
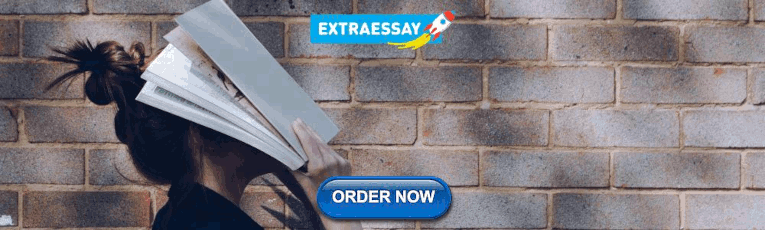
Wolfram|Alpha Widgets Overview Tour Gallery Sign In
Share this page.
- StumbleUpon
- Google Buzz
Output Type
Output width, output height.
To embed this widget in a post, install the Wolfram|Alpha Widget Shortcode Plugin and copy and paste the shortcode above into the HTML source.
To embed a widget in your blog's sidebar, install the Wolfram|Alpha Widget Sidebar Plugin , and copy and paste the Widget ID below into the "id" field:
Save to My Widgets
Build a new widget.
We appreciate your interest in Wolfram|Alpha and will be in touch soon.

Index Assignment problem Hungarian algorithm Solve online
Solver Title

Generating PDF...
- Pre Algebra Order of Operations Factors & Primes Fractions Long Arithmetic Decimals Exponents & Radicals Ratios & Proportions Percent Modulo Number Line Mean, Median & Mode
- Algebra Equations Inequalities System of Equations System of Inequalities Basic Operations Algebraic Properties Partial Fractions Polynomials Rational Expressions Sequences Power Sums Interval Notation Pi (Product) Notation Induction Logical Sets Word Problems
- Pre Calculus Equations Inequalities Scientific Calculator Scientific Notation Arithmetics Complex Numbers Polar/Cartesian Simultaneous Equations System of Inequalities Polynomials Rationales Functions Arithmetic & Comp. Coordinate Geometry Plane Geometry Solid Geometry Conic Sections Trigonometry
- Calculus Derivatives Derivative Applications Limits Integrals Integral Applications Integral Approximation Series ODE Multivariable Calculus Laplace Transform Taylor/Maclaurin Series Fourier Series Fourier Transform
- Functions Line Equations Functions Arithmetic & Comp. Conic Sections Transformation
- Linear Algebra Matrices Vectors
- Trigonometry Identities Proving Identities Trig Equations Trig Inequalities Evaluate Functions Simplify
- Statistics Mean Geometric Mean Quadratic Mean Average Median Mode Order Minimum Maximum Probability Mid-Range Range Standard Deviation Variance Lower Quartile Upper Quartile Interquartile Range Midhinge Standard Normal Distribution
- Physics Mechanics
- Chemistry Chemical Reactions Chemical Properties
- Finance Simple Interest Compound Interest Present Value Future Value
- Economics Point of Diminishing Return
- Conversions Roman Numerals Radical to Exponent Exponent to Radical To Fraction To Decimal To Mixed Number To Improper Fraction Radians to Degrees Degrees to Radians Hexadecimal Scientific Notation Distance Weight Time Volume
- Pre Algebra
- Pre Calculus
- Linear Algebra
- Trigonometry
- Conversions

Most Used Actions
Number line.
- x^{2}-x-6=0
- -x+3\gt 2x+1
- line\:(1,\:2),\:(3,\:1)
- prove\:\tan^2(x)-\sin^2(x)=\tan^2(x)\sin^2(x)
- \frac{d}{dx}(\frac{3x+9}{2-x})
- (\sin^2(\theta))'
- \lim _{x\to 0}(x\ln (x))
- \int e^x\cos (x)dx
- \int_{0}^{\pi}\sin(x)dx
- \sum_{n=0}^{\infty}\frac{3}{2^n}
step-by-step
linear programming calculator
- Practice, practice, practice Math can be an intimidating subject. Each new topic we learn has symbols and problems we have never seen. The unknowing...
Please add a message.
Message received. Thanks for the feedback.
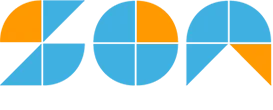
- Mathematicians
- Math Lessons
- Square Roots
- Math Calculators
Linear Programming Calculator + Online Solver With Free Steps
JUMP TO TOPIC
What Is the Linear Programming Calculator?
What is linear programming (lp), representing linear programs.
This online calculator solves the problem of finding the correct solution or optimized output of the desired mathematical models by providing a quick, reliable, and accurate solution.
It just requires the user to enter the objective function along with the system of linear constraints and the solution will be on their screens just in a matter of seconds. The Linear Programming Calculator is the most efficient tool for linear optimization and can be used to solve complex and time-consuming problems and models effectively and logically.
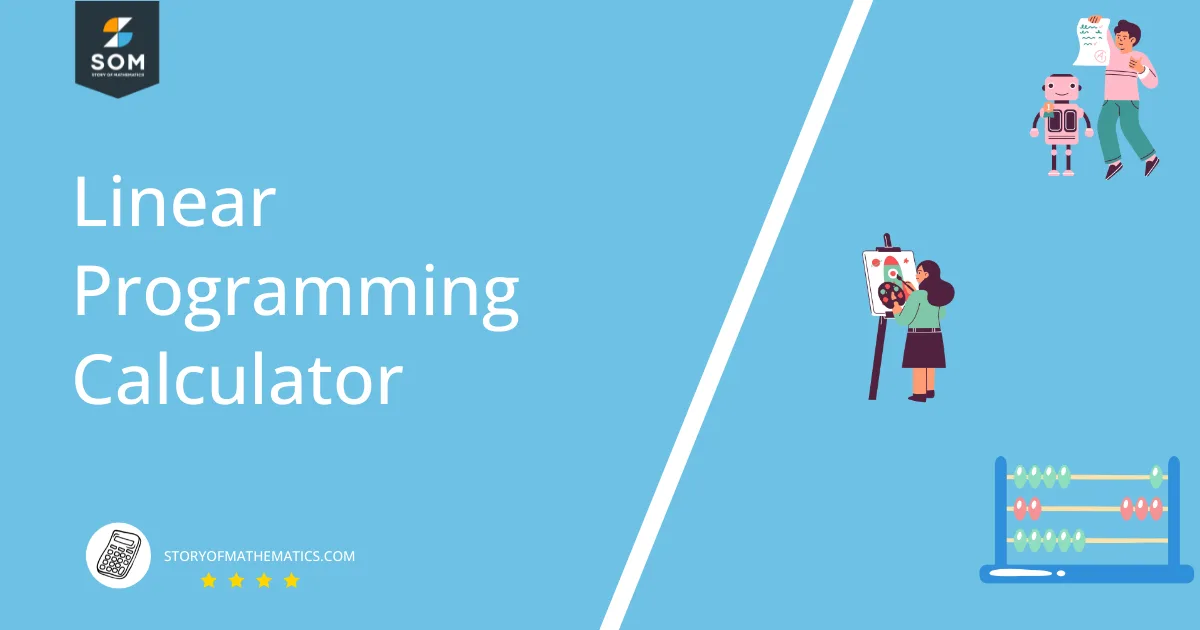
The Linear Programming Calculator is an online calculator that can be used for the linear optimization of various mathematical models.
It is a convenient and user-friendly tool with an easy-to-use interface that helps the user to find the exact and optimized solution for the provided constraints faster than any other mathematical technique applied manually.
The Linear Programming Calculator helps the user to avoid the long mathematical calculations and get the desired answer just by clicking one button.
The calculator can solve problems containing a maximum of nine different variables not more than that. It requires “ , ” as a separator for multiple constraints in a single box.
Letâs find out more about the calculator and how it works.
How To Use a Linear Programming Calculator?
You can use the Linear Programming Calculator by entering the objective function and specifying the constraints. Once you are done entering all the inputs you just have to press the submit button and a detailed solution will be displayed on the screen just in seconds.
Following are the detailed stepwise guidelines to find out the best possible solution for the given objective function with specified constraints. Follow these simple steps and find out the maxima and minima of the functions.
Consider your desired objective function and specify its constraints.
Now, enter the objective function in the tab specified as Objective Function .
After adding the objective function, input the conditions of all constraints in the tab named Subject . The calculator can take a maximum of nine constraints and has more tabs for it under the name More Constraints . To add multiple constraints in a single block, you have to use â,â as a separator.
Once you are done filling all the input fields, select the optimization category from the Optimize drop-down menu. There are three options you can select to find the maxima of the objective function, minima of the objective function or you can select both.
The options in the drop-down menu are given as:
After that, press the Submit button and the optimal solution along with graphs will be displayed in the result window.
Make sure not to add more than nine constraints in the calculator, otherwise it will fail to produce the desired results.
You can view the result window below the calculator layout. The Result window contains the following blocks:
Input Interpretation
This block shows the input entered by the user and how it has been interpreted by the calculator. This block helps the user to figure out if there were any mistakes in the input data.
Global Maximum
This block shows the calculated global maxima of the given objective function. Global maxima are the overall largest value of the objective function.
Global Minimum
This block displays the global minima of the given objective function. Global minima are the overall smallest value of the given function with the specified constraints.Â
This block displays the 3D interpretation of the objective function. It also specifies the maxima and minima points on the 3D plot.
Contour Plot
The contour plot is a 2D representation of the global maxima and global minima of the objective function on the graph.
How Does the Linear Programming Calculator Work?
The Linear Programming Calculator works by computing the best optimal solution of the objective function using the technique of Linear programming, which is also called Linear optimization.
Mathematical optimization is the technique used to find the best possible solution to a mathematical model such as finding the maximum profit or analyzing the size of the cost of a project, etc. It is the type of linear programming that helps to optimize the linear function provided that given constraints are valid.
To understand more about the working of the Linear Programming Calculator , letâs discuss some of the important concepts involved.
Linear Programming is the mathematical programming technique that tends to follow the best optimal solution of a mathematical model under specified conditions which are called constraints. It takes various inequalities applied to a certain mathematical model and finds the optimum solution.
Linear Programming is only subjected to linear equality and inequality constraints. It is only applicable to linear functions that are the first-order functions. The linear function is usually represented by a straight line and the standard form is y = ax + b.
In linear programming , there are three components: decision variables, objective function, and constraints. The usual form of a linear program is given as follows:
The first step is to specify the decision variable that is an unknown element in the problem.
decision variable = xÂ
Then, decide whether the optimization required is the maximum value or the minimum value.
The next step is to write the objective function that can be maximized or minimized. The objective function can be defined as:
\[ X \to C^T \times X \]
Where C is the vector.
Finally, you need to describe the constraints that can be in the form of equalities or inequalities and they must be specified for the given decision variables.
The constraints for the objective function can be defined as:
\[ AX \leq B \]
\[ X \geq 0 \]
Where A and B are the vectors. Therefore, linear programming is an effective technique for the optimization of various mathematical models.
Thus, the Linear Programming Calculator uses the linear programming process to solve the problems in seconds.
Due to its effectiveness, it can be utilized in various fields of study. Mathematicians and businessmen use it widely, and it is a very useful tool for engineers to help them solve complex mathematical models that are formed for various designing, planning, and programming purposes.
A linear program can be represented in various forms. First, it requires the identification of the maximization or minimization of the objective function and then the constraints. The constraints can be either in the form of inequalities $( \leq , \geq )$ or equality ( = ).
A linear program can have decision variables represented as $ x_1, x_2, x_3, âŠâŠ.., x_n $.
Therefore, the general form of a Linear Program is given as:
Minimize or Maximize:
\[ y = c_o + c_1x_1 + c_2x_2 + âŠ. + c_nx_n \]
Subject to:
\[ a_1i x_1+ a_2ix_2 + a_3ix_3 +……. + a_nix_n = b_i \]
\[ a_1ix_1 + a_2ix_2 + a_3ix_3 +……. + a_nix_n \leq b_i \]
\[ a_1ix_1+ a_2ix_1 + a_3ix_2 +……. + a_nix_n \geq b_i \]
Where i = 1,2,3,……..,m.Â
\[ x_k \geq 0 \]
\[ x_k < 0 \]
\[ x_k > 0 \]
Where k = 1,2,3,……..,m.Â
Here $x_k$ is the decision variable and $a_in$, $b_i$, and $c_i$ are the coefficients of objective function.
Solved Examples
Letâs discuss some examples of linear optimization of the mathematical problems using the Linear Programming Calculator.
Maximize and minimize the objective function given as:
\[ 50x_1 + 40x_2 \]
The constraints for the above-mentioned objective function are given as:
\[3x_1 + 1x_2 <= 2700 \]
\[ 6x_1 + 4x_2 >= 600 \]
\[ 5x_1 + 5x_2 = 600 \]
\[ x_1 \geq 0 \]
\[ x_2 \geq 0 \]
Use the calculator to optimize the given function.
Follow the steps mentioned below:
Select the max/min option from the Optimize drop-down menu.
Input the objective function and the functional constraints in the specified blocks.
Now click the submit button to view the results.
