- Privacy Policy
Buy Me a Coffee

Home » ANOVA (Analysis of variance) – Formulas, Types, and Examples
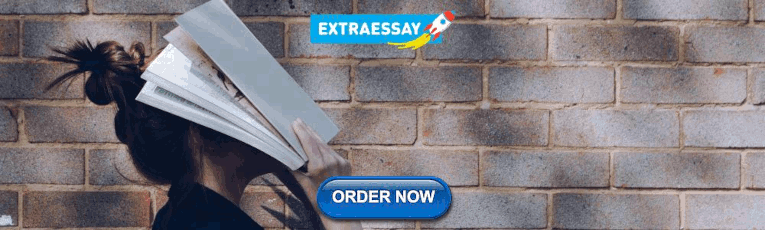
ANOVA (Analysis of variance) – Formulas, Types, and Examples
Table of Contents

Analysis of Variance (ANOVA)
Analysis of Variance (ANOVA) is a statistical method used to test differences between two or more means. It is similar to the t-test, but the t-test is generally used for comparing two means, while ANOVA is used when you have more than two means to compare.
ANOVA is based on comparing the variance (or variation) between the data samples to the variation within each particular sample. If the between-group variance is high and the within-group variance is low, this provides evidence that the means of the groups are significantly different.
ANOVA Terminology
When discussing ANOVA, there are several key terms to understand:
- Factor : This is another term for the independent variable in your analysis. In a one-way ANOVA, there is one factor, while in a two-way ANOVA, there are two factors.
- Levels : These are the different groups or categories within a factor. For example, if the factor is ‘diet’ the levels might be ‘low fat’, ‘medium fat’, and ‘high fat’.
- Response Variable : This is the dependent variable or the outcome that you are measuring.
- Within-group Variance : This is the variance or spread of scores within each level of your factor.
- Between-group Variance : This is the variance or spread of scores between the different levels of your factor.
- Grand Mean : This is the overall mean when you consider all the data together, regardless of the factor level.
- Treatment Sums of Squares (SS) : This represents the between-group variability. It is the sum of the squared differences between the group means and the grand mean.
- Error Sums of Squares (SS) : This represents the within-group variability. It’s the sum of the squared differences between each observation and its group mean.
- Total Sums of Squares (SS) : This is the sum of the Treatment SS and the Error SS. It represents the total variability in the data.
- Degrees of Freedom (df) : The degrees of freedom are the number of values that have the freedom to vary when computing a statistic. For example, if you have ‘n’ observations in one group, then the degrees of freedom for that group is ‘n-1’.
- Mean Square (MS) : Mean Square is the average squared deviation and is calculated by dividing the sum of squares by the corresponding degrees of freedom.
- F-Ratio : This is the test statistic for ANOVAs, and it’s the ratio of the between-group variance to the within-group variance. If the between-group variance is significantly larger than the within-group variance, the F-ratio will be large and likely significant.
- Null Hypothesis (H0) : This is the hypothesis that there is no difference between the group means.
- Alternative Hypothesis (H1) : This is the hypothesis that there is a difference between at least two of the group means.
- p-value : This is the probability of obtaining a test statistic as extreme as the one that was actually observed, assuming that the null hypothesis is true. If the p-value is less than the significance level (usually 0.05), then the null hypothesis is rejected in favor of the alternative hypothesis.
- Post-hoc tests : These are follow-up tests conducted after an ANOVA when the null hypothesis is rejected, to determine which specific groups’ means (levels) are different from each other. Examples include Tukey’s HSD, Scheffe, Bonferroni, among others.
Types of ANOVA
Types of ANOVA are as follows:
One-way (or one-factor) ANOVA
This is the simplest type of ANOVA, which involves one independent variable . For example, comparing the effect of different types of diet (vegetarian, pescatarian, omnivore) on cholesterol level.
Two-way (or two-factor) ANOVA
This involves two independent variables. This allows for testing the effect of each independent variable on the dependent variable , as well as testing if there’s an interaction effect between the independent variables on the dependent variable.
Repeated Measures ANOVA
This is used when the same subjects are measured multiple times under different conditions, or at different points in time. This type of ANOVA is often used in longitudinal studies.
Mixed Design ANOVA
This combines features of both between-subjects (independent groups) and within-subjects (repeated measures) designs. In this model, one factor is a between-subjects variable and the other is a within-subjects variable.
Multivariate Analysis of Variance (MANOVA)
This is used when there are two or more dependent variables. It tests whether changes in the independent variable(s) correspond to changes in the dependent variables.
Analysis of Covariance (ANCOVA)
This combines ANOVA and regression. ANCOVA tests whether certain factors have an effect on the outcome variable after removing the variance for which quantitative covariates (interval variables) account. This allows the comparison of one variable outcome between groups, while statistically controlling for the effect of other continuous variables that are not of primary interest.
Nested ANOVA
This model is used when the groups can be clustered into categories. For example, if you were comparing students’ performance from different classrooms and different schools, “classroom” could be nested within “school.”
ANOVA Formulas
ANOVA Formulas are as follows:
Sum of Squares Total (SST)
This represents the total variability in the data. It is the sum of the squared differences between each observation and the overall mean.
- yi represents each individual data point
- y_mean represents the grand mean (mean of all observations)
Sum of Squares Within (SSW)
This represents the variability within each group or factor level. It is the sum of the squared differences between each observation and its group mean.
- yij represents each individual data point within a group
- y_meani represents the mean of the ith group
Sum of Squares Between (SSB)
This represents the variability between the groups. It is the sum of the squared differences between the group means and the grand mean, multiplied by the number of observations in each group.
- ni represents the number of observations in each group
- y_mean represents the grand mean
Degrees of Freedom
