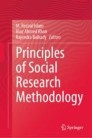
Principles of Social Research Methodology pp 415–426 Cite as
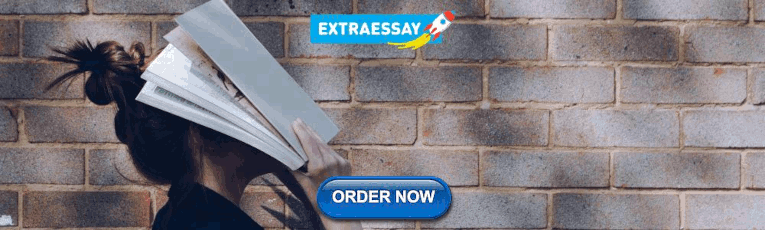
Sampling Techniques for Qualitative Research
- Heather Douglas 4
- First Online: 27 October 2022
2337 Accesses
2 Citations
This chapter explains how to design suitable sampling strategies for qualitative research. The focus of this chapter is purposive (or theoretical) sampling to produce credible and trustworthy explanations of a phenomenon (a specific aspect of society). A specific research question (RQ) guides the methodology (the study design or approach ). It defines the participants, location, and actions to be used to answer the question. Qualitative studies use specific tools and techniques ( methods ) to sample people, organizations, or whatever is to be examined. The methodology guides the selection of tools and techniques for sampling, data analysis, quality assurance, etc. These all vary according to the purpose and design of the study and the RQ. In this chapter, a fake example is used to demonstrate how to apply your sampling strategy in a developing country.
- Phenomenon. Methodology. Research Question. Methods. Tools and Techniques. Purposive Sampling. Sampling Frame. Trustworthiness
This is a preview of subscription content, log in via an institution .
Buying options
- Available as PDF
- Read on any device
- Instant download
- Own it forever
- Available as EPUB and PDF
- Compact, lightweight edition
- Dispatched in 3 to 5 business days
- Free shipping worldwide - see info
- Durable hardcover edition
Tax calculation will be finalised at checkout
Purchases are for personal use only
Douglas, H. (2010). Divergent orientations in social entrepreneurship organisations. In K. Hockerts, J. Robinson, & J. Mair (Eds.), Values and opportunities in social entrepreneurship (pp. 71–95). Palgrave Macmillan.
Chapter Google Scholar
Douglas, H., Eti-Tofinga, B., & Singh, G. (2018a). Contextualising social enterprise in Fiji. Social Enterprise Journal, 14 (2), 208–224. https://doi.org/10.1108/SEJ-05-2017-0032
Article Google Scholar
Douglas, H., Eti-Tofinga, B., & Singh, G. (2018b). Hybrid organisations contributing to wellbeing in small Pacific island countries. Sustainability Accounting, Management and Policy Journal, 9 (4), 490–514. https://doi.org/10.1108/SAMPJ-08-2017-0081
Douglas, H., & Borbasi, S. (2009). Parental perspectives on disability: The story of Sam, Anna, and Marcus. Disabilities: Insights from across fields and around the world, 2 , 201–217.
Google Scholar
Douglas, H. (1999). Community transport in rural Queensland: Using community resources effectively in small communities. Paper presented at the 5th National Rural Health Conference, Adelaide, South Australia, pp. 14–17th March.
Douglas, H. (2006). Action, blastoff, chaos: ABC of successful youth participation. Child, Youth and Environments, 16 (1). Retrieved from http://www.colorado.edu/journals/cye
Douglas, H. (2007). Methodological sampling issues for researching new nonprofit organisations. Paper presented at the 52nd International Council for Small Business (ICSB) 13–15 June, Turku, Finland.
Draper, H., Wilson, S., Flanagan, S., & Ives, J. (2009). Offering payments, reimbursement and incentives to patients and family doctors to encourage participation in research. Family Practice, 26 (3), 231–238. https://doi.org/10.1093/fampra/cmp011
Puamua, P. Q. (1999). Understanding Fijian under-achievement: An integrated perspective. Directions, 21 (2), 100–112.
Download references
Author information
Authors and affiliations.
The University of Queensland, The Royal Society of Queensland, Activation Australia, Brisbane, Australia
Heather Douglas
You can also search for this author in PubMed Google Scholar
Corresponding author
Correspondence to Heather Douglas .
Editor information
Editors and affiliations.
Centre for Family and Child Studies, Research Institute of Humanities and Social Sciences, University of Sharjah, Sharjah, United Arab Emirates
M. Rezaul Islam
Department of Development Studies, University of Dhaka, Dhaka, Bangladesh
Niaz Ahmed Khan
Department of Social Work, School of Humanities, University of Johannesburg, Johannesburg, South Africa
Rajendra Baikady
Rights and permissions
Reprints and permissions
Copyright information
© 2022 The Author(s), under exclusive license to Springer Nature Singapore Pte Ltd.
About this chapter
Cite this chapter.
Douglas, H. (2022). Sampling Techniques for Qualitative Research. In: Islam, M.R., Khan, N.A., Baikady, R. (eds) Principles of Social Research Methodology. Springer, Singapore. https://doi.org/10.1007/978-981-19-5441-2_29
Download citation
DOI : https://doi.org/10.1007/978-981-19-5441-2_29
Published : 27 October 2022
Publisher Name : Springer, Singapore
Print ISBN : 978-981-19-5219-7
Online ISBN : 978-981-19-5441-2
eBook Packages : Social Sciences
Share this chapter
Anyone you share the following link with will be able to read this content:
Sorry, a shareable link is not currently available for this article.
Provided by the Springer Nature SharedIt content-sharing initiative
- Publish with us
Policies and ethics
- Find a journal
- Track your research
Have a language expert improve your writing
Run a free plagiarism check in 10 minutes, generate accurate citations for free.
- Knowledge Base
Methodology
- Sampling Methods | Types, Techniques & Examples
Sampling Methods | Types, Techniques & Examples
Published on September 19, 2019 by Shona McCombes . Revised on June 22, 2023.
When you conduct research about a group of people, it’s rarely possible to collect data from every person in that group. Instead, you select a sample . The sample is the group of individuals who will actually participate in the research.
To draw valid conclusions from your results, you have to carefully decide how you will select a sample that is representative of the group as a whole. This is called a sampling method . There are two primary types of sampling methods that you can use in your research:
- Probability sampling involves random selection, allowing you to make strong statistical inferences about the whole group.
- Non-probability sampling involves non-random selection based on convenience or other criteria, allowing you to easily collect data.
You should clearly explain how you selected your sample in the methodology section of your paper or thesis, as well as how you approached minimizing research bias in your work.
Table of contents
Population vs. sample, probability sampling methods, non-probability sampling methods, other interesting articles, frequently asked questions about sampling.
First, you need to understand the difference between a population and a sample , and identify the target population of your research.
- The population is the entire group that you want to draw conclusions about.
- The sample is the specific group of individuals that you will collect data from.
The population can be defined in terms of geographical location, age, income, or many other characteristics.
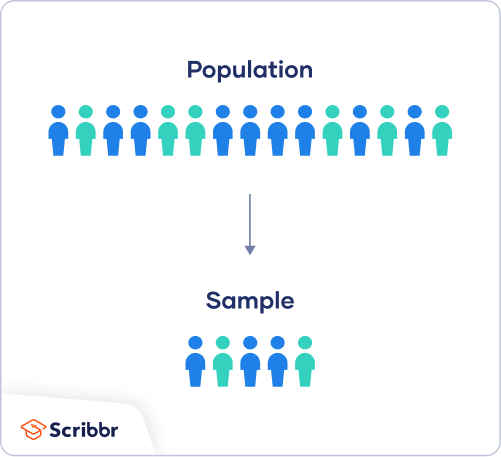
It is important to carefully define your target population according to the purpose and practicalities of your project.
If the population is very large, demographically mixed, and geographically dispersed, it might be difficult to gain access to a representative sample. A lack of a representative sample affects the validity of your results, and can lead to several research biases , particularly sampling bias .
Sampling frame
The sampling frame is the actual list of individuals that the sample will be drawn from. Ideally, it should include the entire target population (and nobody who is not part of that population).
Sample size
The number of individuals you should include in your sample depends on various factors, including the size and variability of the population and your research design. There are different sample size calculators and formulas depending on what you want to achieve with statistical analysis .
Receive feedback on language, structure, and formatting
Professional editors proofread and edit your paper by focusing on:
- Academic style
- Vague sentences
- Style consistency
See an example
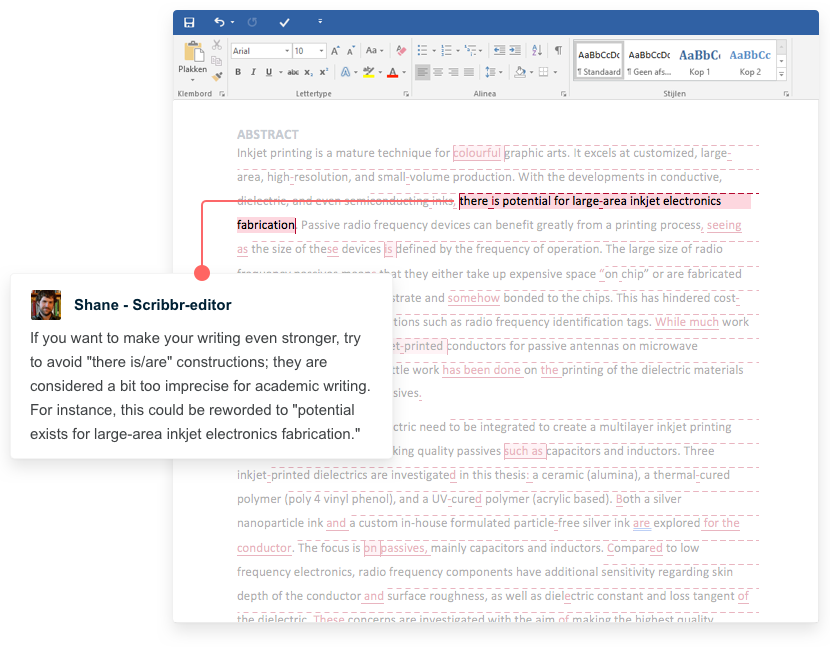
Probability sampling means that every member of the population has a chance of being selected. It is mainly used in quantitative research . If you want to produce results that are representative of the whole population, probability sampling techniques are the most valid choice.
There are four main types of probability sample.
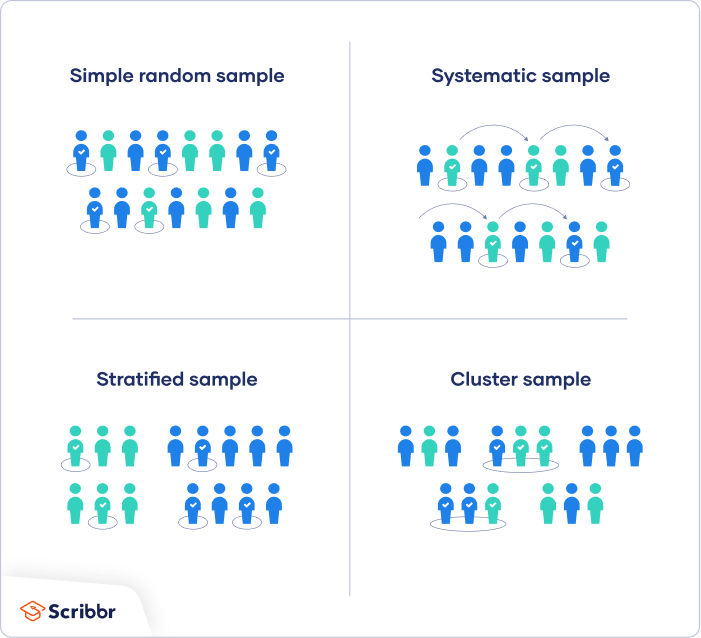
1. Simple random sampling
In a simple random sample, every member of the population has an equal chance of being selected. Your sampling frame should include the whole population.
To conduct this type of sampling, you can use tools like random number generators or other techniques that are based entirely on chance.
2. Systematic sampling
Systematic sampling is similar to simple random sampling, but it is usually slightly easier to conduct. Every member of the population is listed with a number, but instead of randomly generating numbers, individuals are chosen at regular intervals.
If you use this technique, it is important to make sure that there is no hidden pattern in the list that might skew the sample. For example, if the HR database groups employees by team, and team members are listed in order of seniority, there is a risk that your interval might skip over people in junior roles, resulting in a sample that is skewed towards senior employees.
3. Stratified sampling
Stratified sampling involves dividing the population into subpopulations that may differ in important ways. It allows you draw more precise conclusions by ensuring that every subgroup is properly represented in the sample.
To use this sampling method, you divide the population into subgroups (called strata) based on the relevant characteristic (e.g., gender identity, age range, income bracket, job role).
Based on the overall proportions of the population, you calculate how many people should be sampled from each subgroup. Then you use random or systematic sampling to select a sample from each subgroup.
4. Cluster sampling
Cluster sampling also involves dividing the population into subgroups, but each subgroup should have similar characteristics to the whole sample. Instead of sampling individuals from each subgroup, you randomly select entire subgroups.
If it is practically possible, you might include every individual from each sampled cluster. If the clusters themselves are large, you can also sample individuals from within each cluster using one of the techniques above. This is called multistage sampling .
This method is good for dealing with large and dispersed populations, but there is more risk of error in the sample, as there could be substantial differences between clusters. It’s difficult to guarantee that the sampled clusters are really representative of the whole population.
In a non-probability sample, individuals are selected based on non-random criteria, and not every individual has a chance of being included.
This type of sample is easier and cheaper to access, but it has a higher risk of sampling bias . That means the inferences you can make about the population are weaker than with probability samples, and your conclusions may be more limited. If you use a non-probability sample, you should still aim to make it as representative of the population as possible.
Non-probability sampling techniques are often used in exploratory and qualitative research . In these types of research, the aim is not to test a hypothesis about a broad population, but to develop an initial understanding of a small or under-researched population.
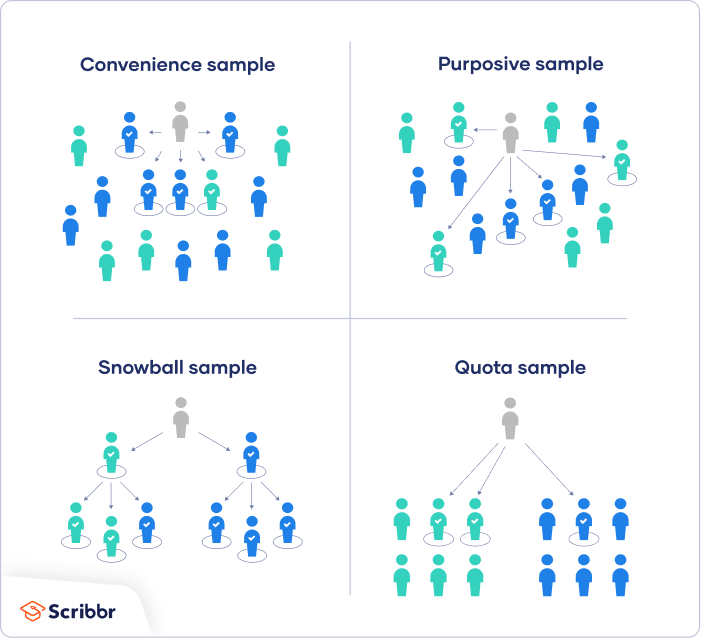
1. Convenience sampling
A convenience sample simply includes the individuals who happen to be most accessible to the researcher.
This is an easy and inexpensive way to gather initial data, but there is no way to tell if the sample is representative of the population, so it can’t produce generalizable results. Convenience samples are at risk for both sampling bias and selection bias .
2. Voluntary response sampling
Similar to a convenience sample, a voluntary response sample is mainly based on ease of access. Instead of the researcher choosing participants and directly contacting them, people volunteer themselves (e.g. by responding to a public online survey).
Voluntary response samples are always at least somewhat biased , as some people will inherently be more likely to volunteer than others, leading to self-selection bias .
3. Purposive sampling
This type of sampling, also known as judgement sampling, involves the researcher using their expertise to select a sample that is most useful to the purposes of the research.
It is often used in qualitative research , where the researcher wants to gain detailed knowledge about a specific phenomenon rather than make statistical inferences, or where the population is very small and specific. An effective purposive sample must have clear criteria and rationale for inclusion. Always make sure to describe your inclusion and exclusion criteria and beware of observer bias affecting your arguments.
4. Snowball sampling
If the population is hard to access, snowball sampling can be used to recruit participants via other participants. The number of people you have access to “snowballs” as you get in contact with more people. The downside here is also representativeness, as you have no way of knowing how representative your sample is due to the reliance on participants recruiting others. This can lead to sampling bias .
5. Quota sampling
Quota sampling relies on the non-random selection of a predetermined number or proportion of units. This is called a quota.
You first divide the population into mutually exclusive subgroups (called strata) and then recruit sample units until you reach your quota. These units share specific characteristics, determined by you prior to forming your strata. The aim of quota sampling is to control what or who makes up your sample.
If you want to know more about statistics , methodology , or research bias , make sure to check out some of our other articles with explanations and examples.
- Student’s t -distribution
- Normal distribution
- Null and Alternative Hypotheses
- Chi square tests
- Confidence interval
- Quartiles & Quantiles
- Cluster sampling
- Stratified sampling
- Data cleansing
- Reproducibility vs Replicability
- Peer review
- Prospective cohort study
Research bias
- Implicit bias
- Cognitive bias
- Placebo effect
- Hawthorne effect
- Hindsight bias
- Affect heuristic
- Social desirability bias
A sample is a subset of individuals from a larger population . Sampling means selecting the group that you will actually collect data from in your research. For example, if you are researching the opinions of students in your university, you could survey a sample of 100 students.
In statistics, sampling allows you to test a hypothesis about the characteristics of a population.
Samples are used to make inferences about populations . Samples are easier to collect data from because they are practical, cost-effective, convenient, and manageable.
Probability sampling means that every member of the target population has a known chance of being included in the sample.
Probability sampling methods include simple random sampling , systematic sampling , stratified sampling , and cluster sampling .
In non-probability sampling , the sample is selected based on non-random criteria, and not every member of the population has a chance of being included.
Common non-probability sampling methods include convenience sampling , voluntary response sampling, purposive sampling , snowball sampling, and quota sampling .
In multistage sampling , or multistage cluster sampling, you draw a sample from a population using smaller and smaller groups at each stage.
This method is often used to collect data from a large, geographically spread group of people in national surveys, for example. You take advantage of hierarchical groupings (e.g., from state to city to neighborhood) to create a sample that’s less expensive and time-consuming to collect data from.
Sampling bias occurs when some members of a population are systematically more likely to be selected in a sample than others.
Cite this Scribbr article
If you want to cite this source, you can copy and paste the citation or click the “Cite this Scribbr article” button to automatically add the citation to our free Citation Generator.
McCombes, S. (2023, June 22). Sampling Methods | Types, Techniques & Examples. Scribbr. Retrieved March 25, 2024, from https://www.scribbr.com/methodology/sampling-methods/
Is this article helpful?
Shona McCombes
Other students also liked, population vs. sample | definitions, differences & examples, simple random sampling | definition, steps & examples, sampling bias and how to avoid it | types & examples, unlimited academic ai-proofreading.
✔ Document error-free in 5minutes ✔ Unlimited document corrections ✔ Specialized in correcting academic texts
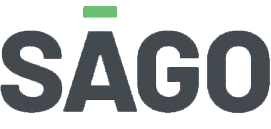
What We Offer
With a comprehensive suite of qualitative and quantitative capabilities and 55 years of experience in the industry, Sago powers insights through adaptive solutions.
- Recruitment
- Communities
- Methodify® Automated research
- QualBoard® Digital Discussions
- QualMeeting® Digital Interviews
- Global Qualitative
- Global Quantitative
- In-Person Facilities
- Research Consulting
- Europe Solutions
- Clinical Research
- Human Factors
- Neuromarketing Tools
- Trial & Jury Consulting
Who We Serve
Form deeper customer connections and make the process of answering your business questions easier. Sago delivers unparalleled access to the audiences you need through adaptive solutions and a consultative approach.
- Consumer Packaged Goods
- Financial Services
- Media Technology
- Marketing Research
With a 55-year legacy of impact, Sago has proven we have what it takes to be a long-standing industry leader and partner. We continually advance our range of expertise to provide our clients with the highest level of confidence.
- Global Offices
- Partnerships & Certifications
- News & Media
- Researcher Events
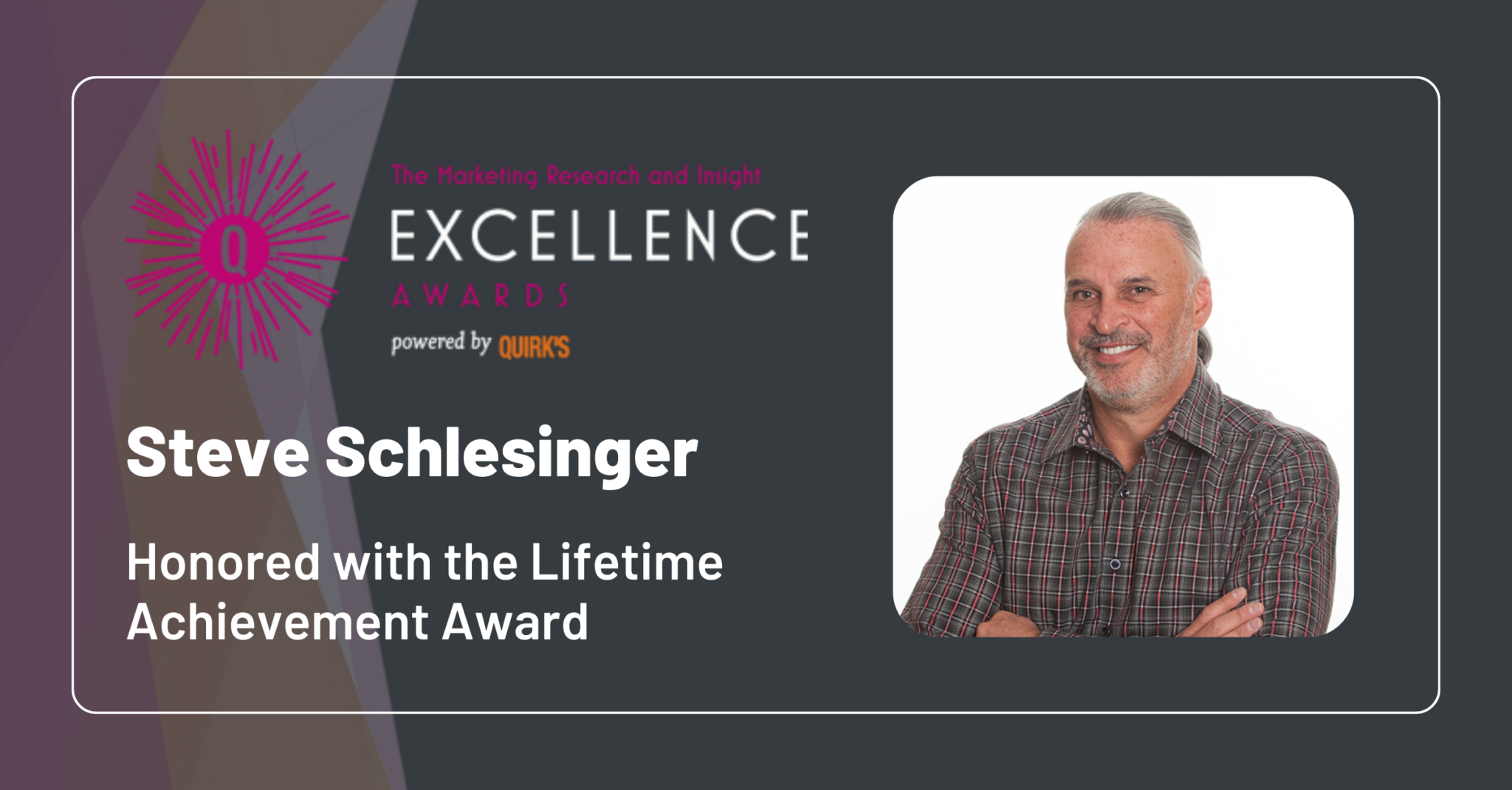
Sago Executive Chairman Steve Schlesinger to Receive Quirk’s Lifetime Achievement Award
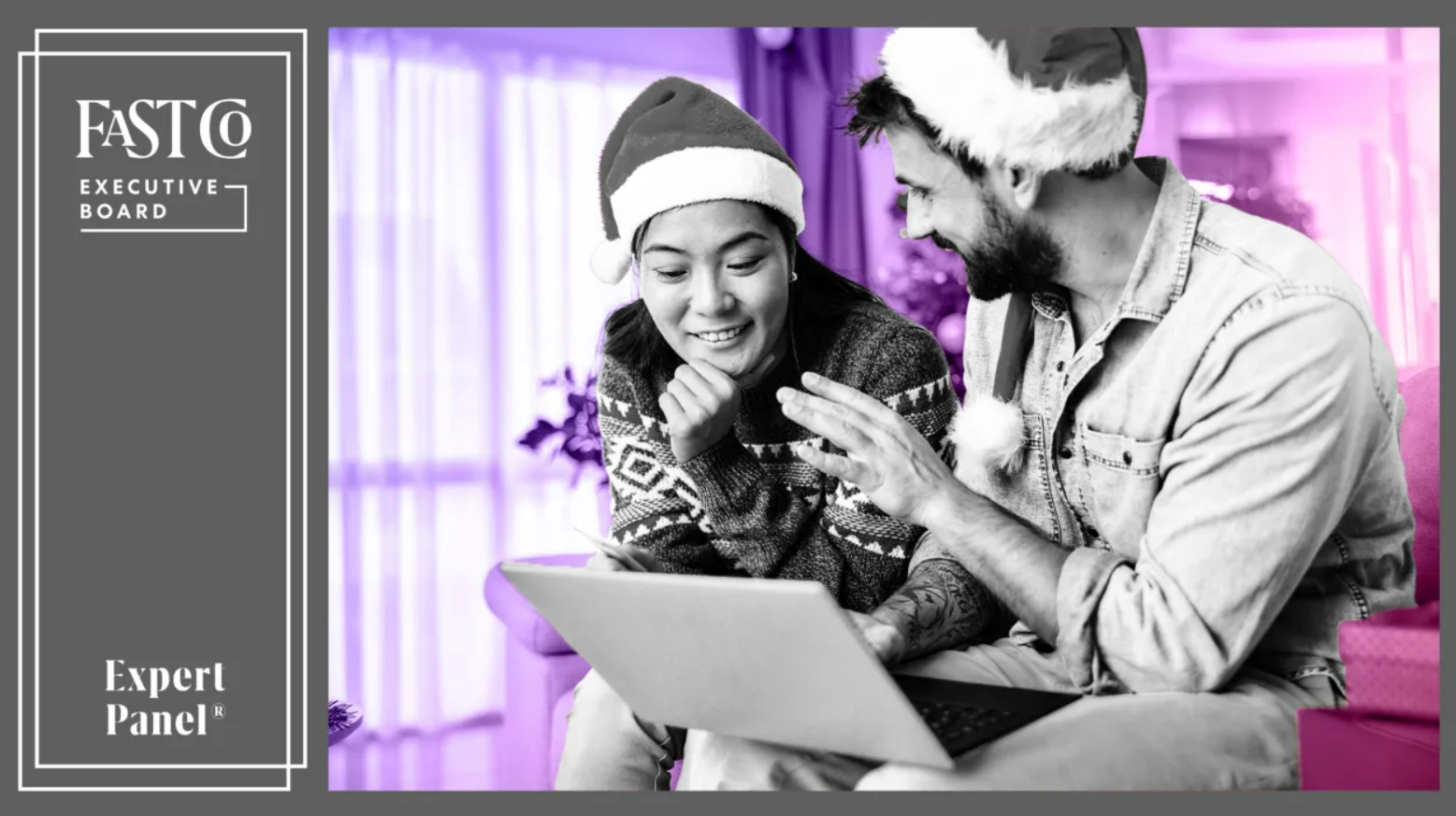
16 Ways to Prepare for the Next Holiday Campaign
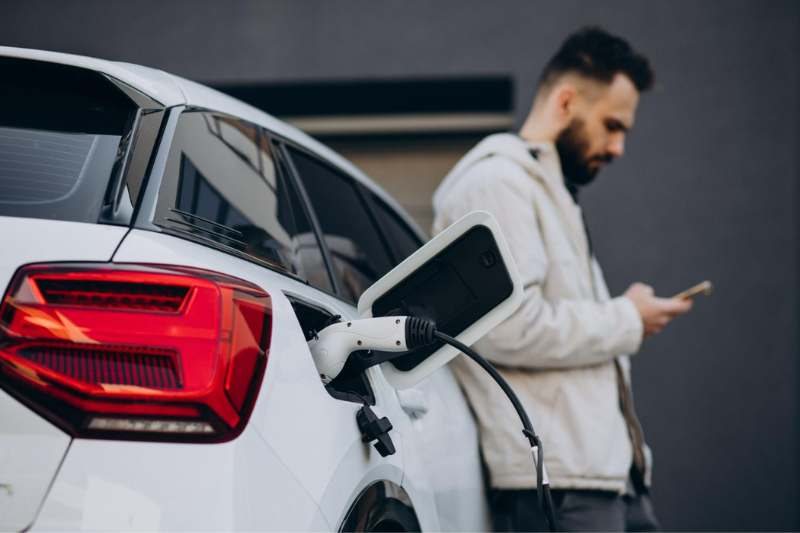
Sago Unveils Electric Vehicle Panel to Drive Industry Success
Drop into your new favorite insights rabbit hole and explore content created by the leading minds in market research.
- Case Studies
- Knowledge Kit
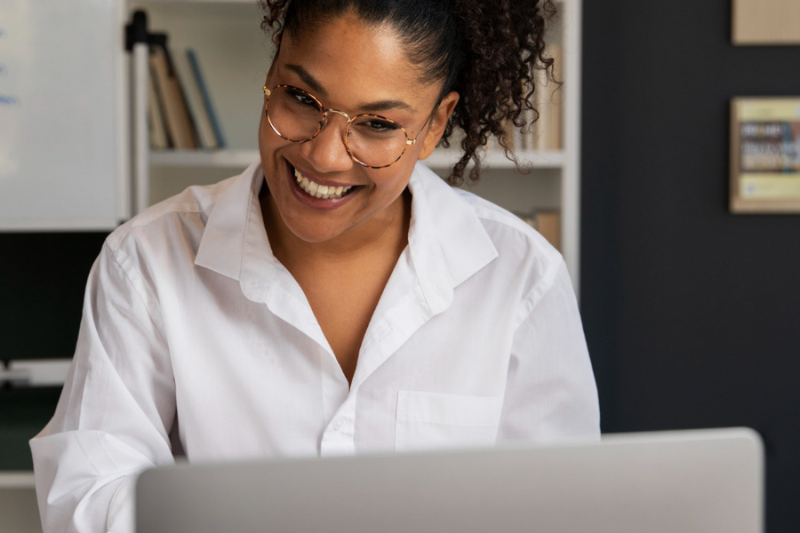
Moderator Minutes: Maximizing Efficient with QualBoard – Streamline Your Projects with Support & AI
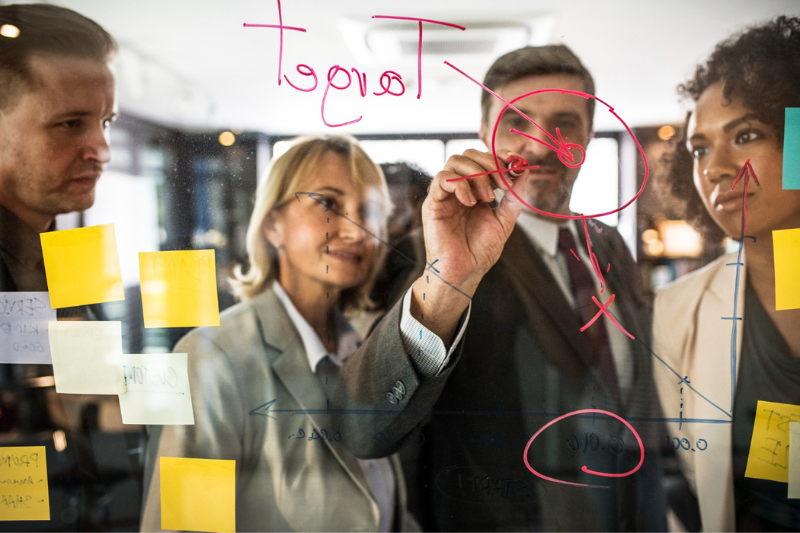
2024 Trends in Research: Fact or Fiction?
Get in touch