The Global maximum of the function is given as:
\[ max( 50x_1 + 40x_2 )_{at ( x_1, x_2 )} = (120, 0 ) \]
The Global minimum of the function is given as:
\[ min ( 50x_1 + 40x_2 )_{at ( x_1, x_2 )} = (60, 60 ) \]
The 3D plot is shown in Figure 1:
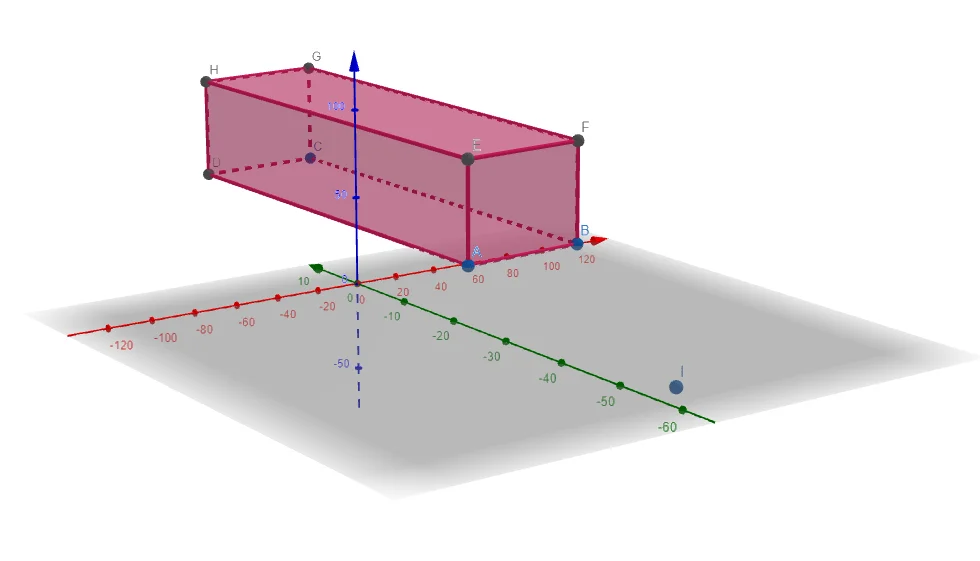
The contour plot is given in Figure 2 below:
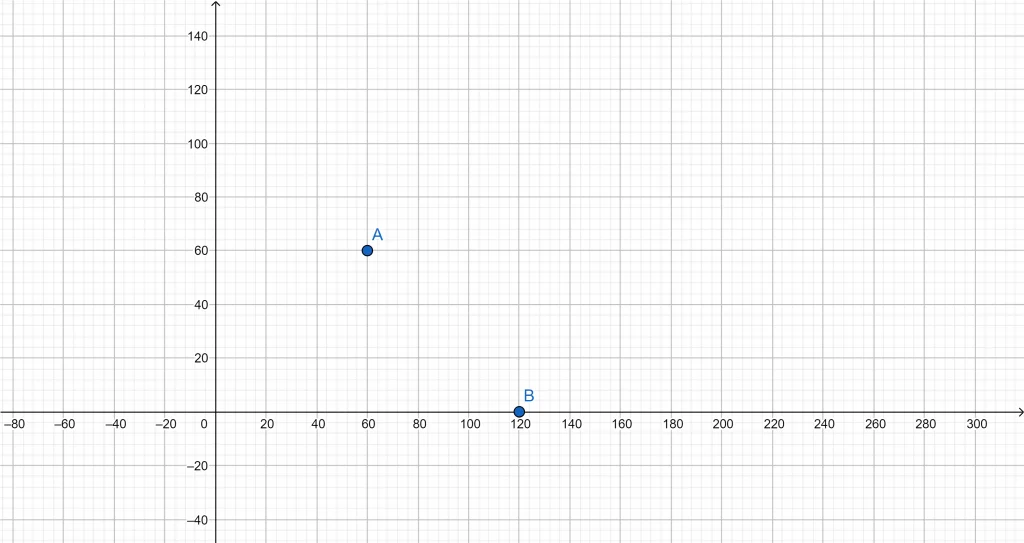
A diet plan chalked by the dietitian contains three types of nutrients from two types of food categories. The nutritional contents under study include proteins, vitamins, and starch. Let the two food categories be $x_1$ and $x_2$.
A specific amount of each nutrient must be consumed each day. The nutritional content of proteins, vitamins, and starch in food $x_1$ is 2, 5, and 7, respectively. For food category $x_2$ the nutritional contents of proteins, vitamins, and starch are 3,6, and, 8, respectively.
The requirement per day of each nutrient is 8, 15, and 7, respectively.
The cost of each category is $2$ per $kg$. Determine the objective function and constraints to find out how much food must be consumed per day to minimize the cost.
The decision variable are $x_1$ and $x_2$.
The objective function is given as:
\[ y = 2x_1 + 2x_2 \]
The various constraints for the given objective function analyzed from the data given above are:
\[ 2x_1 + 3x_2 > 8 \]
\[ 5x_1 + 6x_2 > 15 \]
\[ 7x_1 + 8x_2 > 7 \]
All the constraints are non-negative as the amount of food cannot be negative.
Input all the data in the calculator and press the submit button.
Following results are obtained:
Local Minimum
\[ min( 2x_1 + 2x_2 ) = (0, 2.67)
The 3D representation is shown in figure 3 below:
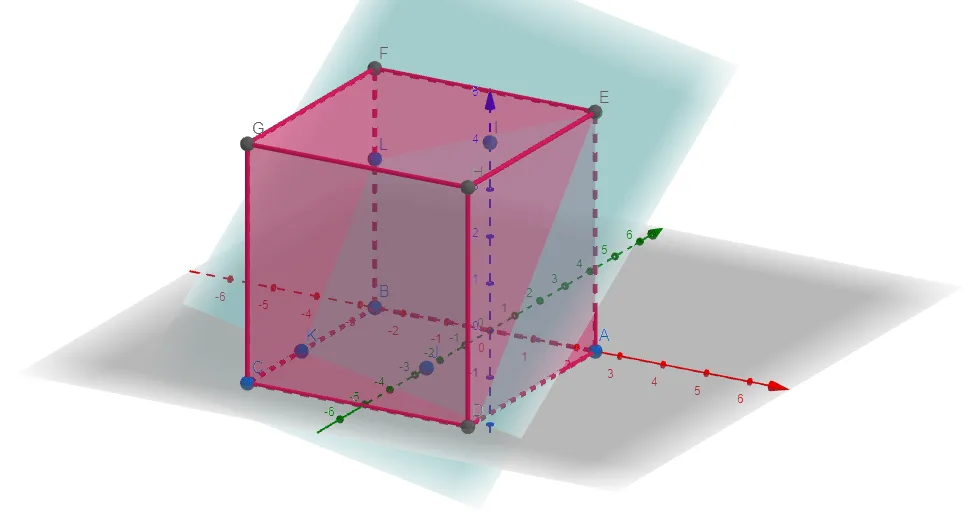
The contour plot is shown in Figure 4:
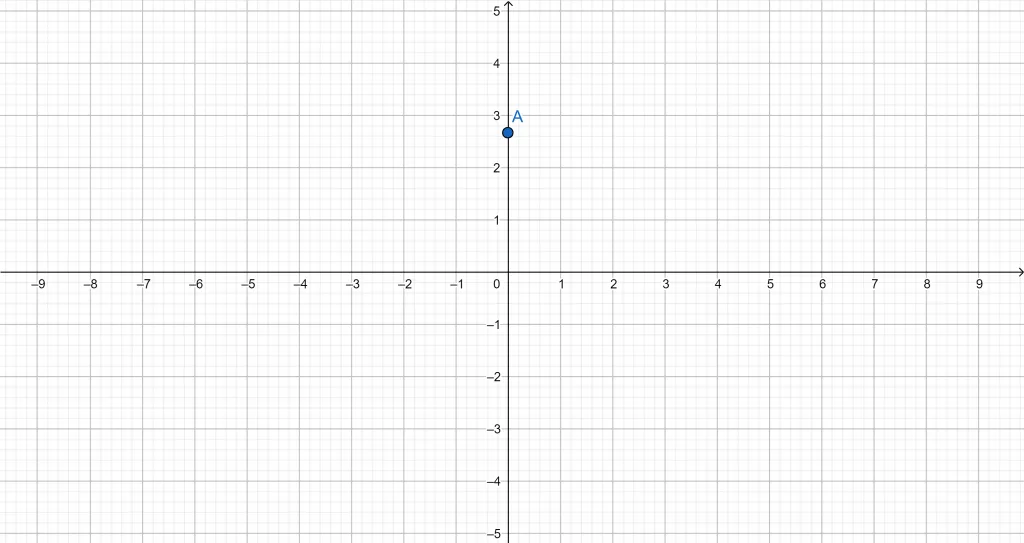
All the Mathematical Images/Graphs are created using GeoGebra.
Triple Integral Calculator < Math Calculators List > Dimensional Analysis Calculator
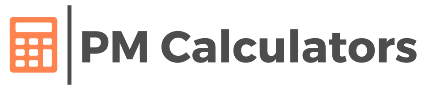
Linear Programming Calculators
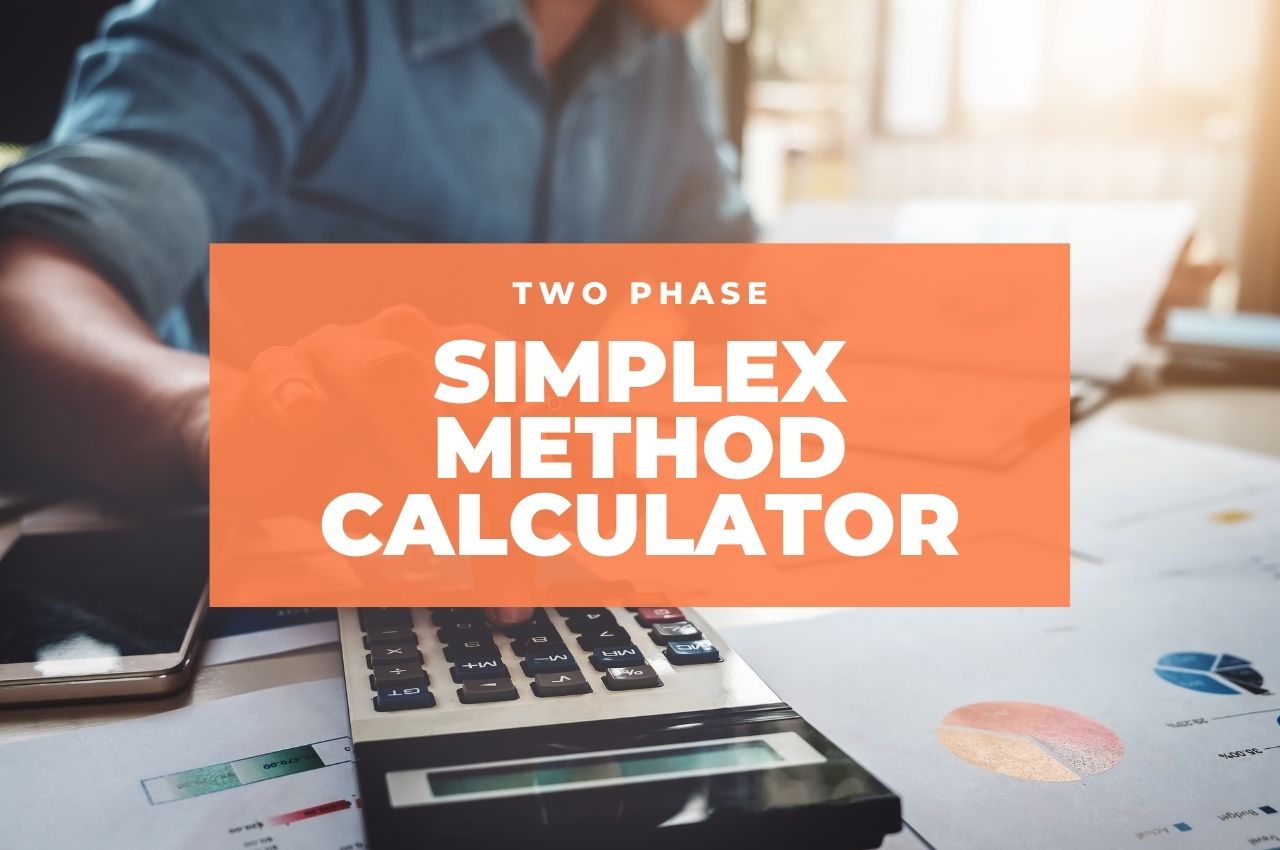
Simplex Method Calculator – Two Phase Online đ„
Find the optimal solution step by step to linear programming problems with our simplex method online calculator. â
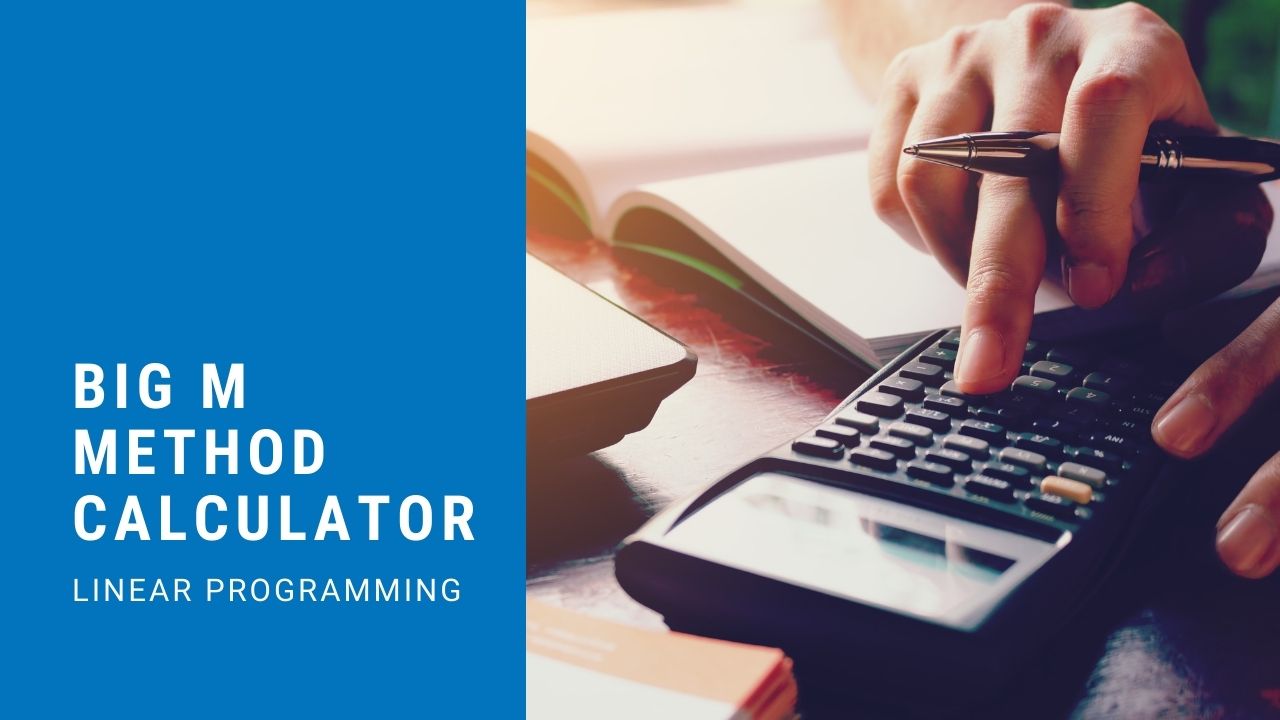
Big M Method Calculator Online – Linear Programming đ„
Solve your linear programming exercises with the big M method calculator online automatically and easily with our online calculator. â
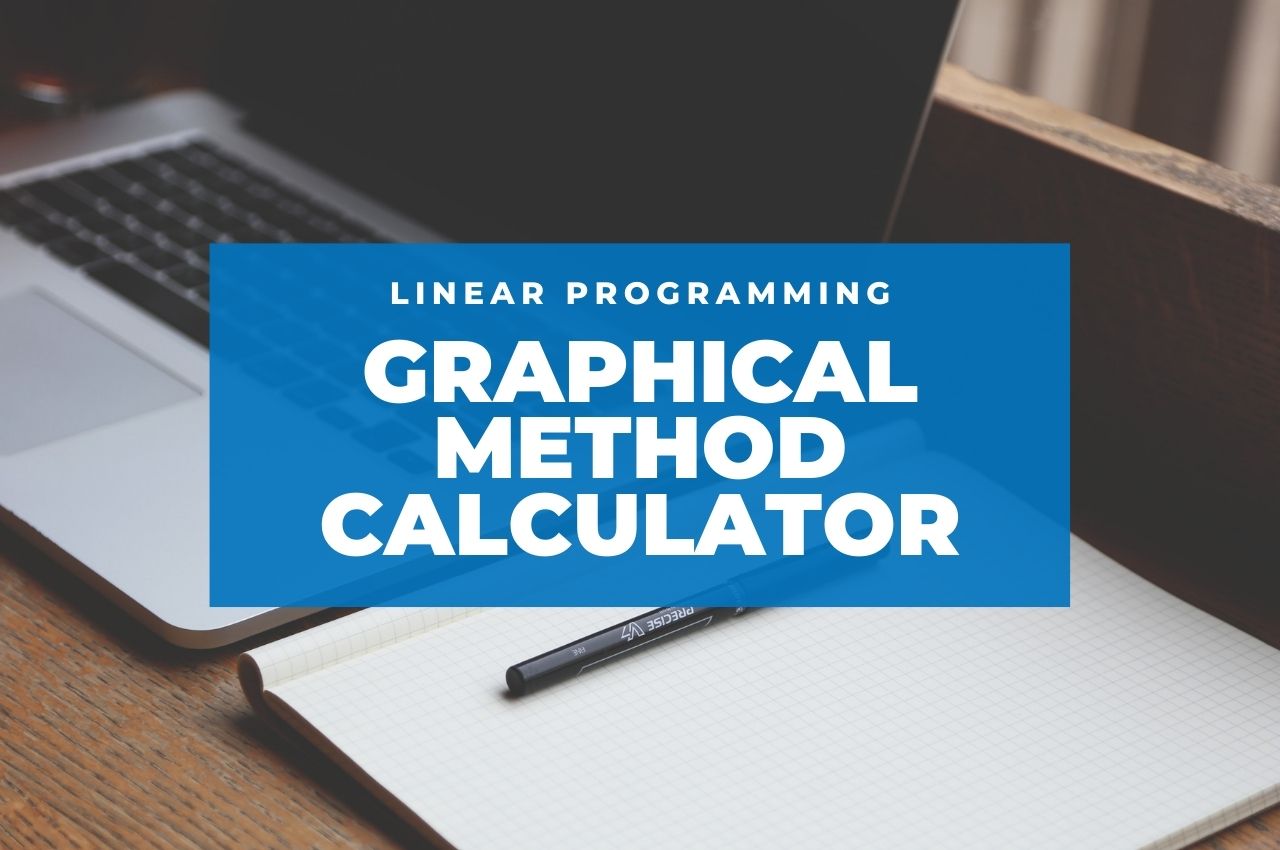
Graphical Method Calculator – Linear Programming đ„
With our Graphical Method Calculator for Linear Programming will quickly solve linear programming problems and display the optimal solution. â
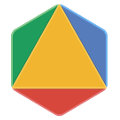
- Google OR-Tools
- Español â AmĂ©rica Latina
- PortuguĂȘs â Brasil
- TiĂȘÌng ViĂȘÌŁt
Linear Sum Assignment Solver
This section describes the linear sum assignment solver , a specialized solver for the simple assignment problem, which can be faster than either the MIP or CP-SAT solver. However, the MIP and CP-SAT solvers can handle a much wider array of problems, so in most cases they are the best option.
Cost matrix
The costs for workers and task are given in the table below.
The following sections present a Python program that solves an assignment problem using the linear sum assignment solver.
Import the libraries
The code that imports the required library is shown below.
Define the data
The following code creates the data for the program.
The array is the cost matrix , whose i , j entry is the cost for worker i to perform task j . There are four workers, corresponding to the rows of the matrix, and four tasks, corresponding to the columns.
Create the solver
The program uses the linear assignment solver, a specialized solver for the assignment problem.
The following code creates the solver.
Add the constraints
The following code adds the costs to the solver by looping over workers and tasks.
Invoke the solver
The following code invokes the solver.
Display the results
The following code displays the solution.
The output below shows the optimal assignment of workers to tasks.
The following graph shows the solution as the dashed edges in the graph. The numbers next to the dashed edges are their costs. The total wait time of this assignment is the sum of the costs for the dashed edges, which is 265.
In graph theory, a set of edges in a bipartite graph that matches every node on the left with exactly one node on the right is called a perfect matching .
The entire program
Here is the entire program.
Solution when workers can't perform all tasks
In the previous example, we assumed that all workers can perform all tasks. But this not always the case - a worker might be unable to perform one or more tasks for various reasons. However, it is easy to modify the program above to handle this.
As an example, suppose that worker 0 is unable to perform task 3. To modify the program to take this into account, make the following changes:
- Change the 0, 3 entry of the cost matrix to the string 'NA' . (Any string will work.) cost = [[90, 76, 75, 'NA'], [35, 85, 55, 65], [125, 95, 90, 105], [45, 110, 95, 115]]
- In the section of the code that assigns costs to the solver, add the line if cost[worker][task] != 'NA': , as shown below. for worker in range(0, rows): for task in range(0, cols): if cost[worker][task] != 'NA': assignment.AddArcWithCost(worker, task, cost[worker][task]) The added line prevents any edge whose entry in the cost matrix is 'NA' from being added to the solver.
