AQA GCSE Geography

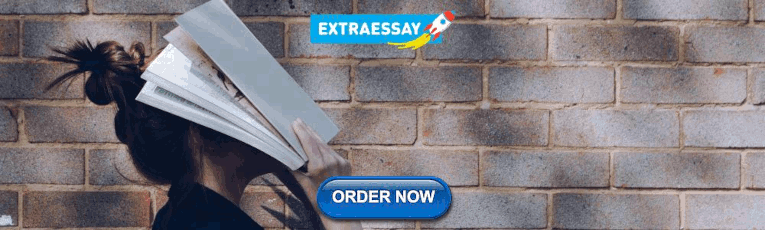
Case Study: River Tees
The River Tees, located in Northern England, flows from its source at Cross Fell in the Pennines travelling 130 km until it reaches its mouth at Teesside, where it meets the North Sea.
With a drainage basin spanning 1,800 km², the River Tees undergoes various processes that shape its course, influenced by both natural and human factors.
Upper Section: Steep-Sided Valleys and High Force Waterfall
In the upper course of the River Tees (near its source), the landscape is characterised by mountains, hills and steep slopes.
There are V-shaped valleys in this region that form as the river descends rapidly through the moorlands. The force of the water erodes the rock, resulting in the formation of steep-sided valleys with a distinctive V-shape. Large rocks within the riverbed contribute to abrasion, which vertically erodes the riverbed.
A notable feature in the upper course is the High Force waterfall. This waterfall is formed by the interaction of a resistant igneous rock called dolerite, locally known as Whin Sill, with a softer rock called limestone.

The hydraulic action erodes the softer rock more rapidly, causing undercutting and leaving the harder rock to overhang. Over time, the unsupported hard rock collapses, causing the waterfall to move upstream and gradually leaving behind a gorge.
Middle Section: Meanders and Flood Plains
As the River Tees enters its middle section, adding tributaries increases its water volume, making the river wider and deeper.
Attrition in the upper section reduces large rocks to smaller pebbles. This reduces friction in the river, allowing it to flow faster. The increased energy from faster flow leads to more hydraulic action.
This process contributes to the formation of meanders, characterised by lateral erosion and the development of a floodplain. These features can be observed around Barnard Castle along the river’s course.
Lower Section: Enlarged Meanders and Levees
In the lower course of the River Tees, the meanders continue to grow, and the floodplain expands further.

This transformation is evident in the areas of Darlington and Yarm. In some locations, meanders may evolve into oxbow lakes.
Another feature present in the lower section is the formation of levees. Levees form when the river overflows during floods and deposits sediment along its banks. Over time, this repeated deposition builds up the levees, which help contain the river within its channel during future flood events.
Estuary: River Tees’ Meeting with the North Sea
Finally, the River Tees reaches its estuary, the point where it flows into the North Sea.
The estuary serves as a transition zone between the freshwater river and the sea’s saltwater, displaying unique ecological characteristics.
You’ve used 10 of your 10 free revision notes for the month
Sign up to get unlimited access to revision notes, quizzes, audio lessons and more.
- Revision Notes
- Unlimited Quizzes
- Audio Content
- Progress Tracking
- No Advertisements

Hi there! Want to study 2x more effectively?
Sign up now for exclusive access to interactive quizzes, audio lessons and more educational tools..
Sign me up!
Last updated: December 27, 2022
Please read these terms and conditions carefully before using our services.
Definitions
For these Terms and Conditions:
- Affiliate means an entity that controls, is controlled by or is under common control with a party, where “control” means ownership of 50% or more of the shares, equity interest or other securities entitled to vote for the election of directors or other managing authority.
- Account means a unique account created for you to access our services or some of our services.
- Country refers to the United Kingdom
- Company refers to Shalom Education Ltd, 86 London Road, (Kingsland Church), Colchester, Essex, CO3 9DW, and may be referred to as ‘we’, ‘us’, ‘our’, or ‘Shalom Education’ in this agreement.
- Device means any device that can access the Service, such as a computer, a mobile phone or a digital tablet.
- Feedback means feedback, innovations or suggestions sent by You regarding the attributes, performance or features of our service.
- Free Trial refers to a limited period of time that may be free when purchasing a subscription.
- Orders mean a request by you to purchase services from us.
- Promotions refer to contests, sweepstakes or other promotions offered by us through the website.
- Services refer to our website, resources and tutoring service.
- Subscriptions refer to the services or access to the service offered on a subscription basis by the company to you.
- Terms and Conditions (also referred to as “Terms”) mean these Terms and Conditions that form the entire agreement between you and Shalom Education Ltd regarding the use of the services we offer.
- Third-party Social Media Service means any services or content (including data, information, products or services) provided by a third party that may be displayed, included or made available on the website.
- Website refers to Shalom-education.com, accessible from https://www.shalom-education.com
- You means the individual accessing or using our services, or the company, or other legal entity on behalf of which such individual is accessing or using our services.
- Tutor refers to an individual who teaches a single pupil or a small group of students who have registered with Shalom Education Ltd.
- Tutee refers to a student or a pupil who has registered for tutoring with Shalom Education Ltd, which is administered through our tutoring platform.
Acknowledgement
Thank you for choosing Shalom Education Tuition for your educational needs. These terms and conditions outline the rules and regulations for the use of our services, and the agreement that will govern your relationship with us.
By accessing or using our services, you accept and agree to be bound by these terms and conditions, and our privacy policy , which describes our policies and procedures for the collection, use, and disclosure of your personal information when you use our website.
It is important that you read both documents carefully before using our services, as they outline your rights and obligations as a user of our services. If you do not agree to these terms and conditions or our privacy policy, please do not use our services. We hope you have a positive and educational experience with Shalom Education Tuition.
Signing up for Tutoring or Membership Accounts
By signing up for tutoring or membership accounts through the website, you confirm that you have the legal ability to enter into a binding contract.
Your information
When you place an order, we may ask you to provide certain information, such as your name, email, phone number, credit card details, and billing address.
You confirm that you have the right to use the payment method you choose, and that the information you provide is accurate and complete. By submitting your information, you give us permission to share it with payment processing third parties to complete your order.
Order cancellation
We reserve the right to cancel your order at any time for various reasons, including but not limited to:
- Unavailability of services (e.g. no tutors available)
- Errors in the description or prices of services
- Errors in your order
- Suspected fraud or illegal activity
Cancelling your order
Any services that you purchase can only be returned in accordance with these terms and conditions. Our Returns Policy forms a part of these Terms and Conditions.