- Account Logins

Different Types of Sampling Techniques in Qualitative Research
- Resources , Blog

Key Takeaways:
- Sampling techniques in qualitative research include purposive, convenience, snowball, and theoretical sampling.
- Choosing the right sampling technique significantly impacts the accuracy and reliability of the research results.
- It’s crucial to consider the potential impact on the bias, sample diversity, and generalizability when choosing a sampling technique for your qualitative research.
Qualitative research seeks to understand social phenomena from the perspective of those experiencing them. It involves collecting non-numerical data such as interviews, observations, and written documents to gain insights into human experiences, attitudes, and behaviors. While qualitative research can provide rich and nuanced insights, the accuracy and generalizability of findings depend on the quality of the sampling process. Sampling is a critical component of qualitative research as it involves selecting a group of participants who can provide valuable insights into the research questions.
This article explores different types of sampling techniques used in qualitative research. First, we’ll provide a comprehensive overview of four standard sampling techniques used in qualitative research. and then compare and contrast these techniques to provide guidance on choosing the most appropriate method for a particular study. Additionally, you’ll find best practices for sampling and learn about ethical considerations researchers need to consider in selecting a sample. Overall, this article aims to help researchers conduct effective and high-quality sampling in qualitative research.
In this Article:
- Purposive Sampling
- Convenience Sampling
- Snowball Sampling
- Theoretical Sampling
Factors to Consider When Choosing a Sampling Technique
Practical approaches to sampling: recommended practices, final thoughts, get expert guidance on your sample needs.
Want expert input on the best sampling technique for your qualitative research project? Book a consultation for trusted advice.
Request a consultation
4 Types of Sampling Techniques and Their Applications
Sampling is a crucial aspect of qualitative research as it determines the representativeness and credibility of the data collected. Several sampling techniques are used in qualitative research, each with strengths and weaknesses. In this section, let’s explore four standard sampling techniques used in qualitative research: purposive sampling, convenience sampling, snowball sampling, and theoretical sampling. We’ll break down the definition of each technique, when to use it, and its advantages and disadvantages.
1. Purposive Sampling
Purposive sampling, or judgmental sampling, is a non-probability sampling technique commonly used in qualitative research. In purposive sampling, researchers intentionally select participants with specific characteristics or unique experiences related to the research question. The goal is to identify and recruit participants who can provide rich and diverse data to enhance the research findings.
Purposive sampling is used when researchers seek to identify individuals or groups with particular knowledge, skills, or experiences relevant to the research question. For instance, in a study examining the experiences of cancer patients undergoing chemotherapy, purposive sampling may be used to recruit participants who have undergone chemotherapy in the past year. Researchers can better understand the phenomenon under investigation by selecting individuals with relevant backgrounds.
Purposive Sampling: Strengths and Weaknesses
Purposive sampling is a powerful tool for researchers seeking to select participants who can provide valuable insight into their research question. This method is advantageous when studying groups with technical characteristics or experiences where a random selection of participants may yield different results.
One of the main advantages of purposive sampling is the ability to improve the quality and accuracy of data collected by selecting participants most relevant to the research question. This approach also enables researchers to collect data from diverse participants with unique perspectives and experiences related to the research question.
However, researchers should also be aware of potential bias when using purposive sampling. The researcher’s judgment may influence the selection of participants, resulting in a biased sample that does not accurately represent the broader population. Another disadvantage is that purposive sampling may not be representative of the more general population, which limits the generalizability of the findings. To guarantee the accuracy and dependability of data obtained through purposive sampling, researchers must provide a clear and transparent justification of their selection criteria and sampling approach. This entails outlining the specific characteristics or experiences required for participants to be included in the study and explaining the rationale behind these criteria. This level of transparency not only helps readers to evaluate the validity of the findings, but also enhances the replicability of the research.
2. Convenience Sampling
When time and resources are limited, researchers may opt for convenience sampling as a quick and cost-effective way to recruit participants. In this non-probability sampling technique, participants are selected based on their accessibility and willingness to participate rather than their suitability for the research question. Qualitative research often uses this approach to generate various perspectives and experiences.
During the COVID-19 pandemic, convenience sampling was a valuable method for researchers to collect data quickly and efficiently from participants who were easily accessible and willing to participate. For example, in a study examining the experiences of university students during the pandemic, convenience sampling allowed researchers to recruit students who were available and willing to share their experiences quickly. While the pandemic may be over, convenience sampling during this time highlights its value in urgent situations where time and resources are limited.
Convenience Sampling: Strengths and Weaknesses
Convenience sampling offers several advantages to researchers, including its ease of implementation and cost-effectiveness. This technique allows researchers to quickly and efficiently recruit participants without spending time and resources identifying and contacting potential participants. Furthermore, convenience sampling can result in a diverse pool of participants, as individuals from various backgrounds and experiences may be more likely to participate.
While convenience sampling has the advantage of being efficient, researchers need to acknowledge its limitations. One of the primary drawbacks of convenience sampling is that it is susceptible to selection bias. Participants who are more easily accessible may not be representative of the broader population, which can limit the generalizability of the findings. Furthermore, convenience sampling may lead to issues with the reliability of the results, as it may not be possible to replicate the study using the same sample or a similar one.
To mitigate these limitations, researchers should carefully define the population of interest and ensure the sample is drawn from that population. For instance, if a study is investigating the experiences of individuals with a particular medical condition, researchers can recruit participants from specialized clinics or support groups for that condition. Researchers can also use statistical techniques such as stratified sampling or weighting to adjust for potential biases in the sample.
3. Snowball Sampling
Snowball sampling, also called referral sampling, is a unique approach researchers use to recruit participants in qualitative research. The technique involves identifying a few initial participants who meet the eligibility criteria and asking them to refer others they know who also fit the requirements. The sample size grows as referrals are added, creating a chain-like structure.
Snowball sampling enables researchers to reach out to individuals who may be hard to locate through traditional sampling methods, such as members of marginalized or hidden communities. For instance, in a study examining the experiences of undocumented immigrants, snowball sampling may be used to identify and recruit participants through referrals from other undocumented immigrants.
Snowball Sampling: Strengths and Weaknesses
Snowball sampling can produce in-depth and detailed data from participants with common characteristics or experiences. Since referrals are made within a network of individuals who share similarities, researchers can gain deep insights into a specific group’s attitudes, behaviors, and perspectives.
4. Theoretical Sampling
Theoretical sampling is a sophisticated and strategic technique that can help researchers develop more in-depth and nuanced theories from their data. Instead of selecting participants based on convenience or accessibility, researchers using theoretical sampling choose participants based on their potential to contribute to the emerging themes and concepts in the data. This approach allows researchers to refine their research question and theory based on the data they collect rather than forcing their data to fit a preconceived idea.
Theoretical sampling is used when researchers conduct grounded theory research and have developed an initial theory or conceptual framework. In a study examining cancer survivors’ experiences, for example, theoretical sampling may be used to identify and recruit participants who can provide new insights into the coping strategies of survivors.
Theoretical Sampling: Strengths and Weaknesses
One of the significant advantages of theoretical sampling is that it allows researchers to refine their research question and theory based on emerging data. This means the research can be highly targeted and focused, leading to a deeper understanding of the phenomenon being studied. Additionally, theoretical sampling can generate rich and in-depth data, as participants are selected based on their potential to provide new insights into the research question.
Participants are selected based on their perceived ability to offer new perspectives on the research question. This means specific perspectives or experiences may be overrepresented in the sample, leading to an incomplete understanding of the phenomenon being studied. Additionally, theoretical sampling can be time-consuming and resource-intensive, as researchers must continuously analyze the data and recruit new participants.
To mitigate the potential for bias, researchers can take several steps. One way to reduce bias is to use a diverse team of researchers to analyze the data and make participant selection decisions. Having multiple perspectives and backgrounds can help prevent researchers from unconsciously selecting participants who fit their preconceived notions or biases.
Another solution would be to use reflexive sampling. Reflexive sampling involves selecting participants aware of the research process and provides insights into how their biases and experiences may influence their perspectives. By including participants who are reflexive about their subjectivity, researchers can generate more nuanced and self-aware findings.
Choosing the proper sampling technique is one of the most critical decisions a researcher makes when conducting a study. The preferred method can significantly impact the accuracy and reliability of the research results.
For instance, purposive sampling provides a more targeted and specific sample, which helps to answer research questions related to that particular population or phenomenon. However, this approach may also introduce bias by limiting the diversity of the sample.
Conversely, convenience sampling may offer a more diverse sample regarding demographics and backgrounds but may also introduce bias by selecting more willing or available participants.
Snowball sampling may help study hard-to-reach populations, but it can also limit the sample’s diversity as participants are selected based on their connections to existing participants.
Theoretical sampling may offer an opportunity to refine the research question and theory based on emerging data, but it can also be time-consuming and resource-intensive.
Additionally, the choice of sampling technique can impact the generalizability of the research findings. Therefore, it’s crucial to consider the potential impact on the bias, sample diversity, and generalizability when choosing a sampling technique. By doing so, researchers can select the most appropriate method for their research question and ensure the validity and reliability of their findings.
Tips for Selecting Participants
When selecting participants for a qualitative research study, it is crucial to consider the research question and the purpose of the study. In addition, researchers should identify the specific characteristics or criteria they seek in their sample and select participants accordingly.
One helpful tip for selecting participants is to use a pre-screening process to ensure potential participants meet the criteria for inclusion in the study. Another technique is using multiple recruitment methods to ensure the sample is diverse and representative of the studied population.
Ensuring Diversity in Samples
Diversity in the sample is important to ensure the study’s findings apply to a wide range of individuals and situations. One way to ensure diversity is to use stratified sampling, which involves dividing the population into subgroups and selecting participants from each subset. This helps establish that the sample is representative of the larger population.
Maintaining Ethical Considerations
When selecting participants for a qualitative research study, it is essential to ensure ethical considerations are taken into account. Researchers must ensure participants are fully informed about the study and provide their voluntary consent to participate. They must also ensure participants understand their rights and that their confidentiality and privacy will be protected.
A qualitative research study’s success hinges on its sampling technique’s effectiveness. The choice of sampling technique must be guided by the research question, the population being studied, and the purpose of the study. Whether purposive, convenience, snowball, or theoretical sampling, the primary goal is to ensure the validity and reliability of the study’s findings.
By thoughtfully weighing the pros and cons of each sampling technique, researchers can make informed decisions that lead to more reliable and accurate results. In conclusion, carefully selecting a sampling technique is integral to the success of a qualitative research study, and a thorough understanding of the available options can make all the difference in achieving high-quality research outcomes.
If you’re interested in improving your research and sampling methods, Sago offers a variety of solutions. Our qualitative research platforms, such as QualBoard and QualMeeting, can assist you in conducting research studies with precision and efficiency. Our robust global panel and recruitment options help you reach the right people. We also offer qualitative and quantitative research services to meet your research needs. Contact us today to learn more about how we can help improve your research outcomes.
Find the Right Sample for Your Qualitative Research
Trust our team to recruit the participants you need using the appropriate techniques. Book a consultation with our team to get started .
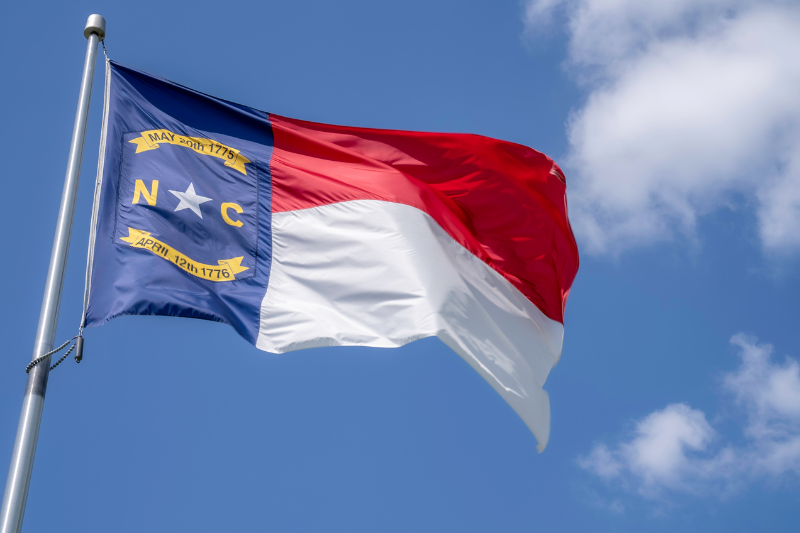
The Deciders February 2024: African American voters in North Carolina
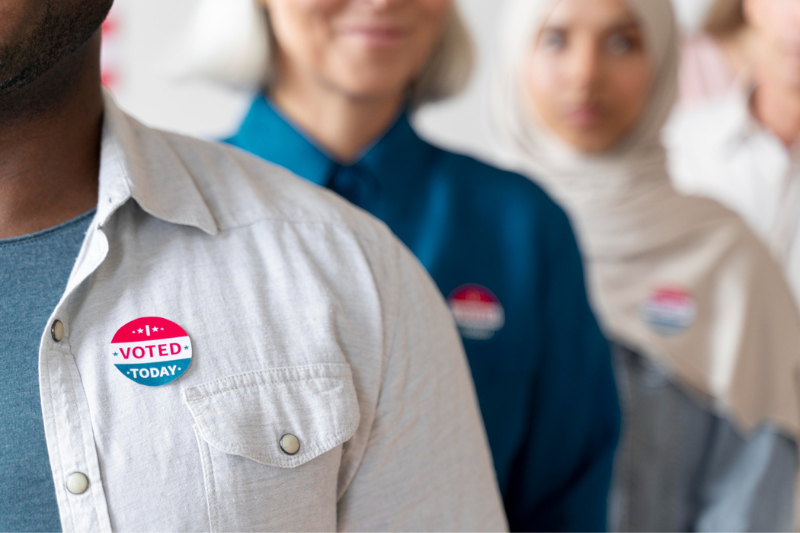
The Swing Voter Project in Michigan – February 2024
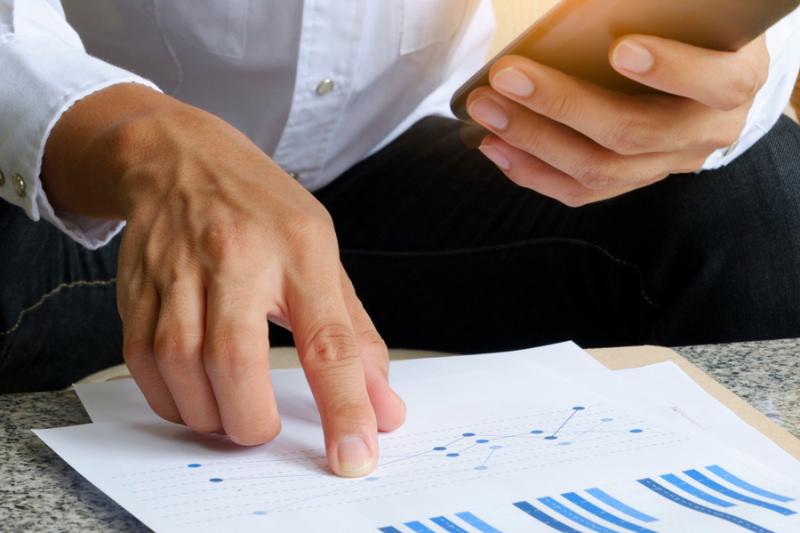
OnDemand: 5 Steps to High-Quality Data: Mitigating the Challenges of Data Quality in Quantitative Research
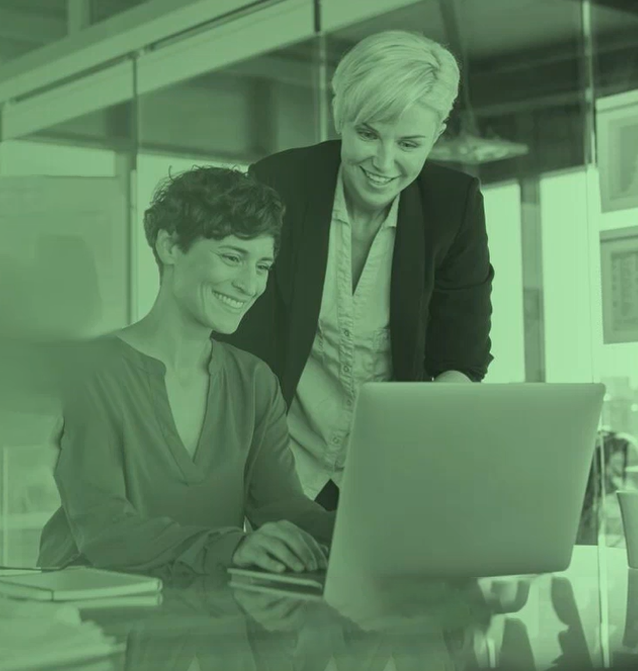
How Leading Brands Use Insights to Win Market Share
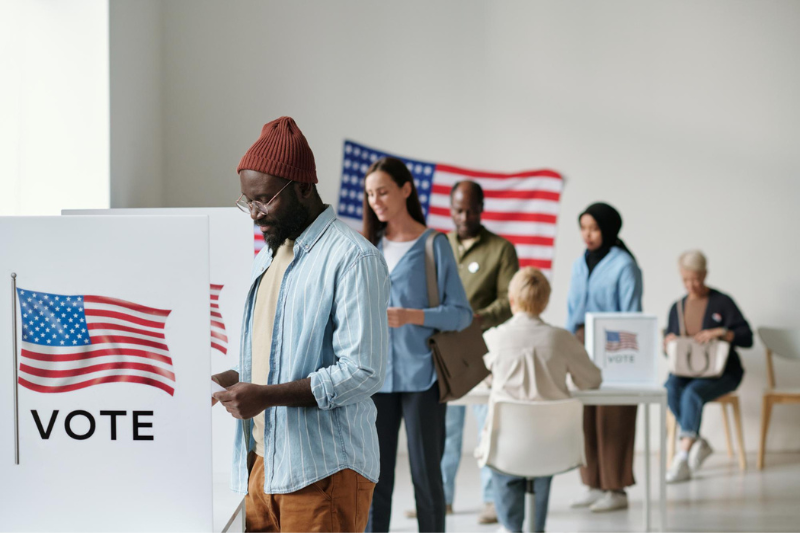
The Deciders January 2024: Trump-Voting Women Who Oppose Dobbs
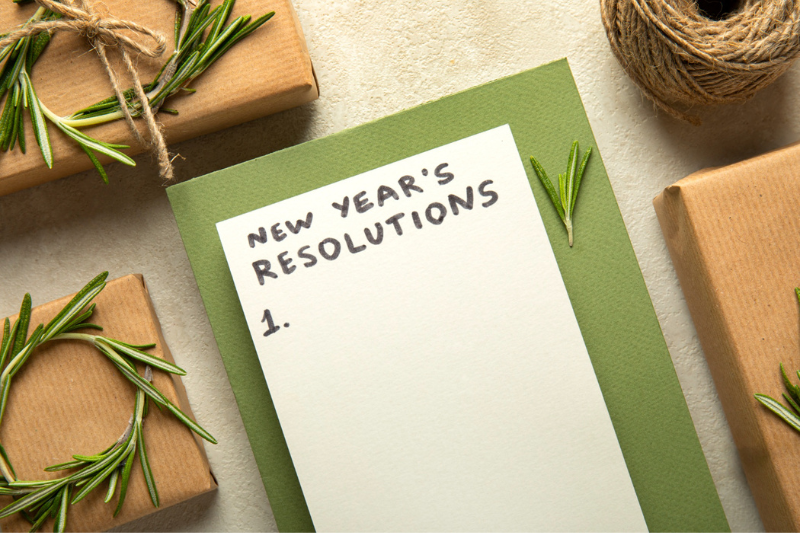
The State of New Year’s Resolutions: A Closer Look at Mindsets and Habits
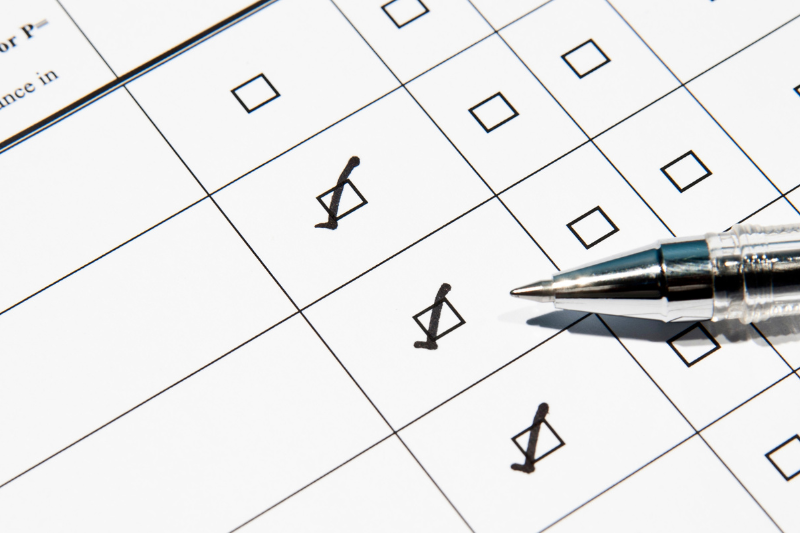
It’s Time to Change the Way We Write Screeners
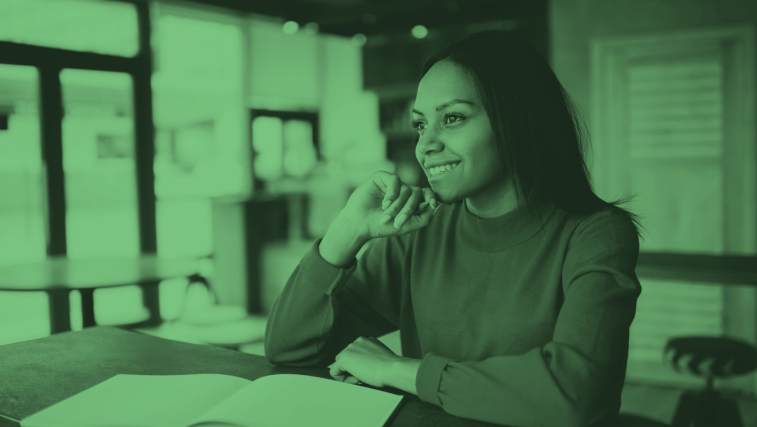
A Closer Look at New Year’s Resolutions
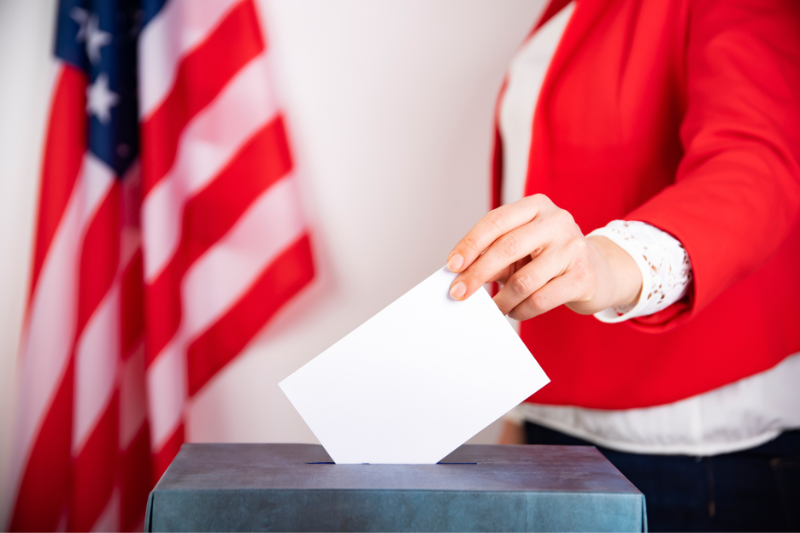
The Swing Voter Project in Nevada – January 2024
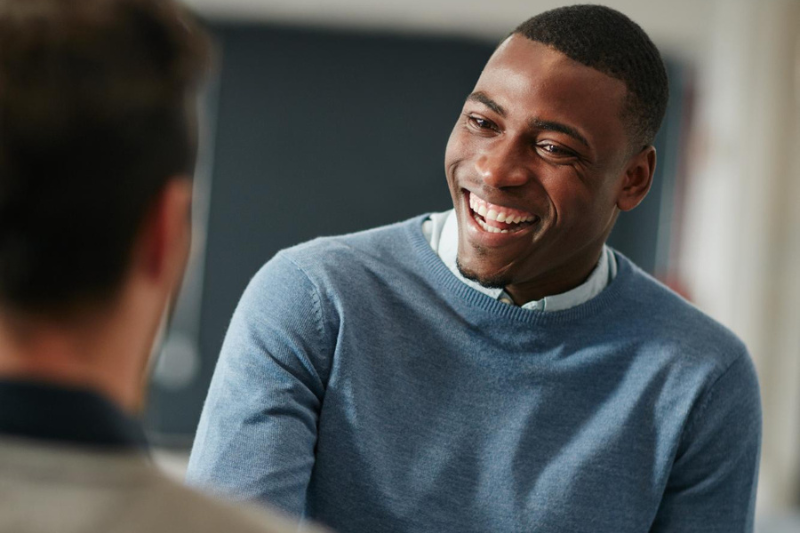
OnDemand: The Secret Sauce to Finding Perfect Participants: Strategies for Recruiting Hard-to-Reach Audiences
Take a deep dive into your favorite market research topics
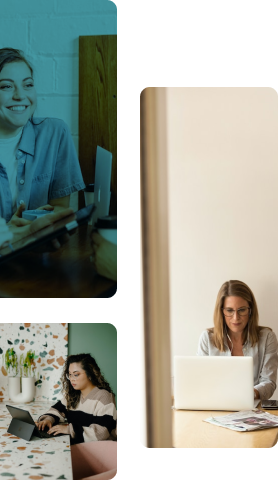
How can we help support you and your research needs?
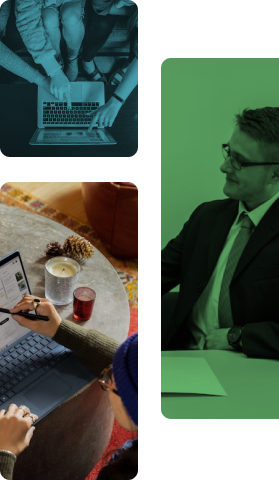
BEFORE YOU GO
Have you considered how to harness AI in your research process? Check out our on-demand webinar for everything you need to know