The degrees of freedom are the number of values that have the freedom to vary when calculating a statistic.
For within groups (dfW):
For between groups (dfB):
For total (dfT):
- N represents the total number of observations
- k represents the number of groups
Mean Squares
Mean squares are the sum of squares divided by the respective degrees of freedom.
Mean Squares Between (MSB):
Mean Squares Within (MSW):
F-Statistic
The F-statistic is used to test whether the variability between the groups is significantly greater than the variability within the groups.
If the F-statistic is significantly higher than what would be expected by chance, we reject the null hypothesis that all group means are equal.
Examples of ANOVA
Examples 1:
Suppose a psychologist wants to test the effect of three different types of exercise (yoga, aerobic exercise, and weight training) on stress reduction. The dependent variable is the stress level, which can be measured using a stress rating scale.
Here are hypothetical stress ratings for a group of participants after they followed each of the exercise regimes for a period:
- Yoga: [3, 2, 2, 1, 2, 2, 3, 2, 1, 2]
- Aerobic Exercise: [2, 3, 3, 2, 3, 2, 3, 3, 2, 2]
- Weight Training: [4, 4, 5, 5, 4, 5, 4, 5, 4, 5]
The psychologist wants to determine if there is a statistically significant difference in stress levels between these different types of exercise.
To conduct the ANOVA:
1. State the hypotheses:
- Null Hypothesis (H0): There is no difference in mean stress levels between the three types of exercise.
- Alternative Hypothesis (H1): There is a difference in mean stress levels between at least two of the types of exercise.
2. Calculate the ANOVA statistics:
- Compute the Sum of Squares Between (SSB), Sum of Squares Within (SSW), and Sum of Squares Total (SST).
- Calculate the Degrees of Freedom (dfB, dfW, dfT).
- Calculate the Mean Squares Between (MSB) and Mean Squares Within (MSW).
- Compute the F-statistic (F = MSB / MSW).
3. Check the p-value associated with the calculated F-statistic.
- If the p-value is less than the chosen significance level (often 0.05), then we reject the null hypothesis in favor of the alternative hypothesis. This suggests there is a statistically significant difference in mean stress levels between the three exercise types.
4. Post-hoc tests
- If we reject the null hypothesis, we conduct a post-hoc test to determine which specific groups’ means (exercise types) are different from each other.
Examples 2:
Suppose an agricultural scientist wants to compare the yield of three varieties of wheat. The scientist randomly selects four fields for each variety and plants them. After harvest, the yield from each field is measured in bushels. Here are the hypothetical yields:
The scientist wants to know if the differences in yields are due to the different varieties or just random variation.
Here’s how to apply the one-way ANOVA to this situation:
- Null Hypothesis (H0): The means of the three populations are equal.
- Alternative Hypothesis (H1): At least one population mean is different.
- Calculate the Degrees of Freedom (dfB for between groups, dfW for within groups, dfT for total).
- If the p-value is less than the chosen significance level (often 0.05), then we reject the null hypothesis in favor of the alternative hypothesis. This would suggest there is a statistically significant difference in mean yields among the three varieties.
- If we reject the null hypothesis, we conduct a post-hoc test to determine which specific groups’ means (wheat varieties) are different from each other.
How to Conduct ANOVA
Conducting an Analysis of Variance (ANOVA) involves several steps. Here’s a general guideline on how to perform it:
- Null Hypothesis (H0): The means of all groups are equal.
- Alternative Hypothesis (H1): At least one group mean is different from the others.
- The significance level (often denoted as α) is usually set at 0.05. This implies that you are willing to accept a 5% chance that you are wrong in rejecting the null hypothesis.
- Data should be collected for each group under study. Make sure that the data meet the assumptions of an ANOVA: normality, independence, and homogeneity of variances.
- Calculate the Degrees of Freedom (df) for each sum of squares (dfB, dfW, dfT).
- Compute the Mean Squares Between (MSB) and Mean Squares Within (MSW) by dividing the sum of squares by the corresponding degrees of freedom.
- Compute the F-statistic as the ratio of MSB to MSW.
- Determine the critical F-value from the F-distribution table using dfB and dfW.
- If the calculated F-statistic is greater than the critical F-value, reject the null hypothesis.
- If the p-value associated with the calculated F-statistic is smaller than the significance level (0.05 typically), you reject the null hypothesis.
- If you rejected the null hypothesis, you can conduct post-hoc tests (like Tukey’s HSD) to determine which specific groups’ means (if you have more than two groups) are different from each other.
- Regardless of the result, report your findings in a clear, understandable manner. This typically includes reporting the test statistic, p-value, and whether the null hypothesis was rejected.
When to use ANOVA
ANOVA (Analysis of Variance) is used when you have three or more groups and you want to compare their means to see if they are significantly different from each other. It is a statistical method that is used in a variety of research scenarios. Here are some examples of when you might use ANOVA:
- Comparing Groups : If you want to compare the performance of more than two groups, for example, testing the effectiveness of different teaching methods on student performance.
- Evaluating Interactions : In a two-way or factorial ANOVA, you can test for an interaction effect. This means you are not only interested in the effect of each individual factor, but also whether the effect of one factor depends on the level of another factor.
- Repeated Measures : If you have measured the same subjects under different conditions or at different time points, you can use repeated measures ANOVA to compare the means of these repeated measures while accounting for the correlation between measures from the same subject.
- Experimental Designs : ANOVA is often used in experimental research designs when subjects are randomly assigned to different conditions and the goal is to compare the means of the conditions.
