Advertisement
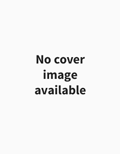
- Previous Article
- Next Article
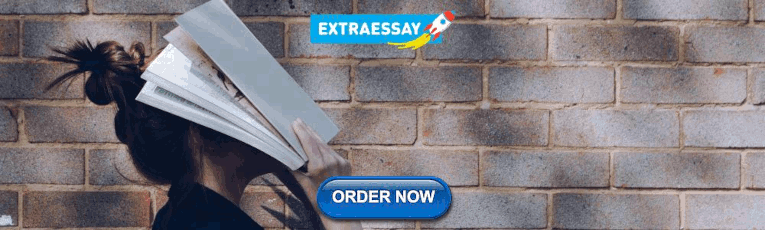
Optimization of Large Gas Pipeline Network--A Case Study in China
- Split-Screen
- Open the PDF for in another window
- View This Citation
- Add to Citation Manager
- Get Permissions
- Search Site
Jin, Lu, and Andrew K. Wojtanowicz. "Optimization of Large Gas Pipeline Network--A Case Study in China." J Can Pet Technol 49 (2010): 36–43. doi: https://doi.org/10.2118/136345-PA
Download citation file:
- Ris (Zotero)
- Reference Manager
In China, annual natural gas consumption is over 67 billion cubic meters with an expected growth rate of 10% per year. Most of the gas is transported from well heads to markets over cross-country gas networks, which requires construction of the West to East Gas Network--one of the largest gas networks in the world. Presently, the network is comprised of four large-diameter pipelines and will include most major gas pipelines in China in the future. The network distributes approximately 30 x 109 m 3 gas per year, of which 3% to 5% is burned to power the gas transportation. At current gas prices, gas transportation costs are roughly 350 million per year, which is a considerable cost that could be reduced by improvements in network design and operation.
This paper reports on a study aimed at optimizing the network to minimize its energy consumption and cost. The large size and complex geometry of the network required breaking the study down into simple components, optimizing operation of the components locally, re-combining the optimized components into the network and optimizing the network globally. This four-step approach employed four different optimization methods, penalty function method, pattern search, enumeration and non-sequential dynamic programming, to solve the problem. The results show that cost savings, because of global optimization, are reduced with increased throughput. For example, increasing the gas rate from 67 - 90 million m 3 /d would reduce operational cost savings because of optimization from 23% - 1.15%. Moreover, the study shows that if the compressors were fully loaded at their maximum rating, the optimized operation would approach the one being presently practiced. Thus, the optimization is effective and much needed when the system does not work at its maximum capacity, a typical case in the present operations of Chinese gas networks.
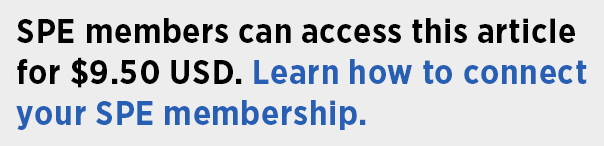
Email Alerts
Suggested reading, affiliations.
- Print ISSN 0021-9487
- Conferences
- Content Alerts
- SPE Member Pricing
- Terms of Use
Sign In or Register to Continue
Sign In or Register
Academia.edu no longer supports Internet Explorer.
To browse Academia.edu and the wider internet faster and more securely, please take a few seconds to upgrade your browser .
Enter the email address you signed up with and we'll email you a reset link.
- We're Hiring!
- Help Center
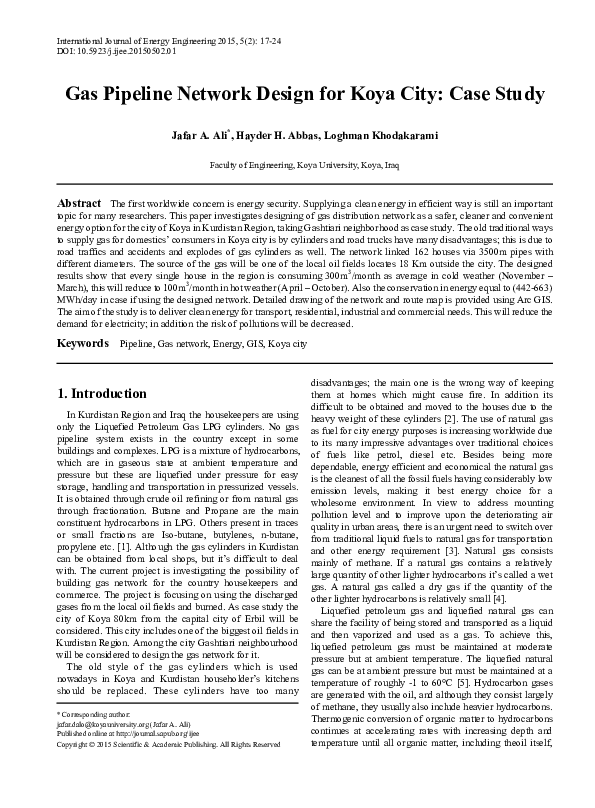
Gas Pipeline Network Design for Koya City: Case Study

2015, International Journal of Energy Engineering
Related Papers
tasya regina
From 2001 to the present, natural gas production in Indonesia dominates petroleum production. Most of the natural gas is used for export until 2013. From 2014 until now, most of the natural gas production is being utilized for domestic with an increasing trend. Domestic gas usage for households is still far below industry and commercial sectors. Domestic gas usage in the household can be done in two ways, namely city gas and Liquefied Petroleum Gas cylinder. The use of city gas is better in terms of price, mitigation, and gas emission. The government plans to build a new city gas network for 4,000 households. This study aim is to propose the design of city gas network, so the construction and operational costs become minimal. This research uses three stages, namely division of region by using the clustering algorithm, the gas network route determination using heuristics algorithm, and determination of the feasibility using the Benefit-Cost Ratio. We have successfully calculated the ...
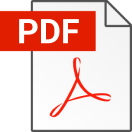
Mohd Amin Abd Majid
Nigerian Journal of Technology
Ibrahim D Muhammad
This work presents the conceptual design of a gas distribution pipeline network for estates in Nigeria using the University of Abuja Staff Quarters as a case study. The problem statement was the aggressive consumption of cooking gas, referred to as liquefied petroleum gas (LPG), without gas pipeline networks infrastructures to homes and estates across Nigeria but relies on cylinders with its attendant danger. The methodology includes the determination of the gas demand from the average monthly gas consumption in each of the households, the elevation head, diameter of the pipelines, gas velocity, gas mass flow rate, head losses and the pressure drop analysis of series (option 1), parallel (option 2) and grid (option 3) options. The results obtained indicate that the best gas distribution design option for the trunk, reticulation and service pipelines was the grid connections to minimize investment costs with equitable pressures at service outlets. In the selected design option, the t...
SN Applied Sciences
ANIRBID SIRCAR
Berge Djebedjian
One of the major parameters that affect the price of natural gas is the cost of its transmission and distribution networks. So it's valuable to reduce its total cost to be affordable for individual customer. Gas networks optimization is raised to focus on the network cost regarding to its design parameters after the revolution in personal computers. This work is a step towards developing a computer code that simulate and optimize gas distribution networks at all networks pressure ranges, i.e. low, medium and high pressure networks. The aim is to reduce the network diameter sizes to a minimum value while fulfilling the constraints of maximum flow velocity and minimum node pressure. In this work, the gradient algorithm is used to solve the hydraulics of the networks, which had never presented in gas networks before. The algorithm was fulfilled and gave an efficient analysis for all gas networks, i.e. at all pressure ranges, at the least time any algorithm can record. Optimization ...
Applied Energy
Xavier Gabarrell , Jordi Oliver-solà , Joan Rieradevall
IOP Conference Series: Materials Science and Engineering
Małgorzata Iwanek
IJRASET Publication
India today has an in depth network of underground pipelines used for the transportation and distribution of gas. Large factories, fertilizer factories and other industrial enterprises are the most consumers in PNG and today, however, with the rise in its popularity, it's currently utilized in the domestic sector similarly as a fuel within the automotive sector in large metropolitan cities. To bring gas to those end users within the boundaries of a significant city, it's necessary to create city gas distribution pipeline networks. India today has an intensive network of underground pipelines used for the transportation and distribution of fossil fuel. Large factories, fertilizer factories and other industrial enterprises are the most consumers in PNG and today, however, with the rise in its popularity, it's currently employed in the domestic sector additionally as a fuel within the automotive sector in large metropolitan cities. To bring gas to those end users within the boundaries of a significant city, it's necessary to create city gas distribution pipeline networks, these networks have already been founded within the cities of Delhi,
Journal of Smart Science and Technology
muhammad ariyon
Trunkline gas network system from Gas Compressor Station (GCS) B to GS.B -GS.B5 total distance of ±18 km. Distance GS.B4-GS.B5 is 9400 m. Gas is the main fuel for engines at gathering stations. A deadlock was found on the GS.B4–GS.B5 pipeline. The solution to this problem is to plan a pipeline between GS.B4–GS.B5 for optimal gas supply. This study conducts an economic analysis of the planning of pipe construction with the same trunkline diameter. Selection of diameter by looking at the effect of pressure drop and erosional velocity using the Pipesim. Then take a problem solving approach by building a system using a pig launcher. Based on the research results, in the existing conditions with a distance of 9400 m using a diameter of 6 and 4 inches there is a high pressure drop. Scenario planning minimizes pressure drop by using equal diameter pipes between 4,6 and 8 inches. The analysis results show that the selected diameter is 6 inches. Pig launcher is designed with safe specs and d...
Energy Exploration & Exploitation
shahram hashemi
Natural gas is transported from production sites to end users through high pressure (5-7 MPa) transmission pipelines. At a consumption point or when passing into a lower pressure pipeline, the pressure of the gas must be reduced. This pressure reduction takes place at City Gate Stations (CGS). Currently in all of Iran's CGSs, valuable pressure exergy (potential energy) contained by the high-pressure natural gas is lost to the environment in throttling valves (expansion valves). In this study, based on a comprehensive program, the inlet and outlet properties and daily flow rates of natural gas through Khorasan province (Iran) CGSs were measured and recorded for a whole year. Based on these data, the amount of energy lost to the environment was calculated. It was found that the yearly average mass flow rate in Khorasan CGSs is 90.5 kg/s, and average energy destruction is 13,240 kW. Assuming a conversion efficiency of 75%, one can extract 9,930 kW of electrical power. Based on Iran...
