionism, meaning that individuals with low closeness-dependence and high anxiety in intimate relationships, i.e., Those who do not adapt well to intimate relationships or who have not experienced success in previous intimate relationships, are prone to perfectionism. Perfectionists are also overly concerned with their own behavior and performance. This group believes that they must be complimented and praised by others, and they show a desire for attention and a fear of being rejected by others ( Stoeber et al., 2017 ). This is why these people tend to experience disappointment in their daily lives and in their interpersonal interactions, resulting in a decline in self-confidence, a decrease in interest and depression.
Implications of the research
This study investigates the mechanisms by which attachment affects depression in university students and has implications for the prevention and intervention of depression in university students. Firstly, it can draw attention to the importance of building secure attachments and creating a good family environment from early childhood to avoid the risk of depression early. At the same time, for attachment characteristics that are difficult to change early in the formative years, it is possible to intervene in depression by changing the mediating variables in this study, guiding individuals to set reasonable goals, and taking more encouraging measures to improve their self-esteem.
Limitations of the research
This study also has certain limitations, firstly, from the research method, the method of questionnaire collection adopted in this study is online collection method, thus there are limitations in controlling the confounding variables. Moreover, the sample size of this study is still not sufficiently random in terms of grade and major, so there may be some errors. Finally, as perfectionism only partially mediates the results from this study and has a low effect size, there are many potential influences in related areas that need to be further explored.
There were significant differences in perfectionism and depression between secure attachment and insecure attachment in university students, with insecure attachment individuals scoring higher in perfectionism and depression than secure attachment individuals.
The closeness-dependence dimension of attachment significantly and negatively predicted perfectionism and depression; the anxiety dimension of attachment significantly and positively predicted perfectionism and depression; and perfectionism partially mediated the effect of both the closeness-dependence dimension of attachment on depression and the anxiety dimension of attachment on depression.
Data availability statement
The raw data supporting the conclusions of this article will be made available by the authors, without undue reservation.
Ethics statement
The studies involving humans were approved by the Ethics Committee of Anhui Agriculture University. The studies were conducted in accordance with the local legislation and institutional requirements. The participants provided their written informed consent to participate in this study.
Author contributions
FF: Conceptualization, Supervision, Writing – review & editing, Funding acquisition, Formal analysis. JW: Writing – original draft, Data curation, Methodology.
The author(s) declare that no financial support was received for the research, authorship, and/or publication of this article.
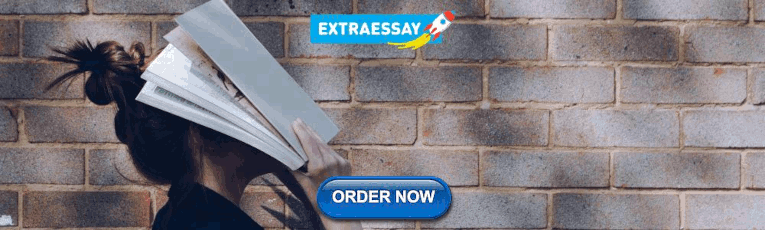
Conflict of interest
The authors declare that the research was conducted in the absence of any commercial or financial relationships that could be construed as a potential conflict of interest.
Publisher’s note
All claims expressed in this article are solely those of the authors and do not necessarily represent those of their affiliated organizations, or those of the publisher, the editors and the reviewers. Any product that may be evaluated in this article, or claim that may be made by its manufacturer, is not guaranteed or endorsed by the publisher.
Bieling, P. J., Israeli, A. L., and Antony, M. M. (2004). Is perfectionism good, bad, or both? Examining models of the perfectionism construct. Pers. Individ. Differ. 36, 1373–1385. doi: 10.1016/S0191-8869(03)00235-6
Crossref Full Text | Google Scholar
Conradi, H. J., and Jonge, P. D. (2009). Recurrent depression and the role of adult attachment: a prospective and a retrospective study. J. Affect. Disord. 116, 93–99. doi: 10.1016/j.jad.2008.10.027
PubMed Abstract | Crossref Full Text | Google Scholar
Flett, G. L., Druckman, T., Hewitt, P. L., and Wekerle, C. (2012). Perfectionism, coping, social support, and depression in maltreated adolescents. J. Ration. Emot. Cogn. Behav. Ther. 30, 118–131. doi: 10.1007/s10942-011-0132-6
Hayes, A. F. (2018). Introduction to mediation, moderation, and conditional process analysis: A regression-based approach . New York: Guilford Press
Google Scholar
He, H., Zeng, Q. L., and Wang, H. S. Q. (2018). Analysis of the influence of left-behind experience on the depression status of rural college students with household registration. Chin. J. Health Educ. 34, 973–978.
Ibrahim, A., Kelly, S., Adams, C., and Glazebrook, C. (2013). A systematic review of studies of depression prevalence in university students. J. Psychiatr. Res. 47, 391–400. doi: 10.1016/j.jpsychires.2012.11.015
Kernis, M., Grannemann, B., and Mathis, L. (1991). Stability of self-esteem as a moderator of the relation between level of self-esteem and depression. J. Pers. Soc. Psychol. 61, 80–84. doi: 10.1037/0022-3514.61.1.80
Lai, Z. Z., Wu, C. Y., Wang, C. C., et al. (2022). The effect of paternity attachment on adolescent depression: a moderated mediation model. Chinese psychological association . Xin Xiang: Henan Normal University Press.
Li, A. L.. (2018). The relationship among undergraduate students’ hardy personality, thinking style, and depression . China West Normal University, Nanchong.
Li, Z., Huang, Z. W., Yang, F. C., et al. (2019). A review of mental health theory: from the perspective of adult attachment. J. Chin. Soc. Med. 36, 146–149.
Li, Y. P., Zhang, G. Y., and Chen, R. J. (2022). Investigation and countermeasures on the depression of college students after the outbreak of COVID-19. J. Psychol.Month. 17, 207–216. doi: 10.19738/j.cnki.psy.2022.23.066
Liu, Y., Liu, Z. H., Duan, H., Chen, G., Liu, W., et al. (2022). The influence of insecure attachment on academic procrastination: the mediating role of perfectionism and rumination. Psychology 10, 407–414. doi: 10.16842/j.cnki.issn2095-5588.2022.07.003
Ma, H. X., Gao, Z. H., Li, J. M., et al. (2010). Relationship between perfectionism and adult attachment of university students. J. Chin. Health Psychol. 18, 717–719. doi: 10.13342/j.cnki.cjhp.2010.06.027
Ma, Q., Qin, L., and Cao, W. (2023). Relationship between depression, attachment and social support of college students. J. Chin. Health Psychol. 31, 294–298. doi: 10.13342/j.cnki.cjhp.2023.02.023
Mikulincer, M., and Shaver, P. R. (2019). Attachment orientations and emotion regulation. Curr. Opin. Psychol. 25, 6–10. doi: 10.1016/j.copsyc.2018.02.006
Nader, H., Hadeis, H., and Usha, B. (2017). The relationship between attachment styles and positive/negative perfectionism, anger, and emotional adjustment among students. Knowl. Res. Appl. Psychol. 17, 11–20.
Rice, K. G., and Ashby, J. S. (2007). An efficient method for classifying perfectionists. J. Couns. Psychol. 54, 72–85. doi: 10.1037/0022-0167.54.1.72
Rice, K. G., and Mirzadeh, S. A. (2000). Perfectionism, attachment, and adjustment. J. Couns. Psychol. 47, 238–250. doi: 10.1037/0022-0167.47.2.238
Scott, J. (2007). The effect of perfectionism and unconditional self-acceptance on depression. J. Ration. Emot. Cogn. Behav. Ther. 25, 35–64. doi: 10.1007/s10942-006-0032-3
Shaver, P. R., Schachner, D. A., and Mikulincer, M. (2005). Attachment style, excessive reassurance seeking, relationship processes, and depression. Personal. Soc. Psychol. Bull. 31, 343–359. doi: 10.1177/0146167204271709
Shi, Y. H., and Wei, P. (2007). A study on the relationship between repression and adult attachment of college students. J. Chin. Health Psychol. 12, 1066–1067. doi: 10.13342/j.cnki.cjhp.2007.12.034
Shi, Z. M., Yang, Q., Xue, Y., et al. (2013). A study of adult attachment types in patients with depression. J. Chin. Clin. Psychol. 21, 616–619.
Stoeber, J., Noland, A. B., Mawenu, T. W. N., Henderson, T. M., and Kent, D. N. P. (2017). Perfectionism, social disconnection, and interpersonal hostility: not all perfectionists don't play nicely with others. Personal. Individ. Differ. 119, 112–117. doi: 10.1016/j.paid.2017.07.008
Su, Z. X., Kang, Y., and Li, J. M. (2011). Study on adolescent depression and the relevant risk factors. J. Health Psychol. 19, 629–631. doi: 10.13342/j.cnki.cjhp.2011.05.024
Wang, M. Y., Liu, J., Wu, X., et al. (2020). A meta-analysis of the prevalence of depression in Chinese college students in recent 10 years. J. Hainan Med. Univ. 26, 686–699.
Wang, Z. Y., Liu, Y. Z., and Yang, Y. (2005). An overview and discussion of the research on the internal working mode of attachment. Adv. Psychol. Sci. 5, 629–639.
Wang, X. D., Wang, X. L., and Ma, H.. (1999). Handbook of China mental health assessment scale . Beijing: China Mental Health Journal Press. 200–202.
Whiffen, V. E., Judd, M. E., and Aube, J. A. (1999). Intimate relationships moderate the association between childhood sexual abuse and depression. J. Interpers. Violence 14, 940–954. doi: 10.1177/088626099014009002
Wu, W. L., Zhang, W., and Liu, X. H. (2004). The reliability and validity of adult attachment scale (AAS-1996 revised edition): a report on its application in China. Sichuan Da Xue Xue Bao Yi Xue Ban 35, 536–538.
Xin-Chun, L., Xiao-Kun, Z., Jing-Jing, D., and Yong-Ling, L. I. (2013). A study on mediating effect of perfectionism between attachment and depression. Chin. J. Clin. Psychol. 21, 658–661. doi: 10.16128/j.cnki.1005-3611.2013.04.005
Xu, W., Liu, Q., Chu, J., et al. (2021). The effect of insecure attachment and negative perfectionism on the relationship between family environment and obsessive-compulsive personality. J. Chin. Clin. Psychol. 29, 328–332. doi: 10.16128/j.cnki.1005-3611.2021.02.022
Yang, J. (2005). Explorative Researeh on adult attachment and its influence upon depression . East China Normal University, Shanghai.
Yao, X. Y., and Zhao, Y. P. (2021). Relationship between adult attachment and mobile phone dependence among college student: the mediating role of emotional expression. J. Psychol. Techn. Appl. 9, 522–529. doi: 10.16842/j.cnki.issn2095-5588.2021.09.002
Zhang, Q. (2021). The influence of types of adult attachment on interpersonal communication. J. Sci. Educ. Lit. 550, 148–150. doi: 10.16871/j.cnki.kjwha.2021.12.046
Zhang, B., Qiu, Z. Y., Jiang, H. B., et al. (2014). Coping styles as a mediator between perfectionism and depression in Chinese college students. J. Nanjing Med. Univ. 14, 319–323. doi: 10.16128/j.cnki.1005-3611.2018.03.039
Zhang, B., Zhou, X. T., and Cai, T. S. (2013). A study on the relationship between perfectionism personality traits and susceptibility to depression. J. Med. Philos. 34, 50–52.
Zi, F., and Zhou, X. (2006). The Chinese frost multidimensional perfectionism scale: an examination of its reliability and validity. J. Chin. Clin. Med. 14, 560–563.
Keywords: collge students, attachment, depression, perfectionism, medating effects
Citation: Fang F and Wang J (2024) The relationship between attachment and depression among college students: the mediating role of perfectionism. Front. Psychol . 15:1352094. doi: 10.3389/fpsyg.2024.1352094
Received: 16 December 2023; Accepted: 02 April 2024; Published: 15 April 2024.