Here are the assumptions that must be met to use ANOVA:
- Normality : The data should be approximately normally distributed.
- Homogeneity of Variances : The variances of the groups you are comparing should be roughly equal. This assumption can be tested using Levene’s test or Bartlett’s test.
- Independence : The observations should be independent of each other. This assumption is met if the data is collected appropriately with no related groups (e.g., twins, matched pairs, repeated measures).
Applications of ANOVA
The Analysis of Variance (ANOVA) is a powerful statistical technique that is used widely across various fields and industries. Here are some of its key applications:
Agriculture
ANOVA is commonly used in agricultural research to compare the effectiveness of different types of fertilizers, crop varieties, or farming methods. For example, an agricultural researcher could use ANOVA to determine if there are significant differences in the yields of several varieties of wheat under the same conditions.
Manufacturing and Quality Control
ANOVA is used to determine if different manufacturing processes or machines produce different levels of product quality. For instance, an engineer might use it to test whether there are differences in the strength of a product based on the machine that produced it.
Marketing Research
Marketers often use ANOVA to test the effectiveness of different advertising strategies. For example, a marketer could use ANOVA to determine whether different marketing messages have a significant impact on consumer purchase intentions.
Healthcare and Medicine
In medical research, ANOVA can be used to compare the effectiveness of different treatments or drugs. For example, a medical researcher could use ANOVA to test whether there are significant differences in recovery times for patients who receive different types of therapy.
ANOVA is used in educational research to compare the effectiveness of different teaching methods or educational interventions. For example, an educator could use it to test whether students perform significantly differently when taught with different teaching methods.
Psychology and Social Sciences
Psychologists and social scientists use ANOVA to compare group means on various psychological and social variables. For example, a psychologist could use it to determine if there are significant differences in stress levels among individuals in different occupations.
Biology and Environmental Sciences
Biologists and environmental scientists use ANOVA to compare different biological and environmental conditions. For example, an environmental scientist could use it to determine if there are significant differences in the levels of a pollutant in different bodies of water.
Advantages of ANOVA
Here are some advantages of using ANOVA:
Comparing Multiple Groups: One of the key advantages of ANOVA is the ability to compare the means of three or more groups. This makes it more powerful and flexible than the t-test, which is limited to comparing only two groups.
Control of Type I Error: When comparing multiple groups, the chances of making a Type I error (false positive) increases. One of the strengths of ANOVA is that it controls the Type I error rate across all comparisons. This is in contrast to performing multiple pairwise t-tests which can inflate the Type I error rate.
Testing Interactions: In factorial ANOVA, you can test not only the main effect of each factor, but also the interaction effect between factors. This can provide valuable insights into how different factors or variables interact with each other.
Handling Continuous and Categorical Variables: ANOVA can handle both continuous and categorical variables . The dependent variable is continuous and the independent variables are categorical.
Robustness: ANOVA is considered robust to violations of normality assumption when group sizes are equal. This means that even if your data do not perfectly meet the normality assumption, you might still get valid results.
Provides Detailed Analysis: ANOVA provides a detailed breakdown of variances and interactions between variables which can be useful in understanding the underlying factors affecting the outcome.
Capability to Handle Complex Experimental Designs: Advanced types of ANOVA (like repeated measures ANOVA, MANOVA, etc.) can handle more complex experimental designs, including those where measurements are taken on the same subjects over time, or when you want to analyze multiple dependent variables at once.
Disadvantages of ANOVA
Some limitations or disadvantages that are important to consider:
Assumptions: ANOVA relies on several assumptions including normality (the data follows a normal distribution), independence (the observations are independent of each other), and homogeneity of variances (the variances of the groups are roughly equal). If these assumptions are violated, the results of the ANOVA may not be valid.
Sensitivity to Outliers: ANOVA can be sensitive to outliers. A single extreme value in one group can affect the sum of squares and consequently influence the F-statistic and the overall result of the test.
Dichotomous Variables: ANOVA is not suitable for dichotomous variables (variables that can take only two values, like yes/no or male/female). It is used to compare the means of groups for a continuous dependent variable.
Lack of Specificity: Although ANOVA can tell you that there is a significant difference between groups, it doesn’t tell you which specific groups are significantly different from each other. You need to carry out further post-hoc tests (like Tukey’s HSD or Bonferroni) for these pairwise comparisons.
Complexity with Multiple Factors: When dealing with multiple factors and interactions in factorial ANOVA, interpretation can become complex. The presence of interaction effects can make main effects difficult to interpret.
Requires Larger Sample Sizes: To detect an effect of a certain size, ANOVA generally requires larger sample sizes than a t-test.
Equal Group Sizes: While not always a strict requirement, ANOVA is most powerful and its assumptions are most likely to be met when groups are of equal or similar sizes.
About the author
Muhammad Hassan
Researcher, Academic Writer, Web developer
You may also like