RELATED PAPERS
International Research Journal of Modernization in Engineering Technology and Science
International Research Journal of Modernization in Engineering Technology and Science (IRJMETS)
Revista: Universidad de La Habana
Amanda Laura Prieto-Valdés
Alpaslan Aydin
Raimund Podloucky
Shafira Setiapuspita
Srikanta Kolay
Dariusz W . Skalski
Christian Lepers
Stavros Alexandris
Bulletin of the Chemical Society of Japan
julia taddei
African Journal of Agricultural Research
Dr. Tanzima Yeasmin
Sylvia Cooper
Thrombosis Research
Jernej Vidmar
Sandra Dias
JTK (Jurnal Tadris Kimiya)
Thelma Aluman
Madras Agricultural Journal
mahender singh
Proceedings of the ... Annual Hawaii International Conference on System Sciences
Murray Jennex
arXiv (Cornell University)
Michel Cozic
James Carter
Episteme. Revista de divulgación en estudios socioterritoriales
Episteme. Revista de divulgación en estudios socioterritoriales.
Tatag Y.E. Siswono
Case Studies in Thermal Engineering
Teerapat Chompookham
Freshwater Biology
James Lazorchak
Nature Climate Change
Bruno Gallo
RELATED TOPICS
- We're Hiring!
- Help Center
- Find new research papers in:
- Health Sciences
- Earth Sciences
- Cognitive Science
- Mathematics
- Computer Science
- Academia ©2024
Advertisement
Optimization of city gas network: a case study from Gujarat, India
- Research Article
- Published: 22 June 2019
- Volume 1 , article number 769 , ( 2019 )
Cite this article
- Anirbid Sircar 1 &
- Kriti Yadav 1
3155 Accesses
Explore all metrics
A natural gas flow network consists of city gate station, district regulator, pipelines, compressors and valves. The performance of such a complex system cannot be studied by analysing individual constituents. Simultaneous performance of integrated system can be expressed using non-linear equations to simulate and forecast operational behaviour. The operational behaviour is subject to various alternative operational conditions also. Nodal analysis is performed to calculate the flow capacity of each network segment and the pressure at each node. Two conditions are evaluated, namely, keeping flow capacity unknown or by making nodal pressure primary unknown. The resulting set of governing equations are then solved simultaneously. Genetic algorithm method is applied to solve the desired target unknown. Compressor governs the energy supply required to transport gas from one end to another. The compressor equation is also incorporated in city gas network simulation. The simulations are performed by straight gas ducts and complex gas ducts. Boundary conditions are dependent on the form in which gas duct is fed. It is assumed that the gas duct takes gas from City Gate Station at x = 0 and supplies the gas to a consumer located at x = L according to a time variable curve demand Q n (L, t). The capacity of the compressor is also changed and it is observed that the demand is usually periodic in nature. Flow demand at individual node of a gas pipeline network is calculated. Pressures at various nodes are also calculated and matched with original pressure at nodes. Percentage deviation error suggest a range between 0 and 1.02%. The aim of this paper is to optimise certain parameters of a gas transmission pipeline network namely flow rate, mass, cost and pressure. This optimization is performed to gas transmission pipeline network of Gandhinagar, district, where Gujarat State Petronet Limited (GSPL) and Sabarmati Gas Limited (SGL) are the major players. The network which has been chosen for pipeline network consists of 10 CNG stations and 10 DRS.
Similar content being viewed by others
Optimal Design of Natural Gas Gathering Systems with Production Capacity Expansion
Sensitivity analysis of gas supply optimization models.
Optimal Control of Compressor Stations in a Coupled Gas-to-Power Network
Avoid common mistakes on your manuscript.
1 Introduction
According to Boston Consulting Group (BCG) in 2017 global gas consumption is estimated to have growth of 3.7% which is double the average annual growth rate from 2010 to 2016. In 2017 Asia and Europe were the leading growth and development in gas sector. The gas consumption in Asia was estimated to have grown by more than 35 bcm (5.3%) in 2017 which has been accounted for the primary energy production. As we know that pipeline networks are the most important way to transport natural gas to end users by using different types of pipeline networks like gathering network, trunk network and distribution network. As the demand and consumption of natural gas increases the evolved pipelines have more complex systems. Flow of gas in pipeline gets affected by friction with the walls of the pipes. The pressure drop here makes more difficult to guarantee the supply security, so that the inlet and outlet pressure of gas remains same. In this case the compressor stations can be used to counterbalance the pressure drop, but it has been found that they consume around 3–5% proportion of gas which flows through the pipeline [ 26 , 31 ].For such issues and challenges an appropriate network is required to satisfy the demand for natural gas while maximizing the operation benefits of the networks. The evaluation of new operation schemes includes a comprehensive and mathematical model which simulates pressure and the mass flow rate of the pipeline network under different operation conditions [ 16 ]. It has been found that the estimated global optimization of operations can be enhanced atleast by 15% of the network operation benefit [ 32 ].
The objective of this paper is to optimize the flow of gas in transmission pipeline network system by using genetic algorithm method. The parameters which are taken in consideration for optimization of system flow rate, pressure, gas consumption, looping condition and cost of the network. Genetic algorithm involves 5 major steps, population, encoding, fitness function, crossover and mutation. Gujarat State Petronet Limited (GSPL) and Sabarmati Gas Limited (SGL) were looking for the optimisation of gas transmission pipeline network so that the cost of the network can be optimised at each node. In this reference present researchers applied genetic algorithm method to a gas pipeline network in Gandhinagar we tried to optimise the cost at each node and the results are encouraging. Optimising the parameters like flow rate, pressure, mass consumption and loop condition leads to the optimization of pipeline network cost.
2 Literature survey
A problem of gas network through compressor stations, including the operation scheme optimization was introduced by O’Neill et al. [ 20 ]. De Wolf and Smeers [ 9 ] modified the problem of O’Neill et al. [ 20 ] by using Piecewise Linear Programing (PLP) approach. De Wolf and Smeers [ 9 ] further represented this solution as “Special ordered sets of type 2” by which the piecewise linear problem can be globally solved by using mixed integer programming code. In 2002 a reduction technique for minimizing fuel consumption by compressors in steady state gas transmission network was proposed by Rios-Mercado et al. [ 24 ]. The base of this technique was novel combination of graph theory and nonlinear functional analysis. This technique can optimize the problem size by without disrupting its mathematical structure.
Differential evolution technique is applied by Babu et al. [ 3 ] to optimize the design of gas transmission pipeline network. The method was generally used for complex and highly complicated non-linear problems. This differential evolution technique was able to find optimised solution which satisfies all the constraints and takes less computational time. To cope up with the forecasted demand for natural gas. Pietrasz et al. [ 22 ] studied the problem of reinforcing regional gas transmission pipeline network. The objective of this study was to optimise the reinforcement costs. Depending on topological decomposition techniques, evolutionary algorithms or search space reduction method is categorised in three sections. It was based on truncated branch and bound leaded to a solution which was locally optimised in the neighbourhood of relaxed solution by giving the continuous relaxation. A technique for optimizing the investment costs on existing gas transmission pipeline network was proposed by Andre et al. [ 2 ]. The network was optimised by two findings first that the location of pipeline segments to be reinforced and second by optimizing sizes for the satisfaction of demands with high pressure for all users. An ant colony optimization technique was proposed by Chebouba et al. [ 7 ] for steady state flow gas pipeline operations. The results of this technique were compared with those which were obtained by employing dynamic programing method which shows that ant colony optimization is an effective way of gas transmission network optimization. In recent times the natural gas pipeline network optimization research have been categorised into two categories. The first category includes methods like regression method including stochastic [ 33 ], linear programming [ 1 ], interval analysis approach [ 4 ], a sequential quadratic method [ 10 ], a non- sequential quadratic method [ 6 ], a bi-level programing method [ 34 ] and economic nonlinear model [ 11 ] and the other category is intelligent method [ 18 ].
3 Study area
Gujarat is India’s first completely covered piped gas state in India. The pipeline networks are being operated by more than one player namely, Gujarat State Petronet Limited (GSPL), GAIL India Limited, Sabarmati Gas Limited (SGL), Adani Gas Limited, Gujarat Gas company limited etc. The major gas distribution source in this state is either from Gandhar field of ONGC or from Oil and Natural Gas Corporation (ONGC’s) General Petroleum Company (GPC) at Hazira. In this paper Gandhinagar, Gujarat, India has been selected as study area for optimisation of pipeline network.
Gandhinagar is a capital of Gujarat which has an aerial extent of 649 km 2 . GSPL and SGL are the major entities working in this region. The pipeline network chosen here for optimisation consists of 10 CNG stations and 10 DRS (Fig. 1 ). This network have 12 nodes between 7 compressors and 2 gas sources as shown in Fig. 2 .
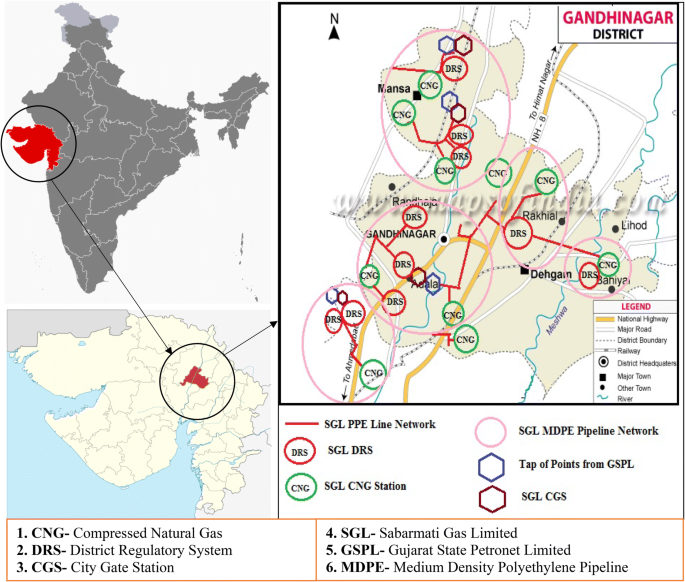
Study area for optimization of CGD network from Gujarat, India
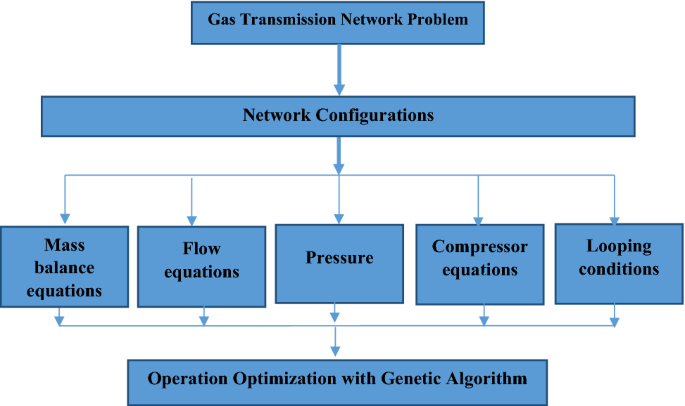
Modified after Woldeyohannes and Majid [ 29 ]
General procedure for mathematical formulation of transmission pipeline network simulation.