Reviewed by:
Copyright © 2024 Fang and Wang. This is an open-access article distributed under the terms of the Creative Commons Attribution License (CC BY) . The use, distribution or reproduction in other forums is permitted, provided the original author(s) and the copyright owner(s) are credited and that the original publication in this journal is cited, in accordance with accepted academic practice. No use, distribution or reproduction is permitted which does not comply with these terms.
*Correspondence: Jingwen Wang, [email protected]
Disclaimer: All claims expressed in this article are solely those of the authors and do not necessarily represent those of their affiliated organizations, or those of the publisher, the editors and the reviewers. Any product that may be evaluated in this article or claim that may be made by its manufacturer is not guaranteed or endorsed by the publisher.
Can carbon emission trading improve regional eco-efficiency? Based on the environmental innovation perspective
- Research Article
- Published: 16 April 2024
Cite this article
- Xiongying Li 1 ,
- Yifan Yu 1 ,
- Junhua Pan 2 &
- Miraj Ahmed Bhuiyan 1
This paper uses green innovation and environmental pollution as the mediating variables to construct a mediating effect model to investigate whether China’s carbon emission trading policy can improve regional eco-efficiency by reducing regional environmental pollution and stimulating green innovation. This study is based on panel data from 30 provinces and municipalities directly under China’s central government and autonomous regions from 2003 to 2019. The eco-efficiency of these provinces is measured using the super-efficiency DEA model and the difference-in-difference method (DID). The results show that (1) China’s emission trading policy significantly improves regional ecological efficiency and the per capita GDP. The improvement can effectively improve regional ecological efficiency. (2) The mediating effect of green innovation and environmental pollution is significant. That is, China’s carbon emission trading policy further improves regional ecological efficiency by stimulating more green innovation and reducing the synergy brought by environmental pollution. (3) There are differences in the feedback of this impact mechanism between different regions: It shows the characteristics of the western region being more significant than the eastern region. The central region has no significant effect. The research conclusion can provide a policy reference for the subsequent unified promotion of the construction of a carbon emission trading market nationwide and a theoretical basis for helping to achieve the “dual carbon” goal.
This is a preview of subscription content, log in via an institution to check access.
Access this article
Price includes VAT (Russian Federation)
Instant access to the full article PDF.
Rent this article via DeepDyve
Institutional subscriptions
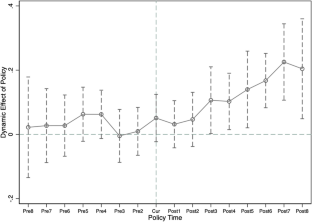
Data Availability
On request.
Ambec S, Barla P (2002) A theoretical foundation of the Porter hypothesis. Econ Lett 75(3):355–360 Elsevier
Article Google Scholar
Arceo A, Rosano M, Biswas WK (2018) Eco-efficiency analysis for remote area power supply selection in Western Australia. Clean Techn Environ Policy 20(3):463–475
Arimura TH, Hibiki A, Johnstone N (2007) An empirical study of environmental R&D: what encourages facilities to be environmentally innovative. In: Environmental policy and corporate behaviour. Edward Elgar Publishing Ltd., pp 142–173
Google Scholar
Baron RM, Kenny DA (1986) The moderator–mediator variable distinction in social psychological research: conceptual, strategic, and statistical considerations. J Pers Soc Psychol 51(6):1173
Article CAS Google Scholar
Borghesi Simone & Cainelli Giulio & Mazzanti Massimiliano, 2012. “European Emission Trading Scheme and environmental innovation: an empirical analysis using CIS data for Italy,” Giornale degli Economisti, GDE (Giornale degli Economisti e Annali di Economia), Bocconi University, vol. 71(1), 71-97.
Calel R, Dechezleprêtre A (2016) Environmental policy and directed technological change: evidence from the European carbon market. Rev Econ Stat 98(1):173–191
Chen F, Ahmad S, Arshad S et al (2022a) Towards achieving eco-efficiency in top 10 polluted countries: the role of green technology and natural resource rents. Gondwana Res 110:114–127
Chen YPV, Zhuo Z, Huang Z et al (2022b) Environmental regulation and ESG of SMEs in China: Porter hypothesis retested. Sci Total Environ 850:157967
Chen Z, Chen J, Song M (2018) The effect of urbanization on environmental pollution in China. Environ Sci Pollut Res 25:35813–35823
Chen Z, Chen J, Song M (2020) The effect of financial development on carbon emission reduction: evidence from China. Energy Econ 86:104664
Clarkson PM, Li Y, Pinnuck M, Richardson GD (2015) The valuation relevance of greenhouse gas emissions under the European Union carbon emissions trading scheme. Eur Account Rev 24(3):551–580
Feng C, Shi B, Kang R (2017) Does environmental policy reduce enterprise innovation?—evidence from China. Sustainability 9(6):872
Fu L, Chen X, Leng Z (2013) Research on eco-efficiency of urban agglomeration based on ultra-efficient DEA model: a case study of Changzhutan “3+5” urban agglomeration. Chinese Mouth Resourc Environ 23(04):169–175
He M, Zhu X, Li H (2023) How does carbon emissions trading scheme affect steel enterprises’ pollution control performance? A quasi natural experiment from China. Sci Total Environ 858:159871
Hu J, Huang N, Shen H (2020a) Can market-incentivized environmental regulation promote technological innovation in enterprises? A natural experiment based on China’s carbon emissions trading mechanism. J Financ Res 01:171–189
Huang S, Du C, Jin X et al (2022) The boundary of Porter hypothesis: the energy and economic impact of China’s carbon neutrality target in 2060. Energies 15(23):9162
Jaffe AB, Newell RG, Stavins RN (2005) A tale of two market failures: technology and environmental policy. Ecol Econ 54(2-3):164–174
Jia Z, Lin B, Wen S (2023) Carbon emissions trading pilot and total factor productivity: also on Porter hypothesis, technology spillover and pollution paradise. Econ Dyn 03:66–86
Jin C, Tsai FS, Gu Q et al (2022) Does the porter hypothesis work well in the emission trading schema pilot? Exploring moderating effects of institutional settings. Res Int Bus Financ 62:101732
Lanjouw JO, Mody A (1996) Innovation and the international diffusion of environmentally responsive technology[J]. Research policy 25(4):549–571
Lanoie P, Laurent-Lucchetti J, Johnstone N, Ambec S (2011) Environmental policy, innovation and performance: new insights on the Porter hypothesis. J Econ Manag Strateg 20:803–842
Lanoie P, Patry M, Lajeunesse R (2008) Environmental regulation and productivity: testing the porter hypothesis. J Prod Anal 30:121–128
Li J, Zhang Y, Wang Z et al (2019) The impact of carbon trading on urban wastewater treatment in China. J Clean Prod 210:145–153
Li K, Lin B (2015) The improvement gap in energy intensity: analysis of China’s thirty provincial regions using the improved DEA (data envelopment analysis) model. Energy 84:589–599
Li K, Lin B (2016) Impact of energy conservation policies on the green productivity in China’s manufacturing sector: evidence from a three-stage DEA model. Appl Energy 168:351–363
Li K, Lin B (2018) The improvement gap in energy intensity: analysis of China’s thirty provincial regions using the improved DEA (data envelopment analysis) model. Energy 84:589–599
Liao W, Dong X, Weng M, Chen X (2020) Economic effects of market-based environmental regulation: carbon emissions trading, green innovation and green economic growth. China Soft Sci 06:159–173
Liu Q, Wang S, Zhang W, Zhan D (2015) Examining the impacts of socioeconomic factors, urban form, and transportation networks on CO 2 emissions in China's megacities. Appl Energy 158:218–228
Liu Q, Wang S, Zhang W, Zhan D (2017b) Examining the impacts of socioeconomic factors, urban form, and transportation networks on CO 2 emissions in China’s megacities. Appl Energy 158:218–228
Liu R, Wang D, Zhang L et al (2019) Can green financial development promote regional ecological efficiency? A case study of China[J]. Natural Hazards 95:325–341
Liu W, Shen J, Wei YD (2023) Spatial restructuring of pollution-intensive enterprises in Foshan China: effects of the changing role of environmental regulation. J Environ Manag 325:116501
Liu Y, Wang L, Long H (2017a) Spatio-temporal analysis of the relationship between urban land expansion and carbon emissions in China. J Clean Prod 142:1074–1083
Lyu Y, Lu Y, Wu S et al (2019) The effect of the belt and road initiative on firms' OFDI: evidence from China's greenfield investment[J]. Economic Research Journal 54(9):187–202
Montgomery WD (1972) Markets in licenses and efficient pollution control programs. J Econ Theory 5(3):395–418
Nie X, Wu J, Wang H et al (2022) Booster or stumbling block? The role of environmental regulation in the coupling path of regional innovation under the Porter hypothesis. Sustainability 14(5):2876
Omrani H, Alizadeh A, Emrouznejad A et al (2022) A robust credibility DEA model with fuzzy perturbation degree: an application to hospitals performance. Expert Syst Appl 189:116021
Pan X, Cheng W, Gao Y et al (2021) Is environmental regulation effective in promoting the quantity and quality of green innovation? Environ Sci Pollut Res 28(5):6232–6241
Passetti E, Tenucci A (2016) Eco-efficiency measurement and the influence of organisational factors: evidence from large Italian companies. J Clean Prod 122:228–239
Popp D (2006) International innovation and diffusion of air pollution control technologies: the effects of NOX and SO2 regulation in the US, Japan, and Germany. J Environ Econ Manag 51(1):46–71
Porter ME, van der Linde C (1995) Toward a new conception of the environment-competitiveness relationship. J Econ Perspect 9(4):97–118
Santikarn M, Churie Kallhauge AN, Bozcaga MO et al (2021) State and trends of carbon pricing 2021[J]
Stavins RN (1995) Transaction costs and tradeable permits. J Environ Econ Manag 29(2):133–148
Tan J, Wang R (2021) Research on evaluation and influencing factors of regional ecological efficiency from the perspective of carbon neutrality. J Environ Manag 294:113030
Temesgen Hordofa T, Minh VH, Maneengam A et al (2023) Does eco-innovation and green investment limit the CO 2 emissions in China? Econ Res-Ekon Istraz 36(1):1–16
Tone K (2001) A slacks-based measure of efficiency in data envelopment analysis. Eur J Oper Res 130(3):498–509
Wang H, Chen W, Wang Q et al (2018) The impact of carbon trading on water resources in China. J Clean Prod 172:2238–2246
Wang J, Zhang Z, Zhang Y et al (2017a) The impact of carbon trading on the emission of chemical oxygen demand in China. J Clean Prod 141:502–508
Wang Y, Li J, Kubota J (2017b) The impact of industrial structure on regional carbon emissions intensity in China. J Clean Prod 164:672–680
Wang W, Wang D, Lu N (2020) Research on the mechanism of carbon emission trading in China to promote low-carbon technology innovation. Chinese Mouth Resourc Environ 30(02):41–48
Wang Y, Li J, Kubota J (2019) The impact of industrial structure on regional carbon emissions intensity in China. J Clean Prod 164:672–680
Wu K, You K, Ren H et al (2022) The impact of industrial agglomeration on ecological efficiency: an empirical analysis based on 244 Chinese cities. Environ Impact Assess Rev 96:106841
Wu R, Lin B (2022) Environmental regulation and its influence on energy-environmental performance: evidence on the Porter hypothesis from China’s iron and steel industry. Resour Conserv Recycl 176:105954
Xie B, Shan ZC (2018) Research on the impact of scientific and technological innovation and environmental regulation on regional ecological efficiency. Ecol Econ 34(04):86–92
Yang B (2009) Research on regional eco-efficiency in China, 2000-2006: an empirical analysis based on DEA method. Econ Geogr 2009(2907):1197–1202
Yang L, Ouyang H, Fang K et al (2015) Evaluation of regional environmental efficiencies in China based on super-efficiency-DEA. Ecol Indic 51:13–19
Yu Y, Zhang W, Zhang N (2018) The potential gains from carbon emissions trading in China’s industrial sectors. Comput Econ 52:1175–1194
Zhang D, Karplus V J, Cassisa C et al (2014) Emissions trading in China: Progress and prospects[J]. Energy policy 75:9–16
Zhang J, Wang Z, Du J (2019) Urbanization, industrial structure and environmental performance: evidence from Chinese cities. J Clean Prod 225:511–521
Zhang J, Wang Z, Du J (2020b) Financial development, environmental regulation and green economic transition: evidence from Chinese cities. J Clean Prod 225:511–521
Zhang J, Zhou C (2016) The impact of foreign direct investment on urban-rural income inequality: evidence from China. Econ Model 53:208–215
Zhang Z, Wang J (2019) The impact of carbon trading on carbon dioxide emissions in China. J Clean Prod 212:1168–1177
Zhang Z, Wang J (2020) Carbon emissions trading in China: features, problems and policy implications. Energy Policy 137:111099
Zhang Z, Wei YM (2016) An overview of current research on EU ETS: evidence from its operating mechanism and economic effect. Appl Energy 174:567–582
Zhang Z, Zhang Y, Wang J et al (2020a) The impact of carbon trading on particulate matter emissions in China. J Clean Prod 256:120435
Zhang ZX (2000) Can China afford to commit itself an emissions cap? An economic and political analysis. Energy Econ 22(6):587–614
Zhang ZX, Baranzini A (2004) What do we know about carbon taxes? An inquiry into their impacts on competitiveness and distribution of income. Energy Policy 32(4):507–518
Zhao X, Ma X, Shang Y et al (2022) Green economic growth and its inherent driving factors in Chinese cities: based on the Metafrontier-global-SBM super-efficiency DEA model. Gondwana Res 106:315–328
Zhou P, Ang BW, Poh KL (2008) A survey of data envelopment analysis in energy and environmental studies. Eur J Oper Res 189(1):1–18
Download references
This paper is funded by the Youth Project of the Guangdong Provincial Research on Philosophy and Social Sciences, “The Mechanism, Effect Measurement and Collaborative Policy Research on Environmental Regulation for High-quality Development in the Guangdong-Hong Kong-Macao Greater Bay Area” (GD21YYJ03).