Cluster Analysis – Types, Methods and Examples

Discriminant Analysis – Methods, Types and...

MANOVA (Multivariate Analysis of Variance) –...

Documentary Analysis – Methods, Applications and...

Graphical Methods – Types, Examples and Guide

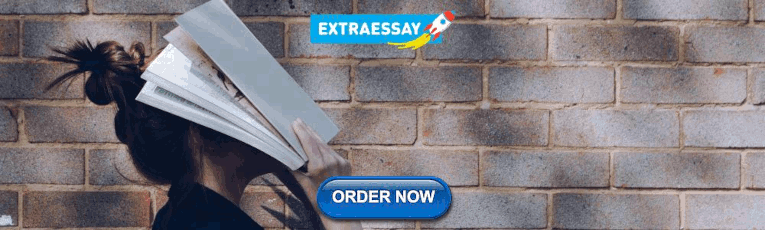
Substantive Framework – Types, Methods and...
If you're seeing this message, it means we're having trouble loading external resources on our website.
If you're behind a web filter, please make sure that the domains *.kastatic.org and *.kasandbox.org are unblocked.
To log in and use all the features of Khan Academy, please enable JavaScript in your browser.
Unit 16: Analysis of variance (ANOVA)
About this unit, analysis of variance (anova).
- ANOVA 1: Calculating SST (total sum of squares) (Opens a modal)
- ANOVA 2: Calculating SSW and SSB (total sum of squares within and between) (Opens a modal)
- ANOVA 3: Hypothesis test with F-statistic (Opens a modal)
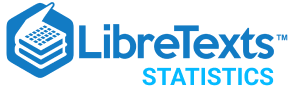
- school Campus Bookshelves
- menu_book Bookshelves
- perm_media Learning Objects
- login Login
- how_to_reg Request Instructor Account
- hub Instructor Commons
- Download Page (PDF)
- Download Full Book (PDF)
- Periodic Table
- Physics Constants
- Scientific Calculator
- Reference & Cite
- Tools expand_more
- Readability
selected template will load here
This action is not available.
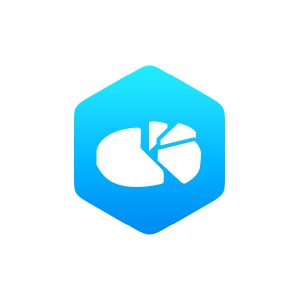
Lab 7: ANOVA
- Last updated
- Save as PDF
- Page ID 9047
Objectives:
- Understand how to perform ANOVA \(F\) test for comparing three or more population means.
- Understand how to use the aov() function in R to construct ANOVA tables.
Definitions:
- ANOVA (analysis of variance)
- treatment groups
- MSTR (mean sum of squares for treatment)
- MSE (mean sum of squares for error)
- \(F\)-distribution
- \(F\) statistic, \(F\) test
Introduction:
In Labs 2 and 6, we considered methods for testing claims about two population means, namely, permutation tests and \(t\) tests. In this lab, we consider a technique for testing claims about three or more population means known as analysis of variance ( ANOVA ). The ANOVA procedure compares the variation in the means of samples taken from the populations. The idea is to partition the variability in all the samples into the variability between each sample and the variability within each sample. If the population means are indeed equal, then the variability between and within each sample should be roughly the same. The ratio of the between and within variability provides a test statistic, and the classical approach, which we consider in this lab, uses a theoretical sampling distribution. In the next lab, we will develop a permutation test approach for performing ANOVA.
Activities:
Getting Organized: If you are already organized, and remember the basic protocol from previous labs, you can skip this section.
Navigate to your class folder structure. Within your "Labs" folder make a subfolder called "Lab7". Next, download the lab notebook .Rmd file for this lab from Blackboard and save it in your "Lab7" folder. There are no datasets used in this lab. You will be working with the Zombies.csv data set on this lab. You should download the data file into your "Lab7" folder from Blackboard.
Within RStudio, navigate to your "Lab7" folder via the file browser in the lower right pane and then click "More > Set as working directory". Get set to write your observations and R commands in an R Markdown file by opening the "lab7_notebook.Rmd" file in RStudio. Remember to add your name as the author in line 3 of the document. For this lab, enter all of your commands into code chunks in the lab notebook. You can still experiment with code in an R script, if you want. To set up an R Script in RStudio, in the upper left corner click “File > New File > R script”. A new tab should open up in the upper left pane of RStudio.
Notation: Before we see how to perform an ANOVA test in R/RStudio, let's formally set up the procedure, starting with defining the notation:
- \(G\) denotes the number of populations/samples
- \(n_i\) denotes the number of observations in the \(i^{\text{th}}\) sample, \(i=1,\ldots,G\)
- \(n=n_1+\cdots+n_G\) denotes the total number of observations
- \(X_{ij}\) denotes the \(j^{\text{th}}\) observation in the \(i^{\text{th}}\) sample, \(j=1,\ldots,n_i\)
- \(\bar{X}_{i\cdot}\) denotes the mean of the \(i^{\text{th}}\) sample
- \(\bar{X}_{\cdot\cdot}\) denotes the grand mean , i.e., the mean of all \(n\) observations in each sample
If we let \(\mu_i\) denote the mean of the \(i^{\text{th}}\) population, then we are testing the following hypotheses with the above sample data:
\(H_0: \mu_1=\mu_2=\cdots=\mu_G \quad\text{vs.}\quad H_A:\ \text{at least one}\ \mu_i\ \text{is different, i.e.,}\ \mu_i\neq\mu_j\ \text{for some}\ i\neq j\)
