
- SUGGESTED TOPICS
- The Magazine
- Newsletters
- Managing Yourself
- Managing Teams
- Work-life Balance
- The Big Idea
- Data & Visuals
- Reading Lists
- Case Selections
- HBR Learning
- Topic Feeds
- Account Settings
- Email Preferences
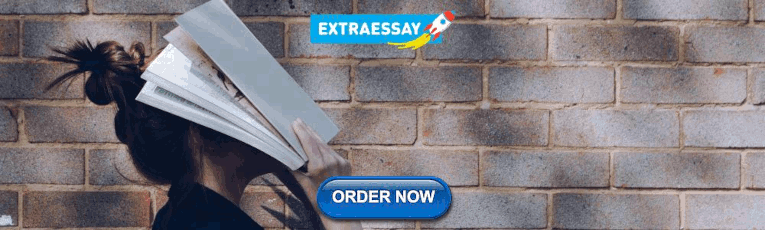
The Power of Healthy Relationships at Work
- Emma Seppälä
- Nicole K. McNichols

Five research-backed principles to cultivate stronger workplace relationships.
Research shows that leaders who prioritize relationships with their employees and lead from a place of positivity and kindness simply do better, and company culture has a bigger influence on employee well-being than salary and benefits. When it comes to cultivating happiness at work, it comes down to fostering positive relationships at work. Citing research from the field of social psychology, the authors outline five core principles that make all relationships, personal or professional, thrive: 1) transparency and authenticity, 2) inspiration, 3) emotional intelligence, 4) self-care, and 5) values.
Kushal Choksi was a successful Wall Street quant who had just entered the doors of the second twin tower on 9/11 when it got hit. As Choksi describes in his best-selling book, On a Wing and a Prayer , his brush with death was a wakeup call. Having mainly focused on wealth acquisition before 9/11, he began to question his approach to work.
- Emma Seppälä , PhD, is a faculty member at the Yale School of Management, faculty director of the Yale School of Management’s Women’s Leadership Program and bestselling author of SOVEREIGN (2024) and The Happiness Track (2017). She is also science director of Stanford University’s Center for Compassion and Altruism Research and Education . Follow her work at emmaseppala.com , http://www.iamsov.com or on Instagram . emmaseppala
- Nicole K. McNichols Ph.D. is an Associate Teaching Professor in the Department of Psychology at the University of Washington where she teaches courses about sex and relationship science in addition to industrial and organizational psychology. Follow her work at www.nicolethesexprofessor.com and on Instagram .
Partner Center
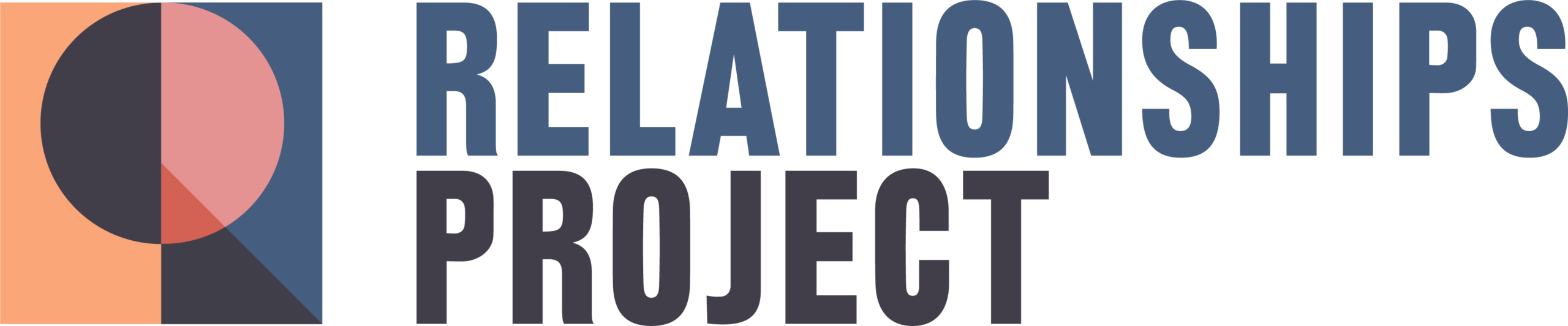
Putting Relationships First
The case for relationship-centred communities, organisations and systems.
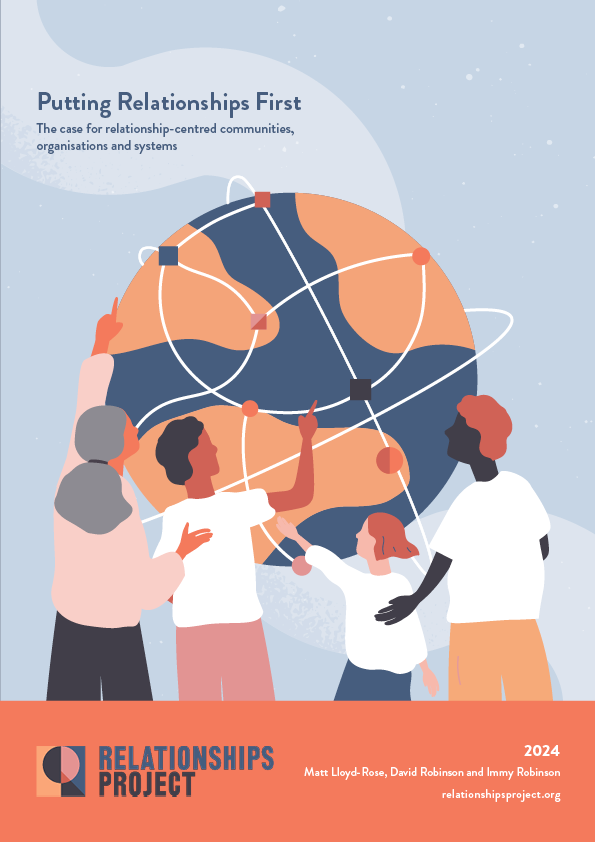
To say that human beings require warm relationships is no touchy-feely idea. It is hard fact. We need nutrition, we need exercise, we need purpose, and we need each other.
Few people argue that relationships don’t matter, but many feel they don’t have the time, capacity or permission to prioritise them. This Case Maker assembles the evidence base for putting relationships first, describing why relationships matter, what great relationship-centred practice looks like, and how it could make an impact in your context.
Use it to…
- Deepen your understanding of the difference that putting relationships first makes in a range of different contexts and scales
- Develop your own narrative of why more investment in relationships will lead to better outcomes in your community or organisation
- Start a discussion and build awareness around the value of relationship-centred practice amongst your team
Rewatch the Launch
On 22nd April Elizabeth Oldfield and Matt Lloyd-Rose were joined by a few of the brilliant people who shared their stories in the Case Maker, namely Sarah Yardley, Christine Frazer and Russ Bellenie. It was a warm, lively and engaging conversation, elevated by some brilliant questions from those who joined us.
In the Case Maker you’ll find…
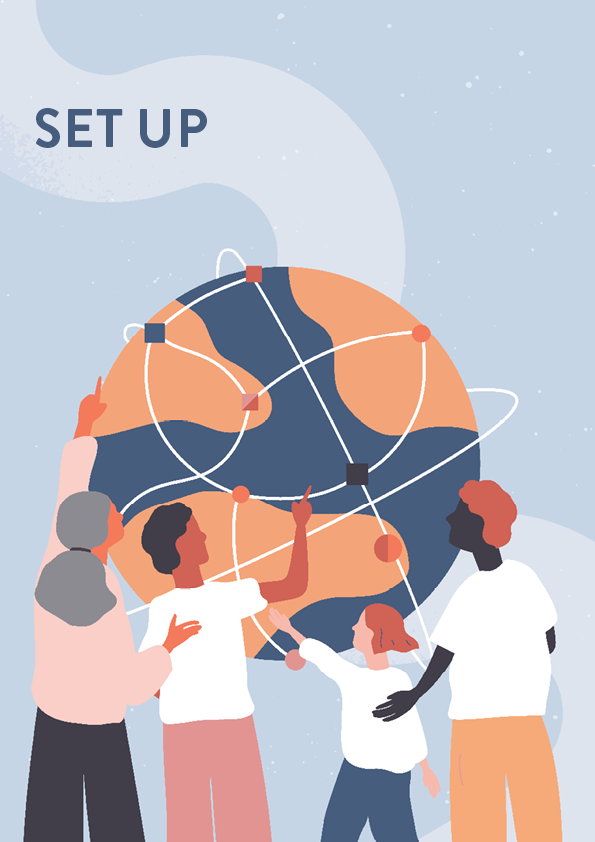
What we mean by relationship-centred practice
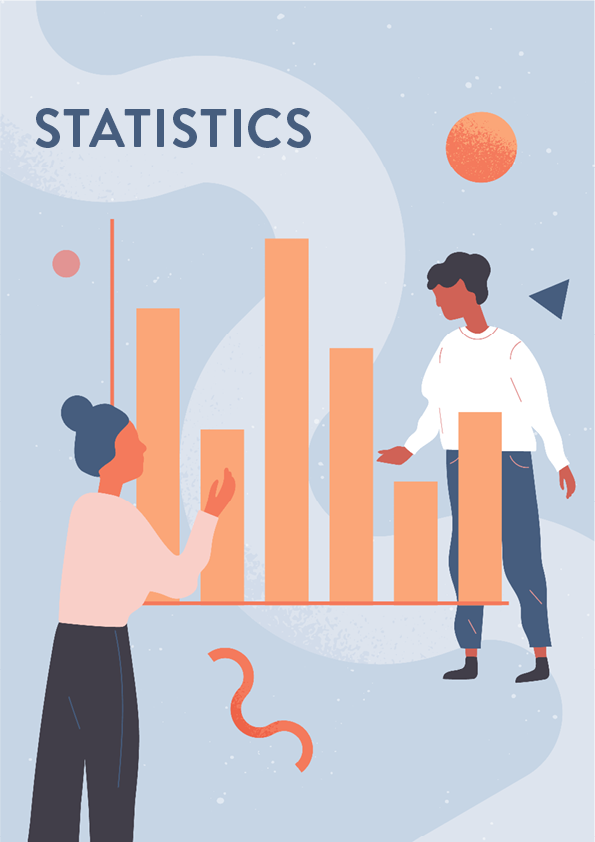
The impact of investing in relationships
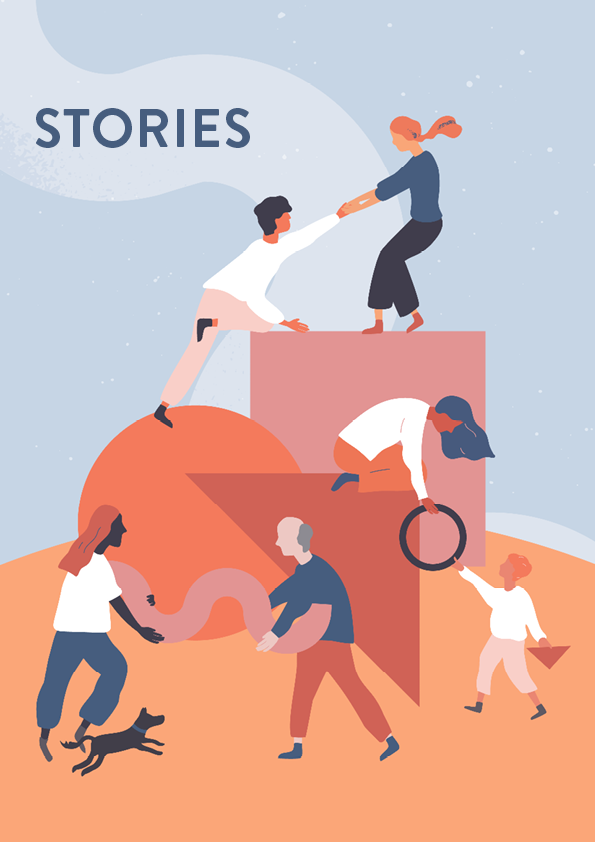
Relationship-centred practice in action
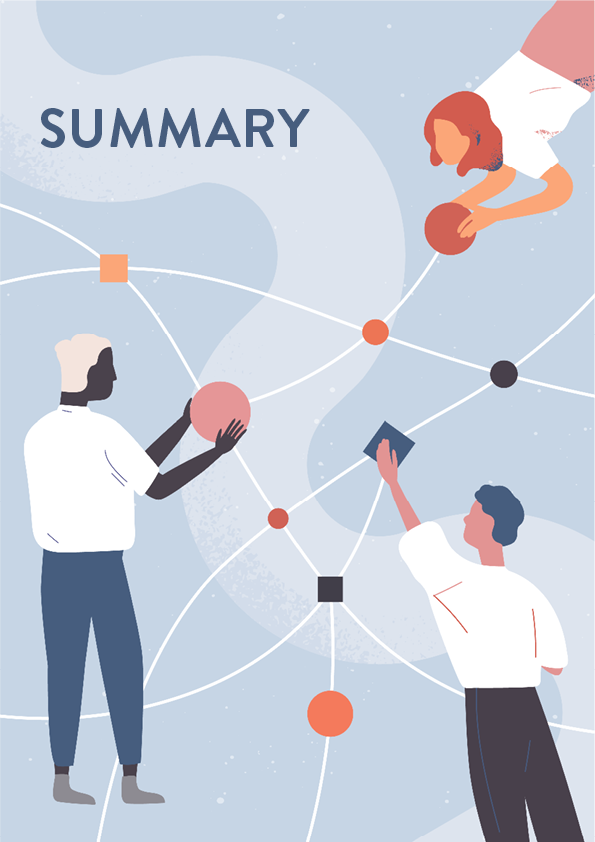
Making your own case for relationships
Better relationships, better outcomes
Good relationships are good for us, we feel better, we live longer and we contribute more. There is no task in adult life, public or personal, which is not done better with an enhanced understanding of relational skills – how to collaborate, how to manage bias and power and parity of esteem, how to forgive, negotiate difference and resolve conflict. Everything works better when we do these things well.
Life expectancy
148 studies examining mortality rates across all age groups, genders and ethnicities show that strong connection increases the likelihood of surviving in any given year by more than 50% ( Holt-Lunstad et al, 2010 )
Reoffending rates
Studies have consistently found that prisoners who maintain close contact with their family members while incarcerated have better post-release outcomes and lower recidivism rates ( Friedmann, 2014 )
Disaster survival
Professor Aldrich has found that the death rate following the 2011 Japan tsunami was up to ten times lower where social connection was strongest, and this was more significant than the height of the sea wall and the height of the wave ( Aldrich, 2023 )
Hospital admissions
In Frome, work on building social networks has led to a 14% reduction in hospital admissions, compared to a national increase of 28%, resulting in a 21% reduction in costs ( Abel et al, 2018 )
Cancer recovery
A longitudinal study of 2,835 women with breast cancer found that those with a network of good relationships were four times more likely to survive than those without ( Kroenke et al, 2006 )
Waiting times
Great Yarmouth Council has reduced waiting lists by 95% by moving away from a standardised model to one in which the council holds individual conversations ( The Guardian, 10th April 2018 )
Medical compliance
A review of over 1,000 scientific abstracts and 280 research manuscripts found that when healthcare providers demonstrate compassion, medication adherence increases by up to 80% and healthcare spending reduces by up to 51% ( Trzeciak and Mazzarelli, 2019 )
Burnout rates
8/10 studies included in a systematic review found a negative association between burnout and empathy, indicating that providing empathy helps reduce burnout rates amongst healthcare professionals ( Wilkinson et al, 2017 )
Relationship-Centred Practice in action
Across the UK and beyond, there are many brilliant examples of relationships making a difference. We’ve compiled a set of stories which illustrate what can happen when we put relationships first. The stories represent different scales, sectors, geographies and types of organisation: from business to community group to charity to large state institution. They are not templates to follow, but rather accounts of some of the relational work that has inspired us. Between them they exemplify many of the key themes, practices and possibilities in relational working, as well as many common barriers and challenges.
We hope these stories provoke thoughts, spark ideas and push the boundaries of what you think is possible.
Find out how we wrote the stories
How did we choose these examples?
We approached people and organisations we have encountered through the work of the Relationships Project to date, including some who had added themselves to the Relationships Map .
Our aim was to give a sense of how relational work looks at different scales and in different contexts.
How did we create these stories?
We joined forces with author and social researcher Matt Lloyd-Rose who conducted semi-structured interviews with people closely involved with each story, asking them to describe the role of relationships in the work they do.
The stories are based on a combination of desk research and the transcripts of those interviews. Interviewees reviewed their story to check it was fair and accurate before they were finalised.
I don’t work in any of these sectors. Are these relevant for me?
Sometimes the most exciting ideas come from sectors and contexts other than our own. We hope you find inspiration and provocation in all of these stories, regardless of whether they reflect the specific work you do.
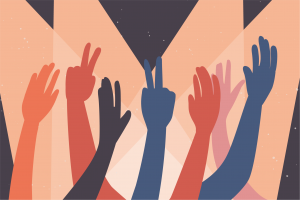
Addressing entrenched social issues relationally not reactively
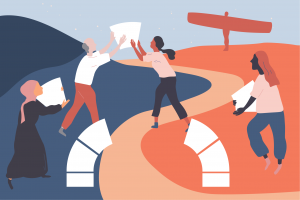
Gateshead Community Bridgebuilders
Empowering communities through devolved decision-making
Narrated by Emily Blad
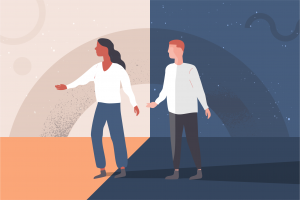
Transforming the lives of prison leavers through reliable relationships
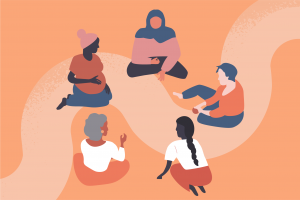
Building social integration through shared ritual and mutual learning
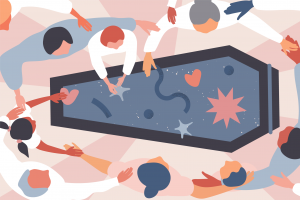
Bringing a more flexible, relational approach to the deathcare sector
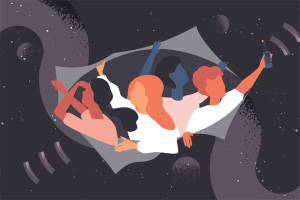
Connecting artists and communities to spark radical imagination
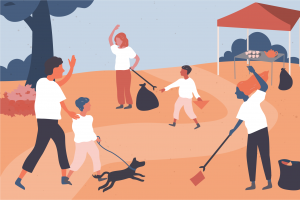
Three Community Groups
Tackling local issues by creating spaces and excuses to connect
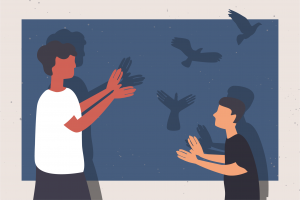
Barking and Deganham's Children's Social Services
Returning social care to its radical, relational roots
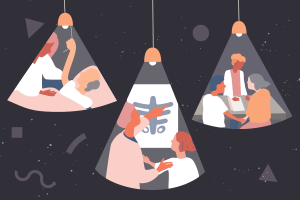
NHS Palliative Care
Pursuing results through relationships in a large, complex system
The Relationships Map
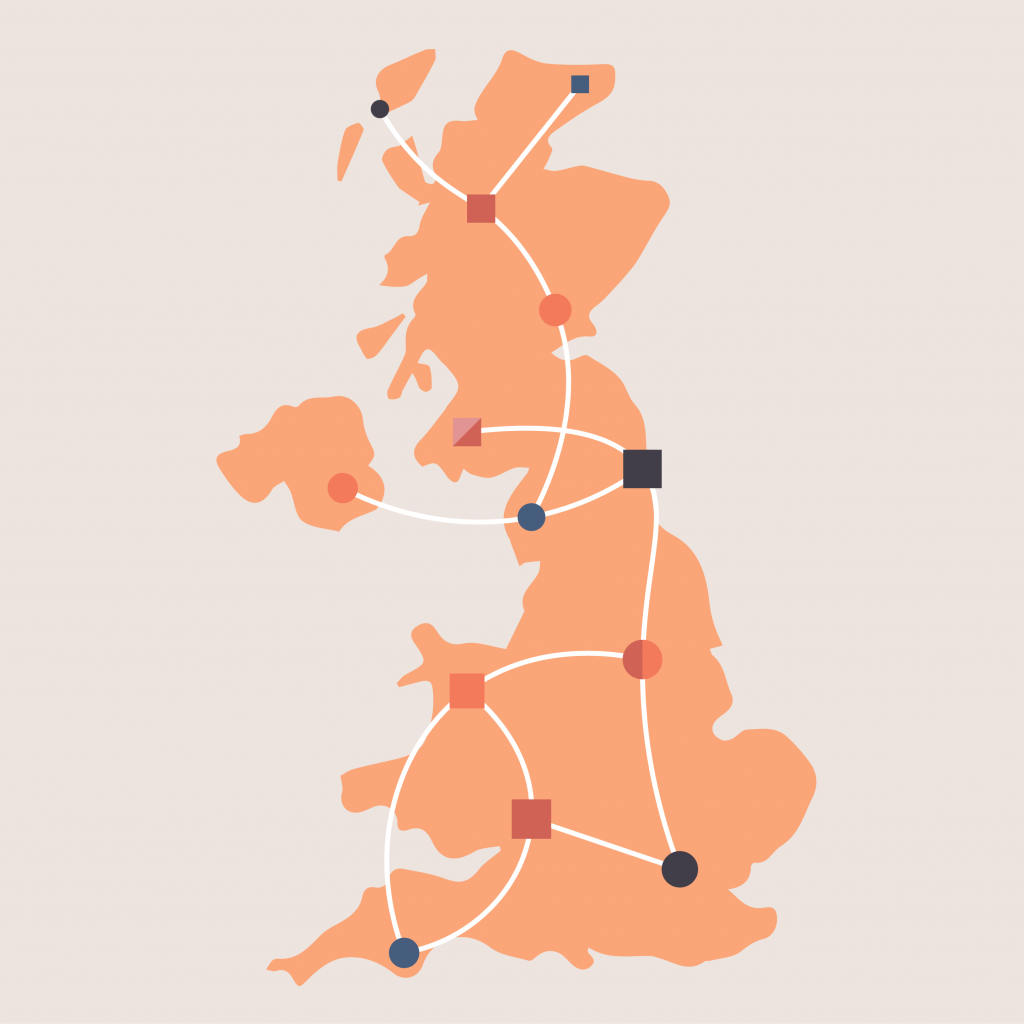
We think that the stories told in this Case Maker are good stories, full of hope and kindness and a special kind of ‘can-do’ spirit, but they are not islands. All over the UK and indeed further afield, relational practice is gathering momentum. The contexts are diverse, as they are here in the Case Maker, but the driving principles are consistent.
On the Relationships Map we have begun to plot the organisations and individuals who are putting relationships first. The Map is more than a directory. It’s a meeting place; a space for finding one another and sharing ideas and resources. Please put your story on the Map, find kindred spirits and help to spread the word.
Making your case
We hope, having engaged with the statistics and stories in this Case Maker, that you’re convinced that everything works better when relationships are put first. And we hope that you feel better equipped to make the case to your colleagues, board members and commissioners that everything works better when relationships are valued.
Here are a few things you can do to turn common sense into common practice in your community, organisation or system…
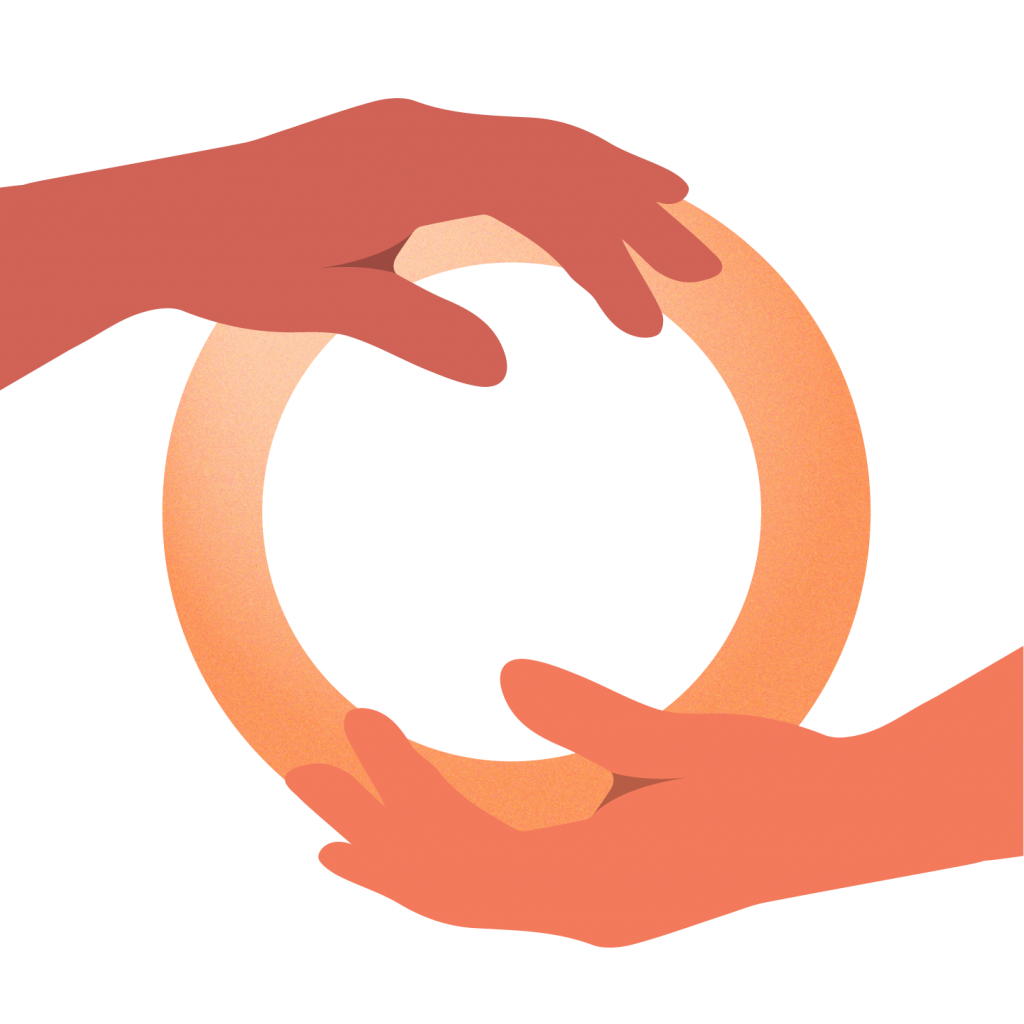
Spread the word
Share the Case Maker and help to spread the word
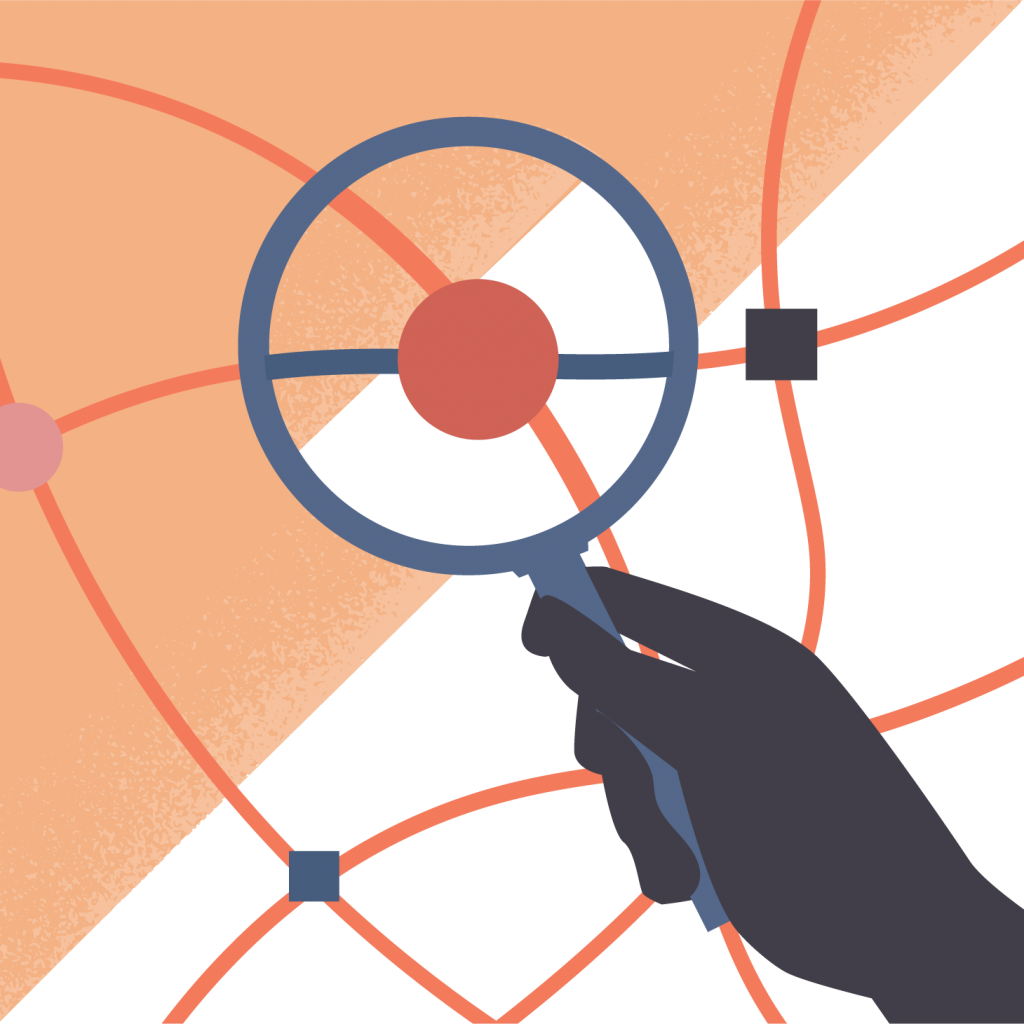
Make your own case
Use the DIY Case Maker to craft your own case, or come along to workshop
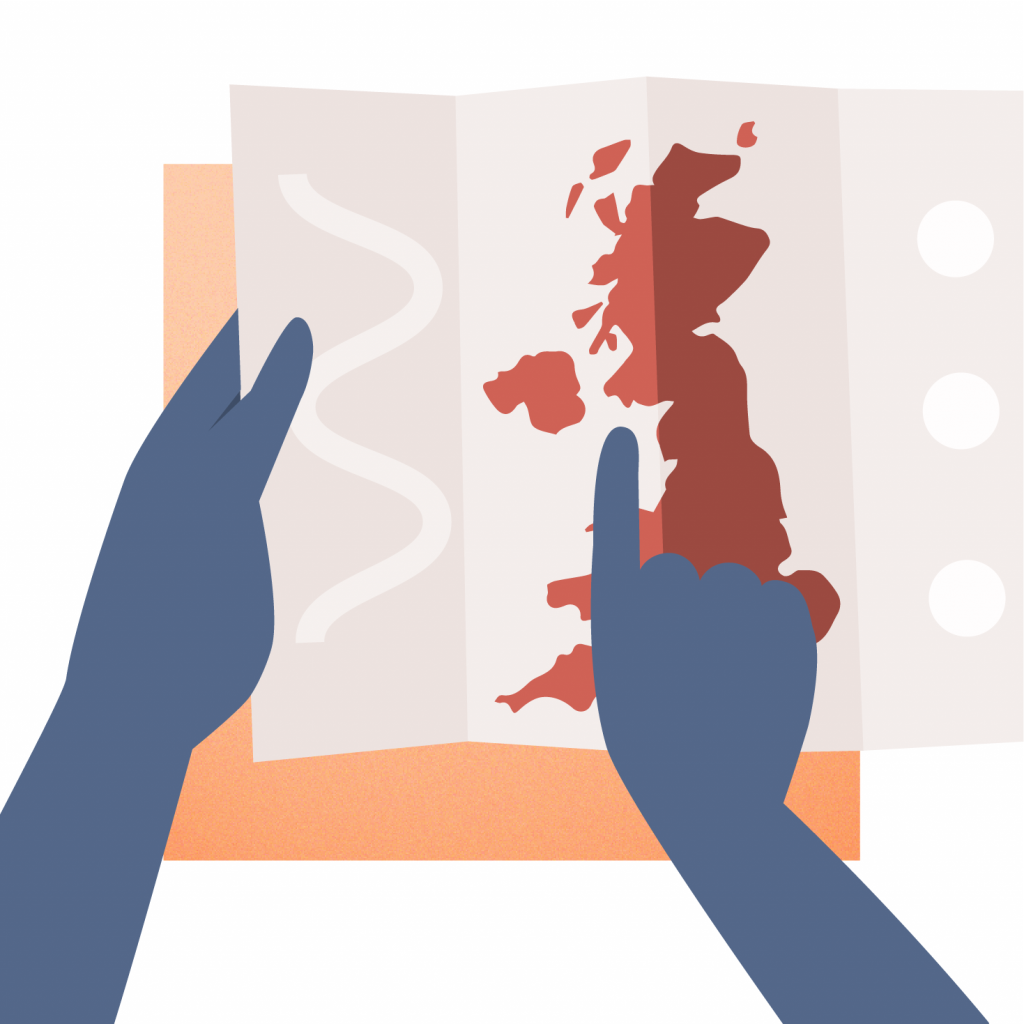
Join the Map
Connect with other relationship-centred practitioners and share your story
Sign up for news and updates
Join our mailing list to keep up to date with the work we're doing, get notified about new reports and tools and hear about upcoming events.
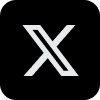
The Weekly “Connect” Meeting
Case study: relationship building.
Share this page
- Share this page on Facebook.
- Share this page on Twitter.
- Share this page on LinkedIn.
- Share this page via email.
- Print this page.
In this section of the module, there are videos demonstrating one way of conducting the “Connect” meeting. Each screen also includes information about what is demonstrated in that video.
Relationship building
As a mentor, being open and receptive to what your students are saying — even when they’re frustrated — can help strengthen your relationship because it shows that you trust them and value their opinions . This mentor maintains a professional approach by showing compassion for the student’s frustration without taking a side.
Icon(s) used on this page:
Thank you for visiting nature.com. You are using a browser version with limited support for CSS. To obtain the best experience, we recommend you use a more up to date browser (or turn off compatibility mode in Internet Explorer). In the meantime, to ensure continued support, we are displaying the site without styles and JavaScript.
- View all journals
- My Account Login
- Explore content
- About the journal
- Publish with us
- Sign up for alerts
- Open access
- Published: 06 May 2021
Interpersonal relationships drive successful team science: an exemplary case-based study
- Hannah B. Love ORCID: orcid.org/0000-0003-0011-1328 1 ,
- Jennifer E. Cross ORCID: orcid.org/0000-0002-5582-4192 2 ,
- Bailey Fosdick ORCID: orcid.org/0000-0003-3736-2219 2 ,
- Kevin R. Crooks 2 ,
- Susan VandeWoude 2 &
- Ellen R. Fisher 3
Humanities and Social Sciences Communications volume 8 , Article number: 106 ( 2021 ) Cite this article
9316 Accesses
13 Citations
3 Altmetric
Metrics details
- Complex networks
- Science, technology and society
Scientists are increasingly charged with solving complex societal, health, and environmental problems. These systemic problems require teams of expert scientists to tackle research questions through collaboration, coordination, creation of shared terminology, and complex social and intellectual processes. Despite the essential need for such interdisciplinary interactions, little research has examined the impact of scientific team support measures like training, facilitation, team building, and expertise. The literature is clear that solving complex problems requires more than contributory expertise, expertise required to contribute to a field or discipline. It also requires interactional expertise, socialised knowledge that includes socialisation into the practices of an expert group. These forms of expertise are often tacit and therefore difficult to access, and studies about how they are intertwined are nearly non-existent. Most of the published work in this area utilises archival data analysis, not individual team behaviour and assessment. This study addresses the call of numerous studies to use mixed-methods and social network analysis to investigate scientific team formation and success. This longitudinal case-based study evaluates the following question: How are scientific productivity, advice, and mentoring networks intertwined on a successful interdisciplinary scientific team? This study used applied social network surveys, participant observation, focus groups, interviews, and historical social network data to assess this specific team and assessed processes and practices to train new scientists over a 15-year period. Four major implications arose from our analysis: (1) interactional expertise and contributory expertise are intertwined in the process of scientific discovery; (2) team size and interdisciplinary knowledge effectively and efficiently train early career scientists; (3) integration of teaching/training, research/discovery, and extension/engagement enhances outcomes; and, (4) interdisciplinary scientific progress benefits significantly when interpersonal relationships among scientists from diverse disciplines are formed. This case-based study increases understanding of the development and processes of an exemplary team and provides valuable insights about interactions that enhance scientific expertise to train interdisciplinary scientists.
Similar content being viewed by others
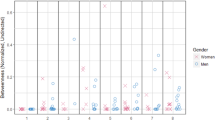
Towards understanding the characteristics of successful and unsuccessful collaborations: a case-based team science study
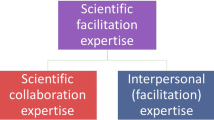
Science facilitation: navigating the intersection of intellectual and interpersonal expertise in scientific collaboration
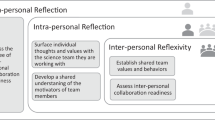
A framework for developing team science expertise using a reflective-reflexive design method (R2DM)
Introduction.
Scientists are increasingly charged with solving complex and large-scale societal, health, and environmental challenges (Read et al., 2016 ; Stokols et al., 2008 ). These systemic problems require interdisciplinary teams to tackle research questions through collaboration, coordination, creation of shared terminology, and complex social and intellectual processes (Barge and Shockley-Zalabak, 2008 ; De Montjoye et al., 2014 ; Fiore, 2008 ). Thus, to successfully approach complex research questions, scientific teams must synthesise knowledge from different disciplines, create a shared terminology, and engage members of a diverse research community (Matthews et al., 2019 ; Read et al., 2016 ). Despite significant time, energy, and money spent on collaboration and interdisciplinary projects, little research has examined the impact of scientific team support measures like training, facilitation, team building, and team performance metrics (Falk-Krzesinski et al., 2011 ; Klein et al., 2009 ).
Studies examining the development of scientific teaming skills that result in successful outcomes are sparse (Fiore, 2008 ; Hall et al., 2018 ; Wooten et al., 2014 ). The earliest studies of collaboration in science used bibliometric data to search for predictors of team success such as team diversity, size, geographical proximity, inter-university collaboration, and repeat collaborations (Borner et al., 2010 ; Cummings and Kiesler, 2008 ; Wuchty et al., 2007 ). Building from these studies, current research focuses on team processes. Literature suggests that to successfully frame a scientific problem, a team must also engage emotionally and interact effectively (Boix Mansilla et al., 2016 ) and that scientific collaboration involve consideration of the process, collaborator, human capital, and other factors that define an scientific collaboration (Bozeman et al., 2013 ; Hall et al., 2019 ; Lee and Bozeman, 2005 ). Similarly, Zhang et al. ( 2020 ) used social network analysis to examine how emotional intelligence is transmitted to team outcomes through team processes. Still more research is needed, and Hall et al. ( 2018 ) called for team science studies that use longitudinal designs and mixed-methods to examine project teams as they develop in order to move beyond bibliometric measures of success and to explore the complex, interacting features in real-world teams.
Fiore ( 2008 ) explained that much of what we know about the science of team science (SciTS), training scientists and team learning in productive team interactions, is anecdotal and not the result of systematic investigation (Fiore, 2008 ). Over a decade later there is still a paucity of research on how scientific teams develop the type of expertise they need to create new knowledge and further scientific discovery (Bammer et al., 2020 ). Bammer et al. ( 2020 ) has identified and defined two types of expertise: (1) contributory expertise, expertise required to make a contribution to a field or discipline (Collins and Evans, 2007 ); and (2) interactional expertise, socialised knowledge that includes socialisation into the practices of an expert group (Bammer et al., 2020 ). These forms of expertise are often tacit, codified by “learning-by-doing,” and augmented from project to project; therefore, they are difficult to measure and rarely documented in literature (Bammer et al., 2020 ).
Wooten et al. ( 2014 ) outlined three types of evaluations—developmental, process, and outcome—needed to understand how teams develop and to provide information about their future success (Wooten et al., 2014 ). A developmental evaluation focuses on the continuous process of team development, and a process evaluation focuses on team interactions, meetings, and engagement (Patton, 2011 ). Both development and process evaluations have the common goal of understanding the team’s future success or failures, also known as the team’s outcomes (e.g., grants, publications, and awards) (Patton, 2011 ). The majority of published work on outcome metrics is evaluated by archival data analysis, not individual team behaviour and assessment (Hall et al., 2018 ). Albeit informative, these studies are based upon limited outcome metrics such as publications and represent only a selective sampling of teams that have achieved success. To collect these three types of evaluation data, it is recommended to engage mixed-methods research such as a combination of social network analysis (SNA), participant observation, surveys, and interviews, although these approaches have not been widely employed (Bennett, 2011 ; Borner et al., 2010 ; Fiore, 2008 ; Hall et al., 2018 ; Wooten et al., 2014 ).
A few key studies have provided insight into successful collaboration strategies. Duhigg ( 2016 ) found that successful teams provided psychological safety, had dependable team members, and relied upon clear roles and structures. In addition, successful teams had meaningful goals, and team members felt like they could make an impact through their work on the team (Duhigg, 2016 ). Similarly, Collins ( 2001 ) explained that in business teams, moving from “Good to Great” required more than selecting the right people; the team needed development and training to achieve their goals (Collins, 2001 ). Woolley et al. ( 2010 ) found that it is not collective intelligence that builds the most effective teams, but rather, how teams interact that predicts their success (Woolley et al., 2010 ). The three traits they identified as most associated with team success included even turn-taking, social sensitivity, and proportion female (when women’s representation nears parity with men) (Woolley et al., 2010 ). Finally, Bammer et al. ( 2020 ) recommended creating a knowledge bank to strengthen knowledge about contributary and interactional expertise in scientific literature to solve complex problems. Collectively, these studies argue that the key to collective intelligence is highly reliant on interpersonal relationships to drive team success.
This article reports on a longitudinal case-based study of an exemplary interdisciplinary scientific team that has been successful in typical scientific outputs, including competing for research awards, publishing academic articles, and training and developing scientists. This analysis examines how scientific productivity, advice, and mentoring networks intertwined to promote team success. The study highlights how the team’s processes to train scientists (e.g., developing mentoring and advice networks) have propelled their scientific productivity, fulfilled the University’s land grant mission (i.e., emphasises research/discovery; education/training; and outreach/engagement) and created contributory and interactional expertise on the team. Team dynamics were evaluated by social network surveys, participant observation, focus groups, interviews, and historical social network data over 15 years to develop theory and evaluate complex relationships contributing to team success (Dozier et al., 2014 ; Greenwood, 1993 ).
Case study selection
The [BLIND] Science of team science (SciTS) team consisted of scientists trained in four different disciplines and research administrators. The SciTS team monitored twenty-five interdisciplinary teams at [BLIND] for 5 years from initiation of team formation to identify team dynamics that related to team success. This case is thus presented as part of an ongoing study of the 25 teams, supported by efforts through the [BLINDED] to encourage and enhance collaborative, interdisciplinary research and scholarship. Team outcomes were recorded annually and included extramural awards, publications, presentations, students trained, and training outcomes. An exemplary case-based study is appropriate when the case is unusual, the issues are theoretically important, and there are practical implications (Yin, 2017 ). Further, cases can illustrate examples of expertise and provide guidance to future teams (Bammer et al., 2020 ). An “exemplary team designation” was given to this team by the SciTS evaluators. Metrics used to designate an exemplary team included: team outcomes; highly interdisciplinary research; longevity of the team; fulfilment of all aspects of the land grant mission (research/discovery; education/training; and outreach/engagement); integration of team members; and use of external reviewers.
Social network survey
The exemplary team included Principle Investigators (PIs), postdoctoral researchers (postdocs), graduate students, undergraduate students, and active collaborators external to the University. The entire team was surveyed annually 2015–2019 about the extent and type of collaboration with other team members. In 2015, the team was asked about prior collaborations, and in subsequent years they were asked about additional interactions since joining the team. Possible collaborative activities included research publications, scientific presentations, grant proposals, and serving on student committees. Team members were also asked the types of relationships they had with each team member, including learning, leadership, mentoring, advice, friendship, and having fun (Supplementary 2 ). Data were collected using a voluntary online survey tool (Organisational Network Analysis Surveys). All subjects were identified by name on the social network survey but are not identified in any network diagrams or analyses. SNA software programmes R Studio (R Studio Team, 2020 ) and UCINET (Borgatti et al., 2014 ) were used to analyse data and Visone (Brandes and Wagner, 2011 ) was used to create visualisations. The response rate for the survey was 94% in 2015, 83% in 2016, 95% in 2017, and 81% in 2018. All data collection methods were performed with the informed consent of the participants and followed Institutional Review Board protocol #19-8622H.
Data from the social network survey were combined to create three different network measures: scientific productivity, mentoring, and advice. The scientific productivity network was a combination of four survey measures: research/consulting, grants, publications, and serving on student committees. Scientific productivity represents a form of cognitive or contributory expertise: expertise required to contribute to a field or discipline (Bammer et al., 2020 ; Boix Mansilla et al., 2016 ). The mentoring and advice networks were created from social network survey questions: “who is your mentor?” and “who do you go to for advice?”, respectively. Mentor and advice are tacit forms of interactional expertise: socialised knowledge that includes socialisation into the practices of an expert group (Collins and Evans, 2007 ). Other studies have also found a connection between social characteristics of interdisciplinary work and other factors like productivity, career paths, and a group’s ability to exchange information, interact, and explore together (Boix Mansilla et al., 2016 ).
Social network data were summarised using average degree, sometimes split into indegree and outdegree. Outdegree is a measure of how many team members a given individual reported getting advice, or mentorship, from. Similarly, the indegree of an individual is a measure of how many other team members reported receiving advice, or mentorship, from that person. Average degree is the average number of immediate connections (i.e., indegree plus outdegree) for a person in a network (Giuffre, 2013 ; R. Hanneman and Riddle, 2005 a, 2005 b). To further explore the mentoring and advice networks, we calculated the average degree/outdegree/indegree of postdocs, graduate students, and faculty separately to directly compare demographic groups.
The advice, mentoring, and scientific productivity networks were directly compared using the Pearson correlation between the corresponding network adjacency matrices. We predicted a positive correlation between the advice, mentoring, and scientific productivity matrices. Statistical significance ( p < 0.05) of correlations was assessed with the network permutation-based method Quadratic Assignment Procedure (QAP) (R. A. Hanneman and Riddle, 2005 a, 2005 b).
Historical social network data
A historical network survey was created to determine how the connections in the network formed, developed, and changed from project-to-project. The historical social network was constructed from three forms of data: interviews with the PIs, a historical narrative written by the PIs describing the team formation process, and team rosters that listed the 81 team members since the inception of the team.
Retrospective team survey
A retrospective team survey was administered at the end of the study to determine what skills team members developed and codified through participating on the team, how membership on the team supported members personally and professionally, and their favourite aspects of the team. The survey was sent to 22 members from the 2018 team roster using Qualtrics (Qualtrics Labs, 2005 ) with an 86% response rate.
Two semi-structured, one-hour interviews were conducted with two PIs in 2018 to learn about the history of the team. The interviews were digitally recorded and transcribed.
Participant observations
Participant observation was conducted from 2015–2019 at four annual three-day, off-campus retreats and 1–2 additional meetings each year. Students, PIs, external collaborators, and families were all invited to attend the retreats and meetings. Field notes about team interactions were recorded immediately after each interaction. The analytic field notes captured how team members interacted across disciplines, tackled scientific problems, and engaged with others at different career stages. Analysis occurred as field notes were written, during observations, and again during data analysis.
An exemplary team
The SciTS Team identified one team from the larger study and designated it as exemplary based on six (tacit and non-tacit) elements. First, the team had outstanding team outcomes. From 2004–2018, notable accomplishments include 33 extramural awards totalling over $5.6 million, including two large federal awards totalling over $4.5 million; 58 peer-reviewed publications with 39 different universities, 13 state agencies, and 11 other organisations; 141 presentations, 21 graduate students and 15 postdocs trained; and receipt of an [BLIND]institution-wide Interdisciplinary Scholarship Team Award. Participants received many individual honours, including one of the PIs being named to the National Academy of Sciences.
Second, this interdisciplinary team combined scientific expertise from many different backgrounds, including ecologists, wildlife biologists, evolutionary biologists, geneticists, veterinarians, and numerous collaborators. Principal Investigators were housed in five main universities: Colorado State University, University of Wyoming, University of Minnesota, University of California-Davis, and University of Tasmania. They also engaged collaborators from national and international universities, federal, state, and local governmental agencies, veterinary centres, and animal shelters. Collectively, team members represented 39 different universities, 11 federal agencies, 13 state agencies, and 11 other organisations listed on their peer-reviewed publications. The team has published globally with co-authors from every continent but Antarctica.
The third element identified was the team’s 15-year history and how they evolved project-to-project (Supplementary Video S1 ). In 2003, a graduate student proposed a collaborative research project between two faculty members who became two of the founding team PIs (Fig. 1 ). The team was formed in 2004 with four members—two faculty PIs, a postdoc, and a Ph.D. student (Fig. 1 ). Initial grant proposals submitted in 2005 and 2006 were not funded; however, in 2007, the team received a large federal research award from the US National Science Foundation (NSF). The team roster increased from four to nine, and a second large expansion occurred after receipt of another NSF award in 2012. By 2014, membership increased to 31 people, and at the end of analysis in 2018, the roster comprised 43 members. Over the course of observation, 81 different individuals, including students, faculty, and collaborators, had participated in research activities supported by the team.
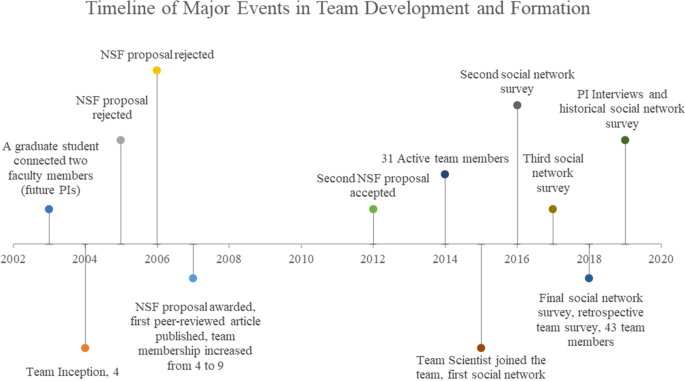
Significant events occurring over 15 years during the development and formation of an exemplary team.
The fourth reason this team was deemed exemplary was because it intertwined the components in the Land Grand mission, including research/discovery, teaching/training, and extension/engagement (Fig. 2 ). The team included undergraduates conducting research and presenting at conferences, graduate students working in multiple labs, and postdocs mentoring all the researchers in the lab. An external advisor said at the end of a retreat, “It’s really cool that students are part of the conversations that are both good/bad/ugly etc. It is not just good. It is not just one-on-one conversations. They hear it all.” A Ph.D. student wrote in the Retrospective Survey about the skills he developed: “I have developed the ability to talk about my research to people outside my field. I have also worked on broadening my understanding of disease ecology as a whole. I have been given the opportunity [to] begin placing my work in the larger framework of ecosystem health.” Faculty also wrote about what they learned, “[I] Learned from leadership of team (especially [blinded], and other PIs) how to develop and conduct research team work well - am using what I am learning to develop new research teams…. how to develop and nurture and respect interpersonal relationships and diversity of opinions. This has been an amazing experience, to be part of a well-functioning team, and to examine why and how that is maintained”
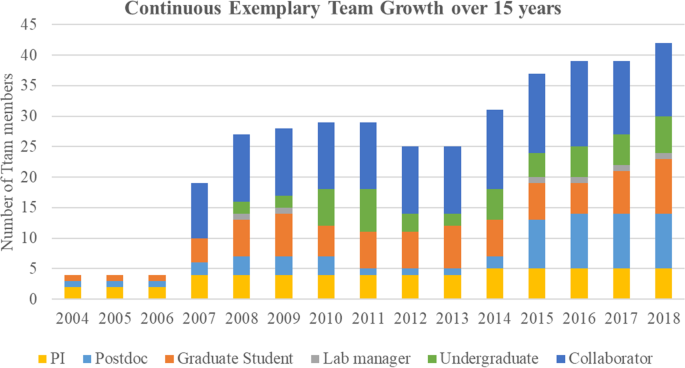
The team grew from 4 members in 2004 to 42 members in 2018. Much of the growth occurred by the addition of students and external collaborators.
Fifth, the team was effective at onboarding and integrating new members. To do so, they used two key strategies (Fig. 3 ). First, 15 of the students held co-advised graduate research positions. This shared model of mentorship provided students with opportunities to work in multiple labs, collaborate with additional team members, and gain a broader academic experience. A Ph.D. student wrote in the Retrospective Survey about the skills she learned from being a member of the team: “Leadership skills, communicating science to those in other fields, scientific writing skills, technical laboratory skills, interpersonal communication skills, data sharing experience, and many others.” The shared model supported the team’s interdisciplinary mission by providing opportunities to train future scientists to communicate, network, and conduct research across disciplines. Second, as team members developed through participation on the team, they assumed more mature scientific roles. Fourteen members of the team changed positions within the team. Many of these transitions were from undergraduate student to Ph.D. student or Ph.D. student to postdoctoral researcher. In 2012, one postdoc became a PI on the grant.
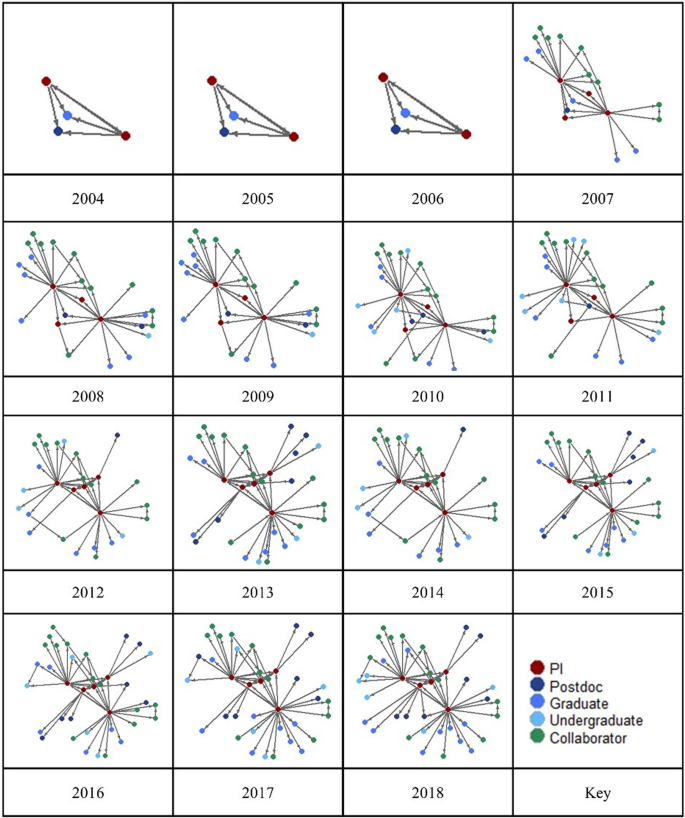
Social network diagrams of team growth and development from 2004–2018. This network reports onboarding and integration of all members, including their primary position when they joined the team. The nodes are sized by average degree (see text). Colours denote different roles on the team.
Finally, the 2018 team retreat included external reviewers. At the end of 2018 team retreat, they were asked if they had any feedback for the team. An external reviewer said: “You can check all of the boxes of a good team.”; “This is a dream team.”; “I am really impressed.”. Another external reviewer said:
The ambitiousness to execute the scope of the project, to have this many PIs, to be able to communicate; the opportunities for new insights; and the opportunities it presents for trainees are rare. There are a lot of people exposed in this. This is a unique experience for someone in training. And it extends to elementary school. I don’t think there are many projects that have this type of scope. I was impressed with just the idea that scientists are taking this across such a great scope and taking on such great questions.
Scientific productivity network
Prior to 2016, the average degree of the scientific productivity network was 8.8 (Fig. 4 ). In 2016, four faculty nodes were in the core of the network, and the periphery nodes included graduate students, postdocs, and external collaborators (Fig. 5 ). The average degree dropped slightly to 6.2 when the team integrated new members and re-formed around new roles and responsibilities on a new grant (Fig. 4 ). In 2017, the average degree peaked at 9.7 (Fig. 4 ) and faculty were still core, but graduate students and postdocs were more central than before (Fig. 5 ). During this time, productivity was at its highest as team members were working together to meet the objectives of a 5-year interdisciplinary NSF award. The network evolved further in 2018; two of the postdoc nodes overlapped with the faculty nodes in the core of the network (Fig. 5 ).
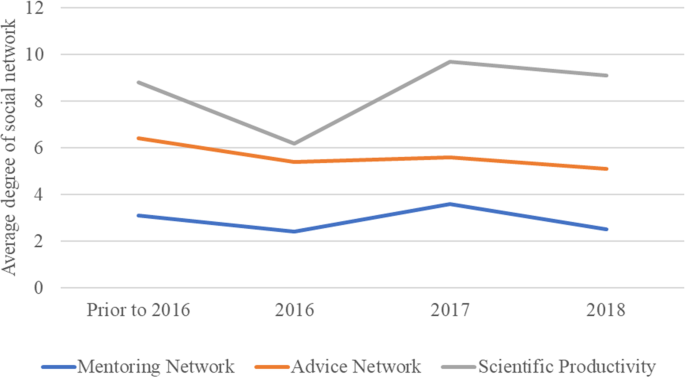
Average degree of social networks diagrams (mentoring, advice, scientific productivity) indicated strong social ties among team members.
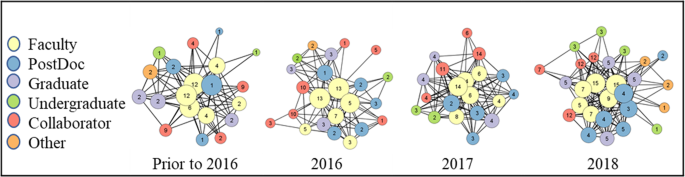
Social network measures of productivity (research/consulting, grants, publications, and serving on student committees) were recorded over time. Each node represents a person on the team, and nodes are sized by average degree (see text). Colours denote different roles on the team. The node label indicates the number of years a person has been part of the team.
Mentoring is integral in the collaborative network
Team members reported between an average of 2.4–3.1 mentors (average outdegree) each year on the team (Fig. 6 ). More specifically, graduate students reported 6.0–7.7 mentors, whereas postdocs reported 2.4–3.5 mentors (Table 1 ). Faculty team members reported having an average of 2.2 to 4.3 mentors on the team (Table 1 ), with the highest average outdegree in 2018.
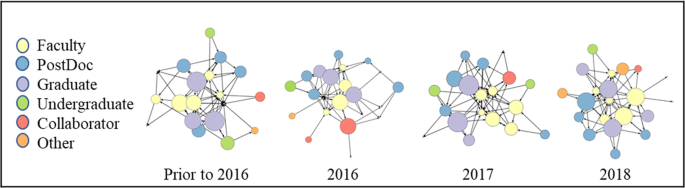
This diagram was created by using participant answers to the social network question, “who is your mentor?” Each circle or node represents a person on the team. The nodes are sized by outdegree to show who reported receiving mentorship. Node size indicates how many mentors an individual reported, and arrows indicate nodes that served as mentors. Colours denote different roles on the team.
The highest indegree for an individual was the lead PI, with an indegree ranging from 13 to 14 each year (i.e., each year, 13–14 team members reported this individual provided mentorship). In response to an interview question about this PIs favourite part of the team, this individual said, “…and of course, I really like the mentorship of the students…They are initially naive, and some people are initially underconfident, but eventually they become fluent in their subject area.” Many students wrote about the mentoring they received from the team. An undergraduate student wrote:
I have improved my communication skills after needing to collaborate with several mentors across different time zones. I’ve also improved willingness to ask questions when I don’t understand a concept. I’ve also learned what concepts I find basic in my field that others outside my discipline are less familiar with.
Faculty also wrote about the mentoring they received, such as, “I continually learn from members in the team and mentorship by the more experienced members has supported my own career progression.”
Advice is integral in the collaborative network
In the 2015–2017 advice network diagrams, the faculty were tightly clustered (Fig. 7 ). In 2018, the cluster separated as postdocs and graduate students joined the centre of the network. On average, team members reported 5.1 to 6.4 people they could go to for advice (Fig. 4 ).
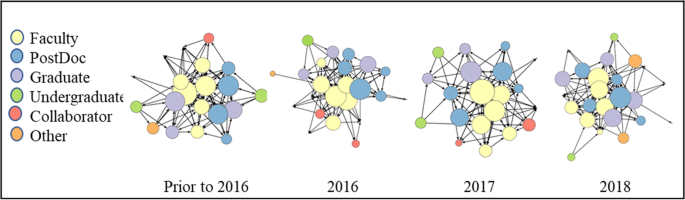
This diagram was created by using participant answers to the social network question, “who do you go to for advice?” Each circle or node represents a person on the team. The nodes are sized by outdegree to show who reported receiving mentorship. Node size indicates how many mentors an individual reported, and arrows indicate nodes that served as mentors. Colours denote different roles on the team.
In a survey, faculty responded to the question, “How has the team supported you personally and professionally?” One faculty member wrote: “Just today I asked three members of the team for professional advice! And got a thoughtful and prompt response from all.” Another team member wrote: “Being a member of the…team has allowed me to develop skills in statistical analysis, scientific writing, and critical thinking. This team has opened my eyes to what is possible to achieve with science and has provided me with opportunities to network and expand my horizons both within the field of study and outside of it.” These quotes further suggest that the mentoring and advice from a large interdisciplinary team were important to train future scientists.
Interpersonal relationships as driver for scientific productivity
The mentoring and advice networks supported and built on the scientific productivity network and vice versa. The correlation between the collaboration, mentoring, and advice networks would not be possible if the networks were not intertwined. In the retrospective survey, a faculty member described how tacit interpersonal relationships were correlated with their scientific productivity:
Being a part of this grant has helped me both personally and professionally by teaching me new skills (disease ecology, team dynamics), developing friendships/mentors from the team, and strengthening my CV and dossier for promotion to early full professorship.
A Ph.D. student also described how the relationships on the large team propelled their research.
Membership on this team has provided me with a lot of mentorship that I would not otherwise receive were I not working on a large multi-disciplinary for my doctoral research. It has also allowed me to network more effectively.
Between 2015 and 2018, the mentor and advice networks were significantly correlated with the scientific productivity network, demonstrating that personal relationships are associated with scientific collaboration (Table 2 ).
To date, the literature examining successful interdisciplinary scientific team skills that result in successful outcomes is sparse (Fiore, 2008 ; Hall et al., 2018 ; Wooten et al., 2014 ). The majority of published work in this area is evaluated by archival data analysis, not individual team behaviour and assessment (Hall et al., 2018 ). This study answers the call of numerous researchers to use mixed-methods and SNA to investigate scientific teams (Bennett, 2011 ; Borner et al., 2010 ; Hall et al., 2018 ; Woolley et al., 2010 ; Wooten et al., 2015 ). Our case-based study also increases understanding of the development and processes of an exemplary team by providing valuable insights about how the interactions that enhance scientific productivity are synergistic with the interactions that train future scientists. There are four major implications of our findings: (1) interactional and contributory expertise are intertwined; (2) team size, tacit knowledge gained from previous project, and interdisciplinary knowledge were used to effectively and efficiently train scientists; (3) the team increased scientific productivity through interpersonal relationships; and (4) the team fulfilled the land grant mission of the University by integrating teaching/training, research/discovery, and extension/engagement into the team’s activities.
Interactive and contributory expertise are intertwined
Previous literature on scientific teams has found that great teams are not built on scientific expertise alone, but on the processes and interactions that build psychological safety, create a shared language, engage members emotionally, and promote effective interactions (Boix Mansilla et al., 2016 ; Hall et al., 2019 ; Senge, 1991 ; Woolley et al., 2010 ; Zhang et al., 2020 ). The team highlighted in this report created a shared language and vision through the mentoring and advice networks that helped fuel the team’s scientific productivity (Hall et al., 2012 ). To solve complex problems requires more than contributory expertise, it also requires interactional expertise (Bammer et al., 2020 ). These forms of expertise are often tacit and internalised through the process of becoming an expert in a field of study (Collins and Evans, 2007 ). Learning-by-doing is augmented from project-to-project, with expertise codified over time (Bammer et al., 2020 ). Further, cognitive, emotional, and interactions are key components of successful collaborations (Boix Mansilla et al., 2016 ; Bozeman et al., 2013 ; Zhang et al., 2020 ). Using social network analysis, our case-based analysis found that the mentoring and advice ties were intertwined with the scientific productivity network.
Training scientists to be experts
The Retrospective Survey asked what personal and professional skills respondents learned from being a member of a team. We hypothesised that many respondents would report tangible skills. Surprisingly, 82% of the open-ended responses were about tacit skills. Students frequently had co-advised graduate research positions, worked in multiple labs, and communicated regularly with practitioners. Moreover, the team translated research to different disciplines within the team, mentored others, and managed interpersonal conflicts. These interactions built expertise because training was not limited to research in a single lab or only in an academic setting. Simple, discrete, and codified knowledge is relatively easy to transfer; however, teams need stronger relationships to gain complex and tacit knowledge, (Attewell, 1992 ; Simonin, 1999 ). On this team, interactions and the ability to practice communication were especially influential for students, junior scientists, and new members. These individuals provided survey responses reporting they learned a wide variety of skills ranging from leadership, scientific and interpersonal communication, networking across disciplines, scientific writing, laboratory techniques, and data sharing standards. Further, respondents noted they had gained experience in developing, nurturing, and respecting interpersonal relationships and diversity of opinions. This was reinforced with participant observation data. In other interdisciplinary groups studied in conjunction with this exemplary team, students were not typically exposed to the inner workings of the team such as leadership meetings. On this team, students were exposed to all conversations, which became an important component of the mentoring and advice structure, serving to train future scientists in all aspects of team integration and leadership development. Belonging to this large interdisciplinary team was effectively training, building, and structuring the team.
Interpersonal relationships increase scientific productivity
Longevity of relationships is an important factor in creating social cohesion, reducing uncertainty, and increasing reliability and reciprocity (Baum et al., 2007 ; Gulati and Gargiulo, 1999 ; Phelps et al., 2012 ). Previous literature has, however, rarely documented the importance of time in building the structure of the network (Phelps et al., 2012 ) and few longitudinal studies of scientific teams exist. Further, it has long been hypothesised that greater interaction among people increases the quality and innovativeness of ideas generated, which may in turn increase productivity (Cimenler et al., 2016 ). Our case-based study found that the mentoring and advice ties existed in a symbiotic relationship with the scientific productivity network where the practices of the team were simultaneously training scientists. This aligns with social network literature that interactions can structure the social network and the network structure influences interactions (Henry, 2009 ; Phelps et al., 2012 ). Second, intentional mentoring programmes have demonstrated a positive relationship between interdisciplinary mentoring and increased research productivity outcomes such as grant funding and publications (Spence et al., 2018 ). Finally, this finding also aligns with literature on the generation of new knowledge (Phelps et al., 2012 ). Knowledge creation has traditionally been framed in terms of individual creativity, but recent studies have placed more emphasis on how the contribution of social dynamics are influential in explaining this process (Boix Mansilla et al., 2016 ; Csikszentmihalyi, 1998 ; Phelps et al., 2012 ; Sawyer, 2003 ; Zhang et al., 2009 ). Thus, while we might think that science drives the team, in this case-based study, the team’s interpersonal relationships were the driver of the team’s scientific productivity.
Fulfilling the land grant mission
As noted above, this exemplary team fulfilled all three goals of the land grant mission. First, the team was training scientists at all levels, from undergraduate students, to graduate students, postdocs, new faculty, and external collaborators, including community partners. In many instances, the training and mentoring was structured in a vertically integrated manner. For example, postdocs were training graduate and undergraduate students, typical of many teams. In addition to the “top-down” scenarios, however, the team also encouraged training that went from the bottom up as well. Effectively, this is a hallmark of successful teams in other sectors such as emergency responders and elite military teams – whomever has the knowledge to drive the issue at hand is the effective “leader” in that mission (Kotler and Wheal, 2008 ). Second, the team excelled in research and discovery, partnering with a diversity of external collaborators to do so. This created a network structure wherein the team clearly utilised the collaborators for mentoring and advice. Organisations with a core-periphery network structure like this team have been reported to be more creative because ties on the periphery, such as external collaborators, can span boundaries and access diverse information (Perry-Smith, 2006 ; Phelps et al., 2012 ). Finally, because the team’s collaborators included community partners and practitioners, they were also influencing policy and practice. This resulted in an overall greater impact for the team’s science and allowed them to tailor their research to best meet the needs of society (Barge and Shockley-Zalabak, 2008 ).
Future research
This study provides a unique contribution to team science literature because it longitudinally studied the development and processes of a successful interdisciplinary team (Wooten et al., 2014 ). Future research on the elements of effective interdisciplinary teaming is required in five key areas. First, identification of best practices that inhibit or support teams is necessary (Fiore, 2008 ; Hall et al., 2018 ; Wooten et al., 2014 ). Second, previous research has found that small teams are best at disrupting science with new ideas and opportunities (Wu et al., 2019 ); however, practices large teams use to create new knowledge have been poorly documented. Third, successful training concepts for graduate students and postdoctoral researchers need additional consideration (Knowlton et al., 2014 ; Ryan et al., 2012 ; Sarraj et al., 2017 ). Fourth, we hypothesise that graduate students act as bridges in teams to connect scientific disciplines and prevent clustering the network. Future research should investigate the role of graduate students in creating knowledge through interdisciplinary teams. Finally, additional research is needed to better recognise and reward scientists who undertake integration and implementation (Bammer et al., 2020 ).
Data availability
The datasets generated during and analysed during the current study are available in the Mountain Scholar repository, https://doi.org/10.25675/10217/214187
Attewell P (1992) Technology diffusion and organizational learning: the case of business computing. Organ Sci 3(1):1–19. https://doi.org/10.1287/orsc.3.1.1
Article Google Scholar
Bammer G, O’Rourke M, O’Connell D, Neuhauser L, Midgley G, Klein JT, … Richardson GP (2020). Expertise in research integration and implementation for tackling complex problems: when is it needed, where can it be found and how can it be strengthened? Palgrave Commun, 6(1). https://doi.org/10.1057/s41599-019-0380-0
Barge JK, Shockley-Zalabak P (2008) Engaged scholarship and the creation of useful organizational knowledge. J Appl Commun Res 36(3):251–265. https://doi.org/10.1080/00909880802172277
Baum JAC, McEvily B, Rowley T (2007) Better with age? Tie longevity and the performance implications of bridging and closure. SSRN (vol. 23). INFORMS. https://doi.org/10.2139/ssrn.1032282
Bennett ML (2011) Collaboration and team science: a field guide-team science toolkit. Retrieved February 19, 2019, from https://www.teamsciencetoolkit.cancer.gov/Public/TSResourceTool.aspx?tid=1&rid=267
Boix Mansilla V, Lamont M, Sato K (2016) Shared cognitive–emotional–interactional platforms: markers and conditions for successful interdisciplinary collaborations. Sci Technol Human Value 41(4):571–612. https://doi.org/10.1177/0162243915614103
Borgatti SP, Everett MG, Freeman LC (2014) UCINET. Encyclopedia of social network analysis and mining. Springer New York, New York, NY, 10.1007/978-1-4614-6170-8_316
Google Scholar
Borner K, Contractor N, Falk-Krzesinski HJ, Fiore SM, Hall KL, Keyton J, Uzzi B (2010). A multi-level systems perspective for the science. Sci Transl Med 2: 49
Bozeman B, Fay D, Slade CP (2013, February 28). Research collaboration in universities and academic entrepreneurship: the-state-of-the-art. J Technol Transf https://doi.org/10.1007/s10961-012-9281-8
Brandes U, Wagner D (2011) Analysis and visualization of social networks. Springer, Berlin, Heidelberg, pp. 321–340. 10.1007/978-3-642-18638-7_15
Cimenler O, Reeves KA, Skvoretz J, Oztekin A (2016) A causal analytic model to evaluate the impact of researchers’ individual innovativeness on their collaborative outputs. J Model Manag 11(2):585–611
Collins H, Evans R (2007) Rethinking expertise. University of Chicago Press, Chicago
Book Google Scholar
Collins JC (2001) Good to great. HarperBusiness, New York, NY
Csikszentmihalyi M (1998) Implications of a systems perspective for the study of creativity. In: Sternberg R (ed) Handbook of creativity (pp. 313–336. Cambridge University Press, Cambridge, England. https://doi.org/10.1017/CBO9780511807916.018
Cummings JN, Kiesler S (2008) Who collaborates successfully? prior experience reduces collaboration barriers in distributed interdisciplinary research. Cscw: 2008 Acm conference on computer supported cooperative work, Conference Proceedings, pp. 437–446. https://doi.org/10.1145/1460563.1460633
De Montjoye YA, Stopczynski A, Shmueli E, Pentland A, Lehmann S (2014) The strength of the strongest ties in collaborative problem solving. Sci Rep 4. https://doi.org/10.1038/srep05277
Dozier AM, Martina CA, O’Dell NL, Fogg TT, Lurie SJ, Rubinstein EP, Pearson TA (2014) Identifying emerging research collaborations and networks: method development. Eval Health Prof 37(1):19–32. https://doi.org/10.1177/0163278713501693
Article PubMed Google Scholar
Duhigg Ch (2016) What google learned from its quest to build the perfect team-The New York Times. Retrieved December 2, 2017, from https://www.nytimes.com/2016/02/28/magazine/what-google-learned-from-its-quest-to-build-the-perfect-team.html
Falk-Krzesinski HJ, Contractor N, Fiore SM, Hall KL, Kane C, Keyton J, Trochim W (2011) Mapping a research agenda for the science of team science. Res Eval 20(2):145–158. https://doi.org/10.3152/095820211X12941371876580
Article PubMed PubMed Central Google Scholar
Fiore SM (2008) Interdisciplinarity as teamwork. Small Group Res 39(3):251–277. https://doi.org/10.1177/1046496408317797
Giuffre K (2013) Communities and networks: using social network analysis to rethink urban and community studies, 1st edn. Polity Press, Cambridge MA, 10.1177/0042098015621842
Greenwood RE (1993) The case study approach. Bus Commun Q 56(4):46–48. https://doi.org/10.1177/108056999305600409
Gulati R, Gargiulo M (1999) Where do interorganizational networks come from? Am J Sociol 104(5):1439–1493. https://doi.org/10.1086/210179
Hall KL, Vogel AL, Croyle RT (2019) Strategies for team science success: handbook of evidence-based principles for cross-disciplinary science and practical lessons learned from health researchers. Springer Nature, Switzerland
Hall KL, Vogel AL, Huang GC, Serrano KJ, Rice EL, Tsakraklides SP, Fiore SM (2018) The science of team science: a review of the empirical evidence and research gaps on collaboration in science. Am Psychol 73(4):532–548. https://doi.org/10.1037/amp0000319
Hall KL, Vogel AL, Stipelman BA, Stokols D, Morgan G, Gehlert S (2012) A four-phase model of transdisciplinary team-based research: goals, team processes, and strategies. Transl Behav Med 2(4):415–430. https://doi.org/10.1007/s13142-012-0167-y
Hanneman RA, Riddle M (2005a) Introduction to social network methods: table of contents. Riverside, CA. Retrieved from http://faculty.ucr.edu/~hanneman/nettext/
Hanneman R, Riddle M (2005b) Introduction to social network methods. Retrieved from http://www.researchgate.net/profile/Robert_Hanneman/publication/235737492_Introduction_to_social_network_methods/links/0deec52261e1577e6c000000.pdf
Henry AD (2009). Society for human ecology the challenge of learning for sustainability: a prolegomenon to. source: human ecology review (Vol. 16). Retrieved from https://www-jstor-org.ezproxy2.library.colostate.edu/stable/pdf/24707537.pdf?refreqid=excelsior%3Ae9ffb98fef69c055ab05ead486b9ca7e
Klein C, DiazGranados D, Salas E, Le H, Burke CS, Lyons R, Goodwin GF (2009) Does team building work? Small Group Res 40(2):181–222. https://doi.org/10.1177/1046496408328821
Knowlton JL, Halvorsen KE, Handler RM, O’Rourke M (2014) Teaching interdisciplinary sustainability science teamwork skills to graduate students using in-person and web-based interactions. Sustainability (Switzerland) 6(12):9428–9440. https://doi.org/10.3390/su6129428
Kotler S, Wheal J (2008) Stealing fire: how silicon valley, the navy seals, and maverick scientists are revolutionising the way we live and work. Visual Comput (vol. 24). Retrieved from https://qyybjydyd01.storage.googleapis.com/MDA2MjQyOTY1NQ==01.pdf
Lee S, Bozeman B (2005) The impact of research collaboration on scientific productivity. Soc Stud Sci 35(5):673–702. https://doi.org/10.1177/0306312705052359
Matthews NE, Cizauskas CA, Layton DS, Stamford L, Shapira P (2019) Collaborating constructively for sustainable biotechnology. Sci Rep 9(1):19033. https://doi.org/10.1038/s41598-019-54331-7
Article ADS CAS PubMed PubMed Central Google Scholar
Patton MQ (2011) Developmental evaluation: applying complexity concepts to enhance innovation and use. Guilford Press
Perry-Smith JE (2006) Social yet creative: the role of social relationships in facilitating individual creativity. Acad Manag J 49(1):85–101. https://doi.org/10.5465/AMJ.2006.20785503
Phelps C, Heidl R, Wadhwa A, Paris H (2012) Agenda knowledge, networks, and knowledge networks: a review and research. J Manag 38(4):1115–1166. https://doi.org/10.1177/0149206311432640
Qualtrics Labs I (2005). Qualtrics Labs, Inc. Provo, Utah, USA
R Studio Team (2020) RStudio: Integrated Development for R. RStudio, PBC, Boston, MA, http://www.rstudio.com/
Read EK, O’Rourke M, Hong GS, Hanson PC, Winslow LA, Crowley S, Weathers KC (2016) Building the team for team science. Ecosphere 7(3):e01291. https://doi.org/10.1002/ecs2.1291
Ryan MM, Yeung RS, Bass M, Kapil M, Slater S, Creedon K (2012) Developing research capacity among graduate students in an interdisciplinary environment. High Educ Res Dev 31(4):557–569. https://doi.org/10.1080/07294360.2011.653956
Sarraj H, Hellmich M, Chao C, Aronson J, Cestone C, Wooten K, Allan, B (2017) Training future team scientists: reflections from translational course. In: Team science training for graduate students and postdocs. Clearwater, FL. Retrieved from www.scienceofteamscience.org
Sawyer RK (2003) Emergence in creativity and development. In: Sawyer RK, John-Steiner V, Moran S., Sternberg RJ, Nakamura J et al. (eds.) Creativity and development. Oxford University Press, Oxford, England, pp. 12–60
Chapter Google Scholar
Senge PM (1991) The fifth discipline, the art and practice of the learning organization. Perform Instruct 30(5):37–37. https://doi.org/10.1002/pfi.4170300510
Simonin BL (1999) Ambiguity and the process of knowledge transfer in strategic alliances. Strateg Manag J 20(7):595–623. 10.1002/(SICI)1097-0266(199907)20:7<595::AID-SMJ47>3.0.CO;2-5
Spence JP, Buddenbaum JL, Bice PJ, Welch JL, Carroll AE (2018) Independent investigator incubator (I3): a comprehensive mentorship program to jumpstart productive research careers for junior faculty. BMC Med Educ 18(1):1–11. https://doi.org/10.1186/s12909-018-1290-3
Stokols D, Misra S, Moser RP, Hall KL, Taylor BK (2008). The ecology of team science. Understanding contextual influences on transdisciplinary collaboration. Am J Prevent Med https://doi.org/10.1016/j.amepre.2008.05.003
Woolley AW, Chabris CF, Pentland A, Hashmi N, Malone TW (2010) Evidence for a collective intelligence factor in the performance of human groups. Science 330(6004):686–688. https://doi.org/10.1126/science.1193147
Article ADS CAS PubMed Google Scholar
Wooten KC, Calhoun WJ, Bhavnani S, Rose RM, Ameredes B, Brasier AR (2015) Evolution of multidisciplinary translational teams (MTTs): insights for accelerating translational innovations. Clin Transl Sci 8(5):542–552. https://doi.org/10.1111/cts.12266
Wooten KC, Rose RM, Ostir GV, Calhoun WJ, Ameredes BT, Brasier AR (2014) Assessing and evaluating multidisciplinary translational teams: a mixed methods approach. Eval Health Profession 37(1):33–49. https://doi.org/10.1177/0163278713504433
Wu L, Wang D, Evans JA (2019) Large teams develop and small teams disrupt science and technology. Nature 1. https://doi.org/10.1038/s41586-019-0941-9
Wuchty S, Jones BF, Uzzi B (2007) The increasing dominance of teams in production of knowledge. Science 316(5827):1036–1039. https://doi.org/10.1126/science.1136099
Yin RK (2017) Case study research and applications: design and methods (6th edn.). Sage Publications
Zhang HH, Ding C, Schutte NS, Li R (2020) How team emotional intelligence connects to task performance: a network approach. Small Group Res 51(4):492–516. https://doi.org/10.1177/1046496419889660
Article CAS Google Scholar
Zhang J, Scardamalia M, Reeve R, Messina R (2009) Designs for collective cognitive responsibility in knowledge-building communities. J Learn Sci 18(1):7–44. https://doi.org/10.1080/10508400802581676
Download references
Acknowledgements
A special thank you to Elizabeth Scodfidio for helping with data, images and more!. The research reported in this publication was supported by Colorado State University’s Office of the Vice President for Research Catalyst for Innovative Partnerships Programme. The content is solely the responsibility of the authors and does not necessarily represent the official views of the Office of the Vice President for Research. Supported by NIH/NCATS Colorado CTSA Grant Number UL1 TR002535. Contents are the authors’ sole responsibility and do not necessarily represent official NIH views. Funding and support were provided by grants from the National Science Foundation’s Ecology of Infectious Diseases Programme (NSF EF-0723676 and NSF EF-1413925).
Author information
Authors and affiliations.
Divergent Science LLC, Fort Collins, CO, USA
Hannah B. Love
Colorado State University, Fort Collins, CO, USA
Jennifer E. Cross, Bailey Fosdick, Kevin R. Crooks & Susan VandeWoude
University of New Mexico and Colorado State University, Fort Collins, CO, USA
Ellen R. Fisher
You can also search for this author in PubMed Google Scholar
Contributions
HBL conceptualised the study, developed the methodology, curated the data, analysed the data, conducted the investigation, worked as the project manager, managed the software, validated the data, created visualisations, reviewed and edited the paper; BF conceptualised the study, developed the methodology, curated the data, analysed the data, managed the software, validated the data, supervised all aspects of the research, created visualisations, reviewed and edited the paper; JC conceptualised the study, developed the methodology, acquired funding, supervised data collection, and reviewed and edited the paper; KC and SV wrote the paper, secured funding, reviewed and edited the paper; and ERF conceptualised the study, developed the methodology, supervised all aspects of the research, acquired funding, created the visualisations, reviewed and edited the paper; All authors reviewed the manuscript.
Corresponding author
Correspondence to Hannah B. Love .
Ethics declarations
Competing interests.
HBL, BF, JC, and ERF declare no competing interests. KC and SV are members of the exemplary team
Additional information
Publisher’s note Springer Nature remains neutral with regard to jurisdictional claims in published maps and institutional affiliations.
Supplementary information
Supplementary information, supplementary data, rights and permissions.
Open Access This article is licensed under a Creative Commons Attribution 4.0 International License, which permits use, sharing, adaptation, distribution and reproduction in any medium or format, as long as you give appropriate credit to the original author(s) and the source, provide a link to the Creative Commons license, and indicate if changes were made. The images or other third party material in this article are included in the article’s Creative Commons license, unless indicated otherwise in a credit line to the material. If material is not included in the article’s Creative Commons license and your intended use is not permitted by statutory regulation or exceeds the permitted use, you will need to obtain permission directly from the copyright holder. To view a copy of this license, visit http://creativecommons.org/licenses/by/4.0/ .
Reprints and permissions
About this article
Cite this article.
Love, H.B., Cross, J.E., Fosdick, B. et al. Interpersonal relationships drive successful team science: an exemplary case-based study. Humanit Soc Sci Commun 8 , 106 (2021). https://doi.org/10.1057/s41599-021-00789-8
Download citation
Received : 02 January 2021
Accepted : 14 April 2021
Published : 06 May 2021
DOI : https://doi.org/10.1057/s41599-021-00789-8
Share this article
Anyone you share the following link with will be able to read this content:
Sorry, a shareable link is not currently available for this article.
Provided by the Springer Nature SharedIt content-sharing initiative
Quick links
- Explore articles by subject
- Guide to authors
- Editorial policies

