Journal of Pharmaceutical Analysis
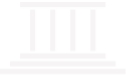
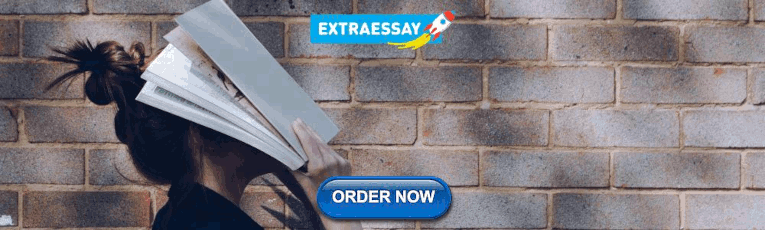
Subject Area and Category
- Analytical Chemistry
- Electrochemistry
- Spectroscopy
- Drug Discovery
- Pharmaceutical Science
Publication type
Information.
How to publish in this journal
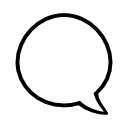
The set of journals have been ranked according to their SJR and divided into four equal groups, four quartiles. Q1 (green) comprises the quarter of the journals with the highest values, Q2 (yellow) the second highest values, Q3 (orange) the third highest values and Q4 (red) the lowest values.
The SJR is a size-independent prestige indicator that ranks journals by their 'average prestige per article'. It is based on the idea that 'all citations are not created equal'. SJR is a measure of scientific influence of journals that accounts for both the number of citations received by a journal and the importance or prestige of the journals where such citations come from It measures the scientific influence of the average article in a journal, it expresses how central to the global scientific discussion an average article of the journal is.
Evolution of the number of published documents. All types of documents are considered, including citable and non citable documents.
This indicator counts the number of citations received by documents from a journal and divides them by the total number of documents published in that journal. The chart shows the evolution of the average number of times documents published in a journal in the past two, three and four years have been cited in the current year. The two years line is equivalent to journal impact factor ™ (Thomson Reuters) metric.
Evolution of the total number of citations and journal's self-citations received by a journal's published documents during the three previous years. Journal Self-citation is defined as the number of citation from a journal citing article to articles published by the same journal.
Evolution of the number of total citation per document and external citation per document (i.e. journal self-citations removed) received by a journal's published documents during the three previous years. External citations are calculated by subtracting the number of self-citations from the total number of citations received by the journal’s documents.
International Collaboration accounts for the articles that have been produced by researchers from several countries. The chart shows the ratio of a journal's documents signed by researchers from more than one country; that is including more than one country address.
Not every article in a journal is considered primary research and therefore "citable", this chart shows the ratio of a journal's articles including substantial research (research articles, conference papers and reviews) in three year windows vs. those documents other than research articles, reviews and conference papers.
Ratio of a journal's items, grouped in three years windows, that have been cited at least once vs. those not cited during the following year.
Leave a comment
Name * Required
Email (will not be published) * Required
* Required Cancel
The users of Scimago Journal & Country Rank have the possibility to dialogue through comments linked to a specific journal. The purpose is to have a forum in which general doubts about the processes of publication in the journal, experiences and other issues derived from the publication of papers are resolved. For topics on particular articles, maintain the dialogue through the usual channels with your editor.
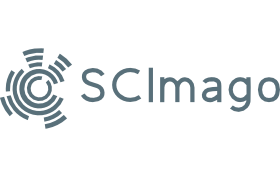
Follow us on @ScimagoJR Scimago Lab , Copyright 2007-2024. Data Source: Scopus®

Cookie settings
Cookie Policy
Legal Notice
Privacy Policy
Identifiers
Linking ISSN (ISSN-L): 2320-0812
URL http://rroij.org/jpa/index.php/jpa/index
Google https://www.google.com/search?q=ISSN+%222320-0812%22
Bing https://www.bing.com/search?q=ISSN+%222320-0812%22
Yahoo https://search.yahoo.com/search?p=ISSN%20%222320-0812%22
National Library of India http://nationallibraryopac.nvli.in/cgi-bin/koha/opac-search.pl?advsearch=1&idx=ns&q=2320-0812&weight_search=1&do=Search&sort_by=relevance
Resource information
Title proper: Research and reviews: journal of pharmaceutical analysis.
Country: India
Medium: Online
Record information
Last modification date: 09/05/2023
Type of record: Confirmed
ISSN Center responsible of the record: ISSN National Centre for India
downloads requested
Discover all the features of the complete ISSN records
Display mode x.
Labelled view
MARC21 view
UNIMARC view

- Paper Archives
- Journal Indexing
- Research Conference
- Add Journal
Searching By
- Search More ...

Description
Last modified: 2013-03-28 02:07:53
- No Archives
Advertisement

- Impact Factor
Top Publication Journals
- Business, Economics & Management
- Chemical & Material Sciences
- Engineering & Computer Science
- Health & Medical Sci
- Humanities, Literature & Arts
- Life Sciences & Earth Sciences
- Physics & Mathematics
- Social Sciences
- 218498 Articles
- 25996 Journals
Journal Impact Factor Report 2021
Submit journal for impact factor evaluation, submit your journal for indexing, journal impact factor report 2020, journal impact factor report 2018, journal impact factor list 2014 ( now online ), getting your journal indexed, 2012 impact factor list.
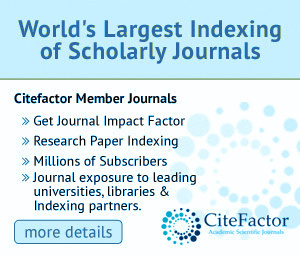
Research & Reviews: Journal of Pharmaceutical Analysis

URL: http://rroij.com/jpa/index.php/jpa
Keywords: Pharmaceutical Analysis
ISSN: 234723402320-0812
EISSN: 2320-0812
Publisher: Research and Reviews
Country: India
Author Ratings

Share With Us
Journal areas list.
- Agriculture
- Biochemistry
- Biotechnology
- Business and Management
- Computer Science
- Environmental Sciences
Information
- Terms & Conditions
- Privacy Policy
Important Links
- Publisher Guidelines
- Journal Indexing
- Paper Indexing
- Impact Factor List
Online Journal Management System
- ✓ Online Submission System
- ✓ Editorial Control Panel
- ✓ Online Review System
- ✓ Online Publishing Tools
Research & Reviews: Journal of Pharmaceutical Analysis
Please submit your article using the form below. Note that all the fields are mandatory. Please also include the full abstract that will help the reviewers to rapidly decide if your article enters their domain of expertise. Also select one or several topics below to help the editors to assign your paper to the right reviewers. After submission you will get a password that will allow you to access the system and eventually modify the information associated with this article. Contact us immediately if you don't receive this confirmation within a few hours.
Submit Manuscript
Author details:, manuscript details.
*Note: If you want to upload more files please click Add More button
Share this page

Current Pharmaceutical Analysis
Limited Time Complementary OA Offer Publish Research Articles With No Charges
Impact Factor : 0.6
Indexed in: Scopus, SCI Expanded, JCR... View all
Volume 20 , Issues 9, 2024
This journal supports open access
Submission for General Articles
Submit to thematic issues.
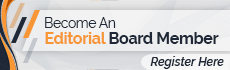
Thematic Issue Issue: {[{issue.issue_title}]}
{[{issue.about_issue}]}
No Text Found
- Submit Abstracts
- Submit Manuscripts Online
- Thematic Issue Proposal
- Animated Abstract Submission

- About Journal
- Editorial Board
- Journal Insight
- Current Issue
- Volumes /Issues
- Author Guidelines
- Graphical Abstracts
- Fabricating and Stating False Information
- Research Misconduct
- Post Publication Discussions and Corrections
- Publishing Ethics and Rectitude
- Increase Visibility of Your Article
- Archiving Policies
- Peer Review Workflow
- Order Your Article Before Print
- Promote Your Article
- Manuscript Transfer Facility
- Editorial Policies
- Allegations from Whistleblowers
- Announcements
- Forthcoming Thematic Issues
- Guest Editor Guidelines
- Editorial Management
- Ethical Guidelines for New Editors
- Reviewer Guidelines
- Abstract Ahead of Print 0
- Article(s) in Press 7
- Free Online Copy
- Most Cited Articles
- Most Accessed Articles
- Highlighted Article
- Most Popular Articles
- Editor's Choice
- Thematic Issues
- Open Access Articles
- Open Access Funding
- Library Recommendation
- Trial Requests
- Advertise With Us
- Meet the Executive Guest Editor(s)
- Brand Ambassador
- Author's Comment & Reviews
- New Journals 2023
- New Journals 2024
- Alert Subscription
Thank you for visiting nature.com. You are using a browser version with limited support for CSS. To obtain the best experience, we recommend you use a more up to date browser (or turn off compatibility mode in Internet Explorer). In the meantime, to ensure continued support, we are displaying the site without styles and JavaScript.
- View all journals
- Explore content
- About the journal
- Publish with us
- Sign up for alerts
Latest science news, discoveries and analysis
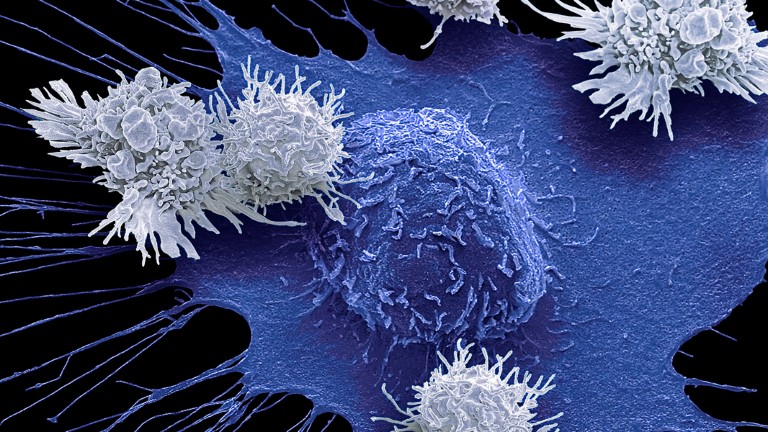
Do cutting-edge CAR-T-cell therapies cause cancer? What the data say
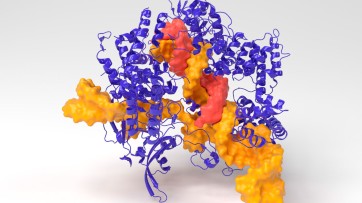
‘ChatGPT for CRISPR’ creates new gene-editing tools
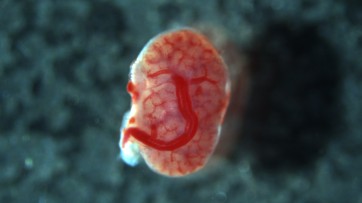
First fetus-to-fetus transplant demonstrated in rats
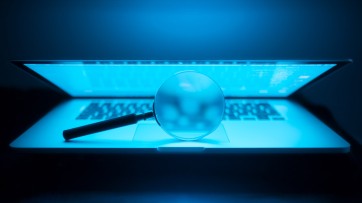
How reliable is this research? Tool flags papers discussed on PubPeer
Epic blazes threaten arctic permafrost. can fire-fighters save it, what china’s mission to collect rocks from the far side could reveal about the moon, bird flu virus has been spreading in us cows for months, rna reveals, superconductivity hunt gets boost from china's $220 million physics 'playground', why doing science is difficult in india today yamini aiyar.
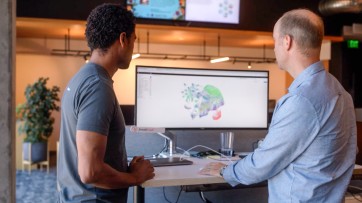
85 million cells — and counting — at your fingertips
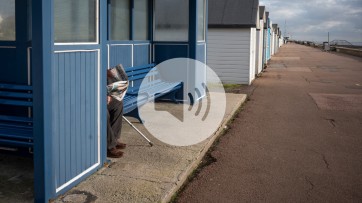
Audio long read: Why loneliness is bad for your health
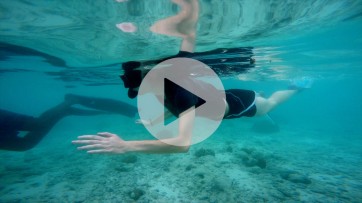
Should the Maldives be creating new land?
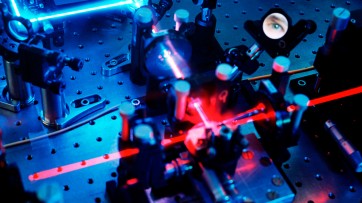
‘Shut up and calculate’: how Einstein lost the battle to explain quantum reality
Could a rare mutation that causes dwarfism also slow ageing, future of humanity institute shuts: what’s next for ‘deep future’ research, bird flu in us cows: is the milk supply safe, nato is boosting ai and climate research as scientific diplomacy remains on ice.
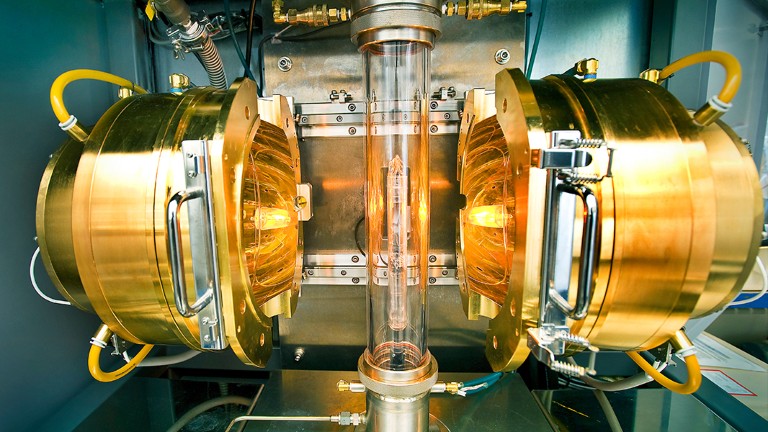
Retractions are part of science, but misconduct isn’t — lessons from a superconductivity lab
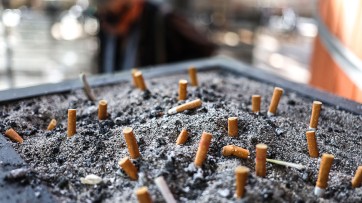
Any plan to make smoking obsolete is the right step
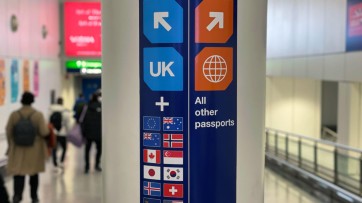
Citizenship privilege harms science
Ecologists: don’t lose touch with the joy of fieldwork chris mantegna, european ruling linking climate change to human rights could be a game changer — here’s how charlotte e. blattner, current issue.
The Maldives is racing to create new land. Why are so many people concerned?
Surprise hybrid origins of a butterfly species, stripped-envelope supernova light curves argue for central engine activity, optical clocks at sea, research analysis.
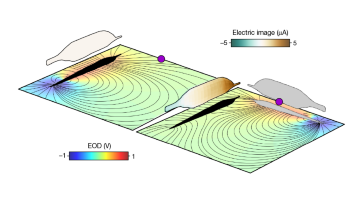
Elephant-nose fish ‘see’ farther by electric sensing when in groups
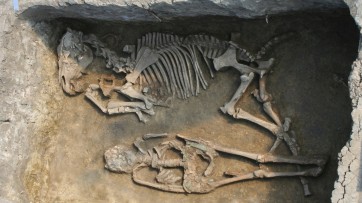
Ancient DNA traces family lines and political shifts in the Avar empire
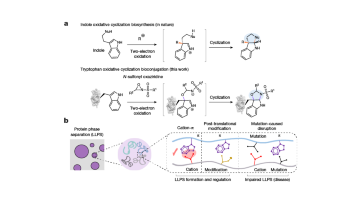
A chemical method for selective labelling of the key amino acid tryptophan
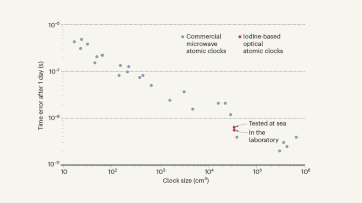
Robust optical clocks promise stable timing in a portable package
How to stop students cramming for exams send them to sea, targeting rna opens therapeutic avenues for timothy syndrome, bioengineered ‘mini-colons’ shed light on cancer progression, galaxy found napping in the primordial universe.
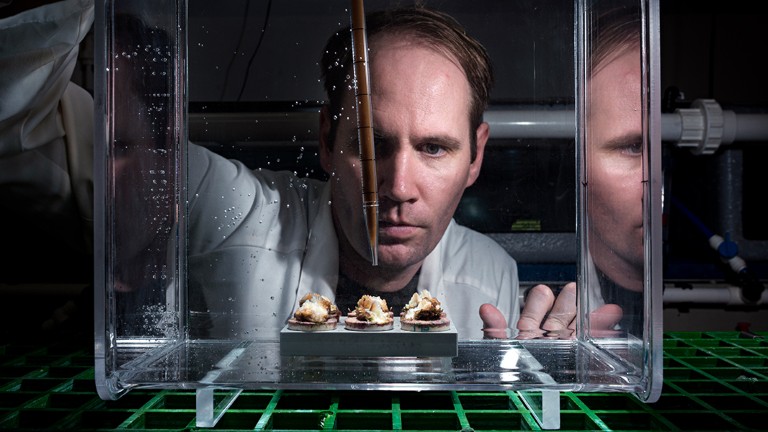
I strive to make the Great Barrier Reef more resilient to heat stress
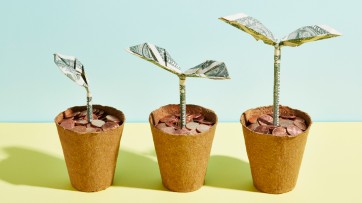
Scientists urged to collect royalties from the ‘magic money tree’
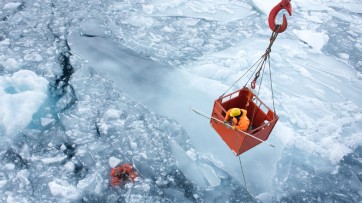
Breaking ice, and helicopter drops: winning photos of working scientists
Want to make a difference try working at an environmental non-profit organization, how ground glass might save crops from drought on a caribbean island, books & culture.
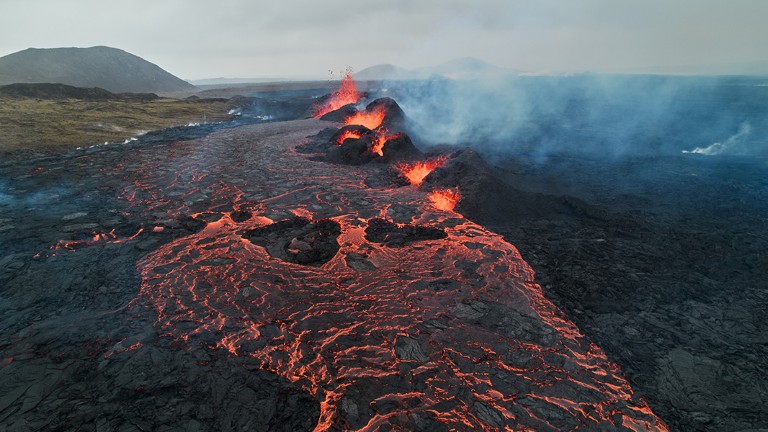
How volcanoes shaped our planet — and why we need to be ready for the next big eruption
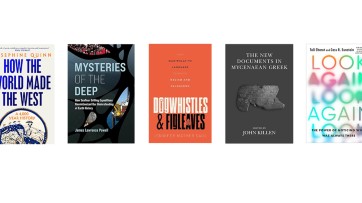
Dogwhistles, drilling and the roots of Western civilization: Books in brief
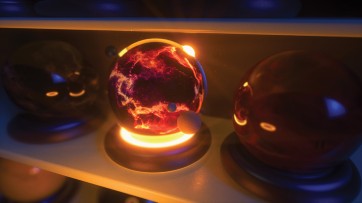
Cosmic rentals
Las borinqueñas remembers the forgotten puerto rican women who tested the first pill, dad always mows on summer saturday mornings, nature podcast.