As discussed in the introduction above, we test these hypotheses by comparing the variability between the sample means to the variability within each sample. For the variability between the samples, we use the mean sum of squares for treatment (MSTR), which is given by
MSTR = \(\displaystyle \frac{1}{G-1}\sum^G_{i=1}n_i(\bar{X}_{i\cdot}-\bar{X}_{\cdot\cdot})^2.\)
For the variability within the samples, we use the mean sum of squares for error (MSE), which is given by
MSE = \(\displaystyle \frac{1}{n-G}\sum^G_{i=1} \sum^{n_i}_{j=1} (X_{ij} - \bar{X}_{i\cdot})^2.\)
Pause for Reflection #1:
Four chemical plants, producing the same products and owned by the same company, discharge liquid waste into streams in the vicinity of their locations. To monitor the extent of pollution created by the liquid waste and determine whether this differs from plant to plant, the company collected random samples of liquid waste from each plant, resulting in the following data.
- State the hypotheses we will test to determine if there is a difference in the mean weight of polluting effluents per gallon in the liquid waste discharged from the four plants. Be sure to define your notation.
- Identify what the values of \(G\) and \(n\) are, and for each sample identify the values of \(n_i\) and \(\bar{X}_{i\cdot}\) are.
- Finally, find the values of the grand mean \(\bar{X}_{\cdot\cdot}\) and the variability between the samples' MSTR.
_____________________________________________________
The ANOVA F Test: If \(H_0\) is true, i.e., the population means are all equal, then the variability between the samples should be roughly the same as the variability within the samples (assuming also that the populations have equal variance). If \(H_0\) is false, then the variability between the samples will be larger than the variability within the samples. Thus, we use the ratio of the between and within variability measures as the test statistic,
\(F = \displaystyle \frac{\text{MSTR}}{\text{MSE}},\)
which has a \(F\) distribution with \((G-1)\) and \((n-G)\) df. The observed test statistic based on the sample data obtained is denoted \(f\), and then its associated \(P\)-value is calculated using the \(F\) distribution as follows:
\(P\text{-value} = P(F\geq f)\)
Note that the \(P\)-value is given by the probability of obtaining a test statistic as large or larger than what was observed, i.e., the \(P\)-value for an ANOVA \(F\) test is always a right-tail probability. This is because "more extreme" in this context would be sample data that produced more between sample variability resulting in a larger ratio of MSTR to MSE.
Pause for Reflection #2:
Return to the pollution example and compute the observed \(F\) statistic using the value of MSTR you found in Reflection #1 and given that MSE = 0.03336. Then use the following code (with the corresponding values of f , G-1 , and n-G substituted in) to calculate the corresponding \(P\)-value:
Based on the \(P\)-value, do the data provide sufficient evidence to indicate a difference in the mean weight of polluting effluents per gallon in the liquid waste discharged from the four plants?
The ANOVA Table: As we can see, there are alot of calculations that go into performing ANOVA. The ANOVA table given below is a tool that summarizes and organizes these calculations in an easy to use format.
Notice how the ANOVA table is arranged:
- the last column with heading Pr(>F) gives the \(P\)-value, so it is easy to read off;
- the second to last column with heading F value gives the observed \(F\) statistic, which the \(P\)-value is based on.
- the first column provides labels for the source of variability, where Factor corresponds to the between samples variability and Error corresponds to the within sample variability;
- the second column gives the corresponding degrees of freedom within each row, note that the sum of the Factor and Error df equals the Total df;
- the third column with heading Sum Sq gives the sum of squares corresponding to each source, note that the sum of squares for the Factor and Error add up to the Total sum of squares (this is where the partitioning of the variability occurs that makes ANOVA possible);
- the fourth column with heading Mean Sq gives the mean sum of squares corresponding to each source, note that these are found by dividing the sum of squares in each row by the corresponding df.
Pause for Reflection #3:
Copy the following partial ANOVA table for the pollution example into your lab notebook and fill in the missing values, denoted by --- . Upload an image of your work into your lab notebook.
Performing the ANOVA F Test in R: Thankfully, there is a function in R that performs the extensive calculations needed to perform ANOVA, given by aov() . Calling the aov() function on the data performs the calculations, and then using the summary() function on the results constructs the ANOVA table. The following code demonstrates how this works in the pollution example. The first step is to format the data in R. Notice that an object Plant is created to store labels for the observed waste amounts so that we can sort the observations into the appropriate treatment groups corresponding to the four populations given by the four plants.