After making these changes and running the modified code, you see the following output:
Notice that the total cost is higher now than it was for the original problem. This is not surprising, since in the original problem the optimal solution assigned worker 0 to task 3, while in the modified problem that assignment is not allowed.
To see what happens if more workers are unable to perform tasks, you can replace more entries of the cost matrix with 'NA' , to denote additional workers who can't perform certain tasks:
When you run the program this time, you get a negative result:
This means there is no way to assign workers to tasks so that each worker performs a different task. You can see why this is so by looking at the graph for the problem (in which there are no edges corresponding to values of 'NA' in the cost matrix).
Since the nodes for the three workers 0, 1, and 2 are only connected to the two nodes for tasks 0 and 1, it not possible to assign distinct tasks to these workers.
The Marriage Theorem
There is a well-known result in graph theory, called The Marriage Theorem , which tells us exactly when you can assign each node on the left to a distinct node on the right, in a bipartite graph like the one above. Such an assignment is called a perfect matching . In a nutshell, the theorem says this is possible if there is no subset of nodes on the left (like the one in the previous example ) whose edges lead to a smaller set of nodes on the right.
More precisely, the theorem says that a bipartite graph has a perfect matching if and only if for any subset S of the nodes on the left side of the graph, the set of nodes on the right side of the graph that are connected by an edge to a node in S is at least as large as S.
Except as otherwise noted, the content of this page is licensed under the Creative Commons Attribution 4.0 License , and code samples are licensed under the Apache 2.0 License . For details, see the Google Developers Site Policies . Java is a registered trademark of Oracle and/or its affiliates.
Last updated 2023-01-18 UTC.
Solver of multiobjective linear optimization problems: description and documents
View the Project on GitHub vOptSolver/vOptSolver
Solver of multiobjective linear optimization problems
vOptSolver is an ecosystem for modeling and solving multiobjective linear optimization problems (MOMIP, MOLP, MOIP, MOCO). It integrates several exact algorithms for computing the set of non-dominated points $Y_N$, and the corresponding complete set of efficient solutions $X_E$, for structured and non-structured optimization problems with at least two objectives.
It is composed of the two julia packages MultiObjectiveAlgorithms (previously vOptGeneric ) and vOptSpecific , and hosts vOPtLib , a library of numerical instances:
- MultiObjectiveAlgorithms.jl which replaces vOptGeneric since February 2023
- vOptSpecific
IMPORTANT (Feb-2023): vOptGeneric.jl has been fully redesigned, and reimplemented. It becomes MultiObjectiveAlgorithms.jl (MOA) , a collection of algorithms for multi-objective optimization integrated to JuMP and MathOptInterface . MOA comes with an enriched list of multi-objective algorithms, especially for solving problems with 3 objectives. Consequently vOptGeneric.jl is no longer under active development. It will remain available on Github at https://github.com/vOptSolver/vOptGeneric.jl. From February 2023, the JuMP-dev organization will continue to maintain the MOA package and transition development over the long term.
Instructions
- Documentation
All bugs, feature requests, pull requests, feedback, etc., are welcome.
Coordinator
Prof. Dr. Xavier Gandibleux, University of Nantes - France (contact)
By alphabetical order (concerning vOptGeneric and vOptSpecific):
- Current contributors: Xavier Gandibleux, Anthony Przybylski, Gauthier Soleilhac.
- Past contributors: Lucas Baussey, Pauline Chatelier, Quentin Delmée, Dorian Dumez, Flavien Lucas, Clément Turcat.
How To Contribute
- in adding your examples (code JuMP + data) solved with vOptGeneric to the collection;
- in plugging your own C/C++/Julia algorithms into vOptSpecific or vOptGeneric;
- in adapting vOptSpecific for windows;
- in sending us your suggestions to improve/extend vOptSolver;
- in telling us when you have completed a work (exercices for students; research; paper; etc.) using vOptSolver;
- in joining the adventure with us as maintainer of the solver, repositories, documents, etc.
In brief, every contributions aiming to share our efforts, our algorithms, our productions around this open source software are welcome.
vOptSolver is distributed under the MIT License .
Xavier Gandibleux. Multi-objective optimization with JuMP. JuliaCon 2023 . Massachusetts Institute of Technology. Cambridge, USA. July 25-29, 2023.
Xavier Gandibleux, Gauthier Soleilhac, Anthony Przybylski. vOptSolver: an ecosystem for multi-objective linear optimization. JuliaCon 2021 . Online and everywhere. July 28-30, 2021. Abstract . Reference to use for citing vOptSolver
Anthony Przybylski. Optimisation combinatoire multi-objectif : mĂ©thodes de rĂ©solution exacte et solveur vOpt. JuliaDayâ2019 : JournĂ©e « Julia et Optimisation ». 17 Juin 2019 . UniversitĂ© de Nantes, France. https://julialang.univ-nantes.fr/journee-julia-et-optimisation
Xavier Gandibleux et Anthony Przybylski, Algorithmes de branch-and-bound multiobjectif et vOptSolver. Tutoriel du GDR CNRS RO, ROADEFâ2018 : 19e Ă©dition du congrĂšs annuel de la SociĂ©tĂ© Française de Recherche OpĂ©rationnelle et dâAide Ă la DĂ©cision. 22 fĂ©vrier 2018, Lorient. Video .