- Methodology
- Open access
- Published: 11 October 2016
Reviewing the research methods literature: principles and strategies illustrated by a systematic overview of sampling in qualitative research
- Stephen J. Gentles 1 , 4 ,
- Cathy Charles 1 ,
- David B. Nicholas 2 ,
- Jenny Ploeg 3 &
- K. Ann McKibbon 1
Systematic Reviews volume 5 , Article number: 172 ( 2016 ) Cite this article
50k Accesses
25 Citations
13 Altmetric
Metrics details
Overviews of methods are potentially useful means to increase clarity and enhance collective understanding of specific methods topics that may be characterized by ambiguity, inconsistency, or a lack of comprehensiveness. This type of review represents a distinct literature synthesis method, although to date, its methodology remains relatively undeveloped despite several aspects that demand unique review procedures. The purpose of this paper is to initiate discussion about what a rigorous systematic approach to reviews of methods, referred to here as systematic methods overviews , might look like by providing tentative suggestions for approaching specific challenges likely to be encountered. The guidance offered here was derived from experience conducting a systematic methods overview on the topic of sampling in qualitative research.
The guidance is organized into several principles that highlight specific objectives for this type of review given the common challenges that must be overcome to achieve them. Optional strategies for achieving each principle are also proposed, along with discussion of how they were successfully implemented in the overview on sampling. We describe seven paired principles and strategies that address the following aspects: delimiting the initial set of publications to consider, searching beyond standard bibliographic databases, searching without the availability of relevant metadata, selecting publications on purposeful conceptual grounds, defining concepts and other information to abstract iteratively, accounting for inconsistent terminology used to describe specific methods topics, and generating rigorous verifiable analytic interpretations. Since a broad aim in systematic methods overviews is to describe and interpret the relevant literature in qualitative terms, we suggest that iterative decision making at various stages of the review process, and a rigorous qualitative approach to analysis are necessary features of this review type.
Conclusions
We believe that the principles and strategies provided here will be useful to anyone choosing to undertake a systematic methods overview. This paper represents an initial effort to promote high quality critical evaluations of the literature regarding problematic methods topics, which have the potential to promote clearer, shared understandings, and accelerate advances in research methods. Further work is warranted to develop more definitive guidance.
Peer Review reports
While reviews of methods are not new, they represent a distinct review type whose methodology remains relatively under-addressed in the literature despite the clear implications for unique review procedures. One of few examples to describe it is a chapter containing reflections of two contributing authors in a book of 21 reviews on methodological topics compiled for the British National Health Service, Health Technology Assessment Program [ 1 ]. Notable is their observation of how the differences between the methods reviews and conventional quantitative systematic reviews, specifically attributable to their varying content and purpose, have implications for defining what qualifies as systematic. While the authors describe general aspects of “systematicity” (including rigorous application of a methodical search, abstraction, and analysis), they also describe a high degree of variation within the category of methods reviews itself and so offer little in the way of concrete guidance. In this paper, we present tentative concrete guidance, in the form of a preliminary set of proposed principles and optional strategies, for a rigorous systematic approach to reviewing and evaluating the literature on quantitative or qualitative methods topics. For purposes of this article, we have used the term systematic methods overview to emphasize the notion of a systematic approach to such reviews.
The conventional focus of rigorous literature reviews (i.e., review types for which systematic methods have been codified, including the various approaches to quantitative systematic reviews [ 2 – 4 ], and the numerous forms of qualitative and mixed methods literature synthesis [ 5 – 10 ]) is to synthesize empirical research findings from multiple studies. By contrast, the focus of overviews of methods, including the systematic approach we advocate, is to synthesize guidance on methods topics. The literature consulted for such reviews may include the methods literature, methods-relevant sections of empirical research reports, or both. Thus, this paper adds to previous work published in this journal—namely, recent preliminary guidance for conducting reviews of theory [ 11 ]—that has extended the application of systematic review methods to novel review types that are concerned with subject matter other than empirical research findings.
Published examples of methods overviews illustrate the varying objectives they can have. One objective is to establish methodological standards for appraisal purposes. For example, reviews of existing quality appraisal standards have been used to propose universal standards for appraising the quality of primary qualitative research [ 12 ] or evaluating qualitative research reports [ 13 ]. A second objective is to survey the methods-relevant sections of empirical research reports to establish current practices on methods use and reporting practices, which Moher and colleagues [ 14 ] recommend as a means for establishing the needs to be addressed in reporting guidelines (see, for example [ 15 , 16 ]). A third objective for a methods review is to offer clarity and enhance collective understanding regarding a specific methods topic that may be characterized by ambiguity, inconsistency, or a lack of comprehensiveness within the available methods literature. An example of this is a overview whose objective was to review the inconsistent definitions of intention-to-treat analysis (the methodologically preferred approach to analyze randomized controlled trial data) that have been offered in the methods literature and propose a solution for improving conceptual clarity [ 17 ]. Such reviews are warranted because students and researchers who must learn or apply research methods typically lack the time to systematically search, retrieve, review, and compare the available literature to develop a thorough and critical sense of the varied approaches regarding certain controversial or ambiguous methods topics.
While systematic methods overviews , as a review type, include both reviews of the methods literature and reviews of methods-relevant sections from empirical study reports, the guidance provided here is primarily applicable to reviews of the methods literature since it was derived from the experience of conducting such a review [ 18 ], described below. To our knowledge, there are no well-developed proposals on how to rigorously conduct such reviews. Such guidance would have the potential to improve the thoroughness and credibility of critical evaluations of the methods literature, which could increase their utility as a tool for generating understandings that advance research methods, both qualitative and quantitative. Our aim in this paper is thus to initiate discussion about what might constitute a rigorous approach to systematic methods overviews. While we hope to promote rigor in the conduct of systematic methods overviews wherever possible, we do not wish to suggest that all methods overviews need be conducted to the same standard. Rather, we believe that the level of rigor may need to be tailored pragmatically to the specific review objectives, which may not always justify the resource requirements of an intensive review process.
The example systematic methods overview on sampling in qualitative research
The principles and strategies we propose in this paper are derived from experience conducting a systematic methods overview on the topic of sampling in qualitative research [ 18 ]. The main objective of that methods overview was to bring clarity and deeper understanding of the prominent concepts related to sampling in qualitative research (purposeful sampling strategies, saturation, etc.). Specifically, we interpreted the available guidance, commenting on areas lacking clarity, consistency, or comprehensiveness (without proposing any recommendations on how to do sampling). This was achieved by a comparative and critical analysis of publications representing the most influential (i.e., highly cited) guidance across several methodological traditions in qualitative research.
The specific methods and procedures for the overview on sampling [ 18 ] from which our proposals are derived were developed both after soliciting initial input from local experts in qualitative research and an expert health librarian (KAM) and through ongoing careful deliberation throughout the review process. To summarize, in that review, we employed a transparent and rigorous approach to search the methods literature, selected publications for inclusion according to a purposeful and iterative process, abstracted textual data using structured abstraction forms, and analyzed (synthesized) the data using a systematic multi-step approach featuring abstraction of text, summary of information in matrices, and analytic comparisons.
For this article, we reflected on both the problems and challenges encountered at different stages of the review and our means for selecting justifiable procedures to deal with them. Several principles were then derived by considering the generic nature of these problems, while the generalizable aspects of the procedures used to address them formed the basis of optional strategies. Further details of the specific methods and procedures used in the overview on qualitative sampling are provided below to illustrate both the types of objectives and challenges that reviewers will likely need to consider and our approach to implementing each of the principles and strategies.
Organization of the guidance into principles and strategies
For the purposes of this article, principles are general statements outlining what we propose are important aims or considerations within a particular review process, given the unique objectives or challenges to be overcome with this type of review. These statements follow the general format, “considering the objective or challenge of X, we propose Y to be an important aim or consideration.” Strategies are optional and flexible approaches for implementing the previous principle outlined. Thus, generic challenges give rise to principles, which in turn give rise to strategies.
We organize the principles and strategies below into three sections corresponding to processes characteristic of most systematic literature synthesis approaches: literature identification and selection ; data abstraction from the publications selected for inclusion; and analysis , including critical appraisal and synthesis of the abstracted data. Within each section, we also describe the specific methodological decisions and procedures used in the overview on sampling in qualitative research [ 18 ] to illustrate how the principles and strategies for each review process were applied and implemented in a specific case. We expect this guidance and accompanying illustrations will be useful for anyone considering engaging in a methods overview, particularly those who may be familiar with conventional systematic review methods but may not yet appreciate some of the challenges specific to reviewing the methods literature.
Results and discussion
Literature identification and selection.
The identification and selection process includes search and retrieval of publications and the development and application of inclusion and exclusion criteria to select the publications that will be abstracted and analyzed in the final review. Literature identification and selection for overviews of the methods literature is challenging and potentially more resource-intensive than for most reviews of empirical research. This is true for several reasons that we describe below, alongside discussion of the potential solutions. Additionally, we suggest in this section how the selection procedures can be chosen to match the specific analytic approach used in methods overviews.
Delimiting a manageable set of publications
One aspect of methods overviews that can make identification and selection challenging is the fact that the universe of literature containing potentially relevant information regarding most methods-related topics is expansive and often unmanageably so. Reviewers are faced with two large categories of literature: the methods literature , where the possible publication types include journal articles, books, and book chapters; and the methods-relevant sections of empirical study reports , where the possible publication types include journal articles, monographs, books, theses, and conference proceedings. In our systematic overview of sampling in qualitative research, exhaustively searching (including retrieval and first-pass screening) all publication types across both categories of literature for information on a single methods-related topic was too burdensome to be feasible. The following proposed principle follows from the need to delimit a manageable set of literature for the review.
Principle #1:
Considering the broad universe of potentially relevant literature, we propose that an important objective early in the identification and selection stage is to delimit a manageable set of methods-relevant publications in accordance with the objectives of the methods overview.
Strategy #1:
To limit the set of methods-relevant publications that must be managed in the selection process, reviewers have the option to initially review only the methods literature, and exclude the methods-relevant sections of empirical study reports, provided this aligns with the review’s particular objectives.
We propose that reviewers are justified in choosing to select only the methods literature when the objective is to map out the range of recognized concepts relevant to a methods topic, to summarize the most authoritative or influential definitions or meanings for methods-related concepts, or to demonstrate a problematic lack of clarity regarding a widely established methods-related concept and potentially make recommendations for a preferred approach to the methods topic in question. For example, in the case of the methods overview on sampling [ 18 ], the primary aim was to define areas lacking in clarity for multiple widely established sampling-related topics. In the review on intention-to-treat in the context of missing outcome data [ 17 ], the authors identified a lack of clarity based on multiple inconsistent definitions in the literature and went on to recommend separating the issue of how to handle missing outcome data from the issue of whether an intention-to-treat analysis can be claimed.
In contrast to strategy #1, it may be appropriate to select the methods-relevant sections of empirical study reports when the objective is to illustrate how a methods concept is operationalized in research practice or reported by authors. For example, one could review all the publications in 2 years’ worth of issues of five high-impact field-related journals to answer questions about how researchers describe implementing a particular method or approach, or to quantify how consistently they define or report using it. Such reviews are often used to highlight gaps in the reporting practices regarding specific methods, which may be used to justify items to address in reporting guidelines (for example, [ 14 – 16 ]).
It is worth recognizing that other authors have advocated broader positions regarding the scope of literature to be considered in a review, expanding on our perspective. Suri [ 10 ] (who, like us, emphasizes how different sampling strategies are suitable for different literature synthesis objectives) has, for example, described a two-stage literature sampling procedure (pp. 96–97). First, reviewers use an initial approach to conduct a broad overview of the field—for reviews of methods topics, this would entail an initial review of the research methods literature. This is followed by a second more focused stage in which practical examples are purposefully selected—for methods reviews, this would involve sampling the empirical literature to illustrate key themes and variations. While this approach is seductive in its capacity to generate more in depth and interpretive analytic findings, some reviewers may consider it too resource-intensive to include the second step no matter how selective the purposeful sampling. In the overview on sampling where we stopped after the first stage [ 18 ], we discussed our selective focus on the methods literature as a limitation that left opportunities for further analysis of the literature. We explicitly recommended, for example, that theoretical sampling was a topic for which a future review of the methods sections of empirical reports was justified to answer specific questions identified in the primary review.
Ultimately, reviewers must make pragmatic decisions that balance resource considerations, combined with informed predictions about the depth and complexity of literature available on their topic, with the stated objectives of their review. The remaining principles and strategies apply primarily to overviews that include the methods literature, although some aspects may be relevant to reviews that include empirical study reports.
Searching beyond standard bibliographic databases
An important reality affecting identification and selection in overviews of the methods literature is the increased likelihood for relevant publications to be located in sources other than journal articles (which is usually not the case for overviews of empirical research, where journal articles generally represent the primary publication type). In the overview on sampling [ 18 ], out of 41 full-text publications retrieved and reviewed, only 4 were journal articles, while 37 were books or book chapters. Since many books and book chapters did not exist electronically, their full text had to be physically retrieved in hardcopy, while 11 publications were retrievable only through interlibrary loan or purchase request. The tasks associated with such retrieval are substantially more time-consuming than electronic retrieval. Since a substantial proportion of methods-related guidance may be located in publication types that are less comprehensively indexed in standard bibliographic databases, identification and retrieval thus become complicated processes.
Principle #2:
Considering that important sources of methods guidance can be located in non-journal publication types (e.g., books, book chapters) that tend to be poorly indexed in standard bibliographic databases, it is important to consider alternative search methods for identifying relevant publications to be further screened for inclusion.
Strategy #2:
To identify books, book chapters, and other non-journal publication types not thoroughly indexed in standard bibliographic databases, reviewers may choose to consult one or more of the following less standard sources: Google Scholar, publisher web sites, or expert opinion.
In the case of the overview on sampling in qualitative research [ 18 ], Google Scholar had two advantages over other standard bibliographic databases: it indexes and returns records of books and book chapters likely to contain guidance on qualitative research methods topics; and it has been validated as providing higher citation counts than ISI Web of Science (a producer of numerous bibliographic databases accessible through institutional subscription) for several non-biomedical disciplines including the social sciences where qualitative research methods are prominently used [ 19 – 21 ]. While we identified numerous useful publications by consulting experts, the author publication lists generated through Google Scholar searches were uniquely useful to identify more recent editions of methods books identified by experts.
Searching without relevant metadata
Determining what publications to select for inclusion in the overview on sampling [ 18 ] could only rarely be accomplished by reviewing the publication’s metadata. This was because for the many books and other non-journal type publications we identified as possibly relevant, the potential content of interest would be located in only a subsection of the publication. In this common scenario for reviews of the methods literature (as opposed to methods overviews that include empirical study reports), reviewers will often be unable to employ standard title, abstract, and keyword database searching or screening as a means for selecting publications.
Principle #3:
Considering that the presence of information about the topic of interest may not be indicated in the metadata for books and similar publication types, it is important to consider other means of identifying potentially useful publications for further screening.
Strategy #3:
One approach to identifying potentially useful books and similar publication types is to consider what classes of such publications (e.g., all methods manuals for a certain research approach) are likely to contain relevant content, then identify, retrieve, and review the full text of corresponding publications to determine whether they contain information on the topic of interest.
In the example of the overview on sampling in qualitative research [ 18 ], the topic of interest (sampling) was one of numerous topics covered in the general qualitative research methods manuals. Consequently, examples from this class of publications first had to be identified for retrieval according to non-keyword-dependent criteria. Thus, all methods manuals within the three research traditions reviewed (grounded theory, phenomenology, and case study) that might contain discussion of sampling were sought through Google Scholar and expert opinion, their full text obtained, and hand-searched for relevant content to determine eligibility. We used tables of contents and index sections of books to aid this hand searching.
Purposefully selecting literature on conceptual grounds
A final consideration in methods overviews relates to the type of analysis used to generate the review findings. Unlike quantitative systematic reviews where reviewers aim for accurate or unbiased quantitative estimates—something that requires identifying and selecting the literature exhaustively to obtain all relevant data available (i.e., a complete sample)—in methods overviews, reviewers must describe and interpret the relevant literature in qualitative terms to achieve review objectives. In other words, the aim in methods overviews is to seek coverage of the qualitative concepts relevant to the methods topic at hand. For example, in the overview of sampling in qualitative research [ 18 ], achieving review objectives entailed providing conceptual coverage of eight sampling-related topics that emerged as key domains. The following principle recognizes that literature sampling should therefore support generating qualitative conceptual data as the input to analysis.
Principle #4:
Since the analytic findings of a systematic methods overview are generated through qualitative description and interpretation of the literature on a specified topic, selection of the literature should be guided by a purposeful strategy designed to achieve adequate conceptual coverage (i.e., representing an appropriate degree of variation in relevant ideas) of the topic according to objectives of the review.
Strategy #4:
One strategy for choosing the purposeful approach to use in selecting the literature according to the review objectives is to consider whether those objectives imply exploring concepts either at a broad overview level, in which case combining maximum variation selection with a strategy that limits yield (e.g., critical case, politically important, or sampling for influence—described below) may be appropriate; or in depth, in which case purposeful approaches aimed at revealing innovative cases will likely be necessary.
In the methods overview on sampling, the implied scope was broad since we set out to review publications on sampling across three divergent qualitative research traditions—grounded theory, phenomenology, and case study—to facilitate making informative conceptual comparisons. Such an approach would be analogous to maximum variation sampling.
At the same time, the purpose of that review was to critically interrogate the clarity, consistency, and comprehensiveness of literature from these traditions that was “most likely to have widely influenced students’ and researchers’ ideas about sampling” (p. 1774) [ 18 ]. In other words, we explicitly set out to review and critique the most established and influential (and therefore dominant) literature, since this represents a common basis of knowledge among students and researchers seeking understanding or practical guidance on sampling in qualitative research. To achieve this objective, we purposefully sampled publications according to the criterion of influence , which we operationalized as how often an author or publication has been referenced in print or informal discourse. This second sampling approach also limited the literature we needed to consider within our broad scope review to a manageable amount.
To operationalize this strategy of sampling for influence , we sought to identify both the most influential authors within a qualitative research tradition (all of whose citations were subsequently screened) and the most influential publications on the topic of interest by non-influential authors. This involved a flexible approach that combined multiple indicators of influence to avoid the dilemma that any single indicator might provide inadequate coverage. These indicators included bibliometric data (h-index for author influence [ 22 ]; number of cites for publication influence), expert opinion, and cross-references in the literature (i.e., snowball sampling). As a final selection criterion, a publication was included only if it made an original contribution in terms of novel guidance regarding sampling or a related concept; thus, purely secondary sources were excluded. Publish or Perish software (Anne-Wil Harzing; available at http://www.harzing.com/resources/publish-or-perish ) was used to generate bibliometric data via the Google Scholar database. Figure 1 illustrates how identification and selection in the methods overview on sampling was a multi-faceted and iterative process. The authors selected as influential, and the publications selected for inclusion or exclusion are listed in Additional file 1 (Matrices 1, 2a, 2b).
Literature identification and selection process used in the methods overview on sampling [ 18 ]
In summary, the strategies of seeking maximum variation and sampling for influence were employed in the sampling overview to meet the specific review objectives described. Reviewers will need to consider the full range of purposeful literature sampling approaches at their disposal in deciding what best matches the specific aims of their own reviews. Suri [ 10 ] has recently retooled Patton’s well-known typology of purposeful sampling strategies (originally intended for primary research) for application to literature synthesis, providing a useful resource in this respect.
Data abstraction
The purpose of data abstraction in rigorous literature reviews is to locate and record all data relevant to the topic of interest from the full text of included publications, making them available for subsequent analysis. Conventionally, a data abstraction form—consisting of numerous distinct conceptually defined fields to which corresponding information from the source publication is recorded—is developed and employed. There are several challenges, however, to the processes of developing the abstraction form and abstracting the data itself when conducting methods overviews, which we address here. Some of these problems and their solutions may be familiar to those who have conducted qualitative literature syntheses, which are similarly conceptual.
Iteratively defining conceptual information to abstract
In the overview on sampling [ 18 ], while we surveyed multiple sources beforehand to develop a list of concepts relevant for abstraction (e.g., purposeful sampling strategies, saturation, sample size), there was no way for us to anticipate some concepts prior to encountering them in the review process. Indeed, in many cases, reviewers are unable to determine the complete set of methods-related concepts that will be the focus of the final review a priori without having systematically reviewed the publications to be included. Thus, defining what information to abstract beforehand may not be feasible.