By running the above code for yourself (already provided in the Lab 7 Notebook), you can check your answers to Reflection #3.
Pause for Reflection #4:
In the above code, explain what the following line does:
In particular, what does the function rep() do?
Zombies: Let's look at another example to see how to use the aov() function given a data set. The Zombies.csv file contains data about the number of zombies killed ( killed ) and by what household weapon ( weapon ) for a sample of 31 apocalypse survivors. Load the data and view it:
Pause for Reflection #5:
Conduct some EDA:
- What are the mean and standard deviation of zombies killed across weapons (hint: the tapply() function will be useful)?
- How many observations of zombies killed are there for each of the weapons (hint: the table() function will be useful)?
- Create side-by-side boxplot to compare the distributions of zombies killed across weapons.
From the EDA, it sure looks as though there are differences in the number of zombies killed by each weapon, but are these differences due to sampling error, or do they represent real differences in zombie-killing effectiveness? To answer that question, we need to run an ANOVA test.
Pause for Reflection #6:
State the hypotheses being tested by the above ANOVA calculations. Report the \(P\)-value you find and state the conclusion.
Assumptions for the ANOVA F Test: In performing the ANOVA \(F\) test, the following assumptions are made:
- the samples are independent
- the populations are normally distributed
- the populations have equal variance
The independence assumption is critical, if the samples are related in some way then a different procedure is needed. Violations of the assumptions of normality and equal variances are less important.
The big problem with non-normality in \(t\) tests is the effect of skewness on one‐sided tests. But ANOVA tests are inherently two sided (we are testing for any differences between means, not differences in one direction) so non-normal distributions generally have little effect as long as the sample sizes are reasonably large.
If the sample sizes \(n_i\) are roughly equal, then unequal variances do not have a great impact, but if the population variances differ, then the actual sampling distribution of the \(F\) statistic could be very different from an \(F\) distribution. In particular, if there is a small sample from a population with large variance, then the \(F\) statistic can explode.
We will run simulations to explore the assumptions for ANOVA: in particular, how does "un-balancedness" (sample sizes not the same) and unequal population variances affect the outcome? We consider the hypotheses
\(H_0: \mu_A = \mu_B = \mu_C \quad\text{vs.}\quad H_A: \) at least one mean is different.
The code below simulates drawing three random samples from populations (called \(A, B, C\)) with the same mean (\(\mu=20\)) and standard deviation (\(\sigma=3\)) and then performs an ANOVA test. Using a significance level of 0.05, the object counter keeps track of how many times the null hypothesis is incorrectly rejected (false positive) and then corresponding proportion is computed.
Pause for Reflection #7:
What type of error is counter keeping track of? Is the proportion given by counter/N close to what you would expect the probability of making that type of error to be?
Pause for Reflection #8:
Alter the code so that the sample size from \(A\) is 10 ( n.A = 10 ) and redo the simulation. What happens to the proportion of times \(H_0\) is rejected?
Pause for Reflection #9:
Alter the code again by increasing the standard deviation of population \(A\) to 9 and trying samples of size 50 and 10 (keeping the other sample sizes to 50). What proportion of times do you reject the null hypothesis in each case?
Pause for Reflection #10:
Explore other scenarios: What if the population means are all different, but the population variances are the same? How do sample sizes affect the outcome? Try with all sample sizes the same and then unequal. Now try different variances and again, with balanced and unbalanced samples.
Record in your lab notebook what scenarios you tried and what results you found, i.e., how the proportion of times \(H_0\) was rejected is impacted.
- No category
STATS-BASILIO-Renz-Tyrone A-232-Assignment-Module-7-ANOVA-test (1)
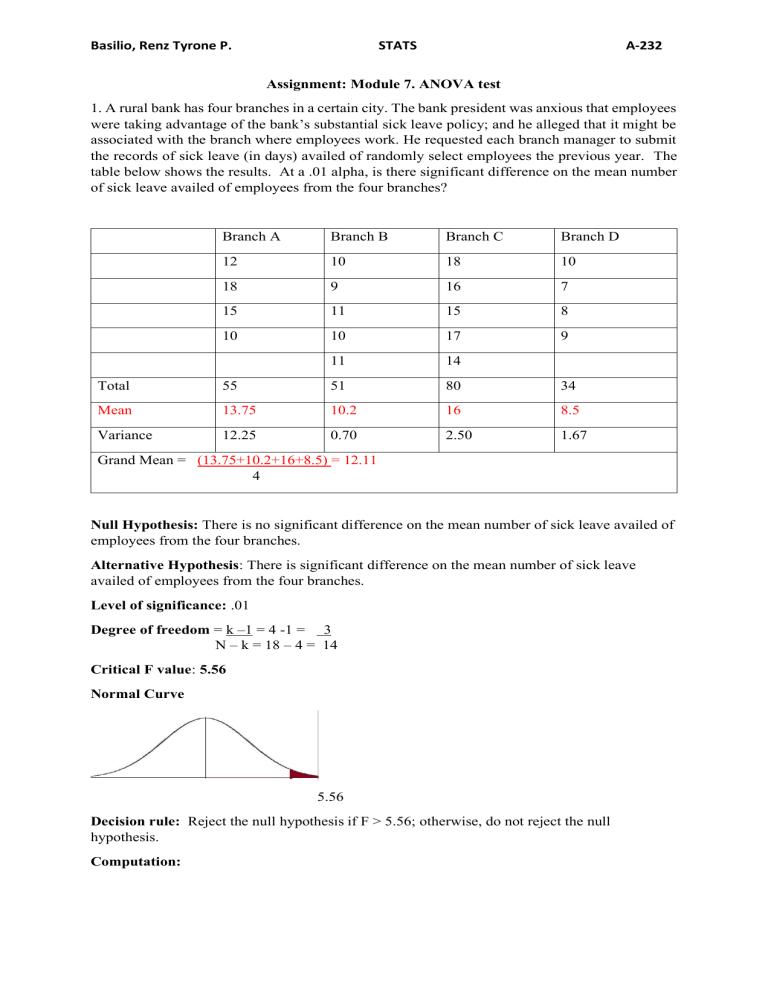
Related documents
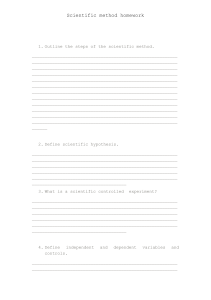
Add this document to collection(s)
You can add this document to your study collection(s)
Add this document to saved
You can add this document to your saved list
Suggest us how to improve StudyLib
(For complaints, use another form )
Input it if you want to receive answer