Latest videos
Nature briefing.
An essential round-up of science news, opinion and analysis, delivered to your inbox every weekday.

Quick links
- Explore articles by subject
- Guide to authors
- Editorial policies
This paper is in the following e-collection/theme issue:
Published on 29.4.2024 in Vol 26 (2024)
The Applications of Artificial Intelligence for Assessing Fall Risk: Systematic Review
Authors of this article:

- Ana González-Castro 1 , PT, MSc ;
- Raquel Leirós-Rodríguez 2 , PT, PhD ;
- Camino Prada-García 3 , MD, PhD ;
- José Alberto Benítez-Andrades 4 , PhD
1 Nursing and Physical Therapy Department, Universidad de León, Ponferrada, Spain
2 SALBIS Research Group, Nursing and Physical Therapy Department, Universidad de León, Ponferrada, Spain
3 Department of Preventive Medicine and Public Health, Universidad de Valladolid, Valladolid, Spain
4 SALBIS Research Group, Department of Electric, Systems and Automatics Engineering, Universidad de León, León, Spain
Corresponding Author:
Ana González-Castro, PT, MSc
Nursing and Physical Therapy Department
Universidad de León
Astorga Ave
Ponferrada, 24401
Phone: 34 987442000
Email: [email protected]
Background: Falls and their consequences are a serious public health problem worldwide. Each year, 37.3 million falls requiring medical attention occur. Therefore, the analysis of fall risk is of great importance for prevention. Artificial intelligence (AI) represents an innovative tool for creating predictive statistical models of fall risk through data analysis.
Objective: The aim of this review was to analyze the available evidence on the applications of AI in the analysis of data related to postural control and fall risk.
Methods: A literature search was conducted in 6 databases with the following inclusion criteria: the articles had to be published within the last 5 years (from 2018 to 2024), they had to apply some method of AI, AI analyses had to be applied to data from samples consisting of humans, and the analyzed sample had to consist of individuals with independent walking with or without the assistance of external orthopedic devices.
Results: We obtained a total of 3858 articles, of which 22 were finally selected. Data extraction for subsequent analysis varied in the different studies: 82% (18/22) of them extracted data through tests or functional assessments, and the remaining 18% (4/22) of them extracted through existing medical records. Different AI techniques were used throughout the articles. All the research included in the review obtained accuracy values of >70% in the predictive models obtained through AI.
Conclusions: The use of AI proves to be a valuable tool for creating predictive models of fall risk. The use of this tool could have a significant socioeconomic impact as it enables the development of low-cost predictive models with a high level of accuracy.
Trial Registration: PROSPERO CRD42023443277; https://tinyurl.com/4sb72ssv
Introduction
According to alarming figures reported by the World Health Organization in 2021, falls cause 37.3 million injuries annually that require medical attention and result in 684,000 deaths [ 1 ]. These figures indicate a significant impact of falls on the health care system and on society, both directly and indirectly [ 2 , 3 ].
Life expectancy has progressively increased over the years, leading to an aging population [ 4 ]. By 2050, it is estimated that 16% of the population will be >65 years of age. In this group, the incidence of falls has steadily risen, becoming the leading cause of accidental injury and death (accounting for 55.8% of such deaths, according to some research) [ 5 , 6 ]. It is estimated that 30% of this population falls at least once a year, negatively impacting their physical and psychological well-being [ 7 , 8 ].
Physically, falls are often associated with severe complications that can lead to extended hospitalizations [ 9 ]. These hospitalizations are usually due to serious injuries, often cranioencephalic trauma, fractures, or soft tissue injuries [ 10 , 11 ]. Psychologically, falls among the older adult population tend to result in self-imposed limitations due to the fear of falling again [ 10 , 12 ]. These limitations lead to social isolation as individuals avoid participating in activities or even individual mobility [ 13 ]. Consequently, falls can lead to psychological conditions such as anxiety and depression [ 14 , 15 ]. Numerous research studies on the risk of falls are currently underway, with ongoing investigations into various innovations and intervention ideas [ 16 - 19 ]. These studies encompass the identification of fall risk factors [ 20 , 21 ], strategies for prevention [ 22 , 23 ], and the outcomes following rehabilitation [ 23 , 24 ].
In the health care field, artificial intelligence (AI) is characterized by data management and processing, offering new possibilities to the health care paradigm [ 24 ]. Some applications of AI in the health care domain include assessing tumor interaction processes [ 25 ], serving as a tool for image-based diagnostics [ 26 , 27 ], participating in virus detection [ 28 ], and, most importantly, as a statistical and predictive method [ 29 - 32 ].
Several publications have combined AI techniques to address health care issues [ 33 - 35 ]. Within the field of predictive models, it is important to understand certain differentiations. In AI, we have machine learning and deep learning [ 36 - 38 ]. Machine learning encompasses a set of techniques applied to data and can be done in a supervised or unsupervised manner [ 39 , 40 ]. On the other hand, deep learning is typically used to work with larger data sets compared to machine learning, and its computational cost is higher [ 41 , 42 ].
Some examples of AI techniques include the gradient boosting machine [ 43 ], learning method, and the long short-term memory (LSTM) [ 44 ] and the convolutional neural network (CNN) [ 45 ], all of them are deep learning methods.
For all the reasons mentioned in the preceding section, it was considered necessary to conduct a systematic review to analyze the scientific evidence of AI applications in the analysis of data related to postural control and the risk of falls.
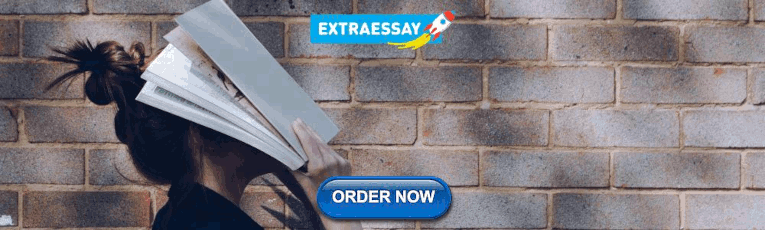
Data Sources and Searches
This systematic review and meta-analysis were prospectively registered on PROSPERO (ID CRD42023443277) and followed the Meta-Analyses of Observational Studies in Epidemiology checklist [ 46 ] and the recommendations of the Cochrane Collaboration [ 47 ].
The search was conducted in January 2024 on the following databases: PubMed, Scopus, ScienceDirect, Web of Science, CINAHL, and Cochrane Library. The Medical Subject Headings (MeSH) terms used for the search included machine learning , artificial intelligent , accidental falls , rehabilitation , and physical therapy specialty . The terms “predictive model” and “algorithms” were also used. These terms were combined using the Boolean operators AND and OR ( Textbox 1 ).
- (“machine learning”[MeSH Terms] OR “artificial intelligent”[MeSH Terms]) AND “accidental falls”[MeSH Terms]
- (“machine learning”[MeSH Terms] OR “artificial intelligent”) AND (“rehabilitation”[MeSH Terms] OR “physical therapy specialty”[MeSH Terms])
- “accidental falls” [Title/Abstract] AND “algorithms” [Title/Abstract]
- “accidental falls”[Title/Abstract] AND “predictive model” [Title/Abstract]
- TITLE-ABS-KEY (“machine learning” OR “artificial intelligent”) AND TITLE-ABS-KEY (“accidental falls”)
- TITLE-ABS-KEY (“machine learning” OR “artificial intelligent”) AND TITLE-ABS-KEY (“rehabilitation” OR “physical therapy specialty”)
- TITLE-ABS-KEY (“accidental falls” AND “algorithms”)
- TITLE-ABS-KEY (“accidental falls” AND “predictive model”)
ScienceDirect
- Title, abstract, keywords: (“machine learning” OR “artificial intelligent”) AND “accidental falls”
- Title, abstract, keywords: (“machine learning” OR “artificial intelligent”) AND (“rehabilitation” OR “physical therapy specialty”)
- Title, abstract, keywords: (“accidental falls” AND “algorithms”)
- Title, abstract, keywords: (“accidental falls” AND “predictive model”)
Web of Science
- TS=(“machine learning” OR “artificial intelligent”) AND TS=“accidental falls”
- TS=(“machine learning” OR “artificial intelligent”) AND TS= (“rehabilitation” OR “physical therapy specialty”)
- AB= (“accidental falls” AND “algorithms”)
- AB= (“accidental falls” AND “predictive model”)
- (MH “machine learning” OR MH “artificial intelligent”) AND MH “accidental falls”
- (MH “machine learning” OR MH “artificial intelligent”) AND (MH “rehabilitation” OR MH “physical therapy specialty”)
- (AB “accidental falls”) AND (AB “algorithms”)
- (AB “accidental falls”) AND (AB “predictive model”)
Cochrane Library
- (“machine learning” OR “artificial intelligent”) in Title Abstract Keyword AND “accidental falls” in Title Abstract Keyword
- (“machine learning” OR “artificial intelligent”) in Title Abstract Keyword AND (“rehabilitation” OR “physical therapy specialty”) in Title Abstract Keyword
- “accidental falls” in Title Abstract Keyword AND “algorithms” in Title Abstract Keyword
- “accidental falls” in Title Abstract Keyword AND “predictive model” in Title Abstract Keyword
Study Selection
After removing duplicates, 2 reviewers (AGC and RLR) independently screened articles for eligibility. In the case of disagreement, a third reviewer (JABA) finally decided whether the study should be included or not. We calculated the κ coefficient and percentage agreement scores to assess reliability before any consensus and estimated the interrater reliability using κ. Interrater reliability was estimated using κ>0.7 indicating a high level of agreement between the reviewers, κ of 0.5 to 0.7 indicating a moderate level of agreement, and κ<0.5 indicating a low level of agreement [ 48 ].
For the selection of results, the inclusion criteria were established as follows: (1) articles should have been published in the last 5 years (from 2018 to the present); (2) they must apply some AI method; (3) AI analyses should be applied to data from samples of humans; and (4) the sample analyzed should consist of people with independent walking, with or without the use of external orthopedic devices.
After screening the data, extracting, obtaining, and screening the titles and abstracts for inclusion criteria, the selected abstracts were obtained in full texts. Titles and abstracts lacking sufficient information regarding inclusion criteria were also obtained as full texts. Full-text articles were selected in case of compliance with inclusion criteria by the 2 reviewers using a data extraction form.
Data Extraction and Quality Assessment
The 2 reviewers mentioned independently extracting data from the included studies using a customized data extraction table in Excel (Microsoft Corporation). In case of disagreement, both reviewers debated until an agreement was reached.
The data extracted from the included articles for further analysis were: demographic information (title, authors, journal, and year), characteristics of the sample (age, inclusion and exclusion criteria, and number of participants), study-specific parameters (study type, AI techniques applied, and data analyzed), and the results obtained. Tables were used to describe both the studies’ characteristics and the extracted data.
Assessment of Risk of Bias
The methodological quality of the selected articles was evaluated using the Critical Review Form for Quantitative Studies [ 49 ]. The ROBINS-E (Risk of Bias in Nonrandomized Studies of Exposures) tool was used to evaluate the risk of bias [ 50 ].
Characteristics of the Selected Studies
A total of 3858 articles were initially retrieved, with 1563 duplicates removed. From the remaining 2295 articles, 2271 were excluded based on the initial selection criteria, leaving 24 articles for the subsequent analysis. In this second analysis, 2 articles were removed as they were systematic reviews, and 22 articles were finally selected [ 51 - 72 ] ( Figure 1 ). After the first reading of all candidate full texts, the kappa score for inclusion of the results of reviewers 1 and 2 was 0.98, indicating a very high level of agreement.
The methodological quality of the 22 analyzed studies (Table S1 in Multimedia Appendix 1 [ 51 , 52 , 54 , 56 , 58 , 59 , 61 , 63 , 64 , 69 , 70 , 72 ]) ranged from 11 points in 2 (9.1%) studies [ 52 , 65 ] to 16 points in 7 (32%) studies [ 53 , 54 , 56 , 63 , 69 - 71 ].

Study Characteristics and Risk of Bias
All the selected articles were cross-sectional observational studies ( Table 1 ).
In total, 34 characteristics affecting the risk of falls were extracted and classified into high fall-risk and low fall-risk groups with the largest sample sizes significantly differing from the rest. Studies based on data collected from various health care systems had larger sample sizes, ranging from 22,515 to 265,225 participants [ 60 , 65 , 67 ]. In contrast, studies that applied some form of evaluation test had sample sizes ranging from 8 participants [ 56 ] to 746 participants [ 55 ].
It is worth noting the various studies conducted by Dubois et al [ 54 , 72 ], whose publications on fall risk and machine learning started in 2018 and progressed until 2021. A total of 9.1% (2/22) of the articles by this author were included in the final selection [ 54 , 72 ]. Both articles used samples with the same characteristics, even though the first one was composed of 43 participants [ 54 ] and the last one had 30 participants [ 72 ]. All 86.4% (19/22) of the articles used samples of individuals aged ≥65 years [ 51 - 60 , 62 - 65 , 68 - 72 ]. In the remaining 13.6% (3/22) of the articles, the ages ranged between 16 and 62 years [ 61 , 66 , 67 ].
Althobaiti et al [ 61 ] used a sample of participants between the ages of 19 and 35 years for their research, where these participants had to reproduce examples of falls for subsequent analysis. In 2022, Ladios-Martin et al [ 67 ] extracted medical data from participants aged >16 years for their research. Finally, in 2023, the study by Maray et al [ 66 ] used 3 types of samples, with ages ranging from 21 to 62 years. Among the 22 selected articles, only 1 (4.5%) of them did not describe the characteristics of its sample [ 52 ].
Finally, regarding the sex of the samples, 13.6% (3/22) of the articles specified in the characteristics of their samples that only female individuals were included among their participants [ 53 , 59 , 70 ].
a AI: artificial intelligence.
b ML: machine learning.
c nd: none described.
d ADL: activities of daily living.
e TUG: Timed Up and Go.
f BBS: Berg Balance Scale.
g ASM: associative skill memories.
h CNN: convolutional neural network.
i FP: fall prevention.
j IMU: inertial measurement unit.
k AUROC: area under the receiver operating characteristic curve.
l AUPR: area under the precision-recall curve.
m MFS: Morse Fall Scale.
n XGB: extreme gradient boosting.
o MCT: motor control test.
p GBM: gradient boosting machine.
q RF: random forest.
r LOOCV: leave-one-out cross-validation.
s LSTM: long short-term memory.
Applied Assessment Procedures
All articles initially analyzed the characteristics of their samples to subsequently create a predictive model of the risk of falls. However, they did not all follow the same evaluation process.
Regarding the applied assessment procedures, 3 main options stood out: studies with tests or assessments accompanied by sensors or accelerometers [ 51 - 57 , 59 , 61 - 63 , 66 , 70 - 72 ], studies with tests or assessments accompanied by cameras [ 68 , 69 ], or studies based on medical records [ 58 , 60 , 65 , 67 ] ( Figure 2 ). Guillan et al [ 64 ] performed a physical and functional evaluation of the participants. In their study, they evaluated parameters such as walking speed, stride frequency and length, and the minimum space between the toes. Afterward, they asked them to record the fall events they had during the past 2 years in a personal diary.