Author information
Authors and affiliations.
School of Economics, Guangdong University of Finance and Economics, Guangzhou, 510320, China
Xiongying Li, Yifan Yu & Miraj Ahmed Bhuiyan
School of Finance, Guangdong University of Finance and Economics, Guangzhou, 510320, China
You can also search for this author in PubMed Google Scholar
Contributions
Xiongying Li: Editing, validation, supervise. Yifan Yu: Writing-initial draft preparation, writing review, editing. Junhua Pan: Writing-initial draft preparation, modeling, software, data collection. Miraj Ahmed Bhuiyan: Editing, supervise. All authors have equally contributed to the study.
Corresponding author
Correspondence to Junhua Pan .
Ethics declarations
Ethical approval.
No unethical issues were performed.
Consent to participate
Consent for publication, competing interests.
The authors declare no competing interests.
Additional information
Responsible Editor: Roula Inglesi-Lotz
Publisher’s Note
Springer Nature remains neutral with regard to jurisdictional claims in published maps and institutional affiliations.
Rights and permissions
Springer Nature or its licensor (e.g. a society or other partner) holds exclusive rights to this article under a publishing agreement with the author(s) or other rightsholder(s); author self-archiving of the accepted manuscript version of this article is solely governed by the terms of such publishing agreement and applicable law.
Reprints and permissions
About this article
Li, X., Yu, Y., Pan, J. et al. Can carbon emission trading improve regional eco-efficiency? Based on the environmental innovation perspective. Environ Sci Pollut Res (2024). https://doi.org/10.1007/s11356-024-33102-6
Download citation
Received : 30 March 2023
Accepted : 22 March 2024
Published : 16 April 2024
DOI : https://doi.org/10.1007/s11356-024-33102-6
Share this article
Anyone you share the following link with will be able to read this content:
Sorry, a shareable link is not currently available for this article.
Provided by the Springer Nature SharedIt content-sharing initiative
- Carbon emission trading
- Regional eco-efficiency
- Difference-in-differences model
- Environmental innovation mechanisms
- Mediation effect model
- Find a journal
- Publish with us
- Track your research

An official website of the United States government
The .gov means it’s official. Federal government websites often end in .gov or .mil. Before sharing sensitive information, make sure you’re on a federal government site.
The site is secure. The https:// ensures that you are connecting to the official website and that any information you provide is encrypted and transmitted securely.
- Publications
- Account settings
Preview improvements coming to the PMC website in October 2024. Learn More or Try it out now .
- Advanced Search
- Journal List
- HHS Author Manuscripts

Guidelines for the Investigation of Mediating Variables in Business Research
David p. mackinnon.
Department of Psychology, Arizona State University, Tempe, AZ 85287-1104, USA
Stefany Coxe
Amanda n. baraldi.
Business theories often specify the mediating mechanisms by which a predictor variable affects an outcome variable. In the last 30 years, investigations of mediating processes have become more widespread with corresponding developments in statistical methods to conduct these tests. The purpose of this article is to provide guidelines for mediation studies by focusing on decisions made prior to the research study that affect the clarity of conclusions from a mediation study, the statistical models for mediation analysis, and methods to improve interpretation of mediation results after the research study. Throughout this article, the importance of a program of experimental and observational research for investigating mediating mechanisms is emphasized.
This piece is the sixth in the Method Corner series featured by this Journal. This series focuses on some of the methodological issues encountered by business psychologists. Past pieces described aggregation of multidimensional constructs ( Johnson et al. 2011 ), methods to identify the importance of regression models ( Tonidandel and LeBreton 2011 ), polynomial regression ( Shanock et al. 2010 ), and method bias ( Conway and Lance 2010 ). The detection of mediators is also a methodological issue important to business psychology. Many theories in business research postulate a mediator ( M ) that transmits the effect of a predictor variable ( X ) to an outcome variable ( Y ) in a causal sequence such that X causes M and M causes Y. In more general terms, a mediating variable explains the process by which one variable causes another. Theories across many disciplines focus on mediating processes and many research questions lend themselves to these models. In intervention research, theory and prior empirical research determine which mediating variables are included as part of study design. If an intervention substantially changes a mediating variable that is causally related to an outcome, then a change in the mediator will produce a change in the outcome. For example, if organizational skills create more efficiency among employees, an employee program teaching organizational skills should increase organizational skills, resulting in greater employee efficiency.
Mediation theory is also applicable to studies that do not include an intervention. An observational variable can serve as a predictor or antecedent variable in a mediation model. For example, it has been suggested that the effects of psychological climate perceptions on performance are mediated by employee work attitudes ( Parker et al. 2003 ), where psychological climate does not represent an intervention but is an observed variable measured for each employee. Psychological climate is an observed variable and is not randomized, thus limiting conclusions regarding the causal nature of the mediating process. The lack of randomization makes it difficult to rule out alternative explanations of the relationship. Examples of alternative explanations include changes in employee work attitudes causing changes in psychological climate or that there is another variable causing changes in both psychological climate and employee work attitudes.
Since the classic articles on mediation by Alwin and Hauser (1975) , Judd and Kenny (1981) , James and Brett (1984) , and Baron and Kenny (1986) , thousands of articles have applied mediation analysis in many fields, including psychology (e.g., Fritz and MacKinnon 2007 ; Mackinnon et al. 2002 ), medicine ( Begg and Leung 2000 ), business ( Chiaburu and Byrne 2009 ; Hung et al. 2009 ), and many other fields (see MacKinnon 2008 ). The popularity of mediation analysis is growing because the method focuses on what is often the central scientific hypothesis: the process by which one variable affects another.
Mediators and Moderators and Confounders and Covariates
Before delving into the details of mediation analysis, we begin with definitions of several key terms that come into play when considering how three variables can be related. These potential relationships are important to understanding when mediation analysis is the appropriate choice for answering specific research questions. When considering the relationship of an independent variable ( X ) and a dependent variable ( Y ), an additional third variable ( Z ) may fill one of several roles. Each role for the third variable describes both a different theoretical model of the relationship between X , Y , and Z , as well as a different approach to the statistical analysis.
A third variable that is both unrelated to the predictor X and has little to no effect on the relationship between X and Y is called a covariate ; a covariate is not often of primary theoretical interest but is used to account for additional variation in the outcome Y. A third variable, Z , can be related to both X and Y in such a way that the inclusion of Z changes the relationship between X and Y. Such a variable is called a confounder , because it confounds or conceals the simple relation between X and Y (see Greenland and Morgenstern 2001 for information on confounders). A variable that is a moderator affects the direction and strength of the relationship between two variables such that the relationship between X and Y is different for varying levels of Z . A moderator is typically expressed as an interaction between the independent variable and the moderator, such that the effect of the independent variable on the dependent variable is conditional on the level of the moderator. A moderator may be a factor in an experimental manipulation with random assignment to varying levels (e.g., time between treatments) or a moderator may be a non-manipulated variable (e.g., age or gender). The understanding of a moderator effect is often a critical component to the generalizability of research findings to other populations, locations, and domains.
The focus of this article is on the third variable as a mediator variable. A simple mediation model is shown in Fig. 1 . A mediation relationship is one in which the independent variable causes the mediator which then causes the dependent variable ( Mackinnon 2008 ). Although variations of the definition of mediation exist in the literature (e.g., Holmbeck 1997 identifies terminological inconsistencies), we will assume a mediator to be a variable that transmits the effect of an independent variable to a dependent variable. Although the terms “mediator” and “mediated effect” will be used throughout this manuscript, other terms are used to describe these variables and effects in different areas of research. For example, the “mediated effect” is often referred to as the “indirect effect” because it represents the effect of the independent variable effect on the dependent variable effect via the mediator variable (i.e., indirectly rather than directly). The “mediator” is sometimes called the “intervening variable” because it is intermediate between the independent and the dependent variables.

Illustration of the mediation model using path diagrams
Though the primary focus of this article is mediation analysis, we feel obligated to spend some time comparing mediation and moderation effects. Both mediation effects and moderation effects are examined in psychological research with some frequency and involve a third variable. This often results in difficulties for researchers who are inexperienced in the nuances of these two types of effects; some may be confused about whether they should be performing a mediation analysis or not, while others may perform a mediation when their research question actually involves moderation (or vice versa). We would like to emphasize that determining whether to investigate a mediator effect versus a moderator effect depends entirely on the research question of interest. In general, moderators provide information on the circumstance under which effects are present , whereas mediators address the mechanisms by which an effect occurs . Mediation effects are exemplified by the question “How did it work?” because mediation examines the means by which the intervention affects outcomes. Moderation effects are exemplified by the question “Who did it work for?” because moderation examines which subgroups (e.g., boys vs. girls) show effects of the intervention on outcomes ( James and Brett 1984 ; Fairchild and MacKinnon 2008 ; MacKinnon 2011 ).
Mediation in Business Psychology
Theories across many substantive disciplines focus on mediating processes as explanations for how and why an antecedent variable is related to an outcome variable; business psychology is no exception. A casual review of the Journal of Business and Psychology found that between 2007 and 2010, over 30 articles purported to be addressing theoretical questions involving mediation or using mediation analysis. Of these, more than 20 articles cited classic mediation sources such as Baron and Kenny (1986) and Sobel (1982) .
Basic Mediation Model
A simple mediation model with one independent variable, X , one mediator, M , and one outcome variable, Y , provides information to investigate mediation by estimating three regression equations. The relationships between X , M , and Y are shown as path diagrams in Fig. 1 . Consider the study by Leach et al. (2009) , in which the relationship between meeting design characteristics ( design ) and perceived meeting effectiveness ( perception ) was mediated by attendees’ involvement during the meeting ( involvement ). In this example, design is the independent variable X , perception is the outcome variable Y , and involvement is the mediator M .