Provide details on what you need help with along with a budget and time limit. Questions are posted anonymously and can be made 100% private.

Studypool matches you to the best tutor to help you with your question. Our tutors are highly qualified and vetted.

Your matched tutor provides personalized help according to your question details. Payment is made only after you have completed your 1-on-1 session and are satisfied with your session.

- Homework Q&A
- Become a Tutor

All Subjects
Mathematics
Programming
Health & Medical
Engineering
Computer Science
Foreign Languages

Access over 20 million homework & study documents
Assignment module 7 anova test.

Sign up to view the full document!

24/7 Homework Help
Stuck on a homework question? Our verified tutors can answer all questions, from basic math to advanced rocket science !

Similar Documents

working on a homework question?

Studypool is powered by Microtutoring TM
Copyright © 2024. Studypool Inc.
Studypool is not sponsored or endorsed by any college or university.
Ongoing Conversations

Access over 20 million homework documents through the notebank

Get on-demand Q&A homework help from verified tutors

Read 1000s of rich book guides covering popular titles

Sign up with Google

Sign up with Facebook
Already have an account? Login
Login with Google
Login with Facebook
Don't have an account? Sign Up
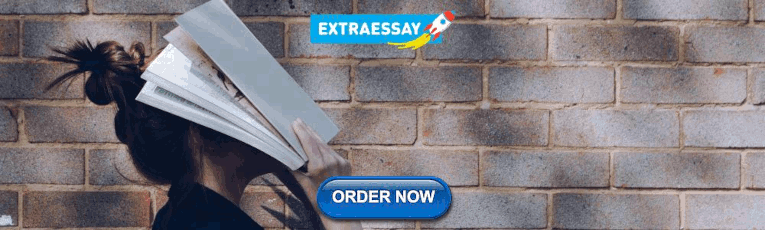
IMAGES
VIDEO
COMMENTS
One-way ANOVA (contʼd) ! Step 6: Write up your results. ! The null hypothesis stated that the means for Assignment 1, Assignment 2, and Assignment 3 are equal. A One-way ANOVA revealed a significant difference among the means for the 3 assignments, F (2, 38) = 9.50, p < .001, η2 = .33. Studentsʼ grades on
n ANOVA test is a way to find out if survey or experiment results are significant. AIn other words, they help you to figure out if you need to reject the null ... STATISTICS Module 7 Assignment ANOVA TEST. Course: BS accountancy. 999+ Documents. Students shared 13228 documents in this course. University: University of Cebu. Info More info. AI ...
Assignment: Module 7. ANOVA test. Read, understand, and analyze the problems carefully. Work on the problem and follow the step by step procedures in solving it - from the null hypothesis to the recommendation. Round off to two decimal places. A rural bank has four branches in a certain city. The bank president was anxious that employees were ...
Analysis of Variance (ANOVA) is a statistical method used to test differences between two or more means. It is similar to the t-test, but the t-test is generally used for comparing two means, while ANOVA is used when you have more than two means to compare. ANOVA is based on comparing the variance (or variation) between the data samples to the ...
Module 7 - ANOVA. Flashcards; Learn; Test; ... Evaluating the mean differences between two or more treatments. evaluates all mean differences simultaneously with one test - regardless of thenumber of means - and thereby avoids the problem of inflated experimentwise alpha. Factor. Independent (or quasi-independent) variable that designates the ...
Step 7: Based on steps 5 and 6, draw a conclusion about H0. If the F\calculated F \calculated from the data is larger than the Fα F α, then you are in the rejection region and you can reject the null hypothesis with (1 − α) ( 1 − α) level of confidence. Note that modern statistical software condenses steps 6 and 7 by providing a p p -value.
ANOVA 1: Calculating SST (total sum of squares) ANOVA 2: Calculating SSW and SSB (total sum of squares within and between) ANOVA 3: Hypothesis test with F-statistic. Analysis of variance, or ANOVA, is an approach to comparing data with multiple means across different groups, and allows us to see patterns and trends within complex and varied ...
SPSS Module 7 Assignment-Factorial ANOVA General Instructions: In this assignment, you will be asked to interpret the results from 1 Factorial ANOVA. As with previous assignments, the Aspelmeier and Pierce text does a wonderful job of explaining how actually run the test in Chapter 9. Follow their instructions on how to interpret the results ...