In general, you have the right to cancel your order and receive a full refund within 14 days of placing it. However, you cannot cancel an order for services that are made to your specifications or are clearly personalised, or for services that you have already received in part.
Money-Back Guarantee: If you are not satisfied with the quality of your tutoring session, you may be eligible for a full or partial refund or credit. To request a refund or credit, please contact us within 24 hours after the end of the session and provide a detailed explanation of your dissatisfaction. We will review your request and, if approved, will issue a refund or credit to your account.
- Please note that refunds or credits may not be available for all types of tutoring services and may be subject to fees or other charges. For more information, please contact us.
Errors and inaccuracies
We strive to provide accurate and up-to-date information about the service we offer, but we cannot guarantee that everything will be completely accurate and up-to-date at all times. Prices, product images, descriptions, availability, and services may be inaccurate, incomplete, or out of date.
We reserve the right to change or update any information, and to correct errors, inaccuracies, or omissions at any time without prior notice.
Prices policy
We reserve the right to change our prices at any time before accepting your order.
All tutoring services and membership accounts purchased on our website must be paid for in full at the time of purchase, for the required time of use. We accept a variety of payment methods, including credit cards, debit cards, and online payment services like PayPal.
Your payment card may be subject to validation checks and authorisation by your card issuer. If we do not receive the necessary authorisation, we cannot be held responsible for any delays or failure to deliver your order.
Subscriptions
Subscription period.
Our tutoring services are available with a pay-as-you-go option or a subscription option that is billed on a monthly or annual basis. The tutoring account subscription will end at the end of the period. You can choose the subscription option that best suits your needs and cancel at any time.
Our membership accounts are billed monthly or annually and do not automatically renew after the period. You can choose to renew your membership account at the end of the period if you wish to continue your membership.
Subscription cancellations
You can cancel your subscription renewal through your account settings or by contacting us. Please note that you will not receive a refund for fees you have already paid for your current subscription period, and you will be able to access the service until the end of your current subscription period.
We need accurate and complete billing information from you, including your full name, address, postal code, telephone number, and valid payment method. If automatic billing fails, you will not receive tutoring services until a payment is made. If payment is not made within a reasonable time period, your account may be terminated.
Fee changes
We reserve the right to modify the subscription fees at any time. Any change in fees will take effect at the end of your current subscription period.
We will give you reasonable notice of any fee changes so you have the opportunity to cancel your subscription before the changes take effect. If you continue to use the service after the fee change, you agree to pay the modified amount.
In general, paid subscription fees are non-refundable. However, we may consider certain refund requests on a case-by-case basis and grant them at our discretion.
We may offer free trials of our subscriptions at our discretion. You may be asked to provide billing information to sign up for a free trial. If you do provide billing information, you will not be charged until the free trial period ends.
On the last day of the free trial, unless you have cancelled your subscription, you will be automatically charged the applicable subscription fees for the plan you have chosen. We reserve the right to modify or cancel free trial offers at any time without notice.
From time to time, we may offer promotions through the Service, such as discounts, special offers, or contests. These promotions may be governed by separate rules and regulations.
If you choose to participate in a promotion, please review the applicable rules and our privacy policy carefully. In the event of a conflict between the promotion rules and these terms and conditions, the promotion rules will take precedence.
Please note that any promotion may be modified or discontinued at any time, and we reserve the right to disqualify any participant who violates the rules or engages in fraudulent or dishonest behaviour. By participating in a promotion, you agree to be bound by the applicable rules and our decisions, which are final and binding in all matters related to the promotion.
User Accounts
In order to access certain features of our services, you may be required to create an account. When you create an account, you agree to provide accurate, complete, and current information about yourself as prompted by the account registration process. If you provide any false, inaccurate, outdated, or incomplete information, or if we have reasonable grounds to suspect that you have done so, we reserve the right to suspend or terminate your account.
You are solely responsible for maintaining the confidentiality of your account and password, and you agree to accept responsibility for all activities that occur under your account. If you believe that your account has been compromised or that there has been any unauthorised access to it, you must notify us immediately.
You may not use a username that is already in use by another user, that belongs to another person or entity without appropriate authorisation, or that is offensive, vulgar, or obscene. We reserve the right to remove or reclaim any username that we determine, in our sole discretion, to be inappropriate, infringing, or otherwise in violation of these terms and conditions.
Tutor Agreements
As a tutor working with Shalom Education, you agree to the following terms and conditions:
Tutor relationships
- You are at least eighteen years of age and have the necessary qualifications and/or experience to provide tuition in the subjects specified on your CV.
- You will not make any agreements with a tutee, any member of the tutee’s family, or the tutee’s guardian that are intended to circumvent the relationship between our service and the tutee and to benefit you at our expense. This includes the exchange of private information (e.g. phone numbers, emails or social media accounts etc).
- You will not, during the period of any tutoring session with a tutee and for a period of six months from the conclusion of the last tutoring session, give any tuition services to that tutee.
- You will use our platform as your only means of communication with tutees, and any other methods are strictly prohibited.
- You will not be abusive towards a tutee or their nominee on our website or any other place.
- You are expected to maintain a professional and respectful relationship with tutees at all times.
- You will not engage in any inappropriate or illegal behaviour while working with tutees, including but not limited to harassment or discrimination.
- You are expected to follow all applicable laws and regulations while providing tutoring services.
Tutor responsibilities
- You will not complete any coursework, essays, or other assignments on behalf of the tutee. However, you can offer support.
- You will be punctual and communicate with us if you are running late or need to reschedule a lesson.
- You are expected to provide high-quality tutoring services to tutees, including preparing appropriate lesson plans.
- You will be responsible for ensuring that you have the necessary resources and equipment to provide effective tutoring services, such as a reliable internet connection and computer if tutoring online.
- You will be expected to keep confidential any personal or sensitive information that you may learn about a tutee while working with them.
Tutor compensation
- You will not request or accept any payments from a tutee, or their nominee.
- The company reserves the right to withhold payment to tutors if it believes lessons are being booked outside our platform.
- The company will pay you a fee for your tutoring services according to the rates agreed between you and the company.
- The company will be responsible for collecting payment from the tutee.
- If you are self-employed, we expect you to provide us with your UTR number and you are expected to pay your own tax to HMRC
Tutor termination
- As a tutor working with the company, you may terminate your relationship with a tutee at any time by providing reasonable notice.
- The company may also terminate your relationship with a tutee at any time, for any reason, such as if the tutee is no longer in need of tutoring services or if the tutee expresses dissatisfaction with your services.
- The company may terminate your use of its service if you breach any of the terms and conditions outlined in this agreement.