In total, 22.7% (5/22) of the studies used the Timed Up and Go test [ 53 , 54 , 69 , 71 , 72 ]. In 18.2% (4/22) of them, the participants performed the test while wearing a sensor to collect data [ 53 , 54 , 71 , 72 ]. In 1 (4.5%) study, the test was recorded with a camera for later analysis [ 69 ]. Another commonly used method in studies was to ask participants to perform everyday tasks or activities of daily living while a sensor collected data for subsequent analysis. Specifically, 18.2% (4/22) of the studies used this method to gather data [ 51 , 56 , 61 , 62 ].
A total of 22.7% (5/22) of the studies asked participants to simulate falls and nonfalls while a sensor collected data [ 52 , 61 - 63 , 66 ]. In this way, the data obtained were used to create the predictive model of falls. As for the tests used, Eichler et al [ 68 ] asked participants to perform the Berg Balance Scale while a camera recorded their performance.
Finally, other authors created their own battery of tests for data extraction [ 55 , 59 , 64 , 70 ]. Gillain et al [ 64 ] used gait records to analyze speed, stride length, frequency, symmetry, regularity, and foot separation. Hu et al [ 59 ] asked their participants to perform normal walking, the postural reflexive response test, and the motor control test. In the study by Noh et al [ 55 ], gait tests were conducted, involving walking 20 m at different speeds. Finally, Greene et al [ 70 ] created a 12-question questionnaire and asked their participants to maintain balance while holding a mobile phone in their hand.
AI Techniques
The selected articles used various techniques within AI. They all had the same objective in applying these techniques, which was to achieve a predictive and classification model for the risk of falls [ 51 - 72 ].
In chronological order, in 2018, Nait Aicha et al [ 51 ] compared single-task learning models with multitask learning, obtaining better evaluation results through multitask learning. In the same year, Dubois et al [ 54 ] applied AI techniques that analyzed multiple parameters to classify the risk of falls in their sample. Qiu et al [ 53 ], also in the same year, used 6 machine learning models (logistic regression, naïve Bayes, decision tree, RF, boosted tree, and support vector machine) in their research.
In contrast, in 2019, Ferrete et al [ 52 ] compared the applicability of 2 different deep learning models: the classifier based on associative skill memories and a CNN classifier. In the same year, after confirming the applicability of AI as a predictive method for the risk of falls, various authors investigated through methods such as the RF to identify factors that can predict and quantify the risk of falls [ 63 , 65 ].
Among the selected articles, 5 (22.7%) were published in 2020 [ 58 - 62 ]. The research conducted by Tunca et al [ 62 ] compared the applicability of deep learning LSTM networks with traditional machine learning applied to the risk of falls. Hu et al [ 59 ] first used cross-validation, where algorithms were trained randomly, and then used the gradient boosting machine algorithm to classify participants as high or low risk. Ye et al [ 60 ] and Hsu et al [ 58 ] both used the extreme gradient boosting (XGBoost) algorithm based on machine learning to create their predictive model. In the same year, Althobaiti et al [ 61 ] trained machine learning models for their research.
In 2021, Lockhart et al [ 57 ] started using 3 deep learning techniques simultaneously with the same goal as before: to create a predictive model for the risk of falls. Specifically, they used the RF, RF with feature engineering, and RF with feature engineering and linear and nonlinear variables. Noh et al [ 55 ], again in the same year, used the XGBoost algorithm, while Roshdibenam et al [ 71 ], on the other hand, used the CNN algorithm for each location of the wearable sensors used in their research. Various machine learning techniques were used for classifying the risk of falls and for balance loss events in the research by Hauth et al [ 56 ]. Dubois et al [ 72 ] used the following algorithms: decision tree, adaptive boosting, neural net, naïve Bayes, k-nearest neighbors, linear support vector machine, radial basis function support vector machine, RF, and quadratic discriminant analysis. Hauth et al [ 56 ], on the other hand, used regularized logistic regression and bidirectional LSTM networks. In the research conducted by Greene et al [ 70 ], AI was used, but the specific procedure that they followed is not described.
Tang et al [ 69 ] published their research with innovation up to that point. In their study, they used a smart gait analyzer with the help of deep learning techniques to assess the diagnostic accuracy of fall risk through vision. Months later, in August 2022, Ladios-Martin et al [ 67 ] published their research, in which they compared 2 deep learning models to achieve the best results in terms of specificity and sensitivity in detecting fall risk. The first model used the Bayesian Point Machine algorithm with a fall prevention variable, and the second one did not use the variable. They obtained better results when using that variable, a mitigating factor defined as a set of care interventions carried out by professionals to prevent the patient from experiencing a fall during hospitalization. Particularly controversial, as its exclusion could obscure the model’s performance. Eichler et al [ 68 ], on the other hand, used machine learning–based classifier training and later tested the performance of RFs in score predictions.
Finally, in January 2023, Maray et al [ 66 ] published their research, linking the previously mentioned terms (AI and fall risk) with 3 wearable devices that are commonly used today. They collected data through these devices and applied transfer learning to generalize the model across heterogeneous devices.
The results of the 22 articles provided promising data, and all of them agreed on the feasibility of applying various AI techniques as a method for predicting and classifying the risk of falls. Specifically, the accuracy values obtained in the studies exceed 70%. Noh et al [ 55 ] achieved the “lowest” accuracy among the studies conducted, with a 70% accuracy rate. Ribeiro et al [ 52 ] obtained an accuracy of 92.7% when using CNN to differentiate between normal gait and fall events. Hsu et al [ 58 ] further demonstrated that the XGBoost model is more sensitive than the Morse Fall Scale. Similarly, in their comparative study, Nait Aicha et al [ 51 ] also showed that a predictive model created from accelerometer data with AI is comparable to conventional models for assessing the risk of falls. More specifically, Dubois et al [ 54 ] concluded that using 1 gait-related parameter (excluding velocity) in combination with another parameter related to seated position allowed for the correct classification of individuals according to their risk of falls.
Principal Findings
The aim of this research was to analyze the scientific evidence regarding the applications of AI in the analysis of data related to postural control and the risk of falls. On the basis of the analysis of results, it can be asserted that the following risk factors were identified in the analyzed studies: age [ 65 ], daily habits [ 65 ], clinical diagnoses [ 65 ], environmental and hygiene factors [ 65 ], sex [ 64 ], stride length [ 55 , 72 ], gait speed [ 55 ], and posture [ 55 ]. This aligns with other research that also identifies sex [ 73 , 74 ], age [ 73 ], and gait speed [ 75 ].
On the other hand, the “fear of falling” has been identified in various studies as a risk factor and a predictor of falls [ 73 , 76 ], but it was not identified in any of the studies included in this review.
As for the characteristics of the analyzed samples, only 9.1% (2/22) of the articles used a sample composed exclusively of women [ 53 , 59 ], and no article used a sample composed exclusively of men. This fact is incongruent with reality, as women have a longer life expectancy than men, and therefore, the number of women aged >65 years is greater than the number of men of the same age [ 77 ]. Furthermore, women experience more falls than men [ 78 ]. The connection between menopause and its consequences, including osteopenia, suggests a higher risk of falls among older women than among men of the same age [ 79 , 80 ].
Within the realm of analysis tools, the most frequently used devices to analyze participants were accelerometers [ 51 - 57 , 59 , 61 - 63 , 66 , 70 - 72 ]. However, only 36.4% (8/22) of the studies provided all the information regarding the characteristics of these devices [ 51 , 53 , 59 , 61 , 63 , 66 , 70 , 72 ]. On the other hand, 18.2% (4/22) of the studies used the term “inertial measurement unit” as the sole description of the devices used [ 55 - 57 , 71 ].
The fact that most of the analyzed procedures involved the use of inertial sensors reflects the current widespread use of these devices for postural control analysis. These sensors, in general (and triaxial accelerometers in particular), have demonstrated great diagnostic capacity for balance [ 81 ]. In addition, they exhibit good sensitivity and reliability, combined with their portability and low economic cost [ 82 ]. Another advantage of triaxial accelerometers is their versatility in both adult and pediatric populations [ 83 - 86 ], although the studies included in this review did not address the pediatric population.
The remaining studies extracted data from cameras [ 68 , 69 ], medical records [ 58 , 60 , 65 , 67 ], and other functional and clinical tests [ 59 , 64 , 70 ]. Regarding the AI techniques used, out of the 18.2% (4/22) of articles that used deep learning techniques [ 52 , 57 , 62 , 71 ], only 4.5% (1/22) did not provide a description of the sample characteristics [ 52 ]. In this case, the authors focused on the AI landscape, while the rest of the articles strike a balance between AI and health sciences.
Regarding the validity of the generated models, only 40.9% (9/22) of the articles assessed this characteristic [ 52 , 53 , 55 , 61 - 64 , 68 , 69 ]. The authors of these 9 (N=22, 40.9%) articles evaluated the validity of the models through accuracy. All the results obtained reflected accuracies exceeding 70%, with Ribeiro et al [ 52 ] achieving a notable accuracy of 92.7% and 100%. Specifically, they obtained a 92.7% accuracy through the CNN model for distinguishing normal gait, the prefall condition, and the falling situation, considering the step before the fall, and 100% when not considering it [ 52 ].
The positive results of sensitivity and specificity can only be compared between the studies of Qiu et al [ 53 ] and Gillain et al [ 64 ], as they were the only ones to take them into account, and in both investigations, they were very high. Similarly, in the case of the F 1 -score, only Althobaiti et al [ 61 ] examined this validity measure. This measure is the result of combining precision and recall into a single figure, and the outcome obtained by these researchers was promising.
Despite these differences, the 22 studies obtained promising results in the health care field [ 51 - 72 ]. Specifically, their outcomes highlight the potential of AI integration into clinical settings. However, further research is necessary to explore how health care professionals can effectively use these predictive models. Consequently, future research should focus on studying the application and integration of the already-developed models. In this context, fall prevention plans could be implemented for the target populations identified by the predictive models. This approach would allow for a retrospective analysis to determine if the combination of predictive models with prevention programs effectively reduces the prevalence of falls in the population.
Limitations
Regarding limitations, the articles showed significant variation in the sample sizes selected. Moreover, even in the study with the largest sample size (with 265,225 participants [ 60 ]), the amount of data analyzed was relatively small. In addition, several of the databases used were not generated specifically for the published research but rather derived from existing medical records [ 58 , 60 , 65 , 67 ]. This could explain the significant variability in the variables analyzed across different studies.
Despite the limitations, this research has strengths, such as being the first systematic review on the use of AI as a tool to analyze postural control and the risk of falls. Furthermore, a total of 6 databases were used for the literature search, and a comprehensive article selection process was carried out by 3 researchers. Finally, only cross-sectional observational studies were selected, and they shared the same objective.
Conclusions
The use of AI in the analysis of data related to postural control and the risk of falls proves to be a valuable tool for creating predictive models of fall risk. It has been identified that most AI studies analyze accelerometer data from sensors, with triaxial accelerometers being the most frequently used.
For future research, it would be beneficial to provide more detailed descriptions of the measurement procedures and the AI techniques used. In addition, exploring larger databases could lead to the development of more robust models.
Conflicts of Interest
None declared.
Quality scores of reviewed studies (Critical Review Form for Quantitative Studies tool results).
PRISMA (Preferred Reporting Items for Systematic Reviews and Meta-Analyses) checklist.
- Step safely: strategies for preventing and managing falls across the life-course. World Health Organization. 2021. URL: https://www.who.int/publications/i/item/978924002191-4 [accessed 2024-04-02]
- Keall MD, Pierse N, Howden-Chapman P, Guria J, Cunningham CW, Baker MG. Cost-benefit analysis of fall injuries prevented by a programme of home modifications: a cluster randomised controlled trial. Inj Prev. Feb 2017;23(1):22-26. [ CrossRef ] [ Medline ]
- Almada M, Brochado P, Portela D, Midão L, Costa E. Prevalence of falls and associated factors among community-dwelling older adults: a cross-sectional study. J Frailty Aging. 2021;10(1):10-16. [ CrossRef ] [ Medline ]
- Menéndez-González L, Izaguirre-Riesgo A, Tranche-Iparraguirre S, Montero-Rodríguez Á, Orts-Cortés MI. [Prevalence and associated factors of frailty in adults over 70 years in the community]. Aten Primaria. Dec 2021;53(10):102128. [ FREE Full text ] [ CrossRef ] [ Medline ]
- Guirguis-Blake JM, Michael YL, Perdue LA, Coppola EL, Beil TL. Interventions to prevent falls in older adults: updated evidence report and systematic review for the US preventive services task force. JAMA. Apr 24, 2018;319(16):1705-1716. [ CrossRef ] [ Medline ]
- Pereira CB, Kanashiro AM. Falls in older adults: a practical approach. Arq Neuropsiquiatr. May 2022;80(5 Suppl 1):313-323. [ FREE Full text ] [ CrossRef ] [ Medline ]
- Byun M, Kim J, Kim M. Physical and psychological factors affecting falls in older patients with arthritis. Int J Environ Res Public Health. Feb 09, 2020;17(3):1098. [ FREE Full text ] [ CrossRef ] [ Medline ]
- Goh HT, Nadarajah M, Hamzah NB, Varadan P, Tan MP. Falls and fear of falling after stroke: a case-control study. PM R. Dec 04, 2016;8(12):1173-1180. [ CrossRef ] [ Medline ]
- Alanazi FK, Lapkin S, Molloy L, Sim J. The impact of safety culture, quality of care, missed care and nurse staffing on patient falls: a multisource association study. J Clin Nurs. Oct 12, 2023;32(19-20):7260-7272. [ CrossRef ] [ Medline ]
- Hossain A, Lall R, Ji C, Bruce J, Underwood M, Lamb SE. Comparison of different statistical models for the analysis of fracture events: findings from the Prevention of Falls Injury Trial (PreFIT). BMC Med Res Methodol. Oct 02, 2023;23(1):216. [ FREE Full text ] [ CrossRef ] [ Medline ]
- Williams CT, Whyman J, Loewenthal J, Chahal K. Managing geriatric patients with falls and fractures. Orthop Clin North Am. Jul 2023;54(3S):e1-12. [ CrossRef ] [ Medline ]
- Gadhvi C, Bean D, Rice D. A systematic review of fear of falling and related constructs after hip fracture: prevalence, measurement, associations with physical function, and interventions. BMC Geriatr. Jun 23, 2023;23(1):385. [ FREE Full text ] [ CrossRef ] [ Medline ]
- Lohman MC, Fallahi A, Mishio Bawa E, Wei J, Merchant AT. Social mediators of the association between depression and falls among older adults. J Aging Health. Aug 12, 2023;35(7-8):593-603. [ CrossRef ] [ Medline ]
- Smith AD, Silva AO, Rodrigues RA, Moreira MA, Nogueira JD, Tura LF. Assessment of risk of falls in elderly living at home. Rev Lat Am Enfermagem. Apr 06, 2017;25:e2754. [ FREE Full text ] [ CrossRef ] [ Medline ]
- Koh V, Matchar DB, Chan A. Physical strength and mental health mediate the association between pain and falls (recurrent and/or injurious) among community-dwelling older adults in Singapore. Arch Gerontol Geriatr. Sep 2023;112:105015. [ CrossRef ] [ Medline ]
- Soh SE, Morgan PE, Hopmans R, Barker AL, Ackerman IN. The feasibility and acceptability of a falls prevention e-learning program for physiotherapists. Physiother Theory Pract. Mar 18, 2023;39(3):631-640. [ CrossRef ] [ Medline ]
- Morat T, Snyders M, Kroeber P, De Luca A, Squeri V, Hochheim M, et al. Evaluation of a novel technology-supported fall prevention intervention - study protocol of a multi-centre randomised controlled trial in older adults at increased risk of falls. BMC Geriatr. Feb 18, 2023;23(1):103. [ FREE Full text ] [ CrossRef ] [ Medline ]
- You T, Koren Y, Butts WJ, Moraes CA, Yeh GY, Wayne PM, et al. Pilot studies of recruitment and feasibility of remote Tai Chi in racially diverse older adults with multisite pain. Contemp Clin Trials. May 2023;128:107164. [ CrossRef ] [ Medline ]