Xavier Gandibleux et Anthony Przybylski, Optimisation combinatoire multiobjectif : deux contributions issues du projet de recherche ANR-DFG vOpt. SPOC16 : 16e journĂ©e âPolyĂšdres et optimisation combinatoireâ . LAMSADE/UniversitĂ© Paris-Dauphine. 15 dĂ©cembre 2017.
Xavier Gandibleux, Pascal Halffmann. Designing and Experimenting with vOptSolver an Algorithm for Computing the Weight Set Decomposition. RAMOOâ2017 : 4th International workshop âRecent Advances in Multi-Objective Optimizationâ , TU-Kaiserslautern, Germany. 2017.
Xavier Gandibleux, Gauthier Soleilhac, Anthony Przybylski, Flavien Lucas, Stefan Ruzika, Pascal Halffmann. vOptSolver, a âget and runâ solver of multiobjective linear optimization problems built on Julia and JuMP. MCDM2017: 24th International Conference on Multiple Criteria Decision Making . July 10-14, 2017. Ottawa (Canada).
Xavier Gandibleux, Gauthier Soleilhac, Anthony Przybylski, Stefan Ruzika. vOptSolver: an open source software environment for multiobjective mathematical optimization. IFORS2017: 21st Conference of the International Federation of Operational Research Societies . July 17-21, 2017. Quebec City (Canada).
The development of vOptSolver started in the ANR/DFG-14-CE35-0034-01 research project vOpt (2015-2019) (link) involving Université de Nantes (France) and University of Koblenz-Landau/University of Kaiserslautern (Germany).
- Solver of multiobjective linear optimization problems for scientifics and practionners
- Easy to formulate a problem, to provide data, to solve a problem, to collect the outputs, to analyze the solutions
- Natural and intuitive use for mathematicians, informaticians, engineers
- Solving needs: methods and algorithms for performing numerical experiments
- Research needs: support and primitives for the development of new algorithms
- Pedagogic needs: environment for practicing of theories and algorithms
Characteristics
- Efficient, flexible, evolutive solver
- Free, open source, multi-platform, reusing existing specifications
- Easy installation, no need of being expert in computer science
- Julia programming language (link)
- JuMP algebraic language (link)
- Usual free (GLPK, Clp/Cbc) and commercial (CPLEX, GUROBI) MIP solvers
Problems managed
- p-LP: Linear Program
- p-MIP: Mixed Integer linear Program
- p-IP: Integer linear Program
- 2-LAP: Linear Assignment Problem
- 2-OSP: One machine Scheduling Problem
- 2-UKP: binary Unidimensional knapsack problem
- 2-UMFLP: Uncapacitated Mixed variables Facility Location Problem
- Forthcoming: [p-PATHS, 2-UDFLP]. Projects : [MKP, SSCFLP, CFLP]
Algorithms integrated
The solving algorithms included compute exact solution(s) corresponding to Y_{lex}, Y_{SN}, or Y_{N}.
- Lexico: compute Y_{lex}, the lexicographic optimal solutions for p-IP (Julia+JuMP)
- Aneja1979: compute Y_{SN} with Aneja & Nair method (also named the dichotomic method) for 2-IP (Julia+JuMP)
- Haimes1971: compute Y_{N} with epsilon-constraint method for 2-IP (Julia+JuMP)
- Chalmet1986: compute Y_N with Chalmet et al. method for 2-IP (Julia+JuMP)
- Forthcoming: algorithms for [{2,3}-LP]. Projects: algorithms for [3-IP, {2,3}-MIP]
- Przybylski2008: 2LAP2008 (C)
- Wassenhove1980: 2OSP1980 (implemented in 2017 in Julia)
- Jorge2010: 2UKP2010 (re-implemented in 2017 in Julia)
- Delmee2017: 2UMFLP2016 (C++)
- Forthcoming:[Gandibleux2006: PATHS (re-implemented in 2018 in Julia); Gandibleux2012: 2UDFLP2012 (re-implemented in 2018 in C)]
- direct with the provided languages (Julia, JuMP)
- standard MOP format (IP, MIP, LP)
- specific problem format (MIP)
- direct with the language (Julia),
- specific problem format (2LAP, 2UKP, 2UFLP)
- standard 2MOP format (IP, MIP, LP)
Information
- Julia is available on macOS, linux, and windows
- vOptSolver (composed of vOptGeneric and vOptSpecific) is free, open source under MIT licence.
- vOptGeneric has been tested with Julia 1.6 on macOS 11.4, linux-Ubuntu 18.04.5 LTS and Windows 10 64 bits.
- vOptSpecific have been tested with Julia 1.6 on macOS 11.4 and linux-Ubuntu 18.04.5 LTS.
Installation and usage Instructions
Refer to the instructions provided for
- vOptGeneric
Documentation and examples
NB: the available documentation is obsolete (written for Julia v0.6.4; new documentation compliant with v1.x is coming).
Old documentation:
- Tutorial (in waiting a user manual) for new users (folder docs)
- Presentations given in conferences (folder talks)
Examples of problems ready to be solved:
- With vOptGeneric (folder examples)
- With vOptSpecific (folder examples)
[Haimes1971] Y.V. Haimes, L.S. Lasdon, D.A. Wismer: On a bicriterion formation of the problems of integrated system identification and system optimization. IEEE Transactions on Systems, Man and Cybernetics , Volume SMC-1, Issue 3, Pages 296-297, July 1971.
[Aneja1979] Y. P. Aneja and K. P. K. Nair: Bicriteria Transportation Problem. Management Science , 25:1, 73-78 1979.
[Wassenhove1980] L. N. Van Wassenhove, L. F. Gelders: Solving a bicriterion scheduling problem. European Journal of Operational Research , Volume 4, Issue 1, Pages 42-48, 1980.
[Chalmet1986] L.G. Chalmet, L. Lemonidis, D.J. Elzinga: An algorithm for the bi-criterion integer programming problem. European Journal of Operational Research , Volume 25, Issue 2, Pages 292-300, 1986.
[Gandibleux2006] X. Gandibleux, F. Beugnies, S. Randriamasy: Martinsâ algorithm revisited for multi-objective shortest path problems with a MaxMin cost function. 4OR: A Quarterly Journal of Operations Research , Springer Verlag, 4 (1), pp.47-59, 2006.
[Przybylski2008] A. Przybylski, X. Gandibleux, M. Ehrgott: Two phase algorithms for the bi-objective assignment problem. European Journal of Operational Research , Volume 185, Issue 2, Pages 509-533, 2008.
[Jorge2010] J. Jorge: Nouvelles propositions pour la résolution exacte du sac à dos multi-objectif unidimensionnel en variables binaires. PhD Thesis (in French), Université de Nantes - France, 2010.
[Gandibleux2012] X. Gandibleux, A. Przybylski , S. Bourougaa, A. Derrien, A. Grimault: Computing the Efficient Frontier for the 0/1 Biobjective Uncapacitated Facility Location Problem CORS/MOPGPâ2012 (10th international conference on Multiple Objective Programming and Goal Programming). June 11-13, 2012, Niagara Falls, Canada.
[Vincent2013] Th. Vincent: CaractĂ©risation des solutions efficaces et algorithmes dâĂ©numĂ©ration exacts pour lâoptimisation multiobjectif en variables mixtes binaires. PhD Thesis (in French), UniversitĂ© de Nantes - France, 2013.
[Delmee2017] Q. DelmĂ©e, X. Gandibleux, A. Przybylski: RĂ©solution exacte du problĂšme de localisation de services bi-objectif sans contrainte de capacitĂ© en variables mixtes. ROADEF2017 (18Ăšme Ă©dition du congrĂšs annuel de la SociĂ©tĂ© Française de Recherche OpĂ©rationnelle et dâAide Ă la DĂ©cision). 22-24 fĂ©vrier 2017, Metz, France.
[Dumez2017] D. Dumez, X. Gandibleux, I. Rusu. Datastructures for Filtering and Storing Non-Dominated Points. MOPGPâ2017: 12th International Conference on Multiple Objective Programming and Goal Programming. 30-31 October 2017, Metz, France.
Terms and acronyms used
- LP: Linear Program
- MIP: Mixed Integer linear Program
- IP: Integer linear program
- CO: Combinatorial Optimization
- MOLP: MultiObjective linear program
- MOIP: MultiObjective Integer linear program
- MOMIP: MultiObjective Mixed Integer linear Program
- MOCO: MultiObjective Combinatorial Optimization
- OSP: One machine Scheduling Problem
- LAP: Linear Assignment Problem
- UKP: Unidimensional 01 Knapsack Problem
- MKP: Multidimensional 01 Knapsack Problem
- UFLP: Uncapacitated Facility Location Problem
- UDFLP: Discrete Uncapacitated Facility Location Problem
- SSCFLP: Single Source Capacitated Facility Location Problem
- UMFLP: Uncapacitated Mixed variables Facility Location Problem
- CFLP: Capacitated Facility Location Problem
- PATHS: shortest paths problem
- Julia: name of the programming language
- JuMP: stands for Julia for Mathematical Optimization, a modeling language for mathematical optimization embedded in Julia
- AVL tree is a self-balancing binary search tree
- API: stands for Application Programming Interface
- GPL: stands for GNU General Public License
- GLPK: stands for GNU Linear Programming Kit, an open source solver
- Clp/Cbc : an open source solver (for LP and MILP respectively) from the COIN-OR project
- CPLEX: a commercial solver
- GUROBI: a commercial solver
- MOP: MultiObjective extension of MPS format
- MapReduce Algorithm
- Linear Programming using Pyomo
- Networking and Professional Development for Machine Learning Careers in the USA
- Predicting Employee Churn in Python
- Airflow Operators

Solving Assignment Problem using Linear Programming in Python
Learn how to use Python PuLP to solve Assignment problems using Linear Programming.
In earlier articles, we have seen various applications of Linear programming such as transportation, transshipment problem, Cargo Loading problem, and shift-scheduling problem. Now In this tutorial, we will focus on another model that comes under the class of linear programming model known as the Assignment problem. Its objective function is similar to transportation problems. Here we minimize the objective function time or cost of manufacturing the products by allocating one job to one machine.
If we want to solve the maximization problem assignment problem then we subtract all the elements of the matrix from the highest element in the matrix or multiply the entire matrix by â1 and continue with the procedure. For solving the assignment problem, we use the Assignment technique or Hungarian method, or Floodâs technique.
The transportation problem is a special case of the linear programming model and the assignment problem is a special case of transportation problem, therefore it is also a special case of the linear programming problem.
In this tutorial, we are going to cover the following topics:
Assignment Problem
A problem that requires pairing two sets of items given a set of paired costs or profit in such a way that the total cost of the pairings is minimized or maximized. The assignment problem is a special case of linear programming.
For example, an operation manager needs to assign four jobs to four machines. The project manager needs to assign four projects to four staff members. Similarly, the marketing manager needs to assign the 4 salespersons to 4 territories. The managerâs goal is to minimize the total time or cost.
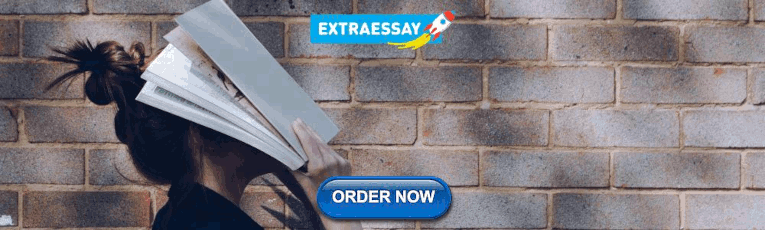
Problem Formulation
A manager has prepared a table that shows the cost of performing each of four jobs by each of four employees. The manager has stated his goal is to develop a set of job assignments that will minimize the total cost of getting all 4 jobs. Â
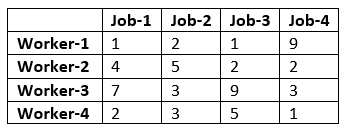
Initialize LP Model
In this step, we will import all the classes and functions of pulp module and create a Minimization LP problem using LpProblem class.
Define Decision Variable
In this step, we will define the decision variables. In our problem, we have two variable lists: workers and jobs. Letâs create them using LpVariable.dicts()  class. LpVariable.dicts()  used with Pythonâs list comprehension. LpVariable.dicts()  will take the following four values:
- First, prefix name of what this variable represents.
- Second is the list of all the variables.
- Third is the lower bound on this variable.
- Fourth variable is the upper bound.
- Fourth is essentially the type of data (discrete or continuous). The options for the fourth parameter are LpContinuous or LpInteger .
Letâs first create a list route for the route between warehouse and project site and create the decision variables using LpVariable.dicts() the method.
Define Objective Function
In this step, we will define the minimum objective function by adding it to the LpProblem object. lpSum(vector)is used here to define multiple linear expressions. It also used list comprehension to add multiple variables.
Define the Constraints
Here, we are adding two types of constraints: Each job can be assigned to only one employee constraint and Each employee can be assigned to only one job. We have added the 2 constraints defined in the problem by adding them to the LpProblem  object.
Solve Model
In this step, we will solve the LP problem by calling solve() method. We can print the final value by using the following for loop.