Principle #5:
Considering the potential impracticality of defining a complete set of relevant methods-related concepts from a body of literature one has not yet systematically read, selecting and defining fields for data abstraction must often be undertaken iteratively. Thus, concepts to be abstracted can be expected to grow and change as data abstraction proceeds.
Strategy #5:
Reviewers can develop an initial form or set of concepts for abstraction purposes according to standard methods (e.g., incorporating expert feedback, pilot testing) and remain attentive to the need to iteratively revise it as concepts are added or modified during the review. Reviewers should document revisions and return to re-abstract data from previously abstracted publications as the new data requirements are determined.
In the sampling overview [ 18 ], we developed and maintained the abstraction form in Microsoft Word. We derived the initial set of abstraction fields from our own knowledge of relevant sampling-related concepts, consultation with local experts, and reviewing a pilot sample of publications. Since the publications in this review included a large proportion of books, the abstraction process often began by flagging the broad sections within a publication containing topic-relevant information for detailed review to identify text to abstract. When reviewing flagged text, the reviewer occasionally encountered an unanticipated concept significant enough to warrant being added as a new field to the abstraction form. For example, a field was added to capture how authors described the timing of sampling decisions, whether before (a priori) or after (ongoing) starting data collection, or whether this was unclear. In these cases, we systematically documented the modification to the form and returned to previously abstracted publications to abstract any information that might be relevant to the new field.
The logic of this strategy is analogous to the logic used in a form of research synthesis called best fit framework synthesis (BFFS) [ 23 – 25 ]. In that method, reviewers initially code evidence using an a priori framework they have selected. When evidence cannot be accommodated by the selected framework, reviewers then develop new themes or concepts from which they construct a new expanded framework. Both the strategy proposed and the BFFS approach to research synthesis are notable for their rigorous and transparent means to adapt a final set of concepts to the content under review.
Accounting for inconsistent terminology
An important complication affecting the abstraction process in methods overviews is that the language used by authors to describe methods-related concepts can easily vary across publications. For example, authors from different qualitative research traditions often use different terms for similar methods-related concepts. Furthermore, as we found in the sampling overview [ 18 ], there may be cases where no identifiable term, phrase, or label for a methods-related concept is used at all, and a description of it is given instead. This can make searching the text for relevant concepts based on keywords unreliable.
Principle #6:
Since accepted terms may not be used consistently to refer to methods concepts, it is necessary to rely on the definitions for concepts, rather than keywords, to identify relevant information in the publication to abstract.
Strategy #6:
An effective means to systematically identify relevant information is to develop and iteratively adjust written definitions for key concepts (corresponding to abstraction fields) that are consistent with and as inclusive of as much of the literature reviewed as possible. Reviewers then seek information that matches these definitions (rather than keywords) when scanning a publication for relevant data to abstract.
In the abstraction process for the sampling overview [ 18 ], we noted the several concepts of interest to the review for which abstraction by keyword was particularly problematic due to inconsistent terminology across publications: sampling , purposeful sampling , sampling strategy , and saturation (for examples, see Additional file 1 , Matrices 3a, 3b, 4). We iteratively developed definitions for these concepts by abstracting text from publications that either provided an explicit definition or from which an implicit definition could be derived, which was recorded in fields dedicated to the concept’s definition. Using a method of constant comparison, we used text from definition fields to inform and modify a centrally maintained definition of the corresponding concept to optimize its fit and inclusiveness with the literature reviewed. Table 1 shows, as an example, the final definition constructed in this way for one of the central concepts of the review, qualitative sampling .
We applied iteratively developed definitions when making decisions about what specific text to abstract for an existing field, which allowed us to abstract concept-relevant data even if no recognized keyword was used. For example, this was the case for the sampling-related concept, saturation , where the relevant text available for abstraction in one publication [ 26 ]—“to continue to collect data until nothing new was being observed or recorded, no matter how long that takes”—was not accompanied by any term or label whatsoever.
This comparative analytic strategy (and our approach to analysis more broadly as described in strategy #7, below) is analogous to the process of reciprocal translation —a technique first introduced for meta-ethnography by Noblit and Hare [ 27 ] that has since been recognized as a common element in a variety of qualitative metasynthesis approaches [ 28 ]. Reciprocal translation, taken broadly, involves making sense of a study’s findings in terms of the findings of the other studies included in the review. In practice, it has been operationalized in different ways. Melendez-Torres and colleagues developed a typology from their review of the metasynthesis literature, describing four overlapping categories of specific operations undertaken in reciprocal translation: visual representation, key paper integration, data reduction and thematic extraction, and line-by-line coding [ 28 ]. The approaches suggested in both strategies #6 and #7, with their emphasis on constant comparison, appear to fall within the line-by-line coding category.
Generating credible and verifiable analytic interpretations
The analysis in a systematic methods overview must support its more general objective, which we suggested above is often to offer clarity and enhance collective understanding regarding a chosen methods topic. In our experience, this involves describing and interpreting the relevant literature in qualitative terms. Furthermore, any interpretative analysis required may entail reaching different levels of abstraction, depending on the more specific objectives of the review. For example, in the overview on sampling [ 18 ], we aimed to produce a comparative analysis of how multiple sampling-related topics were treated differently within and among different qualitative research traditions. To promote credibility of the review, however, not only should one seek a qualitative analytic approach that facilitates reaching varying levels of abstraction but that approach must also ensure that abstract interpretations are supported and justified by the source data and not solely the product of the analyst’s speculative thinking.
Principle #7:
Considering the qualitative nature of the analysis required in systematic methods overviews, it is important to select an analytic method whose interpretations can be verified as being consistent with the literature selected, regardless of the level of abstraction reached.
Strategy #7:
We suggest employing the constant comparative method of analysis [ 29 ] because it supports developing and verifying analytic links to the source data throughout progressively interpretive or abstract levels. In applying this approach, we advise a rigorous approach, documenting how supportive quotes or references to the original texts are carried forward in the successive steps of analysis to allow for easy verification.
The analytic approach used in the methods overview on sampling [ 18 ] comprised four explicit steps, progressing in level of abstraction—data abstraction, matrices, narrative summaries, and final analytic conclusions (Fig. 2 ). While we have positioned data abstraction as the second stage of the generic review process (prior to Analysis), above, we also considered it as an initial step of analysis in the sampling overview for several reasons. First, it involved a process of constant comparisons and iterative decision-making about the fields to add or define during development and modification of the abstraction form, through which we established the range of concepts to be addressed in the review. At the same time, abstraction involved continuous analytic decisions about what textual quotes (ranging in size from short phrases to numerous paragraphs) to record in the fields thus created. This constant comparative process was analogous to open coding in which textual data from publications was compared to conceptual fields (equivalent to codes) or to other instances of data previously abstracted when constructing definitions to optimize their fit with the overall literature as described in strategy #6. Finally, in the data abstraction step, we also recorded our first interpretive thoughts in dedicated fields, providing initial material for the more abstract analytic steps.
Summary of progressive steps of analysis used in the methods overview on sampling [ 18 ]
In the second step of the analysis, we constructed topic-specific matrices , or tables, by copying relevant quotes from abstraction forms into the appropriate cells of matrices (for the complete set of analytic matrices developed in the sampling review, see Additional file 1 (matrices 3 to 10)). Each matrix ranged from one to five pages; row headings, nested three-deep, identified the methodological tradition, author, and publication, respectively; and column headings identified the concepts, which corresponded to abstraction fields. Matrices thus allowed us to make further comparisons across methodological traditions, and between authors within a tradition. In the third step of analysis, we recorded our comparative observations as narrative summaries , in which we used illustrative quotes more sparingly. In the final step, we developed analytic conclusions based on the narrative summaries about the sampling-related concepts within each methodological tradition for which clarity, consistency, or comprehensiveness of the available guidance appeared to be lacking. Higher levels of analysis thus built logically from the lower levels, enabling us to easily verify analytic conclusions by tracing the support for claims by comparing the original text of publications reviewed.
Integrative versus interpretive methods overviews
The analytic product of systematic methods overviews is comparable to qualitative evidence syntheses, since both involve describing and interpreting the relevant literature in qualitative terms. Most qualitative synthesis approaches strive to produce new conceptual understandings that vary in level of interpretation. Dixon-Woods and colleagues [ 30 ] elaborate on a useful distinction, originating from Noblit and Hare [ 27 ], between integrative and interpretive reviews. Integrative reviews focus on summarizing available primary data and involve using largely secure and well defined concepts to do so; definitions are used from an early stage to specify categories for abstraction (or coding) of data, which in turn supports their aggregation; they do not seek as their primary focus to develop or specify new concepts, although they may achieve some theoretical or interpretive functions. For interpretive reviews, meanwhile, the main focus is to develop new concepts and theories that integrate them, with the implication that the concepts developed become fully defined towards the end of the analysis. These two forms are not completely distinct, and “every integrative synthesis will include elements of interpretation, and every interpretive synthesis will include elements of aggregation of data” [ 30 ].
The example methods overview on sampling [ 18 ] could be classified as predominantly integrative because its primary goal was to aggregate influential authors’ ideas on sampling-related concepts; there were also, however, elements of interpretive synthesis since it aimed to develop new ideas about where clarity in guidance on certain sampling-related topics is lacking, and definitions for some concepts were flexible and not fixed until late in the review. We suggest that most systematic methods overviews will be classifiable as predominantly integrative (aggregative). Nevertheless, more highly interpretive methods overviews are also quite possible—for example, when the review objective is to provide a highly critical analysis for the purpose of generating new methodological guidance. In such cases, reviewers may need to sample more deeply (see strategy #4), specifically by selecting empirical research reports (i.e., to go beyond dominant or influential ideas in the methods literature) that are likely to feature innovations or instructive lessons in employing a given method.
In this paper, we have outlined tentative guidance in the form of seven principles and strategies on how to conduct systematic methods overviews, a review type in which methods-relevant literature is systematically analyzed with the aim of offering clarity and enhancing collective understanding regarding a specific methods topic. Our proposals include strategies for delimiting the set of publications to consider, searching beyond standard bibliographic databases, searching without the availability of relevant metadata, selecting publications on purposeful conceptual grounds, defining concepts and other information to abstract iteratively, accounting for inconsistent terminology, and generating credible and verifiable analytic interpretations. We hope the suggestions proposed will be useful to others undertaking reviews on methods topics in future.
As far as we are aware, this is the first published source of concrete guidance for conducting this type of review. It is important to note that our primary objective was to initiate methodological discussion by stimulating reflection on what rigorous methods for this type of review should look like, leaving the development of more complete guidance to future work. While derived from the experience of reviewing a single qualitative methods topic, we believe the principles and strategies provided are generalizable to overviews of both qualitative and quantitative methods topics alike. However, it is expected that additional challenges and insights for conducting such reviews have yet to be defined. Thus, we propose that next steps for developing more definitive guidance should involve an attempt to collect and integrate other reviewers’ perspectives and experiences in conducting systematic methods overviews on a broad range of qualitative and quantitative methods topics. Formalized guidance and standards would improve the quality of future methods overviews, something we believe has important implications for advancing qualitative and quantitative methodology. When undertaken to a high standard, rigorous critical evaluations of the available methods guidance have significant potential to make implicit controversies explicit, and improve the clarity and precision of our understandings of problematic qualitative or quantitative methods issues.
A review process central to most types of rigorous reviews of empirical studies, which we did not explicitly address in a separate review step above, is quality appraisal . The reason we have not treated this as a separate step stems from the different objectives of the primary publications included in overviews of the methods literature (i.e., providing methodological guidance) compared to the primary publications included in the other established review types (i.e., reporting findings from single empirical studies). This is not to say that appraising quality of the methods literature is not an important concern for systematic methods overviews. Rather, appraisal is much more integral to (and difficult to separate from) the analysis step, in which we advocate appraising clarity, consistency, and comprehensiveness—the quality appraisal criteria that we suggest are appropriate for the methods literature. As a second important difference regarding appraisal, we currently advocate appraising the aforementioned aspects at the level of the literature in aggregate rather than at the level of individual publications. One reason for this is that methods guidance from individual publications generally builds on previous literature, and thus we feel that ahistorical judgments about comprehensiveness of single publications lack relevance and utility. Additionally, while different methods authors may express themselves less clearly than others, their guidance can nonetheless be highly influential and useful, and should therefore not be downgraded or ignored based on considerations of clarity—which raises questions about the alternative uses that quality appraisals of individual publications might have. Finally, legitimate variability in the perspectives that methods authors wish to emphasize, and the levels of generality at which they write about methods, makes critiquing individual publications based on the criterion of clarity a complex and potentially problematic endeavor that is beyond the scope of this paper to address. By appraising the current state of the literature at a holistic level, reviewers stand to identify important gaps in understanding that represent valuable opportunities for further methodological development.
To summarize, the principles and strategies provided here may be useful to those seeking to undertake their own systematic methods overview. Additional work is needed, however, to establish guidance that is comprehensive by comparing the experiences from conducting a variety of methods overviews on a range of methods topics. Efforts that further advance standards for systematic methods overviews have the potential to promote high-quality critical evaluations that produce conceptually clear and unified understandings of problematic methods topics, thereby accelerating the advance of research methodology.
Hutton JL, Ashcroft R. What does “systematic” mean for reviews of methods? In: Black N, Brazier J, Fitzpatrick R, Reeves B, editors. Health services research methods: a guide to best practice. London: BMJ Publishing Group; 1998. p. 249–54.
Google Scholar
Cochrane handbook for systematic reviews of interventions. In. Edited by Higgins JPT, Green S, Version 5.1.0 edn: The Cochrane Collaboration; 2011.
Centre for Reviews and Dissemination: Systematic reviews: CRD’s guidance for undertaking reviews in health care . York: Centre for Reviews and Dissemination; 2009.
Liberati A, Altman DG, Tetzlaff J, Mulrow C, Gotzsche PC, Ioannidis JPA, Clarke M, Devereaux PJ, Kleijnen J, Moher D. The PRISMA statement for reporting systematic reviews and meta-analyses of studies that evaluate healthcare interventions: explanation and elaboration. BMJ. 2009;339:b2700–0.
Barnett-Page E, Thomas J. Methods for the synthesis of qualitative research: a critical review. BMC Med Res Methodol. 2009;9(1):59.
Article PubMed PubMed Central Google Scholar
Kastner M, Tricco AC, Soobiah C, Lillie E, Perrier L, Horsley T, Welch V, Cogo E, Antony J, Straus SE. What is the most appropriate knowledge synthesis method to conduct a review? Protocol for a scoping review. BMC Med Res Methodol. 2012;12(1):1–1.
Article Google Scholar
Booth A, Noyes J, Flemming K, Gerhardus A. Guidance on choosing qualitative evidence synthesis methods for use in health technology assessments of complex interventions. In: Integrate-HTA. 2016.
Booth A, Sutton A, Papaioannou D. Systematic approaches to successful literature review. 2nd ed. London: Sage; 2016.
Hannes K, Lockwood C. Synthesizing qualitative research: choosing the right approach. Chichester: Wiley-Blackwell; 2012.
Suri H. Towards methodologically inclusive research syntheses: expanding possibilities. New York: Routledge; 2014.
Campbell M, Egan M, Lorenc T, Bond L, Popham F, Fenton C, Benzeval M. Considering methodological options for reviews of theory: illustrated by a review of theories linking income and health. Syst Rev. 2014;3(1):1–11.
Cohen DJ, Crabtree BF. Evaluative criteria for qualitative research in health care: controversies and recommendations. Ann Fam Med. 2008;6(4):331–9.
Tong A, Sainsbury P, Craig J. Consolidated criteria for reportingqualitative research (COREQ): a 32-item checklist for interviews and focus groups. Int J Qual Health Care. 2007;19(6):349–57.
Article PubMed Google Scholar
Moher D, Schulz KF, Simera I, Altman DG. Guidance for developers of health research reporting guidelines. PLoS Med. 2010;7(2):e1000217.
Moher D, Tetzlaff J, Tricco AC, Sampson M, Altman DG. Epidemiology and reporting characteristics of systematic reviews. PLoS Med. 2007;4(3):e78.
Chan AW, Altman DG. Epidemiology and reporting of randomised trials published in PubMed journals. Lancet. 2005;365(9465):1159–62.
Alshurafa M, Briel M, Akl EA, Haines T, Moayyedi P, Gentles SJ, Rios L, Tran C, Bhatnagar N, Lamontagne F, et al. Inconsistent definitions for intention-to-treat in relation to missing outcome data: systematic review of the methods literature. PLoS One. 2012;7(11):e49163.
Article CAS PubMed PubMed Central Google Scholar
Gentles SJ, Charles C, Ploeg J, McKibbon KA. Sampling in qualitative research: insights from an overview of the methods literature. Qual Rep. 2015;20(11):1772–89.
Harzing A-W, Alakangas S. Google Scholar, Scopus and the Web of Science: a longitudinal and cross-disciplinary comparison. Scientometrics. 2016;106(2):787–804.
Harzing A-WK, van der Wal R. Google Scholar as a new source for citation analysis. Ethics Sci Environ Polit. 2008;8(1):61–73.
Kousha K, Thelwall M. Google Scholar citations and Google Web/URL citations: a multi‐discipline exploratory analysis. J Assoc Inf Sci Technol. 2007;58(7):1055–65.
Hirsch JE. An index to quantify an individual’s scientific research output. Proc Natl Acad Sci U S A. 2005;102(46):16569–72.
Booth A, Carroll C. How to build up the actionable knowledge base: the role of ‘best fit’ framework synthesis for studies of improvement in healthcare. BMJ Quality Safety. 2015;24(11):700–8.
Carroll C, Booth A, Leaviss J, Rick J. “Best fit” framework synthesis: refining the method. BMC Med Res Methodol. 2013;13(1):37.
Carroll C, Booth A, Cooper K. A worked example of “best fit” framework synthesis: a systematic review of views concerning the taking of some potential chemopreventive agents. BMC Med Res Methodol. 2011;11(1):29.
Cohen MZ, Kahn DL, Steeves DL. Hermeneutic phenomenological research: a practical guide for nurse researchers. Thousand Oaks: Sage; 2000.
Noblit GW, Hare RD. Meta-ethnography: synthesizing qualitative studies. Newbury Park: Sage; 1988.
Book Google Scholar
Melendez-Torres GJ, Grant S, Bonell C. A systematic review and critical appraisal of qualitative metasynthetic practice in public health to develop a taxonomy of operations of reciprocal translation. Res Synthesis Methods. 2015;6(4):357–71.
Article CAS Google Scholar
Glaser BG, Strauss A. The discovery of grounded theory. Chicago: Aldine; 1967.
Dixon-Woods M, Agarwal S, Young B, Jones D, Sutton A. Integrative approaches to qualitative and quantitative evidence. In: UK National Health Service. 2004. p. 1–44.
Download references
Acknowledgements
Not applicable.
There was no funding for this work.
Availability of data and materials
The systematic methods overview used as a worked example in this article (Gentles SJ, Charles C, Ploeg J, McKibbon KA: Sampling in qualitative research: insights from an overview of the methods literature. The Qual Rep 2015, 20(11):1772-1789) is available from http://nsuworks.nova.edu/tqr/vol20/iss11/5 .
Authors’ contributions
SJG wrote the first draft of this article, with CC contributing to drafting. All authors contributed to revising the manuscript. All authors except CC (deceased) approved the final draft. SJG, CC, KAB, and JP were involved in developing methods for the systematic methods overview on sampling.
Authors’ information
Competing interests.
The authors declare that they have no competing interests.
Consent for publication
Ethics approval and consent to participate, author information, authors and affiliations.
Department of Clinical Epidemiology and Biostatistics, McMaster University, Hamilton, Ontario, Canada
Stephen J. Gentles, Cathy Charles & K. Ann McKibbon
Faculty of Social Work, University of Calgary, Alberta, Canada
David B. Nicholas
School of Nursing, McMaster University, Hamilton, Ontario, Canada
Jenny Ploeg
CanChild Centre for Childhood Disability Research, McMaster University, 1400 Main Street West, IAHS 408, Hamilton, ON, L8S 1C7, Canada
Stephen J. Gentles
You can also search for this author in PubMed Google Scholar
Corresponding author
Correspondence to Stephen J. Gentles .
Additional information
Cathy Charles is deceased
Additional file
Additional file 1:.
Submitted: Analysis_matrices. (DOC 330 kb)
Rights and permissions
Open Access This article is distributed under the terms of the Creative Commons Attribution 4.0 International License ( http://creativecommons.org/licenses/by/4.0/ ), which permits unrestricted use, distribution, and reproduction in any medium, provided you give appropriate credit to the original author(s) and the source, provide a link to the Creative Commons license, and indicate if changes were made. The Creative Commons Public Domain Dedication waiver ( http://creativecommons.org/publicdomain/zero/1.0/ ) applies to the data made available in this article, unless otherwise stated.
Reprints and permissions
About this article
Cite this article.
Gentles, S.J., Charles, C., Nicholas, D.B. et al. Reviewing the research methods literature: principles and strategies illustrated by a systematic overview of sampling in qualitative research. Syst Rev 5 , 172 (2016). https://doi.org/10.1186/s13643-016-0343-0
Download citation
Received : 06 June 2016
Accepted : 14 September 2016
Published : 11 October 2016
DOI : https://doi.org/10.1186/s13643-016-0343-0
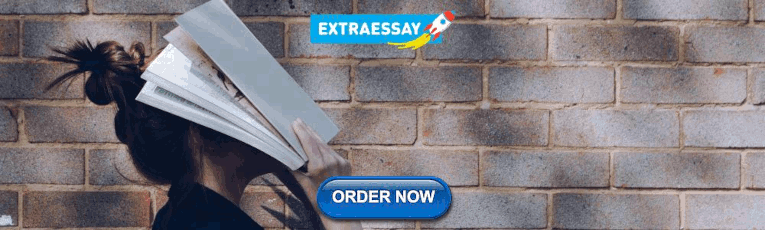
Share this article
Anyone you share the following link with will be able to read this content:
Sorry, a shareable link is not currently available for this article.
Provided by the Springer Nature SharedIt content-sharing initiative
- Systematic review
- Literature selection
- Research methods
- Research methodology
- Overview of methods
- Systematic methods overview
- Review methods
Systematic Reviews
ISSN: 2046-4053
- Submission enquiries: Access here and click Contact Us
- General enquiries: [email protected]
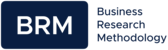
Systematic Sampling
In systematic sampling (also called systematic random sampling) every Nth member of population is selected to be included in the study. It is a probability sampling method. Systematic sampling can be more suitable than simple random sampling because the former can be time-consuming.
It has been stated that “with systematic sampling, every Kth item is selected to produce a sample of size n from a population size of N” [1] . This method requires an approximated frame for a priori but not the full list.
Systematic sampling
As it is the case with any other sampling method, you will have to obtain confirmation from your dissertation supervisor about your choice of systematic sampling, total size of population, size of your sample group and the value of N sample fraction before starting collecting the primary data.
There are three types of systematic random sampling:
1. Systematic random sampling . In this method researcher determines sampling interval and starting from the random point uses this interval to select sample group members.
2. Linear systematic sampling . This method divides N population size into n groups, where each group consists of k Then, sampling interval k=N/n applied to form the sample group.
3. Circular systematic sampling . Population members are arranged in a circular manner and sample group members are chosen in a cyclic manner.
Application of Systematic Sampling: an Example
You can apply systematic sampling in your thesis in the following manner:
1. Label each member of the sample group with a unique identification number (ID) . Accordingly, application of this method requires the knowledge of the size of total target population. You cannot use systematic sampling if you don’t know the size of the total population.
2. Calculate the sampling fraction by dividing the sample size to the total number of the population :