Build Relationships: Self-Revealing Case Studies on Growth and Success
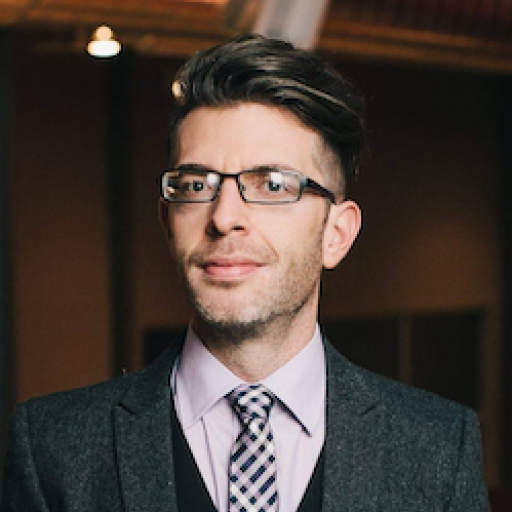
A little over a month ago, I fired off this bombshell of a post …
How I Wrote for Fast Company, Copyblogger, MarketingProfs, and Entrepreneur and Landed My First Nearly $20,000, Three-Month Client … in Less than 120 Days
Despite how outlandishly prideful that headline sounds at first, the point of the post — its secret sauce — came down to three words: “Let’s get rejected.” Naturally, that’s counterintuitive, but check out the post.
Since then, the streak has continued … mostly.
To start, I had another article go up on Copyblogger — “The Ultimate Copy Checklist: 51 Questions to Optimize Every Element of Your Online Copy.” Currently, it’s number two on their most-popular-posts list thanks in large part to the printable poster their awesome design team put together.
In addition, I had a second article accepted to MarketingProfs that’ll be published in a week or two and my first for Unbounce just hit the digital presses a few weeks ago: “4 Steps to Writing Emails with Drastically Higher Open and Click-Through Rates.” On top of all that, I’m looking at a a follow up articles for both Fast Company and Unbounce in January.
But, best of all …
I got rejected.
Yep! How perfect is that?
The story goes like this … over the summer, I wrote an article on storytelling and presenting that Fast Company was kind enough to pick up after I submitted it via cold email.
The waters being warmer, last month I wrote a piece on responsive email, complete with compelling data and these super slick display shots of six of my favorite responsive email newsletters on different devices.
Here’s a couple of ‘em.
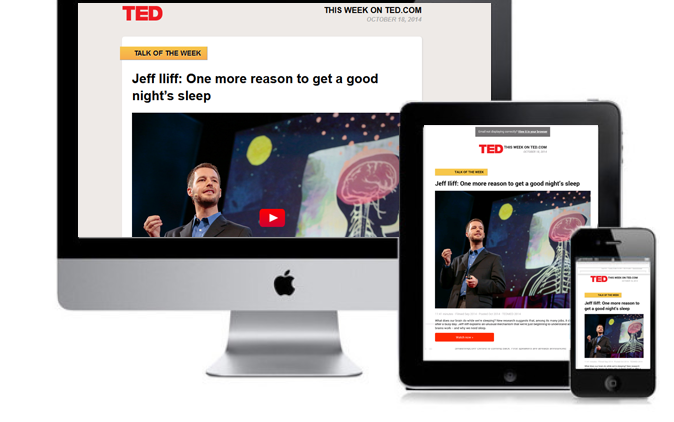
Cool, right?
Well, after a week of waiting … it came:
Dear Aaron,
Thank you for submitting this article for Fast Company’s consideration. Unfortunately, while interesting, this piece is not a right fit for us. I encourage you, however, to place it somewhere else. Please feel free to continue submitting work for our future consideration.
You know the drill …
Enter pain. Enter fear. Enter shame. Enter defensiveness. And, of course, enter those inevitable, manic swings between the land of “how dare they not recognize someone of my literary caliber by immediately publishing my work and renaming their site in my honor” and “of course they didn’t publish it … I can barely string together words, much less justify my existence as a person.”
Like a friend of mine likes to say, “My brain’s constantly telling me I’m either hot s*** or a piece of s***.”
But enough about my existential angst. Pain, fear, shame, defensiveness, and manic swings aside, I eventually picked myself up, dusted off, and reworked the thing for another blog.
More to the point, back some thirty-plus days ago, I promised a more lengthy explanation of how those massive wins — namely writing for Fast Company, Copyblogger, MarketingProfs, Entrepreneur, and now Unbounce — came together.
So today I present the first of three case studies on growth and success:
Build relationships..
Cliches are cliche for a reason. Because they’re true.
“It’s not about what you know. It’s about who you know.”
At the beginning of last summer, when I finally decided to make a go of this whole “freelance writing” thing, I identified a handful of people who — number one — I liked and respected and — number two — mattered.
Demian Farnworth, Copyblogger’s chief copywriter, was at the very top of that list.
I’ve loved Copyblogger (who doesn’t?) for years and dreamed of writing for them.
A few months ago, I noticed Demian’s name kept popping up and always in connection with absolutely stellar content. The first one I really loved was “13 Good Ideas from 13 Dead Copywriters.” And, of course, his “11 Essential Ingredients Every Blog Post Needs” is on just about everybody’s list of the best content of 2014.
So I tracked Demian down, digitally speaking, and found out not only did he run a personal copywriting site — theCopyBot — he also ran an even more personal “personal” site — Fallen + Flawed .
What I found on the second floored me.
Fallen + Flawed is not a marketing site. It isn’t about improving your CTRs, optimizing your headlines, telling your story, or getting your emails opened. In fact, it’s not really about writing at all — although there are posts about blogging .
Fallen + Flawed is about the gospel . It’s about faith and life and struggling and books and marriage and kids and Mexican wrestlers .
It is creative. It is original. It is authentic. And it is phenomenal.
On top of all that, the theological overlaps were deep.
Even within the (for lack of a better word) “evangelical” Christian community (with which I self-identify), there are camps, especially theological camps. And every camp has its patron saints. For the young, reformed community (the camp I belong to), folks like John Piper, D. A. Carson, and Tim Keller are rockstars.
And that’s exactly who Demian was writing about.
I nearly lost my mind.
I was so excited that I started tweeting anything I could get ahold of.
I tweeted his Copyblogger stuff. I tweeted his CopyBot stuff. I tweeted his theological stuff. I even tweeted lines from his fantastic ebook The Messiah | Eleven Meditations from the Book of Mark .
And … I tagged him each and every time.
By now you’ve probably picked up that I’m a little biased, but let me just say that Demian is a super decent dude. I don’t think there’s been a single tweet I tagged him in that he didn’t favorite. Plus, right around the time I started tweeting stuff from his book on Mark, he tweeted back.
Mind = blown.
We threw a few notes around, mostly just polite niceties, and then … I found his coaching page.
This time, I emailed. And — gasp — he emailed back.
I won’t go into detail here about what an amazing coach Demian has been (after all, I’ve probably gushed enough).
Here’s the point …
When it finally came time to submit a guest post to Copyblogger, I did so both through their online submission process as well as a direct email to Demian himself.
From the first — their online process — all I got back was a “Thanks for submitting … but we aren’t accepting unsolicited articles at this time.”
It was the second route — the relationship route — that opened the door.
Now, of course, you gotta have the goods as well as the relationship. I mean, no one’s gonna publish junk. In fact, I’m gonna say a lot more about having “the goods” in the second case study — “Write [bleeping] amazing content” — but for now it’s vitally important to see how central that relationship was.
What made the difference was not first and foremost the words themselves.
What made the difference was that relationship — that authentic, genuine, giving (not just taking) relationship.
A similar situation has developed with Joanna Wiebe of CopyHackers . She and her team — including her awesome copywriting compadre Jen Havice — have been incredibly supportive of both my personal blog and guest posts. I remember the day she signed up for my email list … again: mind = blown.
The same is true of Ann Handley , who after quoting a post of mine in her most recent book, has also been amazing.
So, let me ask you …
Who do you look up to? Who do you admire? Who would you want to connect with? Who do you respect? Who matters in your industry?
Alright … what’s stopping you from reaching out?
Track ‘em down. Share their content. Tag ‘em. Be warm and be personal. Write meaningful comments on their blog. Ask insightful questions. And above all, be real.
Over the next month, I’ll be sharing three more case studies on growth and success. Here’s a preview …
2. Write [bleeping] amazing content.
3. Be a decent freakin’ person.
4. Go after it … again, and again, and again.
Let’s save the world from bad content
Download the …
Comprehensive Content Strategy Roadmap
This is the exact resource I’ve used with clients starting at $10k per month . But, you can have it for free. (Just don’t tell anybody.)

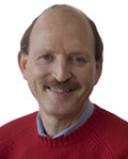
Finding and Deepening a Long-Term Relationship: A Case Study
Five embedded principles and practices..
Posted January 30, 2022 | Reviewed by Abigail Fagan
- The goal of your dating-app profile isn't to attract the most responses; it's to gather good fits. Describe what makes you different.
- Using a "report card" can prevent infatuation's fog from clouding your judgment.
- Even if you feel desperate, have high-standards in a partner. It's better to be single than to join the many unhappy couples.
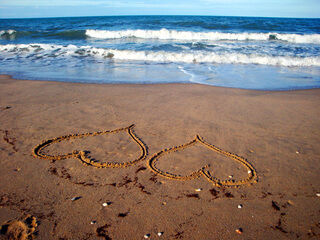
This is part of The Quests series. The others are linked to at the end of this post.
Here we turn to the quest for a good long-term relationship. As in the previous installment, this one begins with a first-person report from "Jeremy." It is a composite of my clients' experiences, and it embeds five keys to finding and deepening a long-term relationship. After, I call those out.
Hi, this is Jeremy again. There are all those songs about love: passionate love, deep love, even lasting love.
But that hasn’t been my experience, although I’ll admit my experience has been kind of limited. Sure, I dated some in college but the longest lasted just three months. I hate the word “hookup” but that’s kind of what most of them were. If I had been sober, maybe I would have had fewer, I don’t know. I told you I’d be honest so I figure I better tell you this: I wasn’t great at it. Sometimes, I was no good at all, if you know what I mean.
My parents are still together although I don’t think they have a marriage made in heaven. They seem to make it work here on earth, although I don’t know what their marriage is really like. Kids don’t know about that. At least I don’t.
But I thought I wanted to try for a real relationship. Actually, I wasn’t sure: So many couples start great but it doesn’t last. And if they get married, half get divorced. I hear divorcing sucks. And if they have kids, it’s even worse.
I knew that bars aren’t good places to meet a real partner—too loud, too much alcohol and weed. There, it comes down mainly to looks. Sometimes I think the 9s and 10s figure they can get by on their looks so they don’t care to be nice, or they don’t work on their personality . I don’t know.
So I told my friends, at least the ones who weren’t screw-ups, that I’m looking to meet someone. I didn’t know exactly what to tell them so I just said “attractive, kind, and well-adjusted.” I even lowered myself to tell my parents that.
I felt I should also use apps—today, even winners use them, by the millions. But what should I say about myself? Something that will attract a lot of responses like, “Successful, kind, fun-loving, communicative guy seeks similar woman?” But I heard that the game isn’t how many responses you get, it’s getting a well-matched one. And that requires you to be honest. Well, at least that’s something I can be. What do you think of this opener? “Kind, recent college grad guy with a decent job (finally), seeks a similar gal.”
Here was my thinking: I wanted to imply that I wasn’t a 10, maybe a 7, and that I wanted to be fair, expecting around the same in a woman. And I used just a little humor , self-effacing humor—that I finally got a job—because I didn’t want to seem like I was trying too hard.
Rather than wait for women to contact me, I reached out so I could be pickier. And I stayed picky: If they didn’t respond within a couple days or seemed at all mean or whose attic seemed dusty, I wrote them off.
My parents made me try Michelle. Well, her texts were okay but the coffee date? All she wanted to talk about was her family and pop culture. And I mean talk—she barely asked me anything. Maybe she was nervous but you gotta make choices—there are a lot of fish in the sea. So, I didn't want to see her again.
A friend insisted that Christine would be a good fit. Yeah, except that there was no chemistry. Remember when I said that sometimes I was no good at all? Well, with Christine, I knew I wouldn’t be.
But the third time was the charm. I felt like I always knew Lily—the conversation just flowed. She wasn’t a 10 but I just couldn’t wait to kiss her, and I could sense that she felt that way too. At the end of our first date, we had the best kiss good-night, but we sensed that we shouldn’t go further that night.
The next day I was flying! I wanted to text her 20 times that day but put myself on a texting diet : I waited until mid-morning and when she responded in 20 minutes, I waited 15, even though I wanted to respond that second.
Fast-forward six months. Lily and I have been living together for two months now. Things are great although the sex isn’t quite as good as it was. And she’s been a little pushy about wanting me to get a better job. But when I rated her on a sort of report card, she’d make Phi Beta Lova: Sex A-, Career A, Kindness B+, Well-adjustedness A-, and that ineffable thing called love: A-.