Equation (1) represents the relationship between the independent variable X and the dependent variable Y :
In terms of the example, this equation represents the relationship between design and perception , where the coefficient c represents the effect of design on perception , i is the intercept, and e 1 is the residual variance (i.e., the part of perception that is not explained by design ). Equation 2 represents the relationship between the independent variable X and the mediator M :
In the example, this equation represents the relationship between design and involvement . Equation 3 represents the somewhat more complex relationship between X , M , and Y :
This equation shows how perception can be predicted by both design and involvement . Since there are two predictors here, both c ′ and b are partial regression coefficients ; each regression coefficient is the effect of that predictor on the outcome, controlling for the effect of the other predictor. Using the example, the b coefficient is the effect of involvement on perception , controlling for design , and the c ′ coefficient is the effect of design on perception , controlling for involvement . There are several important assumptions of this single mediator model, including temporal precedence of the X → M → Y relationship and the assumption that no variables are omitted from the relationship; these assumptions, some of which are testable and some of which are not testable, are discussed later. As an aside, although we are presenting the mediation model in terms of three regression equations, regression is a special case of a structural equation model (SEM) and the methods described can often be done in either the regression or the structural equation model framework. However, structural equation modeling allows for more comprehensive modeling of measurement error, change over time, and multivariate dependent variables that are impossible or cumbersome with multiple regression analysis.
Decisions Prior to Mediation Analysis
Despite the extensive use of complex statistical modeling in the behavioral sciences, the quality of a research project is largely determined by the design decisions that are made before any analysis is done and even before the study is conducted. The conceptualization of a mediation analysis requires forethought about the relationships between the variables of interest and the theoretical meaning behind those relationships. Several other issues are important for researchers to consider prior to conducting a mediation analysis. Some of these decisions are common to all studies, but we will focus on the decisions that are of particular importance when planning a mediation analysis. In any study, a researcher must address the issues of manipulation versus observation, omitted variables that may be influencing results, reliability and validity of measures, and sample size to adequately detect effects. In addition, the theoretical task of choosing which variables will serve as mediators is critical. Table 1 summarizes some of the issues for consideration prior, during, and after mediation analysis.
Summary of issues before, during, and after mediation analysis
Randomization
Random assignment of subjects to experimental conditions is the gold standard for making causal inference about the relationship between two variables. In the case where X represents randomly assigned condition, the coefficients a and c represent causal effects under certain reasonable assumptions. The coefficients b and c ′ represent adjusted relations. Even though there is random assignment to experimental groups, the b and c ′ coefficients do not have a clear interpretation as causal effects because participants select their own value of the mediating variable. This ambiguity of self-selection to value of the mediator is a primary focus of modern causal inference approaches to mediation to be described later. Random assignment to the levels of X is common in many mediation studies but a second random assignment to the value of M (called “double randomization”) is rare and often difficult for ethical or logistical reasons. In double randomization studies, one randomized study evaluates the X to M relation and a second randomized study evaluates the M to Y relation adjusting for X ( MacKinnon 2008 ; MacKinnon and Pirlott 2011 ; Stone-Romero and Rosopa 2011 ; Spencer et al. 2005 ).
A second type of design to obtain some level of randomization of the mediating variable is called a blockage design. In this design, a manipulation is used to block or prevent the mediation process thereby demonstrating that the mediator was crucial ( MacKinnon 2008 ; Robins and Greenland 1992 ). If the blocking manipulation removes the mediation relation, this provides support for a mediational process. As an example of blockage design, return to the example in which the relationship between meeting design characteristics ( design ) and perceived meeting effectiveness ( perception ) was mediated by attendees’ involvement during the meeting ( involvement ). Using a blockage design, participants in the study may be assigned to a blocking treatment condition where deep involvement in the meeting was prevented (e.g., by a mildly distracting task or by not allowing communication with others at the meeting) in addition to the manipulation of the meeting design characteristics. If involvement is a mediator of the design-to-perception relationship, mediation effects should be related to the amount of involvement across groups and participants in the blockage treatment condition should not show as large of a mediated effect as participants in the control condition because the mediating process was blocked. A closely related type of design is the enhancement design which seeks to enhance (rather than eliminate as in the blockage design) the mediated effect in the treatment group. In the meeting perception example, participants in the study may be assigned to an enhancement treatment condition which creates even deeper involvement in the meeting (e.g., by telling them that a promotion will be offered to the person who learns the most from the meeting). If involvement is a mediator of the design to perception relationship, mediation effects should again be related to the amount of involvement across groups and participants in the enhancement treatment condition should show larger mediated effects than the participants in other examples (see other examples in MacKinnon 2008 ; Maxwell et al. 1986 ; Klesges et al. 1986 ).
Several options exist to strengthen causal arguments when randomization of X and/or M is not possible, including the selection of covariates before the study that may explain the X to M and the M to Y relations. Similarly, these covariates may be used in a propensity score model to address omitted variable explanations of mediated effects ( Coffman 2011 ; Jo et al. 2011 ). Instrumental variables may be used to estimate causal effects when randomization (particularly of X ) is not feasible ( MacKinnon 2008 , Chap. 13; Lockhart et al. 2010 ). In addition to statistical adjustments, experimental design methods such as the blockage and enhancement designs can strengthen causal interpretation by focusing on testing the consistency and specificity of mediation relations across different contexts, subgroups, and measures of the mediating and outcome variables ( MacKinnon and Pirlott 2011 ).
Omitted Variables
The term “ignorability” refers to the assumption that the relationship between two variables is unaffected by other variables (such as covariates, confounders, or moderators). Mediation analysis contains two major relationships that may be influenced by other variables: the X → M relationship and the M → Y relationship. Mediation therefore assumes a two part sequential ignorability assumption. There are many issues that arise in the causal interpretation of the single mediator model which stem from the two part sequential ignorability assumption ( Imai et al. 2010 ; Lynch et al. 2008 ; ten Have et al. 2007 ). The ignorability assumption for the X → M relationship can largely be addressed by randomizing the levels of X ; the ignorability assumption for the M → Y relationship is more difficult to justify and represents a challenging aspect of mediation analysis. Ignorability for the M to Y relation assumes randomization of participants at each level of X . In most research, this randomization is not possible and participants usually self-select their value of M. The extent to which sequential ignorability is a valid assumption may differ depending on the type of mediating variable. For example, if the mediators are selected because theory and prior empirical research suggest that they are causally related to the outcome variable, it may be reasonable to conclude that the b effect is known. Thus, it is only required that the levels of M be changed. In this case, the manipulation that changes the X to M relation will have the same expected change in the M to Y relation. Replication experiments can also further clarify the actual mediator from a host of other potential omitted variables. In this respect, replication studies with different manipulations are critical for identifying mediating variables.
Reliability
As in all research, the reliability and validity of proposed measures are best assessed prior to conducting the study. The reliability of a measurement is the extent to which a measure consistently reproduces values of the underlying true score. Valid measures measure the construct they are designed to measure. A program of research is typically needed to develop reliable and valid measures. Measurement is critical to mediation analysis and the search for mediating variables can be considered a measurement problem where science is advanced by more accurate measures of the mediating process ( MacKinnon 2008 ).
Studies on measurement error highlight the need for reliable measures when detecting a mediator. Hoyle and Kenny (1999) demonstrated that as the reliability of M decreases (i.e., as the reliability coefficient departs from one), the observed effect of M on the Y and b is underestimated and the observed effect of X on the Y and c ′ is overestimated. This results in an underestimation of the mediated effect and a decrease in the statistical power to detect the mediated effect. Due to the potential impact unreliability has on masking mediational effects, reliable mediating measures are crucial. One way to increase reliability is to obtain multiple indicators of the variable of interest and create a latent construct representing the variable of interest. The use of a latent variable model allows the estimation of associations between latent variables which are free of measurement error. However, using a latent variable model requires sufficiently large sample sizes and this is not always plausible. Modeling approaches which incorporate the effects of multiple methods such as multiple reporters or item types may yield more reliable relations than those ignoring method effects ( Geiser and Lockhart, under review ) and may provide promising tools for investigating mediation effects with more reliable measures.
Sample Size
Selection of sample size for adequate statistical power is an important part of designing any study. Although a large a sample size is ideal, sample size is often limited for reasons outside the control of a researcher, such as a small available population (e.g., local individuals of a specific age) or financial issues (e.g., excessive time or cost of measurements). However, even with an extremely large sample size, it will be important to obtain some measure of effect size to judge the importance or size of an effect. Mediation studies have traditionally been underpowered because the sample size requirements are much larger than those of simpler models, such as simple linear regression. Fritz and MacKinnon (2007) used simulations to determine sample sizes to obtain 0.80 power for small, medium, and large effect sizes in the single mediator model. A sample size of approximately 74 is required to detect a mediation effect when the path for the X to M relation and the M to Y relation is medium. A more complex mediation model would require a larger sample. The careful use of covariates can decrease the required sample size and repeated measures and longitudinal data can also improve the ability to detect effects. Required sample size and statistical power for more complicated mediation models has been outlined by Thoemmes et al. (2010) using a Monte Carlo approach in a covariance structure analysis program.
Choosing Mediators
The theoretical interpretation of the links in a mediation model can be thought of in terms of the theory for the process underlying the manipulations. These two processes are called the action theory and the conceptual theory ( Chen 1990 ; MacKinnon 2008 ). As shown in Fig. 2 , action theory corresponds to how the manipulation will affect the mediators (the relationship between X and M ) and conceptual theory focuses on how the mediators are related to the outcome of interest (the relationship between M and Y ). Consider an intervention designed to increase knowledge of the benefits of exercise and nutrition designed to increase employee well-being, which is measured as the number of sick days the employee uses. The action theory is that the program will increase the employees’ knowledge of exercise and nutrition. Conceptual theory says that knowledge of the benefits of exercise and nutrition will increase employee well-being, reducing the number of sick days. The use of action and conceptual theory can be used to demonstrate how a manipulation leads to changes in the dependent variable ( Ashby 1956 ; Lipsey 1993 ; MacKinnon 2008 ).

Action and conceptual theory
The informed choice of possible mediators often emerges from action and conceptual theory. Typically, conceptual theory is based on prior research that provides information about the relationship between a potential mediator and the outcome of interest. However, action theory can also inform the selection of mediators, based on what variables are able to be changed by experimental manipulation or intervention. For example, an experimental manipulation can change an individual’s beliefs about a product, but it typically cannot change aspects of an individual’s personality ( Table 1 ).
Table 2 summarizes six methods suggested by Mackinnon (2008) to choose mediators. Depending on the information available and the current state of a particular research area, any one of the six approaches may be a viable approach to choosing mediators. When the area of study is well-researched and a great deal of prior research is available on which to build, mediators can be chosen by performing a literature review to determine empirical relations between potential mediating variables and the outcome variable of interest, by targeting mediators based on an established theoretical framework in the area of substantive research, or based on prior mediation research. In particular, the “theory-driven” approach has received considerable support in the literature ( Chen 1990 ; Lipsey 1993 ; Sidani and Sechrest 1999 ). When the area of research is new and little prior research is available to guide the selection of mediator variables, different approaches to selecting mediators must be employed. Mediators may be identified by studying correlates of the outcome measure to identify mediators based on the conceptual theory of the outcome, using qualitative methods such as focus groups, or on the basis of common sense or intuition about what seems to be the best target of a program. Although these are less scientifically driven methods, they may be a good approach in newly developing fields.
Methods of choosing /mediators
Testing Multiple Mediators
Many studies include more than one mediator of an X – Y relationship. For example, Chen and Chiu (2008) examined several mediators of the relationship between supervisor support of employees and employee organizational citizenship behavior. They found that job satisfaction, person-organization fit, and job tension mediated the relationship between supervisor support and citizenship behavior. When there are multiple mediators, a simple approach is to evaluate one mediator at a time. Using the Chen and Chiu study as an example, one may initially examine the supervisor support → job satisfaction → citizenship behavior relationship. Looking at a single mediator at a time is a useful approach because specific theoretical hypotheses often focus on single mediators rather than groups of mediators. It is also wise to examine any potential moderator or interaction effects (discussed in more detail shortly) at the single-mediator stage. If the study involves many mediators, it will be necessary to implement some control for experiment-wise error due to multiple tests; the alpha or Type I error rate increases rapidly with multiple tests.