- The company may also terminate your use of its service if it determines that you are no longer fit to provide tutoring services or if it receives multiple complaints about your performance.
Tutee Agreements
As a tutee using our service, you agree to the following terms and conditions:
- If you are under 18 years of age, you must have consent from a parent or guardian to register and that parent/guardian must enter into an agreement with Shalom Education to provide tuition services.
- You must contact a tutor through the Shalom Education tutoring platform. Any other means of communication is prohibited.
- You agree not to publish any abusive comments about a tutor or another tutee on the Shalom Education website or any other place. This includes defamatory or derogatory comments.
- You must not request a tutor to complete your coursework, essays, or other assignments given to you in your various schools but can request for support towards that.
- You agree not to make any agreement or arrangement with a tutor which is intended to circumvent the relationship between Shalom Education and the Tutor.
Expectations
- Lessons will be held at the frequency and duration agreed upon with us.
- Shalom Education will provide any necessary materials or resources for the lessons.
- You are expected to be punctual for lessons and to provide adequate notice for cancellations or rescheduling.
- You are expected to provide any necessary materials or resources for the lessons (e.g. pens, pencils or a protractor).
Responsibilities
- If you cancel a lesson less than 12 hours before the lesson is due to commence, you may be charged a cancellation fee of 50% of the lesson fee. If you cancel a lesson less than 2 hours before the scheduled start time of a lesson, you will be charged the full lesson fee.
Dispute Resolution
- If you have any concerns or issues with your tutoring experience, please contact us at [email protected] as soon as possible. We will work with you and your tutor to try to resolve any disputes or issues in a fair and reasonable manner.
Termination
- You may terminate your lessons with a tutor at any time by providing reasonable notice, as specified in your contract with us.
By using our service, you acknowledge that you have read and understand this agreement and agree to be bound by its terms. If you have any questions or concerns, please do not hesitate to contact us.
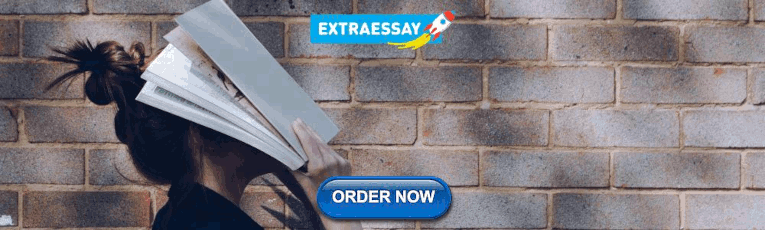
Copyright Policy
We respect the intellectual property rights of others and expect our users to do the same. It is our policy to respond to any claim that content posted on our service infringes the copyright or other intellectual property rights of any person.
If you are a copyright owner or authorised on behalf of one and you believe that your copyrighted work has been copied in a way that constitutes copyright infringement, please provide our copyright agent with the following information:
- An electronic or physical signature of the person authorised to act on behalf of the owner of the copyright’s interests.
- A description of the copyrighted work that you claim has been infringed, including the URL (web page address) of the location where the copyrighted work exists or a copy of the copyrighted work.
- Identification of the URL or other specific location on our service where the material that you claim is infringing is located.
- Your address, telephone number, and email address.
- A statement by you that you have a good faith belief that the disputed use is not authorized by the copyright owner, its agent, or the law.
- A statement by you, made under penalty of perjury, that the information in your notice is accurate and that you are the copyright owner or are authorised to act on the copyright owner’s behalf.
You may be held accountable for damages (including costs and attorneys’ fees) for misrepresenting that any content is infringing your copyright.
Upon receipt of a notification, we will take whatever action, in our sole discretion, we deem appropriate, including removal of the challenged content from our service. If you believe that your content has been removed in error, please contact us at [email protected] .
Intellectual Property
The original content on our services (excluding content provided by you or other users), features, and functionality are and will remain the exclusive property of Shalom Education Ltd and its licensors. This includes, but is not limited to, text, graphics, images, logos, software, and other materials on our website and any proprietary technology used in the operation of our services.
Our service is protected by copyright, trademark, and other laws of both the United Kingdom and foreign countries. Our trademarks and trade dress may not be used in connection with any product or service without the prior written consent of Shalom Education Ltd. All other trademarks not owned by Shalom Education Ltd that appear on our service are the property of their respective owners.
By using our service, you acknowledge and agree that any intellectual property rights, including copyrights, trademarks, patents, and trade secrets, in the content and materials provided by Shalom Education Ltd or accessed through our service are the sole property of Shalom Education Ltd or the respective owners of such rights. You agree not to use, reproduce, modify, distribute, or create derivative works of such content and materials without the express written permission of Shalom Education Ltd or the respective owners of such rights.
You are responsible for protecting your own intellectual property rights and for obtaining any necessary licenses or permissions from the owners of any third-party intellectual property that you may use in connection with your use of our service. Shalom Education Ltd will not be liable for any claims or damages arising from your use of intellectual property that infringes the rights of others.
If you believe that your intellectual property rights have been violated on our service, please contact us at [email protected] .
Your Feedback to Us
By submitting any feedback or suggestions to the company, you agree to assign to the company all rights, titles, and interests in such feedback or suggestions. If for any reason such assignment is ineffective, you agree to grant the company a non-exclusive, perpetual, irrevocable, royalty-free, worldwide right and license to use, reproduce, disclose, sublicense, distribute, modify, and exploit such feedback or suggestions without restriction.
You acknowledge that the company may use your feedback or suggestions for any purpose, including to improve the company’s products or services, and that the company is under no obligation to compensate you for your feedback or suggestions.
Links to Other Websites
Our Service may contain links to third-party websites or services that are not owned or controlled by Shalom Education Ltd. These links are provided for your convenience only and do not imply endorsement by our business of the linked website or service. We have no control over and assume no responsibility for the content, privacy policies, or practices of any third-party websites or services.
By using our Service, you acknowledge and agree that the use of any third-party websites or services is at your own risk. Shalom Education Ltd does not endorse or guarantee the accuracy or reliability of any content or materials on third-party websites or services, and we are not responsible for any errors or omissions.
In no event will Shalom Education Ltd be liable for any damage or loss caused or alleged to be caused by or in connection with the use of or reliance on any content, goods, or services available on or through any third-party websites or services. This limitation of liability applies to all claims.
We recommend that you carefully read the terms and conditions and privacy policies of any third-party websites or services that you visit, as they may differ from the terms and policies of our own services. If you have any concerns or questions about a third-party website or service linked from our service, we encourage you to contact the site or service directly for more information.