I brought Lily home to meet my parents. They love her, or maybe it’s that they’d love to be grandparents. It's probably some combination of the two.
God, I don’t know. Is this it? Is there someone even better for me out there? After we got married, would Lily change? Would I change? Would we tire of each other, mad at each other, join the half of couples that divorce, screaming over who gets the home entertainment unit? Or would we be okay, or better than okay? Or should I stay single at least a while longer? I’m not sure. I’m really not.
The takeaways
Use filtered sources. Bars provide no screen for compatibility beyond looks—anyone can come to a bar. Seeking a partner at a bar screens in people who’d rather drink and talk little—it’s too loud and too public to hear much.
Better: Reach out to well-curated people on the dating apps, ask trusted friends and relatives to set you up, and attend events likely to attract your Mr. or Ms. Long-Term Right: a particular talk, a volunteer opportunity, or a course. For example, if you’re fun-centric, maybe it's a dance class or tennis group lesson. To meet someone practical, you might attend a class on financial planning.
Create an honest dating profile. The goal isn’t to get the most responses, it’s to get a few on-target ones. So, acknowledge not only your strengths but a weakness or difference that the right partner would need to accept, maybe even welcome. For example, if I were single, my dating profile would mention that I like to work unusually long hours. That would screen out the wrong partners and screen in the right ones: those with a rich life independent of their partner.
Reach out. When you do the sifting, you're finding good fits for you. In contrast, if you respond to others, you're tempted to compromise to fit the other person's desires.
The beauty of dating apps is their potential for curation. Have high standards: Sift through many profiles to find a few that you could imagine becoming your special someone. Then, if their initial response is less than a net A-, move on. Sure, you could have guessed wrong, but it’s a probability game: You want to spend your time so it maximizes the chances of your meeting Mr. or Ms. Right.
Chemistry is necessary but far from sufficient. Sex is what differentiates romantic from platonic relationships. So, attraction that's insufficient to last beyond the infatuation phase may be a deal-killer. That’s also true of different sexual appetites: If you’re a daily person and he or she is much less than that, it could be a difficult chasm to bridge.
That said, in the long-term relationship at least, as the rose-colored glasses of infatuation begin to clear, consciously grade the partner and perhaps yourself on sexual compatibility, as well as on kindness, well-adjustedness, financial self-sufficiency, having a rewarding life outside of the relationship, and the key emotional catch-all: love.
In rating yourself on those, do you see an area for growth that you want to address?
Question external pressure. Peers and parents may verbally, or by example, pressure you to “move forward” and marry and perhaps have kids. Weigh their input wisely: Given your situation, to what extent would your life be better or worse, long term, by “moving forward” rather than going slower, or even, at least for a while, going solo?
I read this aloud on YouTube.
Other installments in The Quests series are Finding Good Work , Succeeding on Your First Professional Job, Finding a Good Place to Live , Finding and Growing Money. Finding and Maintaining Diet Discipline , and Finding Peace of Mind .
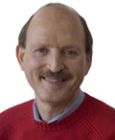
Marty Nemko, Ph.D ., is a career and personal coach based in Oakland, California, and the author of 10 books.
- Find a Therapist
- Find a Treatment Center
- Find a Psychiatrist
- Find a Support Group
- Find Teletherapy
- United States
- Brooklyn, NY
- Chicago, IL
- Houston, TX
- Los Angeles, CA
- New York, NY
- Portland, OR
- San Diego, CA
- San Francisco, CA
- Seattle, WA
- Washington, DC
- Asperger's
- Bipolar Disorder
- Chronic Pain
- Eating Disorders
- Passive Aggression
- Personality
- Goal Setting
- Positive Psychology
- Stopping Smoking
- Low Sexual Desire
- Relationships
- Child Development
- Therapy Center NEW
- Diagnosis Dictionary
- Types of Therapy
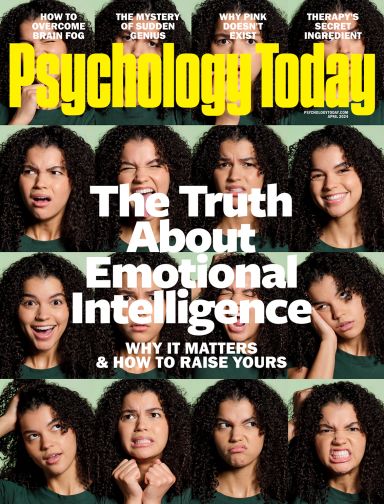
Understanding what emotional intelligence looks like and the steps needed to improve it could light a path to a more emotionally adept world.
- Coronavirus Disease 2019
- Affective Forecasting
- Neuroscience
To read this content please select one of the options below:
Please note you do not have access to teaching notes, relationship building through informal gatherings and technology integrations: a case study.
Library Hi Tech News
ISSN : 0741-9058
Article publication date: 18 January 2024
Issue publication date: 13 February 2024
This case study aims to explore how to provide an informal space to connect members socially, informally and with peers, to provide opportunities for collaboration and informal conversation. The purpose is to analyze the use of technology to promote collaborations and networking within online professional settings.
Design/methodology/approach
In this case study, the authors particularly examine the initiatives as they contribute to the organization, which allows us to understand the interactions between information technology-related tools and online communication.
Successful engagement in informal gatherings was initiated through the planning and implementation with a focus on the following: thematic meetings, time management and participation.
Originality/value
The case study presents an analysis of the use of technology integration into the planning processes that were undertaken by a group of members to enhance informal gatherings for relationship building within a global community of members during the COVID-19 pandemic. The authors suggest ways of engaging users that could be used as a model for organizations alike.
- Informal gatherings
- Relationship building
- Technology integration
- ASIS&T SIG-III
- Community building
- Social interaction
- COVID-19 and online communities
- Social connectedness
Acknowledgements
The authors acknowledge the participation of community members who attended the ICM organized by the ASIS&T SIG-III.
Gala, B. , Reyes, V. , Seifi, L. and Lamba, M. (2024), "Relationship building through informal gatherings and technology integrations: a case study", Library Hi Tech News , Vol. 41 No. 2, pp. 25-28. https://doi.org/10.1108/LHTN-12-2023-0219
Emerald Publishing Limited
Copyright © 2024, Emerald Publishing Limited
Related articles
We’re listening — tell us what you think, something didn’t work….
Report bugs here
All feedback is valuable
Please share your general feedback
Join us on our journey
Platform update page.
Visit emeraldpublishing.com/platformupdate to discover the latest news and updates
Questions & More Information
Answers to the most commonly asked questions here
- Browse All Articles
- Newsletter Sign-Up
CustomerRelationshipManagement →
No results found in working knowledge.
- Were any results found in one of the other content buckets on the left?
- Try removing some search filters.
- Use different search filters.
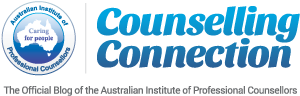
Sign up for your FREE e-newsletter
You’ll regularly recieve powerful strategies for personal development, tips to improve the growth of your counselling practice, the latest industry news and much more.
We’ll keep your information private and never sell, rent, trade or share it with any other organisation. And you can cancel anytime.
Counselling Case Study: Relationship Problems
Mark is 28 and has been married to Sarah for six years. He works for his uncle and they regularly stay back after work to chat. Sarah has threatened to leave him if he does not spend more time with her, but when they are together, they spend most of the time arguing, so he avoids her even more. He loves her, but is finding it hard to put up with her moods. The last few weeks, he has been getting really stressed out and is having trouble sleeping. He’s made a few mistakes at work and his uncle has warned him to pick up his act.
This study deals with the first two of five sessions. The professional counsellor will be using an integrative approach, incorporating Person Centred and Behavioural Therapy techniques in the first session, moving to a Solution Focused approach in the second session. For ease of writing the Professional Counsellor is abbreviated to “C”.
After leaving school at 17, Mark completed a mechanic apprenticeship at a service station owned by his uncle and has worked there ever since. His father died from a heart attack when Mark was six years old and his uncle, who never married, has been a significant influence in his life. He is the youngest of three children, and the only boy in the family. One sister (Anne) is happily married with two children and the other (Erin) is single and works overseas. Mark and his mother have a close relationship, and he was living at home until his marriage.
Some of Mark’s friends are not married and say he was a fool for ‘getting tied down’ so young. Mark used to think that they were just jealous because Sarah is such a ‘knockout’, but lately he has started to wonder if they were right. In the last couple of months, Sarah has been less concerned about her appearance and Mark has commented on this to her. Sarah had been looking for work, but doesn’t seem to do much of anything now.
Three months ago, Sarah found out she can’t have children. According to Mark, she hadn’t spoken about wanting kids so he guessed it wasn’t a big deal to her. When she told him, Mark had joked that at least they wouldn’t have to go into debt to educate them. He thought humour was the best way to go, because he had never been very good at heavy stuff. Sarah had just looked at him and didn’t respond. He asked if she wanted to go out to a movie that night, and she had started to shout at him that he didn’t care about anyone but himself. At that point, he walked out and went to see his brother-in-law, Joe and sister, Anne.
Since then, he and Sarah hardly spoke and when they did it often turned into an argument that ended with Sarah going into the bedroom, slamming the door and crying. Mark usually walked out and drove over to Joe’s place. When Anne tried to talk to Sarah about it, Sarah got angry and told Anne to keep out of it, after all what would she know about it. She had her kids. Joe and Anne had kept their distance since then. Mark talked to his mother, but she said that this was something he and Anne had to work out together. It was she who suggested that Mark come to see C.
Session One
When Mark arrived for the first session, he seemed agitated. C spent some time developing rapport, and eventually Mark seemed to relax a bit. C described the structure of the counselling session, checked if that was ok with Mark, then asked how C could help him.
Mark: “I really wanted Sarah to come; my wife, but she said that I need to sort myself out. I have to tell you, I don’t think counselling is really for men. Women are the ones that like to talk for hours about their problems. I only came here because she insisted and I don’t want her to walk out on me.”
C: “Your marriage is important to you.”
Mark: “Yeah, sure. We’ve had fights before, but they weren’t anything major. And we always made up pretty quickly. But this is different. It seems like whatever I say is wrong, you know? Lately, I haven’t been able to concentrate properly at work and I wake up a lot through the night. I’m feeling really tired and I wish Sarah would get off my case.”
C used encouragers while Mark described what had been happening over the past few months. When he had finished ventilating his immediate concerns, C, moving into Behavioural techniques, summarized and asked Mark to decide what issue he wanted to deal with first. “Mark, you have discussed a number of issues: you are concerned that communication between you and Sarah has been reduced to mostly arguments; you’re unsure how to deal with the fact that Sarah cannot have children; you want to improve your relationship with Sarah; you are worried that Sarah might leave you, and you are feeling very stressed out. What area would you like to work on first?”
Mark: “I just want her to talk to me without arguing. All this is making it really hard for me to concentrate at work, you know.”
C: “Sounds like two goals there, to reduce your stress and to improve communication between Sarah and yourself.”
M: “Yeah, I guess so. If she would just talk to me instead of crying.”
C used open questions and reflections to encourage Mark to look at his feelings. “How do you feel when she goes into the bedroom and starts crying?” Mark: “Well, she’s never been a crier, and I don’t know what to say to her. If I mention not having children, she will probably cry even more.”
C: “So you feel confused about what to do, and anxious that you may upset her even more.”
Mark: “Yes, I just can’t seem to think straight sometimes. Like, I want things to be the way they were, but it’s just getting worse.”
C informed Mark about the use of relaxation techniques to reduce his stress and checked out if he would like to give it a try. “Mark, you appear to be having difficulty coping because you are feeling very stressed. I believe that learning relaxation techniques would decrease the level of stress and help you think more clearly. How does this sound to you?”
Mark: “I’m not into that chanting stuff if that’s what you mean.”
C explained that there are many forms of relaxation and described the deep breathing and muscle tensing method; Mark agreed to do this for 10 minutes twice a day.
As the first session drew to a close, C reviewed the relaxation technique and asked Mark to practise it as often as possible. A second appointment was arranged for the following week.
At the next session, C asked Mark how the relaxation exercise had helped. “I forget to do it some mornings, so I did it for twenty minutes at night instead. I told Sarah what I’m doing and she just leaves me to it. Not sure if it’s making any difference but I’ll keep doing it. It’s nice to have twenty minutes of peace and quiet.” At this point, C moved into a Solution Focused approach.
C congratulated Mark on commencing the relaxation practice, then checked out if it was okay to ask him some different types of questions. Mark agreed and C asked a miracle question. “Imagine that you wake up tomorrow and a miracle has happened. Your problem has been solved. What would other people notice about you that would indicate things are different?”
Mark looked at C, who waited in silence. Eventually Mark responded. “Ok, they would see me and Sarah talking a lot more, without arguing.”
C: “What else would they notice about you?”
Mark: “I would probably be spending more time at home. You know, not staying back so late at work.”
C: “What would they notice that was different about Sarah?”
Mark: “That’s easy. She wouldn’t be crying and yelling all the time.”
C: “So what would she be doing instead?”
Mark: “I guess she would be talking to me, and smiling.”
After spending some time exploring what would be different if the miracle happened, C asked Mark what he had tried in the past to improve communication. Mark revealed that he bought Sarah some flowers and a box of chocolates (his uncle’s suggestion) but it hadn’t really made any difference. C complimented Mark on his efforts and continued with an exception question.
“Can you think of a recent occasion, when you would have expected a quarrel to start and it didn’t?”
Mark furrowed his brow and appeared to be thinking deeply for some time. C waited in silence. Finally, Mark answered. “Actually, about a week ago, I was a bit late home from work and I was expecting another tongue-lashing, but it never came.”
C asked Mark what was different about that night.
Mark: “Well, Sarah was happier.”
C: “How did you know she was happier?”
Mark: “She talked to me, you know, just talked about something she had seen on the telly or something like that.”
C: “And how was that for you, Mark?”
Mark: “Not bad. Actually, it wasn’t too shabby. We did get to chat, and we haven’t done that for ages.”
C: “Can you explain, “Wasn’t too shabby”; I haven’t heard that term before?”
Mark: “Oh, it means it was all good, you know, it was okay.”
C: “So you came home and chatted with Sarah over a cuppa and you found that wasn’t too shabby?” Both smiled
Mark: “I really liked it. I remember thinking I would have come home earlier if I had known it was going to be like that.”
C: “If I was to ask Sarah what was different about that night, what do you think she would say?”
Mark: “Boy, this is getting weird.”
Mark: “Let’s see. She would probably say, “He actually sat and had a cup of coffee with me, instead of just flopping in front of the telly. She’s always griping about that.”
At the appropriate time, C called for a break. “I’d like to take a break and give us both time to consider all the things we’ve talked about. After that, I will give you some feedback.” After the break C summarized what had been discussed and complimented Mark on the work he had put into exploring his problems. He seemed less stressed and had shown that he was committed to improving his relationship with Sarah.
Counselling continued for another three sessions, by which time Mark’s stress had reduced considerably, he was coming home from work earlier and making an effort to talk more to Sarah. The arguments were less frequent and not so heated.
Session Summary
The Person Centred approach allows the client to take the lead and discuss issues as they see them. This encourages the client to talk openly, which was especially useful in this instance since the client showed a reluctance to do so at first.
The Behavioural technique of goal setting is used to clarify what the client wants to achieve out of the sessions.
Solution Focused Therapy, this approach acknowledges that the client has the ability to solve his own problem.
Miracle questions assist the client to examine how they and others would be behaving if the problem were already dealt with. This helps the client to look at their current behaviour and see what they can do to bring about the required change. Exploring what the client has tried in the past highlights that the client is committed to solving the problem. Exception questions help the client to see that there are times when the problem does not occur, and that they have contributed to that situation. This shows the client that they have control over the problem.
Clarifying client’s words, eg. “Not too shabby” shows respect for the client’s language and emphasises that the client is the expert.
Author: Jan McIntyre
Related Case Studies: A Case of Acceptance and Letting Go , A Case of Stress , A Case of Using Logical Consequences
- April 16, 2007
- Case Study , Person-centred , Solution-focused
- Case Studies , Relationship & Families
Comments: 1
Thank you! Very well explained. How to build rapport so that client loosens up.
Leave a Reply Cancel reply
Your email address will not be published. Required fields are marked *

An official website of the United States government
The .gov means it’s official. Federal government websites often end in .gov or .mil. Before sharing sensitive information, make sure you’re on a federal government site.
The site is secure. The https:// ensures that you are connecting to the official website and that any information you provide is encrypted and transmitted securely.
- Publications
- Account settings
Preview improvements coming to the PMC website in October 2024. Learn More or Try it out now .
- Advanced Search
- Journal List
- HHS Author Manuscripts

Creating Good Relationships: Responsiveness, Relationship Quality, and Interpersonal Goals
Amy canevello.
Research Center for Group Dynamics, Institute for Social Research, University of Michigan
Jennifer Crocker
Research Center for Group Dynamics, Institute for Social Research, and Department of Psychology, University of Michigan
Perceived partner responsiveness is a core feature of close, satisfying relationships. But how does responsiveness originate? Can people create relationships characterized by high responsiveness, and consequently, higher quality relationships? We suggest that goals contribute to cycles of responsiveness between people, improving both people’s relationship quality. The present studies examine 1) how interpersonal goals initiate responsiveness processes in close relationships, 2) the self-perpetuating nature of these processes, and 3) how responsiveness evolves dynamically over time through both intrapersonal projection and reciprocal interpersonal relationship processes. In a semester-long study of 115 roommate dyads, actors’ compassionate and self-image goals predicted a cycle of responsiveness between roommates, occurring within weeks and across the semester. In a 3-week study of 65 roommate dyads, actors’ goals again predicted cycles of responsiveness between roommates, which then contributed to both actors’ and partners’ relationship quality. Results suggest that both projection and reciprocation of responsiveness associated with compassionate goals create upward spirals of responsiveness that ultimately enhance relationship quality for both people.
High quality close relationships contribute to mental and physical well-being; poor quality close relationships create stress and undermine health and well-being (e.g., Baumeister & Leary, 1995 ; Uchino, Cacioppo, & Kiecolt-Glaser, 1996 ). Relationship quality depends on beliefs about a relationship partner’s responsiveness--that is, on the perception that a partner understands, values, and supports important aspects of the self. People who perceive their relationship partners as responsive feel close, satisfied, and committed to those relationships ( Reis, Clark, & Holmes, 2004 ).
The present studies focus on the dynamic of responsiveness in dyadic relationships -- relationship processes that promote or undermine reciprocation of responsiveness between relationship partners, affecting both partners’ relationship quality over time. We suggest that people’s interpersonal goals for their relationships, that is their compassionate goals to support others and their self-image goals to create and maintain desired self-images ( Crocker & Canevello, 2008 ), predict positive and negative responsiveness dynamics respectively, changing both people’s relationship quality. In this way, people can create responsive, high-quality relationships for themselves and others.
Responsiveness in Relationships
Responsive relationship partners convey understanding, validation, and caring ( Gable & Reis, 2006 ). They are warm, sensitive to their partners’ feelings, and want to make their partners feel comfortable, valued, listened to, and understood.
Existing theory and research on responsiveness suggests that people’s responsiveness to partners contributes to both their own and partners’ perceptions of responsiveness in the relationship. Lemay and colleagues ( Lemay & Clark, 2008 ; Lemay, Clark, & Feeney, 2007 ) found that people contribute to their own experiences of responsiveness in close relationships; when people report being responsive to relationship partners, they project their responsiveness onto partners and perceive partners as more responsive. Other researchers characterize responsiveness as a transactional process between relationship partners. Reis and Shaver (1988) hypothesize that close relationships develop through an interpersonal process in which actors’ reactions to partners influence partners’ perceptions of actors’ responsiveness. Importantly, Reis and Shaver speculate that goals, motives, needs, and fears of both relationship partners contribute to and result from responsiveness in the relationship. That is, goals and motives predict people’s relationship behaviors and how they interpret partners’ behaviors, which in turn, feed back to predict goals and motives.
The present studies examine both intrapersonal and interpersonal processes of responsiveness and contribute to the responsiveness literature in three important ways. First, as suggested by Reis and Shaver (1988) , interpersonal goals should predict responsiveness processes in close relationships. However, no research that we know of explicitly examines the motivational underpinnings of responsiveness, whether based on projection or reciprocation. We propose that actors’ compassionate goals to support others and self-image goals to construct and maintain desired self-images shape their responsiveness to relationship partners. Through projection, actors’ responsiveness affects their perceptions of their partners’ responsiveness, and hence their own relationship experiences. Through partners’ perceptions and reciprocation of actors’ responsiveness, actors’ responsiveness affects both actors’ and partners’ relationship experiences. We suggest that because projection is an intrapersonal processes and the exchange of responsiveness between relationship partners is an interpersonal process, both can occur simultaneously. That is, people can project their responsiveness onto others, affecting their own relationship experiences, and at the same time, convey responsiveness to relationship partners, affecting partners’ relationship experiences.
Second, projected and reciprocated responsiveness can become self-perpetuating: relationship goals promote or undermine projection and reciprocation of responsiveness, which reinforce both people’s subsequent relationship goals. Thus, through their interpersonal goals, people can create responsive, high-quality relationships for themselves and others and contribute to both people’s goals for the relationship.
Third, to our knowledge, the present studies are the first to examine both immediate and long-term intra- and interpersonal responsiveness dynamics and resulting relationship outcomes as they evolve over time. Previous research suggests that these processes should occur quickly within relationships, guiding people’s relationship experiences and goals in the moment (e.g., Laurenceau, Barrett, & Pietromonaco, 1998 ; Lemay et al., 2007 ). We hypothesize that the effects of compassionate and self-image goals and responsiveness extend over time, predicting change in people’s relationship experiences and goals from day to day and week to week, and that chronic interpersonal goals predict long-term changes in relationship experiences and interpersonal goals over weeks and months. Thus, we propose that projection of responsiveness and reciprocation of responsiveness dynamically affect short-term fluctuations and long-term changes in relationship outcomes.
Figure 1 illustrates our general model in a relationship between an actor (A) and a relationship partner (P). We highlight intra- and interpersonal aspects of the model and detail them below.

Hypothesized theoretical model of interpersonal goals, responsiveness, and relationship quality.
Intrapersonal Process: A’s Compassionate and Self-Image Goals and Responsiveness Predict A’s Relationship Experience
We hypothesize an intrapersonal model examining how people’s goals contribute to their own experiences of responsiveness and resulting relationship goals and quality. Our model extends the intrapersonal projection of responsiveness described by Lemay and colleagues ( Lemay & Clark, 2008 ; Lemay et al., 2007 ), by showing how actors’ goals can be the starting point for change in their responsiveness to partners, which is projected onto partners and leads to change in actors’ goals and relationship outcomes. Paths A–E in Figure 1 show our hypothesized intrapersonal model of goals and responsiveness: A’s interpersonal goals predict change in A’s responsiveness (Path A), which predicts change in A’s perceptions of P’s responsiveness (Path B), with consequences for change in A’s subsequent responsiveness (Path C), goals (Path D), and relationship quality (Path E).
Paths G–K of Figure 1 are a mirror image of the intrapersonal processes in paths A–E, but for partners rather than actors: P’s compassionate goals predict P’s increased and self-image goals predict P’s decreased responsiveness to A (Path G). P’s responsiveness to A predicts P’s increased perceptions of A’s responsiveness (Path H), which then leads to P’s increased responsiveness (Path I), increased compassionate and decreased self-image goals (Path J), and increased relationship quality (Path K).
Below, we present the rationale for each path in the intrapersonal model.
Path A: A’s compassionate and self-image goals predict change in A’s responsiveness
We propose that two types of relationship goals shape responsiveness to relationship partners. Self-image goals focus on constructing, maintaining, and defending desired public and private images of the self ( Crocker & Canevello, 2008 ). When people have self-image goals, they care about what others think of them, but not what others need; consequently they should be less responsive. Compassionate goals focus on supporting others, not to obtain something for the self, but out of concern for others’ well-being ( Crocker & Canevello, 2008 ). When people have compassionate goals, they want to be a constructive force in their interactions with others, and avoid harming them. We suggest that when people have compassionate goals they are more responsive, because they care about others’ well-being. 1
People with chronically high compassionate goals report greater private self-consciousness, lower psychological entitlement, believe that it is possible for both people in a relationship to have their needs met, and believe that it is important that people look out for one another; they trust in and feel closer to others and report both giving and receiving more social support ( Crocker & Canevello, 2008 ). These findings suggest that when people have compassionate goals they understand and trust that when they are responsive to others, they create an environment in which others will respond to them. In contrast, people with chronically high self-image goals report higher psychological entitlement, believe that good outcomes for one person come at the expense of others, and feel that it is important to look out for themselves, even at the expense of others; they report higher loneliness, more conflict with others, and lower interpersonal trust ( Crocker & Canevello, 2008 ). These findings suggest that when people have self-image goals they feel a sense of scarcity and fear that their needs will not be met in collaboration with others. Based on these findings, we propose Path A: When A has the goal to care for and support P, A will become more responsive to P, whereas when A has the goal to create and maintain desired self-images, A will become less responsive to P.
Path B: A’s responsiveness to P predicts A’s increased perceptions of P’s responsiveness
When actors believe they are responsive to partners, they project their own responsiveness onto partners and perceive partners as more responsive ( Kenny & Acitelli, 2001 ; Lemay & Clark, 2008 ; Lemay et al., 2007 ). Several factors might moderate this association. For example, actors who have low self-esteem might feel their partners do not value them and perceive their partners as unresponsive ( Murray, Griffin, Rose, & Bellavia, 2003 ). Also, because of their over-involvement with others and self-neglect, actors high in unmitigated communion might want to see themselves as self-sacrificing and see their partners as unresponsive ( Helgeson & Fritz, 1998 ). However, despite these specific circumstances, in general we expect a strong association between responsiveness and perceptions of partners’ responsiveness. These considerations lead us to propose Path B: A’s responsiveness to P predicts A’s increased perceptions of P’s responsiveness.
Path C: A’s perception of P’s responsiveness predicts A’s increased responsiveness to P
When actors perceive their partners as responsive, they are more responsive in return; when they perceive their partners as unresponsive, actors are less responsive in return ( Fruzzetti, Jacobson, & Blechman, 1990 ; Gable & Reis, 2006 ; Patterson, 1976 ; Plickert, Côté, & Wellman, 2007 ). This may happen for several reasons. People may reciprocate responsiveness out of caring. Actors’ responsiveness strengthens partners’ social bonds to actors, including feelings of caring, connection, and trust, leading partners to want to be responsive to actors (e.g., Brown & Brown, 2006 ; Clark, Fitness, & Brissette, 2004 ). In established communal relationships, partners experience more positive mood and less negative mood when they reciprocate support to actors, compared to when they do not ( Gleason, Iida, Bolger, & Shrout, 2003 ). Even in new relationships, reciprocity of responsiveness may be the result of social exchange norms in which both partners focus on an equal exchange of responsiveness ( Clark & Mills, 1993 ; Mills & Clark, 1982 ). Given this evidence, we propose Path C: A’s perception of P’s responsiveness predicts A’s increased responsiveness to P.
Path D: A’s perception of P’s responsiveness predicts change in A’s compassionate and self-image goals
Actors’ perceptions of partners’ responsiveness should shape actors’ compassionate and self-image goals toward the partner. Actors who perceive partners as responsive feel validated, understood, and cared for ( Reis et al., 2004 ), which fosters a sense of security and permits a shift in focus from protecting the self to supporting others ( Mikulincer, Shaver, Gillath, & Nitzberg, 2005 ; Murray, Holmes, & Collins, 2006 ). In other words, actors’ feelings that partners are responsive to them should foster compassionate goals for partners. Unresponsiveness, on the other hand, conveys a partners’ lack of interest in or concern for actors. Perceptions of partners’ unresponsiveness may signal to actors that they should protect themselves from uncaring partners ( Clark & Monin, 2006 ; Murray et al., 2003 ; Murray, Rose, Bellavia, Holmes, & Kusche, 2002 ) and, as a result, actors should increase in self-image goals. These considerations lead us to propose Path D: A’s perception of P’s responsiveness predicts A’s increased compassionate and decreased self-image goals.
Path E: A’s perception of P’s responsiveness predicts A’s increased relationship quality
Perceived partner responsiveness is crucial to relationship quality ( Clark & Mills, 1993 ; Laurenceau et al., 2004 ; see Reis et al., 2004 for a review). Actors who believe that partners are responsive feel closer, more intimate, and more satisfied with their relationships ( Berg & Archer, 1982 ; Collins & Feeney, 2000 ; Cutrona, Shaffer, Wesner, & Gardner, 2007 ; Davis, 1982 ; Laurenceau et al., 1998 ; Lemay et al., 2007 ). When actors perceive partners as unresponsive, they experience decreased satisfaction, commitment, and closeness in those relationships ( Fincham & Beach, 1999 ; Gottman & Levenson, 1992 ). Consequently, we predict Path E: A’s perception of P’s responsiveness predicts A’s increased relationship quality.
Interpersonal Process: A’s compassionate and self-image goals and responsiveness lead to P’s relationship experience and goals
In addition to this purely intrapersonal process, we hypothesize an interpersonal model in which people’s goals and responsiveness contribute to relationship partners’ experience of actors’ responsiveness, leading to reciprocation of responsiveness and resulting relationship goals and quality. We draw from previous theory and research suggesting that responsiveness is a dyadic process whereby partners perceive actors’ responsiveness and respond in turn (e.g., Collins & Feeney, 2000 ; Reis & Shaver, 1988 ). We hypothesize that actors’ goals can also be the starting point for creating responsiveness dynamics between relationship partners, with consequences for partners’ responsiveness to actors, goals, and relationship quality. Paths A, F, I, J, and K in Figure 1 depict our interpersonal model, in which A’s goals predict change in A’s responsiveness to partners (Path A), which predicts change in P’s perceptions of A’s responsiveness (Path F), with consequences for change in P’s subsequent responsiveness (Path I), goals (Path J), and relationship quality (Path K).
Paths G, L, C, D, and E of Figure 1 are a mirror image of the interpersonal processes in Paths A, F, I, J, and K, but show effects of partners’ goals and responsiveness on change in actors’ relationship experiences: P’s compassionate and self-image goals predict change in P’s responsiveness to A (Path G). P’s responsiveness to A predicts A’s increased perceptions of P’s responsiveness (Path L), which then leads to A’s increased responsiveness, increased compassionate and decreased self-image goals, and increased relationship quality (Paths C, D, and E).
As described previously in our rationale for the intrapersonal model, we propose Path A: that A’s interpersonal goals predict change in A’s responsiveness to P.
Path F: A’s responsiveness predicts P’s increased perceptions of A’s responsiveness
Relationship researchers assume that partners’ perceptions of actors have some basis in actors’ behaviors ( Kelley et al., 1983 ). Most theories of interpersonal relationships assume that actors’ responsiveness to partners predicts partners’ perceptions of actors’ responsiveness (e.g. Bowlby, 1969 ; Gable & Reis, 2006 ; Kelley & Thibaut, 1978 ; I. G. Sarason, Pierce, & Sarason, 1990 ); empirical research supports this prediction ( Abbey, Andrews, & Halman, 1995 ; Bolger, Zuckerman, & Kessler, 2000 ; Collins & Feeney, 2000 ; Feeney & Collins, 2003 ; Lemay & Clark, 2008 ; Vinokur, Schul, & Caplan, 1987 ). For example, in romantic couples when actors disclosed a stressful problem to partners, partners’ reports of their own responsiveness (i.e., responsiveness, listening, understanding, not criticizing, giving support, and expressing concern) positively predicted actors’ perceptions of partners’ responsiveness ( Collins & Feeney, 2000 ). Consequently, we propose Path F: A’s responsiveness to P predicts P’s increased perceptions of A’s responsiveness.
Paths I, J, and K: P’s perceptions of A’s responsiveness and change in P’s relationship experience
Using the same rationale to describe Paths C, D, and E previously, we propose Paths I, J, and K, respectively: P’s perceptions of A’s responsiveness has consequences for P’s increased responsiveness (Path I), increased compassionate and decreased self-image goals (Path J) and increased relationship quality (Path K).
Overview of Present Studies
In two studies of first-semester college freshman roommates, we tested 1) how interpersonal goals initiate projection and reciprocal responsiveness in close relationships, 2) the self-perpetuating nature of these processes, and 3) how responsiveness evolves dynamically over time through both intrapersonal projection and reciprocal interpersonal relationship processes. First semester college students provide an interesting population for examining these processes. Roommates in these samples did not know each other before living together, so their relationships are relatively unbiased by relationship history and past interactions. Unlike most close relationships, previously unacquainted roommates do not self-select into the relationship. At the same time, many first-year students experience significant disruption of their social lives. When they move away from home to attend college, they must build a social network. Their roommates are often the first people they meet and with whom they spend significant time.
Study 1 tested whether students’ compassionate and self-image goals predict a cycle of projected and reciprocal responsiveness between roommates with implications for both people’s relationship goals. Study 2 reports previously unpublished data from the Roommate Goals Study ( Crocker & Canevello, 2008 , Study 2), examining the implications of these processes for both roommates’ relationship quality.
College roommates completed pretest, posttest, and 10 weekly questionnaires, each including measures of compassionate and self-image goals, responsiveness to roommates, and perceived roommates’ responsiveness. We tested associations between students’ goals and 1) the intrapersonal process predicting their own experiences of responsiveness, and 2) the interpersonal process predicting their roommates’ experiences of responsiveness.
We tested a number of alternative explanations and moderators of these processes in Study 1. First, self-disclosure elicits responsiveness from others (e.g., Greene, Derlega, Mathews, Vangelisti, & Perlman, 2006 ; Reis & Patrick, 1996 ; Reis & Shaver, 1988 ). Associations between goals and responsiveness to roommates could be due to perceptions of roommates’ disclosure, and associations between responsiveness to roommates and perceptions of roommates’ responsiveness could be due to disclosure to roommates.
Second, we sought to distinguish responsiveness from social support. Previous research shows that compassionate and self-image goals predict change in perceived available support and supportive behaviors ( Crocker & Canevello, 2008 ). The present studies focus on responsiveness, which we hypothesize is a specific type of support. Support is often broadly defined, including perceptions of support availability and frequency of supportive behaviors (B. R. Sarason, Shearin, Pierce, & Sarason, 1987 ) and includes structural (e.g., group membership or family relationships) and functional components (e.g., providing tangible or emotional support) ( Uchino, 2004 ). Responsiveness refers to people’s sensitivity to partners and desires that partners feel valued, listened to, and understood. Researchers differ in how they view the relation between responsiveness and support; some argue that support is a component of responsiveness (e.g., Reis et al., 2004 ); others conceptualize responsiveness as a subset of social support, distinguishing between responsive and unresponsive support (e.g., Collins & Feeney, in press ). Regardless, researchers agree that responsiveness and support are distinct but related constructs; support providers may not be perceived as responsive. We tested whether support made available to roommates and perceived available social support from roommates explained the effects of responsiveness to roommates and perceptions of roommates’ responsiveness, respectively.
Third, we examined whether negative mood accounts for or moderates the hypothesized associations. For example, the association between interpersonal goals and responsiveness to others might be spurious, if both are associated with anxious or depressed feelings. Feeling anxious or depressed might also moderate these associations. For example, the relation between responsiveness to roommates and perceptions of roommates’ responsiveness may be particularly strong when people do not feel anxious or depressed.
We controlled for students’ self-disclosure to their roommates and their perceptions of their roommates’ disclosure, social support made available to and perceived available support from roommates, and anxiety and depression to rule them out as alternative explanations.
Participants
One hundred fifteen first-semester same-sex freshmen roommate dyads at a large Midwestern university who did not know each other prior to college volunteered for a study of goals and roommate relationships during the fall semester. Via advertisements in the campus newspaper and flyers, we offered each roommate $60 for completing 12 surveys over 10 weeks ($10 for each the pretest and posttest and $4 for each weekly survey) plus a $40 bonus for completing all 12 surveys. One hundred nine pairs (95%) completed the pretest, posttest, and at least 8 weekly surveys. Although 6 pairs completed fewer parts of the study, we retained all data for analyses where possible. 2 Eighty-six pairs (75%) were female. Seventy-five percent of participants reported their race as White or European-American, 2% as Black or African-American, 15% as Asian or Asian-American, and 8% selected other. The racial composition of the sample closely approximated the racial composition of the incoming freshman class. Participants ranged in age from 18 to 21 years ( M = 18.1 years, SD = .36).
In groups of 1 to 8, roommate pairs attended a 1.5 hour session to learn about the study, give their consent, complete the pretest survey, and receive instructions for completing the remaining 11 surveys. All surveys were administered using UM Lessons software. After completing the pretest survey, participants were instructed to complete the 10 weekly online surveys in privacy and not to discuss their responses with each other. The weekly surveys took about 30 minutes to complete and roommates were required to complete weekly surveys within no less than 48 hours of each other. To retain as many participants as possible in the study, participants were given up to 11 weeks to complete the 10 weekly surveys. 3 Once roommates had completed 10 weekly surveys, they completed the posttest survey and were paid for their participation.
Participants completed measures of compassionate and self-image goals, responsiveness to roommates, perceptions of roommates’ responsiveness, disclosure to and from roommates, support made available to roommates, available support from roommates, anxiety, and depression at pretest, posttest, and weekly. At pretest, participants completed questions about demographics (gender, race/ethnicity, age, parental income). Additional measures not germane to the goals of the present investigation were also included.
Self-image and compassionate goals for participants’ relationships with their roommates were measured using a modified measure from Crocker & Canevello (2008) . Pretest and posttest items began with the phrase, “In my relationship with my roommate, I want/try to." Weekly items began with “This week, in my relationship with my roommate, I wanted/tried to.” All items were rated on a scale ranging from 1 ( not at all ) to 5 ( extremely ). Eight items assessed compassionate goals: “be supportive of my roommate;” "have compassion for my roommate's mistakes and weaknesses;" "be aware of the impact my behavior might have on my roommate's feelings;" “make a positive difference in my roommate’s life;” "avoid neglecting my relationship with my roommate;" "avoid being selfish or self-centered;" "be constructive in my comments to my roommate;" and "avoid doing things that aren’t helpful to me or my roommate." Six items reflected self-image goals, including "avoid showing my weaknesses;" “avoid revealing my shortcomings or vulnerabilities;” "avoid the possibility of being wrong;" "convince my roommate that I am right;" "get my roommate to do things my way;" and "avoid being blamed or criticized." Both scales had high internal consistency at pretest (self-image α = .79; compassionate α = .75), posttest (self-image α = .87; compassionate α = .94), and across participants and weeks (self-image goals: .83 < α < .91, M α = .88; compassionate goals: .85 < α < .94, M α = .91).
Responsiveness to roommates and perceptions of roommates’ responsiveness were measured with a 6-item modified version of a responsiveness measure used in previous research ( Cutrona, Hessling, & Suhr, 1997 ; Gore, Cross, & Morris, 2006 ). Participants indicated how they acted toward their roommate in general at pretest and posttest. All items were rated on a scale from 1 ( not at all ) to 5 ( very much ). Items included “I try to make my roommate feel comfortable about him/herself and how he/she feels;” "I try to make my roommate feel valued as a person;" "I try to be sensitive to my roommate’s feelings;" “I really try to understand my roommate’s concerns;" “I really listen to my roommate when he/she talks;” and “I behave warmly toward my roommate.” We measured weekly responsiveness using the same items, asking how participants acted toward their roommate that week. Responsiveness was reliable at pretest (α =.93), posttest (α = .97) and in each weekly survey (.94 < α < .98, M α = .97).
A parallel set of items assessed the extent to which participants believed their roommates responded to them. Pretest and posttest items asked about roommates’ general responsiveness. Sample items included “my roommate tries to make me feel comfortable about myself and how I feel;” and "my roommate tries to make me feel valued as a person." We measured weekly roommate responsiveness with the same items, referring to how roommates acted toward participants that week. Perceptions of roommates’ responsiveness was reliable at pretest (α = .95), posttest (α =.98), and in the weekly surveys (.94 < α < .98, M α = .97).
Disclosure to the roommate and perceptions of roommates’ disclosure were measured with a 5-item modified version of a disclosure measure used by Gore and colleagues (Gore et al., 2006a; Miller, Berg, & Archer, 1983 ). Participants were instructed to indicate the extent to which they discussed each topic with their roommates; pretest and posttest items began with the phrase, “In general, I discuss:." All items were rated on a scale from 1 ( discussed not at all ) to 5 ( discussed fully and completely ) and included “my deepest feelings;” “my worst fears;” “what I like and dislike about myself;” “my close relationships with other people;” and “things I have done which I am proud of.” We measured weekly disclosure using the same instructions and items, beginning with the phrase “This week, I discussed:.” Disclosure to roommates was reliable at pretest (α =.85), posttest (α = .94) and from week to week (.85 < α < .95, M α = .92).
A parallel set of items assessed the extent to which participants believed their roommates self-disclosed. Pretest and posttest items began with the phrase, “In general, my roommate discusses:." Sample items included “his/her deepest feelings;” “his/her worst fears;” and “what he/she likes and dislikes about him/herself.” We measured weekly roommate disclosure with the same items, referring to the extent to which roommates self-disclosed that week. Roommate disclosure was reliable at pretest (α = .89), posttest (α =.94), and in weekly surveys (.89 < α < .95, M α = .93).
Perceived social support availability from roommates and support made available to roommates were measured with the Multidimensional Survey of Perceived Social Support ( Zimet, Dahlem, Zimet, & Farley, 1988 ). Perceived availability pretest and posttest items were preceded with the stem “In general, I feel that.” Weekly items were preceded with the stem “This past week, I felt that.” Sample items included “My roommate really tried to help me” and “I could count on my roommate if things went wrong.” Perceived social support availability was reliable at pretest (α =.93), posttest (α = .96) and from week to week (.93 < α < .97, M α = .96).
Social support made available to roommates was also measured at pretest, posttest and weekly using a parallel set of items. Sample items included “I really tried to help my roommate” and “my roommate can count on me when things go wrong.” Social support made available to roommates was reliable at pretest (α = .92), posttest (α =.95), and in weekly surveys (.86 < α < .96, M α = .94).
Anxiety was assessed with the Speilberger State Anxiety Scale ( Spielberger, Vagg, Barker, Donham, & Westberry, 1980 ). At pretest and posttest, participants rated their anxiety in general on a scale ranging from 1 (never) to 5 (always); in the weekly surveys, they rated their anxiety over the past week on the same scale. Anxiety had high internal consistency at pretest (α =.91), posttest (α =.94), and in each of the weekly surveys (.94 < α < .95, Mα = .94).
Depression was assessed at pretest, posttest, and weekly using the Center for Epidemiological Studies Depression Inventory (CES-D; Radloff, 1997). The CES-D was developed to measure depressive symptoms in community samples and consists of 20 depression-related symptom items rated on a 4 point scale (0–3) based on the amount of time during the past week the respondent has experienced each symptom. Scores can range from 0 to 60. The CES-D had high internal consistency at pretest (α =.86), posttest (α =.89) and each of the weekly surveys (.90 < α < .92, Mα = .91).
Factor Analyses
Table 1 shows the means, standard deviations, and intrapersonal (i.e., within-person) intraclass correlations, which adjust for the degree of nonindependence between dyad members ( Griffin & Gonzalez, 1995 ) for all primary variables in Study 1. Because correlations between compassionate goals and responsiveness to roommates and perceptions of roommates’ responsiveness were high, we conducted exploratory factor analyses (EFA) on these items at pretest and confirmatory factor analyses (CFA) on items at posttest and each week, comparing the fit of a model specifying 2 factors with a model specifying 1 factor.
Study 1 means, standard deviations, and intrapersonal intraclass correlations for all primary pretest, posttest, and mean weekly variables.
N = 230 at pretest, N = 218 at posttest. Chronic scores were calculated by averaging across the weekly reports. Self-image and compassionate goals were measured on a scale ranging from 1 (never) to 5 (always). Responsiveness to roommates and perceptions of roommates’ responsiveness were measured on a scale ranging from 1 (not at all) to 5 (very much).
Compassionate goals and responsiveness to roommates
Compassionate goals and responsiveness to roommates, though correlated, are empirically distinct. EFAs on the pretest items suggested that 2 factors accounted for 48% of the variance: all responsiveness items loaded on the first factor, with loadings ranging between .64 and .99; all compassionate goal items loaded on the second factor, with loadings ranging between .38 and .66. Importantly, no secondary loading exceeded |.28|. We conducted CFAs on items at posttest and each of the 10 weeks (yielding 11 separate sets of CFAs), testing two-factor, 136.84 < χ 2 (76, 218 < N < 230) < 232.48, Mχ 2 (76, 218 < N < 230) = 183.77, and single-factor solutions, 336.77 < χ 2 (77, 218 <N < 230) < 726.72, Mχ 2 (77, 218 < N < 230) = 586.71. For all analyses, two-factor solutions provided significantly better fit, 194.33 < Δχ 2 (1, 218 < N < 230) < 554.95, MΔχ 2 (1, 218 < N < 230) = 402.94.
Compassionate goals and perceptions of roommates’ responsiveness
Compassionate goals and perceptions of roommates’ responsiveness, though correlated, are also empirically distinct. EFAs on the pretest items suggested that 2 factors accounted for 51% of the variance: all responsiveness items loaded on the first factor, with loadings ranging between .74 and .93; all compassionate goal items loaded on the second factor, with loadings ranging between .39 and .63. Importantly, no secondary loading exceeded |.23|. We conducted CFAs on items at posttest and each of the 10 weeks (again, yielding 11 separate sets of CFAs), testing two-factor, 110.55 < χ 2 (76, 218 < N < 230) < 247.82, Mχ 2 (76, 218 < N < 230) = 166.94, and single-factor solutions, 448.56 < χ 2 (76, 218 < N < 230) < 948.77, Mχ 2 (76, 218 < N < 230) = 753.14. For all analyses, two-factor solutions provided significantly better fit, 338.01 < Δχ 2 (1, 218 < N < 230) < 747.22, MΔχ 2 (1, 218 < N < 230) = 586.20.
Overview of Primary Analyses
We conducted data analyses in two phases. In Phase 1 we focused on the intrapersonal associations between goals and responsiveness. We hypothesized that students’ goals would predict change in their responsiveness to roommates (Path A; Figure 1 ), which would predict change in their perceptions of their roommates’ responsiveness (Path B), which would in turn, predict change in their compassionate and self-image goals (Path D). In Phase 2 we focused on the interpersonal associations among these variables to examine how actors’ goals predict change in their responsiveness to partners (Path A), which predicts change in partners’ perceptions of actors’ responsiveness (Path F), which predicts change in partners’ subsequent responsiveness to actors (Path I) and goals (Path J). We tested both the intra- and interpersonal associations 1) within weeks, 2) from week to week using lagged analyses, and 3) across the semester from pretest to posttest.
Importantly, all intra- and interpersonal analyses assess change. For example, in weekly analyses we test whether fluctuations in goals (i.e., the difference between goals that week and that person’s average goals across 10 weeks) predict responsiveness that week; in lagged analyses, we test whether Week 1 goals predict change in responsiveness from Weeks 1 to 2; and in pretest and posttest analyses, we test whether chronic goals predict change in responsiveness from pretest to posttest. Thus, these analyses test the dynamic intra- and interpersonal associations between goals, responsiveness, and perceptions of others’ responsiveness.
General Analytic Strategy
In these data, individuals were nested within dyads and dyads were crossed with weeks ( Kashy, Donnellan, Burt, & McGue, 2008 ). Thus, we controlled for the nonindependence of individuals within dyads in all analyses using the MIXED command in SPSS ( Campbell & Kashy, 2002 ; Campbell, Simpson, Boldry, & Kashy, 2005 ; Kenny, Kashy, & Cook, 2006 ), and because individuals within dyads were indistinguishable, we specified compound symmetry so that intercept variances between dyad members were equal. For all analyses, we structured the data so that each dyad was represented by two lines of data, allowing each participant within a dyad to represent both an actor and a partner (see Campbell & Kashy, 2002 , for a sample arrangement of data). Path models were tested sequentially, with a separate regression equation for each path. For each path, we regressed the criterion on the predictor(s), controlling for all variables preceding that path in the model. All Study 1 path analyses are illustrated in Figure 2 and Figure 3 . Estimates outside of brackets indicate the partial correlation for that association, controlling for previous paths in the model; estimates inside brackets indicate tests of the individual path, not controlling for previous paths in the model. Partial correlations for all analyses were calculated using the method described by Rosenthal and Rosnow (1991) .