A model including all measured mediators should be estimated in addition to single mediator models. This is accomplished by expanding Eq. 3 to include all mediating variables. For example, in the case of three mediating variables, Eq. 3 can be reexpressed as:
Evaluating a model that includes all measured mediators is important because it is possible that the effect of a mediator may change in the presence of other mediators. Recall that the b and c ′ coefficients in these models are partial regression coefficients controlling for all other predictors, so the exclusion of some mediators could potentially change these values in the complete model. Including all measured mediators also produces a model which more closely matches reality, where all potential mediators are present. An additional benefit of multiple mediator models is the identification of mediation pathways that lead to beneficial relations on the outcome measures and mediating pathways that are actually counterproductive. These inconsistent mediation models, defined as mediation models where at least one mediated effect has a different sign than the direct effect, are more clearly identified in multiple mediator models ( MacKinnon et al. 2000 ).
Moderator Effects in Mediation Models
As previously described, moderation is an effect involving a third variable that changes the direction or magnitude of the relationship between two other variables. For example, the relationship between stress and health is moderated by social contacts; individuals with many friends show little relationship between stress and health while individuals with few friends have a strong positive relationship between stress and health ( Cohen and Wills 1985 ).
Moderator relationships can occur with mediation relationships. The combination of moderation and mediation can take on several forms. We briefly discuss these situations involving both mediation and moderation because they occur frequently within psychological research and can be confusing to understand. Moderation of a mediated effect occurs when a moderator variable ( Z ) affects the direction or strength of any or all the mediation regression coefficients. There are a number of ways to incorporate moderator effects into a mediation model. If the moderator is binary, such as gender, moderator effects can be evaluated by conducting analyses by group. Individual regression coefficients or estimates of the mediated effect can be compared across groups using t tests (see MacKinnon 2008 , p. 292). This method is straightforward and makes interpretation simpler, but it can only be used for binary moderators. More complex approaches are needed for continuous moderators and moderators with several categories.
In order to include continuous moderators in a mediation model, the moderators are incorporated into Eqs. 1 – 3 as interaction terms. For example, if a continuous variable, Z, is hypothesized to moderate the effect of X on M , Eq. 2 becomes:
where coefficient f represents the main or conditional effect of the moderator Z on the mediator M and coefficient g represents the interaction or moderator effect of X and Z . Tein et al. (2004) present a framework for testing moderation of all four mediation paths. This framework also allows for the inclusion of baseline covariates. For example, in evaluating a program to improve management and supervisor skills, a researcher may wish to control for pre-program level of skill by including this variable as a covariate.
Timing and Longitudinal Effects
The mediation model is a longitudinal model in that X precedes M and M precedes Y. However, in practice, tests of mediation may be conducted using cross-sectional data. There are a number of problems and limitations with using cross-sectional data to investigate longitudinal mediational processes, as outlined by several researchers ( Cheong et al. 2003 ; Cole and Maxwell 2003 ; MacKinnon 2008 ; Maxwell and Cole 2007 ). Conceptually, a problem arises because mediation is inherently a process that unfolds over time and cross-sectional data do not measure this unfolding over time. Statistically, several studies have shown that estimates of the cross-sectional-mediated effect are severely biased when compared to the estimates of the longitudinal mediated effect ( Maxwell and Cole 2007 ). The bias may be either positive or negative, further complicating the use of cross-sectional data.
The best-designed studies employ repeated measures because power to detect mediated effects is greatly enhanced. In addition, longitudinal studies allow for the measure of change in response to a manipulation ( Cohen 1988 ; Singer and Willet 2003 ). The methodological literature has emphasized the importance of temporal precedence in the investigation of mediation ( Gollob and Reichardt 1991 ; Judd and Kenny 1981 ; Kraemer et al. 2002 ; MacKinnon 1994 ) and has described methods for assessing longitudinal mediation ( Cheong et al. 2003 ; Cole and Maxwell 2003 ; MacKinnon 2008 ; Maxwell and Cole 2007 ). The evaluation of longitudinal mediation models is an important step in advancing mediation methods. Although there are several choices of longitudinal models described in the literature such as autoregressive models and latent change score models, latent growth curve models are a common choice for longitudinal mediation models ( Cheong 2002 ; Cheong et al. 2003 ). More detailed information on longitudinal mediation models can be found in Mitchell and James (2001) and MacKinnon (2008) .
Although longitudinal mediation modeling is the preferred method for evaluation of the mediation process, there are situations where only cross-sectional data are available. For example, secondary mediation analysis of data from a previously collected study may require the use of cross-sectional data. In this case, estimates of the cross-sectional mediated effect may not reflect the longitudinal mediated effect and researchers must provide evidence for temporal relations from theory or empirical research.
Decisions During Mediation Analysis
Recall the path model in Fig. 1 , which shows the directional relations between X , M , and Y for the single-mediator model. For the mediation framework that is most commonly used in psychology ( Baron and Kenny 1986 ; MacKinnon 2008 ), three regression equations are used to describe the relations in this model. These regression equations describe the effect of X on Y ( Eq. 1 ), the effect of X on M ( Eq. 2 ), and the effect of X on Y , controlling for M ( Eq. 3 ). The c coefficient is the total effect of X on Y. The c ′ coefficient is the direct effect of X on Y , controlling for M . The a coefficient corresponds to the “action theory” for the model, whereas the b coefficient corresponds to the “conceptual theory” for the model ( Fig. 2 ).
Baron and Kenny (1986) proposed a causal steps approach to testing whether statistical mediation is present in such a model. The causal steps approach describes a series of tests of regression coefficients that, together, can show mediation is occurring. The first step in this approach is to test whether changes in X produce changes in Y , i.e., whether there is an effect to be mediated. This is determined by the significance of the c regression coefficient in Eq. 1 . If there is no relation between X and Y , the causal steps approach stops. If there is a relation between X and Y , the next step is to determine if there is a relation between X and M by testing the a regression coefficient in Eq. 2 . Given that the independent variable significantly affects the mediator, the next step is to test whether M is related to Y , after controlling for the effect of X on Y. This is shown by testing the significance of the b regression coefficient in Eq. 3 . Finally, it must be shown that the effect of X upon Y , after controlling for M , is not significantly different than zero. The test of the c ′ coefficient in Eq. 3 should not be significant.
The requirements of the causal steps approach that c ≠ 0 and that c ′ = 0 results in reduced statistical power to detect a mediated effect. The requirement that c ≠ 0 is problematic because statistical tests are not absolute; there is always the potential for a Type I or Type II error in this decision. Additionally, if subgroups of participants (e.g., men vs. women) have opposing effects, ignoring these subgroups could result in a non-significant c value. The causal steps mediation approach also requires that c ′ = 0, meaning all effects from X to Y must be transmitted through M ; this type of mediation model is called a complete mediation model . The complete mediation model is the most defensible mediation conclusion from a research study, but it suffers from low statistical power when the causal steps approach is used. The complete mediation model is discussed in more detail shortly.
Modern methods of mediation analysis use regression (as well as structural equation modeling, an expansion of the regression framework) to quantify the mediated effect as a single number for which confidence intervals and significance tests can be calculated. The theory of mediation states that there is a causal relation in a mediation model, such that X causes M and M causes Y. Therefore, the mediated effect of X to Y via M can be quantified as the product of the regression coefficient relating X to M and the regression coefficient relating M to Y , or ab (using Eq. 2 and above). The test of ab can be more powerful than the test of c because it is a more precise explanation of how X affects Y ; the requirement that c be significant is not necessary for mediation to exist. Although modern methods pose that the test of c may not be as important in determining the mediating effect, the test of c is clearly important in its own right. A lack of statistically significant c is important in assessing manipulation and conceptual theory for future studies.
When both M and Y are observed and continuous (so that linear regression or structural equation modeling is used to estimate Eqs. 1 – 3 ) and there are no missing data, it can be shown that the difference between the total effect of X on Y and ( c ), and the direct effect of X on Y and ( c ′) is numerically equivalent to ab . As previously noted, this result holds only for linear models such as linear regression, but not for non-linear models such as logistic regression ( Pearl, in press ). The c – c ′ estimate of the mediated effect reflects that any difference between the total relation between X and Y (the c coefficient) and the direct effect of X on Y while controlling for M (the c ′ coefficient) must be due to the indirect or mediated effect. Some researchers have suggested that only c – c ′ should be used for making causal inferences. The reasoning behind this stance is that, typically, only X is randomly assigned, whereas M is observed or self-selected by the participant. Therefore, the c – c ′ estimate of the mediated effect involves using only regression coefficients that are based upon random assignment to experimental conditions. The point of contention is often irrelevant because the two quantities ab and only c – c ′ are identical in linear regression and structural equation modeling of continuous measures. For logistic regression or other nonlinear statistical methods, the two estimators of the mediated effect may not be equal and may have different meanings ( Imai et al. 2010 ; Pearl 2011).
Complete Versus Partial Mediation
Some researchers (e.g., James and Brett 1984 ) suggest a slightly different approach to quantifying the mediated effect than has been presented here. James and Brett suggest that the model described by Eqs. 1 – 3 implicitly assumes partial mediation , i.e., the mediated path via M accounts for only some of the effect of X on Y. In other words, this approach implies a non-zero direct path or c ′ coefficient. James et al. (2006) suggest an alternative approach that begins from an assumption of complete mediation (sometimes also called “full mediation”), where the c ′ path is assumed to be zero and all effects of X on Y are transmitted through the mediator M . In the complete mediation framework, two regression coefficients are estimated. First, the effect of X on M is estimated using the a coefficient in Eq. 2 above. Second, the effect of M on Y is estimated using the expression
where b ′ is a new regression coefficient representing the relation of M and Y , completely ignoring X . The mediated effect is calculated as ab ′ and reflects the use of this new coefficient.
The complete mediation approach has several attractive features. First, fixing the value of the c ′ path to zero means that, from a structural equation modeling perspective, the complete mediation model is identified and has degrees of freedom, allowing for goodness-of-fit tests. Goodness-of-fit tests allow a researcher to test how closely the model matches the observed data, in addition to testing whether individual paths and the mediated effect are significantly different from zero. Second, the complete mediation model is a more parsimonious explanation of the causal relation from X to M to Y. From a philosophy of science perspective, a simpler or more parsimonious model is preferred. However, complete mediation is uncommon in many areas of psychology so it is likely that there is a direct effect of X on Y , and testing for complete mediation as a first step may not be informative for psychological research; this is a weakness shared by the causal steps approach to mediation described in Judd and Kenny (1981) , which requires that the null hypothesis that the c ′ path is equal to zero is not rejected.
Tests of Mediation and Confidence Limit Estimation
There are many statistical tests to evaluate the mediated effect. Some tests of the mediated effect involve dividing the estimate of the mediated effect by an estimate of its standard error ( Wald 1943 ) and this ratio is then compared to an appropriate statistical distribution, such as the normal distribution. Other tests of the mediated effect are non-parametric, resampling tests such as bootstrapping which use the observed data to determine the distribution and standard error of the mediation estimate. MacKinnon et al. (2002) provide an evaluation of fourteen different methods of evaluating the mediated effect, including methods of calculating the standard errors for ab and c – c ′.
One of the most common tests of the ab mediated effect is based on the multivariate delta standard error ( Sobel 1982 ). The ratio of the mediated effect to its standard error is compared to a standard normal ( z ) distribution to test significance. This method has reduced power because the product of two normally distributed regression coefficients is not normally distributed and instead follows the distribution of the product. The distribution of the product is variable in shape depending on the magnitude of the coefficients and is often asymmetric and highly kurtotic ( Aroian 1947 ; Craig 1936 ).
As described in MacKinnon et al. (2002) , tests of the mediated effect that are based on the distribution of the product have more accurate Type I error rates and have more power than many other tests. Critical values for the distribution of the product produce more accurate confidence intervals for the mediated effect. PRODCLIN (distribution of the PRODuct Confidence Limits for INdirect effects) automates the selection of critical values for the distribution of the product ( MacKinnon et al. 2007 ). The user inputs values of a , b , their respective standard errors, and the desired Type I error rate (e.g., α = .05); the program returns the upper and lower asymmetric confidence limits for the mediated effect. A new version of this program (RMEdiation; Tofighi and MacKinnon 2011 ) now provides several additional capabilities including plots of the distribution of the product and several options for confidence limits. Mackinnon et al. (2004) found that tests of significance based on the distribution of the product outperformed other single-sample methods in terms of Type I error rates, power, and accuracy of confidence limits.