4 Need of optimisation
Optimisation helps to achieve best relative to a set of prioritized criteria or constraints. These includes maximising factors such as productivity, strength, reliability, longevity, efficiency and utilisation. By increasing the efficiency of the existing network by lowering the operational cost one can achieve higher profit from it. Optimisation leads to improvement of four attributes discussed below:
Profitability increase Even a small improvements in gas supply chain can gas market profitability and can be viable in long term business.
Reliability improvement A network optimizer can facilitate to rapidly reallocate production upon equipment failure to satisfy customers and honor contractual delivery commitments.
Investment planning The insights into network bottlenecks and to enable planning for debottlenecking or expansion projects are gained by comprehensive network models.
Flare reduction Minimize environmental and economic penalties from inefficient operations.
5 Mathematical formulation for simulation of gas transmission pipeline network (GTPN)
The gas optimization network is categorised into 2 categories namely optimization of gas distribution network and optimization of gas transmission pipeline. This study is based on the gas transmission network including compressor stations and other gas pipeline constraints. Optimization of gas network is defined as means of objective function searching for optimal design parameters, structures and developments for operation of networks [ 21 ]. Optimization of gas transmission network can be done either in steady state or in transient state [ 27 ].
It has been found that when gas moves by GTPN, it passes through pipelines, valves, regulators and compressors. Friction of gas with the wall of pipes and heat transfer between gas and the surrounding leads to gas pressure drop in the pipeline. In order to boost the gas pressure compressor stations are usually installed. Around 3–5% of the gas is consumed by compressors while compensating the lost pressure of the gas [ 5 , 31 ]. According to Wu [ 30 ] even 1% of improvement on the performance of gas transmission pipeline network of US can result in a saving of 48.6 million dollars. The major constraints of designing and operating of any gas transmission pipeline is minimizing the energy consumption and increasing the gas flow rate through pipes. After observing the above mentioned facts in this paper a mathematical model is used for the simulation of gas transmission pipeline network. This simulation optimizes parameters namely flow rate, pressure, gas consumption, looping condition and cost of the network. Figure 2 depicts the general outflow for mathematical model based on configurations and basic elements of gas transmission pipeline network. Woldeyohannes and Majid [ 29 ] simulation method was adopted in the present study which involves all the basic elements of any gas transmission pipeline network. This method is modified in the present study by adding the compressors equation in governing simulation algorithm. By adding the compressor equation we have optimised the cost at every node and also the cost of whole network, shown in Table 5 .
5.1 Formulation of different Constraints
5.1.1 outflow and inflow rate.
Flow rate depends on the area of the channel that the gas is flowing from and the velocity of gas. The equation for flow rate can be expressed as:
where Q = gas flow rate (m 3 /s), A = area of the channel (m 2 ) and v = velocity of the gas (m/s).
Supply nodes should have gas at an optimum mass flow rate as demanded by consumers. The mass flow rate at supply nodes must be greater than the mass flow rate produced by the well. Hence, \(Q_{imin} \le Q_{i } \le Q_{imax}\) , i = 1, 2, … N n . Where \(Q_{i }\) represents the gas inflow and outflow rate at node ‘i’ (m 3 ), \(Q_{imin}\) is minimum allowable inflow and outflow rate of gas at node ‘i’ (m 3 ) and \(Q_{imax}\) is maximum allowable inflow and outflow rate of gas at node ‘i’ (m 3 ).
5.1.2 Pressure in pipe
The basic equation used for inlet and outlet pressures in gas transmission pipeline can be given as [ 19 ]:
where L = pipe length (m), D = pipe diameter (mm), T s = standard temperature (K), P s = standard pressure (1.01325 bar), f = friction factor, S = gas gravity, Z = gas compressibility factor at the flowing temperature (dimensionless), P i = inlet pressure (bar) and P o = outlet pressure (bar).
The outflow and inflow pressure is similar to mass flow rate, which means \(P_{min} \le P \le P_{max}\) . Where \(P\) is pressure at particular node (MPa), \(P_{min}\) is minimum allowable pressure at particular node (MPa) and \(P_{max}\) is maximum allowable pressure at particular node (MPa).
5.1.3 Loop conditions
In loop piping two or more pipes are connected in such a way that the gas flow can be split among branched pipes and eventually downstream into a single pipe. According to looping condition there is zero pressure drop in a closed loop within the network system [ 17 , 21 ].The loop which is considered here is mentioned in Fig. 2 which includes node points 2, 5, 6, 8, 10 and 12. The equation for single phase general flow can be expressed as:
where Q = gas flow rate through pipe, L = length of the pipe and D = diameter of the pipe.
5.1.4 Pressure drop equation of pipelines
It is the pressure drop in the pipeline as inlet and outlet pressure [ 13 ]. The expression for this can be given as:
where w = flow rate, g = acceleration of gravity, A = cross-sectional area of pipe, V 1 = specific volume of gas at inflow condition (ft 3 /lbm), f = friction factor dimensionless, L = length (ft), D = diameter of the pipe (ft), P i = inflow pressure (psi) and P o = outflow pressure (psi).
5.1.5 Mass flow rate balance equation
According to mass conservation law, the outflow rate and inflow rate should be equal to 0.
where \(C_{i}\) = sets of elements connected with node ‘i’, \(M_{ik}\) = absolute value of inflow (outflow) rate of element k connected with node ‘i’ (m 3 ), \(Q_{i}\) = exchange flow rate between node ‘i’ and outside (inflow is set as positive value and outflow is negative value) (m 3 ) and \(a_{ik}\) = A coefficient, its value is − 1 when gas in element k flow into node ‘i’, the value is 1 when gas in element k flow out of node i.
5.1.6 Compressor equation
The compressor map information is related to outlet pressure, the inlet pressure and flow rate. The relationship between inlet pressure P i and outlet pressure P o with head H is expressed as [ 12 ]:
where H = head, P i = inlet pressure, P o = outlet pressure, m = (k − 1)/k, k is specific heat ration, Ts = inlet gas temperature, Z = compressibility of the gas and R = gas constant.
The compressor performance governing equation for the simulation model can be given as [ 15 ]:
where H = adiabatic head, P i = inlet pressure, P o = outlet pressure, m = (k − 1)/k, k is specific heat ration, Ts = inlet gas temperature, Z = compressibility of the gas, R = gas constant, A H , B H , C H and D H = constants which depend on the unit, Q = flow rate, n = speed and η = adiabatic efficiency.
Equation 8 is a general compressor equation for single compressor. This equation is one of the most significant contribution in order to optimize gas transmission pipeline network.
6 Simulation of optimization of gas network using genetic algorithm
In genetic algorithm method a class of adaptive algorithm are represented whose search methods are based on the simulation of natural genetics. It falls under the class of probabilistic algorithms. The parameters and steps which will be involved in simulation of optimization of gas networking are discussed below. Before starting the genetic algorithm, the sampling interval of each parameters such as flow rate, pressure, loop condition, mass consumption and cost is calculated using the following expression:
where \(\Delta S\) = sampling interval, \(S_{max}\) = maximum value of a particular parameter, \(S_{min}\) = minimum value of a particular parameter, m = encoding base (for binary m = 2) and n = number of bits which represent each parameter in binary code.
The sampling is required to be estimated as it represents the whole population. Let us consider the case of cost which varies from 60 to 74%, and each cost value is binary coded with eight bits. The resolution of sampling for the same is 0.05%.
6.1 Population
The primary set of population is generated randomly and size of population depends upon the complexity of pipeline network [ 14 ]. In order to discover new clones [ 23 ] the initial population should be a large pool of different genes. Including different genes for initial population leads to an algorithm which has enough diversity in the population to get fast and good solutions. In this paper the population size is taken to be 256 (2 8 ). The range of population is taken here as 255.
6.2 Encoding
After the generation of population a standard binary coding is followed. The pipeline parameters need to be converted into binary numbers. As for example, the cost at 1 st node is “70” (hypothetical scenario) which can be encoded as “01000110”. As the population size in present case is 256, so for each parameter around 256 strings of populations will be estimated for optimization. The evaluation of full cycle of genetic algorithm for cost is shown in Table 1 . As the population size is big hence only 4 strings are chosen for manual process, while the algorithm has taken care of whole 256 population.
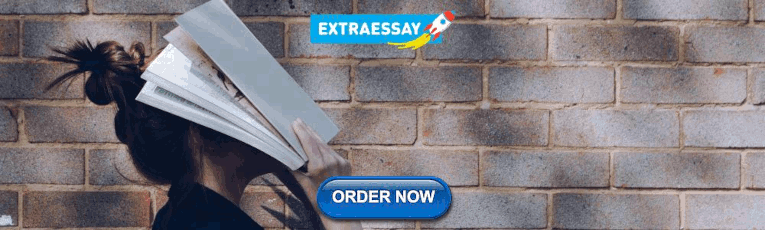
6.3 Fitness function
Fitness function is defined as the ratio of the assessment value of a particular clone to the average assessment of all the clones. In this paper power law by Sadjadi [ 25 ] fitness function f(x) = (x k ) has been applied for each population assessment. For binary representation in this case × is considered as 2 and the k (Problem dependent) value is taken 0.5 as the range of population is 2 8 . The “n” number of population size the fitness for i th chromosome can be given as F i while the average fitness of the i th generation can be calculated as:
The equation for fitness function probability selection [ 8 ] can be expressed as:
where P i = fitness probability and F i = individual parameters fitness.