Shalom Education Ltd reserves the right to terminate or suspend your account at any time, without prior notice or liability, for any reason whatsoever, including but not limited to breach of these terms and conditions, fraudulent or illegal activity, or any other conduct that we deem inappropriate or harmful to our business or other users.
Upon termination, your right to use our services will immediately cease, and any outstanding balances or fees owed to Shalom Education Ltd must be paid in full. If you wish to terminate your account, you may simply stop using our services and contact us to request the closure of your account.
Any personal information or user-generated content associated with your account will be retained in accordance with our privacy policy, unless otherwise required by law.
You acknowledge and agree that Shalom Education Ltd will not be liable to you or any third party for any termination of your access to our services. You further agree that any rights or obligations that survive the termination of your account, such as indemnification or confidentiality obligations, will remain in effect.
“AS IS” and “AS AVAILABLE” Disclaimer
The service is provided to you “As is” and “As available” and with all faults and defects without warranty of any kind. While we make every effort to ensure the accuracy and reliability of our services, we cannot guarantee that they will be error-free or uninterrupted.
To the maximum extent permitted by applicable law, our business and its affiliates and licensors and service providers disclaim all warranties, expressed or implied, including but not limited to warranties of merchantability, fitness for a particular purpose, title and non-infringement. We do not make any representations or warranties that our services will meet your requirements, achieve any intended results, be compatible with any other software or services, operate without interruption, or be error-free.
We do not guarantee the accuracy, completeness, reliability, or timeliness of the information, content, or materials provided through our services.
We do not guarantee that our services or any content or materials provided through our services will be free from viruses, malware, or other harmful components. It is your responsibility to protect your device and system from such threats, and we recommend that you use appropriate security measures and virus protection software.
Some jurisdictions do not allow the exclusion of certain types of warranties or limitations on the applicable statutory rights of a consumer, so some or all of the above exclusions and limitations may not apply to you. In such cases, the exclusions and limitations set forth in this section shall be applied to the greatest extent enforceable under applicable law.
By using our services, you acknowledge and agree that your use is at your own risk, and that you are solely responsible for any damage or loss that may result from your use of our services.
Governing Law and Jurisdiction
These terms and your use of the service shall be governed by and construed in accordance with the laws of the country, excluding its conflicts of law rules. Any disputes arising out of or in connection with these terms or the use of the service shall be resolved through the courts of the country and you hereby consent to the exclusive jurisdiction of such courts.
Disputes Resolution
If you have any concerns or disputes related to the service, you agree to try to resolve the issue informally by contacting us first.
You can try contacting the company through email ([email protected]) or by phone (01206657616) to see if we can come to an agreement or find a solution to your issue. This can be a quick and effective way to address any concerns or issues you may have, without the need for formal legal proceedings.
We will make every effort to address your concerns and reach a mutually satisfactory resolution. If we are unable to resolve the dispute informally, you may choose to bring the matter to alternative dispute resolution through a mediator or arbitrator. Any such alternative dispute resolution proceedings will be conducted in accordance with the laws of the country and will be confidential. You agree that any such dispute will be resolved on an individual basis and that class or collective actions are not permitted.
Severability and Waiver
Severability.
If any provision of these terms and conditions is found to be invalid or unenforceable, that provision will be enforced to the maximum extent possible, and the remaining provisions will remain in full force and effect.
The failure to exercise a right or to require the performance of an obligation under these terms and conditions shall not affect a party’s ability to exercise such right or require such performance at any time in the future. Similarly, the waiver of a breach of these terms and conditions shall not constitute a waiver of any subsequent breach.
Changes to These Terms and Conditions
We may update or change these terms and conditions at any time, at our sole discretion. If we make a material change to these terms, we will provide reasonable notice, such as by posting a notification on our website or through email, at least 30 days before the change takes effect. It is your responsibility to regularly check these terms and conditions for any updates or changes.
By continuing to access or use our Service after any updates or changes to these terms, you agree to be bound by the revised terms. If you do not agree to the updated or changed terms, in whole or in part, please stop using the website and our services.
If you have any questions about these terms and conditions, You can contact us:
- By email: [email protected]
- By visiting this page on our website: https://www.shalom-education.com/contact-us
- By phone number: 01206657616
- Tutor refers to an individual that teaches a single pupil or a small group of students which have registered with Shalom Education Ltd.
- Tutee refers to a student or a pupil that has registered for tutoring with Shalom Education Ltd, which is administered through our tutoring platform.
When you place an order, we may ask you to provide certain information, such as your name, email, phone number, credit card details and billing address.
Username or Email Address
Remember Me
Registration confirmation will be emailed to you.
River Tees Example

It also provides an example of how river basins can be managed against flooding.

River Tees Catchment factors
Physical (natural Factors) The River Tees catchment is located in the north east of England. It has three main rivers, the River Tees, the River Skerne and the River Leven. The River Tees drains the eastern slopes of Cross Fell in the Pennines and flows eastward to the North Sea. The length of the channel from source to sea is approximately 160 kilometres. The River Tees rises on the slopes of Cross Fell at a height of 893metres. The area receives over 2,000 millimetres of rain each year. The rainfall reaches the river quickly because the slopes are steep and very little water can infiltrate due to the impermeable rocks and saturated peat. After heavy rainfall or when the snow melts in the spring the river level can rise quickly and the water also flows downstream quickly. This can cause the ‘Tees roll’ or ‘Tees wave’, when the river level may rise as much as a metre in 15 minutes. There is a long history of flooding along the River Tees, especially in its lower course.
The catchment has areas with distinctly different characteristics. The rivers in the Upper Tees have steep channel gradients and valley sides. In the mid-catchment, the valley widens out and channel slopes become much gentler. The lower catchment is close to sea level and predominantly tidal in nature.
Human Factors
The Tees Barrage forms an artificial barrier between the Tees Estuary and the upstream catchment. This helps maintain water levels for amenity purposes and eliminates tidal effects further upstream. Land use in the west of the area is mainly moorland and pasture. On the lower slopes and middle catchment the land use changes to a greater amount of pasture and woodland.
To the east land use is mainly arable farmland interspersed with large built up areas, including Middlesbrough and Stockton-on-Tees. Approximately 687,000 people live within the catchment area concentrated in the main towns of Darlington, Hartlepool, Middlesbrough, Redcar and Cleveland, and Stockton-on-Tees. There are also large industrial areas such as the ICI chemical works. Flood damage can run into hundreds of thousands of pounds. There is also a huge demand for water for use in homes, industry and agriculture.