Bootstrapping is a resampling technique that is often used to evaluate a test statistic (such as the mediated effect) when the true distribution of the statistic is either unknown or difficult to obtain. The bootstrapping method involves taking many (e.g., 1000) repeated “samples” from the observed sample, calculating the statistic of interest, and producing a distribution based on these values of the statistic. Confidence intervals are obtained empirically, i.e., by observing the value in the bootstrapped distribution beyond which a certain proportion of the test statistics lie. For example, for a two-tailed test with an alpha value of .05, order the 1000 bootstrap statistics from lowest to highest, and choose the value of the (1000 × 0.025) = 25th observation as the lower critical bound of the confidence interval and the value of the (1000 × 0.975) = 975th observation as the upper bound of the confidence interval. Bootstrap methods for determining the significance of the mediated effect generally perform well in terms of power and Type 1 error (see MacKinnon et al. 2004 ). Routines to perform bootstrap analyses are included in many common statistical software programs, including AMOS, M plus , and EQS and programs for conducting bootstrap analyses in SAS and SPSS are also available ( Lockwood and MacKinnon 1998 ; Preacher and Hayes 2004 ). Another advantage of bootstrap methods is that they can be easily expanded as the complexity of the model increases; for example, bootstrapping can easily be applied to a multiple mediator model in which X → M 1 → M 2 → Y , where the mediated effect is calculated as the product of three regression coefficients.
In summary, an ideal method includes an estimate of the mediated effect along with a confidence interval for the indirect effect. Confidence intervals may be made with a bootstrap method or the distribution of the product. The effect size for the paths in the mediation model such as the standardized coefficients or partial correlation measure should be reported. Note that it is important to report statistical tests of the relation of X to Y (i.e., the c coefficient from Eq. 1 in the single mediator model), but this relation is not necessary for mediation to exist. In fact, a test of mediation may be more important when there is not a statistically significant relation of X to Y because the path from X to M represents a test of action theory and the path from M to Y represents a test of conceptual theory. When reporting mediation analyses, it is important to provide detailed information regarding the models tested along with the coefficients from these models (e.g. a , b , c ′, and ab ) and the confidence intervals ( Mackinnon 2008 ).
Decisions Following Mediation Analysis
Several assumptions were made for the regression equations described earlier that can be addressed in the design phase of the study or by appropriate statistical analysis. However, several assumptions are untestable and cannot be completely addressed using design or statistical approaches. These assumptions are related to confounders of the mediated effect, higher order relations between X and M , the causal ordering of X , M , and Y , and measurement error. Many aspects of these assumptions can be addressed by sensitivity analysis, a method of assessing how much the results of an analysis may change due to violation of assumptions. Typically, sensitivity analysis involves systematically changing values of specific parameter values in the model (for example, the a path from X to M ) to determine how much the parameter must change in order to change the substantive interpretation (i.e., significance) or change the estimates by a specific, pre-determined amount (e.g., to change the estimate of the mediated effect by 25%). Sensitivity analysis is one of the most challenging aspects of mediation analysis, but there has been considerable development in these methods in recent years.
Confounders
For the ab estimator of the mediated effect and ordinary least squares regression, the errors in Eqs. 2 and 3 are assumed to be independent. The uncorrelated errors assumption may be violated if there are confounding variables that are omitted from the analysis. Confounders can have a substantial effect on the analysis of the mediated effect. Figure 3 shows how confounders can potentially influence multiple paths in a mediation model. Ideally, measures of the potential confounding variables are included in the statistical model, but if they are not, the confounders may result in biased estimates. As with any study, even when some potential confounders are included in the analysis, there is no guarantee that all possible confounders were included.

Confounders of mediation relations. The true model requires d 1 , d 2 , d 3 , and d 4 , otherwise the coefficients are confounded
Sensitivity analysis is one way to assess the influence of omitted variables on the observed mediation relations. Since randomization of X theoretically eliminates confounders in the X → M relationship, the goal of sensitivity analysis in mediation for experimental studies is typically to assess how large a confounder effect on the M → Y relation (i.e., sequential ignorability) must be in order to invalidate the conclusions of the analysis ( Frank 2000 ; Li et al. 2007 ; Lin et al. 1998 ; Rosenbaum 2002 ). The correlation between the errors in Eqs. 2 and 3 reflects the contribution of omitted variables to the observed relation of M to Y , or the degree to which the assumption of sequential ignorability is violated. Thus, by systematically increasing the correlation between the errors in Eqs. 2 and 3 , one can evaluate how much the b and c ′ coefficients change due to violation of this assumption ( Imai et al. 2010 ).
Promising approaches to improving causal inference by addressing the bias introduced by omitted variables have been proposed ( Frangakis and Rubin 2002 ; Holland 1988 ; Jo 2008 ; Murphy et al. 2001 ; Pearl 2009 , in press ; Robins and Greenland 1992 ; Robins et al. 1992 ; Rubin 2004 ; Shipley 2000 ; Sobel 1998 , 2008 ; Winship and Morgan 1999 ) but most have not been extensively evaluated in simulation studies and applied settings. Vander Weele (2008 , 2010 ) has formalized several useful methods to probe bias in mediation relations when one or both assumptions of Sequential Ignorability have been violated. Imai et al. (2010) describe another method and include a computer program to assess the sensitivity of the results to potential confounders. These methods allow the researcher to draw a conclusion about the direction of the bias by suggesting relations of unmeasured confounders on relations in the mediation model.
X – M Interaction
The standard single mediator model assumes that Eqs. 2 and 3 represent causal relations that are linear, additive, and recursive ( Holland 1988 ; James and Brett 1984 ; James et al. 2006 ; McDonald 1997 ). An additivity assumption implies that there is no interaction between X and M ( Collins et al. 1998 ; Judd and Kenny 1981 ), i.e., the effect of X on Y does not depend on the value of M and the effect of M on Y does not depend on the value of X . The additivity assumption can be directly tested by including the interaction of X and M; if the interaction term is significant, the assumption of additivity is violated. In this context, the mediated effect differs across levels of X and further analyses can explore the size and significance of the mediated effect at different values of X .
Causal Ordering
Since mediation is a causal model, it is important to clearly define the causal chain from X to M to Y. The mediation model makes the assumption that the correct causal order has been specified, such that X causes M and M causes Y. When X is randomly assigned, it is clear that X occurs before M and Y. However, the ordering of M and Y is less clear and theory and prior empirical research can help make the causal ordering more concrete.
Hill (1965) outlined nine considerations for clarifying the ordering of causal relations. These points were initially developed to investigate smoking as a cause of cancer but have applications to establishing causal ordering in mediation models. These are substantive considerations rather than statistical tests, so they require a substantive researcher to carefully evaluate the variables involved. The nine criteria are (1) strength, (2) consistency, (3) specificity, (4) temporality, (5) biological gradient, (6) plausibility, (7) coherence, (8) experiment, and (9) analogy. According to Hill, causality is implied by (1) a stronger relation rather than a weaker relation, (2) consistent findings by multiple people in multiple samples, (3) specific findings (e.g., about a specific disease rather than general unhealthiness), (4) the “cause” occurring prior to the “effect” in time, (5) a larger effect seen with larger exposure to the “cause,” (6) a plausible and sensible mechanism by which the causal relationship occurs, (7) agreement between laboratory and observational studies, (8) experimental evidence of the causal relationship, and (9) similar “causes” resulting in similar “effects.” These criteria can be applied to M and Y (or to X , M , and Y , if X is not randomized) to provide evidence that the presented causal ordering is the correct ordering.
Measurement Error
Mediation analysis assumes that the measures are both reliable and valid ( Baron and Kenny 1986 ; Holland 1988 ; James and Brett 1984 ; MacKinnon 2008 ; McDonald 1997 ). As previously discussed, Hoyle and Kenny (1999) showed that unreliability of the mediator leads to underestimation of the b path and overestimation of c ′ which results in underestimation of the mediated effect and lower power to detect the mediated effect.
After the study is completed, a correction for unreliability in measured variable models can be applied to obtain estimates of coefficients if reliability is assumed to be a certain value (see MacKinnon 2008 , p. 189). This new model results in the estimation of coefficients that have been adjusted for more or less reliable measures. Limitations of this method are that the reliability estimate used may not always be accurate and the correction approach may not adequately address measures that are actually composed of more than one factor. In addition, if there are multiple factors for some measures, the relationship of these factors may have important relationships with other variables in the model that will be ignored ( Bagozzi and Heatherton 1994 ).
Planning for the Next Study
Every study can be thought of as a single piece of an overall body of research; each study builds upon previous studies, resulting in an accumulation of knowledge. Studies involving statistical mediation are no different. In this article, we have discussed a number of potential limitations to the interpretation of mediation analysis, particularly causal inference limitations. For example, we discussed potential confounders of the mediated effect, including experimental design methods that can help rule out the presence of potential confounders and newly developed sensitivity analysis methods that can determine the amount of bias caused by omitted confounders. The consideration of limitations of interpretability and generalizability of results may be especially important for mediation studies because of the number of omitted variables that may affect observed results. As an illustration, consider a study involving a non-randomly assigned X variable. There are several limitations of the interpretation of the mediated effects in this study. The relationship between X and M may be biased for several reasons; the true causal ordering of X and M is potentially unclear and there may be confounders that bias the estimate of the relationship between X and M . A follow-up study can address both of these limitations by incorporating a randomly assigned manipulation of the X variable. Random assignment of the X variable ensures the causal ordering of X and M because X is randomly assigned before M is measured; random assignment also ensures that there are no confounders of the relationship between X and M . If the follow-up study produced results that match the initial study, the researcher can be confident that causal inference based on the X → M relationship is sound. If the follow-up study produces conclusions that differ from the initial study, further research is needed; for example, confounders of the M → Y relationship may be affecting the results. In either situation, multiple studies are typically required to produce a clear picture of the true relationships.
Statistical mediation analysis is a powerful tool for testing the process by which an effect occurs in both experimental and observational studies. In this article, we discussed how design decisions made prior to conducting a study and statistical choices made during analysis influence the conclusions that can be drawn from a study that involves statistical mediation. We also discussed the limitations of interpretation of a mediation process for even well-designed and analyzed studies. The major point is that the investigation of mediation processes requires careful planning and is part of a cumulative program of research using evidence from a variety of sources including clinical observation, qualitative studies, and replication ( MacKinnon 2008 ). Mediation analysis is popular because it directly addresses important theoretical questions about processes by which effects occur. This importance of mediating variables for scientific understanding was identified many years ago ( Lazarsfeld 1955 ; Woodworth 1928 ) and there is now a body of statistical techniques to test and evaluate mediation theory. Business research is an ideal area for the application of these techniques to evaluate whether a variable is truly intermediate in a causal sequence.
Acknowledgments
This article was supported in part by Public Health Service Grant DA09757 from the National Institute on Drug Abuse.
Contributor Information
David P. MacKinnon, Department of Psychology, Arizona State University, Tempe, AZ 85287-1104, USA.
Stefany Coxe, Department of Psychology, Arizona State University, Tempe, AZ 85287-1104, USA.
Amanda N. Baraldi, Department of Psychology, Arizona State University, Tempe, AZ 85287-1104, USA.