- Aldana-Benítez D, Caicedo-Pareja MJ, Sánchez DP, Ordoñez-Mora LT. Dance as a neurorehabilitation strategy: a systematic review. J Bodyw Mov Ther. Jul 2023;35:348-363. [ FREE Full text ] [ CrossRef ] [ Medline ]
- Jawad A, Baattaiah BA, Alharbi MD, Chevidikunnan MF, Khan F. Factors contributing to falls in people with multiple sclerosis: the exploration of the moderation and mediation effects. Mult Scler Relat Disord. Aug 2023;76:104838. [ CrossRef ] [ Medline ]
- Warren C, Rizo E, Decker E, Hasse A. A comprehensive analysis of risk factors associated with inpatient falls. J Patient Saf. Oct 01, 2023;19(6):396-402. [ CrossRef ] [ Medline ]
- Gross M, Roigk P, Schoene D, Ritter Y, Pauly P, Becker C, et al. Bundesinitiative Sturzprävention. [Update of the recommendations of the federal falls prevention initiative-identification and prevention of the risk of falling in older people living at home]. Z Gerontol Geriatr. Oct 11, 2023;56(6):448-457. [ CrossRef ] [ Medline ]
- Li S, Li Y, Liang Q, Yang WJ, Zi R, Wu X, et al. Effects of tele-exercise rehabilitation intervention on women at high risk of osteoporotic fractures: study protocol for a randomised controlled trial. BMJ Open. Nov 07, 2022;12(11):e064328. [ FREE Full text ] [ CrossRef ] [ Medline ]
- Jiang F, Jiang Y, Zhi H, Dong Y, Li H, Ma S, et al. Artificial intelligence in healthcare: past, present and future. Stroke Vasc Neurol. Dec 2017;2(4):230-243. [ FREE Full text ] [ CrossRef ] [ Medline ]
- Ye Y, Wu X, Wang H, Ye H, Zhao K, Yao S, et al. Artificial intelligence-assisted analysis for tumor-immune interaction within the invasive margin of colorectal cancer. Ann Med. Dec 2023;55(1):2215541. [ FREE Full text ] [ CrossRef ] [ Medline ]
- Kuwahara T, Hara K, Mizuno N, Haba S, Okuno N, Fukui T, et al. Current status of artificial intelligence analysis for the treatment of pancreaticobiliary diseases using endoscopic ultrasonography and endoscopic retrograde cholangiopancreatography. DEN Open. Apr 30, 2024;4(1):e267. [ FREE Full text ] [ CrossRef ] [ Medline ]
- Yokote A, Umeno J, Kawasaki K, Fujioka S, Fuyuno Y, Matsuno Y, et al. Small bowel capsule endoscopy examination and open access database with artificial intelligence: the SEE-artificial intelligence project. DEN Open. Apr 22, 2024;4(1):e258. [ FREE Full text ] [ CrossRef ] [ Medline ]
- Ramalingam M, Jaisankar A, Cheng L, Krishnan S, Lan L, Hassan A, et al. Impact of nanotechnology on conventional and artificial intelligence-based biosensing strategies for the detection of viruses. Discov Nano. Dec 01, 2023;18(1):58. [ FREE Full text ] [ CrossRef ] [ Medline ]
- Yerukala Sathipati S, Tsai MJ, Shukla SK, Ho SY. Artificial intelligence-driven pan-cancer analysis reveals miRNA signatures for cancer stage prediction. HGG Adv. Jul 13, 2023;4(3):100190. [ FREE Full text ] [ CrossRef ] [ Medline ]
- Liu J, Dan W, Liu X, Zhong X, Chen C, He Q, et al. Development and validation of predictive model based on deep learning method for classification of dyslipidemia in Chinese medicine. Health Inf Sci Syst. Dec 06, 2023;11(1):21. [ FREE Full text ] [ CrossRef ] [ Medline ]
- Carou-Senra P, Ong JJ, Castro BM, Seoane-Viaño I, Rodríguez-Pombo L, Cabalar P, et al. Predicting pharmaceutical inkjet printing outcomes using machine learning. Int J Pharm X. Dec 2023;5:100181. [ FREE Full text ] [ CrossRef ] [ Medline ]
- Li X, Zhu Y, Zhao W, Shi R, Wang Z, Pan H, et al. Machine learning algorithm to predict the in-hospital mortality in critically ill patients with chronic kidney disease. Ren Fail. Dec 2023;45(1):2212790. [ FREE Full text ] [ CrossRef ] [ Medline ]
- Bonnin M, Müller-Fouarge F, Estienne T, Bekadar S, Pouchy C, Ait Si Selmi T. Artificial intelligence radiographic analysis tool for total knee arthroplasty. J Arthroplasty. Jul 2023;38(7 Suppl 2):S199-207.e2. [ CrossRef ] [ Medline ]
- Kao DP. Intelligent artificial intelligence: present considerations and future implications of machine learning applied to electrocardiogram interpretation. Circ Cardiovasc Qual Outcomes. Sep 2019;12(9):e006021. [ FREE Full text ] [ CrossRef ] [ Medline ]
- van der Stigchel B, van den Bosch K, van Diggelen J, Haselager P. Intelligent decision support in medical triage: are people robust to biased advice? J Public Health (Oxf). Aug 28, 2023;45(3):689-696. [ FREE Full text ] [ CrossRef ] [ Medline ]
- Jakhar D, Kaur I. Artificial intelligence, machine learning and deep learning: definitions and differences. Clin Exp Dermatol. Jan 09, 2020;45(1):131-132. [ CrossRef ] [ Medline ]
- Ghosh M, Thirugnanam A. Introduction to artificial intelligence. In: Srinivasa KG, Siddesh GM, Sekhar SR, editors. Artificial Intelligence for Information Management: A Healthcare Perspective. Cham, Switzerland. Springer; 2021;88-44.
- Taulli T. Artificial Intelligence Basics: A Non-Technical Introduction. Berkeley, CA. Apress Berkeley; 2019.
- Patil S, Joda T, Soffe B, Awan KH, Fageeh HN, Tovani-Palone MR, et al. Efficacy of artificial intelligence in the detection of periodontal bone loss and classification of periodontal diseases: a systematic review. J Am Dent Assoc. Sep 2023;154(9):795-804.e1. [ CrossRef ] [ Medline ]
- Quek LJ, Heikkonen MR, Lau Y. Use of artificial intelligence techniques for detection of mild cognitive impairment: a systematic scoping review. J Clin Nurs. Sep 10, 2023;32(17-18):5752-5762. [ CrossRef ] [ Medline ]
- Tan D, Mohd Nasir NF, Abdul Manan H, Yahya N. Prediction of toxicity outcomes following radiotherapy using deep learning-based models: a systematic review. Cancer Radiother. Sep 2023;27(5):398-406. [ CrossRef ] [ Medline ]
- Rabilloud N, Allaume P, Acosta O, De Crevoisier R, Bourgade R, Loussouarn D, et al. Deep learning methodologies applied to digital pathology in prostate cancer: a systematic review. Diagnostics (Basel). Aug 14, 2023;13(16):2676. [ FREE Full text ] [ CrossRef ] [ Medline ]
- Li K, Yao S, Zhang Z, Cao B, Wilson C, Kalos D, et al. Efficient gradient boosting for prognostic biomarker discovery. Bioinformatics. Mar 04, 2022;38(6):1631-1638. [ FREE Full text ] [ CrossRef ] [ Medline ]
- Chen T, Chen Y, Li H, Gao T, Tu H, Li S. Driver intent-based intersection autonomous driving collision avoidance reinforcement learning algorithm. Sensors (Basel). Dec 16, 2022;22(24):9943. [ FREE Full text ] [ CrossRef ] [ Medline ]
- Huynh QT, Nguyen PH, Le HX, Ngo LT, Trinh NT, Tran MT, et al. Automatic acne object detection and acne severity grading using smartphone images and artificial intelligence. Diagnostics (Basel). Aug 03, 2022;12(8):1879. [ FREE Full text ] [ CrossRef ] [ Medline ]
- Brooke BS, Schwartz TA, Pawlik TM. MOOSE reporting guidelines for meta-analyses of observational studies. JAMA Surg. Aug 01, 2021;156(8):787-788. [ CrossRef ] [ Medline ]
- Scholten RJ, Clarke M, Hetherington J. The Cochrane collaboration. Eur J Clin Nutr. Aug 28, 2005;59 Suppl 1(S1):S147-S196. [ CrossRef ] [ Medline ]
- Warrens MJ. Kappa coefficients for dichotomous-nominal classifications. Adv Data Anal Classif. Apr 07, 2020;15(1):193-208. [ CrossRef ]
- Law M, Stewart D, Letts L, Pollock N, Bosch J. Guidelines for critical review of qualitative studies. McMaster University Occupational Therapy Evidence-Based Practice Research Group. URL: https://www.canchild.ca/system/tenon/assets/attachments/000/000/360/original/qualguide.pdf [accessed 2024-04-05]
- Higgins JP, Morgan RL, Rooney AA, Taylor KW, Thayer KA, Silva RA, et al. Risk of bias in non-randomized studies - of exposure (ROBINS-E). ROBINS-E tool. URL: https://www.riskofbias.info/welcome/robins-e-tool [accessed 2024-04-02]
- Nait Aicha A, Englebienne G, van Schooten KS, Pijnappels M, Kröse B. Deep learning to predict falls in older adults based on daily-life trunk accelerometry. Sensors (Basel). May 22, 2018;18(5):1654. [ FREE Full text ] [ CrossRef ] [ Medline ]
- Ribeiro NF, André J, Costa L, Santos CP. Development of a strategy to predict and detect falls using wearable sensors. J Med Syst. Apr 04, 2019;43(5):134. [ CrossRef ] [ Medline ]
- Qiu H, Rehman RZ, Yu X, Xiong S. Application of wearable inertial sensors and a new test battery for distinguishing retrospective fallers from non-fallers among community-dwelling older people. Sci Rep. Nov 05, 2018;8(1):16349. [ FREE Full text ] [ CrossRef ] [ Medline ]
- Dubois A, Bihl T, Bresciani JP. Automatic measurement of fall risk indicators in timed up and go test. Inform Health Soc Care. Sep 13, 2019;44(3):237-245. [ CrossRef ] [ Medline ]
- Noh B, Youm C, Goh E, Lee M, Park H, Jeon H, et al. XGBoost based machine learning approach to predict the risk of fall in older adults using gait outcomes. Sci Rep. Jun 09, 2021;11(1):12183. [ CrossRef ] [ Medline ]
- Hauth J, Jabri S, Kamran F, Feleke EW, Nigusie K, Ojeda LV, et al. Automated loss-of-balance event identification in older adults at risk of falls during real-world walking using wearable inertial measurement units. Sensors (Basel). Jul 07, 2021;21(14):4661. [ FREE Full text ] [ CrossRef ] [ Medline ]
- Lockhart TE, Soangra R, Yoon H, Wu T, Frames CW, Weaver R. Prediction of fall risk among community-dwelling older adults using a wearable system. Sci Rep. 2021;11(1):20976. [ CrossRef ]
- Hsu YC, Weng HH, Kuo CY, Chu TP, Tsai YH. Prediction of fall events during admission using eXtreme gradient boosting: a comparative validation study. Sci Rep. Oct 08, 2020;10(1):16777. [ FREE Full text ] [ CrossRef ] [ Medline ]
- Hu Y, Bishnoi A, Kaur R, Sowers R, Hernandez ME. Exploration of machine learning to identify community dwelling older adults with balance dysfunction using short duration accelerometer data. In: Proceedings of the 42nd Annual International Conference of the IEEE Engineering in Medicine & Biology Society. 2020. Presented at: EMBC '20; July 20-24, 2020;812-815; Montreal, QC. URL: https://ieeexplore.ieee.org/document/9175871 [ CrossRef ]
- Ye C, Li J, Hao S, Liu M, Jin H, Zheng L, et al. Identification of elders at higher risk for fall with statewide electronic health records and a machine learning algorithm. Int J Med Inform. May 2020;137:104105. [ FREE Full text ] [ CrossRef ] [ Medline ]
- Althobaiti T, Katsigiannis S, Ramzan N. Triaxial accelerometer-based falls and activities of daily life detection using machine learning. Sensors (Basel). Jul 06, 2020;20(13):3777. [ FREE Full text ] [ CrossRef ] [ Medline ]
- Tunca C, Salur G, Ersoy C. Deep learning for fall risk assessment with inertial sensors: utilizing domain knowledge in spatio-temporal gait parameters. IEEE J Biomed Health Inform. Jul 2020;24(7):1994-2005. [ CrossRef ]
- Kim K, Yun G, Park SK, Kim DH. Fall detection for the elderly based on 3-axis accelerometer and depth sensor fusion with random forest classifier. In: Proceedings of the 41st Annual International Conference of the IEEE Engineering in Medicine and Biology Society. 2019. Presented at: EMBC '19; July 23-27, 2019;4611-4614; Berlin, Germany. URL: https://ieeexplore.ieee.org/document/8856698 [ CrossRef ]
- Gillain S, Boutaayamou M, Schwartz C, Brüls O, Bruyère O, Croisier JL, et al. Using supervised learning machine algorithm to identify future fallers based on gait patterns: a two-year longitudinal study. Exp Gerontol. Nov 2019;127:110730. [ FREE Full text ] [ CrossRef ] [ Medline ]
- Lo Y, Lynch SF, Urbanowicz RJ, Olson RS, Ritter AZ, Whitehouse CR, et al. Using machine learning on home health care assessments to predict fall risk. Stud Health Technol Inform. Aug 21, 2019;264:684-688. [ CrossRef ] [ Medline ]
- Maray N, Ngu AH, Ni J, Debnath M, Wang L. Transfer learning on small datasets for improved fall detection. Sensors (Basel). Jan 18, 2023;23(3):1105. [ FREE Full text ] [ CrossRef ] [ Medline ]
- Ladios-Martin M, Cabañero-Martínez MJ, Fernández-de-Maya J, Ballesta-López FJ, Belso-Garzas A, Zamora-Aznar FM, et al. Development of a predictive inpatient falls risk model using machine learning. J Nurs Manag. Nov 30, 2022;30(8):3777-3786. [ CrossRef ] [ Medline ]
- Eichler N, Raz S, Toledano-Shubi A, Livne D, Shimshoni I, Hel-Or H. Automatic and efficient fall risk assessment based on machine learning. Sensors (Basel). Feb 17, 2022;22(4):1557. [ FREE Full text ] [ CrossRef ] [ Medline ]
- Tang YM, Wang YH, Feng XY, Zou QS, Wang Q, Ding J, et al. Diagnostic value of a vision-based intelligent gait analyzer in screening for gait abnormalities. Gait Posture. Jan 2022;91:205-211. [ CrossRef ] [ Medline ]
- Greene BR, McManus K, Ader LG, Caulfield B. Unsupervised assessment of balance and falls risk using a smartphone and machine learning. Sensors (Basel). Jul 13, 2021;21(14):4770. [ FREE Full text ] [ CrossRef ] [ Medline ]
- Roshdibenam V, Jogerst GJ, Butler NR, Baek S. Machine learning prediction of fall risk in older adults using timed up and go test kinematics. Sensors (Basel). May 17, 2021;21(10):3481. [ FREE Full text ] [ CrossRef ] [ Medline ]
- Dubois A, Bihl T, Bresciani JP. Identifying fall risk predictors by monitoring daily activities at home using a depth sensor coupled to machine learning algorithms. Sensors (Basel). Mar 11, 2021;21(6):1957. [ FREE Full text ] [ CrossRef ] [ Medline ]
- Vo MT, Thonglor R, Moncatar TJ, Han TD, Tejativaddhana P, Nakamura K. Fear of falling and associated factors among older adults in Southeast Asia: a systematic review. Public Health. Sep 2023;222:215-228. [ CrossRef ] [ Medline ]
- Torun E, Az A, Akdemir T, Solakoğlu GA, Açiksari K, Güngörer B. Evaluation of the risk factors for falls in the geriatric population presenting to the emergency department. Ulus Travma Acil Cerrahi Derg. Aug 2023;29(8):897-903. [ FREE Full text ] [ CrossRef ] [ Medline ]
- Son NK, Ryu YU, Jeong HW, Jang YH, Kim HD. Comparison of 2 different exercise approaches: Tai Chi versus Otago, in community-dwelling older women. J Geriatr Phys Ther. 2016;39(2):51-57. [ CrossRef ] [ Medline ]
- Sawa R, Doi T, Tsutsumimoto K, Nakakubo S, Kurita S, Kiuchi Y, et al. Overlapping status of frailty and fear of falling: an elevated risk of incident disability in community-dwelling older adults. Aging Clin Exp Res. Sep 11, 2023;35(9):1937-1944. [ FREE Full text ] [ CrossRef ] [ Medline ]
- Calazans JA, Permanyer I. Levels, trends, and determinants of cause-of-death diversity in a global perspective: 1990-2019. BMC Public Health. Apr 05, 2023;23(1):650. [ FREE Full text ] [ CrossRef ] [ Medline ]
- Kakara R, Bergen G, Burns E, Stevens M. Nonfatal and fatal falls among adults aged ≥65 years - United States, 2020-2021. MMWR Morb Mortal Wkly Rep. Sep 01, 2023;72(35):938-943. [ FREE Full text ] [ CrossRef ] [ Medline ]
- Dostan A, Dobson CA, Vanicek N. Relationship between stair ascent gait speed, bone density and gait characteristics of postmenopausal women. PLoS One. Mar 22, 2023;18(3):e0283333. [ FREE Full text ] [ CrossRef ] [ Medline ]
- Zheng Y, Wang X, Zhang ZK, Guo B, Dang L, He B, et al. Bushen Yijing Fang reduces fall risk in late postmenopausal women with osteopenia: a randomized double-blind and placebo-controlled trial. Sci Rep. Feb 14, 2019;9(1):2089. [ FREE Full text ] [ CrossRef ] [ Medline ]
- Woelfle T, Bourguignon L, Lorscheider J, Kappos L, Naegelin Y, Jutzeler CR. Wearable sensor technologies to assess motor functions in people with multiple sclerosis: systematic scoping review and perspective. J Med Internet Res. Jul 27, 2023;25:e44428. [ FREE Full text ] [ CrossRef ] [ Medline ]
- Abdollah V, Dief TN, Ralston J, Ho C, Rouhani H. Investigating the validity of a single tri-axial accelerometer mounted on the head for monitoring the activities of daily living and the timed-up and go test. Gait Posture. Oct 2021;90:137-140. [ CrossRef ] [ Medline ]
- Mielke GI, de Almeida Mendes M, Ekelund U, Rowlands AV, Reichert FF, Crochemore-Silva I. Absolute intensity thresholds for tri-axial wrist and waist accelerometer-measured movement behaviors in adults. Scand J Med Sci Sports. Sep 12, 2023;33(9):1752-1764. [ CrossRef ] [ Medline ]
- Löppönen A, Delecluse C, Suorsa K, Karavirta L, Leskinen T, Meulemans L, et al. Association of sit-to-stand capacity and free-living performance using Thigh-Worn accelerometers among 60- to 90-yr-old adults. Med Sci Sports Exerc. Sep 01, 2023;55(9):1525-1532. [ FREE Full text ] [ CrossRef ] [ Medline ]
- García-Soidán JL, Leirós-Rodríguez R, Romo-Pérez V, García-Liñeira J. Accelerometric assessment of postural balance in children: a systematic review. Diagnostics (Basel). Dec 22, 2020;11(1):8. [ FREE Full text ] [ CrossRef ] [ Medline ]
- Leirós-Rodríguez R, García-Soidán JL, Romo-Pérez V. Analyzing the use of accelerometers as a method of early diagnosis of alterations in balance in elderly people: a systematic review. Sensors (Basel). Sep 09, 2019;19(18):3883. [ FREE Full text ] [ CrossRef ] [ Medline ]
Abbreviations
Edited by A Mavragani; submitted 28.11.23; peer-reviewed by E Andrade, M Behzadifar, A Suárez; comments to author 09.01.24; revised version received 30.01.24; accepted 13.02.24; published 29.04.24.
©Ana González-Castro, Raquel Leirós-Rodríguez, Camino Prada-García, José Alberto Benítez-Andrades. Originally published in the Journal of Medical Internet Research (https://www.jmir.org), 29.04.2024.
This is an open-access article distributed under the terms of the Creative Commons Attribution License (https://creativecommons.org/licenses/by/4.0/), which permits unrestricted use, distribution, and reproduction in any medium, provided the original work, first published in the Journal of Medical Internet Research, is properly cited. The complete bibliographic information, a link to the original publication on https://www.jmir.org/, as well as this copyright and license information must be included.
Research & Reviews: Journal of Pharmaceutical Analysis
e-ISSN: 2320-0812