From the above results, we can infer that Worker-1 will be assigned to Job-1, Worker-2 will be assigned to job-3, Worker-3 will be assigned to Job-2, and Worker-4 will assign with job-4.
In this article, we have learned about Assignment problems, Problem Formulation, and implementation using the python PuLp library. We have solved the Assignment problem using a Linear programming problem in Python. Of course, this is just a simple case study, we can add more constraints to it and make it more complicated. You can also run other case studies on Cargo Loading problems , Staff scheduling problems . In upcoming articles, we will write more on different optimization problems such as transshipment problem, balanced diet problem. You can revise the basics of mathematical concepts in this article and learn about Linear Programming in this article .
- Solving Blending Problem in Python using Gurobi
- Transshipment Problem in Python Using PuLP
You May Also Like
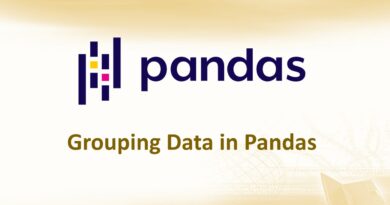
Grouping Data in Pandas
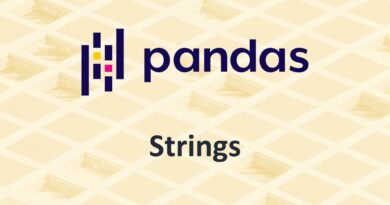
Working with Strings in Pandas
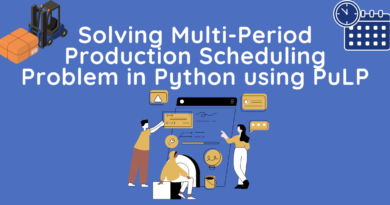
Solving Multi-Period Production Scheduling Problem in Python using PuLP
Assignment Problem Calculator
The valid input data of assigning $n$ tasks to $n$ workers is in the form of $n\times n$ cost matrix $C$ of nonnegative integer numbers, see Matrix Data Input for more detail of entering matrix data. The element $C_{ij}$ is the cost of assigning task $j$ to worker $i$.
- Maths Notes Class 12
- NCERT Solutions Class 12
- RD Sharma Solutions Class 12
- Maths Formulas Class 12
- Maths Previous Year Paper Class 12
- Class 12 Syllabus
- Class 12 Revision Notes
- Physics Notes Class 12
- Chemistry Notes Class 12
- Biology Notes Class 12
- CBSE Class 12 Maths Notes: Chapter Wise Notes 2024
Chapter 1: Relations and Functions
- Types of Functions
- Composite functions - Relations and functions
- Invertible Functions
- Composition of Functions
- Inverse Functions
- Verifying Inverse Functions by Composition
Chapter 2: Inverse Trigonometric Functions
- Inverse Trigonometric Functions
- Graphs of Inverse Trigonometric Functions - Trigonometry | Class 12 Maths
- Properties of Inverse Trigonometric Functions
- Inverse Trigonometric Identities
Chapter 3: Matrices
- Types of Matrices
- Matrix Operations
- Matrix Addition
- Matrix Multiplication
- Transpose of a Matrix
- Symmetric and Skew Symmetric Matrices
- Elementary Operations on Matrices
- Inverse of a Matrix by Elementary Operations - Matrices | Class 12 Maths
- Invertible Matrix
Chapter 4: Determinants
- Determinant of a Matrix with Solved Examples
- Properties of Determinants
- Area of a Triangle using Determinants
- Minors and Cofactors
- Adjoint of a Matrix
- Applications of Matrices and Determinants
Chapter 5: Continuity and Differentiability
- Continuity and Discontinuity in Calculus - Class 12 CBSE
- Differentiability of a Function | Class 12 Maths
- Derivatives of Inverse Functions
- Derivatives of Implicit Functions - Continuity and Differentiability | Class 12 Maths
- Derivatives of Composite Functions
- Derivatives of Inverse Trigonometric Functions | Class 12 Maths
- Derivative of Exponential Functions
- Logarithmic Differentiation - Continuity and Differentiability
- Proofs for the derivatives of eËŁ and ln(x) - Advanced differentiation
- Rolle's Theorem and Lagrange's Mean Value Theorem
- Derivative of Functions in Parametric Forms
- Second Order Derivatives in Continuity and Differentiability | Class 12 Maths
- Mean Value Theorem
- Algebra of Continuous Functions - Continuity and Differentiability | Class 12 Maths
Chapter 6: Applications of Derivatives
- Critical Points
- Derivatives as Rate of Change
- Increasing and Decreasing Functions
- Increasing and Decreasing Intervals
- Tangents and Normals
- Equation of Tangents and Normals
- Relative Minima and Maxima
- Absolute Minima and Maxima
- Concave Function
- Inflection Point
- Curve Sketching
- Approximations & Maxima and Minima - Application of Derivatives | Class 12 Maths
- Higher Order Derivatives
Chapter 7: Integrals
- Integration by Substitution
- Integration by Partial Fractions
- Integration by Parts
- Integration of Trigonometric Functions
- Functions Defined by Integrals
- Definite Integral
- Computing Definite Integrals
- Fundamental Theorem of Calculus
- Finding Derivative with Fundamental Theorem of Calculus
- Evaluating Definite Integrals
- Properties of Definite Integrals
- Definite Integrals of Piecewise Functions
- Improper Integrals
- Riemann Sum
- Riemann Sums in Summation Notation
- Trapezoidal Rule
- Definite Integral as the Limit of a Riemann Sum
- Antiderivatives
- Indefinite Integrals
- Particular Solutions to Differential Equations
- Integration by U-substitution
- Reverse Chain Rule
- Partial Fraction Expansion
- Trigonometric Substitution
Chapter 8: Applications of Integrals
- Area under Simple Curves
- Area Between Two Curves - Calculus
- Area between Polar Curves
- Area as Definite Integral
Chapter 9: Differential Equations
- Differential Equations
- Homogeneous Differential Equations
- Separable Differential Equations
- Exact Equations and Integrating Factors
- Implicit Differentiation
- Implicit differentiation - Advanced Examples
- Advanced Differentiation
- Disguised Derivatives - Advanced differentiation | Class 12 Maths
- Derivative of Inverse Trig Functions
- Logarithmic Differentiation
Chapter 10: Vector Algebra
- Vector Algebra
- Dot and Cross Products on Vectors
- How to Find the Angle Between Two Vectors?
- Section Formula - Vector Algebra
Chapter 11: Three-dimensional Geometry
- Direction Cosines and Direction Ratios
- Equation of a Line in 3D
- Angles Between two Lines in 3D Space
- Shortest Distance Between Two Lines in 3D Space | Class 12 Maths
- Points, Lines and Planes
Chapter 12: Linear Programming
Linear programming.
- Graphical Solution of Linear Programming Problems
Chapter 13: Probability
- Conditional Probability and Independence - Probability | Class 12 Maths
- Multiplication Theorem
- Dependent and Independent Events
- Bayes' Theorem
- Probability Distribution
- Binomial Distribution in Probability
- Binomial Mean and Standard Deviation - Probability | Class 12 Maths
- Bernoulli Trials and Binomial Distribution
- Discrete Random Variable
- Expected Value
- NCERT Solutions for Class 12 Maths -Chapter Wise with PDF
- RD Sharma Class 12 Solutions for Maths
Linear programming is a mathematical concept that is used to find the optimal solution of the linear function. This method uses simple assumptions for optimizing the given function. Linear Programming has a huge real-world application and it is used to solve various types of problems.
Linear programming is used in various industries such as shipping industries, manufacturing industries, transportation industries, telecommunications, and others.
Term “linear programming” consists of two words linear and programming, the word linear tells the relation between various types of variables of degree one used in a problem and the word programming tells us the step-by-step procedure to solve these problems.
In this article, we will learn about linear programming, its examples, formulas, and other concepts in detail.
Table of Content
What is Linear Programming?Â
Components of linear programming, linear programming examples, linear programming problems, types of linear programming problems, linear programming formula, how to solve linear programming problems, linear programming methods, linear programming simplex method, linear programming graphical method, linear programming applications, importance of linear programming, up-to-date applications of linear programming, linear programming in operations research, simplex method.
Linear programming or Linear optimization is a technique that helps us to find the optimum solution for a given problem, an optimum solution is a solution that is the best possible outcome of a given particular problem.
In simple terms, it is the method to find out how to do something in the best possible way. With limited resources, you need to do the optimum utilization of resources and achieve the best possible result in a particular objective such as least cost, highest margin, or least time.Â
The situation that requires a search for the best values of the variables subject to certain constraints is where we use linear programming problems. These situations cannot be handled by the usual calculus and numerical techniques.
Linear Programming Definition
Linear programming is the technique used for optimizing a particular scenario. Using linear programming provides us with the best possible outcome in a given situation. It uses all the available resources in a manner such that they produce the optimum result.
The basic components of a linear programming(LP) problem are:
- Decision Variables: Variables you want to determine to achieve the optimal solution.
- Objective Function: M athematical equation that represents the goal you want to achieve
- Constraints: Limitations or restrictions that your decision variables must follow.
- Non-Negativity Restrictions: In some real-world scenarios, decision variables cannot be negative
Additional Characteristics of Linear Programming
- Finiteness: The number of decision variables and constraints in an LP problem are finite.
- Linearity: The objective function and all constraints must be linear functions of the decision variables . It means the degree of variables should be one.
We can understand the situations in which Linear programming is applied with the help of the example discussed below,
Suppose a delivery man has to deliver 8 packets in a day to the different locations of a city. He has to pick all the packets from A and has to deliver them to points P, Q, R, S, T, U, V, and W. The distance between them is indicated using the lines as shown in the image below. The shortest path followed by the delivery man is calculated using the concept of Linear Programming.
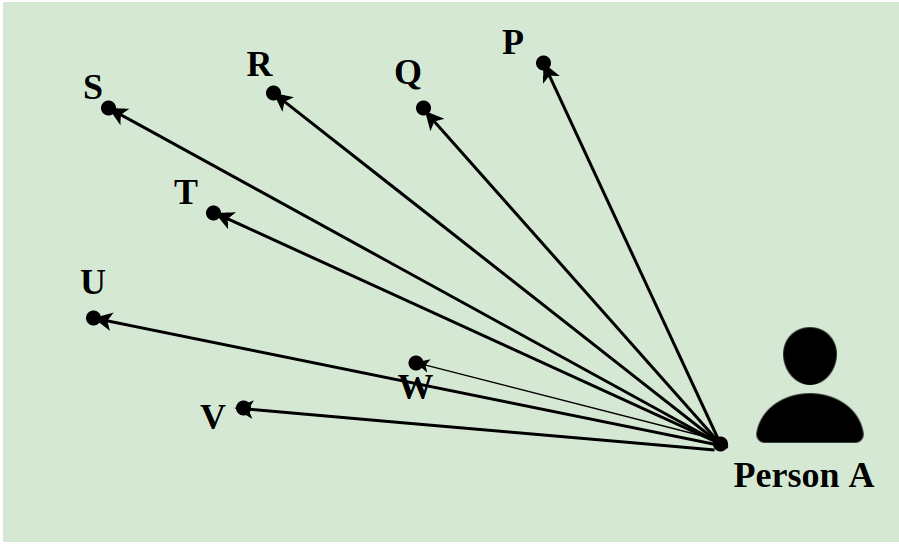
Linear Programming Problems (LPP) involve optimizing a linear function to find the optimal value solution for the function. The optimal value can be either the maximum value or the minimum value.
In LPP, the linear functions are called objective functions. An objective function can have multiple variables, which are subjected to conditions and have to satisfy the linear constraints .
There are many different linear programming problems(LPP) but we will deal with three major linear programming problems in this article.
Manufacturing Problems
Manufacturing problems are a problem that deals with the number of units that should be produced or sold to maximize profits when each product requires fixed manpower, machine hours, and raw materials.
Diet Problems
It is used to calculate the number of different kinds of constituents to be included in the diet to get the minimum cost, subject to the availability of food and their prices.
Transportation Problems
It is used to determine the transportation schedule to find the cheapest way of transporting a product from plants /factories situated at different locations to different markets.
A linear programming problem consists of,
- Decision variables
- Objective function
- Constraints
- Non-Negative restrictions
Decision variables are the variables x, and y, which decide the output of the linear programming problem and represent the final solution.Â
The objective function , generally represented by Z, is the linear function that needs to be optimized according to the given condition to get the final solution.Â
The restrictions imposed on decision variables that limit their values are called constraints.
Now, the general formula of a linear programming problem is,
Objective Function : Z = ax + by
Constraints: cx + dy ℠e, px + qy †r
Non-Negative restrictions: x â„ 0, y â„ 0
In the above condition x, and y are the decision variables.
Before solving the linear programming problems first we have to formulate the problems according to the standard parameters. The steps for solving linear programming problems are,
Step 1: Mark the decision variables in the problem. Step 2: Build the objective function of the problem and check if the function needs to be minimized or maximized. Step 3: Write down all the constraints of the linear problems. Step 4: Ensure non-negative restrictions of the decision variables. Step 5: Now solve the linear programming problem using any method generally we use either the simplex or graphical method.
We use various methods for solving linear programming problems. The two most common methods used are,
- Graphical Method
Let’s learn about these two methods in detail in this article,
One of the most common methods to solve the linear programming problem is the simplex method. In this method, we repeat a specific condition ‘n’ a number of times until an optimum solution is achieved.