Flood events along the River Tees “ The Tees catchment has a long history of flooding with reported flooding dating back over 400 years. Over the years, a number of engineering schemes have been implemented to reduce the risk of flooding in the catchment. At present over 9,600 properties including some key infrastructure buildings are at risk of flooding (not taking into account defences) during the one per cent flood event. In the future due to climate change this number is expected to increase to 11,230 .” David Dangerfield, Director – Yorkshire and North East Environment Agency Tees Catchment Flood Management Plan
Historic events On the night of 16/17th November 1771 the North East of England suffered some of the worst and most destructive flooding on record. The river Tees burst its banks in a number of places with Yarm being one of the areas worst affected. At the height of the flood some stretches of Yarm High Street were submerged in 20 feet of water. The floods were caused by a sudden thaw of the ice in upper Teesdale, and a cloud burst over the Pennines. The rain began in the early hours of Saturday morning and continued heavily throughout the day and night without a break. On the main street alone, six houses were completely destroyed and many more left uninhabitable. The Shambles was washed away and the town’s main church was also damaged. ‘The pews were upturned and tossed about, the pulpit was overturned and several windows were shattered’. Nine of the townspeople lost their lives in the floods. In one home alone where three people were staying, two drowned, and one survived by hanging from the top of a window for nineteen hours with floodwater up to his chin. Source The river flooded again in 1995 and 2015. Within the Tees catchment the main consequences of flooding occur in the urban areas of the catchment. In total there are almost 8,500 residential properties and over 1,200 commercial properties at risk of flooding. This means almost three per cent of the catchment population is at risk from the one per cent flood event.This means that flood management is definitely needed in this catchment

Above - Properties at risk of flooding on River Tees
Flood Management along the Tees
The Flood defence scheme in Yarm Since the 1995 flood event a new flood defence scheme costing £2.1 million has been built. They have also used improved flood warning systems which liaise with the Meteorological Office, police and other emergency services. New development has discouraged building on low-lying and flood-prone land (land is used for activities not damaged by inundation e.g. playing fields, parks, urban forests/walks etc.) In the Yarm area the Environment Agency have;
Put in Reinforced concrete walls with metal flood gates for access by people and vehicles Used Earth Embankments Put in Gabions to protect walls and embankments from erosion Included Fishing platforms, street lighting and replanting to improve the environment Kept building materials in keeping with existing architecture
Cow Green Reservoir

NEXT TOPIC - Hydrographs
©2015 Cool Geography
- Copyright Policy
- Privacy & Cookies
- Testimonials
- Feedback & support

Case Study: River Tees
River valley in the uk – river tees.
The River Tees is located in northeast England. The River Tees flows from the Pennine Hills to the North Sea.
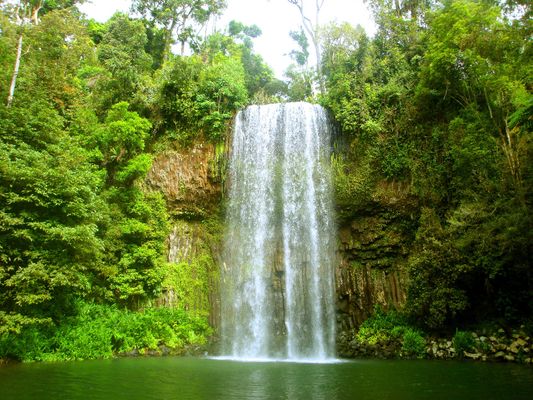
Landforms of erosion
- The High Force is a 20 m high waterfall.
- The High Force was formed by the erosion of limestone (less resistant) which lies underneath a layer of dolerite (more resistant).
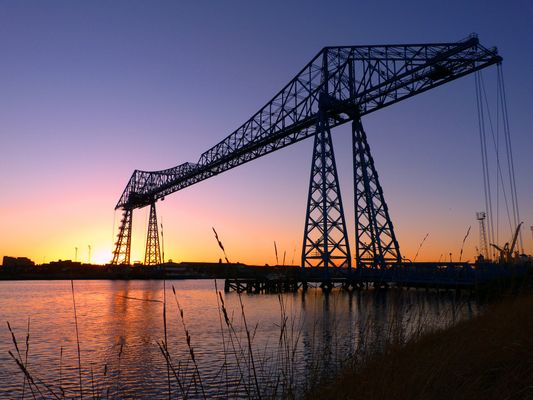
Landforms of deposition
- Landforms of deposition are found in the lower course of the River Tees.
- Floodplains.