- +447389646377
2022: Volume 11, Issue 5
This paper is in the following e-collection/theme issue:
Published on 30.4.2024 in Vol 12 (2024)
Effectiveness of mHealth App–Based Interventions for Increasing Physical Activity and Improving Physical Fitness in Children and Adolescents: Systematic Review and Meta-Analysis
Authors of this article:

- Jun-Wei Wang 1, 2 , MSc ;
- Zhicheng Zhu 3 , MSc ;
- Zhang Shuling 1 , PhD ;
- Jia Fan 1 , MSc ;
- Yu Jin 1 , MSc ;
- Zhan-Le Gao 1 , MSc ;
- Wan-Di Chen 4 , MSc ;
- Xue Li 1 , PhD
1 School of Sport Medicine and Health, Chengdu Sport University, Chengdu, China
2 School of Sports Science, Beijing Sport University, Beijing, China
3 Physical education institute, Xinyu University, Xinyu, China
4 Academic Administration, Chengdu Sport University, Chengdu, China
Corresponding Author:
Xue Li, PhD
School of Sport Medicine and Health
Chengdu Sport University
No.2, Tiyuan Road, Wuhou District
Chengdu, 610041
Phone: 86 13550146822
Email: [email protected]
Background: The COVID-19 pandemic has significantly reduced physical activity (PA) levels and increased sedentary behavior (SB), which can lead to worsening physical fitness (PF). Children and adolescents may benefit from mobile health (mHealth) apps to increase PA and improve PF. However, the effectiveness of mHealth app–based interventions and potential moderators in this population are not yet fully understood.
Objective: This study aims to review and analyze the effectiveness of mHealth app–based interventions in promoting PA and improving PF and identify potential moderators of the efficacy of mHealth app–based interventions in children and adolescents.
Methods: We searched for randomized controlled trials (RCTs) published in the PubMed, Web of Science, EBSCO, and Cochrane Library databases until December 25, 2023, to conduct this meta-analysis. We included articles with intervention groups that investigated the effects of mHealth-based apps on PA and PF among children and adolescents. Due to high heterogeneity, a meta-analysis was conducted using a random effects model. The Cochrane Risk of Bias Assessment Tool was used to evaluate the risk of bias. Subgroup analysis and meta-regression analyses were performed to identify potential influences impacting effect sizes.
Results: We included 28 RCTs with a total of 5643 participants. In general, the risk of bias of included studies was low. Our findings showed that mHealth app–based interventions significantly increased total PA (TPA; standardized mean difference [SMD] 0.29, 95% CI 0.13-0.45; P <.001), reduced SB (SMD –0.97, 95% CI –1.67 to –0.28; P =.006) and BMI (weighted mean difference –0.31 kg/m 2 , 95% CI –0.60 to –0.01 kg/m 2 ; P =.12), and improved muscle strength (SMD 1.97, 95% CI 0.09-3.86; P =.04) and agility (SMD –0.35, 95% CI –0.61 to –0.10; P =.006). However, mHealth app–based interventions insignificantly affected moderate to vigorous PA (MVPA; SMD 0.11, 95% CI –0.04 to 0.25; P <.001), waist circumference (weighted mean difference 0.38 cm, 95% CI –1.28 to 2.04 cm; P =.65), muscular power (SMD 0.01, 95% CI –0.08 to 0.10; P =.81), cardiorespiratory fitness (SMD –0.20, 95% CI –0.45 to 0.05; P =.11), muscular endurance (SMD 0.47, 95% CI –0.08 to 1.02; P =.10), and flexibility (SMD 0.09, 95% CI –0.23 to 0.41; P =.58). Subgroup analyses and meta-regression showed that intervention duration was associated with TPA and MVPA, and age and types of intervention was associated with BMI.
Conclusions: Our meta-analysis suggests that mHealth app–based interventions may yield small-to-large beneficial effects on TPA, SB, BMI, agility, and muscle strength in children and adolescents. Furthermore, age and intervention duration may correlate with the higher effectiveness of mHealth app–based interventions. However, due to the limited number and quality of included studies, the aforementioned conclusions require validation through additional high-quality research.
Trial Registration: PROSPERO CRD42023426532; https://tinyurl.com/25jm4kmf
Introduction
The COVID-19 global pandemic has had adverse effects on the physical fitness (PF) and mental health of children and adolescents [ 1 ]. Before the COVID-19 pandemic outbreak, only approximately 30% of children and adolescents worldwide could meet the recommended levels of physical activity (PA) [ 2 , 3 ]. However, the COVID-19 pandemic has exacerbated this issue by decreasing their levels of PA, increasing sedentary behavior (SB), and leading to a decline in their PF [ 4 ]. A recent study in the United Kingdom discovered that children exhibited lower performance scores on the seated forward bend and 20-meter shuttle run test and higher BMI values in 2020 than in 2019 [ 5 ]. PF is a crucial determinant of children’s and adolescents’ health status [ 6 ], which can be influenced by various factors, including genetic, environmental, and PA-related factors [ 7 ]. Epidemiological studies have established a “dose‒response” relationship between PA and PF, which showed that increased PA levels and reduced SB were positively associated with improved PF in adolescents [ 8 ]. Therefore, the way to increase PA and improve PF is still one of the most important social problems to be solved for children and adolescents.
The rapid advancement of intelligent technology has increased the use of smartphones among young generation and the wide use of mobile health (mHealth) technologies [ 9 , 10 ]. At present, mHealth technologies, including wearable devices, smartphones, tablets, mHealth apps, smartwatches, and pedometers, are commonly used in the field of health care [ 11 ]. Recently, 2 systematic reviews have investigated the impact of mHealth-based interventions on behavioral changes, including PA and SB, in children and adolescents [ 12 , 13 ]. However, these reviews primarily concentrated on specific mHealth technology interventions, including SMS text messaging, wearable devices, web-based interventions, and others. Moreover, these systematic reviews exclusively focus on one or more behavioral changes, encompassing physical inactivity and SB, and the overall quality of the evidence is deemed low [ 13 ]. Current research indicates that app-based interventions on smartphones may represent the most effective strategy [ 13 ]. mHealth app–based interventions are among the most commonly used methods within the realm of mHealth technologies. Among these technologies, mHealth apps have been extensively used in the fitness and medical fields due to their affordability, personalization, and diverse features [ 14 ]. mHealth apps can provide quantitative visual feedback regarding the health behaviors of users, such as their PA; meanwhile, users upload their personal information to app databases, and apps facilitate personalized, long-distance, and low-contact training to improve the healthy development of users [ 15 ]. In recent years, mHealth app–based interventions have shown significant promise in promoting healthy behaviors, including increased PA and reduced SB, among children and adolescents. Nevertheless, there is a lack of systematic reviews comprehensively summarizing the impacts of stand-alone mHealth apps or concerted interventions using apps as one of the multiple components (eg, behavioral counseling combined with app interventions) on various health behaviors, including PF. In addition, studies in this domain have been predominantly centered on adults, with a noticeable dearth of pertinent research within populations such as children and adolescents [ 16 ].
Studies have demonstrated that mHealth app–based interventions can lead to effective outcomes in improving the PA behavior of users [ 17 ]. However, another study [ 18 ] found that such intervention has indicated only small effects on PA and is likely related to potential influencing factors. Furthermore, the efficacy of mHealth app–based interventions on PF is inconsistent. One study linked lower BMI and higher motor competence to the frequency and type of mHealth app use [ 19 ], while another study indicated that mHealth app–based interventions were ineffective in improving PF among adolescents, which is possibly due to the characteristics of the intervention [ 20 ]. The use of theory-based mHealth app interventions may also be more advantageous in increasing PA and enhancing PF in children and adolescents [ 21 ]. Several theoretical paradigms, including self-determination theory (SDT), the transtheoretical model, the health belief model, the theory of planned behavior, and social cognitive theory (SCT), have been used in mHealth app–based interventions [ 22 ]. The number and type of behavior change technique (BCT) clusters may also play a significant role in the effectiveness of mHealth app interventions. Michie et al [ 23 ] provided a standardized taxonomy of BCT that categorizes them into 16 clusters, such as feedback and monitoring, reward and threat, goals and planning, shaping knowledge, social support, and comparison of outcomes. This taxonomy aids in identifying which BCT clusters are more effectively applied to apps, thereby enhancing PA promotion and PF improvement. In conclusion, various factors, including the type of mHealth app, intervention characteristics, theoretical paradigms, and BCT clusters, are important considerations in the effectiveness of mHealth app interventions. Despite the increasing number of articles summarizing interventions based on mHealth apps, a noteworthy research gap persists. Most of these articles concentrate on interventions using commercially available mHealth apps that lack evidence-based behavior change strategies. Nevertheless, a significant proportion of users and patients rely on commercially available app - based mHealth interventions that lack empirical evaluation and rarely incorporate evidence-based behavior change strategies. Furthermore, current research predominantly highlights the intervention effects of mHealth apps on health-related outcome measures. However, there is a notable deficiency in evidence-based mHealth apps intervention programs and studies that integrate various target behaviors.
The first objective of this systematic review aims to evaluate the effectiveness of mHealth app–based interventions in promoting PA and improving PF among children and adolescents. The second objective is to specifically assess moderating effects (eg, age, types of apps, theoretical paradigm, BCT clusters, and intervention duration) on the effectiveness of mHealth app–based interventions in subgroups within these studies. Unlike previous studies, our review contributes evidence-based, high-quality content for potential mHealth app interventions addressing PA and PF. This contribution results from a meticulous evaluation and meta-analysis of relevant randomized controlled trials (RCTs). In addition, we conducted an extensive analysis of key moderating variables using subgroups and meta-regression, encompassing theoretical paradigms, BCT clusters, intervention duration, and more. This comprehensive approach enhances our understanding of the factors influencing intervention effects and facilitates the precise quantification of the intervention program. Our endeavors significantly expand the research scope beyond previous reviews.
Registration and Approval
The systematic reviews were registered on the PROSPERO (CRD42023426532). The literature search, reporting guidance, and implementation process of the study followed the PRISMA (Preferred Reporting Items for Systematic Reviews and Meta-Analyses) guidelines [ 24 ].
Search Strategy
Several databases were searched, including PubMed, Web of Science, EBSCO, and Cochrane Library, to identify relevant RCTs published until December 25, 2023. The search strategy involved a Boolean search using a combination of subject-related words and free words. The following search terms were used: (Child OR Preschool OR Adolescent), (“Mobile health application*” OR “mHealth app*” OR “Portable Software Application*” OR “Mobile Application*” OR App*), and (“Physical Activity” OR PA OR MVPA OR “sedentary behavior” OR SB OR “Physical Fitness”). A detailed search strategy for Web of Science is presented in Multimedia Appendix 1 . We opted to update the searches in the same databases used for the initial search to refine the results for this study. In addition, we examined the references cited in previous reviews to identify additional relevant literature. Concurrently, we reached out to authors of potentially eligible studies to obtain complete data. If >2 attempts were made to contact authors without receiving a response, the study was excluded. The literature search was not restricted by language.
Inclusion and Exclusion Criteria
Inclusion criteria.
The following criteria were included for inclusion in the literature review: (1) The study comprised children and adolescents, aged 3 to 18 years, with the majority falling within this range. Participants did not exhibit physical dysfunction, and overweight or obesity, among other factors, were not exclusionary criteria. Children and adolescents were categorized into 3 groups: preschoolers aged 3-6 years; children aged 7-12 years; and adolescents aged 13-18 years. (2) Interventions using smartphone-based and tablet-based mHealth apps may involve either stand-alone apps (ie, solely apps) or concerted intervention (ie, apps combined with another intervention). The control group comprised genuine controls, such as no interventions, waitlist conditions, and usual clinical care. In addition, active controls, including interventions via apps, were considered. Studies using placebo and sham apps also met the inclusion criteria. (3) The study design was an RCT. (4) Primary outcome measures included PA, SB, performance-related PF (eg, coordination and balance), health-related PF (eg, cardiorespiratory endurance, muscle strength, and body composition), and physiological function (eg, body shape and metabolism).
Exclusion Criteria
The exclusion criteria were as follows: (1) articles written in languages other than English and Chinese; (2) repeated published studies, basic studies, observational studies, reviews, and case series articles; (3) studies for which full text was unavailable or data were incomplete; and (4) mHealth apps that only used SMS text messaging interventions or were incompatible with smartphones or tablets.
Study Selection
After the literature search, the initial search results were imported into EndNote 20 (Thomson ResearchSoft) to remove duplicate articles. Predefined inclusion and exclusion criteria were applied to the literature. Two authors (ZZ and ZS) initially screened the titles and abstracts. Articles meeting the criteria were downloaded, and 1 author (ZZ) thoroughly evaluated the full text based on the inclusion and exclusion criteria, while the other author (ZS) conducted a randomized assessment. Another author (JF) was involved in resolving discrepancies between 2 independent reviewers to determine if a study met the inclusion criteria.
Data Extraction
The included literature was independently extracted by 2 researchers (YJ and ZLG). The extracted information included basic details (eg, authors, publication year, country, and study type), characteristics of the study population (eg, age, gender, and sample size), characteristics of mHealth apps (eg, name, type, theoretical paradigm, and number or type of BCT clusters), intervention characteristics, outcome measures, and indicators related to risk of bias assessment. Two authors (YJ and ZLG) assessed the use of BCT in apps using the taxonomy by Michie [ 23 ]. Relevant information was primarily extracted from the study descriptions; in cases of incomplete data, the original apps were consulted. Disputes were resolved through third-party consultation (WDC).
Risk of Bias Assessment
Two independent (YJ and ZLG) investigators assessed the risk of bias using the Cochrane Working Group’s tool [ 25 ]. Any disagreements were resolved by a third independent researcher (WDC) through consultation. Each study underwent evaluation in 7 domains, and the risk of bias was categorized as unclear, low, or high.
Statistical Analysis
If the number of included papers was <3, then a systematic review was conducted. When a sufficient number of included studies were available for a meta-analysis, we used Revman 5.4 (Nordic Cochrane Center) and Stata 16.0 (StataCorp) to estimate effect sizes, conduct subgroup analysis, and sensitivity analysis. Weighted mean difference (WMD) and 95% CI were used as effect measures when the same measurement method was used. When measurement methods were consistent, the standardized mean difference (SMD) and 95% CI were used. The magnitude of SMD was interpreted as follows: SMD<0.2, negligible; 0.2≤SMD<0.5, small; 0.5≤SMD<0.8, medium; and SMD≥0.8, large [ 26 ].
The study indirectly mentioned the mean and SD, and the MD and SMD were calculated as the postintervention mean and SD based on the Cochrane Handbook [ 27 ]. The study examined the magnitude of heterogeneity using I 2 and P values. If I 2 ≤50% and P ≥.10, a fixed-effect model was used for data analysis. On the contrary, if I 2 >50% and P <.10, a random effect model was used for meta-analysis [ 27 ]. The sources of heterogeneity were identified by subgroup analyses and meta-regression analysis based on type of apps, theoretical paradigm, age, and BCT clusters. The robustness of each study was evaluated using sensitivity analysis, and publication bias was assessed with funnel plots and the Egger test. Results
A total of 12,025 relevant articles were retrieved from PubMed (n=69), Web of Science (n=11,033), EBSCO (n=217), and Cochrane Library (n=706). Duplicate references were removed, which resulted in 6867 articles. Following an initial screening by abstracts, 136 articles were identified, which were then assessed by reading the full text. Finally, 28 articles were chosen for inclusion ( Figure 1 ).

Characteristics of the Included Studies
This study included 28 publications, all of which were RCTs published between 2014 and 2023 [ 20 , 26 , 28 - 49 ]. The sample size included 5643 subjects, ranging from 12 to 1392 participants per study. As shown in Table 1 , the included studies have the following basic characteristics. The age of the participants varied from 3 to 18 years with 5 (18%) of the 28 studies involving preschool children [ 26 , 44 , 46 , 48 , 50 ], 8 (29%) studies involving children [ 28 - 31 , 33 , 36 , 37 , 51 ], and 15 (53%) studies involving adolescents [ 20 , 32 , 34 , 35 , 38 - 43 , 45 , 47 , 49 , 52 , 53 ]. The objectives of the studies differed, and the selection criteria for the target population varied inconsistently. Most studies (16/28, 57%) focused on healthy children and adolescents; however, of the 28 studies, 9 (32%) included participants with overweight and those with obesity [ 29 - 31 , 35 , 36 , 39 , 43 , 45 , 52 ], 2 (7%) involved children with cancer [ 34 , 53 ], and 1 (4%) enrolled youth with congenital heart disease [ 40 ]. Of the 28 studies, 8 (29%) were conducted in Asia [ 31 , 36 , 37 , 39 - 41 , 44 , 45 ], while the remaining studies were performed in Oceania (7/28, 25%) [ 29 , 30 , 35 , 38 , 46 , 50 , 53 ], North America (5/28, 18%) [ 28 , 33 , 34 , 43 , 51 ], and Europe (8/28, 29%) [ 20 , 26 , 32 , 42 , 47 - 49 , 52 ].
This review encompassed 28 studies that used 14 different mHealth apps. These included 9 commercial apps [ 20 , 31 - 33 , 35 , 38 , 41 , 47 , 49 ] and 14 research apps [ 28 - 30 , 39 , 40 , 42 - 46 , 48 , 51 - 53 ], and 5 mHealth apps did not provide the corresponding information [ 26 , 34 , 36 , 37 , 50 ]. The type of intervention used in 15 studies was stand-alone apps [ 20 , 26 , 28 , 32 , 33 , 40 , 41 , 43 - 47 , 49 - 51 ], and 13 studies used concerted intervention [ 29 - 31 , 34 - 39 , 42 , 48 , 52 , 53 ]. Participant engagement with the intervention was mentioned in 28 studies, 8 studies focused on parent-centered [ 26 , 31 , 36 , 37 , 44 , 46 , 48 , 50 ], the remaining studies were child centered. The mHealth apps were based on various theoretical paradigms, including self-regulation theory (SRT), SDT, and SCT. Different numbers or types of BCT clusters were identified, and they ranged from 1 to 7 clusters. Examples of BCT clusters used included goal setting and planning, feedback and monitoring, and behavioral comparison. Interventions duration lasted 2 to 48 weeks ( Table 1 ). The primary and secondary outcome measures included total PA (TPA), moderate to vigorous PA (MVPA), SB, cardiorespiratory fitness (CRF), BMI, waist circumference (WC), muscle strength, agility, flexibility, muscular power, and endurance.
a BCT: behavior change technique.
b SRT: self-regulation theory.
c CRF: cardiorespiratory fitness.
d TPA: total physical activity.
e SB: sedentary behavior.
f MVPA: moderate to vigorous physical activity.
g SDT: self-determination theory.
h PA: physical activity.
i SCT: social cognitive theory.
j WC: waist circumference.
k NR: not reported.
l TTM: transtheoretical model.
m Not available.
Overall, of the 28 studies, 11 (39%) were classified as having a low risk of bias [ 20 , 26 , 28 , 29 , 34 , 35 , 37 , 39 , 51 - 53 ], and a high risk of bias was identified in 17 (61%) studies [ 30 - 33 , 36 , 38 , 40 - 50 ]. The methods for random sequence generation were adequately reported in 28 studies, and 14 (50%) of the 28 studies described allocation concealment protocols [ 20 , 26 , 29 , 31 , 35 , 37 , 39 , 43 , 46 , 48 , 50 - 53 ]. Of the 28 studies, blinding of participants and personnel was unclear in 17 studies [ 20 , 28 - 33 , 38 - 41 , 43 - 45 , 47 , 49 , 51 ], high risk of bias was identified in 7 (25%) studies [ 26 , 34 , 35 , 37 , 50 , 52 , 53 ], and blinding of outcome assessment was unclear in 18 (64%) studies [ 18 , 28 - 34 , 38 , 40 , 41 , 44 , 45 , 47 - 51 , 53 ]. Four studies provided data regarding subjects lost to follow-up [ 30 - 32 , 34 ], while one study had unclear information on this aspect [ 51 ]. None of the 28 studies were found to have selective outcome reporting, and other aspects of bias were evaluated mainly in terms of baseline data and conflicts of interest ( Figure 2 [ 20 , 26 , 28 - 53 ]).

Results of the Meta-Analysis
Effects of mhealth app–based interventions on pa.
Of the 28 studies, 21 (75%) were examined to assess the impact of mHealth app–based interventions on TPA. The heterogeneity test indicated a substantial level of heterogeneity among the studies ( I 2 =75%; P< .001), which led to the adoption of a random effects model for the analysis. The meta-analysis results indicated that the intervention group exhibited higher TPA (SMD 0.29, 95% CI 0.13-0.45; P <.001; Figure 3 [ 20 , 28 , 30 , 35 , 37 , 38 , 40 , 41 , 43 , 46 , 47 , 49 - 51 ]), but the effect size was small.
Of the 28 studies, 14 (50%) reported the impact of mHealth app–based interventions on SB levels. Heterogeneity tests revealed homogeneity between the studies ( I 2 =98%; P< .001), which required analysis using a random effect model. Meta-analysis found that mHealth app–based interventions significantly reduced SB (SMD –0.97, 95% CI –1.67 to –0.28; P =.006; Figure 4 [ 20 , 26 , 29 , 30 , 32 , 34 , 35 , 43 , 44 , 46 , 48 , 53 ]).
Of the 28 studies, 14 (50%) investigated the impact of mHealth app–based interventions on MVPA levels. The heterogeneity test indicated homogeneity among the studies ( I 2 =67%; P <.001), which allowed for analysis using a random effect model. There were no significant differences between the control and intervention groups (SMD 0.11, 95% CI –0.04 to 0.25; P <.001; Figure 5 [ 20 , 26 , 29 , 30 , 33 , 34 , 37 , 38 , 41 , 46 , 48 , 53 ]).

Effects of mHealth App–Based Interventions on BMI and WC
Of the 28 studies, 13 (46%) investigated the effects of mHealth app–based interventions on BMI. Of these 13 studies, 2 (15%) did not directly conduct changes in outcome indicators before and after interventions [ 31 , 52 ] and were only included in systematic reviews. The heterogeneity test demonstrated homogeneity among the studies ( I 2 =32%; P =.12), which enabled analysis using a fixed-effect model. Meta-analysis found that mHealth app–based interventions significantly reduced BMI (WMD –0.31, 95% CI –0.60 to –0.01; P =.12; Figure 6 [ 29 , 30 , 35 - 37 , 39 , 45 , 47 , 49 , 53 ]). The 2 other studies on BMI reported a significant reduction in BMI among obese children with mHealth app–based interventions [ 31 , 52 ], which is consistent with the meta-analysis results.
Of the 28 studies, 9 (32%) reported the effect of mHealth app–based interventions on WC. The heterogeneity tests showed homogeneity among the studies ( I 2 =54%; P =.02), which allowed for analysis using a random effect model. There were no significant differences in WC between the intervention and control groups (WMD 0.38 kg/m 2 , 95% CI –1.28 to 2.04 kg/m 2 ; P =.65; Figure 7 [ 29 , 37 , 39 , 42 , 47 , 49 , 53 ]).

Effects of mHealth App–Based Interventions on PF
Of the 28 studies, 7 (25%) studies investigated the impact of mHealth app–based interventions on CRF. Heterogeneity tests showed homogeneity among the studies ( I 2 =66%; P =.007) and were conducted using a random effect model. No significant differences were found between intervention and control groups in CRF (SMD –0.20 cm, 95% CI –0.45 to 0.05 cm; P =.11; Figure 8 [ 20 , 37 , 42 , 45 , 47 , 53 ]).
Of the 28 studies, 6 (21%) reported the impact of mHealth app–based interventions on muscle strength. Heterogeneity tests revealed homogeneity in the studies ( I 2 =99%; P <.001) and were conducted using a random effect model. Meta-analysis found that mHealth app–based interventions significantly increased muscle strength (SMD 1.97, 95% CI 0.09-3.86; P =.04; Figure 9 [ 29 , 30 , 36 , 44 , 47 , 53 ]).
Of the 28 studies, 5 (18%) investigated the impact of mHealth app–based interventions on muscular power. The heterogeneity test indicated homogeneity among the studies ( I 2 =45%; P =.12), which allowed for analysis using a fixed-effect model. There were no significant differences between the control and intervention groups (SMD 0.01, 95% CI –0.08 to 0.10; P =.81; Figure 10 [ 36 , 37 , 42 , 44 , 47 ]).
Of the 28 studies, 5 (18%) examined the impact of mHealth app–based interventions on muscular endurance. The heterogeneity test indicated a substantial level of heterogeneity among the studies ( I 2 =98%; P< .001), which led to the adoption of a random effects model for the analysis. The meta-analysis results indicated no significant difference in muscle endurance between the intervention and control groups (SMD 0.47, 95% CI –0.08 to 1.02; P =.10; Figure 11 [ 29 , 36 , 37 , 42 , 47 ]).
Of the 28 studies, 3 (11%) investigated the impact of mHealth app–based interventions on agility. The heterogeneity test indicated homogeneity among the studies ( I 2 =0%; P =.87), which allowed for analysis using a fixed-effect model. Meta-analysis found that mHealth app–based interventions significantly improved agility (SMD –0.35, 95% CI –0.61 to –0.10; P =.006; Figure 12 [ 36 , 42 , 44 ]).
Of the 28 studies, 3 (11%) reported the impact of mHealth app–based interventions on flexibility. Heterogeneity tests revealed homogeneity in the studies ( I 2 =52%; P =.12) and were conducted using a random effect model. There were no significant differences in flexibility between the control and intervention groups (SMD 0.09, 95% CI –0.23 to –0.41; P =.58; Figure 13 [ 42 , 44 , 47 ]).