The sampling fraction (also called sampling interval) result is guidance for applying systematic sampling. Sampling fraction is a constant interval used to choose sample group members. For example, if your sampling fraction is equal to 1/5, you will need to choose one in every five cases; that is every fifth case from the sampling frame. In instances where calculations result in a more complicated fraction, especially for large sample sizes, you can round your population to the nearest 10 or 100.
3. The first sample has to be chosen in a random manner . It is important to select the first sample randomly to ensure probability sampling aspect of systematic sampling. In other words, if the first sample is selected from the start of the sample frame all the time, the samples between the sample fractions (samples between every fifth cases in example above) will not have a chance of being included in the sample group. Therefore, the fist case needs to be selected randomly to overcome this issue.
4. Additional members of sample group are chosen by recruiting each Nth subject (5 th subject in example above) among the population.
Let’s illustrate the application of stages above using a specific example.
Suppose your dissertation topic is “A Study into the Impact Leadership Style on Employee Motivation in ABC Company” and you have chosen semi-structured in-depth interview as primary data collection method. ABC Company has 200 operational level employees who could be potentially interviewed. You identified your sample size as 24 subjects, i.e. you will interview 24 employees.
You will have to do the following:
a) Label each employee with a unique number .
b) Calculate the sampling fraction.
Sampling fraction = (Actual Sample Size) / (Total Population) = 24/200 = 3/25.
This sampling fraction can be narrowed down to 1/8. Accordingly, every 8 th member of the sampling frame needs to be selected to participate in the study.
c) Choose the first sample randomly . Suppose you randomly selected the sample #47 as the starting point for selecting samples. Accordingly, your sample group will comprise of ABC Company employees under the following numbers: #47; #55; #63; #71; #79; #87; #95; #103; #111; #119; #127; #135; #143; #151; #159; #167; #175; #183; #191; #199; #7; #15; #23; #31.
Advantages of Systematic Sampling
- When done correctly, this method will approximate the results of simple random sampling.
- Systematic sampling is cost and time efficient. The selection of a sample is very convenient. This is an important aspect of systematic sampling which makes it applicable in many situations.
- Systematic sampling is effectively suitable in collecting data from geographically disperse cases (that do not require face-to-face contact).
- Systematic sampling reduces the probability of contaminating the data
- This method eliminates the phenomenon of clustered selection
Disadvantages of Systematic Sampling
- Systematic sampling can be applied only if the complete list of population is available.
- If there are periodic patterns within the dataset, the sample will be biased.
- If study participants deduce the sampling interval, this can bias the population as non-participants will be different from study participants.
- The risk of over-representation or under-representation of particular patterns
- Greater risk of manipulations with data.
My e-book, The Ultimate Guide to Writing a Dissertation in Business Studies: a step by step approach contains a detailed, yet simple explanation of sampling methods. The e-book explains all stages of the research process starting from the selection of the research area to writing personal reflection. Important elements of dissertations such as research philosophy, research approach, research design, methods of data collection and data analysis are explained in this e-book in simple words.
John Dudovskiy
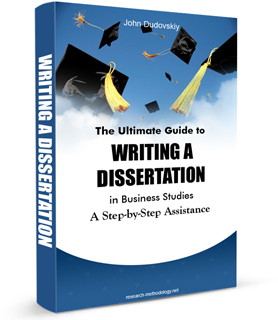
[1] Bajpai, N. (2010) “Business Statistics” Pearson Education India
Have a language expert improve your writing
Run a free plagiarism check in 10 minutes, automatically generate references for free.
- Knowledge Base
- Methodology
Systematic Sampling | A Step-by-Step Guide with Examples
Published on 3 May 2022 by Lauren Thomas . Revised on 18 December 2023.
Systematic sampling is a probability sampling method in which researchers select members of the population at a regular interval (or k ) determined in advance.
If the population order is random or random-like (e.g., alphabetical), then this method will give you a representative sample that can be used to draw conclusions about the population.
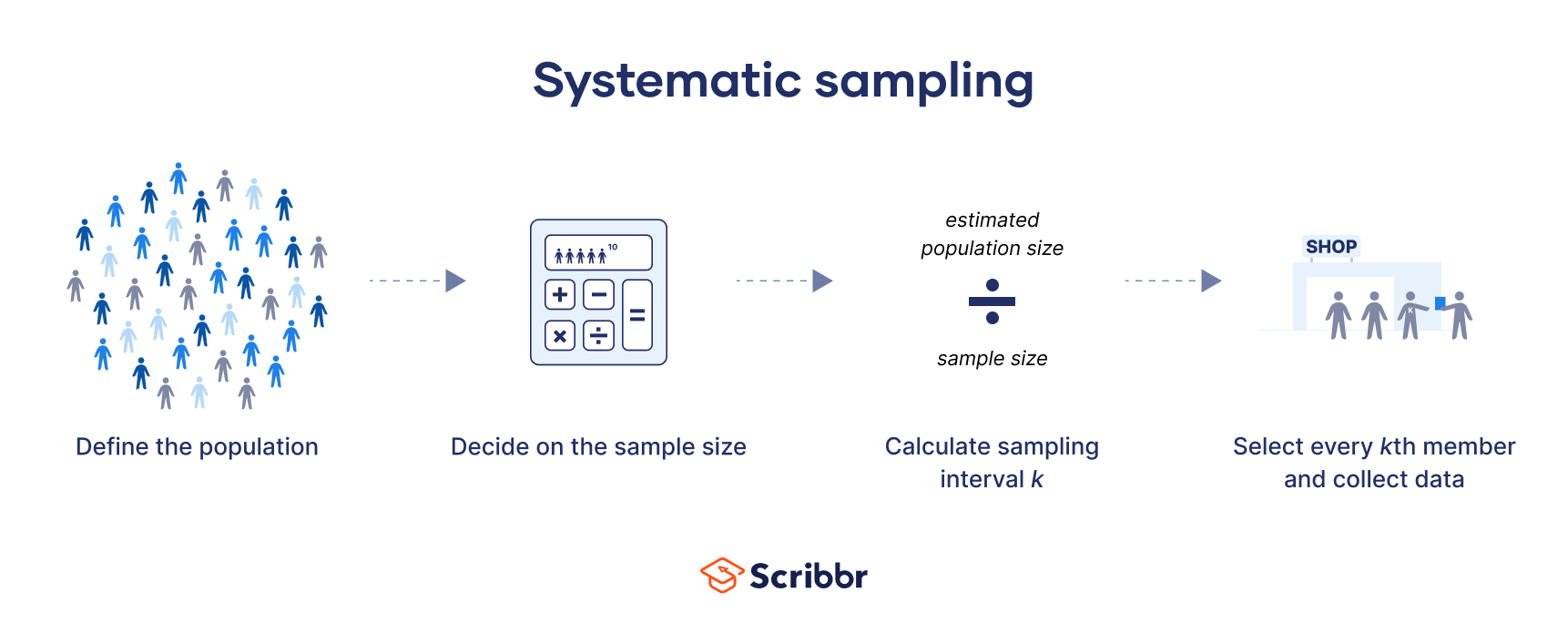
Table of contents
When to use systematic sampling, step 1: define your population, step 2: decide on your sample size, step 3: calculate sampling interval k, step 4: select the sample and collect data, frequently asked questions about systematic sampling.
Systematic sampling is a method that imitates many of the randomisation benefits of simple random sampling but is slightly easier to conduct.
You can use systematic sampling with a list of the entire population, as in simple random sampling . However, unlike with simple random sampling, you can also use this method when you’re unable to access a list of your population in advance.
Order of the population
When using systematic sampling with a population list, it’s essential to consider the order in which your population is listed to ensure that your sample is valid.
If your population is in ascending or descending order, using systematic sampling should still give you a fairly representative sample, as it will include participants from both the bottom and top ends of the population.
For example, if you are sampling from a list of individuals ordered by age, systematic sampling will result in a population drawn from the entire age spectrum. If you instead used simple random sampling, it is possible (although unlikely) that you would end up with only younger or older individuals.
You should not use systematic sampling if your population is ordered cyclically or periodically, as your resulting sample cannot be guaranteed to be representative.
Systematic sampling without a population list
You can use systematic sampling to imitate the randomisation of simple random sampling when you don’t have access to a full list of the population in advance.
Prevent plagiarism, run a free check.
Like other methods of sampling, you must decide upon the population that you are studying.
In systematic sampling, you have two choices for data collection:
- You can select your sample ahead of time from a list and then approach the selected subjects to collect data, or
- You can approach every k th member of your target population to ask them to participate in your study
Listing the population in advance
Ensure that your list contains the entire population and is not in a periodic or cyclic order. Ideally, it should be in a random or random-like (such as alphabetical) order, which will allow you to imitate the randomisation benefits of simple random sampling .
Selecting your sample on the spot
If you cannot access a list in advance, but you are able to physically observe the population, you can also use systematic sampling to select subjects at the moment of data collection.
In this case, ensure that the timing and location of your sampling procedure covers the full population to avoid bias in the results.
Before you choose your interval, you must first decide on your sample size. There are several different ways to choose a sample size, but one of the most common involves using a sample size calculator .
Once you have chosen your desired margin of error and confidence level , estimated total size of the population, and the standard deviation of the variables you are attempting to measure, this calculator will provide you with the sample size you should aim for.
When you know your target sample size, you can calculate your interval, k , by dividing your total estimated population size by your sample size. This can be a rough estimate rather than an exact calculation.
If you already have a list of your population, randomly select a starting point on your list, and from there, select every k th member of the population to include in your sample.
If you don’t have a list, you choose every k th member of the population for your sample at the same time as collecting the data for your study.
As in simple random sampling , you should try to make sure every individual you have chosen for your sample actually participates in your study. If those who decide to participate do so for reasons connected with the variables that you are collecting, this could bias your study.
Systematic sampling is a probability sampling method where researchers select members of the population at a regular interval – for example, by selecting every 15th person on a list of the population. If the population is in a random order, this can imitate the benefits of simple random sampling .
There are three key steps in systematic sampling :
- Define and list your population , ensuring that it is not ordered in a cyclical or periodic order.
- Decide on your sample size and calculate your interval, k , by dividing your population by your target sample size.
- Choose every k th member of the population as your sample.
Probability sampling means that every member of the target population has a known chance of being included in the sample.
Probability sampling methods include simple random sampling , systematic sampling , stratified sampling , and cluster sampling .
Cite this Scribbr article
If you want to cite this source, you can copy and paste the citation or click the ‘Cite this Scribbr article’ button to automatically add the citation to our free Reference Generator.
Thomas, L. (2023, December 18). Systematic Sampling | A Step-by-Step Guide with Examples. Scribbr. Retrieved 25 March 2024, from https://www.scribbr.co.uk/research-methods/systematic-sampling-method/
Is this article helpful?
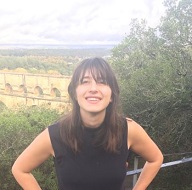
Lauren Thomas
Other students also liked, sampling methods | types, techniques, & examples, simple random sampling | definition, steps & examples, cluster sampling | a simple step-by-step guide with examples.
- Privacy Policy
Buy Me a Coffee