1 Geography Skills
1.1 Mapping
1.1.1 Map Making
1.1.2 OS Maps
1.1.3 Grid References
1.1.4 Contour Lines
1.1.5 Symbols, Scale and Distance
1.1.6 Directions on Maps
1.1.7 Describing Routes
1.1.8 Map Projections
1.1.9 Aerial & Satellite Images
1.1.10 Using Maps to Make Decisions
1.2 Geographical Information Systems
1.2.1 Geographical Information Systems
1.2.2 How do Geographical Information Systems Work?
1.2.3 Using Geographical Information Systems
1.2.4 End of Topic Test - Geography Skills
2 Geology of the UK
2.1 The UK's Rocks
2.1.1 The UK's Main Rock Types
2.1.2 The UK's Landscape
2.1.3 Using Rocks
2.1.4 Weathering
2.2 Case Study: The Peak District
2.2.1 The Peak District
2.2.2 Limestone Landforms
2.2.3 Quarrying
3 Geography of the World
3.1 Geography of America & Europe
3.1.1 North America
3.1.2 South America
3.1.3 Europe
3.1.4 The European Union
3.1.5 The Continents
3.1.6 The Oceans
3.1.7 Longitude
3.1.8 Latitude
3.1.9 End of Topic Test - Geography of the World
4 Development
4.1 Development
4.1.1 Classifying Development
4.1.3 Evaluation of GDP
4.1.4 The Human Development Index
4.1.5 Population Structure
4.1.6 Developing Countries
4.1.7 Emerging Countries
4.1.8 Developed Countries
4.1.9 Comparing Development
4.2 Uneven Development
4.2.1 Consequences of Uneven Development
4.2.2 Physical Factors Affecting Development
4.2.3 Historic Factors Affecting Development
4.2.4 Human & Social Factors Affecting Development
4.2.5 Breaking Out of the Poverty Cycle
4.3 Case Study: Democratic Republic of Congo
4.3.1 The DRC: An Overview
4.3.2 Political & Social Factors Affecting Development
4.3.3 Environmental Factors Affecting the DRC
4.3.4 The DRC: Aid
4.3.5 The Pros & Cons of Aid in DRC
4.3.6 Top-Down vs Bottom-Up in DRC
4.3.7 The DRC: Comparison with the UK
4.3.8 The DRC: Against Malaria Foundation
4.4 Case Study: Nigeria
4.4.1 The Importance & Development of Nigeria
4.4.2 Nigeria's Relationships with the Rest of the World
4.4.3 Urban Growth in Lagos
4.4.4 Population Growth in Lagos
4.4.5 Factors influencing Nigeria's Growth
4.4.6 Nigeria: Comparison with the UK
5 Weather & Climate
5.1 Weather
5.1.1 Weather & Climate
5.1.2 Components of Weather
5.1.3 Temperature
5.1.4 Sunshine, Humidity & Air Pressure
5.1.5 Cloud Cover
5.1.6 Precipitation
5.1.7 Convectional Precipitation
5.1.8 Frontal Precipitation
5.1.9 Relief or Orographic Precipitation
5.1.10 Wind
5.1.11 Extreme Wind
5.1.12 Recording the Weather
5.1.13 Extreme Weather
5.2 Climate
5.2.1 Climate of the British Isles
5.2.2 Comparing Weather & Climate London
5.2.3 Climate of the Tropical Rainforest
5.2.4 End of Topic Test - Weather & Climate
5.3 Tropical Storms
5.3.1 Formation of Tropical Storms
5.3.2 Features of Tropical Storms
5.3.3 The Structure of Tropical Storms
5.3.4 Tropical Storms Case Study: Katrina Effects
5.3.5 Tropical Storms Case Study: Katrina Responses
6 The World of Work
6.1 Tourism
6.1.1 Landscapes
6.1.2 The Growth of Tourism
6.1.3 Benefits of Tourism
6.1.4 Economic Costs of Tourism
6.1.5 Social, Cultural & Environmental Costs of Tourism
6.1.6 Tourism Case Study: Blackpool
6.1.7 Ecotourism
6.1.8 Tourism Case Study: Kenya
7 Natural Resources
7.1.1 What are Rocks?
7.1.2 Types of Rock
7.1.4 The Rock Cycle - Weathering
7.1.5 The Rock Cycle - Erosion
7.1.6 What is Soil?
7.1.7 Soil Profiles
7.1.8 Water
7.1.9 Global Water Demand
7.2 Fossil Fuels
7.2.1 Introduction to Fossil Fuels
7.2.2 Fossil Fuels
7.2.3 The Global Energy Supply
7.2.5 What is Peak Oil?
7.2.6 End of Topic Test - Natural Resources
8.1 River Processes & Landforms
8.1.1 Overview of Rivers
8.1.2 The Bradshaw Model
8.1.3 Erosion
8.1.4 Sediment Transport
8.1.5 River Deposition
8.1.6 River Profiles: Long Profiles
8.1.7 River Profiles: Cross Profiles
8.1.8 Waterfalls & Gorges
8.1.9 Interlocking Spurs
8.1.10 Meanders
8.1.11 Floodplains
8.1.12 Levees
8.1.13 Case Study: River Tees
8.2 Rivers & Flooding
8.2.1 Flood Risk Factors
8.2.2 Flood Management: Hard Engineering
8.2.3 Flood Management: Soft Engineering
8.2.4 Flooding Case Study: Boscastle
8.2.5 Flooding Case Study: Consequences of Boscastle
8.2.6 Flooding Case Study: Responses to Boscastle
8.2.7 Flooding Case Study: Bangladesh
8.2.8 End of Topic Test - Rivers
8.2.9 Rivers Case Study: The Nile
8.2.10 Rivers Case Study: The Mississippi
9.1 Formation of Coastal Landforms
9.1.1 Weathering
9.1.2 Erosion
9.1.3 Headlands & Bays
9.1.4 Caves, Arches & Stacks
9.1.5 Wave-Cut Platforms & Cliffs
9.1.6 Waves
9.1.7 Longshore Drift
9.1.8 Coastal Deposition
9.1.9 Spits, Bars & Sand Dunes
9.2 Coast Management
9.2.1 Management Strategies for Coastal Erosion
9.2.2 Case Study: The Holderness Coast
9.2.3 Case Study: Lyme Regis
9.2.4 End of Topic Test - Coasts
10 Glaciers
10.1 Overview of Glaciers & How They Work
10.1.1 Distribution of Glaciers
10.1.2 Types of Glaciers
10.1.3 The Last Ice Age
10.1.4 Formation & Movement of Glaciers
10.1.5 Shaping of Landscapes by Glaciers
10.1.6 Glacial Landforms Created by Erosion
10.1.7 Glacial Till & Outwash Plain
10.1.8 Moraines
10.1.9 Drumlins & Erratics
10.1.10 End of Topic Tests - Glaciers
10.1.11 Tourism in Glacial Landscapes
10.1.12 Strategies for Coping with Tourists
10.1.13 Case Study - Lake District: Tourism
10.1.14 Case Study - Lake District: Management
11 Tectonics
11.1 Continental Drift & Plate Tectonics
11.1.1 The Theory of Plate Tectonics
11.1.2 The Structure of the Earth
11.1.3 Tectonic Plates
11.1.4 Plate Margins
11.2 Volcanoes
11.2.1 Volcanoes & Their Products
11.2.2 The Development of Volcanoes
11.2.3 Living Near Volcanoes
11.3 Earthquakes
11.3.1 Overview of Earthquakes
11.3.2 Consequences of Earthquakes
11.3.3 Case Study: Christchurch, New Zealand Earthquake
11.4 Tsunamis
11.4.1 Formation of Tsunamis
11.4.2 Case Study: Japan 2010 Tsunami
11.5 Managing the Risk of Volcanoes & Earthquakes