- Alwin DF, Hauser RM. The decomposition of effects in path analysis. American Sociological Review. 1975; 40 :37–47. [ Google Scholar ]
- Aroian LA. The probability function of the product of two normally distributed variables. The Annals of Mathematical Statistics. 1947; 18 :265–271. [ Google Scholar ]
- Ashby WR. An introduction to cybernetics. New York: Wiley; 1956. [ Google Scholar ]
- Bagozzi R, Heatherton T. A general approach to representing multifaceted personality constructs: Application to state self-esteem. Structural Equation Modeling. 1994; 1 :35–67. [ Google Scholar ]
- Baron RM, Kenny DA. The moderator-mediator variable distinction in social psychological research: Conceptual, strategic, and statistical considerations. Journal of Personality and Social Psychology. 1986; 51 :1173–1182. [ PubMed ] [ Google Scholar ]
- Begg CB, Leung DHY. On the use of surrogate end points in randomized trials. Journal of the Royal Statistical Society (Series A—Statistics in Society) 2000; 163 :15–28. [ Google Scholar ]
- Chen HT. Theory-driven evaluations. Newbury Park, CA: Sage; 1990. [ Google Scholar ]
- Chen CC, Chiu SF. An integrative model linking supervisor support and organizational citizenship behavior. Journal of Business and Psychology. 2008; 23 :1–10. [ Google Scholar ]
- Cheong J. Doctoral Dissertation. Arizona State University; Tempe, AZ: 2002. Investigation of a method to evaluate mediating mechanisms to reduce adolescent DUI in a school-based prevention program. [ Google Scholar ]
- Cheong J, MacKinnon DP, Khoo ST. Investigation of mediational processes using parallel process latent growth curve modeling. Structural Equation Modeling: A Multidisciplinary Journal. 2003; 10 :238. [ PMC free article ] [ PubMed ] [ Google Scholar ]
- Chiaburu DS, Byrne ZS. Predicting OCB role definitions: Exchanges with the organization and psychological attachment. Journal of Business and Psychology. 2009; 24 :201–214. [ Google Scholar ]
- Coffman DL. Estimating causal effects in mediation analysis using propensity scores. Structural Equation Modeling. 2011; 18 :357–369. [ PMC free article ] [ PubMed ] [ Google Scholar ]
- Cohen J. Statistical power analysis for the behavioral sciences. 2. Hillsdale, NJ: Lawrence Erlbaum Associates; 1988. [ Google Scholar ]
- Cohen S, Wills TA. Stress, social support and the buffering hypothesis. Psychological Bulletin. 1985; 98 :310–357. [ PubMed ] [ Google Scholar ]
- Cole DA, Maxwell SE. Testing mediational models with longitudinal data: Questions and tips in the use of structural equation modeling. Journal of Abnormal Psychology. 2003; 112 :558–577. [ PubMed ] [ Google Scholar ]
- Collins L, Graham J, Flaherty B. An alternative framework for defining mediation. Multivariate Behavioral Research. 1998; 33 :295–312. [ PubMed ] [ Google Scholar ]
- Conway JM, Lance CE. What reviewers should expect from authors regarding common method bias in organizational research. Journal of Business and Psychology. 2010; 25 :325–334. [ Google Scholar ]
- Craig CC. On the frequency function of xy. The Annals of Mathematical Statistics. 1936; 7 :1–15. [ Google Scholar ]
- Fairchild AJ, MacKinnon DP. A general model for testing mediation and moderation effects. Prevention Science. 2008; 10 :87–99. [ PMC free article ] [ PubMed ] [ Google Scholar ]
- Frangakis CE, Rubin DB. Principal stratification in causal inference. Biometrics. 2002; 58 :21–29. [ PMC free article ] [ PubMed ] [ Google Scholar ]
- Frank KA. Impact of a confounding variable on a regression coefficient. Sociological Methods & Research. 2000; 29 :147–194. [ Google Scholar ]
- Fritz MS, MacKinnon DP. Required sample size to detect the mediated effect. Psychological Science. 2007; 18 :233–239. [ PMC free article ] [ PubMed ] [ Google Scholar ]
- Geiser C, Lockhart GL. A comparison of four approaches to account for method effects in latent state-trait models. (under review) [ PMC free article ] [ PubMed ] [ Google Scholar ]
- Gollob HF, Reichardt CS. Interpreting and estimating indirect effects assuming time lags really matter. In: Collins LM, Horn JL, editors. Best methods for the analysis of change: Recent advances, unanswered questions, future directions. Washington, DC: American Psychological Association; 1991. pp. 243–259. [ Google Scholar ]
- Greenland S, Morgenstern H. Confounding in health research. Annual Review of Public Health. 2001; 22 :189–212. [ PubMed ] [ Google Scholar ]
- Hill A. The environment and disease: Association or causation? Proceedings of the Royal Society of Medicine. 1965; 58 :295–300. [ PMC free article ] [ PubMed ] [ Google Scholar ]
- Holland PW. Causal inference, path analysis, and recursive structural equation models. Sociological Methodology. 1988; 18 :449–484. [ Google Scholar ]
- Holmbeck GN. Toward terminological, conceptual, and statistical clarity in the study of mediators and moderators: Examples from the child-clinical and pediatric psychology literatures. Journal of Consulting and Clinical Psychology. 1997; 65 :599–610. [ PubMed ] [ Google Scholar ]
- Hoyle RH, Kenny DA. Sample size, reliability, and tests of statistical mediation. In: Hoyle RH, editor. Statistical strategies for small sample research. Thousand Oaks, CA: Sage; 1999. pp. 195–222. [ Google Scholar ]
- Hung TK, Chi NW, Lu WL. Exploring the relationships between perceived coworker loafing and counterproductive work behaviors: The mediating role of a revenge motive. Journal of Business and Psychology. 2009; 24 :257–270. [ Google Scholar ]
- Imai K, Keele L, Tingley D. A general approach to causal mediation analysis. Psychological Methods. 2010; 15 :309–334. [ PubMed ] [ Google Scholar ]
- James LR, Brett JM. Mediators, moderators, and tests for mediation. Journal of Applied Psychology. 1984; 69 :307–321. [ Google Scholar ]
- James LR, Mulaik SA, Brett JM. A tale of two methods. Organizational Research Methods. 2006; 9 :233–244. [ Google Scholar ]
- Jo B. Causal inference in randomized experiments with mediational processes. Psychological Methods. 2008; 13 :314–336. [ PMC free article ] [ PubMed ] [ Google Scholar ]
- Jo B, Stuart EA, MacKinnon DP, Vinokur AD. The use of propensity scores in mediation analysis. Multivariate Behavioral Research. 2011; 3 :425–452. [ PMC free article ] [ PubMed ] [ Google Scholar ]
- Johnson RE, Rosen CC, Chang C. To aggregate or not to aggregate: Steps for developing and validating higher-order multidimensional constructs. Journal of Business and Psychology. 2011; 26 :241–248. [ Google Scholar ]
- Judd CM, Kenny DA. Process analysis. Evaluation Review. 1981; 5 :602–619. [ Google Scholar ]
- Klesges RC, Vasey MM, Glasgow RE. A worksite smoking modification competition: Potential for public health impact. American Journal of Public Health. 1986; 76 :198–200. [ PMC free article ] [ PubMed ] [ Google Scholar ]
- Kraemer HC, Wilson GT, Fairburn CG, Agras WS. Mediators and moderators of treatment effects in randomized clinical trials. Archives of General Psychiatry. 2002; 59 :877–884. [ PubMed ] [ Google Scholar ]
- Lazarsfeld PF. Interpretation of statistical relations as a research operation. In: Lazardsfeld PF, Rosenberg M, editors. The language of social research: A reader in the methodology of social research. Glencoe, IL: Free Press; 1955. pp. 115–125. [ Google Scholar ]
- Leach DJ, Rogelberg SG, Warr PB, Burnfield JL. Perceived meeting effectiveness: The role of design characteristics. Journal of Business and Psychology. 2009; 24 :65–76. [ Google Scholar ]
- Li Y, Bienias JL, Bennett DA. Confounding in the estimation of mediation effects. Computational Statistics & Data Analysis. 2007; 51 :3173–3186. [ PMC free article ] [ PubMed ] [ Google Scholar ]
- Lin DY, Psaty BM, Kronmal RA. Assessing the sensitivity of regression results to unmeasured confounders in observational studies. Biometrics. 1998; 54 :948–963. [ PubMed ] [ Google Scholar ]
- Lipsey MW. Theory as method: Small theories of treatments. In: Sechrest LB, Scott AG, editors. Understanding causes and generalizing about them: New directions for program evaluation. San Francisco: Jossey-Bass; 1993. pp. 5–38. [ Google Scholar ]
- Lockhart G, MacKinnon DP. Test of an instrumental variable approach to mediation analysis. In: MacKinnon DP, Lockhart G, editors. New directions in mediating variable analysis; Poster symposium presented at the Annual Meeting of the Society for Prevention Research; Denver, CO. 2010. (Discussants) [ Google Scholar ]
- Lockwood CM, MacKinnon DP. Bootstrapping the standard error of the mediated effect; Proceedings of the twenty-third annual SAS users group international conference; Cary, NC: SAS Institute; 1998. pp. 997–1002. [ Google Scholar ]
- Lynch KG, Cary M, Gallop R, Ten Have TR. Causal mediation analyses for randomized trials. Health Services and Outcomes Research Methodology. 2008; 8 :57–76. [ PMC free article ] [ PubMed ] [ Google Scholar ]
- Mackinnon DP. Analysis of mediating variables in prevention and intervention research. In: Cazares A, Beatty LA, editors. Scientific methods for prevention/intervention research. NIDA Research Monograph Series 139, DHHS Publication No 94-3631. Washington, DC: U.S. Department of Health and Human Services; 1994. pp. 127–153. [ PubMed ] [ Google Scholar ]
- MacKinnon DP. Introduction to statistical mediation analysis. New York: Lawrence Erlbaum Associates; 2008. [ Google Scholar ]
- MacKinnon DP. Integrating mediators and moderators in research design. Research on Social Work Practice. 2011; 21 :675–681. [ PMC free article ] [ PubMed ] [ Google Scholar ]
- MacKinnon DP, Fritz MS, Williams J, Lockwood CM. Distribution of the product confidence limits for the indirect effect: Program PRODCLIN. Behavior Research Methods. 2007; 39 :384–389. [ PMC free article ] [ PubMed ] [ Google Scholar ]
- MacKinnon DP, Krull JL, Lockwood CM. Equivalence of the mediation, confounding, and suppression effect. Prevention Science. 2000; 1 :173–181. [ PMC free article ] [ PubMed ] [ Google Scholar ]
- MacKinnon DP, Lockwood CM, Hoffman JM, West SG, Sheets V. A comparison of methods to test mediation and other intervening variable effects. Psychological Methods. 2002; 7 :83–104. [ PMC free article ] [ PubMed ] [ Google Scholar ]
- MacKinnon DP, Lockwood CM, Williams J. Confidence limits for the indirect effect: Distribution of the product and resampling methods. Multivariate Behavioral Research. 2004; 39 :99–128. [ PMC free article ] [ PubMed ] [ Google Scholar ]
- MacKinnon DP, Pirlott A. The unbearable lightness of b: Approaches to improving causal inference in mediation studies. 2011. (submitted) [ Google Scholar ]
- Maxwell JA, Bashook PG, Sandlow LJ. Combining ethnographic and experimental methods in educational evaluation: A case study. In: Fetterman DM, Pittman MA, editors. Educational evaluation: Ethnography in theory, practice, and politics. Newbury Park, CA: Sage; 1986. pp. 121–143. [ Google Scholar ]
- Maxwell SE, Cole DA. Bias in cross-sectional analyses of longitudinal mediation. Psychological Methods. 2007; 12 :23–44. [ PubMed ] [ Google Scholar ]
- McDonald RP. Haldane’s lungs: A case study in path analysis. Multivariate Behavioral Research. 1997; 32 :1–38. [ PubMed ] [ Google Scholar ]
- Mitchell TR, James LR. Building better theory: Time and the specification of when things happen. Academy of Management Review. 2001; 25 :530–547. [ Google Scholar ]
- Murphy SA, van der Laan MJ, Robins JM. Marginal mean models for dynamic regimes. Journal of the American Statistical Association. 2001; 96 :1410–1423. [ PMC free article ] [ PubMed ] [ Google Scholar ]
- Parker CP, Baltes BB, Young SA, Huff JW, Altmann RA, Lacost HA, et al. Relationships between psychological climate perceptions and work outcomes: A meta-analytic review. Journal of Organizational Behavior. 2003; 24 :389–416. [ Google Scholar ]
- Pearl J. Graphs, causality, and structural equation models. Sociological Methods & Research. 2009; 27 :226–284. [ Google Scholar ]
- Pearl J. The foundations of causal inference. Sociological Methodology. 2010; 40 :75–149. [ Google Scholar ]
- Pearl J. The mediation formula: A guide to the assessment of causal pathways for direct and indirect effects. Prevention Science (in press) [ PubMed ] [ Google Scholar ]
- Preacher KJ, Hayes AF. SPSS and SAS procedures for estimating indirect effects in simple mediation models. Behavior Research Methods, Instruments, & Computers. 2004; 36 :717–731. [ PubMed ] [ Google Scholar ]
- Robins JM, Greenland S. Identifiabilty and exchangeability for direct and indirect effects. Epidemiology. 1992; 3 :143–155. [ PubMed ] [ Google Scholar ]
- Robins JM, Mark SD, Newey WK. Estimating exposure effects by modelling the expectation of exposure conditional on confounders. Biometrics. 1992; 48 :479–495. [ PubMed ] [ Google Scholar ]