The steps required to solve linear programming problems using the simplex method are,
Step 1: Formulate the linear programming problems based on the given constraints. Step 2: Convert all the given inequalities to equations or equalities of the linear programming problems by adding the slack variable to each inequality where ever required. Step 3: Construct the initial simplex table. By representing each constraint equation in a row and writing the objective function at the bottom row. The table so obtained is called the Simplex table. Step 4: Identify the greatest negative entry in the bottom row the column of the element with the highest negative entry is called the pivot column Step 5: Divide the entries of the right-most column with the entries of the respective pivot column, excluding the entries of the bottommost row. Now the row containing the least entry is called the pivot row. The pivot element is obtained by the intersection of the pivot row and the pivot column. Step 6: Using matrix operation and with the help of the pivot element make all the entries in the pivot column to be zero. Step 7: Check for the non-negative entries in the bottommost row if there are no negative entries in the bottom row, end the process else start the process again from step 4. Step 8: The final simplex table so obtained gives the solution to our problem.
Graphical Method is another method than the Simplex method which is used to solve linear programming problems. As the name suggests this method uses graphs to solve the given linear programming problems. This is the best method to solve linear programming problems and requires less effort than the simplex method.Â
While using this method we plot all the inequalities that are subjected to constraints in the given linear programming problems. As soon as all the inequalities of the given LPP are plotted in the XY graph the common region of all the inequalities gives the optimum solution. All the corner points of the feasible region are calculated and the value of the objective function at all those points is calculated then comparing these values we get the optimum solution of the LPP.
Example: Find the maximal and minimal value of z = 6x + 9y when the constraint conditions are,
- 2x + 3y †12
- x and y â„ 0
Step 1 : First convert the inequations into normal equations. Hence the equations will be 2x+3y = 0, x = 0, y = 0 and x + y = 5. Step 2 : Find the points at which 2x + 3y and x + y = 5 cut the x-axis and y-axis. To find the point of intersection of the x-axis put y = 0 in the respective equation and find the point. Similarly for y-axis intersection points put x = 0 in the respective equation. Step 3 : Draw the two lines cutting the x-axis and y-axis. We find that the two axes cut each other at (3,2). Step 4 : For x â„ 0 and y â„ 0, we find that both inequations are followed. Hence the region will include an area region enclosed by two axes and both lines including the origin. The plotted region is shown below in the figure. Step 5 : Find Z for each point and maxima and minima. Coordinates Z = 6x + 9y (0,5) Z = 45 (0,4) Z = 36 (5,0) Z = 30 (6,0) Z = 36 (3,2) Z = 36 Hence, we find that Z = 6x + 9y is maximum at (0,5) and minimum at (5,0).
Linear Programming has applications in various fields. It is used to find the minimum cost of a process when all the constraints of the problems are given. It is used to optimize the transportation cost of the vehicle, etc. Various applications of Linear Programming are
Engineering Industries
Engineering Industries use linear programming to solve design and manufacturing problems and to get the maximum output from a given condition.
Manufacturing Industries
Manufacturing Industries use linear programming to maximize the profit of the companies and to reduce the manufacturing cost.
Energy Industries
Energy companies use linear programming to optimize their production output.
Transportation Industries
Linear programming is also used in transportation industries to find the path to minimize the cost of transportation.
Linear Programming has huge importance in various industries it maximizes the output value while minimizing the input values according to various constraints.
LP is highly applicable when we have multiple conditions while solving a problem and we have to optimize the output of the problem i.e. either we have to find the minimum or the maximum value according to a given condition.
Linear Inequalities Algebraic Solution of Linear Inequalities
Problem 1: A company manufactures and sells two types of products and the cost of production of each unit a and b is rupees 200 and 150 respectively each unit of product yields a profit of 20 rupees and each unit of product b yields a profit of 15 rupees on selling. The company estimates the monthly demand of A and B to be at a maximum of the harvested unit in all the production budget for the month is set at rupees 50000. How many units should the company manufacture to earn maximum profit from its monthly sales from a and b?
Let x = number of units of type A y = Number of units of type B Maximize Z = 40x + 50y Subject to the constraints 3x + y †9 x + 2y †8 and x, y ℠0 Consider the equation, 3x + y = 9 x = 3 y = 0 and x + 2y = 8 x = 8 y = 0 Now, we can determine the maximum value of Z by evaluating the value of Z at the four points (vertices) is shown below Vertices Z = 40x + 50y (0, 0) Z = 40 à 0 + 50 à 0 = Rs. 0 (3, 0) Z = 40 à 3 + 50 à 0 = Rs. 120 (0, 4)  Z = 40 à 0 + 50 à 4 = Rs. 200 (2, 3) Z = 40 à 2 + 50 à 3 = Rs. 230 Maximum profit, Z = Rs. 230 ⎠Number of units of type A is 2 and the number of units of type B is 3.
Problem 2: Maximize Z = 3x + 4y.
Subject to constraints , x + y †450, 2x + y †600 and x, y †0.
We have from the given Constraints (1) X + Y = 450 Putting x = 0, â 0 + y = 450 â y = 450 Putting y = 0, â x + 0 = 450 â x = 450 From, Constraints (2) 2x + y = 600 Putting x = 0, â 0 + y = 600 â y = 600 Putting  y = 0, â 2x + 0 = 600 â x = 300 Now, we have the points co-ordinate Z = 3x + 4y
Therefore, the optimal solution maximum Z = 1800 at co-ordinate x = 0 and y = 450. The graph is given below.
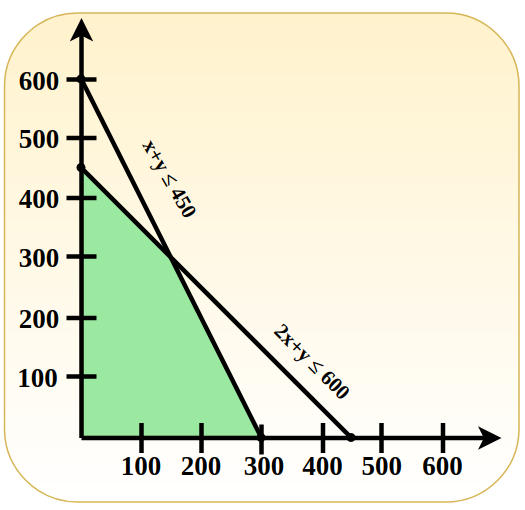
Linear programming, a powerful mathematical technique, is used to solve optimization problems in various industries. Here are some modern applications:
- Supply Chain Optimization : Linear programming helps companies minimize costs and maximize efficiency in their supply chains. It’s used for determining the most cost-effective transportation routes, warehouse operations, and inventory management strategies.
- Energy Management : In the energy sector, linear programming is utilized to optimize the mix of energy production methods. This includes balancing traditional energy sources with renewable ones to reduce costs and environmental impact while meeting demand.
- Telecommunications Network Design : Linear programming aids in designing efficient telecommunications networks. It helps in allocating bandwidth, designing network layouts, and optimizing the flow of data to ensure high-speed communication at lower costs.
- Financial Planning : Businesses and financial analysts use linear programming for portfolio optimization, risk management, and capital budgeting. It helps in making investment decisions that maximize returns while minimizing risk.
- Healthcare Logistics : In healthcare, linear programming is applied to optimize the allocation of resources, such as hospital beds, medical staff, and equipment. It’s crucial for improving patient care, reducing wait times, and managing costs effectively.
- Manufacturing Process Optimization : Linear programming is used to determine the optimal production levels for multiple products within a manufacturing facility, considering constraints like labor, materials, and machine availability.
- Agricultural Planning : Farmers and agricultural planners use linear programming to decide on crop selection, land use, and resource allocation to maximize yields and profits while conserving resources.
- Airline Crew Scheduling : Airlines employ linear programming to schedule crews efficiently, ensuring that flights are staffed in compliance with regulations and minimizing operational costs.
These applications demonstrate the versatility and power of linear programming in solving complex optimization problems across various sectors, showcasing its relevance in today’s data-driven world.
- Core Tool : Linear programming is a foundational tool in operations research for optimizing resources.
- Decision Making : Helps in making the best decisions regarding resource allocation, maximizing profits, or minimizing costs.
- Wide Applications : Used in various fields such as logistics, manufacturing, finance, and healthcare for solving complex problems.
- Modeling Real-World Problems : Transforms real-world problems into mathematical models to find the most efficient solutions.
- Optimization Algorithm : The Simplex Method is a powerful algorithm used in linear programming to find the optimal solution to linear inequalities.
- Step-by-Step Approach : It iteratively moves towards the best solution by navigating the edges of the feasible region defined by constraints.
- Efficiency : Known for its efficiency in solving large-scale linear programming problems.
- Versatility : Applicable in various domains like diet planning, network flows, production scheduling, and more, showcasing its versatility.
Linear Programming – FAQs
What is linear programming.
Linear programming is a mathematical concept which is used to optimize a given linear problem which has a variety of constraints. Using linear programming we the optimum output of the given problem
What are Linear Programming Problems?
Linear Programming Problems (LPP) are the problems which give the optimum solution to the given conditions.
What is Linear Programming Formula?
General Linear Programming Formulas are, Objective Function: Z = ax + by Constraints: px + qy †r, sx + ty †u Non-Negative Restrictions: x ℠0, y ℠0
What are the different types of Linear Programming?
Different types of linear programming methods are, Linear Programming by Simplex Method Linear Programming by R Method Linear Programming by Graphical Method
What are requirements of Linear Programming?
Various requirements of linear programming problems are, Linearity Objective Function Constraints Non-negativity
What are the advantages of Linear Programming?
Various advantages of linear programming are, It provides the optimal solution to any given linear problem. It is easy to use and always gives consistent results It helps to maximize profits and to reduce the input cost.
Please Login to comment...
- Math-Concepts
- Maths-Class-12
- Technical Scripter 2020
- Mathematics
- School Learning
- Technical Scripter
- 10 Best Todoist Alternatives in 2024 (Free)
- How to Get Spotify Premium Free Forever on iOS/Android
- Yahoo Acquires Instagram Co-Founders' AI News Platform Artifact
- OpenAI Introduces DALL-E Editor Interface
- Top 10 R Project Ideas for Beginners in 2024
Improve your Coding Skills with Practice
What kind of Experience do you want to share?