6.4 Expected count
The expected count (Ei) of each string for population size “n” can be given as:
For example if a string is having E i = 2.5 then it will have two outcomes one with probability of 0.1 and other with a probability of 0.5. The minimum count is represented as E i = 0, which is removed from the population. Based upon the expected count each individual get multiple copies.
6.5 Crossover
Crossover is the process of generating better quality genes by exchanging the good information between the particular parents. The crossover probability can be calculated as the ratio of pairs of clones which will be selected for mating to the total number of pairs of clones.
For example in present study single point crossover method is used. In which the designed algorithm can pick a single cross over site randomly. As we can see in Table 2 that is based on the fitness value second string has been replaced by the fourth string. In this case two pairs of strings are evaluated, where the first two strings have crossover site six and last two string have cross over site four. Similarly for the last two strings, the tail bits are exchanged at the crossover site after fourth bit.
6.6 Crossover probability
After the selection of a pair of chromosomes, crossover is performed to generate offspring. Crossover probability is defined as the ration of pairs of chromosomes which will be selected for mating to the total number of pairs of chromosomes. Crossover is performed to generate new chromosomes which will accomplish good qualities of the old chromosomes. Based on the various experiments in this case the crossover probability is taken to be 65%. 65% of crossover probability means that out of 100 pairs of strings, only 65 pairs of randomly chosen strings will have crossover and the rest string pairs will remain unchanged.
6.7 Mutation
Mutation can generate new genes by flipping one or more gene values randomly in a clone. The mutation probability can be calculated as ratio of the bits to be flipped randomly to the total bits of clones [ 28 ].
A multi- directional search is performed by genetic algorithm in order to maintain a population of potential solutions and encourage information formation along with exchange between these directions. A number of populated solutions are developed in this simulated evolution in which the relatively “good” solutions reproduce. The different solutions are distinguished on the basis of evaluation function which plays the role of an environment.
After the individuals are converted into population by determining the fitness function of individual. The simulation further proceeds to crossover selection and mutation probability the simulation needs to be checked whether the termination conditions are satisfied or not. If it meets the desired output than the outputs are the optimization results otherwise it needs to be processed again from the fitness function determination point.
In present study flip bit mutation method is used. In this mutation process, the bits (0 and 1) of the selected genes are flipped by mutation operator. Mutation operator is generated randomly. According to Table 3 the first and third string are having mutation. The last bit of the first string and the fifth bit of the third string have been flipped by the mutation operator.
6.8 Mutation probability
Mutation probability is defined as the ratio of the bits to be flipped randomly to the total bits of the chromosomes. For example a chromosome of length 100 bits have mutation probability of 0.06 that means only six bits randomly chosen will be flipped. In this example the mutation probability value is kept at 12% because the high mutation probability will change the maximum genes of the chromosome, and the algorithm will relapse into a random search for an optimum value.
7 Simulation application
In this paper a 12 node gas transmission network is taken which consist of 2 gas source and 7 compressors (Fig. 3 ). The network was considered as in steady state condition. A High Density Polyethylene (HDPE) type of pipe is used in the segment after source pipeline to consumer segment. The pressure of gas in the transmission pipeline network ranges from 2.2 MPa to 3.45 MPa. The factors like description of pipe, pipeline specification, length of pipe and fitting friction coefficient is described in Table 4 . While the parameters like input/output quantity, quantity constraints and purchasing price of gas of each node before and after optimization are shown in Table 5 .
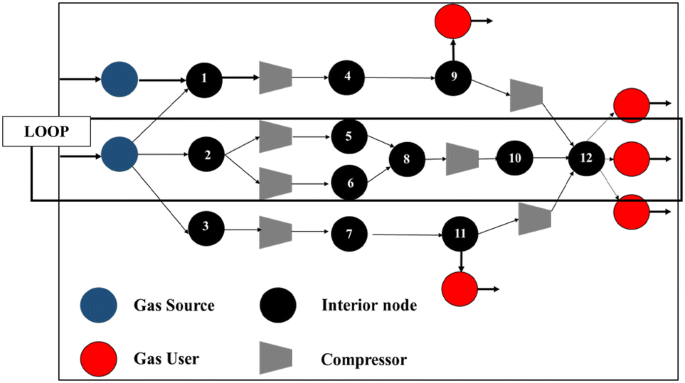
Gas transmission pipeline system consisting of 12 nodes, 7 compressors and 2 gas source
It has been found according to Table 5 that the gas consumption of each node has been optimized between of 30,000–50,000 m 3 /h range, while pressure is optimized in the range of 3.01–3.99 MPa. The total cost before optimization was 276 × 10 4 INR/day whereas after optimization it was found to be 280.32 × 10 4 INR/day.
8 Conclusion
This paper present a mathematical model approach for optimization of gas transmission network. For gas transmission network the algorithm for genetic algorithm is developed in C ++. The objective of this research was to reach the global optimum solution instead of local minima solution for interdependent parameters. In this paper the simulation details are followed by the formulation of different constraints like inlet and outlet pressure, inlet and outlet flow rate, pressure drop equations of pipeline, loop conditions, compressor equations and mass flow rate balance equation and lost at each node. Simulation was performed on the basis of four major steps namely population generation, fitness function, mutation and crossover. The simulation was used to optimize five parameters like pressure, flow rate, mass, loop and cost of a gas transmission network. It was considered that the network was in steady state condition. The simulation was applied on 12 node system where the pressure was optimized between 3.01 and 3.99 MPa range while the gas consumption was optimized between the ranges of 30,000–50,000 m 3 /h. It has been seen that the cost at each node and for the whole system is improved. According to Table 5 it has been seen that the income of the chosen network was 276 × 10 4 INR/day but after the optimization of various parameters involved cost improves to 282.32 × 10 4 INR/day.
Amani H, Kariminezhad H, Kazemzadeh H (2016) Development of natural gas flow rate in pipeline networks based on unsteady state Weymouth equation. J Nat Gas Sci Eng 33:427–437
Article Google Scholar
Andre J, Bonnans F, Cornibert L (2009) Optimization of capacity expansion planning for gas transportation networks. Eur J Oper Res 197(3):1019–1027
Article MathSciNet Google Scholar
Babu BV, Angira R, Chakole PG, Syed Mubeen JH (2003) Optimal design of gas transmission network using differential evolution. In: Proceedings of the second international conference on computational intelligence, robotics, and autonomous systems (CIRAS-2003), Singapore, December 15–18, PS0402, pp 01–07
Bonnans JF, Spiers G, Vie JL (2011) Global optimization of pipe networks by the interval analysis approach: the Belgium network case. INRIA. https://hal.inria.fr/hal-00642932
Borraz-Sanchez C, Rios-Mercado RZ (2004) A non-sequential dynamic programming approach for natural gas network optimization. WSEAS Trans Syst 3:1384–1389
Google Scholar
Borraz-Sánchez C, Ríos-Mercado RZ (2009) Improving the operation of pipeline systems on cyclic structures by tabu search. Comput Chem Eng 33(1): 58–64
Chebouba A, Yalaoui F, Smati A, Amodeo L, Younsi K, Tairi A (2009) Optimization of natural gas pipeline transportation using ant colony optimization. Comput Oper Res 36:1916–1923
Chipperfield A, Fleming P, Pohlheim H, Fonseca C (1994) Genetic algorithm toolbox. University of Sheffield, Sheffield, pp 1–105
De Wolf D, Smeers Y (2000) The gas transmission problem solved by an extension of the simplex algorithm. Manag Sci 46(11):1454–1465
Furey BP (1993) A sequential quadratic programming-based algorithm for optimization of gas networks. Automatica 29:1439–1450
Gopalakrishnan A, Biegler LT (2013) Economic nonlinear model predictive control for periodic optimal operation of gas pipeline networks. Comput Chem Eng 52:90–99
Gresh MT (2000) Compressor performance aerodynamics for the user, 2nd edn. Butterworth Heinemann, Boston
Griffith P (1984) Multiphase flow in pipes. J Petrol Technol 36(3):361–367
Haupt RL, Haupt SE (2004) Practical genetic algorithms, 2nd edn. Wiley Interscience Publication, Hoboke
MATH Google Scholar
Kurz R, Ohanian S (2003) Modeling turbo machinery in pipeline simulations. In: 35th annual meeting of Pipeline Simulation Interest Group (PSIG), Bern, Switzerland
Li CJ, Jia WL, Wu X (2009) Comprehensive valuation on regulation schemes of gas transmission pipelines. In: ASCE proceedings of the international conference on pipelines and trenchless technology, Shanghai, China, pp 581–590
Menon ES (2005) Gas pipeline hydraulics, 1st edn. CRC Press, New York
Book Google Scholar
MohamadiBaghmolaei M, Mahmoudy M, Jafari D (2014) Assessing and optimization of pipeline system performance using intelligent systems. J Nat Gas Sci Eng 18:64–76
Nasr GG (2011) Gas flow and network analysis. Salford University, Salford
O’Neill RP, Williard M, Wilkins B, Pike R (1979) A mathematical programming model for allocation of natural gas. Oper Res 27(5):857–873
Osiadacz AJ (1987) Simulation and analysis of gas networks, 1st edn. Gulf Publishing Company, Houston
Pietrasz S, Antunes M, Cornibert L (2008) The problem of reinforcing regional gas transmission networks: a performance study of three optimization methods. In: EngOpt 2008—international conference on engineering optimization, Rio de Janeiro, Brazil, 1–5 June 2008, pp 1–10
Rezaian A, Alipanah M, Pour HN, Kazemi H (2010) A genetic algorithm approach to determination of optimum diameter of gas transmission pipes. In: Paper SPE-140671-MS presented at the 34th annual SPE international conference and exhibition, Tinapa–Calabar, Nigeria, 31 July–7 August 2010
Rios-Mercado RZ, Wu S, Scout LR, Boyd EA (2002) A reduction technique for natural gas transmission network optimization problems. Ann Oper Res 117:217–234
Sadjadi FA (2004) Comparison of fitness scaling functions in genetic algorithms with applications to optical processing. In: Proceedings of SPIE (5557), SPIE, Bellingham
S’anchez BC, Mercado RZ (2005) A hybrid meta-heuristic approach for natural gas pipeline network optimization. In: LNCS, vol 3636, pp 54–65
Steinbach MC (2007) On PDE solution in transient optimization of gas networks. J Comput Appl Math 203:345–361
Thander B, Sircar A (2014) Hydrocarbon resource estimation: a stochastic approach. J Pet Explor Prod Technol 5(4):445–452
Woldeyohannes AD, Majid MAA (2010) Simulation model for natural gas transmission pipeline network system. Simul Model Pract Theory 19:196–212
Wu S (1998) Steady-state simulation and fuel cost minimization of gas pipeline networks. Department of Mathematics, Ph.D. Thesis, University of Houston
Wu S, Rios-Mercado RZ, Boyd EA, Scott LR (2000) Model relaxations for the fuel cost minimization of steady-state gas pipeline networks. Math Comput Model 31:197–220
Wu Y, Lai KK, Liu YJ (2007) Deterministic global optimization approach to steady-steady distribution gas pipeline. Optim Eng 8(3):259–275
Zavala VM (2014) Stochastic optimal control model for natural gas networks. Comput Chem Eng 64:103–113
Zhang J, Zhu D (1996) A bilevel programming method for pipe network optimization. SIAM J Optim 6:838–857
Download references
Author information
Authors and affiliations.