Sensitivity Analysis
In this study, Stata 16.0 was used to conduct sensitivity analyses on TPA, SB, MVPA, and BMI for evaluating the robustness and reliability of the results. The sensitivity analysis results demonstrated that excluding any single study did not impact the effect size of the mHealth app–based intervention in outcomes such as TPA, SB, and MVPA ( Multimedia Appendices 2 - 4 ), which indicates the robustness and reliability of the study results. In terms of BMI, sensitivity analyses identified 1 study as an outlier [ 37 ] ( Multimedia Appendix 5 ). Removing this study altered the overall effect size, indicating that the study results were not sufficiently robust (SMD 0.03 cm, 95% CI –0.41 to –0.46 cm; P =.90) and should be interpreted with caution.
Subgroup Analyses
Subgroup analyses were conducted to investigate the potential sources of heterogeneity and moderating effects, which considered factors such as age, types of apps, theoretical paradigm, number or type of BCT clusters, and intervention duration. The primary outcome indicators included TPA, BMI, SB, and MVPA. Sensitivity analysis, subgroup analysis, and assessment of publication bias were not conducted for the remaining outcome indicators due to the limited number of included studies.
Subgroup Analyses on the Effect of mHealth App–Based Interventions on TPA
The results of subgroup analyses investigating the impact of mHealth app–based interventions on TPA are presented in Multimedia Appendix 6 . Subgroup analyses indicated no significant difference in types of intervention, theoretical paradigm, and the number of BCT clusters concerning the improvement in TPA in children and adolescents.
Age-based subgroup analysis revealed significant positive effects of interventions for children in the 7-12 years group (SMD 0.42) and adolescents in the 13-18 years group (SMD 0.29) but negligible effect for the preschool children in the 3-6 years group (SMD –0.06).
Subgroup analysis based on types of apps revealed a significantly greater effect size for the commercial apps (SMD 0.51) compared to the research apps intervention (SMD 0.13). Substantial heterogeneity existed between these 2 groups ( I 2 =75%; P <.001), but the 95% CI for the effect size between the 2 groups overlapped.
Subgroup analysis based on intervention duration revealed a significant increase in TPA for the 2- to 4-week intervention (SMD 1.01) and 8- to 12-week intervention (SMD 0.23). Heterogeneity was observed between the 2 groups ( I 2 =75%; P <.001), but the 95% CI for the effect size between the 2 groups overlapped.
Subgroup Analyses on the Effect of mHealth App–Based Interventions on SB
The results of subgroup analyses on SB are presented in Multimedia Appendix 7 . Subgroup analyses showed no significant difference in intervention duration regarding the reduction of SB in children and adolescents.
Age-based subgroup analysis unveiled a notably larger effect size for interventions directed at children aged 7-12 years (SMD –3.78) compared to the group of preschool children aged 3-6 years (SMD –0.92). Heterogeneity was evident among the 2 groups ( I 2 =99%; P <.001), and the 95% CI for the effect size between the 2 groups did not overlap.
Subgroup analysis based on the types of apps demonstrated a significantly stronger effect size for research app interventions (SMD –1.38) than for commercial app interventions (SMD –0.35). Heterogeneity was observed between the 2 groups ( I 2 =98%; P <.001), but the 95% CI for the effect size between the 2 groups overlapped.
Subgroup analysis based on types of intervention revealed a significantly greater effect size for the concerted intervention (SMD –1.47) compared to the stand-alone apps intervention (SMD –0.45). Substantial heterogeneity existed between these 2 groups ( I 2 =98%; P <.001), but the 95% CI for the effect size between the 2 groups overlapped.
Subgroup analyses based on the theoretical paradigm showed a greater effect size for interventions based on the SCT (SMD –0.64) than for interventions based on combination of SCT and other theories (SMD –2.18) and interventions solely based on SRT (SMD 0.05). Substantial heterogeneity existed between these 2 groups ( I 2 =98%; P <.001), but the 95% CI for the effect size between the 2 groups overlapped.
Subgroup analysis based on the number of BCT clusters demonstrated a significant reduction in SB for interventions based on 7-10 BCT clusters (SMD –1.03) and interventions based on 4 BCT clusters (SMD –1.36), and the 95% CI for the effect size between the 2 groups overlapped.
Subgroup analysis based on intervention duration revealed a significant reduction in SB for the 20- to 48-week intervention (SMD –1.42) but had a negligible effect at the 8- to 12-week intervention (SMD –0.63).
Subgroup Analyses on the Effect of mHealth App–Based Interventions on MVPA
The results of subgroup analyses on MVPA are shown in Multimedia Appendix 8 . Subgroup analyses revealed no significant difference in type of apps, types of intervention, and the number of BCT clusters in improving MVPA in children and adolescents, which were not moderators of the effect of mHealth app interventions.
Age-based subgroup analyses unveiled a notably larger effect size for interventions directed at adolescents aged 13-18 years (SMD 0.42) compared to the group of preschool children aged 3-6 years (SMD –0.05) and children aged 7-12 years (SMD 0.11). Heterogeneity was evident among the 2 groups ( I 2 =68%; P <.001), but the 95% CI for the effect size between the 2 groups overlapped.
Subgroup analyses based on theoretical paradigm demonstrated a significant increase in MVPA for SDT-based intervention (SMD 1.03), while no significant effect was observed for SCT (SMD –0.06), combination of SCT and other theories (SMD 0.12), and SRT (SMD –0.14).
Subgroup analysis based on intervention duration revealed a significant increase in MVPA for the 2- to 4-week intervention (SMD 1.42) but negligible effect for the 8- to 12-week intervention (SMD –0.01) and 20- to 48-week intervention (SMD 0.05).
Subgroup Analyses on the Effect of mHealth App–Based Interventions on BMI
The results of the subgroup analyses based on BMI are shown in Multimedia Appendix 9 . Subgroup analyses revealed no significant difference in type of apps, theoretical paradigm, and the number of BCT clusters in decreasing BMI in children and adolescents.
Age-based subgroup analysis revealed a significant decrease in BMI for interventions targeting children aged 7-12 years (WMD –0.59), but no significant difference was observed for adolescents aged 13-18 years (WMD 0.03).
Subgroup analysis based on types of intervention revealed a significantly greater effect size for the concerted intervention (WMD –0.59) compared to the stand-alone apps intervention (WMD 0.34), but the 95% CI for the effect size between the 2 groups overlapped.
Subgroup analysis based on intervention duration revealed a significant reduction in BMI for the 20- to 48-week intervention (WMD –0.57) and the 8- to 12-week intervention (WMD 0.33), and the former showed a clearly superior effect.
Meta-Regression
We conducted meta-regressions for TPA, BMI, SB, and MVPA, focusing on statistically significant moderators identified in the subgroup analyses. The results of the meta-regression for TPA ( P =.03, 95% CI –1.127 to –0.082) and MVPA ( P =.045, 95% CI –2.052 to –0.033) revealed that intervention duration had a potential moderating effect on high heterogeneity; no statistical differences were found for other variables acting as moderators. In the case of BMI, meta-regression results indicated that age ( P =.04, 95% CI 0.041-1.473) and types of intervention ( P =.02, 95% CI –1.648 to –0.205) could be potential moderators for high heterogeneity, whereas other factors did not significantly contribute to explaining high heterogeneity.
Reporting Biases
Funnel plots were used to assess publication bias in the effects of mHealth app–based interventions on TPA, SB, BMI, and MVPA in children and adolescents. The funnel plots exhibited mostly symmetrical patterns in the 4 studies ( Multimedia Appendices 10 - 13 ). In addition, Egger test was performed for TPA ( t 20 =0.01; P =.99), SB ( t 13 =–0.135; P =.20), MVPA ( t 13 =1.22; P =.25), and BMI ( t 12 =–0.07; P =.95), and the results suggested no significant publication bias ( Multimedia Appendices 14 - 17 ).
Principal Findings
We conducted a systematic review and meta-analysis to assess the effectiveness of mHealth app–based interventions in promoting PA and enhancing PF in children and adolescents. This study also examined the potential moderators that may influence the efficacy of these interventions. The findings of this study suggest that mHealth app–based interventions may yield positive effects on TPA, SB, BMI, agility, and muscle strength in children and adolescents. However, no significant effects were observed for MVPA, WC, CRF, muscular power and endurance, and flexibility. Age, theoretical paradigm, BCT clusters, types of intervention, types of apps, and intervention duration were identified as significant moderating factors associated with the increased effectiveness of mHealth app interventions on PA and PF, but the impact on effect size is not entirely consistent.
Overall Effect
The findings of this study indicated that mHealth app–based interventions increased TPA and reduced SB among children and adolescents but had no significant effect on MVPA. Our research findings represent a valuable expansion of recently published systematic reviews; however, they do not entirely align with the results of previous studies. A prior systematic review reported that mHealth-based interventions increased TPA levels and addressed physical inactivity in children and adolescents but did not lead to reduced SB and improved MVPA [ 12 , 13 ]. The inconsistency in the findings may be attributed to the cointervention effect of technologies such as SMS text messaging, wearable devices, web-based interventions, and smartphone apps [ 13 ]. Among these, smartphone app–based interventions might be the most effective strategy. The use of apps may contribute to increased SB time. Nevertheless, results of this study indicate that mHealth app–based interventions can effectively reduce SB in children and adolescents. Distinguishing whether the effect is from stand-alone app interventions or other strategies within concerted interventions is challenging. Subgroup analyses in this study demonstrated the superiority of concerted interventions over stand-alone app interventions. In conclusion, mHealth app–based interventions serve as a valuable adjunct in reducing SB in children and adolescents. Further concerted interventions, such as combining educational policies with mHealth apps interventions, are recommended.
The effectiveness of mHealth app–based interventions was influenced by age and intervention format. Notably, these interventions were more effective in improving PA levels among adolescents compared to children, and greater effects were observed when using mHealth apps than when using only SMS text messaging interventions [ 35 , 50 ]. These findings support the conclusions of this study, but some researchers hold differing views. The study by Trost and Brooks [ 50 ] indicated that an 8-week intervention with Moovosity apps (Kinetica Group Pty Ltd) improved proficiency in fundamental movement skills (FMS) but did not increase PA levels. The phenomenon may be associated with the types of design and goal setting of the apps. The apps in the study were primarily used to increase the FMS of children, which may have resulted in the activity of FMS replacing the original PA. As a result, the TPA of children was unchanged. Another study [ 35 ] identified that targeted app interventions would be effective in reducing SB in adolescents, which must be based on certain theoretical paradigms and BCT clusters. Gamification-based app interventions are also more favorable for increasing TPA and reducing SB levels in children and adolescents and must be combined with the theoretical paradigm, intervention duration, and features of apps [ 55 ]. In conclusion, the reasons for inconsistent intervention results may be related to population characteristics, types of apps, theoretical paradigm, BCT clusters, and intervention duration. Moreover, further research is needed in the future.
Another significant finding of the meta-analysis was that mHealth app–based interventions decreased BMI and improved muscle strength and agility among children and adolescents. However, no significant effects were observed for WC, CRF, muscular power and endurance, and flexibility. These findings are not entirely aligned with the results of previous studies. For example, a previous study reported an increase in PA levels among adolescents and significant reductions in sugary drink consumption and BMI following a 3-month intervention using Fitbit Flex apps (Fitbit, Inc) [ 35 ]. Meanwhile, a different study [ 29 ] indicated that an 18-month app intervention did not lead to significant reductions in BMI and WC, but it improved exercise capacity and reduced screen time. Several potential factors may contribute to the inconsistent results. First, the choice of outcome measure could be a contributing factor. BMI and WC may indicate distinct aspects of obesity; although BMI primarily assesses body size and shape, WC is a measure of abdominal obesity, leading to a weak correlation between the two [ 56 , 57 ]. Moreover, BMI tends to underestimate overweight prevalence when abdominal obesity is considered [ 57 ]. Hence, although mHealth app–based interventions show minor effects on BMI in children and adolescents, they might not induce significant changes in WC. Nonetheless, such interventions can still yield favorable outcomes. Another possible reason for the inconsistency in interventions could be related to the age of the population under study. Some studies have shown that mHealth app–based interventions are effective in reducing body weight and BMI in individuals aged ≥45 years, but they are less effective in children and adolescents [ 58 ]. In addition, our meta-regression analysis revealed that age significantly influences the effectiveness of app - based interventions. This result could be attributed to the fact that children and adolescents are in a period of rapid growth and development, and the effects of the interventions may be masked by the significant changes in height and weight during this stage.
In terms of muscular strength, power, and endurance, previous studies have shown that a 6-month intervention using mHealth apps and trackers resulted in increased muscle strength among children and adolescents but did not have a significant effect on CRF [ 53 ]; Stasiak et al [ 42 ] discovered that a daily conversational agent intervention, using a mobile app for adolescents with obesity, enhanced subjects’ muscular fitness, with no discernible difference between the intervention and control groups. Conversely, other studies have indicated that interventions using AIMFIT apps can positively impact maximal oxygen uptake [ 20 ]; Mateo-Orcajada et al [ 47 ] found that a 10-week after-school intervention for adolescents using mobile step-tracking apps significantly enhanced subjects’ upper limb strength, hamstring and lower back flexibility, explosive power of the lower limbs, as well as abdominal muscular strength and endurance. Various factors can influence the effectiveness of interventions, including subject characteristics, intervention dosage, engagement levels, and willingness to use apps. Some of the studies included in this work involved participants who were patients with cancer [ 53 ] or children with overweight and obesity [ 42 ], and this factor may have influenced the outcomes of the intervention. Furthermore, low user willingness to engage with apps may reduce PA engagement, which consequently impacts the effectiveness of the intervention. Finally, insufficient dosage of mHealth app–based interventions has been associated with a small effect size on TPA in children and adolescents and has shown no increase in MVPA levels. This insufficiency in dosage may explain the lack of intervention effects observed in children.
Moderating Variables on the Effects of mHealth App–Based Interventions for PA and PF
The study results revealed that age moderates the effects of mHealth app interventions on TPA, MVPA, SB, and BMI. Subgroup analysis indicated that mHealth app–based interventions significantly increased TPA and MVPA and reduced SB in children and adolescents aged 7-18 years; increased TPA and MVPA in adolescents aged 7-18 years; and only reduced SB in children aged 3-6 years. It is noteworthy that previous studies have indicated that mHealth app–based interventions did not effectively decrease SB in children and adolescents. This observation may stem from the fact that the use of apps may result in increased SB time, consequently reallocating the time resources of children and adolescents [ 12 ]. However, the findings of this study do not support this conclusion. A separate study reported that the intervention using Fit Survivor apps on SB in adolescents was ineffective, which is possibly due to the characteristics of the population; notably, most participants in these studies had cancer, which may require a longer period of SB to observe significant changes [ 53 ]. The findings regarding BMI indicate that parent-focused mHealth app–based interventions have not been successful in reducing BMI among children and adolescents [ 29 ], somewhat not entirely consistent with the findings of this study. Our meta-regression analysis revealed that age significantly influences the effectiveness of app-based interventions.
This phenomenon may be closely associated with willingness of users and their behavioral intention to use apps. Individual differences, such as age and gender, play a crucial role in moderating willingness and intention of users. In general, behavioral habits and expected performance serve as predictors of willingness and intention of users [ 59 ], and age can increase the frequency of use by moderating behavioral habit and performance [ 60 ], which in turn have an influence on SB. In addition, most of the app intervention is parenting-focused intervention among preschoolers [ 61 ]. The successful implementation of interventions relies on parental involvement, thus parental attitudes significantly influence the participation of preschoolers aged 3-6 years, potentially impacting the efficacy of mHealth app interventions [ 13 ]. In the case of children and adolescents aged 7-18 years, increasing age correlates with improved expected performance [ 62 ]. In adolescents, this performance expectation is closely linked to user intention, bolstering their willingness to engage with apps. However, older adolescents, who prioritize academic achievement, may exhibit reduced effectiveness in app interventions [ 63 ]. In conclusion, the effects of mHealth app–based interventions may vary across different age groups. However, only 4 studies included subjects aged 3-6 years, potentially affecting the generalizability of the findings. Therefore, further validation through a larger number of high-quality studies is warranted to strengthen the conclusions.
Types of Intervention
Concerning types of interventions, there is a lack of systematic reviews analyzing the impacts of stand-alone apps and concerted interventions on PA and PF, including their distinctions. Subgroup analysis revealed that concerted interventions significantly increased TPA and reduced SB and BMI in children and adolescents, whereas stand-alone app interventions had only a modest positive effect on TPA with a small effect size. The findings diverge somewhat from prior research. Some studies indicate that interventions using COOL Passport apps or a combination of COOL Passport apps and game-based interactive platforms do not enhance PA in youth with congenital heart disease [ 40 ]. Conversely, other studies suggest that mobile apps can increase TPA while decreasing SB and BMI in youth [ 46 , 47 ]. This phenomenon may be associated with behavior change interventions. Effective behavior change interventions can enhance health status and reduce health care spending, with theory-based interventions often yielding superior results [ 64 ]. Hence, interventions based on the high-scoring theoretical paradigm, or in combination with other specific high-scoring strategies, are more likely to yield positive outcomes [ 65 ]. In summary, interventions integrating apps with high-scoring strategies are probably more effective than stand-alone apps, although the optimal number and type of strategies remain undetermined.
Theoretical Paradigm
Effective behavior change interventions can improve health status and reduce health care spending, and theory-based interventions generally yielded superior results [ 64 ]. Currently, mHealth app interventions use multiple types of BCT that have been shown to be effective in influencing intervention outcomes [ 21 ]. Of the 28 studies, 8 (29%) did not use theoretical frameworks in their mHealth app interventions [ 31 , 36 , 37 , 45 , 47 , 49 , 50 , 52 ], while the remaining 20 (71%) studies were grounded in theoretical paradigms such as SCT, SDT, and SRT.