Study 1: Intrapersonal (within-person) path analyses of weekly, lagged-week, and pretest and posttest data. NOTE: All estimates are partial correlations; estimates in brackets indicate test of the individual path, not controlling for previous paths in the model. *** p < .001, ** p < .01, * p < .05.

Study 1: Interpersonal (between-person) path analyses of weekly, lagged-week, and pretest and posttest data. NOTE: All estimates are partial correlations; estimates in brackets indicate test of the individual path, not controlling for previous paths in the model. *** p < .001, ** p < .01, * p < .05.
Table 1 shows the means, standard deviations, and intrapersonal (i.e., within-person) intraclass correlations ( Griffin & Gonzalez, 1995 ), for all primary pretest, posttest, and chronic weekly variables. We created measures of chronic compassionate and self-image goals by averaging each measure across the 10 weeks. In general, compassionate goals, responsiveness, and perceptions of roommates’ responsiveness were strongly correlated at pretest and posttest, and across weeks. Self-image goals were less strongly associated with responsiveness and perceptions of roommates’ responsiveness. Because compassionate and self-image goals were significantly correlated, we regressed all outcome variables on compassionate and self-image goals simultaneously. Table 2 shows the interpersonal (i.e. actor-partner) intraclass correlations for all primary variables. Roommates’ compassionate goals, responsiveness and perceptions of roommates’ responsiveness were moderately correlated across time-points; self-image goals predicted fewer partner variables.
Study 1 interpersonal (i.e. actor-partner) intraclass correlations for all pretest, posttest, and mean weekly variables.
Intrapersonal Processes: Students’ Goals Predicting Their Own Responsiveness and Subsequent Goals
Phase 1 analyses test an intrapersonal model in which students’ compassionate and self-image goals predict change in their responsiveness to roommates (Path A; Figure 1 ), which predicts change in their perceptions of roommates’ responsiveness (Path B), which in turn predicts change in students’ subsequent compassionate and self-image goals (Path D). Thus, all Phase 1 analyses use only actor variables as predictors and outcomes. Note that, because the data are structured so that actors and partners are interchangeable, these analyses simultaneously test the process by which partners’ goals lead to partners’ own responsiveness and goals (i.e., Paths G, H, and J).
Weekly associations
First, we examined our hypothesized model within weeks, testing whether weekly interpersonal goals predicted responsiveness to roommates that same week, which then predicted perceptions of roommates’ responsiveness that week. Coefficients for weekly analyses were derived from random-coefficients models using restricted maximum-likelihood estimation, and models included fixed and random effects for the intercept and each predictor. In weekly analyses we person-centered all predictors so that scores represent differences from each individual’s own average across 10 weeks (e.g., Enders & Tofighi, 2007 ; Kreft & de Leeuw, 1998 ; Raudenbush & Bryk, 2002 ).
Path analyses supported our hypothesized model within weeks (see the top of Figure 2 ). Weekly compassionate goals predicted higher and self-image goals predicted lower weekly responsiveness to roommates. Responsiveness to roommates, in turn, positively predicted higher perceptions of roommates’ responsiveness.
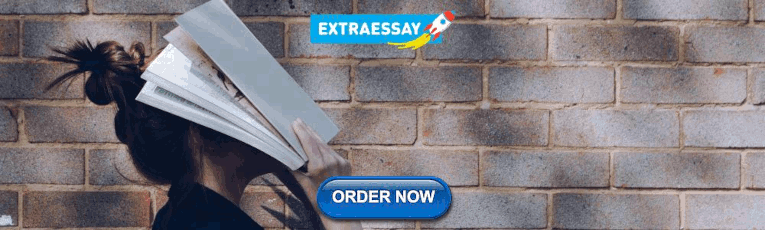
Lagged-week analyses
Next, we tested the lagged-week associations between interpersonal goals, responsiveness to roommates, and perceptions of roommates’ responsiveness. Examination of the temporal sequence of effects across weeks does not demonstrate causality but can shed light on the plausibility or implausibility of causal pathways ( Kenny, 1975 ; Leary, 1995 ; Rogosa, 1980 ; West, Biesanz, & Pitts, 2000 ). For example, evidence that compassionate goals on Week 1 predict responsiveness in Week 2, controlling for responsiveness on Weeks 1 (i.e., testing whether goals one week predict residual change in responsiveness the following week) would be consistent with the hypothesis that compassionate goals cause responsiveness. No association would rule out a causal effect over this time period. Thus, unlike within-week analyses, lagged analyses test the plausibility of causal associations for each hypothesized pathway in our intrapersonal model.
Coefficients for lagged-week analyses were derived from random-coefficients models using restricted maximum-likelihood estimation, with models including fixed and random effects for the intercept and each predictor. We used a residual change strategy to test changes from week to week, regressing the Week N + 1 dependent variable on relevant Week N predictors, controlling for the Week N dependent variable. When change in a variable was a predictor, we entered the Week N and Week N + 1 predictors into the model and interpreted the week N + 1 variable.
We grand mean centered predictors in tests of lagged-week hypotheses because our prediction concerned change in the outcome from week to week. Lagged analyses examine whether change in the outcome from one week to the next is related to levels of the goal (or other predictor), regardless of the source – individual differences or weekly fluctuations around those individual differences. For example, we hypothesize that As’ goals one week predict their responsiveness the following week, controlling for that week’s responsiveness. Person centering predictors tests whether fluctuations in As’ goals from As’ own average goals predict outcomes. Consequently, in our example person-centering predictors in lagged analyses tests whether within-person departures from As’ average goals one week predict As’ responsiveness the following week, controlling for within-person departures from As’ average responsiveness that week. This does not test our lagged hypothesis. Thus, centering on the grand mean for that week is justified and appropriate in these analyses (e.g., Enders & Tofighi, 2007 ). 4
In the lagged-week data, we tested a path model in which goals at Week 1 predict change in responsiveness to roommates from Weeks 1 to 2, which predict simultaneous change in perceptions of roommates’ responsiveness from Weeks 1 to 2, which in turn predict change in compassionate and self-image goals from Weeks 1 to 3. We expected that, in the case of projection, associations between changes in responsiveness to roommates and changes in perceptions of roommates’ responsiveness would be relatively immediate because they occur as a function of perceptions – we expect that when actors become more responsive to roommates, they simultaneously increase their perceptions of roommates’ responsiveness. Accordingly, we hypothesized that change in responsiveness to roommates from Weeks 1 to 2 predicted simultaneous change in perceptions of roommates’ responsiveness.
For each path, we regressed the criterion on the predictor(s), controlling for all variables preceding that path in the model. We tested this path model (i.e., actors’ Week N compassionate and self-image goals predict change in actors’ responsiveness to roommates from Weeks N to N + 1, which predicts change in actors’ perceptions of roommates’ responsiveness from Weeks N to N + 1, which predicts change in actors compassionate and self-image goals from weeks N to N + 2; see the middle of Figure 2 ) in 4 regression equations (except when goals were entered as predictors: because we tested them simultaneously, we were able to test two paths in one equation). Lagged analyses were conducted on all 10 weeks. For simplicity, we refer to Week N as “Week 1,” Week N+1 as “Week 2,” and Week N+2 as “Week 3.”
Lagged-week path analyses supported our hypotheses (see middle of Figure 2 ). Week 1 compassionate goals predicted increased and Week 1 self-image goals predicted decreased responsiveness to roommates from Weeks 1 to 2, which predicted increased perceptions of roommates’ responsiveness from Weeks 1 to 2, which predicted increased compassionate goals and decreased self-image goals from Weeks 1 to 3.
Change across the semester
To test whether and how students’ chronic compassionate and self-image goals contribute to long-term changes in their responsiveness, perceptions of roommates’ responsiveness and goals, we examined a path model in which chronic goals averaged across 10 weeks predicted change in responsiveness to roommates across the semester, which then predicted change in perceptions of the roommates’ responsiveness, which in turn predicted changes in goals from pretest to posttest.
Coefficients for testing change from pretest to posttest were derived from fixed-effects models using restricted maximum-likelihood estimation. We grand mean centered predictors in tests of pretest and posttest hypotheses because we were interested in chronic goals and responsiveness as individual differences. We used a residual change strategy, similar to that used in lagged-week analyses, to test changes from pretest to posttest.
Results partially support our path model (see bottom of Figure 2 ). Chronic compassionate goals predicted increased and chronic self-image goals predicted decreased responsiveness to roommates from pretest to posttest, which predicted change in perceptions of roommates’ responsiveness from pretest to posttest, but perceptions of roommates’ responsiveness did not predict changes in students’ own compassionate and self-image goals from pretest to posttest.
Next, we tested several alternative explanations for and moderators of the associations tested in Figure 2 . We tested whether perceptions of roommates’ responsiveness, disclosure, support, anxiety and depression explained associations in our models by adding the appropriate variables to the path models tested above. Specific analyses for each covariate are described below. Note that our main concern was not whether these covariates were related to each outcome, but whether they could explain or offer an alternative explanation for our findings. Thus we do not report the association between each covariate and outcome variable. Instead, we report associations between our main predictors and outcome variables, controlling for covariates. We also test whether associations in Figure 2 are moderated by anxiety, depression or gender by adding the appropriate main effect and product terms, as described below. Simple slopes for interactions were computed at 1 standard deviation above and below the means of the moderators ( Aiken & West, 1991 ). Because of space considerations, we do not report individual statistics for each covariate test. Instead, we report a summary of results for each covariate; tables of results can be obtained from the first author.
Do perceptions of roommates’ responsiveness explain associations between goals and change in responsiveness to roommates?
Associations between students’ interpersonal goals and changes in their responsiveness to roommates might be attributed to perceptions of roommates’ responsiveness: students’ goals may lead them to be more or less responsiveness to roommates because goals are also associated with perceiving roommates as more or less responsive. We retested the links between compassionate and self-image goals and responsiveness to roommates in all models in Figure 2 , controlling for weekly perceptions of roommates’ responsiveness in weekly analyses, Week 1 perceptions of roommates’ responsiveness in lagged analyses, and chronic perceptions of roommates’ responsiveness in the pretest and posttest analyses. Across all three sets of analyses, all associations between compassionate goals and higher or increased responsiveness remained significant, .17 < pr s < .46, all p s < .001, although perceptions of roommates’ responsiveness predicted higher or increased responsiveness to roommates, .37 < pr s < .40, all p s < .001, across analyses. Thus, students’ perceptions of roommates as more or less responsive do not explain the association between compassionate goals and increased responsiveness to roommates.
On the other hand, 2 of the 3 analyses suggested that associations between students’ self-image goals and lower or decreased responsiveness to roommates could be explained by perceptions of roommates’ responsiveness. Weekly self-image goals no longer predicted weekly responsiveness to roommates, pr = −.03, ns, and chronic self-image goals no longer predicted change in responsiveness from pretest to posttest, pr = −.09, ns. In lagged analyses, Week 1 self-image goals still predicted decreased responsiveness to roommates from Weeks 1 to 2, pr = −.25, p < .001. Thus, students’ self-image goals led to their decreased responsiveness to the extent that they perceived their roommates as less responsive.
Does disclosure explain these associations?
Because others’ disclosure elicits responsiveness and perceptions of roommates’ responsiveness may be a function of people’s own disclosure ( Reis & Shaver, 1988 ), we examined the possibility that associations between responsiveness to roommates and perceptions of roommates’ responsiveness could be explained by perceptions of roommates’ disclosure or disclosure to roommates. We reanalyzed paths in the weekly, lagged-week, and change from pretest to posttest analyses, controlling for the appropriate disclosure variable (i.e., we regressed responsiveness to roommates on goals controlling for perceptions of roommates’ disclosure and we regressed perceptions of roommates’ responsiveness on responsiveness to roommates controlling for disclosure to roommates). In lagged-week analyses we controlled for Week 1 disclosure, or Weeks 1 and 2 disclosure, depending on the specific path we tested. In testing change from pretest to posttest, we controlled for the appropriate chronic or pretest and posttest disclosure variables, again depending on the specific path we tested.
Interpersonal goals predicted responsiveness and responsiveness predicted perceptions of roommates’ responsiveness, independent of disclosure. In 9 of 10 analyses, results remained unchanged when we retested these paths controlling for the appropriate disclosure variables; in the weekly model, the association between weekly self-image goals and responsiveness to roommates became marginally significant when we controlled for perceptions of roommates’ disclosure that week, pr = −.07, p < .06. Thus, people’s interpersonal goals offer an alternative to disclosure in creating responsive close relationships.
Does support availability explain these associations?
These paths might be explained by perceived available support from roommates and support made available to roommates. We reanalyzed all paths, controlling for the appropriate support variable (i.e., when responsiveness to roommates was the criterion, we controlled for support made available to roommates; when perceptions of roommates’ responsiveness or goals were the criterion, we controlled for perceived available support from roommates), using the strategy described above (e.g., in lagged-week analyses we controlled for change in support on the weeks responsiveness variables were included in analyses).
Results remained unchanged when we retested individual paths controlling for the appropriate support variables in 8 of 10 analyses (we did not retest nonsignificant links between perceptions of roommates’ responsiveness and goals). In the weekly model, the association between self-image goals and responsiveness to roommates became nonsignificant when we controlled for perceived available support, pr = −.05, ns, and in the pretest to posttest model, the association between chronic self-image goals and change in responsiveness to roommates became nonsignificant when we controlled for chronic perceived available support, pr = −.09, ns. Thus, self-image goals do not predict change in responsiveness beyond available support: that is, self-image goals may contribute to change in responsiveness because of available support. However, available support cannot explain associations between compassionate goals and change in responsiveness, and support made available to roommates cannot explain the association between students’ responsiveness and their perceptions of roommates’ responsiveness, nor can it explain why students’ perceptions of roommates’ responsiveness predict change in their compassionate goals in the lagged analyses.
Does anxiety or depression explain associations in these models?
We also tested whether the associations in Figure 2 were explained by feeling anxious or depressed. We reanalyzed all paths in weekly, lagged-week, and change from pretest to posttest analyses, controlling for anxiety and depression in separate analyses, using the strategy for testing covariates described above. Results did not change when we controlled for anxiety and depression in 18 of 20 analyses. In the pretest and posttest model, the link between chronic self-image goals and change in responsiveness to roommates became nonsignificant when we controlled for chronic anxiety, pr = −.13, ns, and marginal when we controlled for chronic depression, pr = −.14, p = .07. Thus, anxiety and depression appear to explain why self-image goals lead to longer-term decreases in responsiveness, but they cannot explain why self-image goals lead to decreased responsiveness in weekly and lagged-week analyses, or why compassionate goals lead to higher and increased responsiveness. Anxiety and depression also cannot explain projection of responsiveness or why it leads to increased compassionate goals in the lagged model.
Do associations in these models differ by levels of anxiety or depression?
Because links in the intrapersonal model might depend on negative mood, we tested whether anxiety or depression moderated the simple associations in Figure 2 (i.e., not controlling for other variables in the model), testing 26 separate product terms. Only one was significant: in the weekly model (top of Figure 2 ) anxiety moderated the relation between weekly compassionate goals and weekly responsiveness to roommates, pr = .07, p <.05, such that this association was stronger for those who reported higher anxiety, pr = .33, p <.001, compared to those reporting lower anxiety, pr = .20, p <.001. Results suggested that compassionate goals are beneficial for responsiveness, particularly when anxiety is higher. No other links in the intrapersonal models were moderated by anxiety or depression (all other pr s < |.13|, ns). Thus, results strongly suggest that the processes described by the intrapersonal model do not operate differently depending on negative mood.
Do these associations differ by gender?
Because the intrapersonal process from goals to perceptions of roommates’ responsiveness and change in goals might differ for men and women, we tested whether gender moderated each individual path (i.e., not controlling for other variables in the models) in all models in Figure 2 . In all analyses, gender was treated as a fixed effect (i.e., no random effects were specified in weekly and lagged-week models) and coded such that 1 = men and 2 = women. Gender moderated just 2 of the 13 associations tested (all other pr s < |.07|, ns). First, in the lagged model (the middle of Figure 2 ), gender moderated the association between change in responsiveness to roommates and change in perceptions of roommates’ responsiveness, pr = .25, p < .001, such that the relation was stronger for women, pr = .75, p < .001, than men, pr = .48, p < .001. Second, in tests of pretest to posttest change (the bottom of Figure 2 ), gender moderated the association between change in perceptions of roommates’ responsiveness and change in self-image goals, pr = −.16, p < .05, such that perceptions of roommates’ responsiveness predicted decreased self-image goals for women, pr = −.20, p < .01, but not men, pr = .04, ns .
Summary of intrapersonal processes
These data support our hypothesis that interpersonal goals predict change in responsiveness, which leads to projection of responsiveness: compassionate goals predict increased and self-image goals predict decreased responsiveness to roommates, which predicts increased perceptions of roommates’ responsiveness. This process operates within weeks, from week to week, and across 10 weeks, supporting our hypothesis about the dynamic associations between goals and projection of responsiveness. 5 . Covariates did not consistently account for any of these associations, nor were associations moderated by negative mood or gender. 6
Results were mixed with respect to our hypothesis that the relation between goals and projection is self-perpetuating. Lagged-week analyses supported our hypothesis - increased perceptions of roommates’ responsiveness from Weeks 1 to 2 predicted changes in interpersonal goals from Weeks 1 to 3. However, analyses of change from pretest to posttest did not support this hypothesis – changes in perceptions of roommates’ responsiveness from pretest to posttest did not predict changes in goals from pretest to posttest.
Interpersonal Processes: Actors’ Goals and Responsiveness Predicting Partners’ Goals and Responsiveness
The goal of Phase 2 analyses was to test our interpersonal model whereby actors’ compassionate goals predict their increased and self-image goals predict their decreased responsiveness to partners (Path A; Figure 1 ). Actors’ responsiveness to partners then predicts partners’ increased perceptions of actors’ responsiveness (Path F), which then predicts partners’ increased responsiveness to actors (Path I) and increased compassionate and decreased self-image goals (Path J). Again, note that, because the data are structured so that actors and partners are interchangeable, these analyses simultaneously the process by which partners’ goals predict actors’ responsiveness and goals (i.e., Paths G, L, C, and D). We examined this general model within weeks, from week to week using lagged analyses, and the across the semester using the same analytic strategies described to test our projection (i.e., intrapersonal) hypotheses.
Weekly Associations
We examined our hypothesized interpersonal model within weeks, testing whether actors’ weekly interpersonal goals predicted their responsiveness to roommates that same week, which then predicted partners’ perceptions of actors’ responsiveness that week, which then predicted partners’ interpersonal goals and responsiveness to actors.
Within-week analyses support our hypotheses (see the top of Figure 3 ). On weeks when actors had higher compassionate goals they reported being more responsive to partners, and on weeks when actors had higher self-image goals they reported being less responsive to partners. Actors’ responsiveness to partners predicted partners’ higher perceptions of actors’ responsiveness, which predicts partners’ higher responsiveness to actors and partners’ compassionate goals. Partners’ weekly perceptions of actors’ responsiveness did not predict their own self-image goals that same week.
Again, because lagged analyses provide information about the plausibility of causal pathways, we tested whether actors’ compassionate and self-image goals at Week 1 predicted change in their responsiveness from Weeks 1 to 2, which predicted simultaneous change in partners’ perceptions of actors’ responsiveness from Weeks 1 to 2, which then predicted change in partners’ interpersonal goals and responsiveness to actors from Weeks 1 to 3. We predicted that change in actors’ responsiveness to roommates from Weeks 1 to 2 predicted simultaneous change in partners’ perceptions of actors’ responsiveness from Weeks 1 to 2 because responsiveness transactions between roommates should occur simultaneously (i.e., partners should perceive change in actors’ responsiveness as actors report change in their own responsiveness).
Lagged-week analyses did not support our interpersonal hypotheses (see middle of Figure 3 ). Actors’ Week 1 compassionate goals predicted increased responsiveness and Week 1 self-image goals predicted decreased responsiveness to partners from Weeks 1 to 2, but change in actors’ responsiveness to partners from Weeks 1 to 2 did not predict simultaneous change in partners’ perceptions of actors’ responsiveness from Weeks 1 to 2. Change in partners’ perceptions of actors’ responsiveness from Weeks 1 to 2 positively predicted change in partners’ responsiveness to actors and compassionate goals from Weeks 1 to 3, but did not predict change in partners’ self-image goals from Weeks 1 to 3.
These results do not support the plausibility of causal effects of change in actors’ responsiveness to partners on change in partners’ perceptions of actors’ responsiveness. However, changes in partners’ perceptions of actor’s responsiveness led to their increased responsiveness to actors and compassionate goals the following week.
Change from pretest to posttest
To test whether and how actors’ chronic compassionate and self-image goals contribute to long-term changes in their own responsiveness, and partners’ perceptions of actors’ responsiveness, responsiveness to actors, and goals, we examined a path model in which actors’ chronic goals predicted change in actors’ responsiveness to partners across the semester, which predicted change in partners’ perceptions of actors’ responsiveness, which in turn predicted changes in partners’ goals and responsiveness to actors from pretest to posttest.
Results support our interpersonal model (see bottom of Figure 3 ). Actors’ chronic compassionate goals predict increased and chronic self-image goals predict decreased responsiveness to partners. Change in actors’ responsiveness to partners positively predicted change in partners’ perceptions of actors’ responsiveness from pretest to posttest, which positively predicted change in partners’ responsiveness to actors and compassionate goals and marginally negatively predicted change in partners’ self-image goals across the semester.
Next, we tested several alternative explanations for and moderators of the associations tested in Figure 3 . We tested whether disclosure, available support, anxiety or depression explained associations between actors’ responsiveness to partners and partners’ perceptions of actors’ responsiveness by adding the appropriate variables to the interpersonal path models tested above. Details of these analyses are provided below. Note that, as in tests of covariates in the intrapersonal models, the critical test was whether covariates altered the results of our path models, and not whether the covariates were related to each outcome. Because of this, we do not report the association between each covariate and outcome variable. We also tested whether associations between actors’ responsiveness and partners’ perceptions of actors’ responsiveness were moderated by partners’ own goals, and whether associations unique to the interpersonal models were moderated by anxiety, depression or gender using the strategy described above. Again, because of space considerations, we do not report individual statistics for each covariate test, but instead report a summary of results for each covariate; tables of results can be obtained from the first author.
Do partners’ goals influence how they perceive actors’ responsiveness?
We tested the possibility that the links between actors’ responsiveness and partners’ increased perceptions of actors’ responsiveness were dependent on partners’ goals. For all models in Figure 3 , we tested whether partners’ goals moderated the individual paths (i.e., not controlling for other variables in the models) between actors’ responsiveness to partners and partners’ perceptions of actors’ responsiveness, testing the moderating effect of each goal separately. In the weekly model we tested whether partners’ weekly goals moderated this association; in the lagged analyses we tested whether partners’ Week 2 goals moderated the link between change in actors’ responsiveness to partners from Weeks 1 to 2 and change in partners’ perceptions of actors’ responsiveness from Weeks 1 to 2; in the pretest to posttest analyses we tested whether partners’ posttest goals moderated the link between change in actors’ responsiveness to partners from pretest to posttest and change in partners’ perceptions of actors’ responsiveness from pretest to posttest. Across analyses, partners’ goals did not moderate this association, compassionate goals: −.07 < pr < .02, all ns; self-image goals: all pr s < .02, all ns. Actors’ and partners’ agreement about actors’ responsiveness to partners does not depend on partners’ compassionate or self-image goals.
Does disclosure, available support, anxiety, or depression explain associations between actors’ responsiveness to partners and partners’ perceptions of actors’ responsiveness? 7
We tested associations between actors’ responsiveness to partners and partners’ perceptions of actors’ responsiveness in weekly and change from pretest to posttest models in Figure 3 , separately controlling for partners’ perceptions of actors’ disclosure, partners’ social support available from actors, and partners’ anxiety and depression using a strategy similar to that described for the intrapersonal models. We did not test covariates in the lagged model because there was no association between change in actors’ responsiveness and change in partners’ perceptions of actors’ responsiveness. Results remained unchanged in 7 of 8 tests. Change in actors’ responsiveness to partners from pretest to posttest no longer predicted change in partners’ perceptions of actors’ responsiveness from pretest to posttest when we controlled for change in partners’ support available from actors. Overall, results suggest that actors’ and partners’ agreement about actors’ responsiveness cannot be accounted for by partners’ perceptions of disclosure, anxiety, or depression. However, changes in actors’ responsiveness to partners leads to changes in partners’ perceptions of actors’ responsiveness because actors’ responsiveness is supportive.
Does disclosure, available support, anxiety or depression explain associations between changes in partners’ perceptions of actors’ responsiveness and change in partners’ responsiveness or compassionate goals?
We tested the link from partners’ perceptions of actors’ responsiveness to partners’ responsiveness and compassionate goals, controlling for partners’ perceptions of actors’ disclosure, support available from roommates, anxiety, and depression (in 24 separate analyses). We did not retest nonsignificant links between partners’ perceptions of actors’ responsiveness and partners’ self-image goals. All results remained unchanged, suggesting that partners’ responsiveness reciprocity (i.e., the link between partners’ perceptions of responsiveness and responsiveness to actors) and compassionate goals could not be accounted for by their perceptions of actors’ disclosure, support available from roommates, anxiety, or depression.
Do these associations differ by partners’ levels of anxiety or depression?
We tested whether partners’ anxiety or depression moderated links between actors’ responsiveness and partners’ perceptions of actors’ responsiveness in all models in Figure 3 . We also tested whether partner’s anxiety or depression moderated associations between partners’ perceptions of actors’ responsiveness and partners’ responsiveness to actors in lagged and pretest and posttest models. Partners’ anxiety and depression did not moderate these associations in 9 of 10 tests (all pr s < |.11|, ns). However, in pretest and posttest analyses, depression moderated the link between change in partners’ perceptions of actors’ responsiveness and partners’ responsiveness, pr = −.20, p < .01, such that this association was stronger when partners also reported lower depression (lower depression: pr = .70, p < .001; higher depression: pr = .63, p < .001). Thus, when partners become more depressed, they are less likely to reciprocate increased perceptions of actors’ responsiveness.
We tested whether gender moderated associations unique to the interpersonal models (e.g., links between actors’ responsiveness to partners and partners’ perceptions of actors’ responsiveness in weekly, lagged-week, and change from pretest to posttest analyses; links between partners’ perceptions of actors’ responsiveness and partners’ goals in weekly analyses; and links between partners’ perceptions of actors’ responsiveness and partners’ responsiveness to actors in lagged-week, and change from pretest to posttest analyses), using the same strategy reported for testing whether gender moderated intrapersonal associations. Gender did not moderate any of the 7 paths tested (all pr s < |.13|, all ns).
Study 1 examined intra- and interpersonal models of responsiveness in first-semester college roommates. Results were generally consistent with our hypotheses: students’ compassionate and self-image goals lead to change in their responsiveness to roommates, with consequences for change in both people’s perceptions of responsiveness in the relationship and interpersonal goals. Thus, people’s goals can create their own and others’ responsiveness and goals. In general, these associations were not due to disclosure, available support, anxiety, or depression.
Students’ goals predict their own experiences of responsiveness - their compassionate and self-image goals predict change in their responsiveness to partners, which then predicts projection of their responsiveness onto partners. We also predicted a self-perpetuating cycle between goals and responsiveness in relationships: actors’ perceptions of roommates’ responsiveness in turn predict change in their own compassionate and self-image goals. We found support for this hypothesis in the lagged-week data, but these effects did not appear to have any cumulative effect from pretest to posttest, suggesting projection processes have relatively short-term consequences for people’s goals, but do not affect their goals over the longer-term.
Tests of the interpersonal associations were partially consistent with our hypothesis. We expected agreement between actors and partners on actors’ responsiveness to partners, which should have predicted change in partners’ responsiveness to actors and goals. We found strong evidence for this hypothesis within weeks and over the semester - when actors reported increased responsiveness to partners, partners perceived increased responsiveness from actors, which then led to partners’ increased responsiveness to actors and increased compassionate goals. However, we did not find these associations in the lagged-week analyses – changes in actors’ responsiveness to partners from Weeks 1 to 2 did not predict partners’ perceptions of actors’ responsiveness over that same period, perhaps due to measurement timing; when students were asked to think about their and their roommates’ behaviors over the past week, the two roommates may have simply recalled or drew their responses from different events. More precise measurements of daily goals and responsiveness might show greater agreement between actors’ and partners’ reports. In study 2, we examined these associations in daily measures across three weeks to investigate this possibility.
Study 1 also did not address the implications of being responsive to others for the relationship itself. We predicted that this process of building (or undermining) projected and actual responsiveness between roommates has implications for both people’s perceived relationship quality. We included a measure of relationship quality in Study 2 to address this issue.
Study 1 ruled out self-disclosure, anxiety, and depression as alternative explanations for these processes. Study 1 also ruled out available support as an alternative explanation for associations between change in partners’ perceptions of actors’ responsiveness and change in partner’s responsiveness and compassionate goals and in 2 of 3 analyses, change in available support also could not account for associations between change in actors’ responsiveness to partners to change in partners’ perceptions of actors’ responsiveness. However, self-esteem or esteem for roommates might also account for these associations. For example, if high self-esteem predicts compassionate goals, responsiveness to roommates, and perceived responsiveness of roommates, the associations observed in Study 1 could be spurious. Esteem for roommates might also produce spurious associations if associations with perceptions of responsiveness are simply due to positive evaluations of roommates. Additionally, esteem for roommates may also produce spurious findings if students who hold their roommates in high esteem have more compassionate goals, are more responsive, and perceive their roommates as more responsive.
Alternatively, self-esteem may moderate associations tested in Study 1. Previous research shows that self-esteem moderates associations between perceptions of partners’ regard and relationship behavior and satisfaction ( Murray, Bellavia, Rose, & Griffin, 2003 ; Murray, Griffin, Rose, and Bellavia, 2003 ). In the present context, associations between actors’ compassionate goals and responsiveness to partners, and between perceptions of roommates’ responsiveness and relationship quality might be stronger when actors’ self-esteem is high. We tested self-esteem and esteem for roommates as covariates and self-esteem as a moderator in Study 2.
Study 2 used data from the Roommate Goals Study (Crocker & Canevello, Study 2) to examine how students’ goals predict projected and reciprocal responsiveness between roommates that ultimately influences both peoples’ relationship quality. The general design was similar to Study 1, but over a different time frame; same-sex freshmen roommate dyads completed a series of pretest, posttest, and 21 daily questionnaires, each including measures of compassionate and self-image goals, responsiveness to roommates, perceived roommates’ responsiveness, relationship quality, and self-esteem and esteem for roommates.
Again, we expected both intrapersonal and interpersonal consequences of compassionate and self-image goals. The design of Study 2 allowed us to more closely examine interactions between roommates by assessing students daily instead of weekly. Also, we followed roommate pairs over a shorter time period to examine whether these processes occur in daily interactions. Finally, we included measures of self-esteem and esteem for roommates to rule out these variables as alternative explanations for our hypotheses.
Sixty-five first-semester freshmen roommate dyads at a large Midwestern university who did not know each other prior to college volunteered for a study of goals and roommate relationships during the fall semester. Students completed a pretest survey, 21 daily surveys, and a posttest survey. Sixty-two pairs (95%) completed all 23 surveys; 46 pairs (71%) were female, and 19 (29%) were male. Sixty-eight percent of participants reported their race as White or European-American, 4% as Black or African-American, 16% as Asian or Asian-American, 5% were Latino(a), and 6% selected other. The racial composition of the sample closely approximates the racial composition of the incoming freshman class. Participants ranged in age from 18 to 22 years ( M = 18.2 years).
Roommate pairs attended an initial lab session to learn about the study, give their informed consent, complete the pretest survey, and receive instructions for completing the remaining 22 online surveys. The daily surveys took about 10 min. to complete and roommates were required to complete daily surveys on the same day. Participants were instructed to complete the surveys in privacy and not to discuss their responses with each other. To retain as many participants as possible in the study, participants were given up to 28 days to complete the 21 daily surveys. 8 Once roommates had completed 21 daily surveys, they completed the posttest survey and were paid for their participation. For a detailed description of the procedure see Crocker and Canevello (2008) , Study 2.
Participants completed measures of their compassionate and self-image goals, perceptions of roommates’ responsiveness, responsiveness to the roommate, relationship quality, self-esteem, and esteem for roommates at pretest, posttest, and daily. The pretest measure also included questions about demographics (gender, race/ethnicity, age, parental income). Additional measures not germane to the goals of the present investigation were also included.
Compassionate and self-image goals for participants’ relationships with their roommates were measured at pretest, posttest, and daily, using the measure described in Study 1. 9 Based on exploratory factor analyses, we modified the scales in the following ways: for the compassionate goals scale we dropped “be supportive of my roommate” and “make a positive difference in my roommate’s life” and added "be aware of the impact my behavior might have on my roommate's feelings" and "avoid doing anything that would be harmful to my roommate." For the self-image goals scale we dropped “avoid revealing my shortcomings and vulnerabilities” and added "avoid coming across as unintelligent or incompetent" and "demonstrate my intelligence.” Both scales had high internal consistency at pretest (self-image α = .80; compassionate α = .84), posttest (self-image α = .87; compassionate α = .93), and across days (self-image goals: .75 < α < .87, M α = .83; compassionate goals: .88 < α < .96, M α = .94).
Responsiveness to the roommate and perceptions of roommates’ responsiveness were measured using a 12-item version of the scale used in Study 1. Additional items included “I do things to show my roommate that I care about him/her;” “I try to see things from his/her point of view;” “I am uncaring toward my roommate” (reverse); “I try to show respect for my roommate’s capabilities and talents;” “I don’t really take my roommate’s concerns seriously” (reverse); and “I am sincere when I interact with my roommate.” We measured daily responsiveness with 8 items from the pretest and posttest measure asking how participants acted toward their roommate that day. Responsiveness was reliable at pretest (α =.93), posttest (α = .95) and from day to day (.89 < α < .95, M α = .93).
A parallel set of 12 items assessed the extent to which participants believed their roommates responded to them at pretest and posttest. Sample items included "My roommate seems sensitive to my feelings" and "My roommate is sincere when he/she interacts with me." We removed “My roommate seems uncaring” and “My roommate makes me feel comfortable about myself and my feelings” because in factor analyses, they overlapped with relationship quality. We measured daily roommate responsiveness with 7 items from the pretest and posttest measure asking how roommates acted toward participants that day. Roommate responsiveness was reliable at pretest (α = .96), posttest (α =.94), and from day to day (.84 < α < .92, M α = .89).
Relationship quality included measures of satisfaction, commitment and closeness. Because all scales were highly correlated at each time point (all r s > .67), and we had the same predictions for these indicators of relationship quality, we standardized and averaged these scales to create pretest, posttest, and daily composite relationship quality scores.
In the pretest and posttest, we measured relationship satisfaction with 6 questions: “In general, how satisfied are you with your relationship with your roommate?” “How well does your roommate meet your needs?” “How good is your relationship with your roommate compared to most?” “How many problems are there in your relationship with your roommate?” (reverse scored) “How often do you wish you hadn’t moved in with your roommate?” (reverse scored) and “To what extent has your relationship with your roommate met your original expectations?” Students responded on a scale from 1 ( poorly/not at all/never ) to 5 ( extremely well/completely/often ). We measured commitment using an abbreviated version of the measure developed by Rusbult and colleagues ( Rusbult, Verette, Whitney, Slovik, & Lipkus, 1991 ): “To what extent are you committed to your relationship with your roommate?” “For what length of time would you like your relationship with your roommate to last?” “To what extent are you attached to your roommate?” and “How likely is it that you will end your relationship with your roommate in the near future?” (reverse scored). Students rated items on a 9-point scale (0 = Not at all/< 1 month , 8 = Extremely/ 5+ years ). Two items assessed closeness (e.g., Gore et al, 2006 ): “Relative to all other relationships, how would you characterize your relationship with your roommate?” and “Relative to what you know about other people’s roommate relationships, how would you characterize your relationship with your roommate?” and were rated on a 1 ( not as close as others ) to 5 ( much closer than others ) scale. The relationship quality composite measures had high internal consistency at pretest (α =.90) and posttest (α =.92)
We measured daily relationship satisfaction with 3 questions from the pretest/posttest measure: “How well does your roommate meet your needs today?” “How good is your relationship with your roommate today, compared to most?” and “Today, to what extent do you wish you hadn’t moved in with your roommate?” (reversed). Students responded on a scale from 1 ( poorly/not at all/never ) to 5 ( extremely well/completely/often ). We measured commitment using the four commitment items from the pretest measure, with each item referring to how they felt that day. A single item assessed closeness: “How close do you feel to your roommate today?” and was rated on a 1 ( not at all ) to 5 ( extremely ) scale. The daily relationship quality composite measure had high internal consistency each day of the study (.82 < α < .88, M α = .86).
Self-esteem . The Rosenberg Self-Esteem Inventory ( Rosenberg, 1965 ) was used to assess global self-esteem. In the pretest and posttest surveys, self-esteem was measured using the original 10-item measure. We used an abbreviated version of this measure in the daily surveys, with the stem “Today, to what extent did you feel:” followed by 4 questions: “that you are a person of worth,” “that you are a failure,” (reverse scored) “satisfied with yourself,” and “that you are no good at all,” (reverse scored). Self-esteem had adequate internal consistency at pretest (α = .89), posttest (α = .90), and each day of the study (.83 < α < .93, M α = .90).
Esteem for roommates was measured at pretest, posttest, and in each of the daily surveys. In the pretest and posttest, esteem for roommates was measured with 10 questions, adapted from the Rosenberg self-esteem measure ( Rosenberg, 1965 ), with items assessing evaluation of roommates (not perceptions of roommates’ self-esteem). Sample items included: “I certainly feel my roommate is useless at times,” (reverse scored) “I feel that my roommate has a number of good qualities,” and “I feel that my roommate is a person of worth, at least on an equal basis with others.” Students responded on a scale from 1 ( strongly disagree ) to 5 ( strongly agree ). In the daily surveys, we measured esteem for roommates with the stem “Right now, to what extent do you feel that:” followed by 4 questions: “your roommate is a person of worth,” “your roommate is a failure,” (reverse scored) “you are satisfied with your roommate,” and “your roommate is no good at all,” (reverse scored). Esteem for roommates had adequate internal consistency at pretest (α =.88), posttest (α =.89), and across days (.78 < α < .91, M α = .86).
Table 3 shows the intrapersonal (i.e., within-person) intraclass correlations, which adjust for the degree of nonindependence between dyad members ( Griffin & Gonzalez, 1995 ), means, and standard deviations for all of the main variables in Study 2. As in Study 1, compassionate goals correlated strongly with responsiveness to roommates and perceptions of roommates’ responsiveness. Also, perceptions of roommates’ responsiveness correlated strongly with relationship quality. Following Study 1, we conducted a series of factor analyses to determine whether these measures were empirically distinct.
Study 2 means, standard deviations, and intrapersonal intraclass correlations for all pretest, posttest, and mean daily variables.
N = 130 at pretest, N = 124 at posttest. Chronic scores were calculated by averaging across the 21 daily reports. Responsiveness to the roommate and roommate responsiveness was measured on a scale ranging from 1 (not at all) to 5 (very much). Relationship quality was standardized. Chronic self-image and compassionate goals were measured on a scale ranging from 1 (never) to 5 (always).
Perceptions of roommates’ responsiveness and relationship quality
Perceptions of roommates’ responsiveness and relationship quality are empirically distinct. We conducted EFAs on pretest relationship quality and perceptions of roommates’ responsiveness items. In an initial EFA on pretest items, all but two perceptions of roommates’ responsiveness items (“my roommate makes me feel comfortable about myself and my feelings” and “my roommate seems uncaring”) loaded on the two factors as predicted. After removing those items, a 2-factor solution accounted for 57% of the variance: all perceptions of roommate’s responsiveness items loaded on the first factor, with loadings ranging between |.49| and |.87|; all relationship quality items loaded on the second factor, with loadings ranging between |.49| and |.86|. Importantly, the highest loading on a secondary factor was |.22|. We conducted CFAs on items for each day of the study and at posttest, excluding the two items removed from the EFA above (for a total of 22 separate sets of analyses), testing 2-factor, 120.46 < χ 2 (df = 89 and 208, 124 < N < 130) < 553.274, Mχ 2 (df = 89 and 208, 124 < N < 130) = 232.09, and single-factor solutions, 188.97 < χ 2 (df = 90 and 209, 124 < N < 130) < 860.03, Mχ 2 (df = 90 and 209, 124 < N < 130) =371.45. For all sets of analyses, 2-factor solutions fit significantly better, 63.54 < Δχ 2 (1, 124 < N < 130) < 306.752, MΔχ 2 (1, 124 < N < 130) = 139.36. Because of their overlap with relationship quality, we did not include the two cross-loading items in our final scoring of perceptions of roommates’ responsiveness, nor did we include them in future factor analyses of perceptions of roommates’ responsiveness items.
As in Study 1, the items used to assess compassionate goals and responsiveness to roommates were empirically distinct. We conducted EFAs on pretest compassionate goals and responsiveness to roommate items and CFAs on these items at posttest and each day, comparing the fit of a 2-factor model with that of a single-factor model. In an initial EFA on pretest items, a 2-factor solution accounted for 50% of the variance: all responsiveness items loaded on the first factor, with loadings ranging between |.41| and |.88|; all compassionate goal items loaded on the second factor, with loadings ranging between .50 and .74. No secondary loading exceeded |.17|. We conducted CFAs on items at posttest and across the 21 days, testing 2-factor, 133.52 < χ 2 (df = 89 and 151, 124 < N < 130) < 350.19, M χ2 (df = 89 and 151, 124 < N < 130) = 198.89, and single-factor solutions, 252.81 < χ 2 (df = 90 and 152, 124 < N < 130) < 500.52, M χ 2 (df = 90 and 152, 124 < N < 130) = 394.18. For all sets of analyses, the 2-factor solution fit significantly better, 104.41 < Δχ 2 (1, 124 < N < 130) < 308.15, M Δχ 2 (1, 124 < N < 130) = 195.29.
The items used to assess compassionate goals and perceptions of roommates’ responsiveness are also empirically distinct. We conducted EFAs on pretest compassionate goals and perceptions of roommates’ responsiveness items and CFAs on these items at posttest and each day, comparing the fit of a 2-factor model with that of a single-factor model. An EFA on pretest items showed that a 2-factor solution accounted for 53% of the variance: all perceptions of roommates’ responsiveness items loaded on the first factor, with loadings ranging between |.58| and |.88|; all compassionate goal items loaded on the second factor, with loadings ranging between .44 and .78. No secondary loading exceeded |.27|. We conducted CFAs on items at posttest and across the 21 days, testing 2-factor, 111.09 < χ 2 (df = 76 and 118, 124 < N < 130) < 234.48, M χ2 (df = 76 and 118, 124 < N < 130) = 150.34, and single-factor solutions, 241.75 < χ 2 (df = 77 and 119, 124 < N < 130) < 531.71, M χ 2 (df = 77 and 119, 124 < N < 130) = 395.46. For all sets of analyses, the 2-factor solution fit significantly better, 102.44 < Δχ 2 (1, 124 < N < 130) < 376.90, M Δχ 2 (1, 124 < N < 130) = 245.12.
Overview of Analyses
Data analyses proceeded in two phases. Phase 1 focused on intrapersonal associations and included only actor variables: we tested a model in which students’ goals predict their responsiveness to roommates (Path A), which predicts their perceptions of roommates’ responsiveness (Path B), which then predicts students’ own goals (Path D) and relationship quality (Path E). Phase 2 focused on interpersonal associations, and included actor and partner variables: we tested a model in which actors’ goals predict their responsiveness to partners (Path A), which predicts partners’ perceptions of actors’ responsiveness (Path F), which predicts partners’ goals (Path J) and relationship quality (Path K). As in Study 1, we tested associations in each phase within a given day, from day to day using lagged analyses, and across three weeks (i.e., from pretest to posttest). As in Study 1, all intra- and interpersonal analyses assess change.
Because the structure of the data was similar to Study 1 (persons within dyads and dyads crossed with days; Kashy et al., 2008 ), we arranged the data and conducted analyses using the same strategy as in Study 1, controlling for the appropriate levels of nonindependence using the MIXED command in SPSS, specifying compound symmetry so that intercept variances between dyad members were equal, and testing change using residuals. Again, path models were tested sequentially; for each path, we regressed the criterion on the predictor, controlling for all variables preceding that path in the model. Partial correlations are reported for all analyses ( Rosenthal & Rosnow, 1991 ).
Table 3 shows the means, standard deviations, and intrapersonal (i.e., within-persons) intraclass correlations for all pretest, posttest, and mean daily variables. We created measures of chronic compassionate and self-image goals by averaging each measure across the 21 days. Compassionate goals, responsiveness, and perceptions of roommates’ responsiveness were strongly correlated at pretest and posttest, and across days. Self-image goals were less strongly correlated with responsiveness and perceptions of roommates’ responsiveness, particularly at posttest and in the chronic measures. As in Study 1, compassionate and self-image goals were significantly correlated. To test the independent effects of goals on outcomes, we regressed all outcome variables on compassionate and self-image goals simultaneously. Table 4 shows the interpersonal (i.e. actor-partner) intraclass correlations for all variables. Roommates’ reported compassionate goals, responsiveness and perceptions of roommates’ responsiveness were moderately correlated across time-points; actors’ self-image goals predicted fewer partner variables.
Study 2 interpersonal (i.e. actor-partner) intraclass correlations for all pretest, posttest, and mean daily variables.
Intrapersonal Processes: Students’ Goals Predicting Their Own Responsiveness, Perceptions of Roommates’ Responsiveness and Subsequent Goals and Relationship Quality
Phase 1 analyses test an intrapersonal model in which students’ compassionate and self-image goals predict their responsiveness to roommates (Path A; Figure 1 ), which predicts their perceptions of roommates’ responsiveness (Path B), which in turn predict their subsequent compassionate and self-image goals (Path D) and relationship quality (Path E). All Phase 1 analyses use only actor variables as predictors and outcomes. As in Study 1, because actors and partners are interchangeable, these analyses simultaneously test the process by which partners’ goals lead to partners’ own goals and relationship quality (i.e., Paths G, H, J and K).
Same Day Associations
First, we examined our hypothesized model within days, testing whether daily interpersonal goals predicted daily responsiveness to roommates, which predicted daily perceptions of roommates’ responsiveness, which then predicted daily relationship quality. Following Study 1, coefficients for daily analyses were derived from random-coefficients models using restricted maximum-likelihood estimation, where models included fixed and random effects for the intercept and each predictor. Also, following the rationale for centering described in Study 1 within-week analyses, we person-centered all predictors.
Within-day analyses supported our hypothesized intrapersonal model (see top of Figure 4 ). Daily compassionate goals predicted higher responsiveness to roommates; daily self-image goals did not predict responsiveness to roommates. Responsiveness to roommates predicted higher perceptions of roommates’ responsiveness on that day, which then positively predicted relationship quality on that day.