Centre of Excellence for Geothermal Energy, Pandit Deendayal Petroleum University, Gandhinagar, 382007, India
Anirbid Sircar & Kriti Yadav
You can also search for this author in PubMed Google Scholar
Corresponding author
Correspondence to Kriti Yadav .
Ethics declarations
Conflict of interest.
The authors declare that they have no competing interests.
Additional information
Publisher's note.
Springer Nature remains neutral with regard to jurisdictional claims in published maps and institutional affiliations.
Rights and permissions
Reprints and permissions
About this article
Sircar, A., Yadav, K. Optimization of city gas network: a case study from Gujarat, India. SN Appl. Sci. 1 , 769 (2019). https://doi.org/10.1007/s42452-019-0755-2
Download citation
Received : 22 March 2019
Accepted : 11 June 2019
Published : 22 June 2019
DOI : https://doi.org/10.1007/s42452-019-0755-2
Share this article
Anyone you share the following link with will be able to read this content:
Sorry, a shareable link is not currently available for this article.
Provided by the Springer Nature SharedIt content-sharing initiative
- City gas distribution
- Genetic algorithm
- Find a journal
- Publish with us
- Track your research
- Architecture and Design
- Asian and Pacific Studies
- Business and Economics
- Classical and Ancient Near Eastern Studies
- Computer Sciences
- Cultural Studies
- Engineering
- General Interest
- Geosciences
- Industrial Chemistry
- Islamic and Middle Eastern Studies
- Jewish Studies
- Library and Information Science, Book Studies
- Life Sciences
- Linguistics and Semiotics
- Literary Studies
- Materials Sciences
- Mathematics
- Social Sciences
- Sports and Recreation
- Theology and Religion
- Publish your article
- The role of authors
- Promoting your article
- Abstracting & indexing
- Publishing Ethics
- Why publish with De Gruyter
- How to publish with De Gruyter
- Our book series
- Our subject areas
- Your digital product at De Gruyter
- Contribute to our reference works
- Product information
- Tools & resources
- Product Information
- Promotional Materials
- Orders and Inquiries
- FAQ for Library Suppliers and Book Sellers
- Repository Policy
- Free access policy
- Open Access agreements
- Database portals
- For Authors
- Customer service
- People + Culture
- Journal Management
- How to join us
- Working at De Gruyter
- Mission & Vision
- De Gruyter Foundation
- De Gruyter Ebound
- Our Responsibility
- Partner publishers

Your purchase has been completed. Your documents are now available to view.
Pipeline Power: A Case Study of Strategic Network Investments
We analyze the impact of three controversial pipeline projects on the power structure in the Eurasian network for natural gas. Two pipelines, “Nord Stream” and “South Stream,” allow Russian gas to bypass transit countries, Ukraine and Belarus. The third project, “Nabucco,” aims at diversifying Europe’s gas imports by accessing producers in Middle East and Central Asia. If network power is measured with the Shapley Value we obtain a clear ranking of the projects which corresponds to the observed investment patterns. Nord Stream’s strategic value is huge, easily justifying the high investment cost for Germany and Russia. The additional leverage obtained through South Stream is much smaller and Nabucco is not viable. For the nucleolus in contrast, none of the pipelines has any strategic relevance at all, which contradicts the empirical evidence on investment.
Acknowledgments
This paper is part of a larger collaborative research project on the Eurasian gas network to which Ekaterina Orlova made essential contributions. We are thankful to Johannes H. Reijnierse for providing us with MATLAB code for calculating the nucleolus. We also benefited from comments of seminar participants at Stony Brook Game Theory Festival, UECE Lisbon Meeting, Higher School of Economics Moscow, and the IAEE conference in Austin.
To economize on space only appendix A.1 is published here. The full appendix including A.2 Robustness, A.3 Tables for Robustness, A.4 Tables for the Nucleolus, and A.5 Fragmented Market with Exclusive Access is available at: http://www.ms-hns.de/files/publications/pipeline-power-appendix.pdf .
A.1 Calibration
This appendix describes the functions and parameters used for the calculation of the value function [equation (1) in the main text]. Let f i j ∗ , { i , j } ∈ L ( N ) denote the solution to the program in (1) when solved for the grand coalition, which has access to all resources. To calibrate the model, p i and T ij have to be determined such that f i j ∗ are reasonably close to observed consumption and flows. As it is assumed that the players cooperate effectively, they will make efficient use of the existing network. Hence, for each player the marginal willingness to pay for gas, p i ( q ) will be equal to the local marginal cost of supplying gas, i.e. the nodal cost c i ( q ), which takes into account the physical constraints of the system. This feature is used to calibrate first inverse demand and then supply cost using data on consumption and flows.
Transport costs within Europe are small compared to the cost of producing gas and transporting it to Europe’s borders. As a first approximation, the small differencesr among local costs are neglected, and a common constant supply cost c is assumed. When the program is solved for the grand coalition, none of the links within Europe are capacity constrained. So, nodal costs differ only by the variable transportation cost between connected nodes which are small.
We assume a quadratic utility function so that each consumption node’s willingness to pay for gas is represented with a linear inverse demand function. To reduce the number of parameters, for all consumption nodes the same intercept a + c is assumed. Efficiency requires p i ( q )= a + c – b i q = c for each consumption node i . The slope parameters b i are then calibrated as to replicate the consumption in 2009: b i = a / q i , where q i is the consumption of gas in the consumption node i . As illustrated in Figure 2 , the surplus, which a player obtains from participating in the trade of pipeline gas, depends on three parameters: the difference between the demand intercept and the common supply cost a , its consumption in the base year q i , and its indigenous production q i o . The common supply cost c acts as a shift parameter, which does not affect the surplus.

The Surplus ( S i ).
A change of a , with b i being adjusted, affects all players proportionally. Such a change has little impact on the relative Shapley value (measured in percent of the total), hence, will have little effect on the relative index for bargaining power. However, a determines the absolute size of the surplus and thus, the absolute Shapley value, which is of relevance if the changes in bargaining power are compared to the cost of a pipeline project. It is difficult to support any assumption for a by hard data. Obviously, it will depend a lot on how much time customers are given to substitute to other sources of energy. Making a bold assumption, in the baseline variant a is set equal to 1500 mn €/bcm yielding a total surplus from consuming gas of 949.9 bn €/a. To check the robustness of the results, a “low-surplus” scenario with a =500 mn €/bcm is considered as well. In this case, the total surplus decreases to 334.3 bn €/a. Table 4 presents the resulting values of the slope parameter b i depending on a . All quantities are quoted in bcm/a. All prices or costs are quoted in mn €/bcm, giving the same figure as the more common €/tcm.
Consumption.
a Data for consumption in 2009 are compiled from IEA (2010) and IEA (2011).
b To reduce the number of players, Turkmenistan stands for Kazakhstan, Uzbekistan, and itself.
The parameter c acts as a shift parameter for the demand system and supposed to reflect the typical production and the transportation cost. Accordingly, it is decomposed as c = c P + c ̅ T , where c P reflects a common production cost parameter and c ̅ T an adjustment made for typical transportation cost. These values determine the patterns of production and transport which are presented next.
Table 5 presents the players’ production capacities, production volumes as well as production costs. The production volumes in 2009 are collected from IEA (2010) and IEA (2011) . For the players except Russia and Turkmenistan the production capacities are assumed equal to their production volumes in 2009.
Pipeline Network: Production.
a The global parameter c p is set equal to 20. Production cost of the players, who are net importers, is set equal to zero. The unit cost is given for flows up to 75% of the capacity. For the remaining 25% of capacity the numbers are increased by 20%.
b The Shah Deniz II field will increase Azerbaijan’s current production capacity by 16 bcm/a and serve Nabucco.
c Investment in Iran’s South Pars field will supply an additional 15 bcm/a to Nabucco.
d Northern Iraqi fields will produce an other 10 bcm/a to fill Nabucco’s large capacities.
e To reduce the number of players, Turkmenistan stands for Kazakhstan, Uzbekistan and itself.
The differences in the production cost of existing fields are small compared to differences in the cost of developing new fields. Since meaningful information on wellhead production cost is difficult to obtain, a common supply cost parameter c P is introduced. In accordance with Table 13.6 in IEA (2009) , Δ i accounts for regional differences in wellhead production cost and adjusts c P for each player. For the players, who are net importers, cost of using their indigenous production is ignored. Since it is more difficult to produce at maximal capacity k ij , production cost is assumed to be piecewise linear: T ij ( f )=( c P +Δ i )(min[ f , 0.75* k ij ]+1.2 max[ f –0.75* k ij , 0]). These adjustments help to get more realistic flows for the network, but have only a negligible impact on the estimate of bargaining power. Since the demand system is adjusted to any choice of c P , its absolute value is rather irrelevant and arbitrarily set as c P =20 mn €/bcm.