Home » Systematic Sampling – Types, Method and Examples
Systematic Sampling – Types, Method and Examples
Table of Contents

Systematic Sampling
Definition:
Systematic sampling is a probability sampling technique where a population is divided into equal-size intervals, and a starting point is randomly selected within the first interval. Then, every kth element in the population is selected for the sample, where k is the sampling interval calculated as the population size divided by the desired sample size.
Types of Systematic Sampling
Types of Systematic Sampling are as follows:
- Systematic random sampling: In this method, the first unit is selected at random from the population, and then subsequent units are selected at fixed intervals based on a predetermined pattern. For example, if we want to select a sample of 100 individuals from a population of 1000, we could randomly select one individual from the first 10, and then select every 10th individual after that.
- Linear systematic sampling : In this method, the population is arranged in a line or sequence, and units are selected at fixed intervals along that line. This is useful for populations that are arranged in a linear or sequential order, such as a list of customer names or a street of houses.
- Circular systematic sampling: In this method, the population is arranged in a circle or cycle, and units are selected at fixed intervals around the circle. This is useful for populations that have a circular or cyclical structure, such as days of the week or hours in a day.
- Double sampling: In this method, a small preliminary sample is taken from the population, and then a larger systematic sample is selected from the remaining population. This can be useful for reducing the cost of sampling or for ensuring that the sample is representative of specific subgroups within the population.
- Stratified systematic sampling : In this method, the population is first divided into strata or subgroups based on some characteristic (such as age or income), and then a systematic sample is selected from each stratum. This can help ensure that the sample is representative of the population as a whole, as well as of specific subgroups.
- Cluster systematic sampling: In this method, the population is divided into clusters or groups, and then a systematic sample of clusters is selected, with units within each selected cluster being sampled systematically. This can be useful when it is more practical to sample clusters than individuals (e.g., in large households or communities).
- Random-start systematic sampling: In this method, the starting point for selecting units is chosen randomly, rather than being based on a fixed pattern. This can help reduce bias in the sampling process and increase the representativeness of the sample.
- Multi-stage systematic sampling : In this method, a combination of different sampling methods (such as stratified and cluster sampling) is used to select units from the population. This can help ensure that the sample is representative of the population as a whole, while also allowing for efficient and cost-effective sampling.
Systematic Sampling Method
Here are the basic steps for conducting a Systematic Sample Method:
- Define the population: Determine the larger population you want to sample from.
- Determine the sample size: Decide on the number of units you want to include in your sample.
- Calculate the sampling interval: Divide the population size by the sample size to determine the sampling interval (k). For example, if you want to select a sample of 100 individuals from a population of 1000, the sampling interval would be 10 (i.e., 1000/100 = 10).
- Choose a random starting point: Choose a random number between 1 and k as your starting point.
- Select the sample: From your starting point, select every kth unit in the population until you have your desired sample size.
For example, let’s say you want to conduct a systematic sample of 100 households in a neighborhood of 1000 households. The sampling interval would be 10 (1000/100 = 10). You would choose a random number between 1 and 10 as your starting point (let’s say you choose 5). Then you would select every 10th household starting from the 5th household until you reach 100 households.
Systematic Sampling Formula
The systematic sampling formula can be used to determine the sample size and the interval between selected elements.
The formula for systematic sampling is:
n = N / (k)
- n is the sample size
- N is the population size
- k is the sampling interval
The sampling interval (k) is calculated by dividing the population size (N) by the desired sample size (n). For example, if we want to select a sample of 100 individuals from a population of 1000, the sampling interval would be calculated as follows:
k = N / n k = 1000 / 100 k = 10
In this example, we would select every 10th individual from the population to form the sample.
To use systematic sampling, we first select a random starting point from the population, which is any element between 1 and k. From that starting point, we select every kth element to form the sample. For example, if our starting point is 5, we would select elements 5, 15, 25, 35, and so on until we reach the desired sample size.
Examples of Systematic Sampling
Here are some examples of systematic sampling:
- Quality control : A production line manager wants to check the quality of products produced on a conveyor belt. The manager selects every tenth product that comes out of the belt to ensure that the quality meets the set standards.
- Opinion survey : A polling agency wants to conduct an opinion survey of the residents of a city. The agency selects every 100th person listed in the phonebook to participate in the survey.
- Medical research : A researcher wants to study the effects of a new medication on patients. The researcher selects every fifth patient that comes to a clinic to participate in the study.
- Education research: A researcher wants to study the performance of students in a school. The researcher selects every third student in a class to participate in the study.
- Census survey : A government agency wants to conduct a census survey of a population. The agency selects every 100th household in a region to participate in the survey.
Systematic Sampling Example Situation
Systematic Sampling Example Situation is as follows:
Let’s say we want to conduct a survey of 1000 households in a city to estimate the average monthly water usage. Instead of surveying all 1000 households, we can use systematic sampling to select a representative sample.
To use systematic sampling, we first need to determine the sampling interval (k). Let’s say we want to select a sample of 100 households. Using the formula for systematic sampling, we have:
k = N/n = 1000/100 = 10
This means that we need to select every 10th household from the population to form our sample. We can do this by choosing a random starting point between 1 and 10, and then selecting every 10th household thereafter.
For example, if we randomly select household number 3 as our starting point, we would select the following households for our sample: 3, 13, 23, 33, 43, 53, 63, 73, 83, 93, 103, 113, 123, and so on, until we have selected 100 households in total.
By using systematic sampling, we can obtain a representative sample of the population with less effort and cost compared to surveying all households.
Once we have selected our sample of 100 households, we can survey them to obtain information about their monthly water usage. We can then use the data from the sample to estimate the average monthly water usage of the entire population.
To calculate the estimate of the population average, we can use the formula:
Population average estimate = (sum of sample observations) / sample size
For example, if our sample of 100 households had a total monthly water usage of 10,000 gallons, then our estimate of the population average monthly water usage would be:
Population average estimate = 10,000 / 100 = 100 gallons per household per month
When to Use Systematic Sampling
Systematic Sampling is useful to use in the following situations:
- When The population is large: Systematic sampling is efficient and practical for large populations that are difficult or time-consuming to study in their entirety.
- When The sampling frame is well-ordered : Systematic sampling requires a well-ordered sampling frame where there is no systematic pattern to the arrangement of items.
- When Randomness is desired : Systematic sampling ensures some level of randomness through the use of a randomly selected starting point, which is useful when a simple random sample is not feasible.
- When Resources are limited: Systematic sampling requires fewer resources than other sampling methods, making it a practical option for studies with limited resources.
- When The research question is straightforward: Systematic sampling is useful when the research question is straightforward, and the sample needs to be representative of the population.
- When The sampling interval can be chosen appropriately: The sampling interval needs to be chosen appropriately to avoid bias in the sample. This is possible when the population is homogenous and there are no subgroups that need to be analyzed separately.
Applications of Systematic Sampling
Applications of Systematic Sampling are as follows:
- Agriculture: Systematic sampling is used in agriculture to study the distribution of pests or diseases in crops. A systematic sampling of crops is taken to count the number of diseased or infected plants.
- Market research: Systematic sampling is used in market research to survey customers about their preferences and opinions on products. For example, a company may select every 10th customer who walks into a store to participate in a survey.
- Environmental studies : Systematic sampling is used in environmental studies to measure the quality of air, water, or soil in a particular area. Samples are taken at regular intervals to create a representative sample of the entire area.
- Epidemiology: Systematic sampling is used in epidemiology to study the prevalence of a disease in a population. Samples are taken systematically to estimate the number of people affected by the disease.
- Education research: Systematic sampling is used in education research to study the performance of students. Samples are taken systematically to estimate the average performance of students in a particular subject.
- Quality control : Systematic sampling is used in quality control to check the quality of products during production. Samples are taken systematically at regular intervals to ensure that the quality of products meets the set standards.
Purpose of Systematic Sampling
The purpose of systematic sampling is to obtain a representative sample of a population by selecting every nth item from the population list. It is a convenient and straightforward method that can be used to save time and resources in large-scale studies. Systematic sampling has several purposes:
- Efficiency : Systematic sampling is an efficient method of sampling because it requires fewer resources and less time than other sampling techniques. This is because the sampling intervals are predetermined, and the selection of the sample items is systematic.
- Representation : Systematic sampling ensures that the sample is representative of the population because it covers the entire population list. This reduces the chance of bias in the sample selection process.
- Randomness : Systematic sampling introduces an element of randomness into the sample selection process because the starting point of the sample selection is randomly determined.
- Reliability : Systematic sampling is a reliable method of sampling because it is easy to replicate. This means that the same sample can be obtained from the same population list, and the results will be consistent.
- Cost-effective: Systematic sampling is a cost-effective method of sampling because it requires fewer resources and less time than other sampling techniques. This makes it ideal for large-scale studies or when resources are limited.
Characteristics of Systematic Sampling
The following are some of the characteristics of systematic sampling:
- Regular Sampling Intervals: Systematic sampling involves selecting every kth item from the population, where k is a fixed interval. For example, if the population size is 1000 and the sample size is 100, then the sampling interval is 10 (i.e., 1000/100).
- Random Starting Point : The first item in the sample is selected randomly from the first k items in the population. This helps to avoid any bias that may be introduced by a specific starting point.
- Easy to Implement : Systematic sampling is a straightforward method to implement and is less time-consuming than some other sampling methods.
- Representative Sample : When the sampling interval is chosen appropriately, systematic sampling can provide a representative sample of the population.
- Possibility of Bias : Systematic sampling can introduce bias if there is a pattern in the population that is related to the sampling interval. For example, if a population is sorted by some characteristic and the sampling interval is chosen to match this sorting, then the resulting sample may not be representative of the population.
- Sample Size : The sample size in systematic sampling is determined by dividing the population size by the sampling interval. Therefore, the sample size is limited by the population size and the chosen sampling interval.
Advantages of Systematic Sampling
There are several advantages of systematic sampling, including:
- Ease of Use : Systematic sampling is easy to understand and implement, and it requires fewer resources than other sampling methods.
- Representative Sample: When the sampling interval is chosen appropriately, systematic sampling can provide a representative sample of the population. This means that the sample accurately reflects the characteristics of the population.
- Efficient : Systematic sampling is more efficient than some other sampling methods because it requires less time and effort to select a sample.
- Reduction of Sampling Error : Systematic sampling can help to reduce sampling error, which is the difference between the characteristics of the sample and the population. This is because systematic sampling ensures that each element in the population has an equal chance of being selected for the sample.
- Suitable for Large Populations: Systematic sampling is particularly useful for large populations, where other sampling methods may be impractical or too time-consuming.
- Random Starting Point: The use of a random starting point in systematic sampling reduces the possibility of bias in the sample.
Disadvantages of Systematic Sampling
Disadvantages of Systematic Sampling are as follows:
- Possibility of Bias: Systematic sampling can introduce bias if there is a pattern in the population that is related to the sampling interval. For example, if a population is sorted by some characteristic and the sampling interval is chosen to match this sorting, then the resulting sample may not be representative of the population.
- Limited Sampling Frame: The sampling frame (the list of items in the population) needs to be ordered, and the order should not have any systematic pattern. If the population is not ordered or the order has a pattern, then the sampling frame may not be representative of the population.
- Lack of Flexibility: Systematic sampling requires that the sampling interval is fixed before the sample is selected. This means that if there is a change in the population characteristics, the sampling interval may not be appropriate, and the sample may no longer be representative of the population.
- Possible Overlap: Systematic sampling can result in samples that overlap if the sampling interval is not chosen appropriately. This means that some elements of the population may be selected more than once, while others may not be selected at all.
- Limited Randomness : While systematic sampling uses a random starting point, it does not provide the same level of randomness as other sampling methods such as simple random sampling.
About the author
Muhammad Hassan
Researcher, Academic Writer, Web developer
You may also like

Stratified Random Sampling – Definition, Method...

Purposive Sampling – Methods, Types and Examples

Non-probability Sampling – Types, Methods and...

Cluster Sampling – Types, Method and Examples

Snowball Sampling – Method, Types and Examples

Sampling Methods – Types, Techniques and Examples
Root out friction in every digital experience, super-charge conversion rates, and optimize digital self-service
Uncover insights from any interaction, deliver AI-powered agent coaching, and reduce cost to serve
Increase revenue and loyalty with real-time insights and recommendations delivered to teams on the ground
Know how your people feel and empower managers to improve employee engagement, productivity, and retention
Take action in the moments that matter most along the employee journey and drive bottom line growth
Whatever they’re are saying, wherever they’re saying it, know exactly what’s going on with your people
Get faster, richer insights with qual and quant tools that make powerful market research available to everyone
Run concept tests, pricing studies, prototyping + more with fast, powerful studies designed by UX research experts
Track your brand performance 24/7 and act quickly to respond to opportunities and challenges in your market
Explore the platform powering Experience Management
- Free Account
- For Digital
- For Customer Care
- For Human Resources
- For Researchers
- Financial Services
- All Industries
Popular Use Cases
- Customer Experience
- Employee Experience
- Employee Exit Interviews
- Net Promoter Score
- Voice of Customer
- Customer Success Hub
- Product Documentation
- Training & Certification
- XM Institute
- Popular Resources
- Customer Stories
- Market Research
- Artificial Intelligence
- Partnerships
- Marketplace
The annual gathering of the experience leaders at the world’s iconic brands building breakthrough business results, live in Salt Lake City.
- English/AU & NZ
- Español/Europa
- Español/América Latina
- Português Brasileiro
- REQUEST DEMO
- Experience Management
- Systematic Random Sampling
Try Qualtrics for free
The complete guide to systematic random sampling.
15 min read In this article, we’ll highlight what systematic random sampling is and how you can use it to create random sampling surveys to get a clear understanding of a target population.
If you want a highly effective (and accurate) method for selecting a random sample from a research population, systematic random sampling might be just what you’re looking for.
In this article, we’ll break down what systematic random sampling is and how you can use it to get a clear understanding of a target population.
Start your free 30-day trial of XM for Strategy & Research today
What is systematic random sampling?
Systematic random sampling is a probability sampling method. This means it uses chance and randomization to select sample data that represents a population.
After determining the right sample size , researchers assign a regular interval number they will use to select which members of the target population will be included in the sample.
The sample interval (k) is decided by dividing the population size (N) by the sample size (n).
If you had a list of 1,000 customers (your target population) and you wanted to survey 200 of them, your interval would be 5. This means that you would sample every 5th person in your list of 1,000 customers.
1,000 / 200 = 5
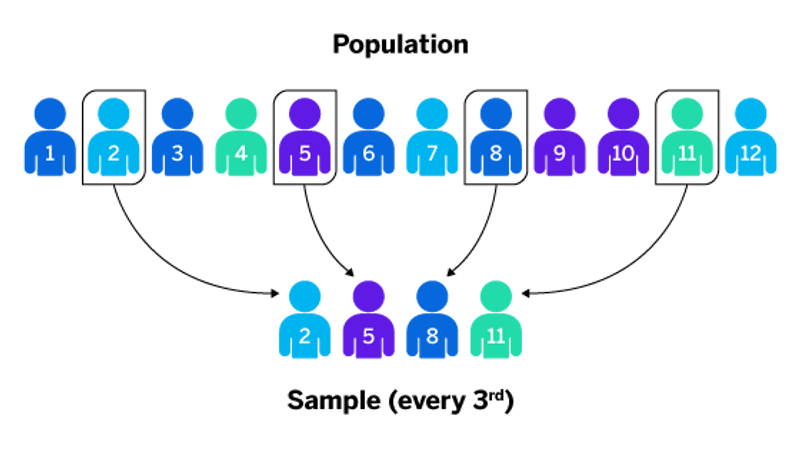
Types of systematic sampling
Although systematic sampling is a relatively simple concept, it can be performed in a few different ways.
Systematic random sampling
This is the ‘classic’ form of systematic sampling where items or individuals are selected at a predetermined interval.
Circular systematic sampling
With circular systematic sampling, the sampling process continues past the end of the population list, until the whole population has been sampled.
Linear systematic sampling
Linear systematic sampling doesn’t repeat, unlike circular systematic sampling. It produces a number using a form of skip logic to select the sample members.
When to use systematic sampling
Systematic sampling can be used whenever you want the benefits of randomly sampling the population you’re studying. It can be especially useful in situations where you don’t have details of the entire population before you begin your study. This is because systematic sampling is rule-based, so you can just apply the interval you’ve chosen to the data.
Systematic random sampling is valuable when you’re on a tight budget or a short timescale, but it may not be right for your project if there is any risk of data manipulation.
What is the risk of bias in systematic sampling?
Systematic sampling can remove some of the unpredictability from a sample, meaning that a researcher could potentially manipulate the results by choosing a starting point that favors their preferred outcome.
However, this risk is very slight because the only random component of the sample is the selection of the starting point. After that, the process moves in a set pattern until the sample is complete. For example, in a population of 21 and starting at 1, using an interval of 3 would result in the numbers 3, 6, 9, 12, 15, 18, and 21 being used.
It’s also important to ensure that your list is not ordered in any way that could introduce bias, e.g. if your list was ordered male, female, male, female, and you picked every other person, you would introduce a great degree of bias .
Benefits of systematic sampling
There are several benefits to using a systematic sample for research.
It’s simple to use
Because of the way systematic sampling is structured, surveys based on it are easy to create and the data is easy to analyze.
This type of sampling is particularly beneficial where the budget is limited as the sample selection process is straightforward.
It provides some control over the process
While systematic sampling retains an element of randomness, it also introduces some control and process into the selection process.
It’s low risk
Systematic sampling carries a low-risk factor because there’s a low chance that the data can be contaminated. This is because of the even distribution of members to form samples.
It’s resistant to bias
After the initial starting point, researchers have little control over who gets selected for systematic sampling. The selection process is truly random, creating a buffer against favoritism when it comes to data collection.
Limitations of systematic sampling
Although there are significant advantages to systematic sampling, it can carry some risks that you need to be aware of.
An assumption that the population size is known
Like all probability sampling techniques, systematic sampling requires that every sample member has a known, non-zero likelihood of being selected.
If the true population sizes are not known and cannot be determined, you can’t use the formula k = N/n to determine the sample interval or likelihood of being selected.
Another challenge is that it can be difficult to achieve the desired sample size if the true population size is not known in advance. If you need a sample of 200 people, you may not know whether to sample every 5th person or every 10th person.
Tip: you can still use systematic sampling if you don’t know the population size
There’s a way to use systematic sampling when the population size is not known in advance — if you are prepared to guess the interval. Imagine you want to conduct an exit poll after an important election. Although you do not know in advance how many people are going to show up at the election site, you can choose to sample every 5th person who leaves the voting location between when it opens and closes.
The true population size will then be approximately 5 times the size of your sample.
You need randomness in the population
If the population you’re assessing has a standard pattern (e.g., the list is ordered male, female, male, female), it can remove the randomness of the sampling interval and inadvertently lead to an unrepresentative sample such as all males. This isn’t common, but it’s worth being aware of before you plan your research project.
Let’s say you were surveying a high school class and wanted to select half of the students for an assessment. If the student list was in a boy, girl, boy, girl pattern and your sampling interval was an even number, you could end up with all boys or all girls in your sample.
To avoid this, make sure your data is sorted in such a way that won’t introduce any kind of repeating pattern — for example, you could ask the children to line up in order of height. That removes any repeating pattern (e.g., boy, girl, boy, girl) that might interfere with the systematic sample. If you had an electronic list of students in the class, you could sort the list in a random way such as alphabetically by student’s first name.
Higher potential for data manipulation
While systematic sampling is reliable and offers a natural degree of unpredictability and randomness, it’s also open to manipulation by individual researchers, albeit in a limited way.
The method the researcher chooses for their sample collection could potentially result in a higher chance of achieving a predetermined outcome, rather than using simple random selection.
How to perform systematic random sampling
Let’s run through the steps for systematic random sampling:
- Confirm the population total
It’s easier to achieve the desired sample size when you know the total population of the sample in advance. Once you have this number, the rest of the sampling process is simple.
- Determine your sample size
When you know the size of the sample you want to draw from the population, you can establish the interval needed to achieve the desired sample size from the population.
There are several factors to consider, including population size, the margin of error (confidence interval), and budget.
For example, you may want a sample size that yields a margin of error of +/- 5 points, or maybe it’s determined by your budget, e.g. you’re giving out $10 gift cards but can only afford 200.
- Determine your sampling interval
For this, you simply divide the total population by the sample size. If the total population size is not known in advance (for example, if you’re conducting an exit poll during an election), then estimate the population size based on historical data (e.g. the previous election) to determine your sampling interval.
- Select your random starting point
Starting your selection at a random number helps to retain the randomness of your selection and removes the risk of cluster or manipulation. The random start number will be between 1 and the sample interval.
E.g. to get a sample of 100 out of 1,000, you would select every 10th person.
- Add your sampling interval until you have the desired sample.
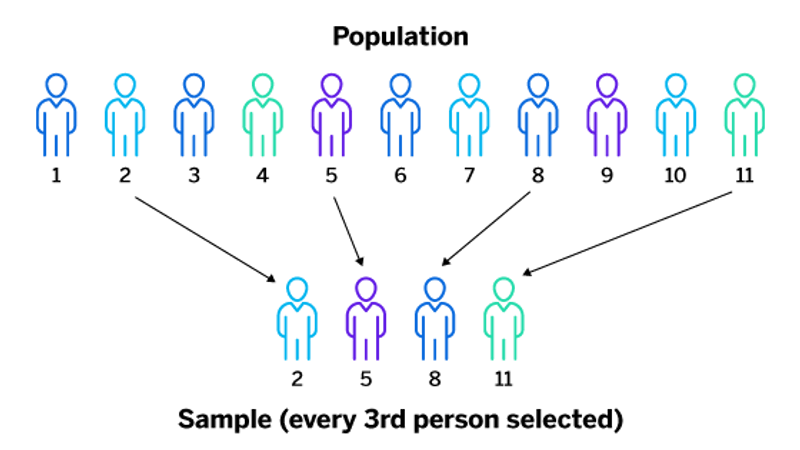
Systematic random sampling use cases and examples
- Systematic random samples can be used in social research — for example, to survey a large number of households by running a list of addresses through the interval selection process.
- It could be used for collecting customer experience feedback, for example by choosing at intervals from a list of people who attended a large conference.
- On a ‘passing traffic’ basis, systematic sampling could be used to survey visitors to a store or shopping mall, drivers using a toll booth, people waiting in line, or calls in a contact center.
How to use systematic sampling
Let’s say a sample size of 20 out of a population of 100 is required. For this study, each member of the population is assigned a number from 001 to 100.
We first calculate the interval by dividing the total number of people in the population (100) by the number we want in the sample (20). This gives us a sample interval of 5.
We then select a number between 1 and the sampling interval from a random number generator. Let’s say we get 4 — this is where we start. We count down the list (using linear systematic sampling or circular systematic sampling) from our starting point (person 4) and select each 5th person.
For example, if we start at 4, the next would be 9, 14, 19, 24, 29, and so on. When you reach the end of the list, you should have your desired number (20) for the sample size.
What are the alternatives to systematic sampling?
Simple random sampling.
A simple random sample is one where every member of the population of interest has an equal probability of being selected, and the selection is done in a completely arbitrary way. You can select randomly from a population using a tool such as a number table or a computer, or by using a draw or lottery system.
Stratified random sampling
Stratified random sampling involves dividing your population into groups (called strata) that are linked by a particular characteristic, such as income bracket or nationality. A random sample is taken from each group, and then these are pooled together to create a random sample of the entire population. The purpose is to make sure each group is represented in your sample.
Cluster sampling
With cluster sampling, groups rather than individual units of the target population are selected at random for the test. These might be pre-existing groups, such as people in certain zip codes or students belonging to an academic year. Cluster sampling can be done by selecting the entire cluster, or in the case of two-stage cluster sampling, by randomly selecting the cluster itself, then selecting at random again within the cluster.
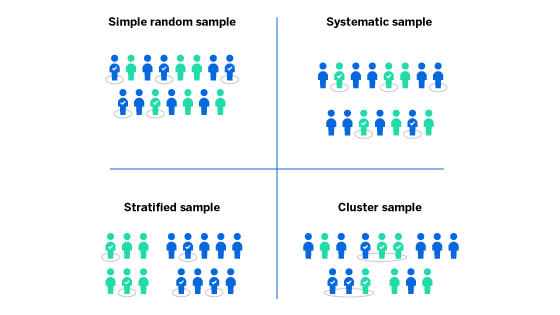
Should you use systematic sampling or simple random sampling?
What’s the difference between a systematic sample and a simple random sample?
In each sampling method, every person has an equal chance of being chosen. It’s also true that both methods could create some bias — e.g. a larger percentage of females in the sample than in the population.
The primary reasons to use a systematic sample in place of a random one are
(1) when the population size is unknown in advance (e.g. an exit poll during an election)
(2) if you don’t have an electronic list or you need to sample on the go. For example, you can use systematic sampling to select 1 out of every 5 people that enter a waiting room at a hospital, whereas with a random sample you would need the complete list of patients for the day, which could present problems if things change or last-minute emergencies turn up.
Want to learn more about sampling?
Choosing the right method for sampling your target audience can make all the difference when it comes to the reliability of your data and analysis.
In our guide to sampling methods and best practices, we explore other probability and non-probability sampling methods , including stratified sampling and cluster sampling, convenience sampling , quota sampling, and more — to give you the information you need to conduct comprehensive research.
If you want to learn more about sampling methods, you can read our ultimate guide to sampling methods and best practices .
How Qualtrics can help
We specialize in providing solutions that empower everyone to gather research insights and take action. With Qualtrics CoreXM , you can make sophisticated research simple.
Whether you’re in marketing, product development, or HR — with CoreXM, you can rapidly turn insights from surveys into actionable strategies.
Featuring advanced and incredibly flexible survey tools, built-in intelligence (driven by iQ™), a fully automated platform, and research services , CoreXM enables you to run the surveys you need to get answers to your most important marketing, branding, customer, and product questions.
Start your free 30-day trial of XM for Strategy & Research today
Related resources
Convenience sampling 18 min read, probability sampling 8 min read, non-probability sampling 17 min read, stratified random sampling 12 min read, simple random sampling 9 min read, sampling methods 15 min read, how to determine sample size 12 min read, request demo.
Ready to learn more about Qualtrics?
- Maths Notes Class 12
- NCERT Solutions Class 12
- RD Sharma Solutions Class 12
- Maths Formulas Class 12
- Maths Previous Year Paper Class 12
- Class 12 Syllabus
- Class 12 Revision Notes
- Physics Notes Class 12
- Chemistry Notes Class 12
- Biology Notes Class 12
- Random Sampling
- Line Integral
- Determinant of 2x2 Matrix
- Exponential Graph
- Domain and Range of Trigonometric Functions
- Derivative of Arctan
- Integration
- Derivative of Sec Square x
- Orthogonal Matrix
- Derivative of Sec x
- Local Maxima and Minima in Calculus
- Triple Integrals
- Algebra of Matrices
- Derivative Rules
- Trigonometry Formulas for Class 12
- Derivative of Tan Inverse x
- Integral of Cos x
- Derivative of Sin 2x
Systematic Random Sampling
Systematic Random Sampling is a method of selecting a sample from a population in a structured and organized manner. It is a valuable technique in research and statistical analysis providing a systematic yet random approach to sample selection ensuring reliable and accurate results. In this article, we will discuss systematic random sampling in detail along with some solved examples and others in detail.
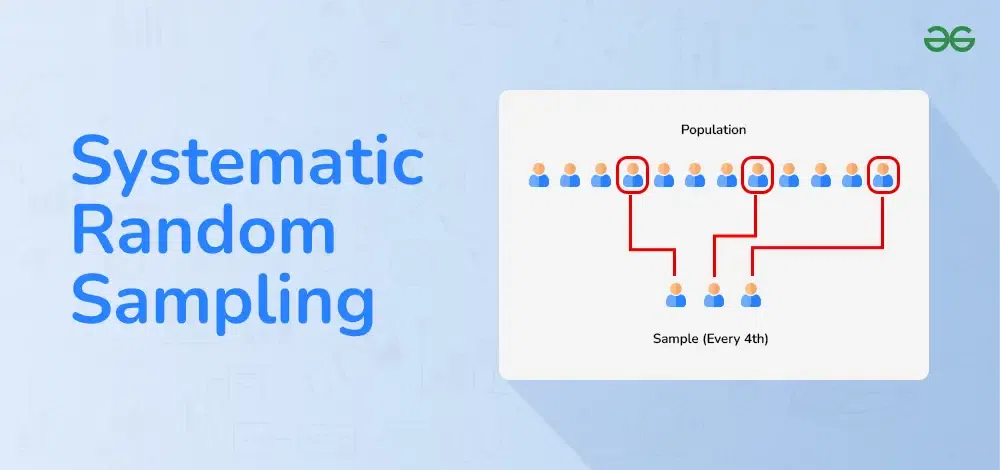
Table of Content
What is Systematic Sampling?
What is systematic random sampling, systematic random sampling examples, advantages and disadvantages of systematic random sampling, why to use systematic random sampling technique, systematic random sampling vs simple random sampling.
Systematic Sampling is a statistical technique where every nth member of a population is chosen for a sample after an initial random starting point.
Here’s how systematic sampling operates:
- Random Start: Initially, a starting point is randomly chosen from the first n elements of the population.
- Interval Selection: Every nth element from this randomly chosen starting point is included in the sample.
- Patterned Selection: This method establishes a structured pattern for selecting elements from the population.
Types of Systematic Sampling
Some of the types of Systematic Sampling are:
- Linear Systematic Sampling: In this method, individuals are chosen at regular intervals from a list. For instance, every 5th person is selected from a given population list after a random starting point.
- Circular Systematic Sampling: Particularly useful for circular or sequential data, this method involves selecting samples at regular intervals, looping back to the beginning when reaching the end of the sequence.
- Stratified Systematic Sampling: The population is divided into distinct strata or subgroups based on specific criteria. Then, systematic sampling is applied within each stratum independently to ensure representation from all groups.
- Cluster Systematic Sampling: Here, the population is divided into clusters, and a systematic sample of clusters is chosen. Then, within the selected clusters, all individuals are sampled systematically..
Systematic random sampling is a method that involves selecting elements from a population at regular intervals using a predetermined pattern. It’s a hybrid approach that combines randomness with a systematic method to ensure a representative sample.
Here’s a detailed breakdown of systematic random sampling:
- Random Start: Initially, a random starting point is chosen from the population. This starting point determines the first element to be included in the sample.
- Regular Intervals: After selecting the initial element randomly, subsequent elements are chosen at fixed intervals. For instance, if every 5th element is to be included in the sample, this pattern is followed consistently.
- Patterned Selection: The selection continues according to the specified interval until the desired sample size is achieved. This systematic approach ensures that each element in the population has an equal chance of being included in the sample.
Systematic random sampling helps avoid bias by ensuring randomness in the selection process while maintaining a systematic structure. This method is particularly useful when the population is large and ordered, providing a balanced representation of the entire population in the sample.
Systematic Random Sampling Definition
Systematic Random Sampling is a statistical technique used to select elements from a population at regular intervals through a systematic and structured approach.
Systematic Sampling involves choosing a starting point at random within the population and then selecting every nth element thereafter according to a predetermined pattern until the desired sample size is achieved.
This method combines systematicity with randomness to ensure that each member of the population has an equal probability of being included in the sample, making it a representative subset of the entire population
Check, Random Sampling
Example 1: Consider a university with 2000 students. To conduct a survey, a systematic random sample of 100 students is needed.
Random Start: A random number between 1 and 10 is chosen as the starting point. Sampling Interval: Every 20th student from the randomly selected starting point is chosen for the survey. If the random start is student number 5, the selected students for the sample will be 5, 25, 45, 65, …, until 100 students are sampled.
Example 2: Suppose a company has a workforce of 500 employees and needs to gather feedback. They aim to survey 50 employees using systematic random sampling.
Random Start: A random number between 1 and 10 is generated to select the starting point. Sampling Interval: Every 10th employee from the randomly chosen starting point is included in the survey sample. If the random start is employee number 3, the sample will include employees 3, 13, 23, 33, …, until 50 employees are surveyed.
Example 3: In a town with 1000 households, a government agency wants to survey 80 households for a census.
Random Start: A random number between 1 and 10 is used as the starting point. Sampling Interval: Every 12th household from the randomly chosen starting point is selected for the census. If the random start is household number 8, the selected households will be 8, 20, 32, 44, …, until 80 households are surveyed.
The advantages and disadvantages of Systematic Random Sampling is tabulated below:
Systematic random sampling is employed for several reasons due to its unique advantages in statistical analysis. Here are the key points explaining why this technique is used:
- Representative Sampling: Systematic random sampling ensures a representative subset of the population, reducing the risk of bias and providing reliable insights into the entire population.
- Efficiency: It offers greater efficiency compared to simple random sampling, especially in large populations, as it maintains randomness while structuring the selection process.
- Systematic Approach: It combines randomness with a systematic approach, making it easier to implement and understand compared to some other sampling methods.
- Reduced Bias: By eliminating personal bias in selecting samples, it provides an impartial method of obtaining a diverse representation from the population.
- Balanced Method: Offers a balanced compromise between systematic selection and randomization, ensuring fairness in the selection process.
- Minimizes Error: It reduces the chances of error due to subjective judgment in selecting samples, enhancing the reliability of the obtained results.
- Saves Time: It can be quicker than other methods, especially when a structured and systematic sampling technique is required for analysis.
The comparison between Systematic Random Sampling and Simple Random Sampling is tabulated below:
How is Sampling Interval Calculated in Systematic Random Sampling
To conduct systematic random sampling, determine the sampling interval (k), randomly select a starting point, and then choose every kth element thereafter until the desired sample size is achieved. The sampling interval (k) is determined by dividing the population size (N) by the desired sample size (n). Mathematically,
Systematic Sampling Method
To calculate systematic random sampling, follow these steps:
- Determine Population Size: Identify the total number of elements in the population you want to sample from.
- Decide Sample Size: Determine the size of the sample required for your study or survey.
- Calculate Sampling Interval: Divide the population size by the desired sample size. This gives the sampling interval (k).
- Sampling Interval (k) = Population Size / Sample Size
- Select a Random Start: Choose a random number between 1 and the sampling interval (k). This number determines the starting point.
- Systematic Selection: Begin from the randomly selected starting point and select every kth element until you reach the desired sample size.
- Ensure Randomness in Selection: It’s crucial to ensure the randomness of the starting point. We can use random number generators or tables to determine the starting point.
- Verify Coverage: Confirm that the systematic selection covers the entire population and that each element has an equal chance of being selected.
This method ensures that each element in the population has an equal chance of being included in the sample, promoting statistical validity.
Why is it Important to Randomly Select the Starting Point in Systematic Random Sampling
Randomly selecting the starting point ensures that the systematic sampling maintains its randomness and avoids introducing bias into the sample selection process.
Benefits of Systematic Random Sampling
The advantages of Systematic Random Sampling are mentioned below:
- Ease of Implementation: It’s relatively straightforward compared to other sampling methods, making it user-friendly.
- Representative Samples: When properly executed, this method can yield samples that accurately represent the larger population.
- Reduced Bias: By following a systematic pattern, it minimizes potential biases introduced by subjective selections.
- Efficiency: It can be more efficient, particularly when dealing with extensive populations, saving time and resources.
- Equal Probability: Every element in the population has an equal chance of selection, ensuring fairness and impartiality.
Where is Systematic Random Sampling Commonly Used
Systematic Random Sampling is commonly used in following situations
- Surveys and Market Research: When conducting surveys it’s often used to select participants systematically from a larger pool ensuring diverse representation.
- Quality Control: In manufacturing, it helps inspect a systematic subset of products to ensure consistent quality.
- Ecological Studies: Sampling trees in a forest or species in an ecosystem can employ this method to study biodiversity patterns.
- Education Research: To gather student feedback or test scores from a large educational institution, this method helps in systematic data collection.
- Clinical Trials: In medical research, it’s utilized to select patients systematically from a pool for drug testing or treatment studies.
Also, Check
- Probability Theory
- Introduction to Statistics
Systematic Random Sampling – Solved Examples
Example 1: Given Population size (N): 100, Desired sample size (n): 10. Calculate Systematic Random Sampling.
Sampling interval (k) = N/n = 100/10 = 10 Randomly select a starting point: 7 Selected Sample: 7, 17, 27, 37, 47, 57, 67, 77, 87, 97
Example 2: Given Population size (N): 150, Desired sample size (n): 15. Calculate Systematic Random Sampling.
Sampling interval (k) = N/n = 150/15 = 10 Randomly select a starting point: 4 Selected Sample: 4, 14, 24, 34, 44, 54, 64, 74, 84, 94, 104, 114, 124, 134, 144
Example 3: Given Population size (N): 90, Desired sample size (n): 9. Calculate Systematic Random Sampling.
Sampling interval (k) = N/n = 90/9 = 10 Randomly select a starting point: 6 Selected Sample: 6, 16, 26, 36, 46, 56, 66, 76, 86
Example 4: Given Population size (N): 160, Desired sample size (n): 16. Calculate Systematic Random Sampling.
Sampling interval (k) = N/n = 160/16 = 10 Randomly select a starting point: 3 Selected Sample: 3, 13, 23, 33, 43, 53, 63, 73, 83, 93, 103, 113, 123, 133, 143, 153
Example 5: Given Population size (N): 80, Desired sample size (n): 8. Calculate Systematic Random Sampling.
Sampling interval (k) = N/n = 80/8 = 10 Randomly select a starting point: 2 Selected Sample: 2, 12, 22, 32, 42, 52, 62, 72
Systematic Random Sampling Practice Problems
Problem 1: Given Population size (N): 120, Desired sample size (n): 10. Calculate Systematic Random Sampling.
Problem 2: Given Population size (N): 80, Desired sample size (n): 4. Calculate Systematic Random Sampling.
Problem 3: Given Population size (N): 60, Desired sample size (n): 8. Calculate Systematic Random Sampling.
Problem 4: Given Population size (N): 40, Desired sample size (n): 6. Calculate Systematic Random Sampling.
Problem 5: Given Population size (N): 20, Desired sample size (n): 4. Calculate Systematic Random Sampling.
Systematic Random Sampling: FAQs
Is systemic random sampling qualitative or quantitative.
Systematic Random Sampling is a Quantitative Sampling Technique
What Challenges Associated with Systematic Random Sampling?
One challenge is that if there is an unseen periodicity or pattern in the population, it may coincide with the sampling interval, potentially leading to biased results. Additionally, it may be impractical if the population is not easily ordered.
Can Systematic Random Sampling be used with Large Populations?
Yes, systematic random sampling is suitable for large populations. Its efficiency makes it feasible for selecting representative samples even when dealing with extensive datasets.
How does Systematic Random Sampling compare to other Sampling Methods?
Systematic random sampling strikes a balance between simplicity and representativeness. It may be more efficient than simple random sampling and less prone to bias than some non-random methods.
What Steps can be Taken to Enhance the Reliability of Systematic Random Sampling Results?
Ensuring a truly random starting point, using a suitable sampling interval, and being mindful of potential biases or patterns in the population are key steps to enhance the reliability of systematic random sampling results.
Is there a Risk of Introducing Bias in Systematic Random Sampling?
While systematic random sampling aims to be unbiased, there’s a risk if there is an unnoticed pattern in the population that aligns with the sampling interval potentially leading to biased results.
Can Systematic Random Sampling be Applied to Qualitative Research?
Yes, systematic random sampling can be adapted for qualitative research such as selecting interviews or cases systematically from a larger pool providing a structured yet unbiased approach.
How is Systematic Sampling different from Random Sampling?
Systematic sampling involves selecting every nth element from a population after an initial random start, whereas random sampling involves selecting individuals randomly from a population without any order or sequence.
What is the Formula for Random Sampling?
The formula for random sampling is often expressed as P(E)= n/N, where P(E) is the probability of selecting a specific individual, n is the number of individuals to select and N is the total population size.
What is Stratified Random Sampling?
Stratified random sampling involves dividing a population into subgroups (strata) based on specific characteristics and then randomly selecting individuals from each subgroup, ensuring representation from each subgroup in the sample for a more comprehensive analysis.
What is Solvin Formula?
“Solvin Formula” is not a familiar term in the context of sampling or statistics. If it refers to a specific formula or concept, providing more context would help clarify its meaning.
Please Login to comment...
- Geeks Premier League 2023
- Math-Statistics
- Maths-Class-12
- Geeks Premier League
- Mathematics
- School Learning
- WhatsApp To Launch New App Lock Feature
- Top Design Resources for Icons
- Node.js 21 is here: What’s new
- Zoom: World’s Most Innovative Companies of 2024
- 30 OOPs Interview Questions and Answers (2024)
Improve your Coding Skills with Practice
What kind of Experience do you want to share?