Study 2: Intrapersonal (within-person) path analyses of daily, lagged-day, and pretest and posttest data. NOTE: All estimates are partial correlations; estimates in brackets indicate test of the individual path, not controlling for previous paths in the model. *** p < .001, ** p < .01, * p < .05.
Lagged-Day Analyses
We next examined our intrapersonal model using lagged-day analyses to test the plausibility of causal paths in our model. Using the strategy described in Study 1 lagged analyses, i.e., random-coefficients models using restricted maximum-likelihood estimation with all predictors grand mean centered, we constructed MIXED models that regressed one day’s criterion on the previous day’s predictor and criterion.
Using the logic from Study 1’s intrapersonal lagged-week path model, we hypothesized a lagged-day path model in which goals at Day 1 predict change in responsiveness to roommates from Days 1 to 2, which predict simultaneous change in perceptions of roommates’ responsiveness from Days 1 to 2, which in turn predict change in compassionate and self-image goals and relationship quality from Days 1 to 3.
Results supported the intrapersonal model (see middle of Figure 4 ). Day 1 compassionate goals predicted increased responsiveness to roommates and Day 1 self-image goals predicted decreased responsiveness to roommates from Days 1 to 2. Change in responsiveness to roommates from Days 1 to 2 positively predicted change in perceptions of roommates’ responsiveness from Days 1 to 2, which in turn, positively predicted change in relationship quality and compassionate goals and but did not predict change in self-image goals from Days 1 to 3.
These analyses support the plausibility of a causal chain from goals to responsiveness to roommates and perceptions of roommates’ responsiveness to relationship quality. Compassionate goals predict increased responsiveness and perceptions of roommates’ responsiveness across days, which results in increased relationship quality and compassionate goals and decreased self-image goals, while self-image goals predict decreased responsiveness and perceptions of roommates’ responsiveness across days, which results in decreased relationship quality and compassionate goals and increased self-image goals.
To test whether and how students’ chronic compassionate and self-image goals contribute to changes in their responsiveness, perceptions of roommates’ responsiveness, goals, and relationship quality over time, we tested a path model in which chronic goals averaged across 21 days predicted change in responsiveness to roommates from pretest to posttest, which then predicted change in perceptions of the roommates’ responsiveness, which in turn predicted changes in goals and relationship quality from pretest to posttest. Again, following Study 1, coefficients for testing change from pretest to posttest were derived from fixed-effects models using restricted maximum-likelihood estimation and we grand mean centered all predictors.
Results testing change across 3 weeks supported our intrapersonal model (see bottom of Figure 4 ) and suggest that effects of goals accumulate over time, resulting in longer-term changes in compassionate and self-image goals and relationship quality. Chronic compassionate goals predict increased and chronic self-image goals predict decreased responsiveness to roommates from pretest to posttest, which predicted increased perceptions of roommates’ responsiveness from pretest to posttest, which predicted increased relationship quality and compassionate goals, and decreased self-image goals.
As in Study 1, we tested several alternative explanations for and moderators of these associations, using the same statistical strategies described in Study 1. We tested whether perceptions of roommates’ responsiveness, self-esteem, or esteem for roommates explained associations in Figure 4 . We also test whether these associations were moderated by self-esteem or gender. Finally, we also tested an alternative model suggesting that perceptions of roommates’ responsiveness result from relationship quality (as compared to our hypothesis that relationship quality results from perceptions of roommates’ responsiveness). As in Study 1, we used the procedure recommended by Aiken and West (1991) to examine simple slopes. Specific analyses for each covariate are described below. As in Study 1, our main concern was whether these covariates offered an alternative explanation for our findings. Thus, we report the associations between our predictor and outcome variables, controlling for covariates, but do not report whether covariates were related to each outcome, not controlling for our predictors. Again, tables of these analyses including covariates can be obtained from the first author.
We tested whether associations between students’ interpersonal goals and changes in their responsiveness to roommates could be explained by reciprocity of perceptions of roommates’ responsiveness by retesting the links between compassionate and self-image goals and responsiveness to roommates (all models in Figure 4 ), controlling for daily perceptions of roommates’ responsiveness in daily analyses, Day 1 perceptions of roommates’ responsiveness in lagged analyses, and chronic perceptions of roommates’ responsiveness in the pretest and posttest analyses. As in Study 1, perceptions of roommates responsiveness predicted higher or increased responsiveness to roommates across all three sets of analyses, .19 < pr s < .60, all p s < .001. Associations between compassionate goals and higher and increased responsiveness remained significant in daily and lagged analyses, daily: pr = .26, p < .001; lagged: pr = .33, p < .001. In pretest and posttest analyses, chronic compassionate goals no longer predicted change in responsiveness to roommates when we controlled for chronic perceptions of roommates’ responsiveness, pr = .10, ns.
The associations between students’ self-image goals and decreased responsiveness to roommates were not explained by perceptions of roommates’ responsiveness. In lagged analyses and analyses of change from pretest to posttest, self-image goals predicted decreased responsiveness to roommates, when we controlled for perceptions of roommates’ responsiveness, both pr s = −.21, both p s < .05. The association between daily self-image goals and responsiveness to roommates was not significant in the original model. Overall, these results suggest that students’ interpersonal goals predict change in their responsiveness to roommates, but not because they also perceive roommates as more or less responsive.
Does self-esteem or esteem for roommates explain these associations?
We tested whether the hypothesized processes were due simply to students’ esteem for themselves or their roommates. We reanalyzed all models in the daily, lagged-day, and change from pretest to posttest data, controlling for these covariates separately, using the analytic strategy from Study 1. Results remained unchanged in 31of 32 analyses. In the pretest to posttest model (bottom of Figure 4 ) the association between change in perceptions of roommates’ responsiveness and change in self-image goals became marginal when we controlled for change in esteem for roommates, pr = −.21, p < .08, however change in esteem for roommates did not significantly predict change in self-image goals, pr = −.10, ns. Thus, the associations tested here cannot be accounted for by self-esteem or esteem for roommates.
Does self-esteem moderate these associations?
We also tested whether each individual path in the models in Figure 4 was moderated by self-esteem (not controlling for other variables in the model). Overall, the intrapersonal process did not depend on self-esteem. Only 2 of 16 interactions were significant; self-esteem moderated the association between self-image goals and decreased responsiveness so that it was stronger with lower self-esteem in the lagged day analyses, pr = .14, p < .05 (low self-esteem, pr = −.21, p < .001, high self-esteem, pr = −.02, ns), but weaker with lower self-esteem in pretest to posttest analyses, pr = −.20, p < .05, (low self-esteem, pr = −.19, p < .05, high self-esteem, pr = −.36, p < .001). For all other moderation analyses pr s < |.12|, ns.
As in Study 1, responsiveness dynamics may differ by gender. We tested whether gender moderated each individual path (i.e., not controlling for other variables in the models) in each intrapersonal model, using the strategy described in Study 1. Gender did not moderate any of the 16 associations in Figure 4 , all pr s < |.17|, ns.
Does relationship quality lead to perceptions of roommates’ responsiveness?
It is possible that students’ relationship quality leads to changes in their perceptions of roommates’ responsiveness. To address this, we tested alternative orders of perceptions of roommate’s responsiveness and relationship quality in lagged-day and change from pretest to posttest analyses. (We did not test this alternative order in the daily data because the cross-sectional nature of those data do not speak to the plausibility of causal associations and either order would provide virtually the same result.) Change in relationship quality from Days 1 to 2 did not predict change in perceptions of roommates’ responsiveness from Days 1 to 3, pr = .06, ns. Chronic perceptions of roommates’ responsiveness averaged across 21 days predicted increased relationship quality, pr = .23, p < .05; chronic relationship quality averaged across 21 days did not predict change in perceptions of roommates’ responsiveness, pr = .10, ns. (We did not test whether change in relationship quality from pretest to posttest would predict change in perceptions of roommates’ responsiveness from pretest to posttest because this test of simultaneous change would provide the same result for either ordering.) Thus, these analyses do not support the idea that perceptions of roommates’ responsiveness are simply a function of relationship quality.
These results replicate and extend the intrapersonal process found in Study 1: goals predict change in responsiveness to roommates, which predicts increased projection of responsiveness, which predicts changes in goals and relationship quality. 10 Self-esteem and esteem for roommates did not account for these associations and they were not moderated by self-esteem. 11
Thus, goals have immediate implications for responsiveness, projection of responsiveness, goals, and relationship quality. These effects held in lagged day analyses and predicted change in goals and relationship quality across three weeks. However, these analyses do not address whether students’ goals predict their roommates’ experiences. The second phase of analyses addresses this issue.
Interpersonal Processes: Actors’ Goals and Responsiveness Predicting Partners’ Responsiveness and Relationship Quality
Phase 2 analyses test whether partners perceive actors’ responsiveness and whether these perceptions predict partners’ own goals and relationship quality. We tested an interpersonal model in which actors’ goals predict actors’ responsiveness to partners (Path A), which predicts partners’ perceptions of actors’ responsiveness (Path F), which predicts partners’ goals and relationship quality (Paths J and K, respectively). These analyses simultaneously examine the process by which partners’ goals predict actors’ goals and relationship quality (i.e., Paths G, L, D, and E). We examined evidence supporting this model within days, from day to day, and across three weeks, using the same analytic strategy described in Study 1 interpersonal analyses.
Within-day analyses support our hypotheses (see top of Figure 5 ). On days actors had higher compassionate goals, they reported being more responsive to partners; actors’ daily self-image goals were unrelated to their daily responsiveness to partners. Actors’ daily responsiveness to partners predicted partners’ higher perceptions of actors’ responsiveness, which then predicted partners’ higher relationship quality and compassionate goals, but did not predict self-image goals.

Study 2: Interpersonal (between-person) path analyses of daily, lagged-day, and pretest and posttest data. NOTE: All estimates are partial correlations; estimates in brackets
Following the logic from Study 1’s interpersonal lagged-week path model, we hypothesized an interpersonal lagged-day path model in which actors’ goals at Day 1 predict change in their responsiveness to roommates from Days 1 to 2, which predict simultaneous change in partners’ perceptions of actors’ responsiveness from Days 1 to 2, which in turn predict change in partners’ compassionate and self-image goals and relationship quality from Days 1 to 3.
Lagged-day tests of the path model support the plausibility of causal effects in our hypothesized interpersonal model (see middle of Figure 5 ). Actors’ compassionate goals on Day 1 predicted increased responsiveness to partners from Days 1 to 2; actors’ self-image goals on Day 1 predicted decreased responsiveness to partners from Days 1 to 2. Change in actors’ responsiveness to partners from Days 1 to 2 positively predicted simultaneous change in partners’ perceptions of actors’ responsiveness from Days 1 to 2, which positively predicted change in partners’ relationship quality and compassionate goals from Days 1 to 3. Change in partners’ perceptions of actors’ responsiveness from Days 1 to 2 did not predict change in partners’ self-image goals from Days 1 to 3.
Changes Across 3 Weeks
The interpersonal effects of actors’ goals accumulated over time, indirectly predicting change in partners’ relationship quality and goals over three weeks (see bottom of Figure 5 ). Actors’ chronic compassionate goals predicted increased responsiveness and actors’ chronic self-image goals predicted decreased responsiveness to partners from pretest to posttest. Change in actors’ responsiveness to partners positively predicted change in partners’ perceptions of actors’ responsiveness, which positively predicted change in partners’ relationship quality and compassionate goals from pretest to posttest, but did not predict change in partners’ self-image goals.
As in Study 1, we tested whether partners’ goals moderate their perceptions of actors’ responsiveness, whether self-esteem or esteem for roommates explain associations unique to the interpersonal model, and whether those associations are moderated by self-esteem or gender, using the same analytic strategies described in Study 1.
As in Study 1, we tested the possibility that the links between actors’ responsiveness and partners’ perceptions of actors’ responsiveness were dependent on partners’ goals. For all models in Figure 5 , we tested whether partners’ goals moderated the individual paths (i.e., not controlling for other variables in the models) between actors’ responsiveness to partners and partners’ perceptions of actors’ responsiveness, using the strategy described in Study 1. Across daily, lagged, and pretest and posttest analyses, partners’ goals did not moderate this association, compassionate goals: −.09 < pr < .02, all ns; self-image goals: −.04 < pr < .02, all ns. Again, actors’ and partners’ agreement about actors’ responsiveness does not depend on partners’ compassionate or self-image goals.
To rule out the possibility that esteem for roommates or self-esteem explained associations unique to the interpersonal model, we retested the links between actors’ responsiveness to partners and partners’ perceptions of actors’ responsiveness, controlling for partners’ self-esteem or esteem for roommates in separate analyses. We tested these links in all models in Figure 5 using the analytic strategy described previously. In the weekly data, we also tested whether partners’ self-esteem or esteem for roommates accounted for associations between partners’ daily perceptions of actors’ responsiveness and partners’ compassionate and self-image goals. Results remained unchanged in all 5 analyses.
We also tested whether self-esteem moderated associations between actors’ responsiveness and partners’ perceptions of actors’ responsiveness in all models and associations between partners’ perceptions of actors’ responsiveness and partners’ compassionate and self-image goals in the weekly model. None of the 5 product terms tested were significant (all pr s < |.15|, ns).
We tested whether gender moderated associations unique to the interpersonal models (i.e., paths from actors’ responsiveness to partners to partners’ perceptions of actors’ responsiveness in daily, lagged-day, and change from pretest to posttest analyses; and paths from perceptions of actors’ responsiveness to partners’ compassionate and self-image goals in daily analyses); gender did not moderate any of the 5 paths tested (all pr s < |.13|, ns).
Study 2 results strongly support our overall hypothesis that people’s goals can create their own and others’ responsiveness and relationship quality. First, we replicated and extended the intrapersonal associations found in Study 1. Again, students’ goals predicted their responsiveness to roommates, led them to project their responsiveness onto roommates, which then contributed to their goals and relationship quality. We found evidence for this process within days, from day to day, and across the study. Second, interpersonal results supported our hypothesis that students’ goals predict their responsiveness to their roommates, which lead roommates’ to feel responded to, which in turn lead to roommates’ relationship quality and goals. In contrast to Study 1, interpersonal effects in Study 2 were quite clear, suggesting that these processes occur in a more immediate time frame (daily rather than weekly), possibly because in daily reports actors and partners are more likely to base their reports on the same events.
GENERAL DISCUSSION
People who perceive others as responsive become responsive themselves and perceive their partners as more responsive, leading to high quality relationships for both the partner and the self. But what creates perceived partner responsiveness in relationships? The studies reported here explore the processes that promote or undermine responsiveness over time. We incorporate and build on two models in the responsiveness literature: an intrapersonal projection model in which responsiveness predicts perceptions of others’ responsiveness and one’s own relationship outcomes (e.g., Lemay & Clark, 2008 ), and an interactive dyadic model in which actors’ responsiveness to partners predicts partners’ perceptions of actors’ responsiveness (e.g., Reis & Shaver, 1988 ).
Indeed, our data are consistent with the hypothesis that students project their responsiveness onto their roommates. Across data sets, results consistently show that responsiveness to roommates strongly predicts perceptions of roommates’ responsiveness (i.e., projection). 12 However, they also support the hypothesis that responsiveness is a dyadic process, as suggested by Reis and Shaver (1988) – in 5 of 6 models tested, actors’ responsiveness to partners predicted partners’ increased perceptions of actors’ responsiveness. Approximately one-third of the total variance in responsiveness to roommates occurred at the dyad level (30% in Study 1 and 39% in Study 2). Just over one-third of the total variance in perceptions of roommates’ responsiveness occurred at the dyad level (34% in Study 1 and 38% in Study 2). If responsiveness was strictly an individual projection phenomenon, then we should not find variance in responsiveness and perceptions of roommates’ responsiveness at the dyad level. Because a portion of variance in responsiveness and perceptions of roommates’ responsiveness occurs at the dyad level, and these roommates did not have a long relationship history or self-select into their roommate relationships, the portion of variance that occurs at the dyad level likely indicates dyadic processes. Given inconsistent findings in the literature regarding whether responsiveness is a real behavioral phenomenon, our data are important because they consistently support the hypothesis that responsiveness is a dyadic process, in addition to a process of projection.
Results from the current studies also suggest that interpersonal goals provide one avenue for creating responsiveness in relationships, initiating a self-perpetuating relation between goals and responsiveness projection and reciprocation, and demonstrate that these processes are dynamic and extend over time. We consider each of these contributions in turn.
Compassionate and Self-Image Goals Predict Responsiveness in Relationships
Although Reis and Shaver (1988) speculate that actors’ goals and motives play a role in responsiveness processes, no research that we know of has examined these associations. Our data indicate that interpersonal goals can create or undermine responsiveness in close relationships. Across two studies, when people had compassionate goals, they became more responsive to relationship partners. When people had self-image goals, they became less responsive.
Our data show that interpersonal goals, particularly compassionate goals, predict responsiveness. Reis and Shaver’s theorizing focused mainly on disclosure as the mechanism by which responsiveness is created -- actors reveal personally relevant thoughts and feelings to partners and when partners are responsive, actors perceive that responsiveness. Although empirical research supports the Reis and Shaver hypothesis that self-disclosures lead to perceptions of partners’ responsiveness ( Laurenceau et al., 1998 ; Laurenceau, Barrett, & Rovine, 2005 ; Manne et al., 2004 ), disclosure cannot explain associations between compassionate goals, responsiveness to roommates, and perceptions of roommates’ responsiveness in these data. In Study 1, disclosure did not account for 10 of the 11 associations leading to responsiveness or perceptions of roommate’s responsiveness. Thus, these data suggest that responding to partners is an alternative route to creating responsive partners, and that actors’ goals to support partners out of concern for their well-being (versus trying to control what partners think of them) can begin this process. Through their compassionate and self-image goals, people create their own relationship experiences; when they are responsive to others, people project their responsiveness onto others, perceiving them as more responsive, with consequences for people’s own relationship quality. Through their goals, people also create others’ experiences; when, because of their interpersonal goals, people are responsive to partners, partners perceive their responsiveness, which has consequences for partners’ relationship quality.
Perceptions of roommates’ responsiveness did not explain why compassionate goals predict responsiveness to roommates. In only 1 of 6 analyses across both samples did the association between compassionate goals and responsiveness drop to nonsignificance when we controlled for perceptions of roommates’ responsiveness. Thus, people with compassionate goals are not responsive simply because they reciprocate perceived responsiveness in their partners. Perceptions of roommates’ responsiveness may, however, explain why self-image goals predict lower responsiveness. Perceptions of roommates’ responsiveness accounted for the link between weekly self-image goals and responsiveness and between chronic self-image goals and change in responsiveness from pretest to posttest in Study 1. Thus, people with self-image goals are less responsive, apparently, because they perceive others as less responsive.
The association between compassionate goals and responsiveness to roommates was remarkably robust. We examined whether several potential confounds could explain the effects of compassionate goals on responsiveness to roommates. Neither support availability nor negative affect (i.e., anxiety and depression; Study 1) or self-esteem (Study 2) consistently accounted for associations between actors’ compassionate goals, responsiveness, and perceptions of roommates’ responsiveness, nor did they account for associations between actors’ responsiveness, partners’ perceptions of actors’ responsiveness, partners’ responsiveness, and partners’ goals. 13 We also examined whether negative affect in Study 1, or self-esteem in Study 2 moderated the effects of compassionate goals; they did not. The processes we describe – compassionate goals lead to increased responsiveness, which leads to both people’s increased perceptions of the others responsiveness, which then leads to changes in both people’s goals and partners’ increased reciprocated responsiveness -- cannot be attributed to, nor do they depend on, affect or feeling that support is available. Compassionate goals appear to have unique implications for, responsiveness, perceptions of others’ responsiveness, and change in people’s own and partners’ interpersonal goals and relationship quality.
In contrast, the effects of self-image goals on responsiveness to roommates were explained by perceived available support, anxiety, and depression in several analyses, although the effects were inconsistent across weekly, lagged, and pretest to posttest analyses (Study 1). These results suggest that self-image and compassionate goals relate to responsiveness to others through different processes. Self-image goals predict responsiveness through affect and feeling that support is available; compassionate goals predict responsiveness through some other process, unrelated to affect and available support.
Self-Perpetuating Associations between Goals and Responsiveness in Relationships
These studies provide strong evidence for a powerful self-perpetuating virtuous cycle from compassionate goals to responsiveness to relationship quality and subsequent goals. Across all analyses, compassionate goals predicted increased responsiveness and perceptions of roommates’ responsiveness predicted increased compassionate goals in 10 of 12 analyses. Only two findings did not support an effect of perceptions of responsiveness on compassionate goals: first, in Study 1, change in perceptions of partners’ responsiveness from pretest to posttest did not predict change in compassionate goals from pretest to posttest; second, in Study 2, change in perceptions of partners’ responsiveness from Days 1 to 2 only marginally predicted increased compassionate goals from Days 1 to 3. Both of these exceptions occurred in intrapersonal models, suggesting that projection of responsiveness alone may not always be sufficient to create this mutually reinforcing process; it may depend on partners’ responses or behaviors. Overall, these data support our hypothesis that compassionate goals perpetuate future compassionate goals in the self and others.
Of course, upward spirals of compassionate goals and responsiveness cannot continue indefinitely. Future research should address the conditions or situations that cause responsiveness dynamics to asymptote or reverse. We speculate that responsiveness uses attentional resources. Even when people want to support others, they may not always have the attentional capacity to be sensitive to others’ emotional states. Thus, attentional demands may interrupt the upward spiral of compassionate goals and responsiveness. Furthermore, demands on partners’ attention and partners’ lack of motivation to be supportive may interrupt the interpersonal aspects of this upward spiral.
The present studies provide weaker evidence for a vicious cycle from self-image goals to responsiveness. Self-image goals predicted decreased responsiveness to roommates, but perceptions of responsiveness did not predict subsequent self-image goals. However, self-image goals may indirectly have consequences for future compassionate goals; self-image goals contribute to decreased responsiveness, which ultimately leads to decreased compassionate goals for both partners. Thus, the present studies more strongly indicate the benefits of one person’s compassionate goals for both people’s relationship quality than the detriments of self-image goals.
Responsiveness Processes are Dynamic and Extend over Time
The present studies demonstrate the dynamic nature of associations among goals, responsiveness reciprocation, and relationship quality over time. Perceptions of partners’ responsiveness vary from interaction to interaction (e.g., Laurenceau et al., 1998 ), but little research has investigated whether and how these discrete interactions influence long-term relationship experiences. Both the intrapersonal and the interpersonal responsiveness processes in the present studies occurred within a day, and had lingering consequences over several days, suggesting the power of interpersonal goals to create lasting effects on responsiveness. Goals and responsiveness also have cumulative consequences over a semester. Roommates’ chronic goals over weeks and months predicted long-term changes in both people’s responsiveness and perceptions of the others’ responsiveness, with consequences for both people’s goals and relationship quality. Because they operate over the long-term, compassionate goals may create a foundation for enduring, sustainable high-quality relationships.
Examining these processes over different time periods also allowed us to rule out alternative explanations. Within-day and -week analyses suggest that these processes fluctuate within people and are not due to individual differences. For example, on days and weeks when people have higher compassionate goals, they are more responsive to roommates; on days and weeks when those same people have higher self-image goals they are less responsive to roommates. Individual differences (e.g., chronic goals, gender, or social desirability) cannot account for daily and weekly within-person fluctuations in these processes. The lagged analyses and tests of change from pretest to posttest indicate the plausibility of causal paths in these models. Overall, these data do not allow us to rule out causal paths from interpersonal goals to responsiveness to both people’s perceptions of partners’ responsiveness to both people’s compassionate goals and relationship quality in daily assessment across 3 weeks. Tests of change across three weeks and a semester also speak to the cumulative effect of these processes over time. Students’ chronic compassionate goals accumulate to predict long-term changes in responsiveness between roommates. Taken together, these data suggest that interpersonal goals may provide a potential point of intervention – changing people’s daily and weekly goals toward others can change both people’s experiences that day or week, and the following days and weeks, with potential consequences for long-term goal and relationship change.
As a methodological note, results of these studies suggest that the interpersonal processes captured by diary methods may depend on the length of diary intervals. Our data show that the links between actors’ responsiveness to partners and partners’ perceptions of actors’ responsiveness differ depending on whether diaries were completed daily or weekly. In daily and lagged-day analyses (Study 2), roommates showed strong agreement on actors’ responsiveness, suggesting that roommates were drawing from similar events in recalling and reporting their daily relationship experiences. However, we did not find strong actor-partner agreement in weekly assessments (Study 1). Specifically, in lagged-week analyses actors’ Week 1 self-reported responsiveness did not predict change in partners’ perceptions of actors' responsiveness from Weeks 1 to 2. Further, in pretest to posttest change analyses, the association between actors’ responsiveness and partners’ perceptions of actors’ responsiveness became nonsignificant when we controlled for change in partners’ perceived available support. These data suggest that participants report on similar events and experiences in daily reports, however there may be a disconnect between what actors report and what partners perceive when reporting their experiences over longer periods of time (in this case 1 week). Actors may recall their own intentions to be responsive to partners, but these may be independent of partners’ accounts, which may be based on roommates’ supportive behaviors rather than perceptions of roommates’ responsiveness. Thus, daily measures may capture each person’s experiences, but weekly measures may capture each person’s most salient or personally relevant experiences, which may differ for each relationship partner.
Responsiveness and Social Support
Responsiveness and support are theoretically distinct constructs; responsiveness involves tuning into and reacting to others’ states, whereas support involves providing help, information, and assistance, but not necessarily attending to others’ emotional and psychological needs. In the current data, only 2 of 17 significant associations in Figure 2 and Figure 3 could be explained by support, providing empirical evidence that support and responsiveness, although related, are distinct constructs. Previous work also suggests a distinction between support and responsiveness. For example, Collins and colleagues ( Collins, Guichard, Ford, & Feeney, 2006 ) propose that effective caregiving (i.e., support) involves both responsiveness and sensitivity to partners’ signals. Caregiving lacking either responsiveness or sensitivity to partners’ signals is ineffective, consistent with our distinction between responsiveness and support. Whereas effective caregiving predicts improved perceived relationship quality, mood, and self-esteem for recipients, ineffective caregiving does not ( Feeney, 2004 ; Feeney & Collins, 2003 ).
The distinction between support and responsiveness raises many questions about the nature and functions of support versus responsiveness. For example, is responsiveness a subset of support or vice versa? What consequences does each have for relationship outcomes and functioning, and for both relationship partners’ mental and physical health? Theoretical and empirical distinctions between responsiveness and support may clarify their different effects and perhaps resolve issues such as why support is sometimes unhelpful.
These studies are consistent with the hypotheses that interpersonal goals can promote or undermine reciprocal responsiveness between relationship partners and that process can lead to both partners’ relationship quality and interpersonal goals. However, the generalizability of these findings to other types of relationships has yet to be established. The close relationships in these studies were relatively new and the processes shown here were relatively unaffected by relationship history or self-selection into relationships, making them ideally suited for examining relationship processes because they rule out several alternative explanations. We expect that these responsiveness dynamics apply to other types of relationships, for example, between romantic, family, or work dyads. Factors such as relationship history or being in a high-stakes relationship may attenuate or amplify the effects of goals. For example, in relationships with long histories, responsiveness may be affected more strongly by past events rather than by current goals or perceptions of partners’ responsiveness. In high-stakes relationships, for example between firefighters whose lives depend on coworkers, the effects of goals may be amplified because the responsiveness of others to current needs may have life or death consequences. Of course, these are empirical questions to be addressed in future studies.
The nature of the samples may also limit the generalizability of the findings. Both samples were largely female (75% in Study 1 and 71% in Study 2). Despite the relatively small number of men, we tested whether gender moderated the associations found in both studies. Few associations differed by gender relative to the number of tests conducted -- only 2 of 40 product terms between the two samples were significant. Furthermore, results of these moderation analyses were inconsistent between samples – the two paths that were moderated by gender in Study 1 were not consistent between models and did not replicate in Study 2. Additional research with larger samples involving more males would clarify the generalizability of the findings across genders.
Because goals and responsiveness were not manipulated, these studies do not enable us to specify causal relationships among variables. However, several aspects of the results are consistent with causal associations among the variables. Temporal associations among variables in the lagged-week and lagged-day analyses and tests of change from pretest to posttest are consistent with causal links in our path models. Although these effects could be due to unmeasured variables, we ruled out disclosure, social support, self-esteem, and esteem for roommates as alternative explanations. Both studies also provided evidence of an interpersonal process in which actors’ goals and responsiveness lead to changes in partners’ perceptions, behaviors, and goals. Overall, these results support the plausibility of a causal effect of interpersonal goals on responsiveness to roommates, reciprocal associations between people’s responsiveness, and causal effects of perceived partners’ responsiveness on relationship quality and interpersonal goals.
Finally, although exploratory and confirmatory factor analyses across samples suggest that compassionate goals, responsiveness to roommates, perceptions of roommates’ responsiveness, and relationship quality are separate constructs, examination of zero-order correlations in Table 1 and Table 3 suggest a relatively high degree of overlap between these variables. This may lead some to question our findings. However, it is important to remember that our analyses assess change in these constructs over time . If our goals, responsiveness, and relationship quality measures did, in fact, tap the same construct, tests of change would not be as strong or consistent across analyses and samples. At best, we would expect instability in our effects (e.g., compassionate goals would carry significant variance in some cases, but not others).
That said, we acknowledge this limitation and suggest that future research include more specific measures of responsiveness to others and perceptions of others’ responsiveness. In this research, we used standard measures of responsiveness, which included items assessing both participants’ intentions (i.e., what they were trying to do) and behaviors (i.e., what they actually did). Given that our goals measure captures intentions, it is not surprising that these scales were strongly correlated. Future research should distinguish between enacted responsiveness and responsive intentions.
Additional Theoretical Mechanisms
Overall, results from these two studies support our argument that interpersonal goals lead to both people’s relationship quality through the projection of responsiveness and responsiveness dynamics between relationship partners. However, these results generate additional questions, including how interpersonal goals lead to responsiveness and how responsiveness is communicated between partners. We suggest that goals translate to responsiveness through affect and constructive and destructive beliefs about relationship problems.
When people have self-image goals, they report feeling afraid and confused when interacting with their roommates (e.g., Crocker & Canevello, 2008 ) and endorse unconstructive beliefs about relationships (e.g,. believing that people should take care of themselves, even at the expense of others and blaming the other person for relationship problems; Canevello & Crocker, 2009 ; Crocker & Canevello, 2008 ). We suggest that when people have compassionate goals, fear, confusion, and unconstructive beliefs about relationship problems render them insensitive to partners’ needs, resulting in decreased responsiveness.
When people have compassionate goals, they report feeling caring and connected when they interact with their roommates, (e.g., Crocker & Canevello, 2008 ) and they endorse constructive beliefs about relationship problems (e.g., believing that people should take care of each other and sharing responsibility for relationship problems; Canevello & Crocker, 2009 ; Crocker & Canevello, 2008 ). We suggest that when people have compassionate goals, caring, connection, and constructive beliefs about relationship problems lead to a heightened sensitivity to partners’ needs, resulting in increased responsiveness.
We also suggest that responsiveness is communicated to relationship partners through responsive support behaviors (e.g., Collins & Feeney, in press ). That is, partners’ perceptions of actors’ responsiveness should depend on the match between actors’ behaviors and partners’ needs. When actors’ responsive behaviors attend to partners’ needs, partners should report higher perceptions of actors’ responsiveness. When actors’ responsive behaviors do not address supports sought by partners, partners should report lower perceptions of actors’ responsiveness.
Perceived partner responsiveness is a core feature of close, satisfying relationships and can be created in at least two ways: through an intrapersonal process of projecting own responsiveness onto others or through an interpersonal process whereby people’s reported responsiveness is perceived by partners. But how does responsiveness originate? Our findings suggest that people’s interpersonal goals (to either support others or construct and maintain desired images of the self) can initiate or inhibit responsiveness and its projection and reciprocation in relationships, which predicts relationship quality and reinforces interpersonal goals for both relationship partners. In light of these data, we suggest that people not only can create the types of relationships that they want - those characterized by high responsiveness, and consequently, higher quality, but they also can create responsive, high quality relationships for others.
Acknowledgments
The project described was supported by Grant Number R01MH058869 from the National Institute of Mental Health. The content is solely the responsibility of the authors and does not necessarily represent the official views of the National Institute of Mental Health or the National Institutes of Health.
We are grateful to Samir Adhia, Laura Diskin, George Payapilly, Cristina Popa, Robby Uppal, Megan Villwock, and Claire Woodward for their assistance with data collection and to Riia Luhtanen, Kate Oddi, Juliana G. Breines, Dominik Mischkowski, and Mary Liu for their invaluable assistance in both of these studies. We are also grateful to our friends at Learning as Leadership for inspiring our research on self-image and compassionate goals.
The following manuscript is the final accepted manuscript. It has not been subjected to the final copyediting, fact-checking, and proofreading required for formal publication. It is not the definitive, publisher-authenticated version. The American Psychological Association and its Council of Editors disclaim any responsibility or liabilities for errors or omissions of this manuscript version, any version derived from this manuscript by NIH, or other third parties. The published version is available at www.apa.org/journals/psp
Similarly, being responsive does not necessitate having compassionate goals. People may be responsive to others without intending to be caring or compassionate. For example, Cathy may be responsive to Dan, not because she has compassionate goals, but because she wants Dan to like her. She may make Dan feel comfortable about himself and valued as a person, be sensitive to his feelings, and understand his concerns, but Cathy may not have compassionate goals to make a positive difference in Dan’s life or avoid being selfish or self-centered. Although compassionate goals are distinguishable from responsiveness, we hypothesize that when people have compassionate goals, they typically are responsive.
2 Of the remaining five percent of respondents, one pair completed the pretest and 9 weekly surveys; two pairs completed the pretest, posttest, and 5 weekly surveys; and three pairs completed the pretest and less than 6 weekly surveys.
3 Participants reported that completing the weekly surveys did not strongly affect their reports or their roommate relationships during Study 1. In the posttest measure, students rated the influence of the weekly records on scales ranging from 1 ( not at all ) to 7 ( very much ). Although we do not have a control group for comparison, participants did not report that it was particularly difficult to complete the weekly surveys ( M = 3.58) and felt that their weekly surveys were accurate ( M = 5.33). Completing the records did increase how much they thought about their roommates and relationships ( M = 4.34) and positive thoughts about the relationships ( M = 3.35). However, they did not report that completing the surveys affected their behavior ( M = 2.61) or the occurrence of negative ( M = 1.94) or positive ( M = 2.67) events between roommates.
4 Although several authors provide guidelines for centering in multilevel models (e.g., Kreft & de Leeuw, 1998 ; Raudenbush & Bryk, 2002 ), they also discourage “thoughtless application” of these guidelines ( Enders & Tofighi, 2007 , p. 136) and, instead, encourage centering strategies based on specific substantive research questions.
5 We examined whether compassionate and self-image goals interacted to predict responsiveness to roommates. Weekly goals did not interact to predict weekly responsiveness or change in responsiveness to the following week, both pr s < |.04|, ns. Chronic goals did interact to predict change in responsiveness to roommates from pretest to posttest, pr = .15, p < .05, such that the association between chronic compassionate goals and increased responsiveness to roommates was stronger with lower self-image goals, pr = .59, p < .001, compared to higher self-image goals pr = .58, p < .001.
6 Previous data from our lab suggests that social desirability positively predicts chronic compassionate goals and negatively predicts chronic self-image goals ( Crocker & Canevello, 2008 , Study 1). In Study 1, pretest social desirability positively correlated with chronic self-image goals, r = .23, p < .01, and negatively correlated with chronic compassionate goals, r = −.22, p < .01. Importantly, when we regressed posttest outcomes on pretest social desirability and pretest outcome variables, social desirability did not predict change in responsiveness to roommates ( pr = .09, ns), nor did it predict change in perceptions of roommates’ responsiveness ( pr = .05, ns). Thus, although social desirability is associated with interpersonal goals, it cannot account for associations between goals and changes in outcomes from pretest to posttest. Social desirability also cannot account for within week analyses because person centered predictors remove the influence of individual differences. Finally, lagged analyses test whether goals on a particular week predict changes in outcomes the following week. Because social desirability is a stable personality factor, we see no reason why it would explain the lagged associations between goals and outcomes.
7 We tested alternative explanations for associations unique to the interpersonal models in Figure 3 . Results for other paths are redundant with test of covariates in the intrapersonal models in Figure 2 .
8 Participants reported that completing daily surveys did not strongly affect their reports or their roommate relationships during Study 2. As in Study 1, students rated the influence of the daily records on scales ranging from 1 ( not at all ) to 7 ( very much ) in the Study 2 posttest measure. Again, we do not have a control group for comparison, but participants did not report that it was particularly difficult to complete the weekly surveys ( M = 3.62) and felt that their weekly surveys were accurate ( M = 5.16). Completing the records did increase how much they thought about their roommates and relationships ( M = 4.88) and positive thoughts about the relationships ( M = 3.72). However, although they reported that that completing the surveys affected their behavior somewhat ( M = 3.19), they did not affect the occurrence of negative ( M = 2.01) or positive ( M = 2.95) relationship events.
9 Compassionate goal items differ between studies because Study 2 preliminary factor analyses suggested that we include “be aware of the impact my behavior might have on my roommates’ feelings,” and “avoid doing anything that would be harmful to my roommate.” We included “be supportive of my roommate” and “make a positive difference in my roommates’ life” in the compassionate goals measure in Study 1 because in factor analyses, it loaded strongly with other compassionate goal items and did not load with responsiveness items. Because factor analyses in Study 2 suggested that it loaded equally with compassionate goal and responsiveness items, we removed this item in Study 2 to reduce overlap between measures. Self-image goal items differ between studies because preliminary factor analyses suggested different solutions. Importantly, although the goals measures differ slightly between studies, they provide consistent results.
10 We examined whether compassionate and self-image goals interacted to predict responsiveness to roommates. Goals did not interact to predict responsiveness or change in responsiveness in daily, lagged, or pretest to posttest analyses, all pr s < |.10|, ns.
11 In Study 2, pretest social desirability does not predict chronic self-image ( r = .13, ns) or compassionate goals ( r = −.05, ns). Importantly, when we regress posttest outcomes on pretest social desirability and pretest outcome variables, social desirability does not predict change in responsiveness to roommates, perceptions of roommates’ responsiveness, relationship quality, compassionate goals, or self-image goals, −.05 < prs < = .05, all ns. Again, social desirability also cannot account for within week analyses because person centered predictors remove the influence of individual differences, and because social desirability is a stable personality factor, we see no reason why it would explain the lagged associations between goals and outcomes.
12 We also tested whether compassionate goals moderate projection (i.e., the relation between A’s responsiveness and A’s perceptions of Ps’ responsiveness). In Study 1 weekly analyses, A’s compassionate goals moderated this association, pr = −.07, p <.05, such that the association was stronger for lower compassionate goals, pr = .38, p <.001, than higher compassionate goals, pr = .28, p <.001. In Study 2 daily analyses, the product between A’s compassionate goals and A’s responsiveness was marginal, pr = −.09, p <.07. Again, the association was stronger for lower compassionate goals, pr = .63, p <.001, than higher compassionate goals, pr = .55, p <.001. Thus, these data are consistent with the hypothesis that goals moderate projection, such that projection is stronger when people have lower compassionate goals. However, they do not suggest that people never project when they have compassionate goals. They simply project less.
13 It is important to note that support accounts for the association between change in actors’ responsiveness to partners and change in partners’ perceptions of actors’ responsiveness from pretest to posttest. This result may indicate that partners detect responsiveness through supportive behavior. However, we hesitate to overstate or over-interpret this finding because 1) it did not replicate in within-week or lagged-week analyses and 2) we did not specifically test mediation.
Contributor Information
Amy Canevello, Research Center for Group Dynamics, Institute for Social Research, University of Michigan.
Jennifer Crocker, Research Center for Group Dynamics, Institute for Social Research, and Department of Psychology, University of Michigan.
- Abbey A, Andrews FM, Halman LJ. Provision and receipt of social support and disregard: What is their impact on the marital life quality of infertile and fertile couples? Journal of Personality and Social Psychology. 1995; 68 :455–469. [ PubMed ] [ Google Scholar ]
- Aiken LS, West SG. Multiple Regression: Testing and Interpreting Interactions. Newbury Park: Sage; 1991. [ Google Scholar ]
- Baumeister RF, Leary MR. The need to belong: Desire for interpersonal attachments as a fundamental human motivation. Psychological Bulletin. 1995; 117 :497–529. [ PubMed ] [ Google Scholar ]
- Berg JH, Archer RL. Responses to self-disclosure and interaction goals. Journal of Experimental Social Psychology. 1982; 18 :501–512. [ Google Scholar ]
- Bolger N, Zuckerman A, Kessler RC. Invisible support and adjustment to stress. Journal of Personality and Social Psychology. 2000; 79 :953–961. [ PubMed ] [ Google Scholar ]
- Bowlby J. Attachment and loss: Vol. 1. Attachment. New York: Basic Books; 1969. [ Google Scholar ]
- Brown SL, Brown RM. Selective investment theory: Recasting the functional significance of close relationships. Psychological Inquiry. 2006; 17 :1–29. [ Google Scholar ]
- Campbell L, Kashy DA. Estimating actor, partner, and interaction effects for dyadic data using PROC MIXED and HLM: A user-friendly guide. Personal Relationships. 2002; 9 :327–342. [ Google Scholar ]
- Campbell L, Simpson JA, Boldry J, Kashy DA. Perceptions of conflict and support in romantic relationships: The role of attachment anxiety. Journal of Personality and Social Psychology. 2005; 88 :510–531. [ PubMed ] [ Google Scholar ]
- Canevello A, Crocker J. University of Michigan; 2009. Interpersonal goals predict constructive beliefs about relationships. [ Google Scholar ]
- Clark MS, Fitness J, Brissette I. Understanding people's perceptions of relationships is crucial to understanding their emotional lives. In: Brewer MB, Hewstone M, editors. Emotion and motivation. Malden, MA: US: Blackwell Publishing; 2004. pp. 21–46. [ Google Scholar ]
- Clark MS, Mills J. The difference between communal and exchange relationships: What it is and is not. Personality and Social Psychology Bulletin. 1993; 19 :684–691. [ Google Scholar ]
- Clark MS, Monin JK. Giving and receiving communal responsiveness as love. In: Sternberg RJ, Weis K, editors. The new psychology of love. New Haven, CT: US: Yale University Press; 2006. pp. 200–221. [ Google Scholar ]
- Collins NL, Feeney BC. A safe haven: An attachment theory perspective on support seeking and caregiving in intimate relationships. Journal of Personality and Social Psychology. 2000; 78 :1053–1073. [ PubMed ] [ Google Scholar ]
- Collins NL, Feeney BC. An attachment theoretical perspective on social support dynamics in couples: Normative processes and individual differences. In: Sullivan K, Davila J, editors. Support Processes in Intimate Relationships. Oxford: University Press; (in press) [ Google Scholar ]
- Collins NL, Guichard AC, Ford MiB, Feeney BC. Responding to need in intimate relationships: Normative processes and individual differences. In: Mikulincer M, Goodman GS, editors. Dynamics of romantic love: Attachment, caregiving, and sex. New York, NY: US: Guilford Press; 2006. pp. 149–189. [ Google Scholar ]
- Crocker J, Canevello A. Creating and undermining social support in communal relationships: The roles of compassionate and self-image goals. Journal of Personality and Social Psychology. 2008; 95 :555–575. [ PubMed ] [ Google Scholar ]
- Cutrona CE, Hessling RM, Suhr JA. The influence of husband and wife personality on marital social support interactions. Personal Relationships. 1997; 4 :379–393. [ Google Scholar ]
- Cutrona CE, Shaffer PA, Wesner KA, Gardner KA. Optimally matching support and perceived spousal sensitivity. Journal of Family Psychology. 2007; 21 :754–758. [ PubMed ] [ Google Scholar ]
- Davis D. Determinants of responsiveness in dyadic interaction. In: Ickes W, Knowles ES, editors. Personality, Roles, and Social Behavior. New York: Springer-Verlag; 1982. [ Google Scholar ]
- Enders CK, Tofighi D. Centering predictor variables in cross-sectional multilevel models: A new look at an old issue. Psychological Methods. 2007; 12 :121–138. [ PubMed ] [ Google Scholar ]
- Feeney BC. A secure base: Responsive support of goal strivings and exploration in adult intimate relationships. Journal of Personality and Social Psychology. 2004; 87 :631–648. [ PubMed ] [ Google Scholar ]
- Feeney BC, Collins NL. Motivations for caregiving in adult intimate relationships: Influences on caregiving behavior and relationship functioning. Personality and Social Psychology Bulletin. 2003; 29 :950–968. [ PubMed ] [ Google Scholar ]
- Fincham FD, Beach SRH. Marriage in the new millennium: Is there a place for social cognition in marital research? Journal of Social and Personal Relationships. 1999; 16 :685–704. [ Google Scholar ]
- Fruzzetti AE, Jacobson NS, Blechman EA. Emotions and the family: For better or for worse. Hillsdale, NJ, England: Lawrence Erlbaum Associates, Inc; 1990. Toward a behavioral conceptualization of adult intimacy: Implications for marital therapy; pp. 117–135. [ Google Scholar ]
- Gable SL, Reis HT. Intimacy and the self: An iterative model of the self and close relationships. In: Noller P, Feeney JA, editors. Close relationships: Functions, forms and processes. Hove, England: Psychology Press/Taylor & Francis (UK); 2006. pp. 211–225. [ Google Scholar ]
- Gleason MEJ, Iida M, Bolger N, Shrout PE. Daily supportive equity in close relationships. Personality and Social Psychology Bulletin. 2003; 29 :1036–1045. [ PubMed ] [ Google Scholar ]
- Gore JS, Cross SE, Morris ML. Let’s be friends: Relational self-construal and the development of intimacy. Personal Relationships. 2006; 13 :83–102. [ Google Scholar ]
- Gottman JM, Levenson RW. Marital processes predictive of later dissolution: Behavior, physiology, and health. Journal of Personality and Social Psychology. 1992; 63 :221–233. [ PubMed ] [ Google Scholar ]
- Greene K, Derlega VJ, Mathews A, Vangelisti AL, Perlman D. The Cambridge handbook of personal relationships. New York, NY: US: Cambridge University Press; 2006. Self-disclosure in personal relationships; pp. 409–427. [ Google Scholar ]
- Griffin D, Gonzalez R. Correlational analysis of dyad-level data in the exchangeable case. Psychological Bulletin. 1995; 118 :430–439. [ Google Scholar ]
- Helgeson VS, Fritz HL. A theory of unmitigated communion. Personality and Social Psychology Review. 1998; 2 :173–183. [ PubMed ] [ Google Scholar ]
- Kashy DA, Donnellan MB, Burt SA, McGue M. Growth curve models for indistinguishable dyads using multilevel modeling and structural equation modeling: The case of adolescent twins' conflict with their mothers. Developmental Psychology. 2008; 44 :316–329. [ PubMed ] [ Google Scholar ]
- Kelley HH, Berscheid E, Christensen A, Harvey JH, Huston TL, Levinger G, et al. Analyzing close relationships. In: Kelley HH, Berscheid E, Christensen A, Harvey JH, Huston TL, Levinger G, McClintock E, Peplau LA, Peterson DR, editors. Close Relationships. New York: Freeman; 1983. pp. 20–67. [ Google Scholar ]
- Kelley HH, Thibaut JW. Interpersonal relations : A theory of interdependence. New York: Wiley; 1978. [ Google Scholar ]
- Kenny DA. Cross-lagged panel correlation: A test for spuriousness. Psychological Bulletin. 1975; 82 :887–903. [ Google Scholar ]
- Kenny DA, Acitelli LK. Accuracy and bias in the perception of the partner in a close relationship. Journal of Personality and Social Psychology. 2001; 80 :439–448. [ PubMed ] [ Google Scholar ]
- Kenny DA, Kashy DA, Cook WL. Dyadic data analysis. New York, NY: US: Guilford Press; 2006. [ Google Scholar ]
- Kreft I, de Leeuw J. Introducing Multilevel Modeling. London: Sage; 1998. [ Google Scholar ]
- Laurenceau J-P, Barrett LF, Pietromonaco PR. Intimacy as an interpersonal process: The importance of self-disclosure, partner disclosure, and perceived partner responsiveness in interpersonal exchanges. Journal of Personality and Social Psychology. 1998; 74 :1238–1251. [ PubMed ] [ Google Scholar ]
- Laurenceau J-P, Barrett LF, Rovine MJ. The interpersonal process model of intimacy in marriage: A daily-diary and multilevel modeling approach. Journal of Family Psychology. 2005; 19 :314–323. [ PubMed ] [ Google Scholar ]
- Laurenceau J-P, Rivera LM, Schaffer AR, Pietromonaco PR, Mashek DJ, Aron AP. Handbook of closeness and intimacy. Mahwah, NJ, US: Lawrence Erlbaum Associates Publishers; 2004. Intimacy as an interpersonal process: Current status and future directions; pp. 61–78. [ Google Scholar ]
- Leary MR. Self-presentation: Impression management and interpersonal behavior. Madison, WI: US: Brown & Benchmark Publishers; 1995. [ Google Scholar ]
- Lemay EP, Jr, Clark MS. How the head liberates the heart: Projection of communal responsiveness guides relationship promotion. Journal of Personality and Social Psychology. 2008; 94 :647–671. [ PubMed ] [ Google Scholar ]
- Lemay EP, Jr, Clark MS, Feeney BC. Projection of responsiveness to needs and the construction of satisfying communal relationships. Journal of Personality and Social Psychology. 2007; 92 :834–853. [ PubMed ] [ Google Scholar ]
- Manne S, Ostroff J, Rini C, Fox K, Goldstein L, Grana G. The interpersonal process model of intimacy: The role of self-disclosure, partner disclosure, and partner responsiveness in interactions between breast cancer patients and their partners. Journal of Family Psychology. 2004; 18 :589–599. [ PubMed ] [ Google Scholar ]
- Mikulincer M, Shaver PR, Gillath O, Nitzberg RA. Attachment, Caregiving, and Altruism: Boosting Attachment Security Increases Compassion and Helping. Journal of Personality and Social Psychology. 2005; 89 (5):817–839. [ PubMed ] [ Google Scholar ]
- Miller LC, Berg JH, Archer RL. Openers: Individuals who elicit intimate self-disclosure. Journal of Personality and Social Psychology. 1983; 44 :1234–1244. [ Google Scholar ]
- Mills J, Clark MS. Exchange and communal relationships. In: Wheeler L, editor. Review of personality and social psychology. Vol. 3. Beverly Hills, CA: Sage; 1982. pp. 121–144. [ Google Scholar ]
- Murray SL, Griffin DW, Rose P, Bellavia GM. Calibrating the sociometer: The relational contingencies of self-esteem. Journal of Personality and Social Psychology. 2003; 85 :63–84. [ PubMed ] [ Google Scholar ]
- Murray SL, Holmes JG, Collins NL. Optimizing assurance: The risk regulation system in relationships. Psychological Bulletin. 2006; 132 :641–666. [ PubMed ] [ Google Scholar ]
- Murray SL, Rose P, Bellavia GM, Holmes JG, Kusche AG. When rejection stings: How self-esteem constrains relationship-enhancement processes. Journal of Personality and Social Psychology. 2002; 83 :556–573. [ PubMed ] [ Google Scholar ]
- Patterson ML. An arousal model of interpersonal intimacy. Psychological Review. 1976; 83 :235–245. [ Google Scholar ]
- Plickert G, Côté RR, Wellman B. It's not who you know, it's how you know them: Who exchanges what with whom? Social Networks. 2007; 29 :405–429. [ Google Scholar ]
- Raudenbush SW, Bryk AS. Hierarchical linear models: Applications and data analysis methods. (Second ed.) Thousand Oaks: Sage; 2002. [ Google Scholar ]
- Reis HT, Clark MS, Holmes JG. Perceived partner responsiveness as an organizing construct in the study of intimacy and closeness. In: Mashek DJ, Aron AP, editors. Handbook of closeness and intimacy. Mahwah, NJ: US: Lawrence Erlbaum Associates Publishers; 2004. pp. 201–225. [ Google Scholar ]
- Reis HT, Patrick BC. Attachment and intimacy: Component processes. In: Higgins ET, Kruglanski AW, editors. Social psychology: Handbook of basic principles. New York, NY: US: Guilford Press; 1996. pp. 523–563. [ Google Scholar ]
- Reis HT, Shaver P. Intimacy as an interpersonal process. In: Duck S, Hay DF, Hobfoll SE, Ickes W, Montgomery BM, editors. Handbook of personal relationships: Theory, research and interventions. Oxford, England: John Wiley & Sons; 1988. pp. 367–389. [ Google Scholar ]
- Rogosa D. A critique of cross-lagged correlation. Psychological Bulletin. 1980; 88 :245–258. [ Google Scholar ]
- Rosenberg M. Society and the adolescent self-image. Princeton, NJ: Princeton University Press; 1965. [ Google Scholar ]
- Rosenthal R, Rosnow R. Essentials of behavioral reserach: Methods and data analysis. (2nd ed.) New York: McGraw-Hill; 1991. [ Google Scholar ]
- Rusbult CE, Verette J, Whitney GA, Slovik LF, Lipkus I. Accommodation processes in close relationships: Theory and preliminary empirical evidence. Journal of Personality and Social Psychology. 1991; 60 :53–78. [ Google Scholar ]
- Sarason BR, Shearin EN, Pierce GR, Sarason IG. Interrelations of social support measures: Theoretical and practical implications. Journal of Personality and Social Psychology. 1987; 52 :813–832. [ Google Scholar ]
- Sarason IG, Pierce GR, Sarason BR. Social support and interactional processes: A triadic hypothesis. Journal of Social and Personal Relationships. 1990; 7 :495–506. [ Google Scholar ]
- Spielberger CD, Vagg PR, Barker LR, Donham GW, Westberry LG, editors. The factor structure of the state-trait anxiety inventory. Vol. 7. Washington, D. C: Hemisphere; 1980. [ Google Scholar ]
- Uchino BN. Social support and physical health: Understanding the health consequences of relationships. New Haven, CT: US: Yale University Press; 2004. [ Google Scholar ]
- Uchino BN, Cacioppo JT, Kiecolt-Glaser JK. The relationship between social support and physiological processes: A review with emphasis on underlying mechanisms and implications for health. Psychological Bulletin. 1996; 119 :488–531. [ PubMed ] [ Google Scholar ]
- Vinokur A, Schul Y, Caplan RD. Determinants of perceived social support: Interpersonal transactions, personal outlook, and transient affective states. Journal of Personality and Social Psychology. 1987; 53 :1137–1145. [ PubMed ] [ Google Scholar ]
- West SG, Biesanz JC, Pitts SC, editors. Causal inference and generalization in field settings: Experimental and quasi-experimental designs. Cambridge University Press; 2000. [ Google Scholar ]
- Zimet GD, Dahlem NW, Zimet SG, Farley GK. The multidimensional scale of perceived social support. Journal of Personality Assessment. 1988; 52 :30–41. [ PubMed ] [ Google Scholar ]
- Subcontractors
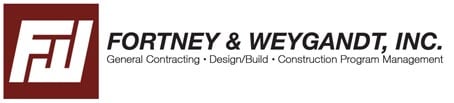
- Corporate History
- Founder's Page
- Why F&W
- General Contracting
- Rollout Program Management
- Design Build
- Value Engineering
- Restaurants
- Multi-Family
- Senior Living
- Educational
- All Project Profiles
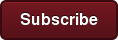
Building An Enduring Relationship - A Case Study
By: Greg Freeh | Dec 08, 2016 | Topics: News
We often write about the importance Fortney & Weygandt, Inc. places on building mutually beneficial relationships with our clients. We firmly believe that loyalty and trust are the foundations of our relationships with our clients. Trust builds confidence and confidence builds repeat customers.
We have been honored to have forged an excellent relationship over the last couple of years with a national extended stay hotel brand, which serves as an excellent case study of how everyone at Fortney & Weygandt works to build a strong client relationship.
The process with this particular client began in August of 2013 when Fortney & Weygandt’s Business Development Department learned of the company’s plans to build a hotel in a nearby city and made an initial contact to inquire about qualifying as a general contractor to be able to bid on the upcoming hotel and future projects. There were a number of follow-up contacts over the ensuing months, but nothing of any real significance. Almost a year later the hotel chain’s growth strategy was pretty much set and the Director of Construction called to request a conference call wherein we learned of their plans to build 40 corporate-owned hotels over the next five years and they were looking for general contractors with design-build capabilities to partner with. We were asked to provide a conceptual budget for a new prototype design, which we did. They were very pleased with our numbers and two months later representatives from the hotel travelled to Cleveland to meet and further discuss their plans and how Fortney & Weygandt could factor into those plans.
As the hotel chain and Fortney & Weygandt continued to communicate, exchanging information and sharing ideas, both organizations’ confidence in each other strengthened. Soon Fortney & Weygandt received a call from one of the hotel’s franchisee telling us that they had been referred to us by the corporate office and requesting us to bid their newest hotel. Once again our numbers prevailed and within a few months ground was broken for the first hotel we would build with that brand name.
During the course of construction for that first hotel, our project team demonstrated the Fortney & Weygandt commitment to continuously communicate with both the owner and the architect to keep them apprised of the project’s progress and any potential issues in order to prevent issues from becoming problems. The fact that every day our team, both in the field and in the office, earned the owner’s trust by demonstrating our competency, reassured our client beyond any doubt that they made the right choice selecting Fortney & Weygandt.
Our reputation within the brand family spread and soon we were bidding another hotel for another franchisee that had actually traveled to the site of the first hotel under construction to view the quality of workmanship, observe the level of coordination and leadership and talk with the project team. We soon broke ground on a hotel for that franchisee. Not long after, we began bidding on corporate-owned hotels.
As of this writing, two hotels have been completed with two more under way. In addition, we have or will soon bid on six more hotels of this brand in six separate states, both franchisee-owned and corporate-owned.
This case study is just the most recent example of how valuable we consider our relationships with our clients and business associates to be and how our 37 years of working hard to earn the trust and loyalty of our clients have resulted in so many repeat customers.
To view our completed hotel projects, click here .
To learn more about Fortney & Weygandt's services, click here.
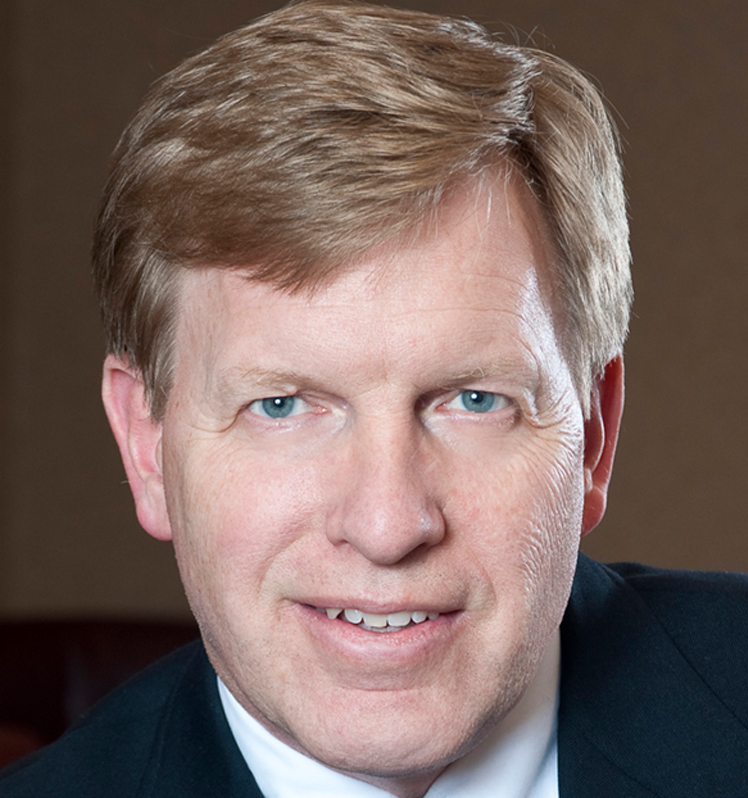
As CEO, he collaborates with the management team to achieve the company’s growth strategies. He oversees the day-to-day operations of the company, providing overall management leadership for all department leaders. He ensures that business operations are efficient and effective while optimizing the company’s staffing and resources. Greg actively develops and monitors strategies for ensuring the company’s long-term financial viability.
Associations:

- Market Segments
- Projects Bidding
Case Study with Suzy Myers Jackson at Spark Youth NYC: Diversifying Revenue Beyond a Gala through Relationship Building The Business Behind Fundraising
Suzy Myers Jackson didn’t expect to have a career in fundraising, but when the opportunity to lead an organization that aligned with her passion for theatre presented itself, she hit the ground running. After 17 years at Opening Act New York, Suzy became the Executive Director of SparkYouth NYC. Now, she’s bringing a refreshing style of tried and true fundraising opportunities to expand opportunities for young people in New York City. What You Will Discover: ✔️ We all learn from experience, but establishing a process to evaluate and adapt to those experiences can make all the difference for your organization ✔️ Skills you have developed prior to becoming a fundraiser will always come in handy, like theatre! ✔️ Prioritizing relationship building leading up to the event makes a tremendous impact on how successful the event is for your organization ✔️ Relationships held by members of your Board of Directors can bring new energy to your events and lead to future connections —————————————— Suzy Myers Jackson is the Executive Director of SparkYouthNYC, an organization that ignites growth in community-based, youth-centered organizations through a three-pronged model of financial investment (trust-based, multi-year, unrestricted support), capacity building (SparkYouth's unique Cofactor Lab), and peer support (Executive Director Leadership Collective). Suzy became Executive Director at SparkYouth following 5 years of service on SparkYouth's Board of Directors and 6 years as a SparkYouth grantee. Suzy’s nonprofit background spans nearly 20 years and encompasses a diverse range of expertise, including: fundraising, board development, nonprofit management, strategic planning, leadership coaching, and program development. Suzy was the longtime Executive Director of Opening Act, where she grew the organization’s reach from 3 to 57 of NYC’s most high-need public schools and increased revenue by 80 fold. Under her leadership the organization became a leader in Social Emotional Learning outcomes. Suzy has also been a consultant for such organizations and corporations as Harlem Educational Activities Fund, HBO, the Center for Anti-Violence Education, Youth INC, Brandworkers, STEM From Dance, and INCLUDEnyc. She has developed multiple campaigns and professional development workshops that have been implemented by organizations to raise funds, deepen relationships with key stakeholders, and build a thriving organizational culture. Suzy received her BFA from NYU’s Tisch School of the Arts. She currently lives in Brooklyn with her partner and young sons. LinkedIn: https://www.linkedin.com/in/suzy-myers-jackson-6157419/ Website: https://sparkyouthnyc.org/ —————————————— Welcome to the Business Behind Fundraising podcast, where you’ll discover how to raise the kind of money your big vision requires without adding more events, appeals, or grant applications. Learn how to stop blocking overall revenue growth and start attracting investment-level donors with Sherry Quam Taylor. Sherry Quam Taylor’s unique approach and success combine her background of scaling businesses with her decade-long experience advising nonprofit leadership teams. With out-of-the-box principles and a myth-busting methodology, proven results, and an ability to see solutions to revenue problems that others overlook, her clients regularly add 7-figures of revenue to their bottom line. If you need a true partner to show you how to fully finance your entire mission, both programs, AND overhead, year after year… You’re in the right place! #nonprofits #podcast
- Episode Website
- More Episodes
Asking the better questions that unlock new answers to the working world's most complex issues.
Trending topics
AI insights
EY podcasts
EY webcasts
Operations leaders
Technology leaders
Marketing and growth leaders
Cybersecurity and privacy leaders
Risk leaders
EY Center for Board Matters
EY helps clients create long-term value for all stakeholders. Enabled by data and technology, our services and solutions provide trust through assurance and help clients transform, grow and operate.
Artificial Intelligence (AI)
Strategy, transaction and transformation consulting
Technology transformation
Tax function operations
Climate change and sustainability services
EY Ecosystems
Supply chain and operations
EY Partner Ecosystem
Explore Services
We bring together extraordinary people, like you, to build a better working world.
Experienced professionals
MBA and advanced-degree students
Student and entry level programs
Contract workers
EY-Parthenon careers
Discover how EY insights and services are helping to reframe the future of your industry.
Case studies
Energy and resources
How data analytics can strengthen supply chain performance
13-Jul-2023 Ben Williams
How Takeda harnessed the power of the metaverse for positive human impact
26-Jun-2023 Edwina Fitzmaurice
Banking and Capital Markets
How cutting back infused higher quality in transaction monitoring
11-Jul-2023 Ron V. Giammarco
At EY, our purpose is building a better working world. The insights and services we provide help to create long-term value for clients, people and society, and to build trust in the capital markets.
EY is now carbon negative
19-Sep-2022 Carmine Di Sibio
Our commitment to audit quality
13-Nov-2023 Julie A. Boland
No results have been found
Recent Searches
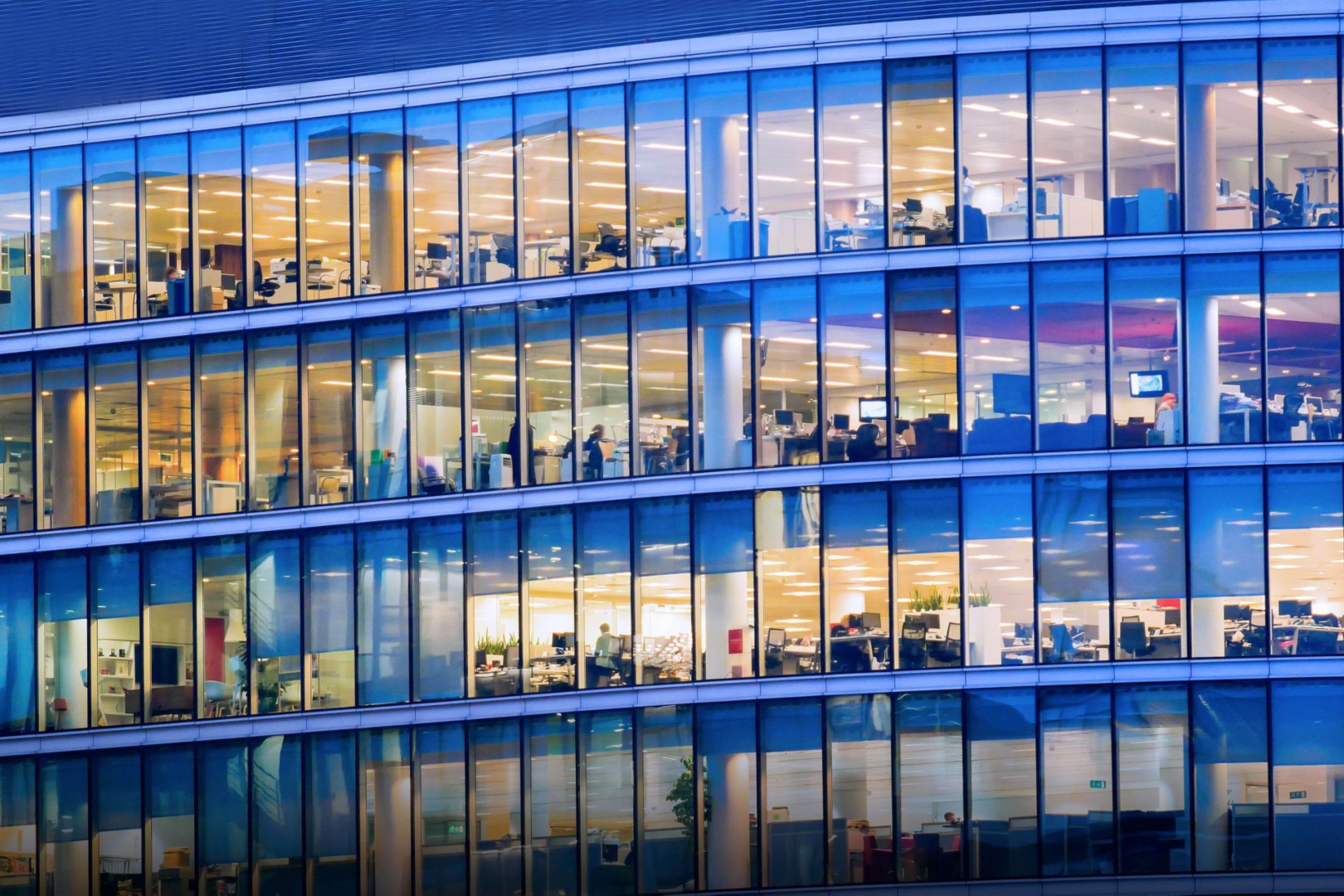
BEPS 2.0: as policies evolve, engagement is key
It remains to be seen whether the US will align its tax law with the OECD/G20’s global BEPS 2.0 rules. MNEs will feel the impact in 2024. Learn more.
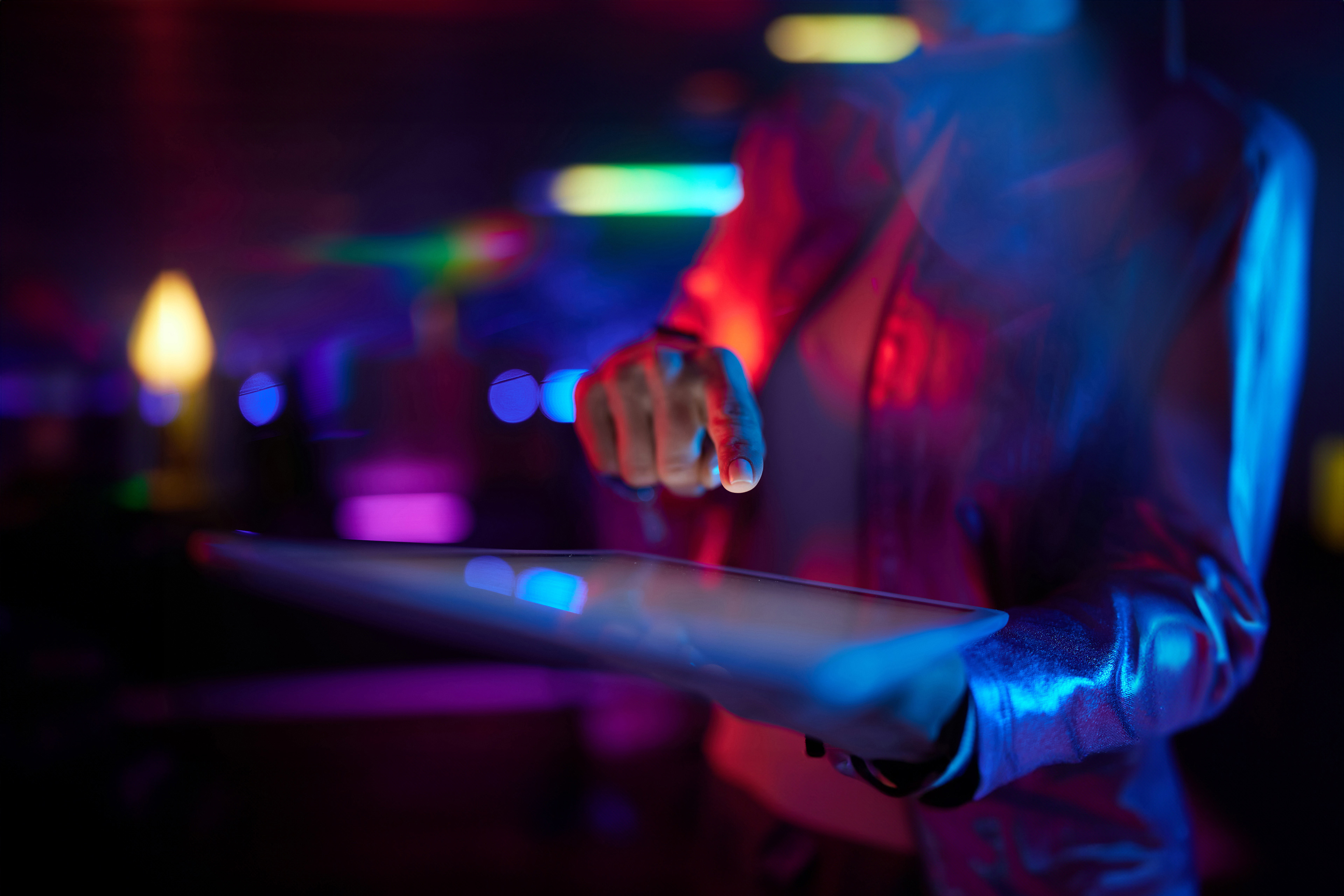
How GenAI strategy can transform innovation
Companies considering or investing in a transformative GenAI strategy should tie generative artificial intelligence use cases to revenue, cost and expense. Learn more
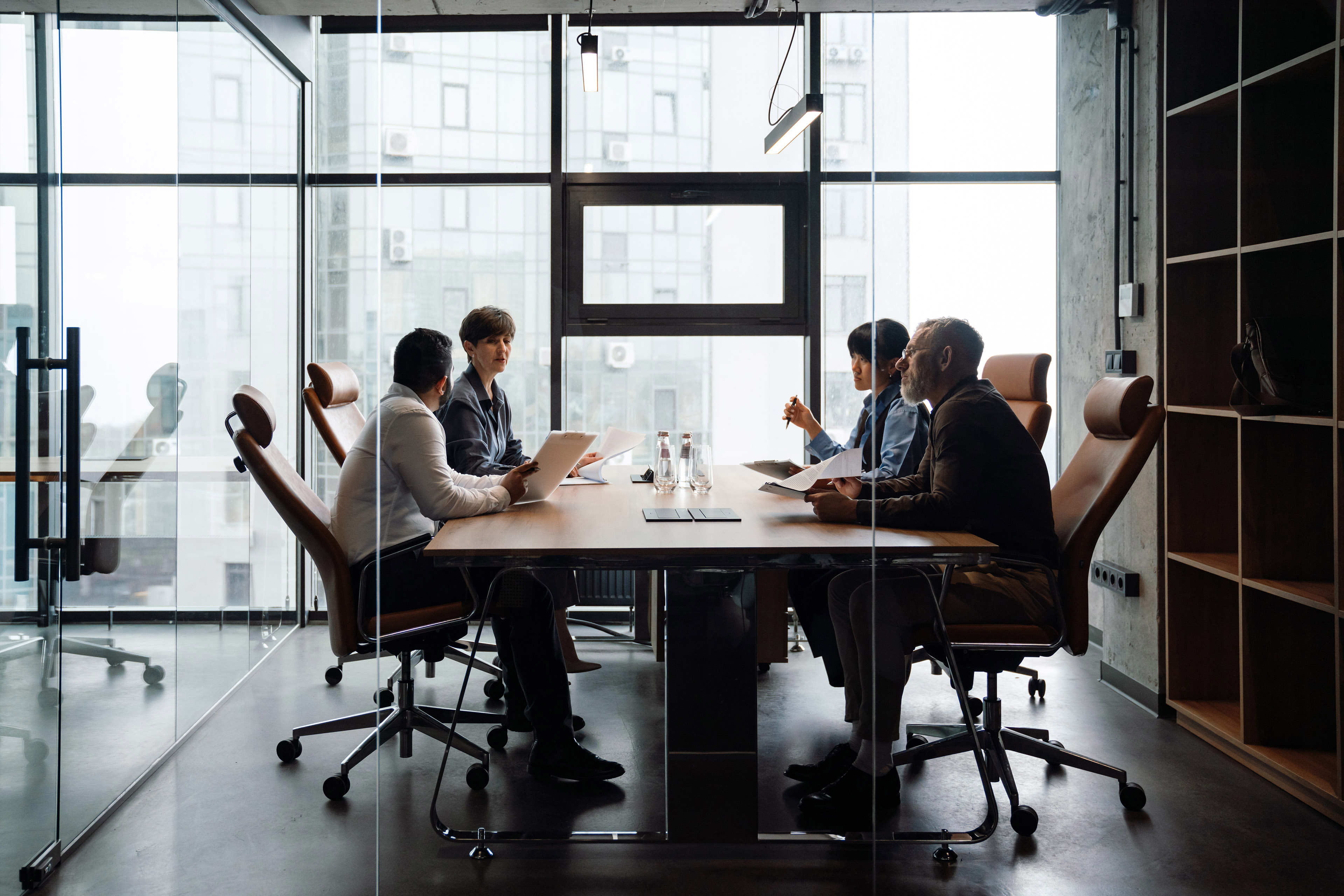
Top five private equity trends for 2024
Read about the five key trends private equity firms will emphasize in 2024 as they create value
Select your location
close expand_more
Consulting at EY is building a better working world by realizing business transformation through the power of people, technology and innovation.
The nature of work is evolving fast, we’re all being asked to adopt new behaviors — to be more innovative, more agile, more collaborative, more everything.
Business today is anything but usual. There is an urgency demanding we look ahead through a new lens, reframe the future and ask new and different questions:
- How do you create customer intimacy without proximity?
- Where does employee centricity meet the future of work?
- How can technology at speed create competitive advantage?
- Where does innovation at scale meet the new "S-curve" of growth?
By placing humans at the center, leveraging technology at speed and enabling innovation at scale, our clients are transforming to realize long-term value for people, business and society as a whole.
How organizations can stop skyrocketing AI use from fueling anxiety</p> "> How organizations can stop skyrocketing AI use from fueling anxiety
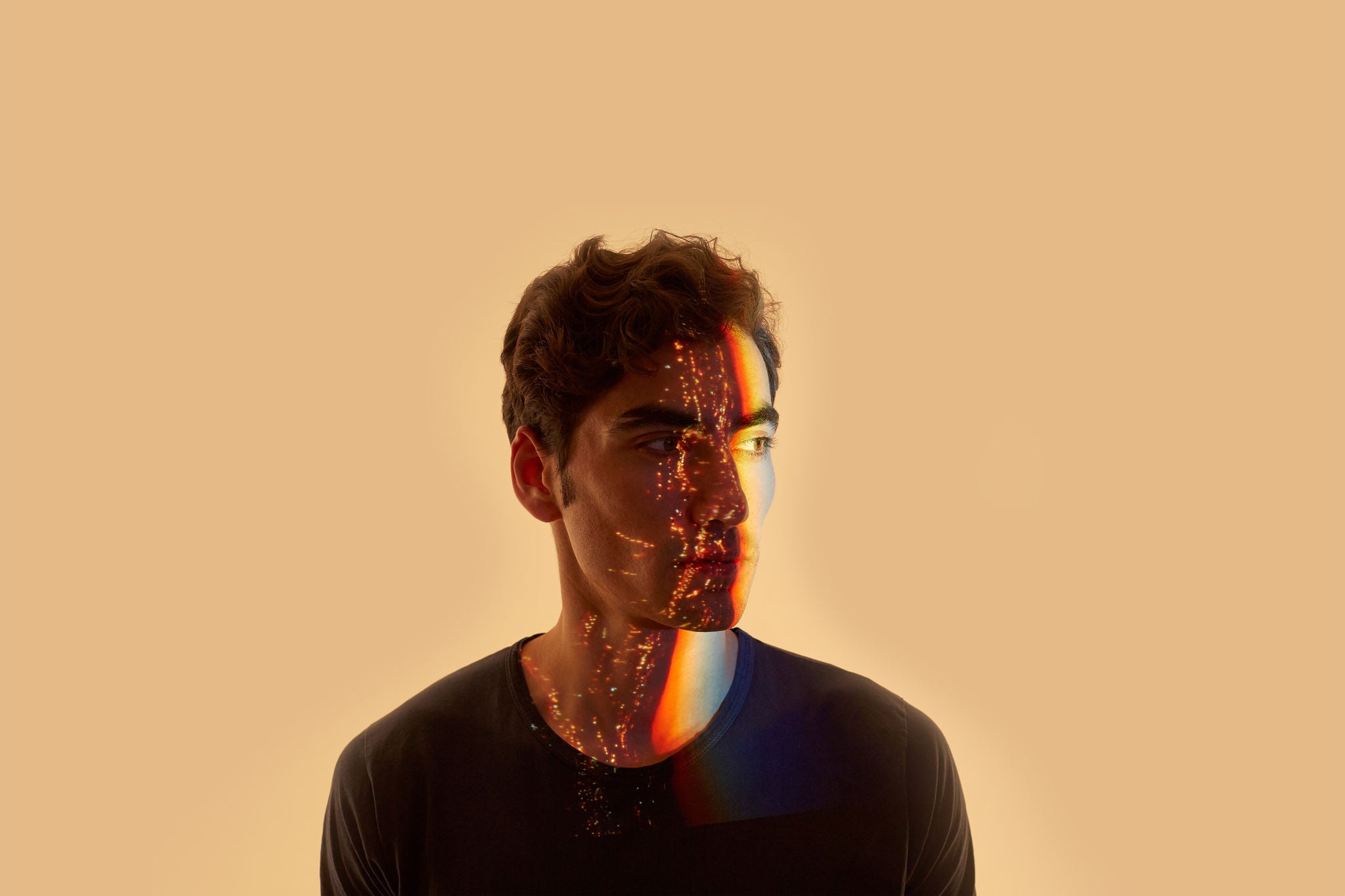
Discover c-suite executive agendas
- Technology leaders' agenda
- Operation leaders' agenda
- Marketing leaders' agenda
- Cyber and privacy leaders' agenda
- Risk leaders' agenda
- Finance leaders’ agenda
Explore our case study library
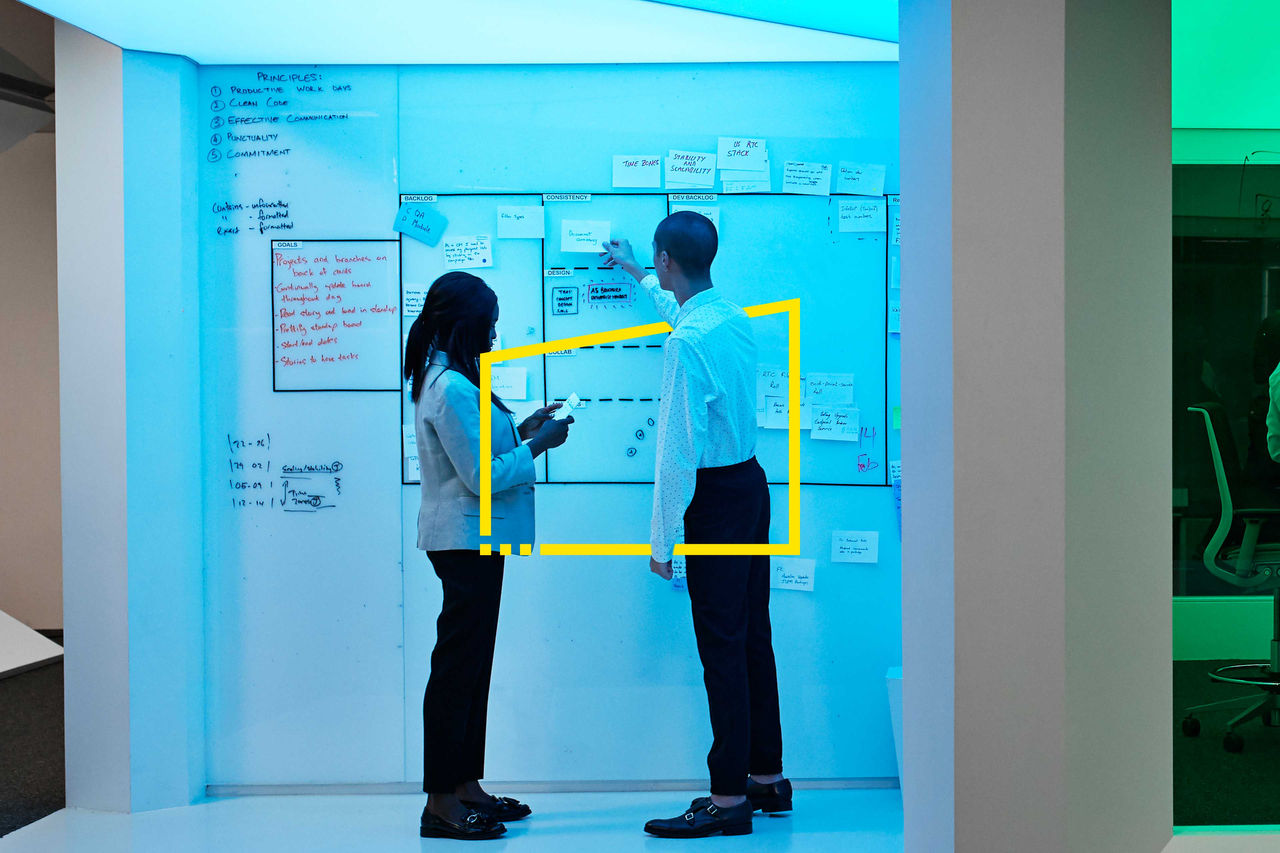
- Our case studies
- Our latest thinking
Explore our case studies
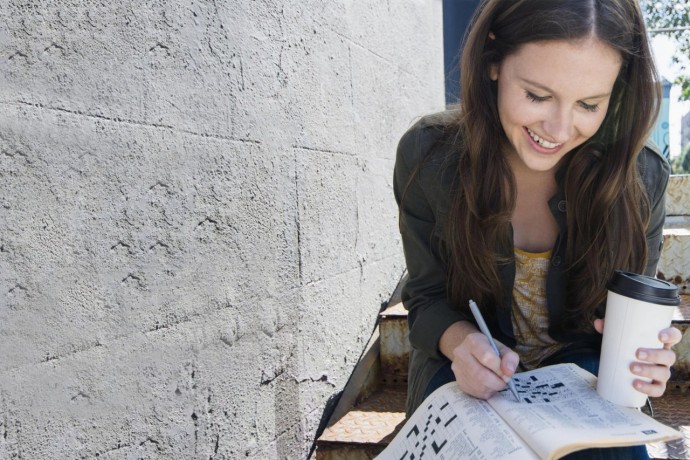
Natural language processing turns search speed into light speed
A managed care company saves hundreds of hours in compliance verification with a new, AI-enabled database. Learn more in this case study.
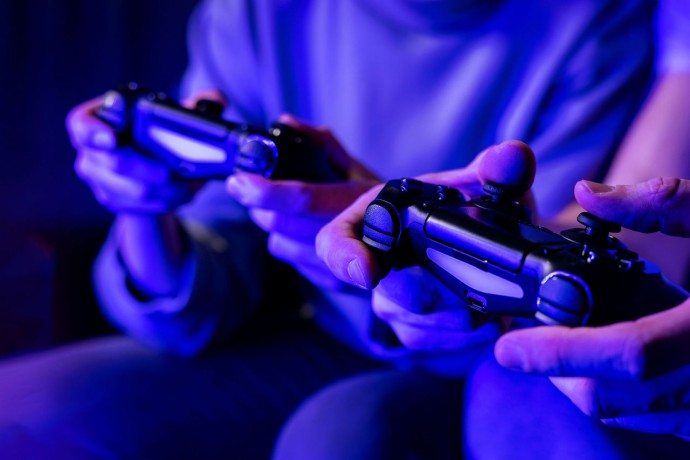
How strong data management becomes a real game-changer
For one video game company, a data-driven culture meant gigs of innovation. Learn more.
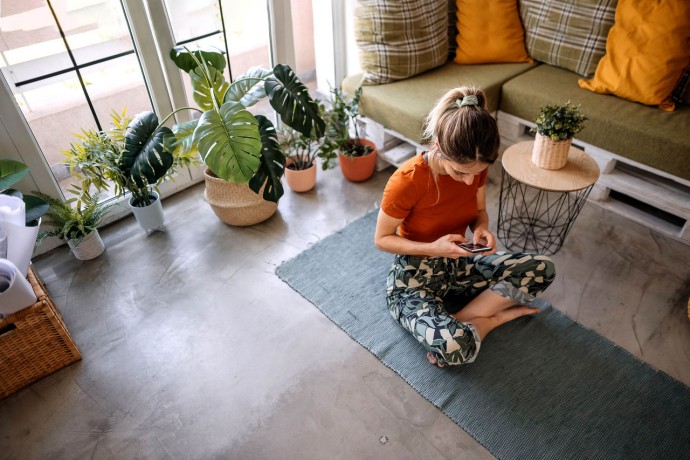
How a major health provider is putting care into patients’ hands
Learn how a leading health care system used digital transformation to enhance its telehealth application and better serve both patients and providers.
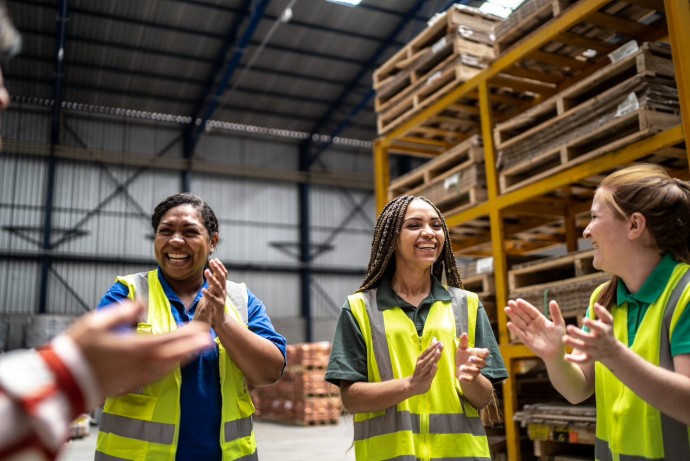
How Ownership Works used a digital springboard to launch a movement
EY teams helped create an online presence — providing employees with the opportunity to build wealth at work.
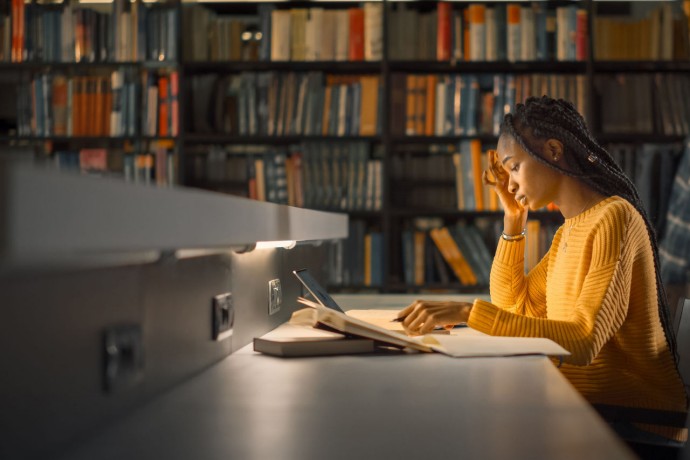
Why cybersecurity should be required reading for higher education
Following a full cybersecurity assessment, a university lands on a solution that can identify, triage and manage data risks.
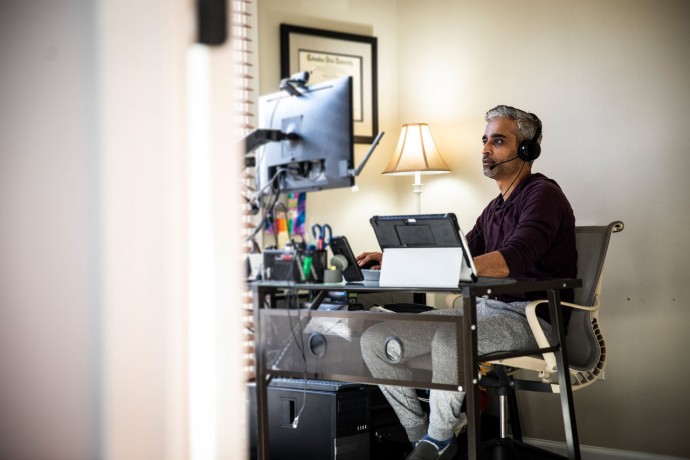
How EY is delivering an exceptional experience for its workforce
Learn how EY People Advisory Services has helped the global EY organization realize one of the largest end-to-end HR transformations on record.
Explore our latest thinking
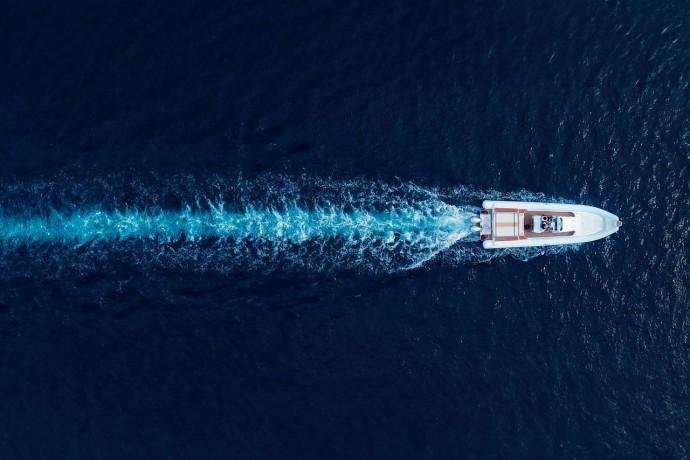
Can core platform modernization position a bank for future success?
Case study: how one regional bank used core platform modernization to build a strong foundation for future profitability.
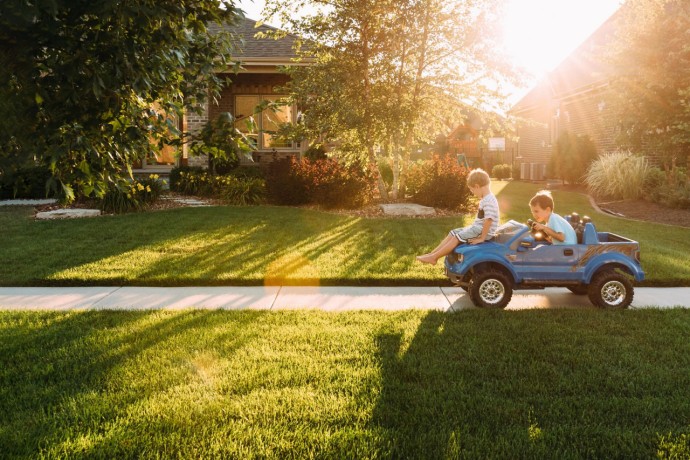
Case study: Intelligent automation shifts a state agency into higher gear
In this case study, learn how a state agency used robotic process automation to serve its customers with speed and convenience.
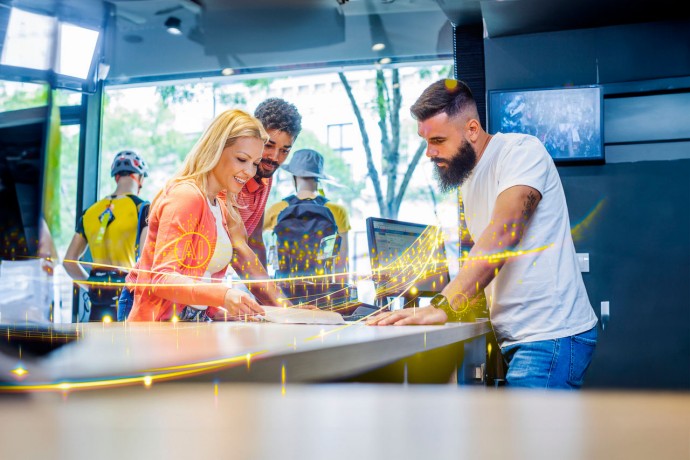
What strategic ops mean for your business
Many executives think of operations as a cost center. A 180-degree shift in thinking could lead to competitive advantage. Learn more.
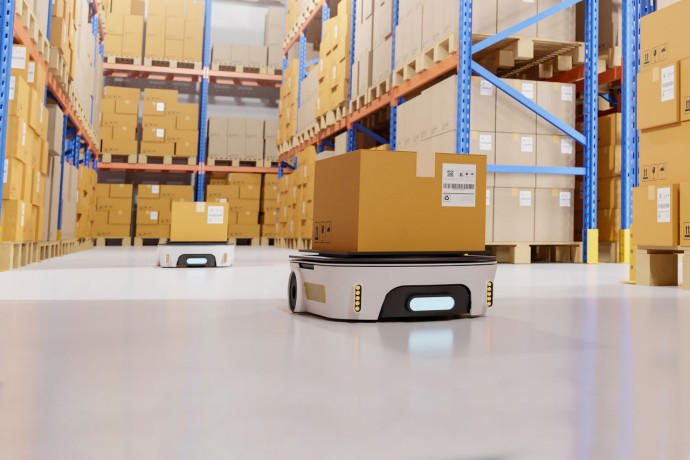
How companies are achieving distribution transformation at speed and scale
Read how organizations can improve global supply chain efficiency through an integrated network of distribution centers.
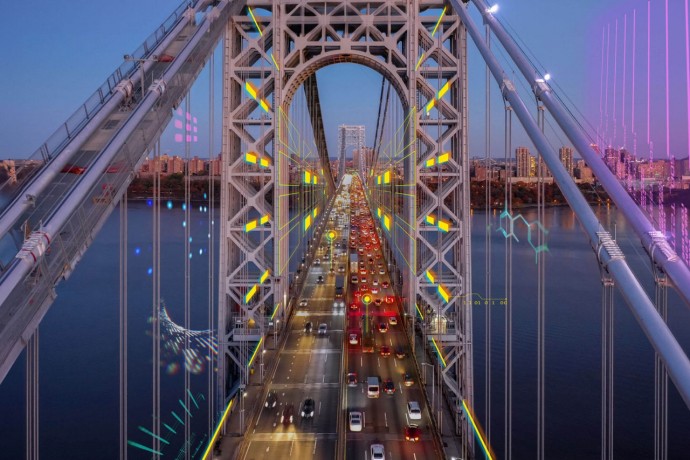
AI Summit NYC: How leaders are tapping the potential of AI
Read session summaries and watch videos from AI Summit NYC where leaders demonstrate how AI in business boosts efficiency and productivity and drives growth.
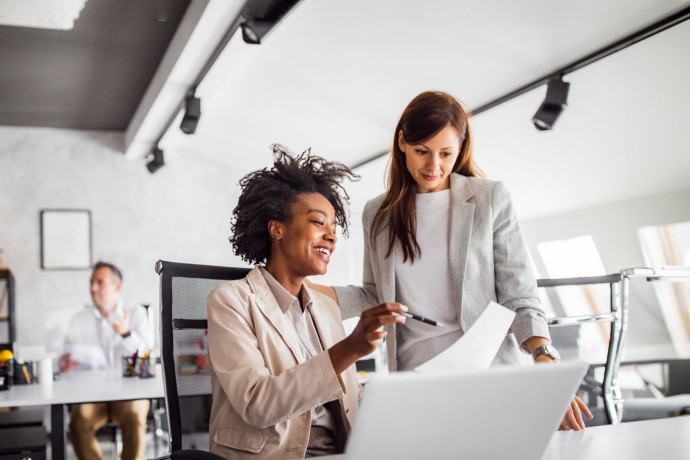
Six ways CFOs and CSCOs can collectively drive value
Despite the challenges of frequently diverging objectives, the value that these executives can collaboratively unlock is transformative. Learn more.
EY Consulting case studies are a window into how we work alongside our clients to deliver strategic, sustainable growth and success.
How EY can help
Transformation EQ™ services
EY Transformation EQ™ services help your business implement leading practices and harness the power of your people to drive transformation success. Learn more.
Service Leader