The total cost of transporting gas consists of, in principle, operating cost and capacity cost. Since capacity costs of existing pipelines are sunk, they are not taken into account. This simplification is based on the assumption that bargaining among rational players should not be influenced by sunk cost. The operating cost is composed by management and maintenance cost and energy cost, which are proportional to the length of the pipeline as well as to the quantity of gas transported. The operating cost is represented as a piecewise linear function: T i j ( f ) = c i j T ∗ ( min [ f , 0.75 ∗ k i j ] + 1.2 ∗ max [ f − 0.75 ∗ k i j , 0 ] ) , where k ij denotes maximal capacity. Per unit transportation costs are constant, but only up to three quarter of the pipe capacity and increased by 20% for the remaining quarter. Capacities of the pipelines linking the players’ transit nodes are collected from ENTSOG (2010) and public sources. Flows in 2009 are compiled from IEA (2010) and IEA (2011) . Capacities of the pipelines which are connected to areas outside of the regional scope are limited to flows through them in 2009. The pipeline capacities and the flows through them are presented in the first two columns of Tables 6 and 7 .
Pipeline Network A.
a The unit cost is given for flows up to 75% of the capacity. For the remaining 25% of capacity the numbers are increased by 20%.
b To reduce the number of players, Turkmenistan stands for Kazakhstan, Uzbekistan and itself.
Pipeline Network B.
To calculate the link specific cost parameter c i j T , for onshore pipelines universal operating cost of 0.3 mn €/bcm/100 km is assumed. For offshore pipelines operating cost is 50% higher to account for higher pressure and increased difficulties of maintenance. These coefficients are then multiplied with the distance between the nodes to obtain the link specific operating cost as shown in column 3 of Tables 6 and 7 .
Having specified the production cost by c P and Δ i , as well as the link specific transportation cost by c i j T , the only free parameter is the “typical” transport cost c ̅ T . To determine a value, the optimization program (1) is run for the grand coalition to find that c ̅ T =19 mn €/bcm yields a solution f i j ∗ which closely replicates the empirical data on consumption and flows in the system.
In the model the LNG gas is considered as nonstrategic since a single LNG exporter’s market share in the Eurasian gas trade is small relative to the market power of the suppliers of the pipeline gas. Incorporation of the global LNG market into a cooperative game would be challenging. Since the LNG gas is a common source so that actions of players outside of the considered coalition would have to be taken into account. They will form alternative coalitions which may tap the LNG market and change the availability of the LNG supplies. Since the focus of the paper is on pipeline gas, the LNG market is not modeled explicitly.
The LNG regasification plants, also called terminals, are represented as LNG links with capacities limited to flow in 2009. The LNG regasification capacities and imports through them are compiled from GIE (2010) , IEA (2010) and IEA (2011) . Comparing Tables 13.5 and 13.6 in IEA (2009) , the total cost (sum of production, liquefaction, transportation and regasification costs) of gas which is imported through the LNG terminals is assumed as 2 c P . Similar to the production and transportation costs, total cost of LNG is assumed to be piecewise linear: T ij ( f )=2 c P (min[ f , 0.75* k ij ]+1.2 max[ f –0.75* k ij , 0]). Figures for the LNG links are given in Table 8 .
Pipeline Network: LNG Regasification Plants.
a The global parameter c p is set equal to 20. The unit cost is given for flows up to 75% of the capacity. For the remaining 25% of capacity the numbers are increased by 20%.
New Projects
Information about the pipeline projects is obtained from various public sources. Cost estimates of the project consortia are supplemented by own estimates if figures are unavailable, outdated or subject to review. A rather high discount rate of 15% is used to translate capital expenditures into annualized capacity cost. This rate is a common hurdle rate in the gas industry and reflects the real option nature of the investment and depreciation. Table 9 collects the parameters for the new pipelines.
Pipeline Network: New Pipelines.
a Existing capacity as compiled from ENTSOG (2010) and public sources+planned capacity.
b Data are compiled from IEA (2010) and IEA (2011).
c Currently gas flows from Center-East to Balkan. The projects plan to revert the flow.
d This part of the project is referred to as Trans-Caspian.
e Currently gas flows from Balkan to Turkey. The project plans to revert the flow.
Bloch, F. and M. O. Jackson (2007) “The Formation of Networks with Transfers among Players,” Journal of Economic Theory, 133(1):83–110. [Network Theory] 10.1016/j.jet.2005.10.003 Search in Google Scholar
BP (2009) “Statistical Review of World Energy.” Published in June 2009. Available at http://www.bp.com/statisticalreview . Search in Google Scholar
BP (2010) “Statistical Review of World Energy.” Published in June 2010. Available at http://www.bp.com/statisticalreview . Search in Google Scholar
Brandenburger, A. M. and B. J. Nalebuff (1997) Co-Opetition . New York, New York, U.S.A: Doubleday Broadway Books. Search in Google Scholar
Bruce, C. (2005) Fraternal Friction Or Fraternal Fiction?: The Gas Factor in Russian-Belarusian Relations . Oxford, England: Oxford Institute for Energy Studies. Search in Google Scholar
Cobanli, O. (2014) “Central Asian Gas in Eurasian Power Game,” Energy Policy, 68:348–370. 10.1016/j.enpol.2013.12.027 Search in Google Scholar
Dixit, A. K. (1994) Investment under Uncertainty . Princeton, New Jersey, USA: Princeton University Press. 10.1515/9781400830176 Search in Google Scholar
EC (2007) Communication from the Commission to the Council and the European Parlemant: Priority Interconnection Plan. COM(2006) 846 final. Search in Google Scholar
EEGA (2010) Nord Stream: Russian land section nearly three times more expensive than German OPAL. Visited on 13 March 2014. Available at http://www.eegas.com/pipecost2010-05e.htm . Search in Google Scholar
Elliott, M. (2011) “Inefficiencies in Networked Markets.” Unpublished manuscript, California Institute of Technology . Search in Google Scholar
Energy Charter Secretariat (2007) Putting a Price on Energy – International Pricing Mechanisms for Oil and Gas. Technical report, Energy Charter Secretariat. Search in Google Scholar
ENTSOG (2010) Capacity Map Dataset in Excel Format. Version June 2010. Available at http://www.entsog.eu/mapsdata.html . Search in Google Scholar
EU (2006) “1364/2006/EC: Laying Down Guidelines for Trans-European Energy Networks and Repealing Decision 96/391/EC and Decision No 1229/2003/EC,” Official Journal of the European Union, L262(1). Search in Google Scholar
EU (2009) “Directive 2009/73/EC of the European Parliament and of the Council of 13 July 2009 Concerning Common Rules for the Internal Market in Natural Gas and Repealing Directive 2003/55/Ec,” Official Journal of the European Union, L211(94). Search in Google Scholar
EurActiv (2011) Oettinger zu Nabucco: Jahr der Entscheidung. Published on 20 March 2011. Available at http://www.euractiv.de/energie-und-klimaschutz/artikel/oettinger-nabucco-jahr-der-entscheidung-004571 . Search in Google Scholar
Evans, R. A. (1996) “Value, Consistency, and Random Coalition Formation,” Games and Economic Behavior, 12(1):68–80. 10.1006/game.1996.0005 Search in Google Scholar
GIE (2010) GLE Map Dataset in Excel-format. Version June 2010. Available at http://www.gie.eu/maps_data/lng.asp . Search in Google Scholar
Gul, F. (1989) “Bargaining Foundations of Shapley Value,” Econometrica: Journal of the Econometric Society, 57(1):81–95. 10.2307/1912573 Search in Google Scholar
Hirschman, A. O. (1969) National Power and the Structure of Foreign Trade . Berkeley, Los Angeles, USA: University of California Press. Search in Google Scholar
Hubert, F. and S. Ikonnikova (2011a) “Hold-Up and Strategic Investment in International Transport Networks: Gas Pipelines in North Western Europe.” Working paper. Available at http://www.ms-hns.de/files/publications/holdup_-_strategic_investment_-_gas.pdf . Search in Google Scholar
Hubert, F. and S. Ikonnikova (2011b) “Investment Options and Bargaining Power in the Eurasian Supply Chain for Natural Gas,” Journal of Industrial Economics, 59(1):85–116. 10.1111/j.1467-6451.2011.00447.x Search in Google Scholar
Hubert, F. and E. Orlova (2014a) “Competition or Countervailing Power for the European Gas Market.” Working paper. Available at http://www.ms-hns.de/files/publications/competition_countervailing.pdf . Search in Google Scholar
Hubert, F. and E. Orlova (2014b) “Network Access and Market Power.” Working paper. 10.1016/j.eneco.2018.09.020 Search in Google Scholar
Hubert, F. and I. Suleymanova (2008) “Strategic Investment in International Gas-Transport Systems: A Dynamic Analysis of the Hold-Up Problem.” Discussion papers: German Institute for Economic Research (DIW). 10.2139/ssrn.1429257 Search in Google Scholar
IEA (2009) World Energy Outlook 2009. Paris. Search in Google Scholar
IEA (2010) Gas Trade Flows in Europe. Viewed on 15 October 2013. Available at http://www.iea.org/gtf/index.asp . Search in Google Scholar
IEA (2011) Natural Gas Information 2011. Paris. Search in Google Scholar
Inderst, R. and C. Wey (2003) “Bargaining, Mergers, and Technology Choice in Bilaterally Oligopolistic Industries,” RAND Journal of Economics, 34(1):1–19. 10.2307/3087440 Search in Google Scholar
Jackson, M. O. (2010) Social and Economic Networks . Princeton, New Jersey, USA: Princeton University Press. 10.2307/j.ctvcm4gh1 Search in Google Scholar
Jackson, M. O. and A. Wolinsky (1996) “A Strategic Model of Social and Economic Networks,” Journal of Economic Theory, 71(1):44–74. 10.1007/978-3-540-24790-6_3 Search in Google Scholar
Jamestown Foundation (2006) Azerbaijan Spearheading Initiative on Trans-Caspian Gas Pipeline. Published on 30 March 2006. Available at http://www.jamestown.org/single/?no_cache=1&tx_ttnews%5Bttnews%5D=31531\#.UyDeG1FdUgE . Search in Google Scholar
Jamestown Foundation (2014) SCP, TANAP, TAP: Segments of the Southern Gas Corridor to Europe. Publisched on 15 January 2014. Available at http://www.jamestown.org/single/?tx_ttnews%5Bany_of_the_words%5D=Statoil&tx_ttnews%5Btt_news%5D=41821\\&tx_ttnews%5BbackPid\%5D=7&cHash=afc2067be4307e56147de91d55eb4da4\#.UyrId3VdWlh . Search in Google Scholar
Manea, M. (2011) “Bargaining in Stationary Networks,” The American Economic Review, 101(5):2042–2080. 10.1257/aer.101.5.2042 Search in Google Scholar