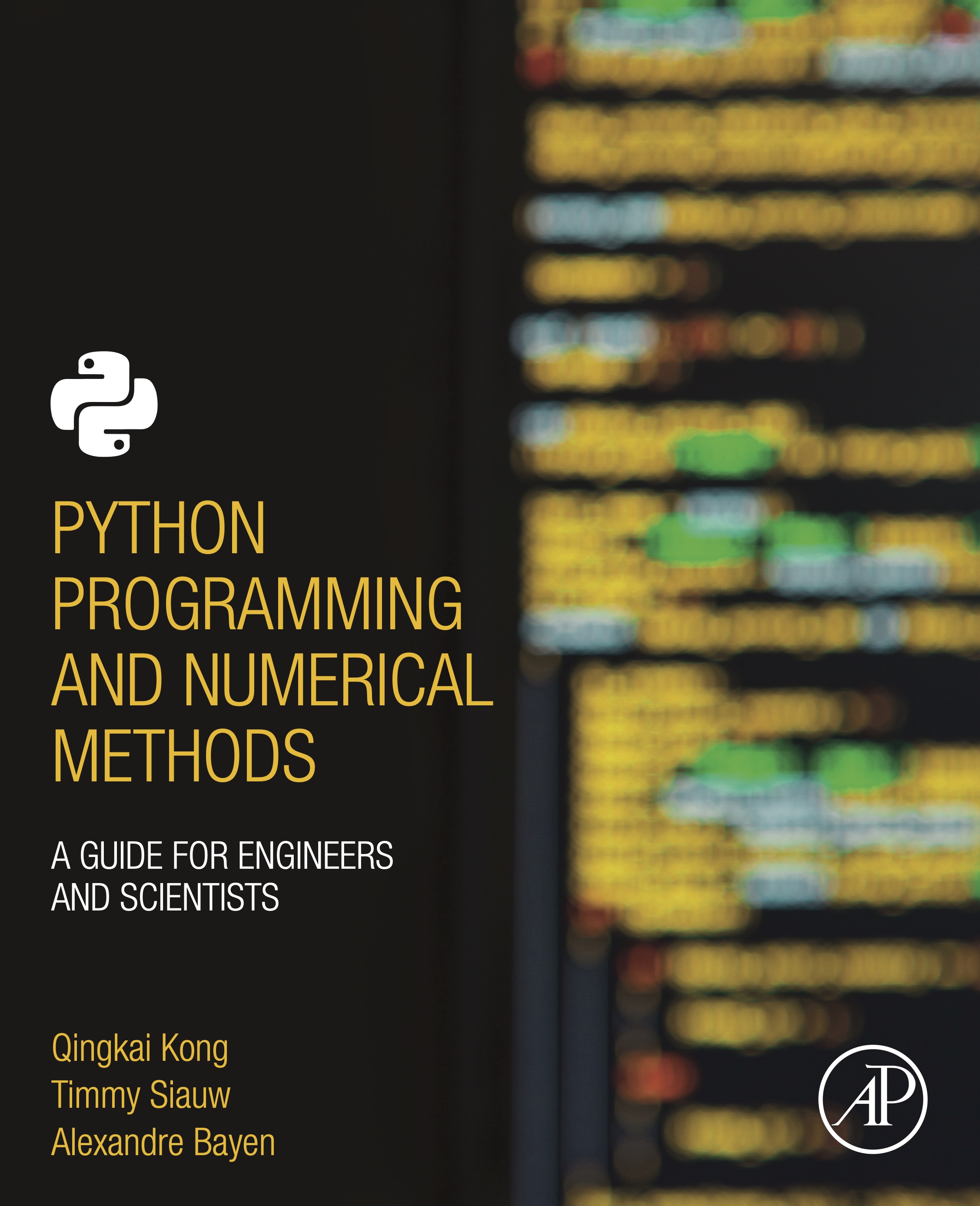
Python Numerical Methods
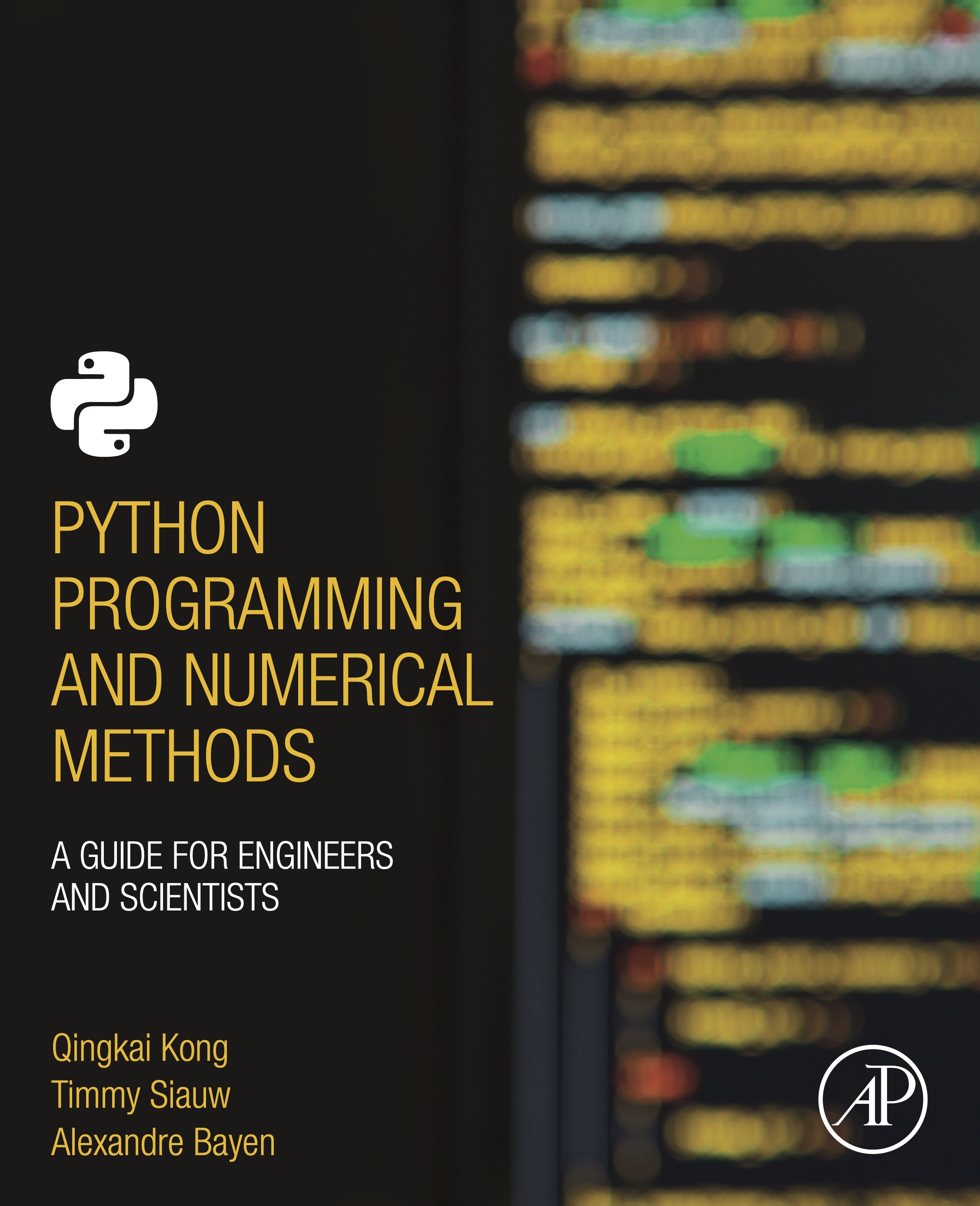
This notebook contains an excerpt from the Python Programming and Numerical Methods - A Guide for Engineers and Scientists , the content is also available at Berkeley Python Numerical Methods .
The copyright of the book belongs to Elsevier. We also have this interactive book online for a better learning experience. The code is released under the MIT license . If you find this content useful, please consider supporting the work on Elsevier or Amazon !
< CHAPTER 14. Linear Algebra and Systems of Linear Equations | Contents | 14.2 Linear Transformations >
Basics of Linear Algebra ¶
Before we introduce the systems of linear equations, letâs first introduce some basics of linear algebra, which will be used to describe and solve the linear equations. We will just cover the very basics of it in this chapter, and you can explore more by reading a linear algebra book.
We discussed the data structure - sets in chapter 2 before, here we will take a look of it from mathematics point of view using the mathematical languages. In mathematics, a set is a collection of objects. As we shown before, sets are usually denoted by braces {}. For example, \(S = {orange, apple, banana}\) means âS is the set containing âorangeâ, âappleâ, and âbananaââ.
The empty set is the set containing no objects and is typically denoted by empty braces such as \(\{\}\) or by \(\emptyset\) . Given two sets, \(A\) and \(B\) , the union of \(A\) and \(B\) is denoted by \(A \cup B\) and equal to the set containing all the elements of \(A\) and \(B\) . The intersect of \(A\) and \(B\) is denoted by \(A \cap B\) and equal to the set containing all the elements that belong to both \(A\) and \(B\) . In set notation, a colon is used to mean âsuch thatâ . The usage of these terms will become apparent shortly. The symbol \(\in\) is used to denote that an object is contained in a set. For example \(a \in A\) means â \(a\) is a member of \(A\) â or â \(a\) is in \(A\) .â A backslash, \(\backslash\) , in set notation means set minus . So if \(a\in A\) then \(A\backslash a\) means â \(A\) minus the element, \(a\) .â
There are several standard sets related to numbers, for example natural numbers , whole numbers , integers , rational numbers , irrational numbers , real numbers , and complex numbers . A description of each set and the symbol used to denote them is shown in the following table.
TRY IT! Let \(S\) be the set of all real \((x,y)\) pairs such that \(x^2 + y^2 = 1\) . Write \(S\) using set notation.
\(S = \{(x,y) : x,y \in {\mathbb{R}}, x^2 + y^2 = 1\}\)
The set \({\mathbb{R}}^n\) is the set of all \(n\) -tuples of real numbers. In set notation this is \({\mathbb{R}}^n = \{(x_1, x_2, x_3, \cdots, x_n): x_1, x_2, x_3, \cdots, x_n \in {\mathbb{R}}\}\) . For example, the set \({\mathbb{R}}^3\) represents the set of real triples, \((x,y,z)\) coordinates, in three-dimensional space.
A vector in \({\mathbb{R}}^n\) is an \(n\) -tuple, or point, in \({\mathbb{R}}^n\) . Vectors can be written horizontally (i.e., with the elements of the vector next to each other) in a row vector , or vertically (i.e., with the elements of the vector on top of each other) in a column vector . If the context of a vector is ambiguous, it usually means the vector is a column vector. The \(i\) -th element of a vector, \(v\) , is denoted by \(v_i\) . The transpose of a column vector is a row vector of the same length, and the transpose of a row vector is a column vector. In mathematics, the transpose is denoted by a superscript \(T\) , or \(v^T\) . The zero vector is the vector in \({\mathbb{R}}^n\) containing all zeros.
The norm of a vector is a measure of its length. There are many ways of defining the length of a vector depending on the metric used (i.e., the distance formula chosen). The most common is called the \(L_2\) norm, which is computed according to the distance formula you are probably familiar with from grade school. The \(L_2\) norm of a vector \(v\) is denoted by \(\Vert v \Vert_{2}\) and \(\Vert v \Vert_{2} = \sqrt{\sum_i v_i^2}\) . This is sometimes also called Euclidian length and refers to the âphysicalâ length of a vector in one-, two-, or three-dimensional space. The \(L_1\) norm, or âManhattan Distance,â is computed as \(\Vert v \Vert_{1} = \sum_i |v_i|\) , and is named after the grid-like road structure in New York City. In general, the p-norm , \(L_p\) , of a vector is \(\Vert v \Vert_{p} = \sqrt[p]{(\sum_i v_i^p)}\) . The \(L_\infty\) norm is the \(p\) -norm, where \(p = \infty\) . The \(L_\infty\) norm is written as \(||v||_\infty\) and it is equal to the maximum absolute value in \(v\) .
TRY IT! Create a row vector and column vector, and show the shape of the vectors.
Note! In Python, the row vector and column vector are a little bit tricky. You can see from the above in order to get the 1 row and 4 columns or 4 rows and 1 column vectors, we have to use list of list to specify it. You can define np.array([1,2,3,4]), but you will soon notice that it doesnât contain information about row or column.
TRY IT! Transpose the row vector we defined above into a column vector and calculate the \(L_1\) , \(L_2\) , and \(L_\infty\) norm of it. Verify that the \(L_\infty\) norm of a vector is equivalent to the maximum value of the elements in the vector.
Vector addition is defined as the pairwise addition of each of the elements of the added vectors. For example, if \(v\) and \(w\) are vectors in \({\mathbb{R}}^n\) , then \(u = v + w\) is defined as \(u_i = v_i + w_i\) .
Vector multiplication can be defined in several ways depending on the context. Scalar multiplication of a vector is the product of a vector and a scalar (i.e., a number in \({\mathbb{R}}\) ). Scalar multiplication is defined as the product of each element of the vector by the scalar. More specifically, if \(\alpha\) is a scalar and \(v\) is a vector, then \(u = \alpha v\) is defined as \(u_i = \alpha v_i\) . Note that this is exactly how Python implements scalar multiplication with a vector.
TRY IT! Show that \(a(v + w) = av + aw\) (i.e., scalar multiplication of a vector distributes across vector addition).
By vector addition, \(u = v + w\) is the vector with \(u_i = v_i + w_i\) . By scalar multiplication of a vector, \(x = \alpha u\) is the vector with \(x_i = \alpha(v_i + w_i)\) . Since \(\alpha, v_i\) , and \(w_i\) are scalars, multiplication distributes and \(x_i = \alpha v_i + \alpha w_i\) . Therefore, \(a(v + w) = av + aw\) .
The dot product of two vectors is the sum of the product of the respective elements in each vector and is denoted by \(\cdot\) , and \(v \cdot w\) is read âv dot w.â Therefore for \(v\) and \(w\) \(\in {\mathbb{R}}^n, d = v\cdot w\) is defined as \(d = \sum_{i = 1}^{n} v_iw_i\) . The angle between two vectors , \(\theta\) , is defined by the formula:
The dot product is a measure of how similarly directed the two vectors are. For example, the vectors (1,1) and (2,2) are parallel. If you compute the angle between them using the dot product, you will find that \(\theta = 0\) . If the angle between the vectors, \(\theta = \pi/2\) , then the vectors are said to be perpendicular or orthogonal , and the dot product is 0.
TRY IT! Compute the angle between the vectors \(v = [10, 9, 3]\) and \(w = [2, 5, 12]\) .
Finally, the cross product between two vectors, \(v\) and \(w\) , is written \(v \times w\) . It is defined by \(v \times w = \Vert v \Vert_{2}\Vert w \Vert_{2}\sin{(\theta)} \textit{n}\) , where \(\theta\) is the angle between the \(v\) and \(w\) (which can be computed from the dot product) and \(n\) is a vector perpendicular to both \(v\) and \(w\) with unit length (i.e., the length is one). The geometric interpretation of the cross product is a vector perpendicular to both \(v\) and \(w\) with length equal to the area enclosed by the parallelogram created by the two vectors.
TRY IT! Given the vectors \(v = [0, 2, 0]\) and \(w = [3, 0, 0]\) , use the Numpy function cross to compute the cross product of v and w.
Assuming that \(S\) is a set in which addition and scalar multiplication are defined, a linear combination of \(S\) is defined as $ \( \sum \alpha_i s_i, \) $
where \(\alpha_i\) is any real number and \(s_i\) is the \(i^{\text{th}}\) object in \(S\) . Sometimes the \(\alpha_i\) values are called the coefficients of \(s_i\) . Linear combinations can be used to describe numerous things. For example, a grocery bill can be written \(\displaystyle{\sum c_i n_i}\) , where \(c_i\) is the cost of item \(i\) and \(n_i\) is the number of item \(i\) purchased. Thus, the total cost is a linear combination of the items purchased.
A set is called linearly independent if no object in the set can be written as a linear combination of the other objects in the set. For the purposes of this book, we will only consider the linear independence of a set of vectors. A set of vectors that is not linearly independent is linearly dependent .