The most commonly used theoretical paradigms in the research are SCT and SDT, and interventions based on these frameworks have demonstrated positive effects on TPA, MVPA, and SB in children and adolescents. Subgroup analysis revealed that interventions based on SDT significantly increased TPA and MVPA in children and adolescents, while interventions based on SCT significantly reduced SB in this population. Previous studies have consistently shown that interventions based on SCT effectively improve PA among adolescents, which aligns with the findings of this study [ 54 ]. SCT is commonly used to elucidate the mechanisms underlying the improvement in PA through behavior change interventions. SCT posits that PA is influenced by personal, social, and environmental factors. Personal factors, including self-efficacy, self-management, and expected performance, play a crucial role in enhancing PA and reducing SB. mHealth apps can effectively promote the increase in PA and the reduction in SB by modifying these factors [ 66 ].
SDT is frequently used to account for variations in individual behavior resulting from differences in intrinsic and extrinsic motivation. Intrinsic motivation, which is a crucial predictor of PA levels, is lacking in approximately 40% of Europeans; this situation leads to failure to meet the recommended PA levels [ 67 ]. In contrast to extrinsic motivation, intrinsic motivation drives the initiation and sustained engagement in individual behaviors. Thus, interventions grounded in SDT can effectively enhance leisure-time PA and PA in physical education classes and reduce SB among adolescents by fostering intrinsic motivation [ 68 ]. Furthermore, interventions using mHealth apps based on the health belief model and theory of planned behavior may yield positive effects on increasing PA in children and adolescents. However, which theory yields optimal intervention effects requires further investigation. In conclusion, interventions based on SCT or SDT within mHealth apps may likely yield more effective results. However, due to the limited number of included studies, further validation through a larger volume of high-quality research is necessary to bolster these conclusions.
BCT Clusters
BCT clusters represent specific implementation strategies in behavior change interventions. Previous studies have demonstrated that interventions incorporating a specific number of BCT clusters are more effective in promoting the PA of users. However, further investigation is needed to determine the optimal combination of the number and type of BCT clusters to achieve optimal intervention effects [ 69 ].
The BCT clusters that emerged frequently in this study were feedback and monitoring (n=22), goals and planning (n=19), shaping knowledge (n=12), and social support (n=11). A previous study [ 70 ] focusing on app interventions targeting PA and SB in adults also identified feedback and monitoring as well as goals and planning as the most commonly used BCT clusters. Another study [ 16 ] also highlighted the positive effects of mHealth app–based interventions on PA and SB, particularly when specific BCT clusters such as goals and planning, feedback and monitoring, and social support were implemented. Consequently, specific BCT clusters, including goals and planning, feedback, and monitoring, play a crucial role in influencing the effectiveness of mHealth app–based interventions, ensuring increased TPA, decreased SB, and reduced BMI by enhancing the willingness and engagement of users [ 71 ].
Limited research exists on the impact of varying numbers of BCT clusters implemented on PA and PF in children and adolescents. In this study, we discovered that mHealth app–based interventions incorporating 4 and 7-10 BCT clusters resulted in a significant reduction in SB among child adolescents, and the latter showed a greater effect size than the former. Conroy et al [ 72 ] reported a mean of 4.2 for the use of BCT in app. The number of studies included in the analysis varies, and most studies used 4 BCT clusters, which is slightly lower than the number of BCT used in previous studies. This discrepancy may be attributed to the characteristics of the subjects [ 73 ]. Moreover, more BCT clusters in mHealth apps do not necessarily lead to better outcomes. Excessive use of BCT can decrease the willingness of users and their frequency of app use [ 74 ]. Thus, further research is needed to determine the optimal number of BCT clusters.
This phenomenon appears to be strongly associated with the willingness of users and their engagement in using apps. Feedback and monitoring, which involves design patterns enabling users to track their performance or status, play a crucial role in enhancing the trust, motivation, and engagement with the app of users. Consequently, this situation leads to the effective promotion of PA levels and reduction in BMI [ 75 ]. Similarly, goals and planning, which involve planned behaviors and the conversion of the intentions of users into actionable steps through self-regulation and self-efficacy, have been shown to improve PA and reduce BMI [ 76 ].
Types of Apps and Intervention Duration
The available evidence suggests that commercial apps did not yield improvements in PA or reductions in SB among children and adolescents [ 20 , 31 - 33 , 35 ]. However, Jasmine et al [ 77 ] found that integrating commercial apps with web-based social networking platforms was effective in improving PA. However, a certain use frequency was needed, and a significant correlation between frequency of use and PA was observed. Our study discovered that research apps significantly reduced SB, while commercial apps increased TPA in children and adolescents, but the effect size was small. However, the effect size was small for commercial apps, which contrasts with our expectations. This discrepancy may be attributed to the design and characteristics of the apps. Commercial app developers often prioritize user interface simplification and the inclusion of complex features to enhance app engagement. However, they may overlook incorporating theoretical frameworks and BCT clusters that are closely associated with intervention effectiveness [ 78 ].
An intriguing finding emerged regarding the duration of the interventions. The 2- to 4-week and 8- to 12-week interventions significantly increased TPA, but the former demonstrated superior effectiveness to the latter; 8- to 12-week interventions significantly increased MVPA. Furthermore, the 20- to 48-week intervention significantly reduced SB and BMI in children and adolescents. This finding aligns with the results of previous studies that short-term mHealth app interventions effectively increased the PA of children, while longer interventions yielded diminished outcomes [ 40 , 51 ]. The results of this study support the notion that intervention effects are closely linked to the willingness of participants to engage. The reason is that shortly after engaging in an mHealth app–based intervention, children and adolescents will experience a sensitive attraction period [ 79 ]; prolonged interventions may lead to diminished interest and compliance, thereby weakening the impact. Another plausible explanation is that behavior change interventions rooted in theoretical frameworks yield better outcomes, but they require sufficient time for users to develop habitual behaviors conducive to lasting positive changes, and interventions targeting SB and BMI take even longer. In addition, our meta-regression analysis revealed that intervention duration significantly influences the effectiveness of app - based interventions.
Limitations
This study has several limitations. The search was restricted to English literature, which may introduce language bias and affect the reliability of the findings. Only a limited number of published articles were included, which potentially omit unpublished manuscripts and other sources (eg, conference papers and dissertations) that could contribute to publication bias. The outcome measures used in this study had inconsistent units, and the interpretation of the results using SMD as effect indicators requires caution. PF encompasses multiple dimensions, and different studies focused on various subdimensions.
Most studies (21/28, 75%) encompassed multiple metrics, and some studies (11/28, 39%) were not primarily designed to enhance PA and PF. These factors could have biased the results, potentially limiting the effect sizes and hindering a full reflection of the true effects in this study. Despite our extensive meta-regression and subgroup analyses, only age and intervention period demonstrated significant associations. Heterogeneity for some outcome metrics remained high, and the 95% CIs for the effect size overlapped between groups. Consequently, caution is advised when interpreting partial conclusions from subgroups. Our findings indicate heterogeneity in some studies, and the source of this heterogeneity remains unclear. The effectiveness of mHealth app–based interventions may vary among children and adolescents based on demographics (eg, ethnic backgrounds, regions, genders, and BMI), economic levels, intervention models (parent centered vs child centered), and the degree of app individualization. These factors could contribute to the observed heterogeneity. However, due to limitations stemming from the number and characteristics of included studies, these factors were not analyzed in this study. In conclusion, the findings of this systematic review and meta-analysis should be interpreted cautiously.
Conclusions
The findings from a systematic review and meta-analysis indicate that mHealth app–based interventions hold significant potential as a therapeutic strategy for increasing TPA levels, reducing BMI and SB, and improving agility and muscle strength among children and adolescents. However, these interventions insignificantly affected MVPA, WC, CRF, muscular power and endurance, and flexibility, which are crucial for promoting PA and enhancing PF. Age, app types, types of intervention, theoretical paradigms, BCT clusters, and intervention duration emerged as important moderating variables that influence the effectiveness of mHealth app–based interventions. These moderating effects should be considered during the design, preparation, and promotion of interventions.
Considering the potential benefits of using mHealth apps, future research should explore the combination of different types and quantities of mHealth app–based interventions to determine the optimal approach for increasing PA levels and improving PF in children and adolescents. Furthermore, future studies could investigate the impact of additional influences on the intervention effectiveness of mHealth app interventions, such as demographics characteristics, economic levels, and intervention models.
Acknowledgments
The authors would like to thank the staff and faculty at the Institute of Sports Medicine and Health at Chengdu Sport University, which offered the funding support and the place to conduct the study. This study was funded by the National Social Science Fund (grant 22BTY063), the Key R&D Projects of the Sichuan Provincial Science and Technology Plan Project (grant 2022YFS0053), the Soft Science Project of Sichuan Provincial Department of Science and Technology (grant 2022JDR0314), the Key Project of Sichuan Provincial Philosophy and Social Science Foundation (grant CJJ23ND77), the Sports Medicine Key Laboratory of Sichuan Province and Sports Medicine Key Laboratory of State Sport General Administration (grant 2024-A002), and the “14th Five Year Plan” Scientific Research and Innovation Team of Chengdu Sport University (grant 23CXTD02).
Authors' Contributions
JWW designed the study protocol and drafted the report; ZZ, ZS, and JF searched the literature, analyzed the data, and interpreted the results; YJ, ZLG, and WDC contributed to the study protocol and reviewed the manuscript; and XL reviewed and revised the manuscript.
Conflicts of Interest
None declared.
Literature search strategy.
Sensitivity analyses results on total physical activity.
Sensitivity analyses results on sedentary behavior.
Sensitivity analyses results on moderate to vigorous physical activity.
Sensitivity analyses results on BMI.
Summary of subgroup analysis results of mobile health app–based interventions on total physical activity.
Summary of subgroup analysis results of mobile health app–based interventions on sedentary behavior.
Summary of subgroup analysis results of mobile health app–based interventions on moderate to vigorous physical activity.
Summary of subgroup analysis results of mobile health app–based interventions on BMI.
Funnel plot of total physical activity.
Funnel plot of sedentary behavior.
Funnel plot of BMI.
Funnel plot of moderate to vigorous physical activity.
Egger test results on total physical activity.
Egger test results sedentary behavior.
Egger test results moderate to vigorous physical activity.
Egger test results BMI.
PRISMA 2020 Checklist.
- Singh S, Roy D, Sinha K, Parveen S, Sharma G, Joshi G. Impact of COVID-19 and lockdown on mental health of children and adolescents: a narrative review with recommendations. Psychiatry Res. Nov 2020;293:113429. [ FREE Full text ] [ CrossRef ] [ Medline ]
- Guthold R, Stevens GA, Riley LM, Bull FC. Global trends in insufficient physical activity among adolescents: a pooled analysis of 298 population-based surveys with 1·6 million participants. Lancet Child Adolesc Health. Jan 2020;4(1):23-35. [ FREE Full text ] [ CrossRef ] [ Medline ]
- Neil-Sztramko SE, Caldwell H, Dobbins M. School-based physical activity programs for promoting physical activity and fitness in children and adolescents aged 6 to 18. Cochrane Database Syst Rev. Sep 23, 2021;9(9):CD007651. [ FREE Full text ] [ CrossRef ] [ Medline ]
- Cachón-Zagalaz J, Sánchez-Zafra M, Sanabrias-Moreno D, González-Valero G, Lara-Sánchez AJ, Zagalaz-Sánchez ML. Systematic review of the literature about the effects of the COVID-19 pandemic on the lives of school children. Front Psychol. 2020;11:569348. [ FREE Full text ] [ CrossRef ] [ Medline ]
- Basterfield L, Burn NL, Galna B, Batten H, Goffe L, Karoblyte G, et al. Changes in children's physical fitness, BMI and health-related quality of life after the first 2020 COVID-19 lockdown in England: a longitudinal study. J Sports Sci. May 2022;40(10):1088-1096. [ CrossRef ] [ Medline ]
- Ortega FB, Ruiz JR, Castillo MJ, Sjöström M. Physical fitness in childhood and adolescence: a powerful marker of health. Int J Obes (Lond). Jan 2008;32(1):1-11. [ CrossRef ] [ Medline ]
- Lämmle L, Worth A, Bös K. Socio-demographic correlates of physical activity and physical fitness in German children and adolescents. Eur J Public Health. Dec 2012;22(6):880-884. [ CrossRef ] [ Medline ]
- Júdice PB, Silva AM, Berria J, Petroski EL, Ekelund U, Sardinha LB. Sedentary patterns, physical activity and health-related physical fitness in youth: a cross-sectional study. Int J Behav Nutr Phys Act. Mar 04, 2017;14(1):25. [ FREE Full text ] [ CrossRef ] [ Medline ]
- Krebs P, Duncan DT. Health app use among US mobile phone owners: a national survey. JMIR Mhealth Uhealth. Nov 04, 2015;3(4):e101. [ FREE Full text ] [ CrossRef ] [ Medline ]
- Brannon EE, Cushing CC. A systematic review: is there an app for that? Translational science of pediatric behavior change for physical activity and dietary interventions. J Pediatr Psychol. May 2015;40(4):373-384. [ CrossRef ] [ Medline ]
- Rising CJ, Jensen RE, Moser RP, Oh A. Characterizing the US population by patterns of mobile health use for health and behavioral tracking: analysis of the national cancer institute's health information national trends survey data. J Med Internet Res. May 14, 2020;22(5):e16299. [ FREE Full text ] [ CrossRef ] [ Medline ]
- Baumann H, Fiedler J, Wunsch K, Woll A, Wollesen B. mHealth interventions to reduce physical inactivity and sedentary behavior in children and adolescents: systematic review and meta-analysis of randomized controlled trials. JMIR Mhealth Uhealth. May 11, 2022;10(5):e35920. [ FREE Full text ] [ CrossRef ] [ Medline ]
- He Z, Wu H, Yu F, Fu J, Sun S, Huang T, et al. Effects of smartphone-based interventions on physical activity in children and adolescents: systematic review and meta-analysis. JMIR Mhealth Uhealth. Feb 01, 2021;9(2):e22601. [ FREE Full text ] [ CrossRef ] [ Medline ]
- Byambasuren O, Sanders S, Beller E, Glasziou P. Prescribable mHealth apps identified from an overview of systematic reviews. NPJ Digit Med. 2018;1:12. [ FREE Full text ] [ CrossRef ] [ Medline ]
- Higgins JP. Smartphone applications for patients' health and fitness. Am J Med. Jan 2016;129(1):11-19. [ CrossRef ] [ Medline ]
- Yerrakalva D, Yerrakalva D, Hajna S, Griffin S. Effects of mobile health app interventions on sedentary time, physical activity, and fitness in older adults: systematic review and meta-analysis. J Med Internet Res. Nov 28, 2019;21(11):e14343. [ FREE Full text ] [ CrossRef ] [ Medline ]
- Domin A, Spruijt-Metz D, Theisen D, Ouzzahra Y, Vögele C. Smartphone-based interventions for physical activity promotion: scoping review of the evidence over the last 10 years. JMIR Mhealth Uhealth. Jul 21, 2021;9(7):e24308. [ FREE Full text ] [ CrossRef ] [ Medline ]
- Direito A, Carraça E, Rawstorn J, Whittaker R, Maddison R. mHealth technologies to influence physical activity and sedentary behaviors: behavior change techniques, systematic review and meta-analysis of randomized controlled trials. Ann Behav Med. Apr 2017;51(2):226-239. [ CrossRef ] [ Medline ]
- Litman L, Rosen Z, Spierer D, Weinberger-Litman S, Goldschein A, Robinson J. Mobile exercise apps and increased leisure time exercise activity: a moderated mediation analysis of the role of self-efficacy and barriers. J Med Internet Res. Aug 14, 2015;17(8):e195. [ FREE Full text ] [ CrossRef ] [ Medline ]
- Direito A, Jiang Y, Whittaker R, Maddison R. Apps for IMproving FITness and increasing physical activity among young people: the AIMFIT pragmatic randomized controlled trial. J Med Internet Res. Aug 27, 2015;17(8):e210. [ FREE Full text ] [ CrossRef ] [ Medline ]
- Fiedler J, Eckert T, Wunsch K, Woll A. Key facets to build up eHealth and mHealth interventions to enhance physical activity, sedentary behavior and nutrition in healthy subjects - an umbrella review. BMC Public Health. Oct 23, 2020;20(1):1605. [ FREE Full text ] [ CrossRef ] [ Medline ]
- Yang Q, Van Stee SK. The comparative effectiveness of mobile phone interventions in improving health outcomes: meta-analytic review. JMIR Mhealth Uhealth. Apr 03, 2019;7(4):e11244. [ FREE Full text ] [ CrossRef ] [ Medline ]
- Michie S, Richardson M, Johnston M, Abraham C, Francis J, Hardeman W, et al. The behavior change technique taxonomy (v1) of 93 hierarchically clustered techniques: building an international consensus for the reporting of behavior change interventions. Ann Behav Med. Aug 2013;46(1):81-95. [ FREE Full text ] [ CrossRef ] [ Medline ]
- Jüni P, Egger M. PRISMAtic reporting of systematic reviews and meta-analyses. Lancet. Oct 10, 2009;374(9697):1221-1223. [ CrossRef ] [ Medline ]
- Higgins JP, Altman DG, Gøtzsche PC, Jüni P, Moher D, Oxman AD, Cochrane Bias Methods Group, et al. Cochrane Statistical Methods Group. The Cochrane Collaboration's tool for assessing risk of bias in randomised trials. BMJ. Oct 18, 2011;343:d5928. [ FREE Full text ] [ CrossRef ] [ Medline ]
- Nyström CD, Sandin S, Henriksson P, Henriksson H, Trolle-Lagerros Y, Larsson C, et al. Mobile-based intervention intended to stop obesity in preschool-aged children: the MINISTOP randomized controlled trial. Am J Clin Nutr. Jun 2017;105(6):1327-1335. [ FREE Full text ] [ CrossRef ] [ Medline ]
- Higgins JP, Thomas J, Chandler J, Cumpston MJ, Li T, Page M, et al. Cochrane Handbook for Systematic Reviews of Interventions. Hoboken, NJ. John Wiley & Sons; 2019.
- Garde A, Umedaly A, Abulnaga SM, Junker A, Chanoine JP, Johnson M, et al. Evaluation of a novel mobile exergame in a school-based environment. Cyberpsychol Behav Soc Netw. Mar 2016;19(3):186-192. [ CrossRef ] [ Medline ]
- Lubans DR, Smith JJ, Plotnikoff RC, Dally KA, Okely AD, Salmon J, et al. Assessing the sustained impact of a school-based obesity prevention program for adolescent boys: the ATLAS cluster randomized controlled trial. Int J Behav Nutr Phys Act. Aug 20, 2016;13:92. [ FREE Full text ] [ CrossRef ] [ Medline ]
- Smith JJ, Morgan PJ, Plotnikoff RC, Dally KA, Salmon J, Okely AD, et al. Smart-phone obesity prevention trial for adolescent boys in low-income communities: the ATLAS RCT. Pediatrics. Sep 2014;134(3):e723-e731. [ CrossRef ] [ Medline ]
- Fernandez-Luque L, Singh M, Ofli F, Mejova YA, Weber I, Aupetit M, et al. Erratum to: implementing 360° quantified self for childhood obesity: feasibility study and experiences from a weight loss camp in Qatar. BMC Med Inform Decis Mak. May 12, 2017;17(1):62. [ FREE Full text ] [ CrossRef ] [ Medline ]
- Pyky R, Koivumaa-Honkanen H, Leinonen AM, Ahola R, Hirvonen N, Enwald H, et al. Effect of tailored, gamified, mobile physical activity intervention on life satisfaction and self-rated health in young adolescent men: a population-based, randomized controlled trial (MOPO study). Comput Human Behav. Jul 2017;72:13-22. [ CrossRef ]
- Gaudet J, Gallant F, Bélanger M. A bit of fit: minimalist intervention in adolescents based on a physical activity tracker. JMIR Mhealth Uhealth. Jul 06, 2017;5(7):e92. [ FREE Full text ] [ CrossRef ] [ Medline ]
- Mendoza JA, Baker KS, Moreno MA, Whitlock K, Abbey-Lambertz M, Waite A, et al. A Fitbit and Facebook mHealth intervention for promoting physical activity among adolescent and young adult childhood cancer survivors: a pilot study. Pediatr Blood Cancer. Dec 2017;64(12). [ CrossRef ] [ Medline ]