There are 4 modules in this course. This second course in statistical modeling will introduce students to the study of the analysis of variance (ANOVA), analysis of covariance (ANCOVA), and experimental design. ANOVA and ANCOVA, presented as a type of linear regression model, will provide the mathematical basis for designing experiments for ...
The ANOVA F Test: If \(H_0\) is true, i.e., the population means are all equal, then the variability between the samples should be roughly the same as the variability within the samples (assuming also that the populations have equal variance). If \(H_0\) is false, then the variability between the samples will be larger than the variability ...
Question: SPSS Module 7 Assignment-Factorial ANOVA General Instructions: In this assignment, you will be asked to interpret the results from 1 Factorial ANOVA. ... A One-Way ANOVA was used to test the hypothesis leading to the rejection of the null hypothesis, indicating a significant difference between the means, F(3, 96)=4.61, p<.05. Tukey ...
MODULE 7 ANOVA branch branch branch branch 12 10 18 10 18 16 15 11 15 10 10 17 11 14 total 55 51 80 34 mean 13.75 10.2 16 variance 12.25 0.70 2.50 1.67 grand. ... Agbuya- Busstat Module 8 CHI- Square TEST; Statistics Module 7 - ANOVA; Assignment Module 1 - Types of Variables; Download. 0 0. Was this document helpful? 0 0. Save Share. Premium.
Module 7. ANOVA TEST - Free download as PDF File (.pdf), Text File (.txt) or read online for free.
A-231 Assignment: Module 7. ANOVA test Read, understand, and analyze the problems carefully. Work on the problem and follow the step by step procedures in solving it - from the null hypothesis to the recommendation. Round off to two decimal places.
Assignment: Module 7. ANOVA test Read, understand, and analyze the problems carefully. Work on the problem and follow the step by step procedures in solving it - from the null hypothesis to the recommendation. Round off to two decimal places. 1. A rural bank has four branches in a certain city.
Zuniga, Khryzelle G. B-212 Assignment: Module 7. ANOVA test Read, understand, and analyze the problems carefully. Work on the problem and follow the step-by-step procedures in solving it - from the null hypothesis to the recommendation. Round off to two decimal places.
Module 7. Analysis of Variance. Analysis of Variance (ANOVA) Compare and determine significant differences between the means of three or more independent groups. An extension of t-test since it analyzes the differences between the means of more than two independent groups.
Basilio, Renz Tyrone P. STATS A-232 Assignment: Module 7. ANOVA test 1. A rural bank has four branches in a certain city. The bank president was anxious that employees were taking advantage of the bank's substantial sick leave policy; and he alleged that it might be associated with the branch where employees work.
1. A rural bank has four branches in a certain city. The bank president was anxious that employeeswere taking advantage of the bank's substantial sick leave policy; and he alleged that it might be
Assignment-Module-7 - anova test. anova test. Course. Accountancy (A201) 145 Documents. Students shared 145 documents in this course. University City College of Angeles . Academic year: 2019/2020. Uploaded by: bella tan. City College of Angeles . 0 followers. 0 Uploads 0 upvotes. Follow. Recommended for you. 2.
Question: SPSS Module 7 Assignment-Factorial ANOVA General Instructions: In this assignment, you will be asked to interpret the results from 1 previous assignments, the Aspelmeier and Chapter 9. Follow their instructions on how to interpret the results for this assignment. Factorial ANOVA. As with Pierce text does a wonderful job of explaining ...
week 7 assignment u07a1 anova complete the following problems within this word document. (do not submit other files.) show your work for problem sets that ... The results of each test in the following table are similar to the way in which the data were given in their article. Indep enden t Variab les. Life Satisfaction. M SD F p. Sex 0. Men 3 0 ...