An official website of the United States government
The .gov means it’s official. Federal government websites often end in .gov or .mil. Before sharing sensitive information, make sure you’re on a federal government site.
The site is secure. The https:// ensures that you are connecting to the official website and that any information you provide is encrypted and transmitted securely.
- Publications
- Account settings
Preview improvements coming to the PMC website in October 2024. Learn More or Try it out now .
- Advanced Search
- Journal List
- HHS Author Manuscripts

Purposeful sampling for qualitative data collection and analysis in mixed method implementation research
Lawrence a. palinkas.
1 School of Social Work, University of Southern California, Los Angeles, CA 90089-0411
Sarah M. Horwitz
2 Department of Child and Adolescent Psychiatry, New York University, New York, NY
Carla A. Green
3 Center for Health Research, Kaiser Permanente Northwest, Portland, OR
Jennifer P. Wisdom
4 George Washington University, Washington DC
Naihua Duan
5 New York State Neuropsychiatric Institute and Department of Psychiatry, Columbia University, New York, NY
Kimberly Hoagwood
Purposeful sampling is widely used in qualitative research for the identification and selection of information-rich cases related to the phenomenon of interest. Although there are several different purposeful sampling strategies, criterion sampling appears to be used most commonly in implementation research. However, combining sampling strategies may be more appropriate to the aims of implementation research and more consistent with recent developments in quantitative methods. This paper reviews the principles and practice of purposeful sampling in implementation research, summarizes types and categories of purposeful sampling strategies and provides a set of recommendations for use of single strategy or multistage strategy designs, particularly for state implementation research.
Recently there have been several calls for the use of mixed method designs in implementation research ( Proctor et al., 2009 ; Landsverk et al., 2012 ; Palinkas et al. 2011 ; Aarons et al., 2012). This has been precipitated by the realization that the challenges of implementing evidence-based and other innovative practices, treatments, interventions and programs are sufficiently complex that a single methodological approach is often inadequate. This is particularly true of efforts to implement evidence-based practices (EBPs) in statewide systems where relationships among key stakeholders extend both vertically (from state to local organizations) and horizontally (between organizations located in different parts of a state). As in other areas of research, mixed method designs are viewed as preferable in implementation research because they provide a better understanding of research issues than either qualitative or quantitative approaches alone ( Palinkas et al., 2011 ). In such designs, qualitative methods are used to explore and obtain depth of understanding as to the reasons for success or failure to implement evidence-based practice or to identify strategies for facilitating implementation while quantitative methods are used to test and confirm hypotheses based on an existing conceptual model and obtain breadth of understanding of predictors of successful implementation ( Teddlie & Tashakkori, 2003 ).
Sampling strategies for quantitative methods used in mixed methods designs in implementation research are generally well-established and based on probability theory. In contrast, sampling strategies for qualitative methods in implementation studies are less explicit and often less evident. Although the samples for qualitative inquiry are generally assumed to be selected purposefully to yield cases that are “information rich” (Patton, 2001), there are no clear guidelines for conducting purposeful sampling in mixed methods implementation studies, particularly when studies have more than one specific objective. Moreover, it is not entirely clear what forms of purposeful sampling are most appropriate for the challenges of using both quantitative and qualitative methods in the mixed methods designs used in implementation research. Such a consideration requires a determination of the objectives of each methodology and the potential impact of selecting one strategy to achieve one objective on the selection of other strategies to achieve additional objectives.
In this paper, we present different approaches to the use of purposeful sampling strategies in implementation research. We begin with a review of the principles and practice of purposeful sampling in implementation research, a summary of the types and categories of purposeful sampling strategies, and a set of recommendations for matching the appropriate single strategy or multistage strategy to study aims and quantitative method designs.
Principles of Purposeful Sampling
Purposeful sampling is a technique widely used in qualitative research for the identification and selection of information-rich cases for the most effective use of limited resources ( Patton, 2002 ). This involves identifying and selecting individuals or groups of individuals that are especially knowledgeable about or experienced with a phenomenon of interest ( Cresswell & Plano Clark, 2011 ). In addition to knowledge and experience, Bernard (2002) and Spradley (1979) note the importance of availability and willingness to participate, and the ability to communicate experiences and opinions in an articulate, expressive, and reflective manner. In contrast, probabilistic or random sampling is used to ensure the generalizability of findings by minimizing the potential for bias in selection and to control for the potential influence of known and unknown confounders.
As Morse and Niehaus (2009) observe, whether the methodology employed is quantitative or qualitative, sampling methods are intended to maximize efficiency and validity. Nevertheless, sampling must be consistent with the aims and assumptions inherent in the use of either method. Qualitative methods are, for the most part, intended to achieve depth of understanding while quantitative methods are intended to achieve breadth of understanding ( Patton, 2002 ). Qualitative methods place primary emphasis on saturation (i.e., obtaining a comprehensive understanding by continuing to sample until no new substantive information is acquired) ( Miles & Huberman, 1994 ). Quantitative methods place primary emphasis on generalizability (i.e., ensuring that the knowledge gained is representative of the population from which the sample was drawn). Each methodology, in turn, has different expectations and standards for determining the number of participants required to achieve its aims. Quantitative methods rely on established formulae for avoiding Type I and Type II errors, while qualitative methods often rely on precedents for determining number of participants based on type of analysis proposed (e.g., 3-6 participants interviewed multiple times in a phenomenological study versus 20-30 participants interviewed once or twice in a grounded theory study), level of detail required, and emphasis of homogeneity (requiring smaller samples) versus heterogeneity (requiring larger samples) ( Guest, Bunce & Johnson., 2006 ; Morse & Niehaus, 2009 ; Padgett, 2008 ).
Types of purposeful sampling designs
There exist numerous purposeful sampling designs. Examples include the selection of extreme or deviant (outlier) cases for the purpose of learning from an unusual manifestations of phenomena of interest; the selection of cases with maximum variation for the purpose of documenting unique or diverse variations that have emerged in adapting to different conditions, and to identify important common patterns that cut across variations; and the selection of homogeneous cases for the purpose of reducing variation, simplifying analysis, and facilitating group interviewing. A list of some of these strategies and examples of their use in implementation research is provided in Table 1 .
Purposeful sampling strategies in implementation research
Embedded in each strategy is the ability to compare and contrast, to identify similarities and differences in the phenomenon of interest. Nevertheless, some of these strategies (e.g., maximum variation sampling, extreme case sampling, intensity sampling, and purposeful random sampling) are used to identify and expand the range of variation or differences, similar to the use of quantitative measures to describe the variability or dispersion of values for a particular variable or variables, while other strategies (e.g., homogeneous sampling, typical case sampling, criterion sampling, and snowball sampling) are used to narrow the range of variation and focus on similarities. The latter are similar to the use of quantitative central tendency measures (e.g., mean, median, and mode). Moreover, certain strategies, like stratified purposeful sampling or opportunistic or emergent sampling, are designed to achieve both goals. As Patton (2002 , p. 240) explains, “the purpose of a stratified purposeful sample is to capture major variations rather than to identify a common core, although the latter may also emerge in the analysis. Each of the strata would constitute a fairly homogeneous sample.”
Challenges to use of purposeful sampling
Despite its wide use, there are numerous challenges in identifying and applying the appropriate purposeful sampling strategy in any study. For instance, the range of variation in a sample from which purposive sample is to be taken is often not really known at the outset of a study. To set as the goal the sampling of information-rich informants that cover the range of variation assumes one knows that range of variation. Consequently, an iterative approach of sampling and re-sampling to draw an appropriate sample is usually recommended to make certain the theoretical saturation occurs ( Miles & Huberman, 1994 ). However, that saturation may be determined a-priori on the basis of an existing theory or conceptual framework, or it may emerge from the data themselves, as in a grounded theory approach ( Glaser & Strauss, 1967 ). Second, there are a not insignificant number in the qualitative methods field who resist or refuse systematic sampling of any kind and reject the limiting nature of such realist, systematic, or positivist approaches. This includes critics of interventions and “bottom up” case studies and critiques. However, even those who equate purposeful sampling with systematic sampling must offer a rationale for selecting study participants that is linked with the aims of the investigation (i.e., why recruit these individuals for this particular study? What qualifies them to address the aims of the study?). While systematic sampling may be associated with a post-positivist tradition of qualitative data collection and analysis, such sampling is not inherently limited to such analyses and the need for such sampling is not inherently limited to post-positivist qualitative approaches ( Patton, 2002 ).
Purposeful Sampling in Implementation Research
Characteristics of implementation research.
In implementation research, quantitative and qualitative methods often play important roles, either simultaneously or sequentially, for the purpose of answering the same question through convergence of results from different sources, answering related questions in a complementary fashion, using one set of methods to expand or explain the results obtained from use of the other set of methods, using one set of methods to develop questionnaires or conceptual models that inform the use of the other set, and using one set of methods to identify the sample for analysis using the other set of methods ( Palinkas et al., 2011 ). A review of mixed method designs in implementation research conducted by Palinkas and colleagues (2011) revealed seven different sequential and simultaneous structural arrangements, five different functions of mixed methods, and three different ways of linking quantitative and qualitative data together. However, this review did not consider the sampling strategies involved in the types of quantitative and qualitative methods common to implementation research, nor did it consider the consequences of the sampling strategy selected for one method or set of methods on the choice of sampling strategy for the other method or set of methods. For instance, one of the most significant challenges to sampling in sequential mixed method designs lies in the limitations the initial method may place on sampling for the subsequent method. As Morse and Neihaus (2009) observe, when the initial method is qualitative, the sample selected may be too small and lack randomization necessary to fulfill the assumptions for a subsequent quantitative analysis. On the other hand, when the initial method is quantitative, the sample selected may be too large for each individual to be included in qualitative inquiry and lack purposeful selection to reduce the sample size to one more appropriate for qualitative research. The fact that potential participants were recruited and selected at random does not necessarily make them information rich.
A re-examination of the 22 studies and an additional 6 studies published since 2009 revealed that only 5 studies ( Aarons & Palinkas, 2007 ; Bachman et al., 2009 ; Palinkas et al., 2011 ; Palinkas et al., 2012 ; Slade et al., 2003) made a specific reference to purposeful sampling. An additional three studies ( Henke et al., 2008 ; Proctor et al., 2007 ; Swain et al., 2010 ) did not make explicit reference to purposeful sampling but did provide a rationale for sample selection. The remaining 20 studies provided no description of the sampling strategy used to identify participants for qualitative data collection and analysis; however, a rationale could be inferred based on a description of who were recruited and selected for participation. Of the 28 studies, 3 used more than one sampling strategy. Twenty-one of the 28 studies (75%) used some form of criterion sampling. In most instances, the criterion used is related to the individual’s role, either in the research project (i.e., trainer, team leader), or the agency (program director, clinical supervisor, clinician); in other words, criterion of inclusion in a certain category (criterion-i), in contrast to cases that are external to a specific criterion (criterion-e). For instance, in a series of studies based on the National Implementing Evidence-Based Practices Project, participants included semi-structured interviews with consultant trainers and program leaders at each study site ( Brunette et al., 2008 ; Marshall et al., 2008 ; Marty et al., 2007; Rapp et al., 2010 ; Woltmann et al., 2008 ). Six studies used some form of maximum variation sampling to ensure representativeness and diversity of organizations and individual practitioners. Two studies used intensity sampling to make contrasts. Aarons and Palinkas (2007) , for example, purposefully selected 15 child welfare case managers representing those having the most positive and those having the most negative views of SafeCare, an evidence-based prevention intervention, based on results of a web-based quantitative survey asking about the perceived value and usefulness of SafeCare. Kramer and Burns (2008) recruited and interviewed clinicians providing usual care and clinicians who dropped out of a study prior to consent to contrast with clinicians who provided the intervention under investigation. One study ( Hoagwood et al., 2007 ), used a typical case approach to identify participants for a qualitative assessment of the challenges faced in implementing a trauma-focused intervention for youth. One study ( Green & Aarons, 2011 ) used a combined snowball sampling/criterion-i strategy by asking recruited program managers to identify clinicians, administrative support staff, and consumers for project recruitment. County mental directors, agency directors, and program managers were recruited to represent the policy interests of implementation while clinicians, administrative support staff and consumers were recruited to represent the direct practice perspectives of EBP implementation.
Table 2 below provides a description of the use of different purposeful sampling strategies in mixed methods implementation studies. Criterion-i sampling was most frequently used in mixed methods implementation studies that employed a simultaneous design where the qualitative method was secondary to the quantitative method or studies that employed a simultaneous structure where the qualitative and quantitative methods were assigned equal priority. These mixed method designs were used to complement the depth of understanding afforded by the qualitative methods with the breadth of understanding afforded by the quantitative methods (n = 13), to explain or elaborate upon the findings of one set of methods (usually quantitative) with the findings from the other set of methods (n = 10), or to seek convergence through triangulation of results or quantifying qualitative data (n = 8). The process of mixing methods in the large majority (n = 18) of these studies involved embedding the qualitative study within the larger quantitative study. In one study (Goia & Dziadosz, 2008), criterion sampling was used in a simultaneous design where quantitative and qualitative data were merged together in a complementary fashion, and in two studies (Aarons et al., 2012; Zazelli et al., 2008 ), quantitative and qualitative data were connected together, one in sequential design for the purpose of developing a conceptual model ( Zazelli et al., 2008 ), and one in a simultaneous design for the purpose of complementing one another (Aarons et al., 2012). Three of the six studies that used maximum variation sampling used a simultaneous structure with quantitative methods taking priority over qualitative methods and a process of embedding the qualitative methods in a larger quantitative study ( Henke et al., 2008 ; Palinkas et al., 2010; Slade et al., 2008 ). Two of the six studies used maximum variation sampling in a sequential design ( Aarons et al., 2009 ; Zazelli et al., 2008 ) and one in a simultaneous design (Henke et al., 2010) for the purpose of development, and three used it in a simultaneous design for complementarity ( Bachman et al., 2009 ; Henke et al., 2008; Palinkas, Ell, Hansen, Cabassa, & Wells, 2011 ). The two studies relying upon intensity sampling used a simultaneous structure for the purpose of either convergence or expansion, and both studies involved a qualitative study embedded in a larger quantitative study ( Aarons & Palinkas, 2007 ; Kramer & Burns, 2008 ). The single typical case study involved a simultaneous design where the qualitative study was embedded in a larger quantitative study for the purpose of complementarity ( Hoagwood et al., 2007 ). The snowball/maximum variation study involved a sequential design where the qualitative study was merged into the quantitative data for the purpose of convergence and conceptual model development ( Green & Aarons, 2011 ). Although not used in any of the 28 implementation studies examined here, another common sequential sampling strategy is using criteria sampling of the larger quantitative sample to produce a second-stage qualitative sample in a manner similar to maximum variation sampling, except that the former narrows the range of variation while the latter expands the range.
Purposeful sampling strategies and mixed method designs in implementation research
Criterion-i sampling as a purposeful sampling strategy shares many characteristics with random probability sampling, despite having different aims and different procedures for identifying and selecting potential participants. In both instances, study participants are drawn from agencies, organizations or systems involved in the implementation process. Individuals are selected based on the assumption that they possess knowledge and experience with the phenomenon of interest (i.e., the implementation of an EBP) and thus will be able to provide information that is both detailed (depth) and generalizable (breadth). Participants for a qualitative study, usually service providers, consumers, agency directors, or state policy-makers, are drawn from the larger sample of participants in the quantitative study. They are selected from the larger sample because they meet the same criteria, in this case, playing a specific role in the organization and/or implementation process. To some extent, they are assumed to be “representative” of that role, although implementation studies rarely explain the rationale for selecting only some and not all of the available role representatives (i.e., recruiting 15 providers from an agency for semi-structured interviews out of an available sample of 25 providers). From the perspective of qualitative methodology, participants who meet or exceed a specific criterion or criteria possess intimate (or, at the very least, greater) knowledge of the phenomenon of interest by virtue of their experience, making them information-rich cases.
However, criterion sampling may not be the most appropriate strategy for implementation research because by attempting to capture both breadth and depth of understanding, it may actually be inadequate to the task of accomplishing either. Although qualitative methods are often contrasted with quantitative methods on the basis of depth versus breadth, they actually require elements of both in order to provide a comprehensive understanding of the phenomenon of interest. Ideally, the goal of achieving theoretical saturation by providing as much detail as possible involves selection of individuals or cases that can ensure all aspects of that phenomenon are included in the examination and that any one aspect is thoroughly examined. This goal, therefore, requires an approach that sequentially or simultaneously expands and narrows the field of view, respectively. By selecting only individuals who meet a specific criterion defined on the basis of their role in the implementation process or who have a specific experience (e.g., engaged only in an implementation defined as successful or only in one defined as unsuccessful), one may fail to capture the experiences or activities of other groups playing other roles in the process. For instance, a focus only on practitioners may fail to capture the insights, experiences, and activities of consumers, family members, agency directors, administrative staff, or state policy leaders in the implementation process, thus limiting the breadth of understanding of that process. On the other hand, selecting participants on the basis of whether they were a practitioner, consumer, director, staff, or any of the above, may fail to identify those with the greatest experience or most knowledgeable or most able to communicate what they know and/or have experienced, thus limiting the depth of understanding of the implementation process.
To address the potential limitations of criterion sampling, other purposeful sampling strategies should be considered and possibly adopted in implementation research ( Figure 1 ). For instance, strategies placing greater emphasis on breadth and variation such as maximum variation, extreme case, confirming and disconfirming case sampling are better suited for an examination of differences, while strategies placing greater emphasis on depth and similarity such as homogeneous, snowball, and typical case sampling are better suited for an examination of commonalities or similarities, even though both types of sampling strategies include a focus on both differences and similarities. Alternatives to criterion sampling may be more appropriate to the specific functions of mixed methods, however. For instance, using qualitative methods for the purpose of complementarity may require that a sampling strategy emphasize similarity if it is to achieve depth of understanding or explore and develop hypotheses that complement a quantitative probability sampling strategy achieving breadth of understanding and testing hypotheses ( Kemper et al., 2003 ). Similarly, mixed methods that address related questions for the purpose of expanding or explaining results or developing new measures or conceptual models may require a purposeful sampling strategy aiming for similarity that complements probability sampling aiming for variation or dispersion. A narrowly focused purposeful sampling strategy for qualitative analysis that “complements” a broader focused probability sample for quantitative analysis may help to achieve a balance between increasing inference quality/trustworthiness (internal validity) and generalizability/transferability (external validity). A single method that focuses only on a broad view may decrease internal validity at the expense of external validity ( Kemper et al., 2003 ). On the other hand, the aim of convergence (answering the same question with either method) may suggest use of a purposeful sampling strategy that aims for breadth that parallels the quantitative probability sampling strategy.