TRY IT! Given the row vectors \(v = [0, 3, 2]\) , \(w = [4, 1, 1]\) , and \(u = [0, -2, 0]\) , write the vector \(x = [-8, -1, 4]\) as a linear combination of \(v\) , \(w\) , and \(u\) .
TRY IT! Determine by inspection whether the following set of vectors is linearly independent: \(v = [1, 1, 0]\) , \(w = [1, 0, 0]\) , \(u = [0, 0, 1]\) .
Clearly \(u\) is linearly independent from \(v\) and \(w\) because only \(u\) has a nonzero third element. The vectors \(v\) and \(w\) are also linearly independent because only \(v\) has a nonzero second element. Therefore, \(v, w\) , and \(u\) are linearly independent.
An \({m} \times {n}\) matrix is a rectangular table of numbers consisting of \(m\) rows and \(n\) columns. The norm of a matrix can be considered as a particular kind of vector norm, if we treat the \({m} \times {n}\) elements of \(M\) are the elements of an \(mn\) dimensional vector, then the p-norm of this vector can be write as:
You can calculate the matrix norm using the same norm function in Numpy as that for vector.
Matrix addition and scalar multiplication for matrices work the same way as for vectors. However, matrix multiplication between two matrices, \(P\) and \(Q\) , is defined when \(P\) is an \({m} \times {p}\) matrix and \(Q\) is a \({p} \times {n}\) matrix. The result of \(M = PQ\) is a matrix \(M\) that is \(m \times n\) . The dimension with size \(p\) is called the inner matrix dimension , and the inner matrix dimensions must match (i.e., the number of columns in \(P\) and the number of rows in \(Q\) must be the same) for matrix multiplication to be defined. The dimensions \(m\) and \(n\) are called the outer matrix dimensions . Formally, if \(P\) is \({m} \times {p}\) and Q is \({p} \times {n}\) , then \(M = PQ\) is defined as
The product of two matrices \(P\) and \(Q\) in Python is achieved by using the dot method in Numpy. The transpose of a matrix is a reversal of its rows with its columns. The transpose is denoted by a superscript, \(T\) , such as \(M^T\) is the transpose of matrix \(M\) . In Python, the method T for an Numpy array is used to get the transpose. For example, if \(M\) is a matrix, then \(M.T\) is its transpose.
TRY IT! Let the Python matrices \(P = [[1, 7], [2, 3], [5, 0]]\) and \(Q = [[2, 6, 3, 1], [1, 2, 3, 4]]\) . Compute the matrix product of \(P\) and \(Q\) . Show that the product of \(Q\) and \(P\) will produce an error.
A square matrix is an \({n} \times {n}\) matrix; that is, it has the same number of rows as columns. The determinant is an important property of square matrices. The determinant is denoted by \(det(M)\) , both in mathematics and in Numpyâs linalg package, sometimes it is also denoted as \(|M|\) . Some examples in the uses of a determinant will be described later.
In the case of a \(2 \times 2\) matrix, the determinant is:
Similarly, in the case of a \(3 \times 3\) matrix, the determinant is:
We can use similar approach to calculate the determinant for higher the dimension of the matrix, but it is much easier to calculate using Python. We will see an example below how to calculate the determinant in Python.
The identity matrix is a square matrix with ones on the diagonal and zeros elsewhere. The identity matrix is usually denoted by \(I\) , and is analagous to the real number identity, 1. That is, multiplying any matrix by \(I\) (of compatible size) will produce the same matrix.
TRY IT! Use Python to find the determinant of the matrix \(M = [[0, 2, 1, 3], [3, 2, 8, 1], [1, 0, 0, 3], [0, 3, 2, 1]]\) . Use the np.eye function to produce a \({4} \times {4}\) identity matrix, \(I\) . Multiply \(M\) by \(I\) to show that the result is \(M\) .
The inverse of a square matrix \(M\) is a matrix of the same size, \(N\) , such that \(M \cdot N = I\) . The inverse of a matrix is analagous to the inverse of real numbers. For example, the inverse of 3 is \(\frac{1}{3}\) because \((3)(\frac{1}{3}) = 1\) . A matrix is said to be invertible if it has an inverse. The inverse of a matrix is unique; that is, for an invertible matrix, there is only one inverse for that matrix. If \(M\) is a square matrix, its inverse is denoted by \(M^{-1}\) in mathematics, and it can be computed in Python using the function inv from Numpyâs linalg package.
For a \(2 \times 2\) matrix, the analytic solution of the matrix inverse is:
The calculation of the matrix inverse for the analytic solution becomes complicated with increasing matrix dimension, there are many other methods can make things easier, such as Gaussian elimination, Newtonâs method, Eigendecomposition and so on. We will introduce some of these methods after we learn how to solve a system of linear equations, because the process is essentially the same.
Recall that 0 has no inverse for multiplication in the real-numbers setting. Similarly, there are matrices that do not have inverses. These matrices are called singular . Matrices that do have an inverse are called nonsingular .
One way to determine if a matrix is singular is by computing its determinant. If the determinant is 0, then the matrix is singular; if not, the matrix is nonsingular.
TRY IT! The matrix \(M\) (in the previous example) has a nonzero determinant. Compute the inverse of \(M\) . Show that the matrix \(P = [[0, 1, 0], [0, 0, 0], [1, 0, 1]]\) has a determinant value of 0 and therefore has no inverse.
A matrix that is close to being singular (i.e., the determinant is close to 0) is called ill-conditioned . Although ill-conditioned matrices have inverses, they are problematic numerically in the same way that dividing a number by a very, very small number is problematic. That is, it can result in computations that result in overflow, underflow, or numbers small enough to result in significant round-off errors (If you forget these concepts, refresh yourself with materials in chapter 9). The condition number is a measure of how ill-conditioned a matrix is, and it can be computed using Numpyâs function cond from linalg . The higher the condition number, the closer the matrix is to being singular.
The rank . of an \({m} \times {n}\) matrix \(A\) is the number of linearly independent columns or rows of \(A\) , and is denoted by rank( \(A\) ). It can be shown that the number of linearly independent rows is always equal to the number of linearly independent columns for any matrix. A matrix is called full rank . if rank \((A)=\min(m,n)\) . The matrix, \(A\) , is also full rank if all of its columns are linearly independent. An augmented matrix . is a matrix, \(A\) , concatenated with a vector, \(y\) , and is written \([A,y]\) . This is commonly read â \(A\) augmented with \(y\) .â You can use np.concatenate to concatenate the them. If \(rank([A,y]) = {rank}(A) + 1\) , then the vector, \(y\) , is ânewâ information. That is, it cannot be created as a linear combination of the columns in \(A\) . The rank is an important property of matrices because of its relationship to solutions of linear equations, which is discussed in the last section of this chapter.
TRY IT! Matrix \(A = [[1, 1, 0], [0, 1, 0], [1, 0, 1]]\) , compute the condition number and rank for this matrix. If \(y = [[1], [2], [1]]\) , get the augmented matrix [A, y].
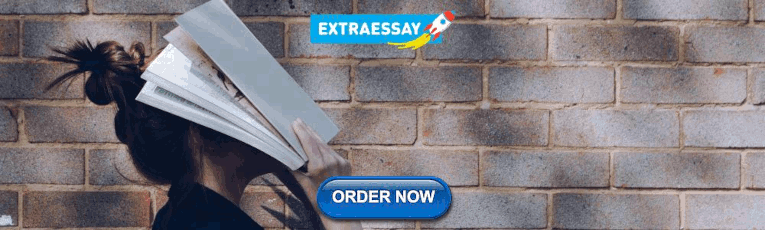
IMAGES
VIDEO
COMMENTS
Solution Help. Hungarian method calculator. 1. A computer centre has 3expert programmers. The centre wants 3 application programmes to be developed. The head of thecomputer centre, after studying carefully the programmes to be developed, estimates the computer time in minutes required by the experts for the application programmes as follows.
Linear Programming. Linear programming solver with up to 9 variables. New constraints could be added by using commas to separate them. Get the free "Linear Programming Solver" widget for your website, blog, Wordpress, Blogger, or iGoogle. Find more Mathematics widgets in Wolfram|Alpha.
Solve an assignment problem online. Fill in the cost matrix of an assignment problem and click on 'Solve'. The optimal assignment will be determined and a step by step explanation of the hungarian algorithm will be given. Fill in the cost matrix (random cost matrix):
Operation Research calculators - Solve linear programming problems of Operations Research, step-by-step online. ... 1.1 Balanced Assignment Problem (Using Hungarian method) 1. A department has five employess with five jobs to be permormed. The time (in hours) each men will take to perform each job is given in the effectiveness matrix. ...
linear programming calculator. en. Related Symbolab blog posts. Practice, practice, practice ... Each new topic we learn has symbols and problems we have never seen. The unknowing... Enter a problem. Cooking Calculators. Cooking Measurement Converter Cooking Ingredient Converter Cake Pan Converter More calculators. Fitness Calculators.
The use of our calculator is very simple and intuitive, however, we will explain its use step by step: Before starting, you must have made the approach of the model to be optimized. Remember that for the graphical method we normally work with 2 decision variables. You must enter the coefficients of the objective function and the constraints.
Linear Programming Calculator + Online Solver With Free Steps. Linear Programming Calculator is a free online calculator that provides the best optimal solution for the given mathematical model.. This online calculator solves the problem of finding the correct solution or optimized output of the desired mathematical models by providing a quick, reliable, and accurate solution.
Big M Method Calculator Online - Linear Programming đ„. Solve your linear programming exercises with the big M method calculator online automatically and easily with our online calculator. .
The program uses the linear assignment solver, a specialized solver for the assignment problem. The following code creates the solver. Note: The linear sum assignment solver only accepts integer values for the weights and values. The section Using a solver with non-integer data shows how to use the solver if your data contains non-integer values.
Solve linear programming problems in the browser. Find optimal values of variables based on their attributes, constraints and optimization objective. Under the hood a linear programming solver finds a solution of a system of linear equations. With StatSim Solve you can solve linear programming problems online without writing code.
2-LAP: Linear Assignment Problem; 2-OSP: One machine Scheduling Problem; 2-UKP: binary Unidimensional knapsack problem; ... GLPK: stands for GNU Linear Programming Kit, an open source solver; Clp/Cbc : an open source solver (for LP and MILP respectively) from the COIN-OR project;
Assignment Problem. A problem that requires pairing two sets of items given a set of paired costs or profit in such a way that the total cost of the pairings is minimized or maximized. The assignment problem is a special case of linear programming. For example, an operation manager needs to assign four jobs to four machines.
It shows how to solve an assignment linear programming model in Solver Add-in in Excel. It finds the optimum assignment of multiple tasks to multiple workers...
A simple calculator and some simple steps to use it. Follow the below-mentioned procedure to use the Linear Programming Calculator at its best. Step 1: Enter the Objective Function into the input bar. Step 2: Enter the constraints into the respective input bar. Step 3: Click on the "Optimize the Function" button.
Small Linear Programming Problem. Consider the following linear programming problem: You need to find x and y such that the red, blue, and yellow inequalities, as well as the inequalities x â„ 0 and y â„ 0, are satisfied. At the same time, your solution must correspond to the largest possible value of z.
The assignment problem is a special case of the transportation problem, which is a special case of the minimum cost flow problem, which in turn is a special case of a linear program. While it is possible to solve any of these problems using the simplex algorithm , each specialization has a smaller solution space and thus more efficient ...
When trying to solve for assignments given a cost matrix, what is the difference between. using Scipy's linear_sum_assignment function (which I think uses the Hungarian method). describing the LP problem using a objective function with many boolean variables, add in the appropriate constraints and send it to a solver, such as through scipy.optimize.linprog?
Explore math with our beautiful, free online graphing calculator. Graph functions, plot points, visualize algebraic equations, add sliders, animate graphs, and more. Linear Programming Project Graph | Desmos
The procedure to use the linear programming calculator is as follows: Step 1: Enter the objective function, constraints in the respective input field. Step 2: Now click the button "Submit" to get the optimal solution. Step 3: Finally, the best optimal solution and the graph will be displayed in the new window.
The transportation problem is a special linear programming problem. This calculator finds the initial solution by the North-West Corner Method or the Least Cost Method. If necessary the initial solution will be improved by the MODI method. The solution is accompanied by a large number of illustrations. You can solve your problem or see examples ...
In which we introduce linear programming. 1 Linear Programming A linear program is an optimization problem in which we have a collection of variables, which can take real values, and we want to nd an assignment of values to the variables that satis es a given collection of linear inequalities and that maximizes or minimizes a given linear function.
The linear assigment problems with the objecttive of maximizing profit or minimizing cost can be solved with this calculator. For example, you want to hire three workers to do three tasks. The cost/task of the workers is shown in the table below. You want to do the worker-task assignment in such a way that minimize the total cost. Solving this ...
Linear Programming Problems. Linear Programming Problems (LPP) involve optimizing a linear function to find the optimal value solution for the function.The optimal value can be either the maximum value or the minimum value. In LPP, the linear functions are called objective functions.An objective function can have multiple variables, which are subjected to conditions and have to satisfy the ...
The rank. of an m Ă n matrix A is the number of linearly independent columns or rows of A, and is denoted by rank ( A ). It can be shown that the number of linearly independent rows is always equal to the number of linearly independent columns for any matrix. A matrix is called full rank. if rank (A) = min (m, n).