11.5.1 Coping With Earthquakes & Volcanoes
11.5.2 End of Topic Test - Tectonics
12 Climate Change
12.1 The Causes & Consequences of Climate Change
12.1.1 Evidence for Climate Change
12.1.2 Natural Causes of Climate Change
12.1.3 Human Causes of Climate Change
12.1.4 The Greenhouse Effect
12.1.5 Effects of Climate Change on the Environment
12.1.6 Effects of Climate Change on People
12.1.7 Climate Change Predictions
12.1.8 Uncertainty About Future Climate Change
12.1.9 Mitigating Against Climate Change
12.1.10 Adapting to Climate Change
12.1.11 Case Study: Bangladesh
13 Global Population & Inequality
13.1 Global Populations
13.1.1 World Population
13.1.2 Population Structure
13.1.3 Ageing Populations
13.1.4 Youthful Populations
13.1.5 Population Control
13.1.6 Mexico to USA Migration
13.1.7 End of Topic Test - Development & Population
14 Urbanisation
14.1 Urbanisation
14.1.1 Rural Characterisitcs
14.1.2 Urban Characteristics
14.1.3 Urbanisation Growth
14.1.4 The Land Use Model
14.1.5 Rural-Urban Pull Factors
14.1.6 Rural-Urban Push Factors
14.1.7 The Impacts of Migration
14.1.8 Challenges of Urban Areas in Developed Countries
14.1.9 Challenges of Urban Areas in Developing Countries
14.1.10 Urban Sustainability
14.1.11 Case Study: China's Urbanisation
14.1.12 Major UK Cities
14.1.13 Urbanisation in the UK
14.1.14 End of Topic Test- Urbanisation
14.1.15 End of Topic Test - Urban Issues
15 Ecosystems
15.1 The Major Biomes
15.1.1 Distribution of Major Biomes
15.1.2 What Affects the Distribution of Biomes?
15.1.3 Biome Features: Tropical Forests
15.1.4 Biome Features: Temperate Forests
15.1.5 Biome Features: Tundra
15.1.6 Biome Features: Deserts
15.1.7 Biome Features: Tropical Grasslands
15.1.8 Biome Features: Temperate Grasslands
15.2 Case Study: The Amazon Rainforest
15.2.1 Interdependence of Rainforest Ecosystems
15.2.2 Nutrient Cycling in Tropical Rainforests
15.2.3 Deforestation in the Amazon
15.2.4 Impacts of Deforestation in the Amazon
15.2.5 Protecting the Amazon
15.2.6 Adaptations of Plants to Rainforests
15.2.7 Adaptations of Animals to Rainforests
16 Life in an Emerging Country
16.1 Case Studies
16.1.1 Mumbai: Opportunities
16.1.2 Mumbai: Challenges
17 Analysis of Africa
17.1 Africa
17.1.1 Desert Biomes in Africa
17.1.2 The Semi-Desert Biome
17.1.3 The Savanna Biome
17.1.4 Overview of Tropical Rainforests
17.1.5 Colonisation History
17.1.6 Population Distribution in Africa
17.1.7 Economic Resources in Africa
17.1.8 Urbanisation in Africa
17.1.9 Africa's Location
17.1.10 Physical Geography of Africa
17.1.11 Desertification in Africa
17.1.12 Reducing the Risk of Desertification
17.1.13 Case Study: The Sahara Desert - Opportunities
17.1.14 Case Study: The Sahara Desert - Development
18 Analysis of India
18.1 India - Physical Geography
18.1.1 Geographical Location of India
18.1.2 Physical Geography of India
18.1.3 India's Climate
18.1.4 Natural Disasters in India
18.1.5 Case Study: The Thar Desert
18.1.6 Case Study: The Thar Desert - Challenges
18.2 India - Human Geography
18.2.1 Population Distribution in India
18.2.2 Urabinsation in India
18.2.3 The History of India
18.2.4 Economic Resources in India
19 Analysis of the Middle East
19.1 The Middle East
19.1.1 Physical Geography of the Middle East
19.1.2 Human Geography of the Middle East
19.1.3 Climate Zones in the Middle East
19.1.4 Climate Comparison with the UK
19.1.5 Oil & Natural Gas in the Middle East
19.1.6 Water in the Middle East
19.1.7 Population of the Middle East
19.1.8 Development Case Studies: The UAE
19.1.9 Development Case Studies: Yemen
19.1.10 Supporting Development in Yemen
19.1.11 Connection to the UK
19.1.12 Importance of Oil
19.1.13 Oil & Tourism in the UAE
20 Analysis of Bangladesh
20.1 Bangladesh Physical Geography
20.1.1 Location of Bangladesh
20.1.2 Climate of Bangladesh
20.1.3 Rivers in Bangladesh
20.1.4 Flooding in Bangladesh
20.2 Bangladesh Human Geography
20.2.1 Population Structure in Bangladesh
20.2.2 Urbanisation in Bangladesh
20.2.3 Bangladesh's Economy
20.2.4 Energy & Sustainability in Bangladesh
21 Analysis of Russia
21.1 Russia's Physical Geography
21.1.1 Russia's Climate
21.1.2 Russia's Landscape
21.2 Russia's Human Geography
21.2.1 Population of Russia
21.2.2 Russia's Economy
21.2.3 Energy & Sustainability in Russia
Jump to other topics
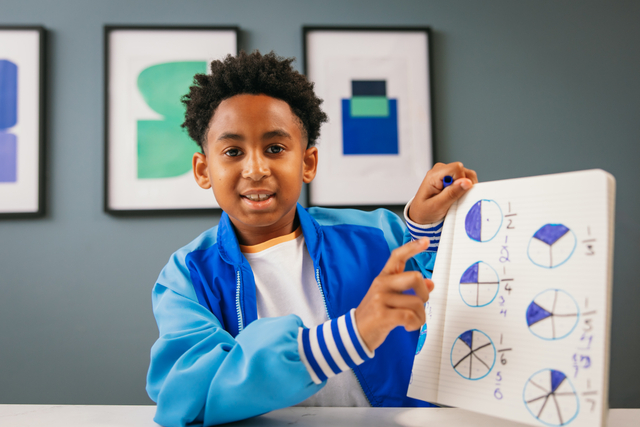
Unlock your full potential with GoStudent tutoring
Affordable 1:1 tutoring from the comfort of your home
Tutors are matched to your specific learning needs
30+ school subjects covered
Flood Risk Factors
- International
- Schools directory
- Resources Jobs Schools directory News Search
GCSE Geography - River Tees case study
Subject: Geography
Age range: 14-16
Resource type: Lesson (complete)

Last updated
4 August 2022
- Share through email
- Share through twitter
- Share through linkedin
- Share through facebook
- Share through pinterest
Complete lesson - Linked to OCR 2016 Geography syllabus Based of case study sheet (included) and YouTube link PowerPoint self explanatory
- Do it Now task - review of UK landscapes and geomorphic processes learning Case study of River Tees
- Location of River Tees
- Changing landscape downstream - upper, middle and lower course
- Human activity - Cow Green reservoir, farming and human settlement
- Extended question to assess application of knowledge (model answer included)
Tes paid licence How can I reuse this?
Your rating is required to reflect your happiness.
It's good to leave some feedback.
Something went wrong, please try again later.
This resource hasn't been reviewed yet
To ensure quality for our reviews, only customers who have purchased this resource can review it
Report this resource to let us know if it violates our terms and conditions. Our customer service team will review your report and will be in touch.