Purposeful and Random Sampling Strategies for Mixed Method Implementation Studies
- (1) Priority and sequencing of Qualitative (QUAL) and Quantitative (QUAN) can be reversed.
- (2) Refers to emphasis of sampling strategy.

Furthermore, the specific nature of implementation research suggests that a multistage purposeful sampling strategy be used. Three different multistage sampling strategies are illustrated in Figure 1 below. Several qualitative methodologists recommend sampling for variation (breadth) before sampling for commonalities (depth) ( Glaser, 1978 ; Bernard, 2002 ) (Multistage I). Also known as a “funnel approach”, this strategy is often recommended when conducting semi-structured interviews ( Spradley, 1979 ) or focus groups ( Morgan, 1997 ). This approach begins with a broad view of the topic and then proceeds to narrow down the conversation to very specific components of the topic. However, as noted earlier, the lack of a clear understanding of the nature of the range may require an iterative approach where each stage of data analysis helps to determine subsequent means of data collection and analysis ( Denzen, 1978 ; Patton, 2001) (Multistage II). Similarly, multistage purposeful sampling designs like opportunistic or emergent sampling, allow the option of adding to a sample to take advantage of unforeseen opportunities after data collection has been initiated (Patton, 2001, p. 240) (Multistage III). Multistage I models generally involve two stages, while a Multistage II model requires a minimum of 3 stages, alternating from sampling for variation to sampling for similarity. A Multistage III model begins with sampling for variation and ends with sampling for similarity, but may involve one or more intervening stages of sampling for variation or similarity as the need or opportunity arises.
Multistage purposeful sampling is also consistent with the use of hybrid designs to simultaneously examine intervention effectiveness and implementation. An extension of the concept of “practical clinical trials” ( Tunis, Stryer & Clancey, 2003 ), effectiveness-implementation hybrid designs provide benefits such as more rapid translational gains in clinical intervention uptake, more effective implementation strategies, and more useful information for researchers and decision makers ( Curran et al., 2012 ). Such designs may give equal priority to the testing of clinical treatments and implementation strategies (Hybrid Type 2) or give priority to the testing of treatment effectiveness (Hybrid Type 1) or implementation strategy (Hybrid Type 3). Curran and colleagues (2012) suggest that evaluation of the intervention’s effectiveness will require or involve use of quantitative measures while evaluation of the implementation process will require or involve use of mixed methods. When conducting a Hybrid Type 1 design (conducting a process evaluation of implementation in the context of a clinical effectiveness trial), the qualitative data could be used to inform the findings of the effectiveness trial. Thus, an effectiveness trial that finds substantial variation might purposefully select participants using a broader strategy like sampling for disconfirming cases to account for the variation. For instance, group randomized trials require knowledge of the contexts and circumstances similar and different across sites to account for inevitable site differences in interventions and assist local implementations of an intervention ( Bloom & Michalopoulos, 2013 ; Raudenbush & Liu, 2000 ). Alternatively, a narrow strategy may be used to account for the lack of variation. In either instance, the choice of a purposeful sampling strategy is determined by the outcomes of the quantitative analysis that is based on a probability sampling strategy. In Hybrid Type 2 and Type 3 designs where the implementation process is given equal or greater priority than the effectiveness trial, the purposeful sampling strategy must be first and foremost consistent with the aims of the implementation study, which may be to understand variation, central tendencies, or both. In all three instances, the sampling strategy employed for the implementation study may vary based on the priority assigned to that study relative to the effectiveness trial. For instance, purposeful sampling for a Hybrid Type 1 design may give higher priority to variation and comparison to understand the parameters of implementation processes or context as a contribution to an understanding of effectiveness outcomes (i.e., using qualitative data to expand upon or explain the results of the effectiveness trial), In effect, these process measures could be seen as modifiers of innovation/EBP outcome. In contrast, purposeful sampling for a Hybrid Type 3 design may give higher priority to similarity and depth to understand the core features of successful outcomes only.
Finally, multistage sampling strategies may be more consistent with innovations in experimental designs representing alternatives to the classic randomized controlled trial in community-based settings that have greater feasibility, acceptability, and external validity. While RCT designs provide the highest level of evidence, “in many clinical and community settings, and especially in studies with underserved populations and low resource settings, randomization may not be feasible or acceptable” ( Glasgow, et al., 2005 , p. 554). Randomized trials are also “relatively poor in assessing the benefit from complex public health or medical interventions that account for individual preferences for or against certain interventions, differential adherence or attrition, or varying dosage or tailoring of an intervention to individual needs” ( Brown et al., 2009 , p. 2). Several alternatives to the randomized design have been proposed, such as “interrupted time series,” “multiple baseline across settings” or “regression-discontinuity” designs. Optimal designs represent one such alternative to the classic RCT and are addressed in detail by Duan and colleagues (this issue) . Like purposeful sampling, optimal designs are intended to capture information-rich cases, usually identified as individuals most likely to benefit from the experimental intervention. The goal here is not to identify the typical or average patient, but patients who represent one end of the variation in an extreme case, intensity sampling, or criterion sampling strategy. Hence, a sampling strategy that begins by sampling for variation at the first stage and then sampling for homogeneity within a specific parameter of that variation (i.e., one end or the other of the distribution) at the second stage would seem the best approach for identifying an “optimal” sample for the clinical trial.
Another alternative to the classic RCT are the adaptive designs proposed by Brown and colleagues ( Brown et al, 2006 ; Brown et al., 2008 ; Brown et al., 2009 ). Adaptive designs are a sequence of trials that draw on the results of existing studies to determine the next stage of evaluation research. They use cumulative knowledge of current treatment successes or failures to change qualities of the ongoing trial. An adaptive intervention modifies what an individual subject (or community for a group-based trial) receives in response to his or her preferences or initial responses to an intervention. Consistent with multistage sampling in qualitative research, the design is somewhat iterative in nature in the sense that information gained from analysis of data collected at the first stage influences the nature of the data collected, and the way they are collected, at subsequent stages ( Denzen, 1978 ). Furthermore, many of these adaptive designs may benefit from a multistage purposeful sampling strategy at early phases of the clinical trial to identify the range of variation and core characteristics of study participants. This information can then be used for the purposes of identifying optimal dose of treatment, limiting sample size, randomizing participants into different enrollment procedures, determining who should be eligible for random assignment (as in the optimal design) to maximize treatment adherence and minimize dropout, or identifying incentives and motives that may be used to encourage participation in the trial itself.
Alternatives to the classic RCT design may also be desirable in studies that adopt a community-based participatory research framework ( Minkler & Wallerstein, 2003 ), considered to be an important tool on conducting implementation research ( Palinkas & Soydan, 2012 ). Such frameworks suggest that identification and recruitment of potential study participants will place greater emphasis on the priorities and “local knowledge” of community partners than on the need to sample for variation or uniformity. In this instance, the first stage of sampling may approximate the strategy of sampling politically important cases ( Patton, 2002 ) at the first stage, followed by other sampling strategies intended to maximize variations in stakeholder opinions or experience.
On the basis of this review, the following recommendations are offered for the use of purposeful sampling in mixed method implementation research. First, many mixed methods studies in health services research and implementation science do not clearly identify or provide a rationale for the sampling procedure for either quantitative or qualitative components of the study ( Wisdom et al., 2011 ), so a primary recommendation is for researchers to clearly describe their sampling strategies and provide the rationale for the strategy.
Second, use of a single stage strategy for purposeful sampling for qualitative portions of a mixed methods implementation study should adhere to the same general principles that govern all forms of sampling, qualitative or quantitative. Kemper and colleagues (2003) identify seven such principles: 1) the sampling strategy should stem logically from the conceptual framework as well as the research questions being addressed by the study; 2) the sample should be able to generate a thorough database on the type of phenomenon under study; 3) the sample should at least allow the possibility of drawing clear inferences and credible explanations from the data; 4) the sampling strategy must be ethical; 5) the sampling plan should be feasible; 6) the sampling plan should allow the researcher to transfer/generalize the conclusions of the study to other settings or populations; and 7) the sampling scheme should be as efficient as practical.
Third, the field of implementation research is at a stage itself where qualitative methods are intended primarily to explore the barriers and facilitators of EBP implementation and to develop new conceptual models of implementation process and outcomes. This is especially important in state implementation research, where fiscal necessities are driving policy reforms for which knowledge about EBP implementation barriers and facilitators are urgently needed. Thus a multistage strategy for purposeful sampling should begin first with a broader view with an emphasis on variation or dispersion and move to a narrow view with an emphasis on similarity or central tendencies. Such a strategy is necessary for the task of finding the optimal balance between internal and external validity.
Fourth, if we assume that probability sampling will be the preferred strategy for the quantitative components of most implementation research, the selection of a single or multistage purposeful sampling strategy should be based, in part, on how it relates to the probability sample, either for the purpose of answering the same question (in which case a strategy emphasizing variation and dispersion is preferred) or the for answering related questions (in which case, a strategy emphasizing similarity and central tendencies is preferred).
Fifth, it should be kept in mind that all sampling procedures, whether purposeful or probability, are designed to capture elements of both similarity and differences, of both centrality and dispersion, because both elements are essential to the task of generating new knowledge through the processes of comparison and contrast. Selecting a strategy that gives emphasis to one does not mean that it cannot be used for the other. Having said that, our analysis has assumed at least some degree of concordance between breadth of understanding associated with quantitative probability sampling and purposeful sampling strategies that emphasize variation on the one hand, and between the depth of understanding and purposeful sampling strategies that emphasize similarity on the other hand. While there may be some merit to that assumption, depth of understanding requires both an understanding of variation and common elements.
Finally, it should also be kept in mind that quantitative data can be generated from a purposeful sampling strategy and qualitative data can be generated from a probability sampling strategy. Each set of data is suited to a specific objective and each must adhere to a specific set of assumptions and requirements. Nevertheless, the promise of mixed methods, like the promise of implementation science, lies in its ability to move beyond the confines of existing methodological approaches and develop innovative solutions to important and complex problems. For states engaged in EBP implementation, the need for these solutions is urgent.

Multistage Purposeful Sampling Strategies
Acknowledgments
This study was funded through a grant from the National Institute of Mental Health (P30-MH090322: K. Hoagwood, PI).
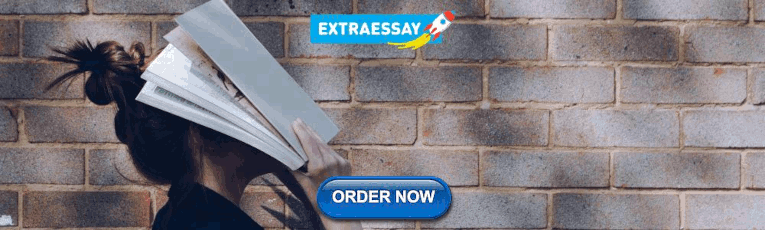
IMAGES
VIDEO
COMMENTS
When to use systematic sampling. Systematic sampling is a method that imitates many of the randomization benefits of simple random sampling, but is slightly easier to conduct.. You can use systematic sampling with a list of the entire population, like you would in simple random sampling.However, unlike with simple random sampling, you can also use this method when you're unable to access a ...
The entire purpose of "probability-based random sampling is generalization from the sample to a population."(Patton, 2002: 273). ... Similarly, Shaw and Holland (2014) argue, "there is little logic in attempting random or probability sampling with qualitative research studies. The people, settings, or text we choose to include in new ...
The usually small sample size in qualitative research depends on the information richness of the data, the variety of participants (or other units), the broadness of the research question and the phenomenon, the data collection method (e.g., individual or group interviews) and the type of sampling strategy.
Abstract. The chapter discusses different types of sampling methods used in qualitative research to select information-rich cases. Two types of sampling techniques are discussed in the past ...
Qualitative evidence syntheses, also known as systematic reviews of qualitative research, aim to explore people's perceptions and experiences of the world around them by synthesizing data from studies across a range of settings. ... Purposeful random sampling • Randomly select from the list of included studies for inclusion in the analysis ...
Sampling can be divided in a number of different ways. At a basic level, with the exception of total population sampling you will often see the divide between random sampling of a representative population and non-random sampling. Clearly, for many more quantitative-minded researchers, non-random sampling is the second-choice approach as it creates
Systematic random sampling is a probability sampling method. This means it uses chance and randomisation to select sample data that represents a population. After determining the right sample size, researchers assign a regular interval number they will use to select which members of the target population will be included in the sample.
five random sampling schemes (i.e., simple random sampling, stratified random sampling, cluster random sampling, systematic random sampling, and multi-stage random sampling). Conversely, if the goal is not to generalize to a population but to obtain insights into a phenomenon, individuals, or events, as is most often the case in interpretivist
Purposive Sampling. Purposive (or purposeful) sampling is a non-probability technique used to deliberately select the best sources of data to meet the purpose of the study. Purposive sampling is sometimes referred to as theoretical or selective or specific sampling. Theoretical sampling is used in qualitative research when a study is designed ...
Another type of sampling discussed by some authors is "systematic random sample.". The steps for this method are: Make a list of all the potential recruits. Using a random method (described earlier) to select a starting point (example number 4) Select this number and every fifth number from this starting point.
Example: Simple random sampling. You want to select a simple random sample of 1000 employees of a social media marketing company. You assign a number to every employee in the company database from 1 to 1000, and use a random number generator to select 100 numbers. 2. Systematic sampling.
In this section, let's explore four standard sampling techniques used in qualitative research: purposive sampling, convenience sampling, snowball sampling, and theoretical sampling. We'll break down the definition of each technique, when to use it, and its advantages and disadvantages. 1. Purposive Sampling.
The example systematic methods overview on sampling in qualitative research. The principles and strategies we propose in this paper are derived from experience conducting a systematic methods overview on the topic of sampling in qualitative research . The main objective of that methods overview was to bring clarity and deeper understanding of ...
Systematic Sampling. In systematic sampling (also called systematic random sampling) every Nth member of population is selected to be included in the study. It is a probability sampling method. Systematic sampling can be more suitable than simple random sampling because the former can be time-consuming. It has been stated that "with ...
Systematic sampling without a population list. You can use systematic sampling to imitate the randomisation of simple random sampling when you don't have access to a full list of the population in advance. Research example You run a department store and are interested in how you can improve the shopping experience for your customers. To ...
Sample selection was carried out using the Systematic Random Sampling method (Bhardwaj, 2019), involving regular sampling at specified intervals from the sorted sample frame or selecting specific ...
Regular Sampling Intervals: Systematic sampling involves selecting every kth item from the population, where k is a fixed interval. For example, if the population size is 1000 and the sample size is 100, then the sampling interval is 10 (i.e., 1000/100). Random Starting Point: The first item in the sample is selected randomly from the first k ...
Sampling types. There are two major categories of sampling methods ( figure 1 ): 1; probability sampling methods where all subjects in the target population have equal chances to be selected in the sample [ 1, 2] and 2; non-probability sampling methods where the sample population is selected in a non-systematic process that does not guarantee ...
We first calculate the interval by dividing the total number of people in the population (100) by the number we want in the sample (20). This gives us a sample interval of 5. We then select a number between 1 and the sampling interval from a random number generator. Let's say we get 4 — this is where we start.
Random Sampling. Definition. A systematic process of selecting subjects or units for examination and analysis that does not take contextual or local features into account. When is it used? Random sampling is typically used in experimental and quasi-experimental designs. Random sampling typically involves the generation of large samples.
Abstract. In gerontology the most recognized and elaborate discourse about sampling is generally thought to be in quantitative research associated with survey research and medical research. But sampling has long been a central concern in the social and humanistic inquiry, albeit in a different guise suited to the different goals.
Systematic Random Sampling is a method of selecting a sample from a population in a structured and organized manner. It is a valuable technique in research and statistical analysis providing a systematic yet random approach to sample selection ensuring reliable and accurate results.
Principles of Purposeful Sampling. Purposeful sampling is a technique widely used in qualitative research for the identification and selection of information-rich cases for the most effective use of limited resources (Patton, 2002).This involves identifying and selecting individuals or groups of individuals that are especially knowledgeable about or experienced with a phenomenon of interest ...