Maschler, M., B. Peleg and L. S. Shapley (1979) “Geometric Properties of the Kernel, Nucleolus, and Related Solution Concepts,” Mathematics of Operations Research, 4(4):303–338. 10.1287/moor.4.4.303 Search in Google Scholar
Myerson, R. B. (1980) “Conference Structures and Fair Allocation Rules,” International Journal of Game Theory, 9(3):169–182. 10.1007/BF01781371 Search in Google Scholar
Natural Gas Europe (2011) Nabucco Cost Speculation Rises. Published on 24 October 2011. Available at http://www.naturalgaseurope.com/nabucco-costs-speculation-rises-3172 . Search in Google Scholar
Natural Gas Europe (2013) Gazprom Raises Full Cost South Stream Project to over 56 billion. Published on 10 December 2013. Available at http://www.naturalgaseurope.com/gazprom-raises-full-cost-south-stream-over-56-billion . Search in Google Scholar
Netherlands Ministry of Economic Affairs, Agriculture and Innovation (2010) Economic impact of the Dutch gas hub strategy on Netherlands. Available at http://www.rijksoverheid.nl/bestanden/documenten-en-publicaties/rapporten/2010/12/08/dutch-gas-hub-strategy-on-the-netherlands/10183259-bijlage.pdf . Search in Google Scholar
New York Times (2011) European Pipeline Project Faces Formidable Obstacles. Published on 7 March 2011. Available at http://www.nytimes.com/2011/03/08/business/global/08nabucco.html?pagewanted=all&_r=0 . Search in Google Scholar
Nord Stream (2013) Fact Sheet: Nord Stream by Numbers. Visited on 13 March 2014. Available at https://www.nord-stream.com/download/document/177/?language=en . Search in Google Scholar
Pirani, S., J. P. Stern and K. Yafimava (2009) The Russo-Ukrainian Gas Dispute of January 2009: A Comprehensive Assessment . Oxford: Oxford Institute for Energy Studies. 10.26889/9781901795851 Search in Google Scholar
Potters, J. A., J. H. Reijnierse and M. Ansing (1996) “Computing the Nucleolus by Solving a Prolonged Simplex Algorithm,” Mathematics of Operations Research, 21(3):757–768. 10.1287/moor.21.3.757 Search in Google Scholar
Reuters (2013a) BP to buy Stake in Azeri Gas Pipeline Project TANAP. Published on 6 December 2013. Available at http://uk.reuters.com/article/2013/12/06/turkey-gas-tanap-idUKL5N0JL2TP20131206 . Search in Google Scholar
Reuters (2013b) Gazprom sees South Stream costing $39 bln. Published on 29 January 2013. Available at http://uk.reuters.com/article/2013/01/29/gazprom-southstream-idUKL5N0AYAW620130129 . Search in Google Scholar
Reuters (2013c) Gazprom’s Domestic Costs for South Stream Pipeline rise by 45 pct. Published on 9 December 2013. Available at http://uk.reuters.com/article/2013/12/09/energy-gazprom-southstream-idUKL6N0JO2YX20131209 . Search in Google Scholar
Rianovosti (2009) Gazprom Signs Deals with Transit States to spur South Stream. Published on 15 May 2009. Available at http://en.ria.ru/russia/20090515/155027053.html . Search in Google Scholar
Schmeidler, D. (1969) “The Nucleolus of a Characteristic Function Game,” SIAM Journal on Applied Mathematics, 17(6):1163–1170. 10.1137/0117107 Search in Google Scholar
Shapley, L. (1953) “A Value for n-Person Games,” Annals of Mathematics Study, 28:307–317. 10.1515/9781400881970-018 Search in Google Scholar
Smeers, Y. (2008) Gas Models and Three Difficult Objectives. Discussion Paper: ECORE. Available at http://www.ecore.be/DPs/dp_1203678243.pdf . Search in Google Scholar
South Stream (2010) South Stream is Estimated to Cost EUR 15.5 billion. Visited on 30 November 2010. Available at http://south-stream.info/index.php?id=70L=1 . Search in Google Scholar
Stern, J. P. (2005) The Future of Russian gas and Gazprom . Oxford, England: Oxford University Press. Search in Google Scholar
Stole, L. A. and J. Zwiebel (1996a) “Intra-Firm Bargaining under Non-Binding Contracts,” The Review of Economic Studies, 63(3):375–410. 10.2307/2297888 Search in Google Scholar
Stole, L. A. and J. Zwiebel (1996b) “Organizational Design and Technology Choice under Intrafirm Bargaining,” The American Economic Review, 86(1):195–222. 10.1257/000282803321455421 Search in Google Scholar
TAP (2013) Trans Adriatic Pipeline. Visited on 30 July 2013. Available at http://www.trans-adriatic-pipeline.com/index.php . Search in Google Scholar
Young, H. P. (1985a) “Monotonic Solutions of Cooperative Games,” International Journal of Game Theory, 14(2):65–72. 10.1007/BF01769885 Search in Google Scholar
Young, H. P. (1985b) “Producer Incentives in Cost Allocation,” Econometrica: Journal of the Econometric Society, 53(4):757–765. 10.2307/1912652 Search in Google Scholar
©2016 Walter de Gruyter GmbH, Berlin/Boston
- X / Twitter
Supplementary Materials
Please login or register with De Gruyter to order this product.
Journal and Issue
Articles in the same issue.

An official website of the United States government
Here’s how you know
Official websites use .gov A .gov website belongs to an official government organization in the United States.
Secure .gov websites use HTTPS A lock ( Lock A locked padlock ) or https:// means you’ve safely connected to the .gov website. Share sensitive information only on official, secure websites.
Free Cyber Services #protect2024 Secure Our World Shields Up Report A Cyber Issue
The Attack on Colonial Pipeline: What We’ve Learned & What We’ve Done Over the Past Two Years
Jen Easterly, CISA Director
Tom Fanning, Chairman and CEO of Southern Company and Chair of CISA’s Cybersecurity Advisory Committee
Today marks two years since a watershed moment in the short but turbulent history of cybersecurity. On May 7, 2021, a ransomware attack on Colonial Pipeline captured headlines around the world with pictures of snaking lines of cars at gas stations across the eastern seaboard and panicked Americans filling bags with fuel, fearful of not being able to get to work or get their kids to school. This was the moment when the vulnerability of our highly connected society became a nationwide reality and a kitchen table issue.
The good news is that since that event, the Biden-Harris Administration has made significant strides in our collective cyber defense, harnessing the full power of the U.S. government to address the full spectrum of the threat. At the Cybersecurity and Infrastructure Security Agency (CISA), we have been laser focused on improving resilience across our Nation’s critical infrastructure. Recognizing that organizations need a simple way to access actionable and timely cybersecurity information, we developed stopransomware.gov to provide a central location for alerts and guidance for businesses and individuals. Recognizing that only cohesive collaboration across government will scale to meet the threat, we launched the Joint Ransomware Task Force with our FBI partners to orchestrate the federal government’s response to the epidemic of ransomware. And recognizing the need to bring together industry, government, and internal partners and tear down siloes that create gaps for the adversary, we established the Joint Cyber Defense Collaborative (JCDC) —a concept born out of the U.S. Cyberspace Solarium Commission on which one of us served as a Commissioner—to catalyze a community of experts on the front lines of cyber defense—from across the public and private sectors—to share insights and information in real time to understand threats and drive down risk to the nation.
Since its establishment, the JCDC led the national response to one of the most extensive software vulnerabilities discovered; played a central role in CISA’s Shields Up campaign to protect critical infrastructure from potential Russian cyber-attacks; and, along with our partners at the Transportation Security Administration (TSA), brought together more than 25 major pipeline operators and industrial control systems partners to strengthen security practices to safeguard the operational technology networks critical to pipeline operations, efforts that complement the Security Directives TSA issued in the aftermath of the attack on Colonial Pipeline. Separately, with the support of Congress, we expanded our capability known as “CyberSentry” which enables heightened visibility into and more rapid detection of cyber threats that could target our nation’s most critical operational technology networks. Finally, we worked to help organizations of all sizes and skill levels prioritize the most impactful cybersecurity investments with the introduction of cybersecurity performance goals, or CPGs .
While we should welcome this progress, much work remains to ensure the security and resilience of our critical infrastructure in light of complex threats and increasing geopolitical tension. The U.S. Intelligence Community issued a stark warning of a potential future in its recent Annual Assessment , noting that “If Beijing feared that a major conflict with the United States were imminent, it almost certainly would consider undertaking aggressive cyber operations against U.S. homeland critical infrastructure…China almost certainly is capable of launching cyber-attacks that could disrupt critical infrastructure services within the United States, including against oil and gas pipelines, and rail systems.”
We cannot afford to dismiss this warning. We must do everything today to be prepared for such a scenario. First, we must ensure that the technology that underpins the services that Americans rely on every hour of every day is safe and secure . For too long, we have sacrificed security for features and speed to market, leaving us increasingly vulnerable, with the burden of security placed on those least able to bear it. As listed in one of the core pillars in the President’s National Cyber Strategy we need security to be built into the creation of new technology—as a foundational imperative—rather than bolted on at the end requiring continuous security updates from consumers.
Second, we need to prioritize cybersecurity at the highest levels. The days of relegating cybersecurity to the CIO or the CISO must end. CEOs and Boards of Directors must embrace cyber risk as a matter of good governance and prioritize cybersecurity as a strategic imperative and business enabler.
Third, we must continue to invest in the JCDC model of persistent and proactive operational collaboration between government and industry where the default is to share information on malicious cyber activity, knowing that a threat to one is a threat to all.
Finally, we need to normalize cyber risks for the general public with the recognition that cyber-attacks are a reality for the foreseeable future. We cannot completely prevent attacks from happening, but we can minimize their impact by building resilience into our infrastructure and into our society. We need to look no further than our Ukrainian partners for an example of the power of societal resilience.