- Rosenbaum PR. Covariance adjustment in randomized experiments and observational studies. Statistical Science. 2002; 17 :286–327. [ Google Scholar ]
- Rubin DB. Direct and indirect causal effects via potential outcomes. Scandinavian Journal of Statistics. 2004; 31 :161–170. [ Google Scholar ]
- Shanock LR, Baran BE, Gentry WA, Pattison SC, Heggestad ED. Polynomial regression with response surface analysis: A powerful approach for examining moderation and overcoming limitations of difference scores. Journal of Business and Psychology. 2010; 25 :543–554. [ Google Scholar ]
- Shipley B. A new inferential test for path models based on directed acyclic graphs. Structural Equation Modeling. 2000; 7 :206–218. [ Google Scholar ]
- Sidani S, Sechrest L. Putting program theory into operation. American Journal of Evaluation. 1999; 20 :227–238. [ Google Scholar ]
- Singer JD, Willet JB. Applied longitudinal data analysis: Modeling change and event occurrence. London: Oxford University Press; 2003. [ Google Scholar ]
- Sobel ME. Asymptotic confidence intervals for indirect effects in structural equation models. Sociological Methodology. 1982; 13 :290–312. [ Google Scholar ]
- Sobel ME. Causal inference in statistical models of the process of socioeconomic achievement: A Case study. Sociological Methods and Research. 1998; 27 :318–348. [ Google Scholar ]
- Sobel ME. Identification of causal parameters in randomized studies with mediating variables. Journal of Educational and Behavioral Statistics. 2008; 33 :230–251. [ Google Scholar ]
- Spencer SJ, Zanna MP, Fong GT. Establishing a causal chain: Why experiments are often more effective than mediational analyses in examining psychological processes. Journal of Personality and Social Psychology. 2005; 89 :845–851. [ PubMed ] [ Google Scholar ]
- Stone-Romero EF, Rosopa PJ. Experimental tests of mediation: Prospects, problems, and some solutions. Organizational Research Methods. 2011; 14 :631–646. [ Google Scholar ]
- Tein J, Sandler IN, MacKinnon DP, Wolchik SA. How did it work? Who did it work for? Mediation in the context of a moderated prevention effect for children of divorce. Journal of Consulting and Clinical Psychology. 2004; 72 :617–624. [ PMC free article ] [ PubMed ] [ Google Scholar ]
- ten Have TRT, Joffe MM, Lynch KG, Brown GK, Maisto SA, Beck AT. Causal mediation analyses with rank preserving models. Biometrics. 2007; 63 :926–934. [ PubMed ] [ Google Scholar ]
- Thoemmes F, MacKinnon DP, Reiser MR. Power analysis for complex mediational designs using Monte Carlo methods. Structural Equation Modeling. 2010; 17 :510–534. [ PMC free article ] [ PubMed ] [ Google Scholar ]
- Tofighi D, MacKinnon DP. RMediation: An R package for mediation analysis confidence intevals. Behavior Research Methods. 2011; 43 :692–700. [ PMC free article ] [ PubMed ] [ Google Scholar ]
- Tonidandel S, LeBreton JM. Relative importance analysis: A useful supplement to regression analysis. Journal of Business and Psychology. 2011; 26 :1–9. [ Google Scholar ]
- VanderWeele TJ. Simple relations between principal stratification and direct and indirect effects. Statistics and Probability Letters. 2008; 78 :2957–2962. [ Google Scholar ]
- VanderWeele TJ. Bias formulas for sensitivity analysis for direct and indirect effects. Epidemiology. 2010; 21 :540–551. [ PMC free article ] [ PubMed ] [ Google Scholar ]
- Wald A. Tests of statistical hypotheses concerning several parameters when the number of observations is large. Transactions of the American Mathematical Society. 1943; 54 :426–482. [ Google Scholar ]
- Winship C, Morgan SL. The estimation of causal effects from observational data. Annual Review of Sociology. 1999; 25 :659–706. [ Google Scholar ]
- Woodworth RS. Dynamic psychology. In: Murchison C, editor. Psychologies of 1925. Worcester, MA: Clark Universal Academy Press, Inc; 1928. pp. 111–126. [ Google Scholar ]
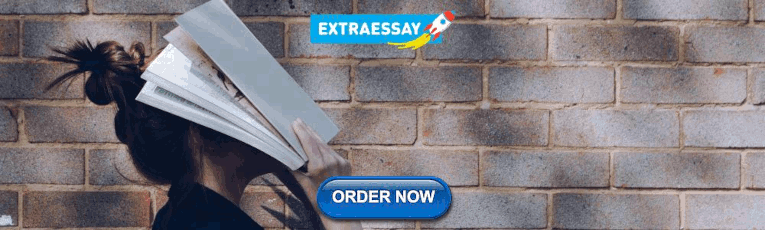
IMAGES
VIDEO
COMMENTS
Hi Sean, according to the three steps model (Dudley, Benuzillo and Carrico, 2004; Pardo and Román, 2013)., we can test hypothesis of mediator variable in three steps: (X -> Y; X -> M; X and M -> Y). Then, we must use the Sobel test to make sure that the effect is significant after using the mediator variable.
Published on March 1, 2021 by Pritha Bhandari . Revised on June 22, 2023. A mediating variable (or mediator) explains the process through which two variables are related, while a moderating variable (or moderator) affects the strength and direction of that relationship. Including mediators and moderators in your research helps you go beyond ...
The primary hypothesis of interest in a mediation analysis is to see whether the effect of the independent variable (intervention) on the outcome can be mediated by a change in the mediating variable. In a full mediation process, the effect is 100% mediated by the mediator, that is, in the presence of the mediator, the pathway connecting the ...
Mediation was initially hypothesized as a variable in the middle of a causal chain. Previously, most of the epidemiological reports focused on evaluating the simple association between E and Y as in Figure 1A. However, as in Figure 1B, it is shown that an E affects a mediator (M), which in turn affects an Y. The M fully mediates the effect from ...
Mediation, or an indirect effect, is said to occur when the causal effect of an in-dependent variable (X) on a dependent variable (Y) is transmittedby a mediator (M). In other words, X affects Y because X affects M, and M, in turn, affects Y. Mediation effect and indirect effect are often used interchangeably (as they
Abstract. This contribution in the "Best (but Oft-Forgotten) Practices" series considers mediation analysis. A mediator (sometimes referred to as an intermediate variable, surrogate endpoint, or intermediate endpoint) is a third variable that explains how or why ≥2 other variables relate in a putative causal pathway.
4.1 Introduction. Mediation analysis is a powerful statistical technique used to understand the relationship between two variables and how one variable influences the other through a mediator variable. To illustrate the importance of mediation analysis, consider the following caselet. Suppose a researcher is interested in examining the ...
Mediation Analysis. A mediational hypothesis is a kind of statistical test that assumes that the relationship between an independent and dependent variable is mediated by (or accounted for by) a third variable. This is what is known as a mediating variable. The goal of the mediating variable is to completely mediate the relationship so that ...
Mediation analysis is widely used to study whether the effect of an independent variable on an outcome is transmitted through a mediator. Bayesian methods have become increasingly popular for mediation analysis. However, limited research has been done on formal Bayesian hypothesis testing of mediation. Although hypothesis testing using Bayes factor for a single path is readily available, how ...
Using statistical mediation analysis, researchers can test causal hypotheses about how and why an independent and dependent variable are related (Hayes 2013; MacKinnon 2008).Many psychological theories involve causal assumptions linking a first construct (the cause) to a second construct (the effect) explaining the relation with intermediate constructs (mediators).
Introduction. Without respect to a given statistical model, mediation processes are framed in terms of intermediate variables between an independent variable and a dependent variable, with a minimum of three variables required in total: X, M, and Y, where X is the independent variable (IV), Y is the dependent variable (DV), and M is the (hypothesized) mediator variable that is supposed to ...
1. Testing Mediation with Regression Analysis. Mediation is a hypothesized causal chain in which one variable affects a second variable that, in turn, affects a third variable. The intervening variable, M, is the mediator. It "mediates" the relationship. between a predictor, X, and an outcome. Graphically, mediation can be depicted in the ...
Thus, a mediating or mediator variable is part of a causal chain. Hypotheses link variables, in causal assertions. An hypothesis may describe whether or not a relationship exists, possible causal direction of the relationship ("null" hypotheses are directionless), the mechanics (how) of the relationship; even the form of the relationship.
The mediator variable in mediation hypothesis can be caused by the outcome variable. This happens when the initial variable is a manipulated variable—then it cannot be caused either by the mediator or the outcome in mediation hypothesis. However, since both the mediator and the outcome variables are not manipulated, they may cause each other ...
1 What are Mediation and Moderation?. Mediation analysis tests a hypothetical causal chain where one variable X affects a second variable M and, in turn, that variable affects a third variable Y. Mediators describe the how or why of a (typically well-established) relationship between two other variables and are sometimes called intermediary variables since they often describe the process ...
Mediating variables are prominent in psychological theory and research. A mediating variable transmits the effect of an independent variable on a dependent variable. ... The results of the randomized study along with the predictions of different theories are used to provide evidence for a mediation hypothesis and suggest further studies to ...
the mediator variable M enters the model, the direct effect would be reduced since some of the ... The Hypotheses Required in Determining the Mediation Effects Hypothesis 1: X 1
The primary hypothesis of interest in a mediation analysis is to see whether the effect of the independent variable (intervention) on the outcome can be mediated by a change in the mediating variable. In a full mediation process, the effect is 100% mediated by the mediator, that is, in the presence of the mediator, the
1. Landau, S. and Everitt, B. S. (2004). A Handbook of Statistical analyses using SPSS. 2.Howitt, D. and Cramer, D. (2008). Introduction to SPSS. It appears that the design you are doing is a ...
A hypothesis has an independent variable and a dependent variable. Independent variables are controlled by the experiment. Dependent variables rely on the independent variables. The point of the experiment is to test the validity of what the hypothesis says about how the variables are related.
Correlation analysis of attachment characteristics, depression, and perfectionism among university students. Pearson correlation analysis was conducted using SPSS 26.0 on the three variables of attachment characteristics, depression and perfectionism, and the results indicated that all three variables were correlated, which was consistent with the premise of the mediating effect test.
X= the independent variable, Y= the dependent variable, and M= the mediating variable. The mediation model decomposes the total effect of X on Y (c), into two parts: the indirect effect of X on Y, quantified by ab (the product of a and b), and the direct effect of X on Y with the effect of the mediator removed, quantified by c′. c=ab+c′
Another important third variable is the confounding variable that causes both X and Y such that failure to adjust for the confounding variable will confound or lead to incorrect conclusions about the relation of X to Y.A confounding variable differs from a mediating variable in that the confounding variable is not in a causal sequence but the confounding variable is related to both X and Y.
This paper uses green innovation and environmental pollution as the mediating variables to construct a mediating effect model to investigate whether China's carbon emission trading policy can improve regional eco-efficiency by reducing regional environmental pollution and stimulating green innovation. This study is based on panel data from 30 provinces and municipalities directly under China ...
Research learning engagement is the basic element of master's students' innovation output, and the supervisor is the first responsible body for master's students' cultivation. Exploring the influence of the supervisor-postgraduate relationship on master's students' research learning engagement, with a focus on the mediating role of academic aspiration, is of great significance ...
Many theories in business research postulate a mediator ( M) that transmits the effect of a predictor variable ( X) to an outcome variable ( Y) in a causal sequence such that X causes M and M causes Y. In more general terms, a mediating variable explains the process by which one variable causes another. Theories across many disciplines focus on ...