EY Global People Advisory Services Tax Deputy Leader
Business reinvention consulting services
EY Business reinvention consulting services can help your business deliver long-term value for customers, employees, businesses and society. Learn more.
Evolving technology and data through cloud services
EY’s Business transformation through cloud services can help your business unlock the agility, efficiency and innovation of composable business services. Learn more.
Commercial excellence services
EY Commercial excellence helps organizations unlock, create and sustain new organic value in a fast-changing world.
Customer experience services
The EY Customer Experience solution can help your business integrate CX programs that help deliver sustainable long-term value. Discover more.
Cybersecurity Transformation
Discover how EY's Cybersecurity Transformation solution can help your organization design, deliver, and maintain cybersecurity programs.
Data and Insight-driven Transformation solution
EY Data and Insight-Driven Transformation teams can help you use technology and data to modernize and innovate your business and prepare for future disruption. Learn more.
</b>Enterprise Resilience" href="https://www.ey.com/en_us/services/consulting/enterprise-resilience"> Enterprise Resilience
Discover how EY can help transform your business to navigate disruption with agility, stay competitive in the market and help generate long-term value.
Internal Audit Transformation Services
EY IA services can help your business define the IA vision to create value from thought leadership, digital insights, and risk management. Learn more.
Organization and Workforce Transformation
Our Organization and Workforce Transformation solution can help build workforce capabilities needed to realize organizational strategy. Read more.
People Experience solution
EY People Experience can help you build insight, develop interventions and scale people experience capabilities. Learn more.
Product and Service Innovation consulting services
EY Product and Service Innovation teams can help your business identify, define and design products and services that deliver long-term value. Discover more.
EY Supply Chain Transformation solution
Discover how EY's Supply Chain Transformation solution can help your business move towards fully autonomous, connected supply chains that drive business growth.
Sustainability governance, risk and compliance solution
EY Sustainability GRC can help you implement a digitally enabled ESG framework that is embedded in your operating model and meets regulatory requirements.
Transformation delivery solution
EY Transformation Delivery solution improves your confidence over time in achieving your transformation outcomes. Find out how.
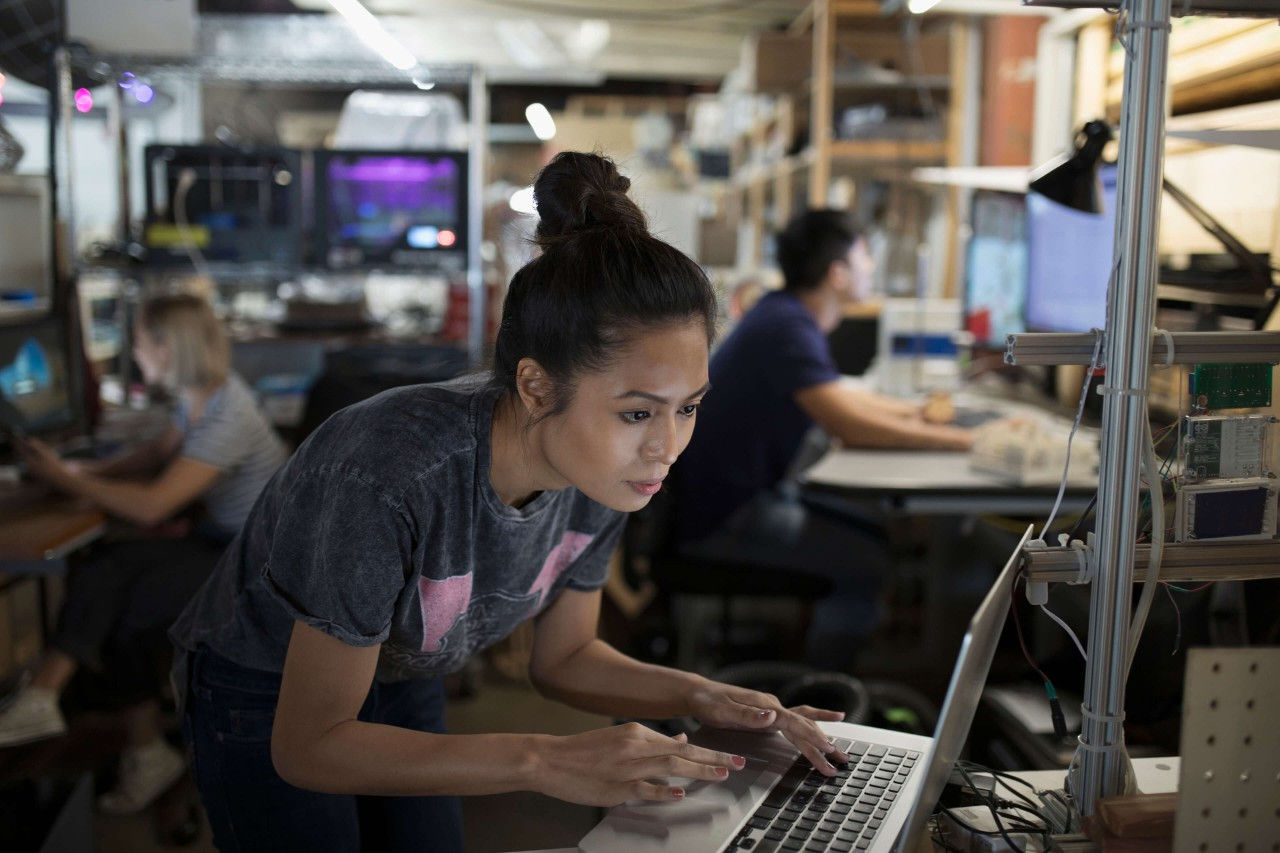
Transformational leader. Innovator. Technologist. Complex problem solver. Strategist. Husband. Father. Mentor. Volunteer. Auto enthusiast.
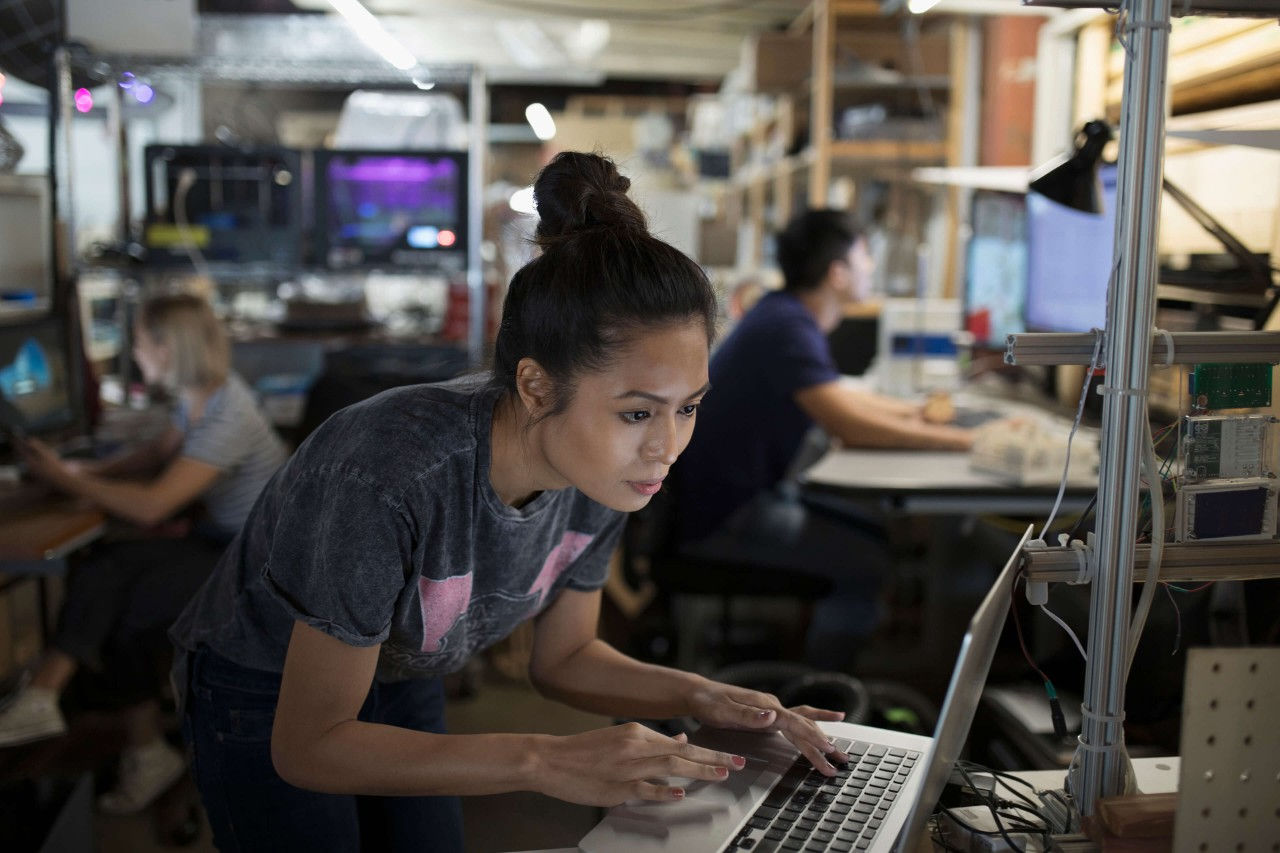
Errol Gardner
Helping clients embrace disruption to drive change. Passionate about inclusion and mentoring. Avid Manchester United fan.
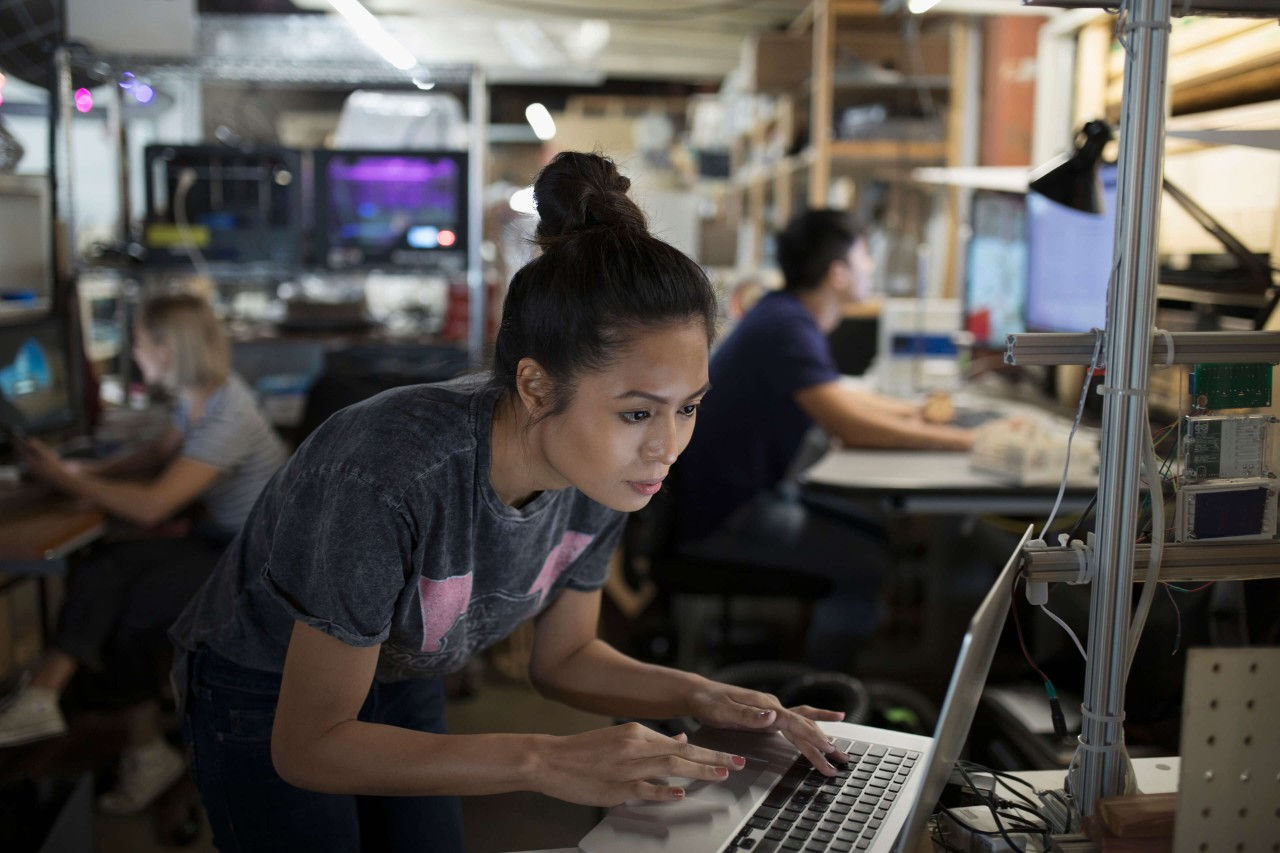
Lisa Caldwell
Recognized for building business models, systems and processes that drive profitable growth in highly competitive markets while championing the next generation of leaders.
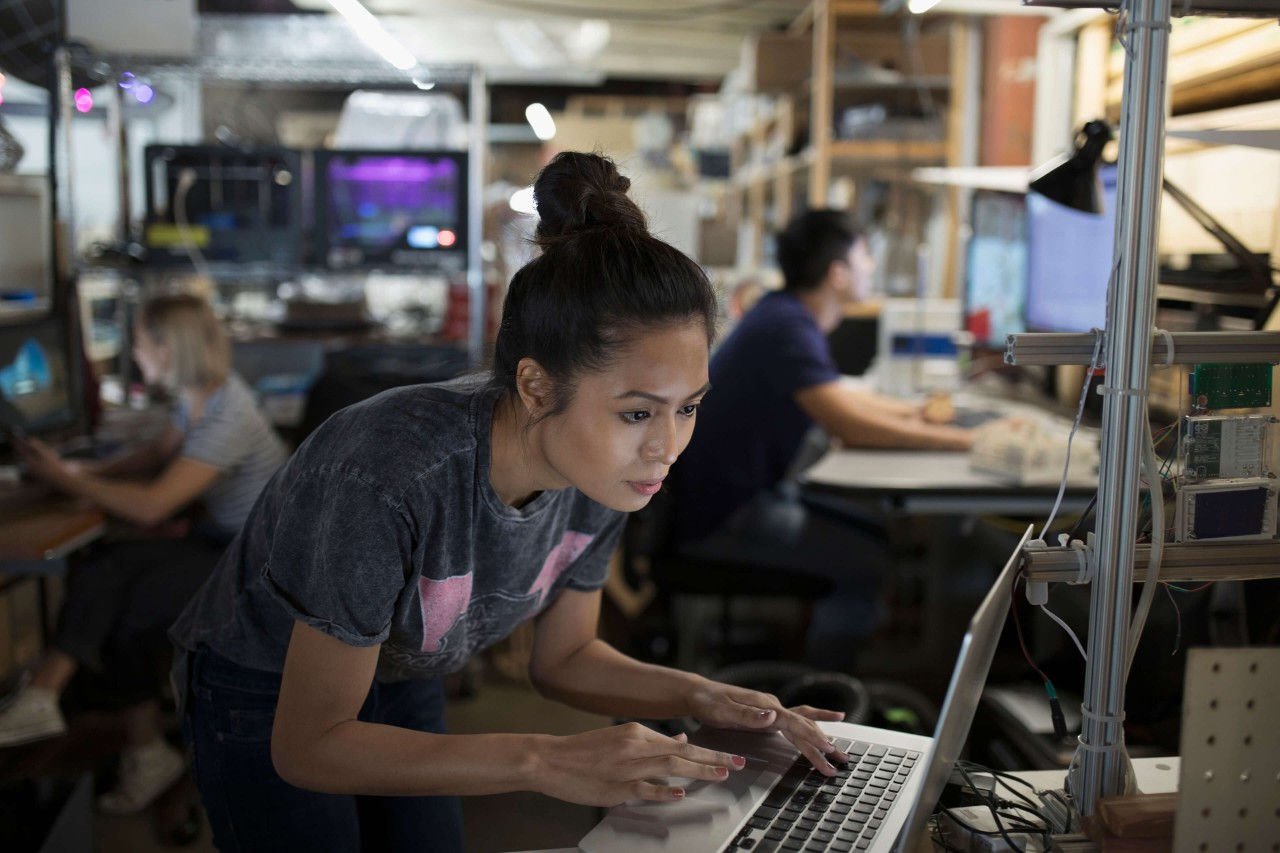
Focused on driving technology transformation, innovation and strategy. Husband. Father. Volunteer. Golfer. Hockey fan. Supports diversity and inclusion.
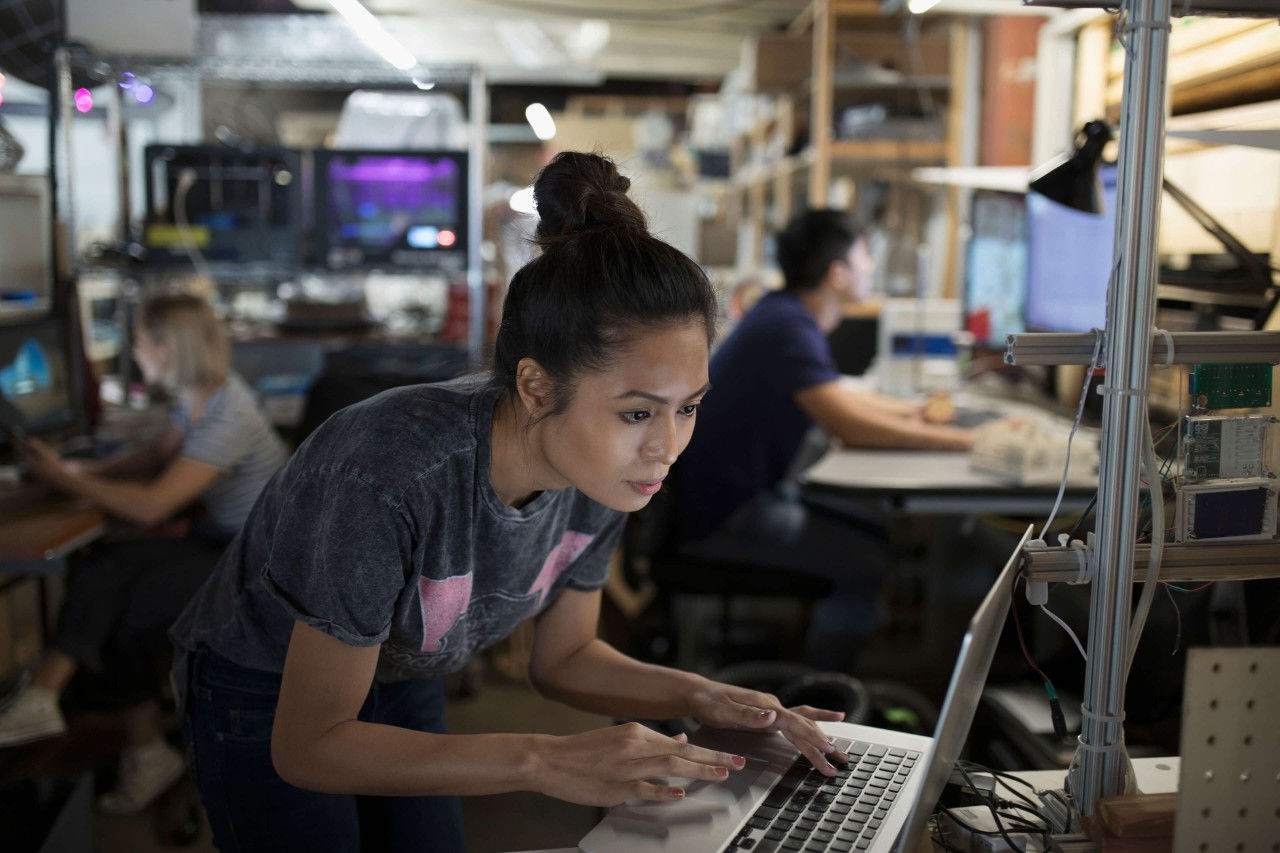
Nalika Nanayakkara
Wealth and Asset management industry leader. Passionate about innovative market solutions as well as helping underserved demographic groups get the financial advice they need.
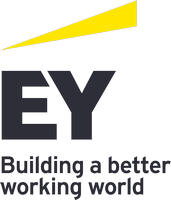
- Connect with us
- Our locations
- Do Not Sell or Share My Personal Information
- Legal and privacy
- Accessibility
- Open Facebook profile
- Open X profile
- Open LinkedIn profile
- Open Youtube profile
EY refers to the global organization, and may refer to one or more, of the member firms of Ernst & Young Global Limited, each of which is a separate legal entity. Ernst & Young Global Limited, a UK company limited by guarantee, does not provide services to clients.
Building a New Foundation with High-Quality Instructional Materials
Passaic public schools and tntp partner to uplevel the curriculum, ensuring every student has access to complex, grade-appropriate, and equity-focused assignments., the challenge, insight + courage + action, an assessment audit uncovers a deeper challenge.
In early 2021, TNTP worked with Passaic Public Schools, a district in northern New Jersey, about 10 miles from New York City, to conduct an assessment audit. District stakeholders worried they were over-testing and that exams were either too easy or difficult. In addition, district leadership wanted to determine whether assessment materials aligned with the New Jersey Student Learning Standards.
The findings uncovered a more foundational issue—the curriculum itself, particularly the instructional materials such as textbooks and worksheets. In many cases, students were being taught from materials that were below grade level or lacking complexity. Since educators weren’t working from high-level texts, classroom instruction was not rigorous enough.
High-quality instructional materials are fundamental for student achievement, one of the four criteria TNTP’s The Opportunity Myth deems essential to a successful educational experience.
Swift , Thorough Action to Reimagine the Curriculum
Passaic leadership immediately extended its partnership with TNTP to conduct a complete curricular audit and classroom-level diagnostic, released in the fall of 2021. The findings showed that only 45% of the students’ assignments were grade appropriate. The lack of high-quality materials was also impacting the other three pillars of high-quality instruction: a mere 23% of lessons were rooted in strong instruction, in which students were asked to do intellectually heavy lifting. Only 50% of students were deeply engaged in the content, while only 37% of students had teachers with high expectations for them. Yet, the review also uncovered a promising fact: when students were given the chance to engage in standards-aligned grade-appropriate assignments, they rose to the occasion, and the percentage of students able to meet the target standards doubled.
Instructional staff understood that access to high-quality instructional materials is, at its heart, an equity issue. The Opportunity Myth shows that classrooms that serve predominantly students from higher-income backgrounds spent twice as much time on grade-appropriate assignments than did classrooms with students from low-income backgrounds, and this seemed to be playing out in Passaic, where 86% of the district’s 15,000 students qualified for free or reduced lunch. Educators were determined to interrupt the opportunity gap.
A Materials Review That Centers Equity for Multi l ingual Learners
Harnessing TNTP’s Curriculum Implementation Framework, Passaic leaders began by convening a task force to design a vision for standout ELA and math education that would guide the selection of new instructional materials. Then, content specialists began reviewing materials for ELA for grades K through 8 and math for grades 3 through 12.
Central to Passaic’s efforts was an unwavering commitment to finding vendors that offered the same curriculum in both English and Spanish, since 93% of Passaic’s families identify as Latinx. Leaders also wanted to ensure continuity across schools, since students often move between schools within the district.
Finding dual-language materials proved more challenging than expected. Content-area specialists and bilingual supervisors examined numerous options, discovering that because of inequities in the markets, materials that were offered in both English and Spanish often didn’t meet the standards that define high-quality instructional materials; conversely, high quality materials often were only offered in English.
Yet Passaic leaders weren’t willing to compromise on the materials’ quality, nor on offering them in both Spanish and English. Leaders also felt it was critical from an equity standpoint that all students in a grade learn same curriculum, so purchasing high quality materials in English and different ones in Spanish also was not an option.
Next, Passaic worked with TNTP to assemble a diverse group of stakeholders to conduct an in-depth review of the few materials that met the criteria. They assessed whether the materials were rich in content and driven by learning science, and examined whether the texts were aligned with college- and career-ready standards. They also looked closely at whether they were culturally responsive and equity centered. The task force also reviewed performance reports on the materials that other organizations had conducted and invited publishers to give presentations.
TNTP worked with Passaic to establish criteria on which to assess the materials and determine which options best meet their needs. The group held discussions and ultimately used the criteria to vote, selecting Amplify CKLA and Amplify Caminos as the ELA and SLA vendor, and Carnegie Learning and Illustrative Math for the math curricula.
Once these key decisions were made, with support from TNTP, the district immediately began creating their strategic implementation plan. From setting clear goals for implementation in alignment with their new content visions, to creating yearlong professional learning plans for key stakeholders including school leaders, coaches, and teachers, to aligning the district’s teacher development strategy to its new instructional priorities, to implementing a progress monitoring approach, each layer of the plan was strategic and intended to build the investment and skills for instructional staff at all levels that would be required to achieve their desired results.
High Quality Materials and Students Meeting Challenges
Passaic began implementing the new curriculum in the 2022-2023 school year, and early figures showed immense promise. The percentage of in-class lessons using grade-level content jumped from just 47% in October 2021 to 90% in November 2022, while the percentage of assignments with grade-level content increased from 45% to 79%. The new curriculum strongly impacted the in-class teaching, with the percentage of lessons with strong instruction jumping from 19% in October 2021 to 50% in November 2022. More than 80% of teachers feel that the instructional materials benefit their students, and more than half feel students are more engaged with the new curriculum than with the old one.
In addition, leaders were encouraged by the fact that students mastered grade-level standards as soon as they had the chance. In October 2021, students were meeting grade-level standards in only 30% of their work; by April 2023, that figure had climbed to 74%. Bilingual classrooms demonstrated comparable growth to non-bilingual classrooms, with grade-appropriate assignments increasing from 17% to 50% and lessons with strong instruction increasing from 17% to 50%.
Passaic extended its partnership with TNTP to continue rolling out the curriculum and to monitor progress. The district is also partnering with TNTP to refine its vision for bilingual instruction and ensure that teachers are fully leveraging the new materials in support of this vision. Passaic also applied this process to select new materials for its high schools during the 2022-2023 school year, and is now implementing HMH – Into Literature high-quality instructional materials at the high school level.
School leaders praised the breadth of experience TNTP brought to the project, having guided other districts through selecting high quality instructional materials. “Partner with TNTP—that would truthfully and honestly be my first advice [to districts embarking on a similar project]. It’s so helpful to have a thought partner who has done this work in other places,” said Lisa Rowbotham, Passaic’s Director of Elementary and Secondary Education. “You might have colleagues in other districts you can call, but they can’t give you that level of support to say, ‘We’ve worked across the United States, and this is what we’ve experienced.’ It gives you a completely different understanding of this work.”
Related Topics
- Accelerated Learning
- Curriculum and Instruction
- Multilingual Learners
Stay in the Know
Sign up for updates on our latest research, insights, and high-impact work.
" * " indicates required fields
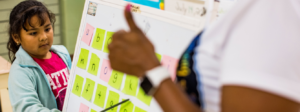
Blog Post | April 1, 2024
Join TNTP in Embracing Multilingualism as a Superpower
As we celebrate Multilingual Advocacy Month, TNTP is deepening our support through research, policy, and opportunities for authentic engagement.
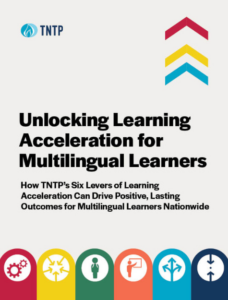
Publication | March 4, 2024
Unlocking Learning Acceleration for Multilingual Learners
Our report, "Unlocking Learning Acceleration for Multilingual Learners," highlights innovative strategies from six districts and key recommendations to supercharge ML success.
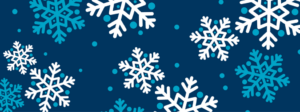
Blog Post | December 13, 2023
TNTP Celebrates Our Most Impactful Year Yet
Reflections from TNTP’s CEO on a year of extraordinary collective impact.
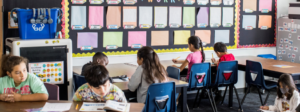
Blog Post | November 16, 2023
Is Your School On-Track with the Science of Reading?
Key questions for school leaders to assess, reflect, and course-correct their schools’ progress towards effective implementation of an evidence-based literacy approach.
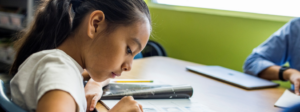
Blog Post | October 3, 2023
What is the Science of Reading, and Why Does it Matter?
Learn why we believe this movement presents a once-in-a-generation opportunity to transform literacy nationwide for all students.
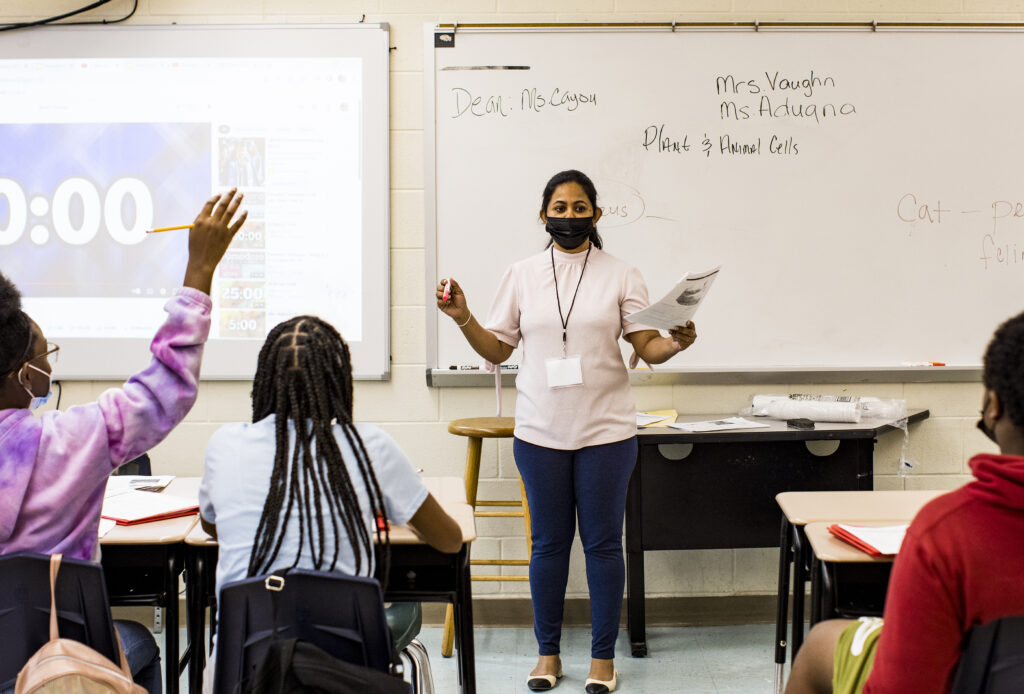
Imali Ariyarathne, seventh-grade teacher at Langston Hughes Academy, introduces her students to the captivating world of science.
TNTP is the nation’s leading research, policy, and consulting organization dedicated to transforming America’s public education system, so that every generation thrives.
Today, we work side-by-side with educators, system leaders, and communities across 39 states and over 6,000 districts nationwide to reach ambitious goals for student success.
Yet the possibilities we imagine push far beyond the walls of school and the education field alone. We are catalyzing a movement across sectors to create multiple pathways for young people to achieve academic, economic, and social mobility.
Saeed Taraky fled Afghanistan. Now he helps refugees/immigrants build new lives in Erie
Taraky succeeds niken astari carpenter, who was the city's first liaison to the new american/immigrant communities. carpenter resigned in may 2023 to explore other opportunities..
Saeedullah Taraky came to Erie less than two years ago during a harrowing time for both his family and the people of his native Afghanistan.
The country’s government began crumbling in August 2021 after its capital city of Kabul was captured by the Taliban, despite efforts of the U.S. and NATO to stabilize the country.
U.S. troops were scheduled to withdraw from Afghanistan within two weeks of the Taliban’s takeover, ending a two-decade war that started after the Sept. 11, 2001 terrorist attacks.
“I was working with the U.S. military back in Afghanistan as an advisor for almost 10 years," said Taraky, 34, who commonly goes by the first name Saeed. He began working for Erie Mayor Joe Schember’s administration in February as the city’s liaison to immigrants, refugees and new Americans.
“We were forced to leave the country after the collapse. We finally came to Erie in September 2022 and it was a shock for me,” Taraky said. “It’s not easy to leave your country, your family, your friends and loved ones.
“But we feel welcome in Erie,” Taraky said. “I’m looking forward to this new opportunity.”
Taraky succeeds Niken Astari Carpenter, the city’s first liaison to the new American/immigrant communities, who resigned in May 2023 to explore other opportunities.
Schember created the new position of immigrant and refugee liaison, and appointed Carpenter to the post, when he took office in January 2018, as part of a concerted effort to reach out to Erie’s immigrant and refugee community. The position's annual salary is $45,000, according to the city of Erie's 2024 budget.
Previous coverage: Niken Astari Carpenter, Erie's first new American/immigrant liaison, resigns
Carpenter had served as a judge in her native Indonesia for 12 years before moving to the United States.
Taraky speaks five languages including Urdu (Pakistani); Dari and Pashto (Afghan; Pashto is also spoken in Pakistan and Iran) Hindi; and English.
He is a former case manager for Catholic Charities Counseling & Adoption Services — where he helped new Americans with myriad resettlement issues such as employment, legal status, government benefits and cultural orientation — and Taraky earned a diploma in business administration from the Southern Alberta Institute of Technology in Calgary, Canada.
He also helped launch Erie's first Afghan Community Center, which opened in December in the 4800 block of Peach Street. Taraky is the center's president/CEO.
The Erie Times-News recently sat down with Taraky to discuss his new position and how he plans to approach it. This interview has been edited for length and clarity.
Building relationships
Question: What do you consider crucial in terms of helping people resettle here in Erie, and getting them to trust/accept assistance from local government?
Answer: The immigrants and new American families here need government resources. My job here will be to help the new Americans in different ways. Like civic engagement, connecting immigrants and refugees with the long-term residents of Erie. For example we're working to (showcase) refugees' culture, food and the skills they have in terms of jobs at different exhibitions and events. We will have orientations for the new American community, working with other organizations, to show them what city government is all about and the ways we can help them feel more comfortable in the community. We have a lot of refugees who have much to offer to Erie.
Employment a focus
What other things do you want to focus on in this new position?
Employment would be one. Refugees and new Americans need to work and want to work. We can connect them with different organizations and businesses for employment. There are grants available for refugees and immigrants so they can start a business and I can help them as part of my job to apply for the grants. Immigrants and refugees are already contributing a lot to the economy of Erie. They are bringing new services to Erie, new food, new products. We want that to continue.
The challenges of resettlement
What makes you a good fit for this job?
I myself am a refugee. I know the process of coming to the United States from another country and it's not easy when you leave your country for a different environment and culture. I understand the language barriers and the legal adjustments. Getting employment right away might not be easy. These are some of the the challenges that refugees face and it takes time overcome them. That's what motivates me to help other refugees. I want to help them navigate those challenges.
'Cultural orientations'
Tell me about something else that you're working on.
I'm working with Erie's police department and Erie's fire department to have cultural orientations with the immigrant and refugee communities so they can learn more about what law enforcement is in this country. It is not scary. Law enforcement is helping people here. They can explain that if you're in trouble, you can call 9-1-1. My goal is to have these on a monthly basis and even invite code enforcement or (other) emergency responders.
Welcoming new Americans
If someone asked you why city government needs a liaison to the new American community, what would be your response?
Erie welcomes new immigrants and new refugees. We need new people in the city for employment, people who bring new skills and culture and experience to Erie. We have a lot of refugees in Erie and we need to welcome them and help them be comfortable here.
Contact Kevin Flowers at [email protected] . Follow him on X at @ETNflowers .
- Open access
- Published: 19 April 2024
GbyE: an integrated tool for genome widely association study and genome selection based on genetic by environmental interaction
- Xinrui Liu 1 , 2 ,
- Mingxiu Wang 1 ,
- Jie Qin 1 ,
- Yaxin Liu 1 ,
- Shikai Wang 1 ,
- Shiyu Wu 1 ,
- Ming Zhang 1 ,
- Jincheng Zhong 1 &
- Jiabo Wang 1
BMC Genomics volume 25 , Article number: 386 ( 2024 ) Cite this article
257 Accesses
Metrics details
The growth and development of organism were dependent on the effect of genetic, environment, and their interaction. In recent decades, lots of candidate additive genetic markers and genes had been detected by using genome-widely association study (GWAS). However, restricted to computing power and practical tool, the interactive effect of markers and genes were not revealed clearly. And utilization of these interactive markers is difficult in the breeding and prediction, such as genome selection (GS).
Through the Power-FDR curve, the GbyE algorithm can detect more significant genetic loci at different levels of genetic correlation and heritability, especially at low heritability levels. The additive effect of GbyE exhibits high significance on certain chromosomes, while the interactive effect detects more significant sites on other chromosomes, which were not detected in the first two parts. In prediction accuracy testing, in most cases of heritability and genetic correlation, the majority of prediction accuracy of GbyE is significantly higher than that of the mean method, regardless of whether the rrBLUP model or BGLR model is used for statistics. The GbyE algorithm improves the prediction accuracy of the three Bayesian models BRR, BayesA, and BayesLASSO using information from genetic by environmental interaction (G × E) and increases the prediction accuracy by 9.4%, 9.1%, and 11%, respectively, relative to the Mean value method. The GbyE algorithm is significantly superior to the mean method in the absence of a single environment, regardless of the combination of heritability and genetic correlation, especially in the case of high genetic correlation and heritability.
Conclusions
Therefore, this study constructed a new genotype design model program (GbyE) for GWAS and GS using Kronecker product. which was able to clearly estimate the additive and interactive effects separately. The results showed that GbyE can provide higher statistical power for the GWAS and more prediction accuracy of the GS models. In addition, GbyE gives varying degrees of improvement of prediction accuracy in three Bayesian models (BRR, BayesA, and BayesCpi). Whatever the phenotype were missed in the single environment or multiple environments, the GbyE also makes better prediction for inference population set. This study helps us understand the interactive relationship between genomic and environment in the complex traits. The GbyE source code is available at the GitHub website ( https://github.com/liu-xinrui/GbyE ).
Peer Review reports
Genetic by environmental interaction (G × E) is crucial of explaining individual traits and has gained increasing attention in research. It refers to the influence of genetic factors on susceptibility to environmental factors. In-depth study of G × E contributes to a deeper understanding of the relationship between individual growth, living environment and phenotypes. Genetic factors play a role in most human diseases at the molecular or cellular level, but environmental factors also contribute significantly. Researchers aim to uncover the mechanisms behind complex diseases and quantitative traits by investigating the interactions between organisms and their environment. Common, complex, or rare human diseases are often considered as outcomes resulting from the interplay of genes, environmental factors, and their interactions. Analyzing the joint effects of genes and the environment can provide valuable insights into the underlying pathway mechanisms of diseases. For instance, researchers have successfully identified potential loci associated with asthma risk through G × E interactions [ 1 ], and have explored predisposing factors for challenging-to-treat diseases like cancer [ 2 , 3 ], rhinitis [ 4 ], and depression [ 5 ].
However, two main methods are currently being used by breeders in agricultural production to increase crop yields and livestock productivity [ 6 ]. The first is to develop varieties with relatively low G × E effect to ensure stable production performance in different environments. The second is to use information from different environments to improve the statistical power of genome-wide association study (GWAS) to reveal potential loci of complex traits. The first method requires long-term commitment, while the second method clearly has faster returns. In GWAS, the use of multiple environments or phenotypes for association studies has become increasingly important. This not only improves the statistical power of environmental susceptibility traits[ 7 ], but also allows to detect signaling loci for G × E. There are significant challenges when using multiple environments or phenotypes for GWAS, mainly because most diseases and quantitative traits have numerous associated loci with minimal impact [ 8 ], and thus it is impossible to determine the effect size regulated by environment in these loci. The current detection strategy for G × E is based on complex statistical model, often requiring the use of a large number of samples to detect important signals [ 9 , 10 ]. In GS, breeders can use whole genome marker data to identify and select target strains in the early stages of animal and plant production [ 11 , 12 , 13 ]. Initially, GS models, similar to GWAS models, could only analyze a single environment or phenotype [ 14 ]. To improve the predictive accuracy of the models, higher marker densities are often required, allowing the proportion of genetic variation explained by these markers to be increased, indirectly obtaining higher predictive accuracy. It is worth mentioning that the consideration of G × E and multiple phenotypes in GS models [ 15 ] has been widely studied in different plant and animal breeding [ 16 ]. GS models that allow G × E have been developed [ 17 ] and most of them have modeled and interpreted G × E using structured covariates [ 18 ]. In these studies, most of the GS models provided more predictive accuracy when combined with G × E compared to single environment (or phenotype) analysis. Hence, there is need to develop models that leverage G × E information for GWAS and GS studies.
This study developed a novel genotype-by-environment method based on R, termed GbyE, which leverages the interaction among multiple environments or phenotypes to enhance the association study and prediction performance of environmental susceptibility traits. The method enables the identification of mutation sites that exhibit G × E interactions in specific environments. To evaluate the performance of the method, simulation experiments were conducted using a dataset comprising 282 corn samples. Importantly, this method can be seamlessly integrated into any GWAS and GS analysis.
Materials and methods
Support packages.
The development purpose of GbyE is to apply it to GWAS and GS research, therefore it uses the genome association and prediction integrated tool (GAPIT) [ 19 ], Bayesian Generalized Linear Regression (BGLR) [ 20 ], and Ridge Regression Best Linear Unbiased Prediction (rrBLUP) [ 21 ]package as support packages, where GbyE only provides conversion of interactive formats and file generation. In order to simplify the operation of the GbyE function package, the basic calculation package is attached to this package to support the operation of GbyE, including four function packages GbyE.Simulation.R (Dual environment phenotype simulation based on heritability, genetic correlation, and QTL quantity), GbyE.Calculate.R (For numerical genotype and phenotype data, this package can be used to process interactive genotype files of GbyE), GbyE.Power.FDR.R (Calculate the statistical power and false discovery rate (FDR) of GWAS), and GbyE.Comparison.Pvalue.R (GbyE generates redundant calculations in GWAS calculations, and SNP effect loci with minimal p -values can be filtered by this package).
Samples and sequencing data
In this study, a small volume of data was used for software simulation analysis, which is widely used in testing tasks of software such as GAPIT, TASSEL, and rMPV. The demonstration data comes from 282 inbred lines of maize, including 4 phenotypic data. In any case, there are no missing phenotypes in these data, and this dataset can be obtained from the website of GAPIT ( https://zzlab.net/GAPIT/index.html , accessed on May 1, 2022). Among them, our phenotype data was simulated using a self-made R simulation function, and the Mean and GbyE phenotype files were calculated. Convert this format to HapMap format using PLINK v1.09 and scripts written by oneself.
Simulated traits
Phenotype simulation was performed by modifying the GAPIT.Phenotype.Simulation function in the GAPIT. Based on the input parameter NQTN, the random selected markers’ genotype from whole genome were used to simulate genetic effect in the simulated trait. The genotype effects of these selected QTNs were randomly sampled from a multivariate normal distribution, the correlation value between these normal distribution was used to define the genetic relationship between each environments. The additive heritability ( \({{\text{h}}}_{{\text{g}}}^{2}\) ) was used to scale the relationship between additive genetic variance and phenotype variance. The simulated phenotype conditions in this paper are set as follows: 1) The three levels of \({{\text{h}}}_{{\text{g}}}^{2}\) were set at 0.8, 0.5, and 0.2, representing high ( \({{\text{h}}}_{{\text{h}}}^{2}\) ), median ( \({{\text{h}}}_{{\text{m}}}^{2}\) ) and low ( \({{\text{h}}}_{{\text{l}}}^{2}\) ) heritability; 2) Genetic correlation were set three levels 0.8, 0.5, 0.2 representing high ( \({{\text{R}}}_{{\text{h}}}\) ), medium ( \({{\text{R}}}_{{\text{m}}}\) ) and low ( \({{\text{R}}}_{{\text{l}}}\) ) genetic correlation; 3) 20 pre-set effect loci of QTL. The phenotype values in each environment were simulated together following above parameters.
Genetic by environment interaction model
The pipeline analysis process of GbyE includes three steps: data preprocessing, production converted, Association analysis. Normalize the phenotype data matrix Y of the dual environment and perform GbyE conversion to generate phenotype data in GbyE.Y format. The genotype data format, such as hapmap, vcf, bed and other formats firstly need to be converted into numerical genotype format (homozygotes were coded as 0 or 2, heterozygotes were coded as 1) using software or scripts such as GAPIT, PLINK, etc. The environment (E) matrix is environment index matrix. The G (n × m) originally of genotype matrix was converted as GbyE.GD(2n × 2 m) \(\left[\begin{array}{cc}G& 0\\ G& G\end{array}\right]\) during the Kronecker product, and the Y vector (n × 1) was also converted as the GbyE.Y vector (2n × 1) after normalization. The duplicated data format indicated different environments, genetic effect, and populations. The genomic data we used in the analysis was still retained the whole genome information. The first column of E is the additive effect, which was the average genetic effect among environments. The others columns of E are the interactive effect, which should be less one column than the number of environments. Because it need to avoid the linear dependent in the model. In the GbyE algorithm, we coded the first environment as background as default, that means the genotype in the first environment are 0, the others are 1. Then the Kronecker product of G and environment index matrix was named as GbyE.GD. The interactive effect part of the GbyE.GD matrix in the GWAS and GS were the relative values based on the first environment (Fig. 1 ). The GbyE environmental interaction matrix can be easily obtained by constructing the interaction matrix E (e.g., Eq. 1 ) such that the genotype matrix G is Kronecker-product with the design interaction matrix E (e.g., Eq. 2 ), in which \(\left[\begin{array}{c}G\\ G\end{array}\right]\) matrix is defined as additive effect and \(\left[\begin{array}{c}0\\ G\end{array}\right]\) matrix is defined as interactive effect. \(\left[\begin{array}{cc}G& 0\\ G& G\end{array}\right]\) matrix is called gene by environment interaction matrix, hereinafter referred to as the GbyE matrix. The phenotype file (GbyE.Y) and genotype file (GbyE.GD) after transformation by GbyE will be inputted into the GWAS and GS models and computed as standard phenotype and genotype files.
where G is the matrix of whole genotype and E is the design matrix for exploring interactive effects. GbyE mainly uses the Kronecker product of the genetic matrix (G) and the environmental matrix (E) as the genotype for subsequent GWAS as a way to distinguish between additive and interactive effects.
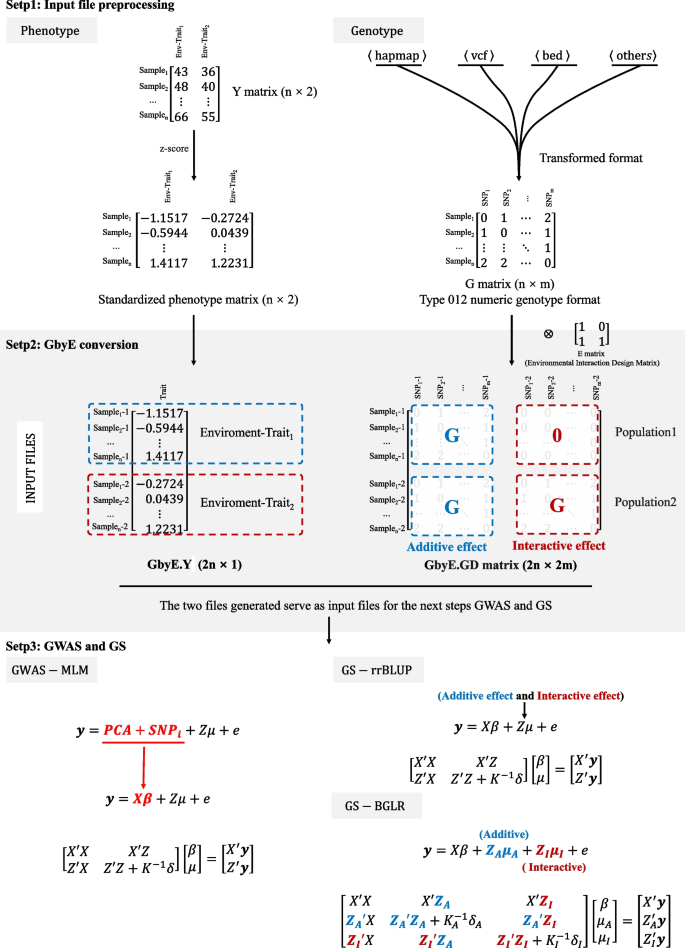
The workflow pipeline of GbyE. The GbyE contains three main steps. (Step 1) Preprocessing of phenotype and genotype data,. The phenotype values in each environment was normalized respectively. Meanwhile, all genotype from HapMap, VCF, BED, and other types were converted to numeric genotype; (Step 2) Generate GbyE phenotype and interactive genotype matrix through the transformation of GbyE. In GbyE.GD matrix, the blue characters indicate additive effect, and red ones indicate interactive effect; (Step 3) The MLM and rrBLUP and BGLR were used to perform GWAS and GS
Association analysis model
The mixed linear model (MLM) of GAPIT is used as the basic model for GWAS analysis, and the principal component analysis (PCA) parameter is set to 3. Then the p -values of detection results are sorted and their power and FDR values are calculated. General expression of MLM (Fig. 1 ):
where Y is the vector of phenotypic measures (2n × 1); PCA and SNP i were defined as fixed effects, with a size of (2n × 2 m); Z is the incidence matrix of random effects; μ is the random effect vector, which follows the normal distribution μ ~ N(0, \({\delta }_{G}^{2}\) K) with mean vector of 0 and variance covariance matrix of \({\delta }_{G}^{2}\) K, where the \({\delta }_{G}^{2}\) is the total genetic variance including additive variance and interactive variance, the K is the kinship matrix built with all genotype including additive genotype and interactive genotype; e is a random error vector, and its elements need not be independent and identically distributed, e ~ N(0, \({\delta }_{e}^{2}\) I), where the \({\delta }_{e}^{2}\) is the residual and environment variance, the I is the design matrix.
Detectivity of GWAS
In the GWAS results, the list of markers following the order of P-values was used to evaluate detectivity of GWAS methods. When all simulated QTNs were detected, the power of the GWAS method was considered as 1 (100%). From the list of markers, following increasing of the criterion of real QTN, the power values will be increasing. The FDR indicates the rate between the wrong criterion of real QTNs and the number of all un-QTNs. The mean of 100 cycles was used to consider as the reference value for statistical power comparison. Here, we used a commonly used method in GWAS research with multiple traits or environmental phenotypes as a comparison[ 22 ]. This method obtains the mean of phenotypic values under different conditions as the phenotypic values for GWAS analysis, called the Mean value method, Compare the calculation results of GbyE with the additive and interactive effects of the mean method to evaluate the detection power of the GbyE strategy. Through the comprehensive analysis of these evaluation indicators, we aim to comprehensively evaluate the statistical power of the GbyE strategy in GWAS and provide a reference for future optimization research.
Among them, the formulae for calculating Power and FDR are as follows:
where \({{\text{n}}}_{{\text{i}}}\) indicates whether the i-th detection is true, true is 1, false is 0; \({{\text{m}}}_{{\text{r}}}\) is the total number of all true QTLs in the sample size; the maximum value of Power is 1.
where \({{\text{N}}}_{{\text{i}}}\) represents the i-th true value detected in the pseudogene, true is 1, false is 0. and cumulative calculation; \({{\text{M}}}_{{\text{f}}}\) is the number of all labeled un-QTNs in the total samples; the maximum value of FDR is 1.
Genomic prediction
To comparison the prediction accuracy of different GS models using GbyE, we performed rrBLUP, Bayesian methods using R packages. All phenotype of reference population and genotype of all population were used to train the model and predict genomic estimated breeding value (gEBV) of all individuals. The correlation between real phenotypes and gEBV of inference population was considered as prediction accuracy. fivefold cross-validation and 100 times repeats was performed to avoid over prediction and reduce bias. In order to distinguish the additive and interactive effects in GbyE, we designed two lists of additive and interactive effects in the "ETA" of BGLR, and put the additive and interactive effects into the model as two kinships for random objects. However, it was not possible to load the gene effects of the two lists in rrBLUP, so the additive and interactive genotypes together were used to calculate whole genetic kinship in rrBLUP (Fig. 1 ). Relevant parameters in BGLR are set as follows: 1) model set to "RRB"; 2) nIter is set to "12000"; 3) burnIn is set to "10000". The results of the above operations are averaged over 100 cycles. We also validated the GbyE method using four other Bayesian methods (BayesA, BayesB, BayesCpi, and Bayesian LASSO) in addition to RRB in BGLR.
Partial missing phentoype in the prediction
In this study, we artificially missed phenotype values in the single and double environments in the whole population from 281 inbred maize datasets. In the missing single environment case, the inference set in the cross-validation was selected from whole population, and each individual in the inference were only missed phenotypes in the one environment. The phenotype in the other environment was kept. The genotypes were always kept. In the case of missing double environments, both phenotypes and genotypes of environment 1 and environment 2 are missing, and the model can only predict phenotypic values in the two missing environments through the effects of other markers. In addition, the data were standardized and unstandardized to assess whether standardization had an effect on the estimation of the model. This experiment was tested using the "ML" method in rrBLUP to ensure the efficiency of the model.
GWAS statistical power of models at different heritabilities and genetic correlations
Power-FDR plots were used to demonstrate the detection efficiency of GbyE at three genetic correlation and three genetic power levels, with a total of nine different scenarios simulated (from left to right for high and low genetic correlation and from top to bottom for high and low genetic power). In order to distinguish whether the effect of improving the detection ability of genome-wide association analysis in GbyE is an additive effect or an effect of environmental interactions, we plotted their Power-FDR curves separately and added the traditional Mean method for comparative analysis. As shown in Fig. 2 , GbyE algorithm can detect more statistically significant genetic loci with lower FDR under any genetic background. However, in the combination with low heritability (Fig. 2 A, B, C), the interactive effect detected more real loci than GbyE under low FDR, but with the continued increase of FDR, GbyE detected more real loci than other groups. Under the combination with high heritability, all groups have high statistical power at low FDR, but with the increase of FDR, the statistical effect of GbyE gradually highlights. From the analysis of heritability combinations at all levels, the effect of heritability on interactive effect is not obvious, but GbyE always maintains the highest statistical power. The average detection power of GWAS in GbyE can be increased by about 20%, and with the decrease of genetic correlation, the effect of GbyE gradually highlights, indicating that the G × E plays a role.
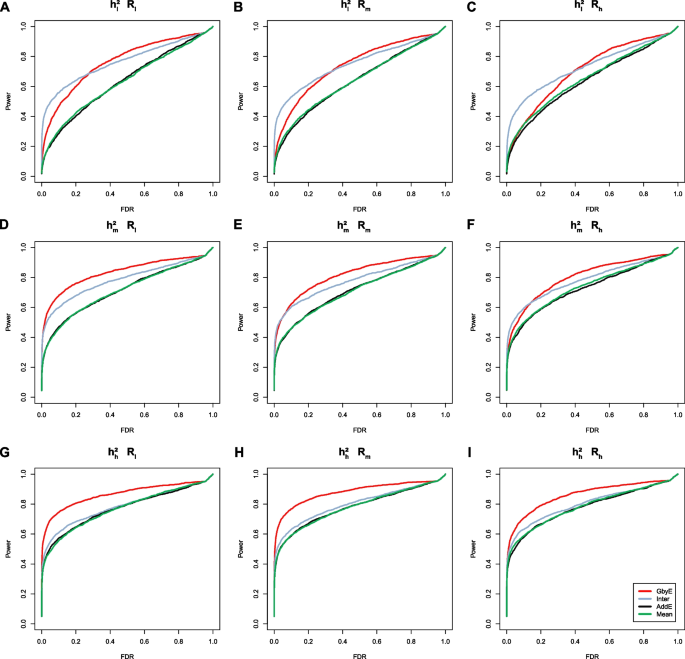
The power-FDR testing in simulated traits. Comparing the efficacy of the GbyE algorithm with the conventional mean method in terms of detection power and FDR. From left to right, the three levels of genetic correlation are indicated in order of low, medium and high. From top to bottom, the three levels of heritability, low, medium and high, are indicated in order. (1) Inter: Interactive section extracted from GbyE; (2) AddE: Additive section extracted from GbyE; (3) \({{\text{h}}}_{{\text{l}}}^{2}\) , \({{\text{h}}}_{{\text{m}}}^{2}\) , \({{\text{h}}}_{{\text{g}}}^{2}\) : Low, medium, high heritability; (4) \({{\text{R}}}_{{\text{l}}}\) , \({{\text{R}}}_{{\text{m}}}\) , \({{\text{R}}}_{{\text{l}}}\) : where R stands for genetic correlation, represents three levels of low, medium and high
Resolution of additive and interactive effect
The output results of GbyE could be understood as resolution of additive and interactive genetic effect. Hence, we created a combined Manhattan plots with Mean result from MLM, additive, and interactive results from GbyE. As shown in Fig. 3 , true marker loci were detected on chromosomes 1, 6 and 9 in Mean, and the same loci were detected on chromosomes 1 and 6 for the additive result in GbyE (the common loci detected jointly by the two results were marked as solid gray lines in the figure). All known pseudo QTNs were labeled with gray dots in the circle. Total 20 pseudo QTNs were simulated in such trait (The heritability is set to 0.9, and the genetic correlation is set to 0.1). Although the additive section in GbyE did not catch the locus on chromosome 9 yet (those p-values of markers did not show above the significance threshold (p-value < 3.23 × 10 –6 )), it has shown high significance relative to other markers of the same chromosome. In the reciprocal effect of GbyE, we detected more significant loci on chromosomes 1, 2, 3 and 10, and these loci were not detected in either of the two previous sections. An integrate QQ plot (Fig. 3 D) shows that the overall statistical power of the additive section in Mean and GbyE are close, nevertheless, the interactive section in the GbyE provided a bit of inflation.
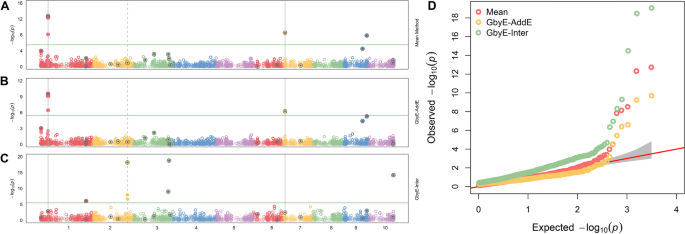
Manhattan statistical comparison plot. Manhattan comparison plots of mean ( A ), additive ( B ) and gene-environment interactive sections ( C ) at a heritability of 0.9 and genetic correlation of 0.1. Different colors are used in the diagram to distinguish between different chromosomes (X-axis). Loci with reinforcing circles and centroids are set up as real QTN loci. Consecutive loci found in both parts are shown as id lines, and loci found separately in the reciprocal effect only are shown as dashed lines. Parallel horizontal lines indicate significance thresholds ( p -value < 3.23 × 10 –6 ). D Quantile–quantile plots of simulated phenotypes for demo data from genome-wide association studies. x-axis indicates expected values of log p -values and y-axis is observed values of log p -values. The diagonal coefficients in red are 1. GbyE-inter is the interactive section in GbyE; GbyE-AddE is the additive section in GbyE
Genomic selection in assumption codistribution
The prediction accuracy of GbyE was significantly higher than the Mean value method by model statistics of rrBLUP in most cases of heritability and genetic correlation (Fig. 4 ). The prediction accuracy of the additive effect was close to that of Mean value method, which was consistent with the situation under the low hereditary. The prediction accuracy of interactive sections in GbyE remains at the same level as in GbyE, and interactive section plays an important role in the model. We observed that in \({{\text{h}}}_{{\text{l}}}^{2}{{\text{R}}}_{{\text{h}}}\) (Fig. 4 C), \({{\text{h}}}_{{\text{m}}}^{2}{{\text{R}}}_{{\text{h}}}\) (Fig. 4 F), \({{\text{h}}}_{{\text{h}}}^{2}{{\text{R}}}_{{\text{l}}}\) (Fig. 4 G), the prediction accuracy of GbyE was slightly higher than the Mean value method, but there was no significant difference overall. In addition, we only observed that the prediction accuracy of GbyE was slightly lower than the Mean value method in \({{\text{h}}}_{{\text{h}}}^{2}{{\text{R}}}_{{\text{l}}}\) (Fig. 4 H), but there was still no significant difference between GbyE and Mean value methods. Under the combination of low heritability and genetic correlation, the prediction accuracy of Mean value method and additive effect model remained at a similar level. However, with the continuous increase of heritability and genetic correlation, the difference in prediction accuracy between the two gradually increases. In summary, the GbyE algorithm can improve the accuracy of GS by capturing information on multiple environment or trait effects under the rrBLUP model.
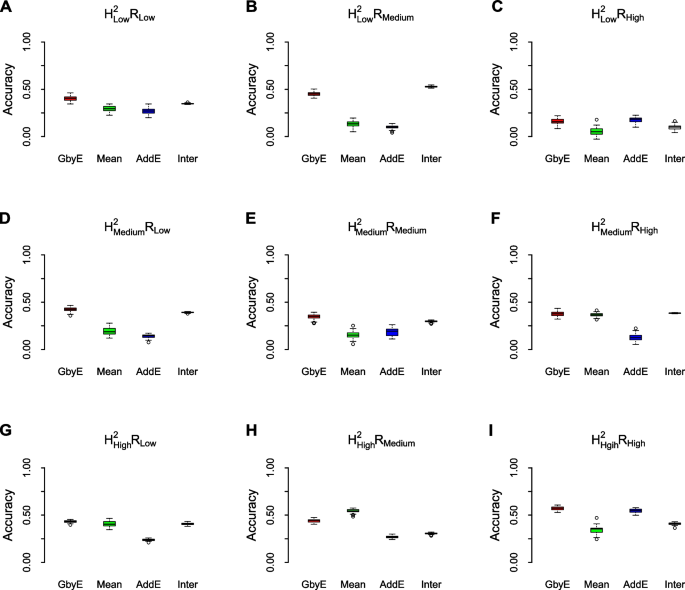
Box-plot of model prediction accuracy. The prediction accuracy (pearson's correlation coefficient) of the GbyE algorithm was compared with the tradition al Mean value method in a simulation experiment of genomic selection under the rrBLUP operating environment. The effect of different levels of heritability and genetic correlation on the prediction accuracy of genomic selection was simulated in this experiment. Each row from top to bottom represents low heritability ( \({{\text{h}}}_{{\text{l}}}^{2}\) ), medium heritability ( \({{\text{h}}}_{{\text{m}}}^{2}\) ) and high heritability ( \({{\text{h}}}_{{\text{h}}}^{2}\) ), respectively; each column from left to right represents low genetic correlation ( \({{\text{R}}}_{{\text{l}}}\) ), medium genetic correlation ( \({{\text{R}}}_{{\text{m}}}\) ) and high genetic correlation ( \({{\text{R}}}_{{\text{h}}}\) ), respectively; The X-axis shows the different test methods and effects, and the Y-axis shows the prediction accuracy
Genomic selection in assumption un-codistribution
The overall performance of GbyE under the 'BRR' statistical model based on the BGLR package remained consistent with rrBLUP, maintaining high predictive accuracy in most cases of heritability and genetic relatedness (Fig. S1 ). However, when the heritability is set to low and medium, the difference between the prediction accuracy of GbyE algorithm and Mean value method gradually decreases with the continuous increase of genetic correlation, and there is no statistically significant difference between the two. The prediction accuracy of the model by GbyE in \({{\text{h}}}_{{\text{h}}}^{2}{{\text{R}}}_{{\text{l}}}\) (Fig. S1 G) and \({{\text{h}}}_{{\text{h}}}^{2}{{\text{R}}}_{{\text{h}}}\) (Fig. S1 I) is significantly higher than that by Mean value method when the heritability is set to be high. On the contrary, when the genetic correlation is set to medium, there is no significant difference between GbyE and Mean value method in improving the prediction accuracy of the model, and the overall mean of GbyE is lower than Mean. When GbyE has relatively high heritability and low genetic correlation, its prediction accuracy is significantly higher than the mean method, such as \({{\text{h}}}_{{\text{m}}}^{2}{{\text{R}}}_{{\text{l}}}\) (Fig. S1 D), \({{\text{h}}}_{{\text{h}}}^{2}{{\text{R}}}_{{\text{l}}}\) (Fig. S1 G), and \({{\text{h}}}_{{\text{h}}}^{2}{{\text{R}}}_{{\text{m}}}\) (Fig. S1 H). Therefore, GbyE is more suitable for situations with high heritability and low genetic correlation.
Adaptability of Bayesian models
Next, we tested a more complex Bayesian model. The GbyE algorithm and Mean value method were combined with five Bayesian algorithms in BGLR for GS analysis, and the computing R script was used for phenotypic simulation test, where heritability and genetic correlation were both set to 0.5. The results indicate that among the three Bayesian models of RRB, BayesA, and BayesLASSO, the predictive accuracy of GbyE is significantly higher than that of Mean value method (Fig. 5 ). In contrast, under the Bayesian models of BayesB and BayesCpi, the prediction accuracy of GbyE is lower than that of the Mean value method. The GbyE algorithm improves the prediction accuracy of the three Bayesian models BRR, BayesA, and BayesLASSO using information from G × E and increases the prediction accuracy by 9.4%, 9.1%, and 11%, respectively, relative to the Mean value method. However, the predictive accuracy of the BayesB model decreased by 11.3%, while the BayescCpi model decreased by 6%.
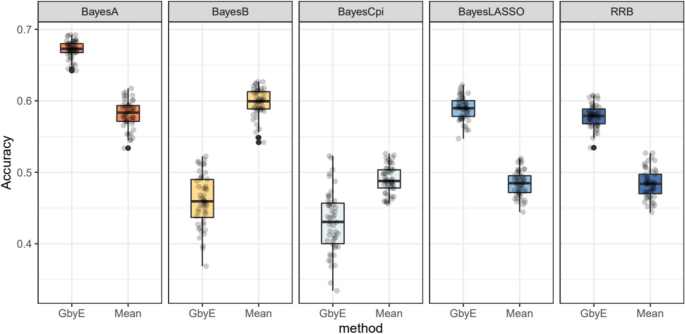
Relative prediction accuracy histogram for different Bayesian models. The X-axis is the Bayesian approach based on BGLR, and the Y-axis is the relative prediction accuracy. Where we normalize the prediction accuracy of Mean (the prediction accuracy is all adjusted to 1); the prediction accuracy of GbyE is the increase or decrease value relative to Mean in the same group of models
Impact of all and partial environmental missing
We tested missing the environmental by using simulated data. In the case of the simulated data, we simulated a total of nine situations with different heritability and genetic correlations (Fig. 6 ) and conducted tests on single and dual environment missing. The improvement in prediction accuracy by the GbyE algorithm was found to be significantly higher than the Mean value method in single environment deletion, regardless of the combination of heritability and genetic correlation. In the case of \({{\text{h}}}_{{\text{h}}}^{2}{{\text{R}}}_{{\text{h}}}\) , the prediction accuracy of GbyE is higher than 0.5, which is the highest value among all simulated combinations. When GbyE estimates the phenotypic values of Environment 1 and Environment 2 separately, its predictive accuracy seems too accurate. On the other hand, when the phenotypic values of both environments are missing on the same genotype, the predictive accuracy of GbyE does not show a significant decrease, and even maintains accuracy comparable to that of a single environment missing. However, when GbyE estimates Environment 1 and Environment 2 separately, the prediction accuracy significantly decreases compared to when a single environment is missing, and the prediction accuracy of Environment 1 and Environment 2 in \({{\text{h}}}_{{\text{l}}}^{2}{{\text{R}}}_{{\text{m}}}\) is extremely low (Fig. 6 B). In addition, the prediction accuracy of GbyE is lower than Mean values only in \({{\text{h}}}_{{\text{l}}}^{2}{{\text{R}}}_{{\text{h}}}\) , whether it is missing in a single or dual environment.
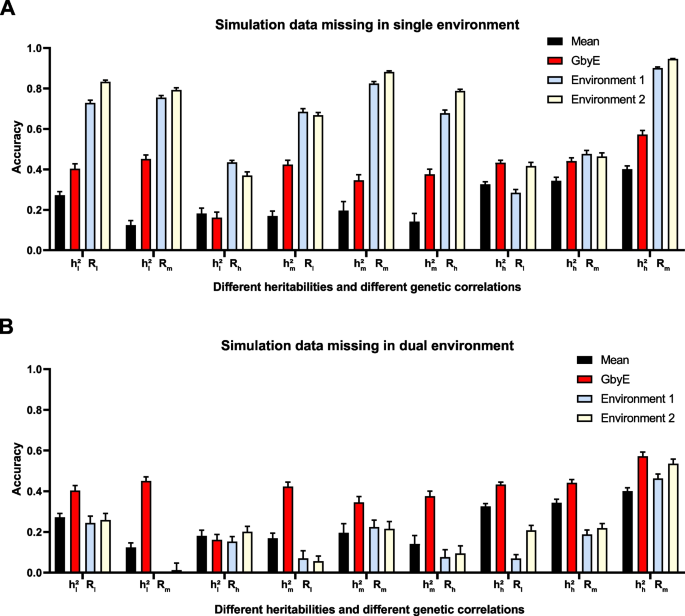
Prediction accuracy of simulated data in single and dual environment absence. The prediction effect of GbyE was divided into two parts, environment 1 and environment 2, to compare the prediction accuracy of GbyE when predicting these two parts separately. This includes simulations with missing phenotypes and genotypes in environment 1 only ( A ) and simulations with missing in both environments ( B ). The horizontal coordinates of the graph indicate the different combinations of heritabilities and genetic correlations of the simulations
The phenotype of organisms is usually controlled by multiple factors, mainly genetic [ 23 ] and environmental factors [ 24 ], and their interactive factors. The phenotype of quantitative traits is often influenced by these three factors [ 25 , 26 ]. However, based on the computing limitation and lack of special tool, the interactive effect always was ignored in most GWAS and GS research, and it is difficult to distinguish additive and interactive effects. The rate between all additive genetic variance and phenotype variance was named as narrow sense heritability. The accuracy square of prediction of additive GS model is considered that can not surpass narrow sense heritability. In this study, the additive effects in GbyE are essentially equivalent to the detectability of traditional models, the key advantage of GbyE is the interactive section. More significant markers with interactive effects were detected. Detecting two genetic effects (additive and interactive sections) in GWAS and GS is a boost to computational complexity, while obtaining genotypes for genetic interactions by Kronecker product is an efficient means. This allows the estimation of additive and interactive genetic effects separately during the analysis, and ultimately the estimated genetic effects for each GbyE genotype (including additive and interactive genetic effect markers) are placed in a t-distribution for p -value calculation, and the significance of each genotype is considered by multiple testing. The GbyE also expanded the estimated heritability as generalized heritability which could be explained as the rate between total genetics variance and phenotype variance.
The genetic correlation among traits in multiple environments is the major immanent cause of GbyE. When the genetic correlation level is high, then additive genetic effects will play primary impact in the total genetic effect, and interactive genetic effects with different traits or environments are often at lower levels [ 27 ]. Therefore, the statistical power of the GbyE algorithm did not improve significantly compared with the traditional method (Mean value) when simulating high levels of genetic correlation. On the contrary, in the case of low levels of genetic correlation, the genetic variance of additive effects is relatively low and the genetic variance of interactive effects is major. At this time, GbyE utilizes multiple environments or traits to highlight the statistical power. Since the GbyE algorithm obtains additive, environmental, and interactive information by encoding numerical genotypes, it only increases the volume of SNP data and can be applied to any traditional GWAS association statistical model. However, this may slightly increase the correlation operation time of the GWAS model, but compared to other multi environment or trait models [ 28 , 29 ], GbyE only needs to perform a complete traditional GWAS once to obtain the results.
In GS, rrBLUP algorithm is a linear mixed model-based prediction method that assumes all markers provide genetic effects and their values following a normal distribution [ 30 ]. In contrast, the BGLR model is a linear mixed model, which assumes that gene effects are randomly drawn from a multivariate normal distribution and genotype effects are randomly drawn from a multivariate Gaussian process, which takes into account potential pleiotropy and polygenic effects and allows inferring the effects of single gene while estimating genomic values [ 31 ]. The algorithm typically uses Markov Chain Monte Carlo methods for estimation of the ratio between genetic variances and residual variances [ 32 , 33 ]. The model has been able to take into account more biological features and complexity, and therefore the overall improvement of the GbyE algorithm under BGLR is smaller than Mean method. In addition, the length of the Markov chain set on the BGLR package is often above 20,000 to obtain stable parameters and to undergo longer iterations to make the chain stable [ 34 ]. GbyE is effective in improving the statistical power of the model under most Bayesian statistical models. In the case of the phenotypes we simulated, more iterations cannot be provided for the BayesB and BayesCpi models because of the limitation of computation time, which causes low prediction accuracy. It is worth noting that the prediction accuracy of BayesCpi may also be influenced by the number of QTLs [ 35 ], and the prediction accuracy of BayesB is often related to the distribution of different allele frequencies (from rare to common variants) at random loci [ 36 ].
The overall statistical power of GbyE was significantly higher in missing single environment than in missing double environment, because in the case of missing single environment, GbyE can take full advantage of the information from the phenotype in the second environment. And the correlation between two environments can also affect the detectability of the GbyE algorithm in different ways. On the one hand, a high correlation between two environments can improve the predictive accuracy of the GbyE algorithm by using the information from one environment to predict the breeding values in the other environment, even if there is only few relationship with that environment [ 37 , 38 ]. On the other hand, when two environments are extremely uncorrelated, GbyE algorithm trained in one environment may not export well to another environment, which may lead to a decrease in prediction accuracy [ 39 ]. In the testing, we found that when the GbyE algorithm uses a GS model trained in one environment and tested in another environment, the high correlation between environments may result to the model capturing similarities between environments unrelated to G × E information [ 40 ]. However, when estimating the breeding values for each environment separately, GbyE still made effective predictions using the genotypes in that environment and maintained high prediction accuracy. As expected, the additive effect calculates the average genetic effect between environments, and its predictive effect does not differ much from the mean method. The interactive effect, however, has one less column than the number of environments, and it calculates the relative values between environments, a component that has a direct impact on the predictive effect. The correlation between the two environments may have both positive and negative effects on the detectability of the GbyE, so it is important to carefully consider the relationship between the two environments in subsequent in development and testing.
A key advantage of the GbyE algorithm is that it can be applied to almost all current genome-wide association and prediction. However, the focus of GbyE is still on estimating additive and interactive effects separately, so that it is easy to determine which portion of the is playing a role in the computational estimation.. The GbyE algorithm may have implications for the design of future GS studies. For example, the model could be used to identify the best environments or traits to include in GS studies in order to maximize prediction accuracy. It is particularly important to test the model on large datasets with different genetic backgrounds and environmental conditions to ensure that it can accurately predict genome-wide effects in a variety of contexts.
GbyE can simulate the effects of gene-environment interactions by building genotype files for multiple environments or multiple traits, normalizing the effects of multiple environments and multiple traits on marker effects. It also enables higher statistical power and prediction accuracy for GWAS and GS. The additive and interactive effects of genes under genetic roles could be revealed clearly, which makes it possible to utilize environmental information to improve the statistical power and prediction accuracy of traditional models, thus helping us to better understand the interactions between genes and the environment.
Availability of data and materials
The GbyE source code, demo script, and demo data are freely available on the GitHub website ( https://github.com/liu-xinrui/GbyE ).
Abbreviations
- Genome-widely association study
Genome selection
Genetic by environmental interaction
Genome association and prediction integrated tool
Mixed linear model
Bayesian generalized linear regression
Ridge regression best linear unbiased prediction
False discovery rate
Principal component analysis
Genomic estimated breeding value
Maazi H, Hartiala JA, Suzuki Y, Crow AL, Shafiei Jahani P, Lam J, Patel N, Rigas D, Han Y, Huang P. A GWAS approach identifies Dapp1 as a determinant of air pollution-induced airway hyperreactivity. PLoS Genet. 2019;15(12):e1008528.
Article PubMed PubMed Central Google Scholar
Simonds NI, Ghazarian AA, Pimentel CB, Schully SD, Ellison GL, Gillanders EM, Mechanic LE. Review of the gene-environment interaction literature in cancer: what do we know? Genet Epidemiol. 2016;40(5):356–65.
Wang X, Chen H, Kapoor PM, Su Y-R, Bolla MK, Dennis J, Dunning AM, Lush M, Wang Q, Michailidou K. A Genome-Wide Gene-Based Gene-Environment Interaction Study of Breast Cancer in More than 90,000 Women. Cancer research communications. 2022;2(4):211–9.
Article CAS PubMed PubMed Central Google Scholar
Chen R-X, Dai M-D, Zhang Q-Z, Lu M-P, Wang M-L, Yin M, Zhu X-J, Wu Z-F, Zhang Z-D, Cheng L. TLR Signaling Pathway Gene Polymorphisms, Gene-Gene and Gene-Environment Interactions in Allergic Rhinitis. Journal of Inflammation Research. 2022;15:3613–30.
Zhao M-Z, Song X-S, Ma J-S. Gene× environment interaction in major depressive disorder. World Journal of Clinical Cases. 2021;9(31):9368.
Falconer DS. The problem of environment and selection. Am Nat. 1952;86(830):293–8.
Article Google Scholar
Kim J, Zhang Y, Pan W. Powerful and adaptive testing for multi-trait and multi-SNP associations with GWAS and sequencing data. Genetics. 2016;203(2):715–31.
Visscher PM, Wray NR, Zhang Q, Sklar P, McCarthy MI, Brown MA, Yang J. 10 years of GWAS discovery: biology, function, and translation. The American Journal of Human Genetics. 2017;101(1):5–22.
Article CAS PubMed Google Scholar
van Os J, Rutten BP. Gene-environment-wide interaction studies in psychiatry. Am J Psychiatry. 2009;166(9):964–6.
Article PubMed Google Scholar
Winham SJ, Biernacka JM. Gene–environment interactions in genome-wide association studies: current approaches and new directions. Journal of Child Psychology Psychiatry. 2013;54(10):1120–34.
Windhausen VS, Atlin GN, Hickey JM, Crossa J, Jannink J-L, Sorrells ME, Raman B, Cairns JE, Tarekegne A, Semagn K. Effectiveness of genomic prediction of maize hybrid performance in different breeding populations and environments. G3: Genes|Genomes|Genetics. 2012;2(11):1427–36.
Xu S, Zhu D, Zhang Q. Predicting hybrid performance in rice using genomic best linear unbiased prediction. Proc Natl Acad Sci. 2014;111(34):12456–61.
Zhao Y, Mette M, Gowda M, Longin C, Reif J. Bridging the gap between marker-assisted and genomic selection of heading time and plant height in hybrid wheat. Heredity. 2014;112(6):638–45.
Crossa J, Perez P, Hickey J, Burgueno J, Ornella L, Cerón-Rojas J, Zhang X, Dreisigacker S, Babu R, Li Y. Genomic prediction in CIMMYT maize and wheat breeding programs. Heredity. 2014;112(1):48–60.
Crossa J, Pérez-Rodríguez P, Cuevas J, Montesinos-López O, Jarquín D, De Los CG, Burgueño J, González-Camacho JM, Pérez-Elizalde S, Beyene Y. Genomic selection in plant breeding: methods, models, and perspectives. Trends Plant Sci. 2017;22(11):961–75.
Roorkiwal M, Jarquin D, Singh MK, Gaur PM, Bharadwaj C, Rathore A, Howard R, Srinivasan S, Jain A, Garg V. Genomic-enabled prediction models using multi-environment trials to estimate the effect of genotype× environment interaction on prediction accuracy in chickpea. Sci Rep. 2018;8(1):11701.
Burgueño J, de los Campos G, Weigel K, Crossa J. Genomic prediction of breeding values when modeling genotype× environment interaction using pedigree and dense molecular markers. Crop Science. 2012;52(2):707–19.
Jarquín D, Crossa J, Lacaze X, Du Cheyron P, Daucourt J, Lorgeou J, Piraux F, Guerreiro L, Pérez P, Calus M. A reaction norm model for genomic selection using high-dimensional genomic and environmental data. Theoretical applied genetics. 2014;127:595–607.
Wang JB, Zhang ZW. GAPIT Version 3: boosting power and accuracy for genomic association and prediction. Genomics Proteomics Bioinformatics. 2021;19(4):629–40.
Pérez P, de Los CG. Genome-wide regression and prediction with the BGLR statistical package. Genetics. 2014;198(2):483–95.
Endelman JB. Ridge Regression and other kernels for genomic selection with R package rrBLUP. Plant Genome J. 2011;4:250–5.
Turley P, Walters RK, Maghzian O, Okbay A, Lee JJ, Fontana MA, Nguyen-Viet TA, Wedow R, Zacher M. Furlotte NAJNg. Multi-trait analysis of genome-wide association summary statistics using MTAG. 2018;50(2):229–37.
CAS Google Scholar
Falconer DS. Introduction to quantitative genetics. Pearson Education India; 1996.
Google Scholar
Lynch M, Walsh B. Genetics and analysis of quantitative traits, vol. 1: Sinauer Sunderland, MA. 1998.
Mackay TF. The genetic architecture of quantitative traits. Annu Rev Genet. 2001;35(1):303–39.
Visscher PM, Hill WG, Wray NR. Heritability in the genomics era—concepts and misconceptions. Nat Rev Genet. 2008;9(4):255–66.
Van der Sluis S, Posthuma D, Dolan CV. TATES: efficient multivariate genotype-phenotype analysis for genome-wide association studies. PLoS Genet. 2013;9(1):e1003235.
O’Reilly PF, Hoggart CJ, Pomyen Y, Calboli FC, Elliott P, Jarvelin M-R, Coin LJ. MultiPhen: joint model of multiple phenotypes can increase discovery in GWAS. PLoS ONE. 2012;7(5):e34861.
Chung J, Jun GR, Dupuis J, Farrer LA. Comparison of methods for multivariate gene-based association tests for complex diseases using common variants. Eur J Hum Genet. 2019;27(5):811–23.
Pérez-Rodríguez P, Gianola D, González-Camacho JM, Crossa J, Manès Y, Dreisigacker S. Comparison between linear and non-parametric regression models for genome-enabled prediction in wheat. G3: Genes|Genomes|Genetics. 2012;2(12):1595–16605.
VanRaden PM. Efficient methods to compute genomic predictions. J Dairy Sci. 2008;91(11):4414–23.
Meuwissen TH, Hayes BJ, Goddard M. Prediction of total genetic value using genome-wide dense marker maps. Genetics. 2001;157(4):1819–29.
de Los CG, Hickey JM, Pong-Wong R, Daetwyler HD, Calus MP. Whole-genome regression and prediction methods applied to plant and animal breeding. Genetics. 2013;193(2):327–45.
Andrieu C, De Freitas N, Doucet A, Jordan MI. An introduction to MCMC for machine learning. Mach Learn. 2003;50:5–43.
Daetwyler HD, Pong-Wong R, Villanueva B, Woolliams JA. The impact of genetic architecture on genome-wide evaluation methods. Genetics. 2010;185(3):1021–31.
Clark SA, Hickey JM, Van der Werf JH. Different models of genetic variation and their effect on genomic evaluation. Genet Sel Evol. 2011;43(1):1–9.
Yang J, Benyamin B, McEvoy BP, Gordon S, Henders AK, Nyholt DR, Madden PA, Heath AC, Martin NG, Montgomery GW. Common SNPs explain a large proportion of the heritability for human height. Nat Genet. 2010;42(7):565–9.
González-Recio O, Forni S. Genome-wide prediction of discrete traits using Bayesian regressions and machine learning. Genet Sel Evol. 2011;43:1–12.
Korte A, Farlow A. The advantages and limitations of trait analysis with GWAS: a review. Plant Methods. 2013;9(1):1–9.
Gauderman WJ. Sample size requirements for matched case-control studies of gene–environment interaction. Stat Med. 2002;21(1):35–50.
Download references
Acknowledgements
Thank you to all colleagues in the laboratory for their continuous help.
This project was partially funded by the National Key Research and Development Project of China, China (2022YFD1601601), the Heilongjiang Province Key Research and Development Project, China (2022ZX02B09), the Qinghai Science and Technology Program, China (2022-NK-110), Sichuan Science and Technology Program, China (Award #s 2021YJ0269 and 2021YJ0266), the Program of Chinese National Beef Cattle and Yak Industrial Technology System, China (Award #s CARS-37), and Fundamental Research Funds for the Central Universities, China (Southwest Minzu University, Award #s ZYN2023097).
Author information
Authors and affiliations.
Key Laboratory of Qinghai-Tibetan Plateau Animal Genetic Resource Reservation and Utilization, Sichuan Province and Ministry of Education, Southwest Minzu University, Chengdu, 6110041, China
Xinrui Liu, Mingxiu Wang, Jie Qin, Yaxin Liu, Shikai Wang, Shiyu Wu, Ming Zhang, Jincheng Zhong & Jiabo Wang
Nanchong Academy of Agricultural Sciences, Nanchong, 637000, China
You can also search for this author in PubMed Google Scholar
Contributions
JW and XL conceived and designed the project. XL managed the entire trial, conducted software code development, software testing, and visualization. MW, JQ, YL, SW, MZ and SW helped with data collection and analysis. JQ, and YL assisted with laboratory analyses. JW, and XL had primary responsibility for the content in the final manuscript. JZ supervised the research. JW designed software and project methodology. All authors approved the final manuscript. All authors have reviewed the manuscript.
Corresponding author
Correspondence to Jiabo Wang .
Ethics declarations
Ethics approval and consent to participate.
Not applicable.
Consent for publication
Competing interests.
The authors have declared no competing interests.
Additional information
Publisher’s note.
Springer Nature remains neutral with regard to jurisdictional claims in published maps and institutional affiliations.
Supplementary Information
Supplementary material 1., rights and permissions.
Open Access This article is licensed under a Creative Commons Attribution 4.0 International License, which permits use, sharing, adaptation, distribution and reproduction in any medium or format, as long as you give appropriate credit to the original author(s) and the source, provide a link to the Creative Commons licence, and indicate if changes were made. The images or other third party material in this article are included in the article's Creative Commons licence, unless indicated otherwise in a credit line to the material. If material is not included in the article's Creative Commons licence and your intended use is not permitted by statutory regulation or exceeds the permitted use, you will need to obtain permission directly from the copyright holder. To view a copy of this licence, visit http://creativecommons.org/licenses/by/4.0/ . The Creative Commons Public Domain Dedication waiver ( http://creativecommons.org/publicdomain/zero/1.0/ ) applies to the data made available in this article, unless otherwise stated in a credit line to the data.
Reprints and permissions
About this article
Cite this article.
Liu, X., Wang, M., Qin, J. et al. GbyE: an integrated tool for genome widely association study and genome selection based on genetic by environmental interaction. BMC Genomics 25 , 386 (2024). https://doi.org/10.1186/s12864-024-10310-5
Download citation
Received : 27 December 2023
Accepted : 15 April 2024
Published : 19 April 2024
DOI : https://doi.org/10.1186/s12864-024-10310-5
Share this article
Anyone you share the following link with will be able to read this content:
Sorry, a shareable link is not currently available for this article.
Provided by the Springer Nature SharedIt content-sharing initiative
- Genomic selection
BMC Genomics
ISSN: 1471-2164
- Submission enquiries: [email protected]
- General enquiries: [email protected]
Firefighter hurt, building destroyed in massive Corbin fire
CORBIN, Ky. (WKYT)—A firefighter was hurt, and a building was destroyed in a major fire that took multiple departments to put out Monday afternoon.
It happened on the Cumberland Gap Parkway, just off Interstate 75′s Exit 29, which is a busy place. The big fire captured the attention of many on Monday.
“As I came this way, it kept getting blacker and bigger. It was some sight definitely,” said Deena Cox, who feared something exploded from all the thick black rolling smoke and flames.
Multiple fire departments were called to help fight the fire and police were also called.
“We were actually called out early on to help traffic control. And then when we found out some homeless were possibly staying there, we were called to a different capacity,” said Gilbert Acciardo with the Laurel County Sheriff’s Office.
The person in question was located Tuesday morning. One firefighter was hurt.
“I am grateful we have responders that respond so quickly. Here so fast. It could have gotten worse with it being behind a gas station,” said Cox.
Captain Eric Philpot of the Corbin Fire Department is the injured firefighter. He was hurt when debris fell on him, but he is expected to make a full recovery.
They say he was airlifted to the University of Kentucky hospital only for precautionary reasons, and he was released late Monday night.
The cause of the fire is under investigation by the ATF and the state fire marshal.
The fire destroyed a vacant building that was once used as a garage for semi trucks. It was once part of a truck stop that closed years ago, but the area is seeing a bit of a resurgence now with a lot of construction taking place.
Copyright 2024 WKYT. All rights reserved.