- Chen J, Guedes CM, Lung AE. Smartphone-based healthy weight management intervention for Chinese American adolescents: short-term efficacy and factors associated with decreased weight. J Adolesc Health. Apr 2019;64(4):443-449. [ CrossRef ] [ Medline ]
- Kahana R, Kremer S, Dekel Dahari M, Kodesh E. The effect of incorporating an exergame application in a multidisciplinary weight management program on physical activity and fitness indices in children with overweight and obesity. Children (Basel). Dec 29, 2021;9(1):18. [ FREE Full text ] [ CrossRef ] [ Medline ]
- Liu Z, Gao P, Gao AY, Lin Y, Feng XX, Zhang F, et al. Effectiveness of a multifaceted intervention for prevention of obesity in primary school children in China: a cluster randomized clinical trial. JAMA Pediatr. Jan 01, 2022;176(1):e214375. [ FREE Full text ] [ CrossRef ] [ Medline ]
- Ridgers ND, Timperio A, Ball K, Lai SK, Brown H, Macfarlane S, et al. Effect of commercial wearables and digital behaviour change resources on the physical activity of adolescents attending schools in socio-economically disadvantaged areas: the RAW-PA cluster-randomised controlled trial. Int J Behav Nutr Phys Act. Apr 12, 2021;18(1):52. [ FREE Full text ] [ CrossRef ] [ Medline ]
- Likhitweerawong N, Boonchooduang N, Kittisakmontri K, Chonchaiya W, Louthrenoo O. Effectiveness of mobile application on changing weight, healthy eating habits, and quality of life in children and adolescents with obesity: a randomized controlled trial. BMC Pediatr. Nov 10, 2021;21(1):499. [ FREE Full text ] [ CrossRef ] [ Medline ]
- Lin PJ, Fanjiang YY, Wang JK, Lu CW, Lin KC, Cheong IM, et al. Long-term effectiveness of an mHealth-tailored physical activity intervention in youth with congenital heart disease: a randomized controlled trial. J Adv Nurs. Aug 2021;77(8):3494-3506. [ CrossRef ] [ Medline ]
- Seah ML, Koh KT. The efficacy of using mobile applications in changing adolescent girls’ physical activity behaviour during weekends. Eur Phys Educ Rev. Jun 17, 2020;27(1):113-131. [ CrossRef ]
- Stasinaki A, Büchter D, Shih CI, Heldt K, Güsewell S, Brogle B, et al. Effects of a novel mobile health intervention compared to a multi-component behaviour changing program on body mass index, physical capacities and stress parameters in adolescents with obesity: a randomized controlled trial. BMC Pediatr. Jul 09, 2021;21(1):308. [ FREE Full text ] [ CrossRef ] [ Medline ]
- Tugault-Lafleur CN, De-Jongh González O, Macdonald J, Bradbury J, Warshawski T, Ball GD, et al. Efficacy of the Aim2Be intervention in changing lifestyle behaviors among adolescents with overweight and obesity: randomized controlled trial. J Med Internet Res. Apr 25, 2023;25:e38545. [ FREE Full text ] [ CrossRef ] [ Medline ]
- Han X, Tian Z, Zhao M, Zhou Z. An intervention pattern of family parent-child physical activity based on a smartphone app for preschool children during COVID-19. Biomed Res Int. 2022;2022:2777079. [ FREE Full text ] [ CrossRef ] [ Medline ]
- Oh W, An Y, Min S, Park C. Comparative effectiveness of artificial intelligence-based interactive home exercise applications in adolescents with obesity. Sensors (Basel). Sep 28, 2022;22(19):7352. [ FREE Full text ] [ CrossRef ] [ Medline ]
- Staiano AE, Newton RL, Beyl RA, Kracht CL, Hendrick CA, Viverito M, et al. mHealth intervention for motor skills: a randomized controlled trial. Pediatrics. May 01, 2022;149(5):e2021053362. [ FREE Full text ] [ CrossRef ] [ Medline ]
- Mateo-Orcajada A, Abenza-Cano L, Albaladejo-Saura MD, Vaquero-Cristóbal R. Mandatory after-school use of step tracker apps improves physical activity, body composition and fitness of adolescents. Educ Inf Technol (Dordr). Jan 24, 2023.:1-32. [ FREE Full text ] [ CrossRef ] [ Medline ]
- Alexandrou C, Henriksson H, Henström M, Henriksson P, Delisle Nyström C, Bendtsen M, et al. Effectiveness of a smartphone app (MINISTOP 2.0) integrated in primary child health care to promote healthy diet and physical activity behaviors and prevent obesity in preschool-aged children: randomized controlled trial. Int J Behav Nutr Phys Act. Feb 21, 2023;20(1):22. [ FREE Full text ] [ CrossRef ] [ Medline ]
- Mateo-Orcajada A, Vaquero-Cristóbal R, Abenza-Cano L. Influence of Pokémon go playing style on physical activity and its effect on Kinanthropometry variables and body composition in adolescents. J Phys Act Health. Oct 01, 2023;20(10):934-947. [ CrossRef ] [ Medline ]
- Trost SG, Brookes DS. Effectiveness of a novel digital application to promote fundamental movement skills in 3- to 6-year-old children: a randomized controlled trial. J Sports Sci. Feb 2021;39(4):453-459. [ CrossRef ] [ Medline ]
- Garde A, Chowdhury M, Rollinson AU, Johnson M, Prescod P, Chanoine JP, et al. A multi-week assessment of a mobile exergame intervention in an elementary school. Games Health J. Feb 2018;7(1):1-8. [ CrossRef ] [ Medline ]
- Browne S, Kechadi MT, O'Donnell S, Dow M, Tully L, Doyle G, et al. Mobile health apps in pediatric obesity treatment: process outcomes from a feasibility study of a multicomponent intervention. JMIR Mhealth Uhealth. Jul 08, 2020;8(7):e16925. [ FREE Full text ] [ CrossRef ] [ Medline ]
- Devine KA, Viola A, Levonyan-Radloff K, Mackowski N, Bozzini B, Chandler A, et al. Feasibility of FitSurvivor: a technology-enhanced group-based fitness intervention for adolescent and young adult survivors of childhood cancer. Pediatr Blood Cancer. Sep 26, 2020;67(9):e28530. [ FREE Full text ] [ CrossRef ] [ Medline ]
- Liu J, Zeng M, Wang D, Zhang Y, Shang B, Ma X. Applying social cognitive theory in predicting physical activity among Chinese adolescents: a cross-sectional study with multigroup structural equation model. Front Psychol. 2021;12:695241. [ FREE Full text ] [ CrossRef ] [ Medline ]
- Khamzina M, Parab KV, An R, Bullard T, Grigsby-Toussaint DS. Impact of Pokémon Go on physical activity: a systematic review and meta-analysis. Am J Prev Med. Feb 2020;58(2):270-282. [ CrossRef ] [ Medline ]
- Tully L, Burls A, Sorensen J, El-Moslemany R, O'Malley G. Mobile health for pediatric weight management: systematic scoping review. JMIR Mhealth Uhealth. Jun 03, 2020;8(6):e16214. [ FREE Full text ] [ CrossRef ] [ Medline ]
- Booth ML, Hunter C, Gore CJ, Bauman A, Owen N. The relationship between body mass index and waist circumference: implications for estimates of the population prevalence of overweight. Int J Obes Relat Metab Disord. Aug 2000;24(8):1058-1061. [ CrossRef ] [ Medline ]
- Emberson MA, Lalande A, Wang D, McDonough DJ, Liu W, Gao Z. Effectiveness of smartphone-based physical activity interventions on individuals' health outcomes: a systematic review. Biomed Res Int. 2021;2021:6296896. [ FREE Full text ] [ CrossRef ] [ Medline ]
- Venkatesh V, Thong JY, Xu X. Consumer acceptance and use of information technology: extending the unified theory of acceptance and use of technology. MIS Q. 2012;36(1):157. [ CrossRef ]
- Schoeppe S, Alley S, Van Lippevelde W, Bray NA, Williams SL, Duncan MJ, et al. Efficacy of interventions that use apps to improve diet, physical activity and sedentary behaviour: a systematic review. Int J Behav Nutr Phys Act. Dec 07, 2016;13(1):127. [ FREE Full text ] [ CrossRef ] [ Medline ]
- Webster EK, Kracht CL, Newton Jr RL, Beyl RA, Staiano AE. Intervention to improve preschool children's fundamental motor skills: protocol for a parent-focused, mobile app-based comparative effectiveness trial. JMIR Res Protoc. Oct 20, 2020;9(10):e19943. [ FREE Full text ] [ CrossRef ] [ Medline ]
- Mateo-Orcajada A, Vaquero-Cristóbal R, Abenza-Cano L. Gender and academic year as moderators of the efficacy of mobile app interventions to promote physical activity in adolescents: a randomized controlled trial. Humanit Soc Sci Commun. Dec 22, 2023;10(1). [ FREE Full text ] [ CrossRef ]
- Morales-Vives F, Camps E, Dueñas JM. Predicting academic achievement in adolescents: the role of maturity, intelligence and personality. Psicothema. Feb 2020;32(1):84-91. [ CrossRef ] [ Medline ]
- Connell LE, Carey RN, de Bruin M, Rothman AJ, Johnston M, Kelly MP, et al. Links between behavior change techniques and mechanisms of action: an expert consensus study. Ann Behav Med. Jul 17, 2019;53(8):708-720. [ FREE Full text ] [ CrossRef ] [ Medline ]
- Hale K, Capra S, Bauer J. A framework to assist health professionals in recommending high-quality apps for supporting chronic disease self-management: illustrative assessment of type 2 diabetes apps. JMIR Mhealth Uhealth. Sep 14, 2015;3(3):e87. [ FREE Full text ] [ CrossRef ] [ Medline ]
- Elizabeth Morghen S, Robert WM. Pilot study of social cognitive theory variables as correlates of physical activity among adolescents with pediatric multiple sclerosis. Mult Scler Relat Disord. Jul 2020;42:102026. [ CrossRef ] [ Medline ]
- Ng JY, Ntoumanis N, Thøgersen-Ntoumani C, Deci EL, Ryan RM, Duda JL, et al. Self-determination theory applied to health contexts: a meta-analysis. Perspect Psychol Sci. Jul 2012;7(4):325-340. [ CrossRef ] [ Medline ]
- Nogg KA, Vaughn AA, Levy SS, Blashill AJ. Motivation for physical activity among U.S. adolescents: a self-determination theory perspective. Ann Behav Med. Mar 16, 2021;55(2):133-143. [ CrossRef ] [ Medline ]
- Hu L, Ping L. Can sports and fitness apps promote physical exercise? Evidence from a meta-analysis. China Sports Sci Technol. 2021;58(06):94-103. [ FREE Full text ] [ CrossRef ]
- Bardus M, van Beurden SB, Smith JR, Abraham C. A review and content analysis of engagement, functionality, aesthetics, information quality, and change techniques in the most popular commercial apps for weight management. Int J Behav Nutr Phys Act. Mar 10, 2016;13:35. [ FREE Full text ] [ CrossRef ] [ Medline ]
- Muellmann S, Forberger S, Möllers T, Zeeb H, Pischke CR. Effectiveness of eHealth interventions for the promotion of physical activity in older adults: a systematic review protocol. Syst Rev. Mar 16, 2016;5:47. [ FREE Full text ] [ CrossRef ] [ Medline ]
- Conroy DE, Yang CH, Maher JP. Behavior change techniques in top-ranked mobile apps for physical activity. Am J Prev Med. Jun 2014;46(6):649-652. [ CrossRef ] [ Medline ]
- Schoeppe S, Alley S, Rebar AL, Hayman M, Bray NA, Van Lippevelde W, et al. Apps to improve diet, physical activity and sedentary behaviour in children and adolescents: a review of quality, features and behaviour change techniques. Int J Behav Nutr Phys Act. Jun 24, 2017;14(1):83. [ FREE Full text ] [ CrossRef ] [ Medline ]
- Bondaronek P, Alkhaldi G, Slee A, Hamilton FL, Murray E. Quality of publicly available physical activity apps: review and content analysis. JMIR Mhealth Uhealth. Mar 21, 2018;6(3):e53. [ FREE Full text ] [ CrossRef ] [ Medline ]
- Vinnikova A, Lu L, Wei J, Fang G, Yan J. The use of smartphone fitness applications: the role of self-efficacy and self-regulation. Int J Environ Res Public Health. Oct 20, 2020;17(20):7639. [ FREE Full text ] [ CrossRef ] [ Medline ]
- Wang YS, Lin HH, Luarn P. Predicting consumer intention to use mobile service. Inf Syst J. Mar 02, 2006;16(2):157-179. [ FREE Full text ] [ CrossRef ]
- Petersen JM, Kemps E, Lewis LK, Prichard I. Associations between commercial app use and physical activity: cross-sectional study. J Med Internet Res. Jun 03, 2020;22(6):e17152. [ FREE Full text ] [ CrossRef ] [ Medline ]
- Desmet M, Franssen S, Varol T, Fillon A, Thivel D, Roefs A, et al. A smartphone application as a personalized treatment tool for adolescents with overweight: an explorative qualitative study. BMC Public Health. Apr 14, 2023;23(1):696. [ FREE Full text ] [ CrossRef ] [ Medline ]
- Egilsson E, Bjarnason R, Njardvik U. Usage and weekly attrition in a smartphone-based health behavior intervention for adolescents: pilot randomized controlled trial. JMIR Form Res. Feb 17, 2021;5(2):e21432. [ FREE Full text ] [ CrossRef ] [ Medline ]
Abbreviations
Edited by L Buis; submitted 01.08.23; peer-reviewed by M Hammersley, H Baumann, O Byambasuren, M Zhou; comments to author 13.09.23; revised version received 11.02.24; accepted 14.03.24; published 30.04.24.
©Jun-Wei Wang, Zhicheng Zhu, Zhang Shuling, Jia Fan, Yu Jin, Zhan-Le Gao, Wan-Di Chen, Xue Li. Originally published in JMIR mHealth and uHealth (https://mhealth.jmir.org), 30.04.2024.
This is an open-access article distributed under the terms of the Creative Commons Attribution License (https://creativecommons.org/licenses/by/4.0/), which permits unrestricted use, distribution, and reproduction in any medium, provided the original work, first published in JMIR mHealth and uHealth, is properly cited. The complete bibliographic information, a link to the original publication on https://mhealth.jmir.org/, as well as this copyright and license information must be included.
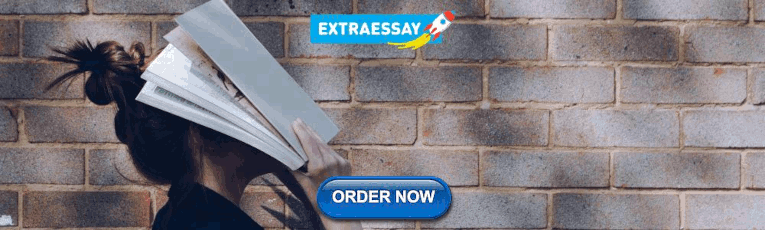
IMAGES
VIDEO
COMMENTS
Welcome to the Journal Index Copernicus value (ICV) : 73.15. Research & Reviews: Journal of Pharmaceutical Analysis (e-ISSN: 2320-0812) an International journal publishing quarterly (Electronic) on the aspect of Pharmaceutical Analysis for an effective scientific reading and public view with an aim to reach the world wide researchers.. The manuscript would be considered under the specific ...
The journal of the Xi'an Jiaotong University Journal of Pharmaceutical Analysis (JPA), launched in 2011, is an official journal of Xi'an Jiaotong University. JPA is a monthly peer-reviewed open access journal that publishes significant Original research articles, Review papers, Short communications, News, Research highlight, and Editorial in the field of Analysis of Pharmacy, broadly covering ...
Read the latest articles of Journal of Pharmaceutical Analysis at ScienceDirect.com, Elsevier's leading platform of peer-reviewed scholarly literature ... Research article Open access Analysis of docosanol using GC/MS: Method development, ... select article Application of microfluidic chip technology in pharmaceutical analysis: A review ...
Journal of Pharmaceutical Analysis (JPA) requests that chemical structures be prepared according to the guidelines below. ... Peer review This journal operates a double anonymized review process. All contributions will be initially assessed by the editor for suitability for the journal. ... Research data This journal encourages and enables you ...
Scope. Journal of Pharmaceutical Analysis (JPA) is a peer-reviewed open access journal for original research articles, review articles and short communications related to all aspects of pharmaceutical analysis. JPA was launched in 2011, and published bimonthly by Xi'an Jiaotong University. This journal is indexed in SCIE, Scopus, PubMed Central ...
VCA Rahul Kasar, Ashish Gogia, Kartik Shah. Research & Reviews: Journal of Pharmaceutical Analysis 2 (4) , 2013. 10. 2013. Development and Validation of a RP-HPLC Method for Simultaneous Estimation of Diacerein and Aceclofenac in Pharmaceutical Dosage Form. N Nyola. Journal of Pharmaceutical Analysis 1 (1), 8. , 2012.
ISSN 2320-0812 (Online) | Research and reviews: journal of pharmaceutical analysis. Skip to main content. Leave this field blank . Log In; Automatic login IP; PUBLISHERS' AREA DISCOVER ISSN ... Title proper: Research and reviews: journal of pharmaceutical analysis. Country: India. Medium: Online. Record information. Last modification date: 09 ...
Description Research & Reviews: Journal of Pharmaceutical Analysis (ISSN: 2320?0812) an online International journal publishing quarterly on the aspect of Pharmaceutical Analysis for an effective scientific reading and public view with an aim to reach the world wide researchers.
Volume 14, Issue 3, 2024. Abstract: Triterpenoids widely exist in nature, displaying a variety of pharmacological activities. Determining triterpenoids in different matrices, especially in biological samples holds great significance. High-performance liquid chromatography (HPLC) has become the predominant method for triterpenoids analysis due ...
Explore the latest full-text research PDFs, articles, conference papers, preprints and more on PHARMACEUTICAL ANALYSIS. Find methods information, sources, references or conduct a literature review ...
This review is aimed at focusing the role of various analytical instruments in the assay of pharmaceuticals and giving a thorough literature survey of the instrumentation involved in pharmaceutical analysis. The review also highlights the advancement of the techniques beginning from the older titrimetric method and reaching the advanced ...
Research & Reviews: Journal of Pharmaceutical Analysis (e-ISSN: 2320-0812, p-ISSN: 2347-2340) an International journal publishing quarterly (Electronic and Print version) on the aspect of Pharmaceutical Analysis for an effective scientific reading and public view with an aim to reach the world wide researchers. The manuscript would be ...
Research & Reviews: Journal of Pharmaceutical Analysis. Please submit your article using the form below. Note that all the fields are mandatory. Please also include the full abstract that will help the reviewers to rapidly decide if your article enters their domain of expertise. Also select one or several topics below to help the editors to ...
Research & Reviews: Journal of Pharmaceutical Analysis 22 e-ISSN: 2320-0812 p-ISSN: 2347-2340 RRJPA | Volume 5 | Issue 2 | July - September, 2016 A Review on High Performance Liquid Chromatography (HPLC) Mukthi Thammana* Department of Pharmacy, Vignan Institute of Pharmaceutical Technology, Duvvada, Visakhapatanam, Andhra Pradesh, India
Research and development (R&D) productivity has long been a topic of concern amongst industry analysts (Drug Inf. J. 34, 1169-1194; 2000). In general, R&D productivity can be thought of as ...
Current Pharmaceutical Analysis. Limited Time Complementary OA Offer Publish Research Articles With No Charges. Impact Factor: 0.6. Indexed in: Scopus, SCI Expanded, JCR... View all. Volume 20 , Issues 9, 2024. Back Journal Home. Call For Paper.
The Journal of Pharmaceutical Analysis (JPA) is a broad-based journal founded on two key tenets: To publish the most exciting researches in the field of Pharmaceutical analysis to it : Second to provide a rapid turn-around time possible for reviewing and publishing of articles freely for research, teaching and reference purposes. It is ...
Find breaking science news and analysis from the world's leading research journal. Latest science news and analysis from the world's leading research journal. ... Andrew Robinson reviews five of ...
Read the latest articles of Journal of Pharmaceutical Analysis at ScienceDirect.com, Elsevier's leading platform of peer-reviewed scholarly literature ... Review paper; Original articles; Short communications; Receive an update when the latest issues in this journal are published. ... Research article Open access
Heavy metal soil pollution severely threatens human health and food safety. This study used PRISMA to systematically review heavy metal-contaminated soil remediation patents in the Derwent Patent Database from 2003 to 2023. A total of 1744 patents were selected. The results of the analysis show that related patent applications are growing around the world. Among them, China has the most ...
Background: This paper explores the widely discussed relationship between electronic media use and sleep quality, indicating negative effects due to various factors. However, existing meta-analyses on the topic have some limitations. Objective: The study aims to analyze and compare the impacts of different digital media types, such as smartphones, online games, and social media, on sleep quality.
Furthermore, a descriptive analysis of 74 postinfection and 153 postvaccination HZ studies found no significant differences on gender or age (<50 and ≥50 years) following COVID-19 infection. Notably, 44.0% of the HZ cases postinfection appeared within the first week, with 69.5% resolving within 10 days, predominantly presenting as skin lesions.
Research article Open access Protective effects of dioscin against Parkinson's disease via regulating bile acid metabolism through remodeling gut microbiome/GLP-1 signaling Zhang Mao, Haochen Hui, Xuerong Zhao, Lina Xu, ...
Background: Falls and their consequences are a serious public health problem worldwide. Each year, 37.3 million falls requiring medical attention occur. Therefore, the analysis of fall risk is of great importance for prevention. Artificial intelligence (AI) represents an innovative tool for creating predictive statistical models of fall risk through data analysis.
Conceivable Mechanisms of Clozapine Propagated Dyslipidemia- A Short Review. Pearl Pinto, Sivaprakasam Chinnarasu, Mohanraj Sadasivam, Louis Cojandaraj*. Abstract. Full-text. PDF. Mobile Full-text. Research & Reviews is a scientific organization that drives the progress of research through open access journals Volume 11, Issue 5 | 2022.
Read the latest articles of Journal of Pharmaceutical Analysis at ScienceDirect.com, Elsevier's leading platform of peer-reviewed scholarly literature ... Research article Open access ... Review article Open access Multidisciplinary strategies to enhance therapeutic effects of flavonoids from Epimedii Folium: Integration of herbal medicine ...
Background: The COVID-19 pandemic has significantly reduced physical activity (PA) levels and increased sedentary behavior (SB), which can lead to worsening physical fitness (PF). Children and adolescents may benefit from mobile health (mHealth) apps to increase PA and improve PF. However, the effectiveness of mHealth app-based interventions and potential moderators in this population are ...