These changes are not easy, but we need to hold ourselves accountable to the hard lessons learned from two years ago. Are we going to make the choices that will lead us to a secure, resilient, and prosperous future or are we going to allow inaction to dictate a future in which our national security and our way of life hang in the balance? We have proven that it can be done but only if we act now…together.
Related Articles
Secure by design turns 1, resilient together with priority telecommunications services (pts), ncswic releases roles and responsibilities: statewide interoperability coordinators document, safecom publishes safecom governance operating processes and procedures (march 2024 update).
Paper Information
- Paper Submission
Journal Information
- About This Journal
- Editorial Board
- Current Issue
- Author Guidelines
International Journal of Energy Engineering
p-ISSN: 2163-1891 e-ISSN: 2163-1905
2015; 5(2): 17-24
doi:10.5923/j.ijee.20150502.01
Gas Pipeline Network Design for Koya City: Case Study
Jafar A. Ali , Hayder H. Abbas , Loghman Khodakarami
Faculty of Engineering, Koya University, Koya, Iraq
Copyright © 2015 Scientific & Academic Publishing. All Rights Reserved.
The first worldwide concern is energy security. Supplying a clean energy in efficient way is still an important topic for many researchers. This paper investigates designing of gas distribution network as a safer, cleaner and convenient energy option for the city of Koya in Kurdistan Region, taking Gashtiari neighborhood as case study. The old traditional ways to supply gas for domestics’ consumers in Koya city is by cylinders and road trucks have many disadvantages; this is due to road traffics and accidents and explodes of gas cylinders as well. The network linked 162 houses via 3500m pipes with different diameters. The source of the gas will be one of the local oil fields locates 18 Km outside the city. The designed results show that every single house in the region is consuming 300m 3 /month as average in cold weather (November – March), this will reduce to 100m 3 /month in hot weather (April – October). Also the conservation in energy equal to (442-663) MWh/day in case if using the designed network. Detailed drawing of the network and route map is provided using Arc GIS. The aim of the study is to deliver clean energy for transport, residential, industrial and commercial needs. This will reduce the demand for electricity; in addition the risk of pollutions will be decreased.
Keywords: Pipeline, Gas network, Energy, GIS, Koya city
Cite this paper: Jafar A. Ali , Hayder H. Abbas , Loghman Khodakarami , Gas Pipeline Network Design for Koya City: Case Study, International Journal of Energy Engineering , Vol. 5 No. 2, 2015, pp. 17-24. doi: 10.5923/j.ijee.20150502.01.
Article Outline
1. introduction, 2. koya city energy consumption, 3. main gas pipeline, 4. detailed design drawing, 5. cost and revenue, 6. result and discussion, 7. conclusions.
PIPELINE OPEN KNOWLEDGE BASE
19 th Pipeline Technology Conference 8-11 April 2024, BERLIN
Proceedings Publication Date 02 May 2017

To view the video or download the paper please register here for free
You already have access? Sign in now.
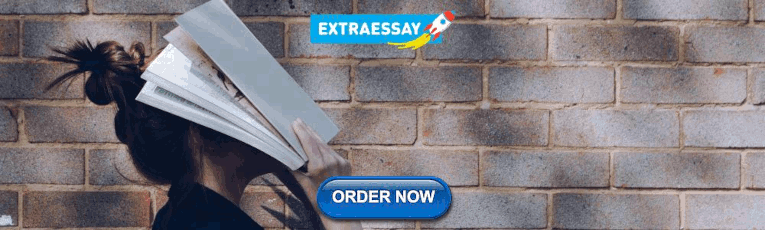
COMMENTS
Natural gas pipeline network case studies. Gas transmission pipeline networks are used to move massive amounts of high-pressure sales gas from processing plants to distribution systems and large industrial users (Mokhatab and Poe, 2012). In this study, we mainly consider uncertainties that arise from two sources. One source is the uncertainty ...
Abstract. This paper presents a group maintenance scheduling case study for a water distribution network. This water pipeline network presents the challenge of maintaining aging pipelines with the associated increases in annual maintenance costs. The case study focuses on developing an effective pipeline replacement planning for the water utility.
A case study is conducted on a real municipal gas pipeline network distributed in an urban area with 4 km × 2 km around covering more than 6500 gas pipelines. The traditional model and the proposed data-driven model are employed to evaluate the post-event risk of the pipeline network.
A Case Study for a Pipeline Network F. Li, L. Ma, Y. Sun and J. Mathew Abstract This paper presents a group maintenance scheduling case study for a water distribution network. This water pipeline network presents the challenge of maintaining aging pipelines with the associated increases in annual maintenance costs.
A case study is carried out using PLS by considering a . ... (Boye et al., 2017). Pipeline network is made up of collection of many components such as pipes and other flow control devices ...
case study. Levelized cost of CO 2 pipelines calculated for the region range from 0.14 to 0.75 GBP per tonne of CO 2. This is a preliminary study and is useful for obtaining quick techno-economic assessment of CO 2 pipelines. Keywords: Case Study, CO 2 transport, Pipeline, Network Design, Techno-economic analysis
Abstract. In China, annual natural gas consumption is over 67 billion cubic meters with an expected growth rate of 10% per year. Most of the gas is transported from well heads to markets over cross-country gas networks, which requires construction of the West to East Gas Network--one of the largest gas networks in the world. Presently, the network is comprised of four large-diameter pipelines ...
The first step in the pipeline network generation process is the clustering of nodes currently being considered in the study area. The study area has several nodes, which are in the immediate vicinity of each other. ... Effect of terrain, environment and infrastructure on potential CO 2 pipeline corridors: a case-study from North-Central USA.
In this paper, various issues to be considered in CO 2 pipeline design for carbon capture and storage applications are discussed. Also, different techno-economic correlations for CO 2 pipelines are assessed using the Humber region as a case study. Levelized cost of CO 2 pipelines calculated for the region range from 0.14 to 0.75 GBP per tonne ...
A case study on a real gas pipeline network consisting of more than 6500 pipelines verifies the effectiveness of the proposed model. Introduction. Urban lifelines which include power, gas, water supply sewerage and other systems play important roles in maintaining city function. As a complex network distributed in the cities, the lifeline ...
The operating pressure of the pipeline network varies from 3.5 to 22.75 MPa, as depicted in Fig. 6 b. Additionally, ... As a case study, the BBN model was used to calculate the failure probability for Alberta's oil and gas transmission pipelines, with the final results indicating that more pipe segments can fail due to burst rather than leak ...
This paper shows a case study where system-level damage potentials for the City of Los Angeles water pipeline network were assessed using a stochastic method. The study considers both the distribution of earthquake-induced shaking and ground deformations, and the locations of the pipe network within the areas of varying shaking and ground ...
Request PDF | Optimization of Large Gas Pipeline Network--A Case Study in China | In China, annual natural gas consumption is over 67 billion cubic meters with an expected growth rate of 10% per year.
This work presents the conceptual design of a gas distribution pipeline network for estates in Nigeria using the University of Abuja Staff Quarters as a case study. The problem statement was the aggressive consumption of cooking gas, referred to as liquefied petroleum gas (LPG), without gas pipeline networks infrastructures to homes and estates ...
study investigates the process of building pipelines network to distribute gas for Koya city residents. The gas source will be one of the local oil field and transmit to the city via pipeline. The need for an optimum route is more important as this can reduce a huge operational cost. Gas storage system is needed for peaks shaving in gas
Building an additional pipeline to bring WEPII's gas to Hong Kong by the end of 2012, however, would be no easy task and would require top-notch project management. A HIGHLY COMPLEX PROJECT Connecting the WEPII network from mainland China to Hong Kong presented numerous, complex challenges to all involved.
Presently, the network is comprised of four large-diameter pipelines and will include most major gas pipelines in China in the future. The network distributes approximately 30 x 10 9 m 3 gas per year, of which 3% to 5% is burned to power the gas transportation. At current gas prices, gas transportation costs are roughly USD 350 million per year ...
A natural gas flow network consists of city gate station, district regulator, pipelines, compressors and valves. The performance of such a complex system cannot be studied by analysing individual constituents. Simultaneous performance of integrated system can be expressed using non-linear equations to simulate and forecast operational behaviour. The operational behaviour is subject to various ...
This paper reports on an extended-period simulation for predictions of the flows and pressures of a municipal water supply system. The program has been developed within the scope of the Real-Time Intelligent Systems Project at the University of Regina, Saskatchewan, Canada. The aim of this project is to develop a real-time decision support system for monitoring and control of the water ...
transmission network is highly vulnerable to demand fluctuations. Also, results indicate that employing both pipeline fortification and back-up pipeline strategies have numerous impacts on the resiliency of the NG network. Important managerial insights are obtained from the model implementation in a case study. Keywords: Natural Gas Transmission
We analyze the impact of three controversial pipeline projects on the power structure in the Eurasian network for natural gas. Two pipelines, "Nord Stream" and "South Stream," allow Russian gas to bypass transit countries, Ukraine and Belarus. The third project, "Nabucco," aims at diversifying Europe's gas imports by accessing producers in Middle East and Central Asia.
Today marks two years since a watershed moment in the short but turbulent history of cybersecurity. On May 7, 2021, a ransomware attack on Colonial Pipeline captured headlines around the world with pictures of snaking lines of cars at gas stations across the eastern seaboard and panicked Americans filling bags with fuel, fearful of not being able to get to work or get their kids to school.
From the survey results show 82% using EWHS, and 95% using EH and kerosene, the total number of householders in Koya is 12489. Based on 10 hours working, the range of energy consumption in the city is (442 - 663) MW.h/day. Therefore, using the gas pipeline in the city is something can't be over locked. 3.
The third case study is related to decommissioning a subsea pipeline in the Gulf of Mexico. The paper concludes with considerations that offshore operators need to focus on and how inline isolation technology can assist in platform decommissioning and pipeline network reconfiguration.
This is supported by a case study on implementing reliable monitoring of oil and gas pipeline installations. Network parameters like node-discovery, node-mobility, data security, link connectivity, data aggregation, information knowledge discovery and quality of service provisioning have been reviewed. ... "Network Challenges for Cyber Physical ...