One person killed when tires came off semi on I-75

A decade after gruesome Bardstown deaths, family gives new details in the Netherland case

Police provide update in death of Grammy-winning musician Mandisa

Police say 10-year-old boy awoke to find his parents and 3 brothers shot to death

Lexington family determined to solve 2018 murder case
Latest news.

Lexington mother accused of trying to kill her 7-month-old

WATCH | Jim Caldwell's FastCast

Jim Caldwell's Forecast | A couple of cooler ones before the big surge

WATCH | Kids Art Day: Earth Day special at Crank & Boom Craft Ice Cream

LexTran to vote on new budget, including proposed funding for microtransit study
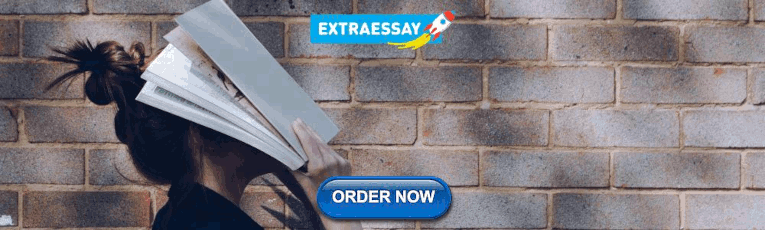
IMAGES
VIDEO
COMMENTS
Case Study Cards. Download our case study cards, get your team together, and explore ideas for strengthening your relationships by following one of the activities suggested in the cards. The cards are designed for use in workshops, meetings or sessions. We recommend printing the cards so that people can read them, move them and stick them up on ...
Welcome to our Case Study Collection: inspiring examples of charities, schools, businesses, governments and more who are putting relationships at the heart of what they do, and reaping the rewards. By providing an easy way to find practical examples of good relational practice, the collection serves as a useful jumping-off point for thinking ...
Rebuilding Relationships Across Teams in a Hybrid Workplace. by. Ron Carucci. November 10, 2021. Illustration by Vickie Sieczka. Summary. The coming year of inventing our way toward whatever our ...
Kushal Choksi was a successful Wall Street quant who had just entered the doors of the second twin tower on 9/11 when it got hit. As Choksi describes in his best-selling book, On a Wing and a ...
The contexts are diverse, as they are here in the Case Maker, but the driving principles are consistent. On the Relationships Map we have begun to plot the organisations and individuals who are putting relationships first. The Map is more than a directory. It's a meeting place; a space for finding one another and sharing ideas and resources.
Relationship building. As a mentor, being open and receptive to what your students are saying — even when they're frustrated — can help strengthen your relationship because it shows that you trust them and value their opinions. This mentor maintains a professional approach by showing compassion for the student's frustration without ...
Our case-based study found that the mentoring and advice ties existed in a symbiotic relationship with the scientific productivity network where the practices of the team were simultaneously ...
Over the next month, I'll be sharing three more case studies on growth and success. Here's a preview …. 2. Write [bleeping] amazing content. 3. Be a decent freakin' person. 4. Go after it … again, and again, and again. .
It is a composite of my clients' experiences, and it embeds five keys to finding and deepening a long-term relationship. After, I call those out. Hi, this is Jeremy again. There are all those ...
People who seem like they're paying attention often aren't—even when they're smiling and nodding toward the speaker. Research by Alison Wood Brooks, Hanne Collins, and colleagues reveals just how prone the mind is to wandering, and sheds light on ways to stay tuned in to the conversation. 03 Oct 2023. Research Event.
Emphasis is on the unfolding of the client-therapist interaction and the development of the therapeutic relationship in their case study, rather than on discrete relationship variables (e.g., empathy, alliance, self-disclosure) or on schools of psychotherapy. In examining the process of change in the case studies, the authors of each chapter ...
Our case study demonstrates that relationship building is the key f oundation to successful extension. Yet even the most pervasive and basic models of social and adult learning do not
You want to create a positive feeling of achievement and teamwork so that everyone feels great and eager to work with you in the future. 8. Demonstrate passion and empathy. When engaging with them, project managers can build healthy stakeholder relationships by displaying a high degree of passion, empathy, and honesty.
Building Strategic Relationships. Wednesday, March 10, 2021. 10:30 AM - 2:00 PM EST. Speaker (s): Gord Arbeau, Associate Vice-President Advancement and External Relations, Brock University, and Liesl Elder, Chief Development Officer, University of Oxford. Topical Key Concepts. Concepts covered for understanding of a topic/mastery of a competency.
Originality/value. The case study presents an analysis of the use of technology integration into the planning processes that were undertaken by a group of members to enhance informal gatherings for relationship building within a global community of members during the COVID-19 pandemic.
Dimension: Building trust and cultivating partnership relationships Indicator Case Study Activities researchers and practitioners routinely work together. The partners in this case study worked together from December 2018 to April 2020. Before the partnership began, the researcher approached the RP consultants about her interest in
Although case studies have been discussed extensively in the literature, little has been written about the specific steps one may use to conduct case study research effectively (Gagnon, 2010; Hancock & Algozzine, 2016).Baskarada (2014) also emphasized the need to have a succinct guideline that can be practically followed as it is actually tough to execute a case study well in practice.
A case study is one of the most commonly used methodologies of social research. This article attempts to look into the various dimensions of a case study research strategy, the different epistemological strands which determine the particular case study type and approach adopted in the field, discusses the factors which can enhance the effectiveness of a case study research, and the debate ...
New research on customer relationship management from Harvard Business School faculty on issues including ways to increase loyalty, determining and using customer lifetime value calculations, and the effect of using Groupon-type vouchers to promote customer growth. ... Bill George discusses his case study, "Facebook Confronts a Crisis of Trust ...
This study deals with the first two of five sessions. The professional counsellor will be using an integrative approach, incorporating Person Centred and Behavioural Therapy techniques in the first session, moving to a Solution Focused approach in the second session. For ease of writing the Professional Counsellor is abbreviated to "C".
Study 1 tested whether students' compassionate and self-image goals predict a cycle of projected and reciprocal responsiveness between roommates with implications for both people's relationship goals. Study 2 reports previously unpublished data from the Roommate Goals Study (Crocker & Canevello, 2008, Study 2), examining the implications of ...
TRUST, SAFETY, AND RELATIONSHIP BUILDING: A CASE STUDY OF ALTERNATIVE SCHOOL EDUCATION Kermit D. Blakley Ed. D. Educational Leadership Submitted in fulfillment of the requirements of Doctor of Education in the Foster G. McGaw Graduate School National College of Education National Louis University June, 2013
Building An Enduring Relationship - A Case Study. We often write about the importance Fortney & Weygandt, Inc. places on building mutually beneficial relationships with our clients. We firmly believe that loyalty and trust are the foundations of our relationships with our clients. Trust builds confidence and confidence builds repeat customers.
️ Prioritizing relationship building leading up to the event makes a tremendous impact on how successful the event is for your organization ️ Relationships held by members of your Board of Directors can bring new energy to your events and lead to future connections ——————————————
Consulting at EY is building a better working world by realizing business transformation through the power of people, technology and innovation. The nature of work is evolving fast, we're all being asked to adopt new behaviors — to be more innovative, more agile, more collaborative, more everything. Business today is anything but usual.
High Quality Materials and Students Meeting Challenges. Passaic began implementing the new curriculum in the 2022-2023 school year, and early figures showed immense promise. The percentage of in-class lessons using grade-level content jumped from just 47% in October 2021 to 90% in November 2022, while the percentage of assignments with grade ...
The study presents 12 actionable tactics brands and marketers can take to build loyalty with their customers Intuit Inc. (Nasdaq: INTU), the global financial technology platform that makes Intuit TurboTax , Credit Karma , QuickBooks , and Mailchimp , today released a new report, "The Science of Loyalty," focused on the drivers behind consumer behavior and tangible insights that marketers ...
Saeed Taraky fled Afghanistan. Now he helps refugees/immigrants build new lives in Erie Taraky succeeds Niken Astari Carpenter, who was the city's first liaison to the new American/immigrant ...
The growth and development of organism were dependent on the effect of genetic, environment, and their interaction. In recent decades, lots of candidate additive genetic markers and genes had been detected by using genome-widely association study (GWAS). However, restricted to computing power and practical tool, the interactive effect of markers and genes were not revealed clearly.
Fire destroyed a former garage where semi trucks were worked on beside I-75 Exit 29 in Laurel County. (PHIL PENDLETON) CORBIN, Ky. (WKYT)—A firefighter was hurt, and a building was destroyed in ...