Not quite what you were looking for? Search by keyword to find the right resource:
- 0 Shopping Cart
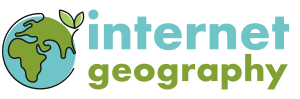
GCSE Geography Rivers Revision
Landforms of the River Tees Quiz
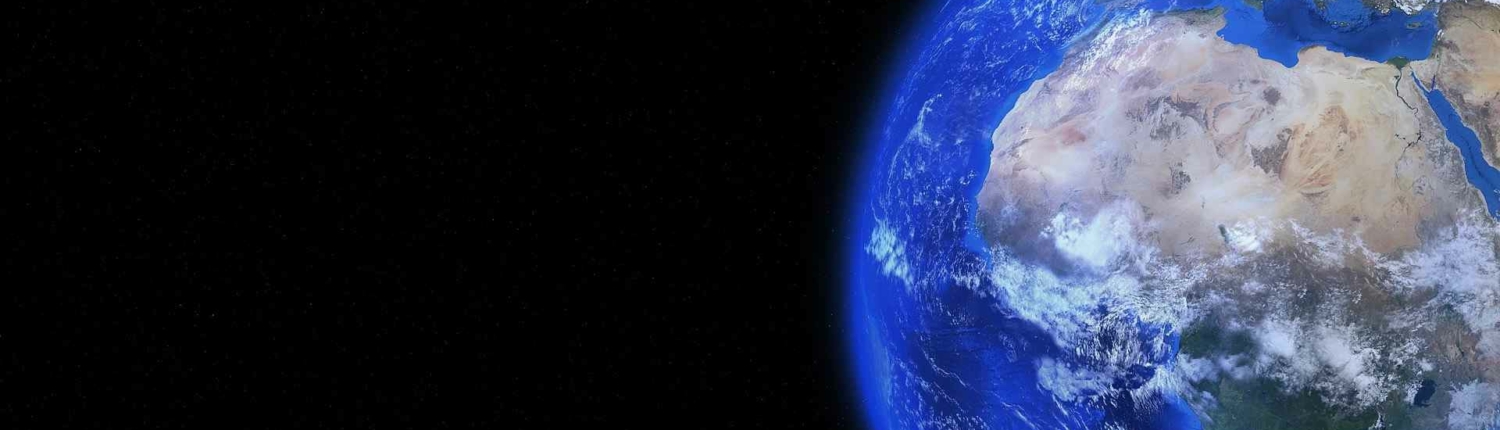
GCSE Geography Revision > Rivers > Landforms of the River Tees Quiz
Study the map showing major rivers in the UK. Identify the location of the River Tees.
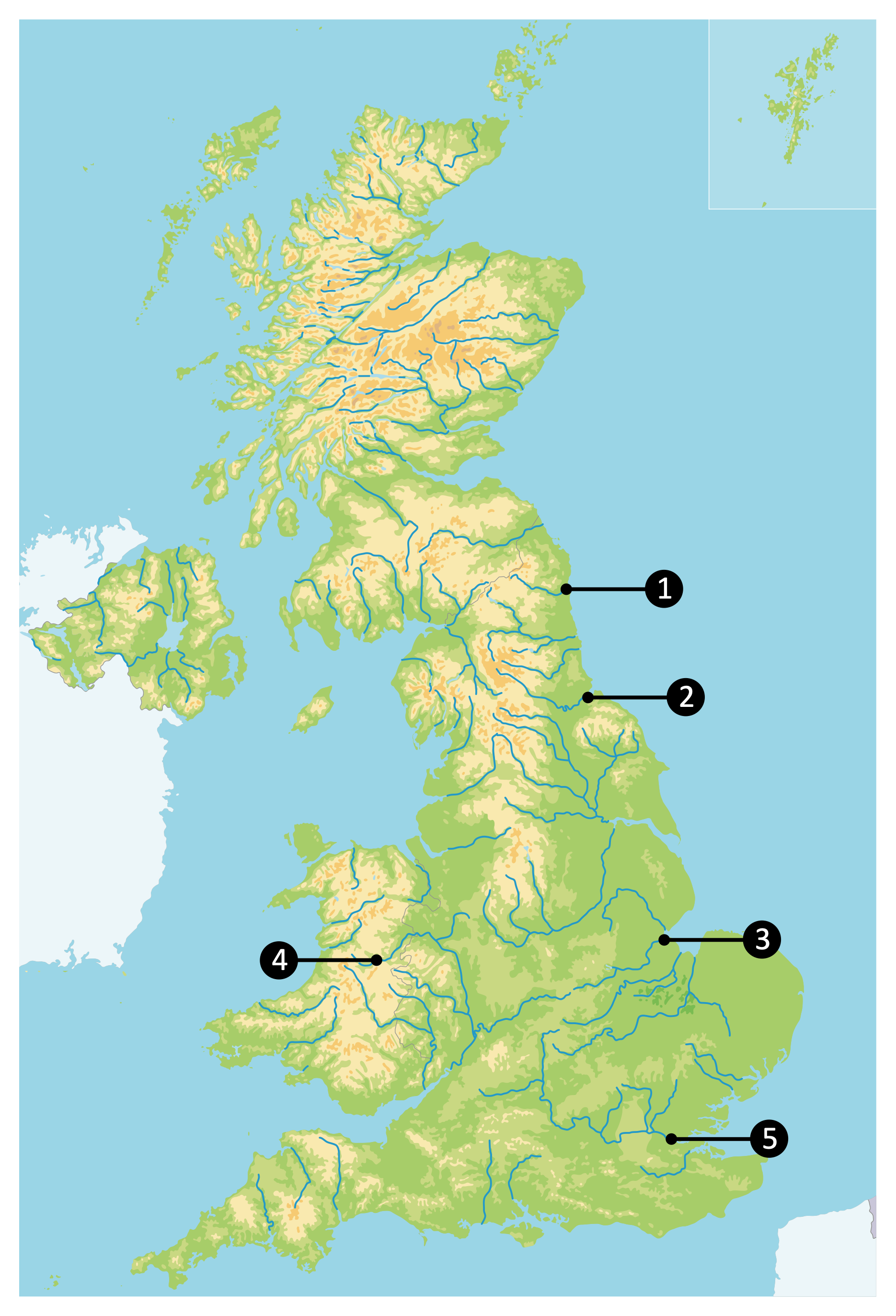
True or false? The River Tees is the longest river in the UK.
What is the length of the river tees, what direction does the river tees flow, where is the source of the river tees, which of the following is a waterfall on the river tees, true or false the image above shows a waterfall on the river tees..
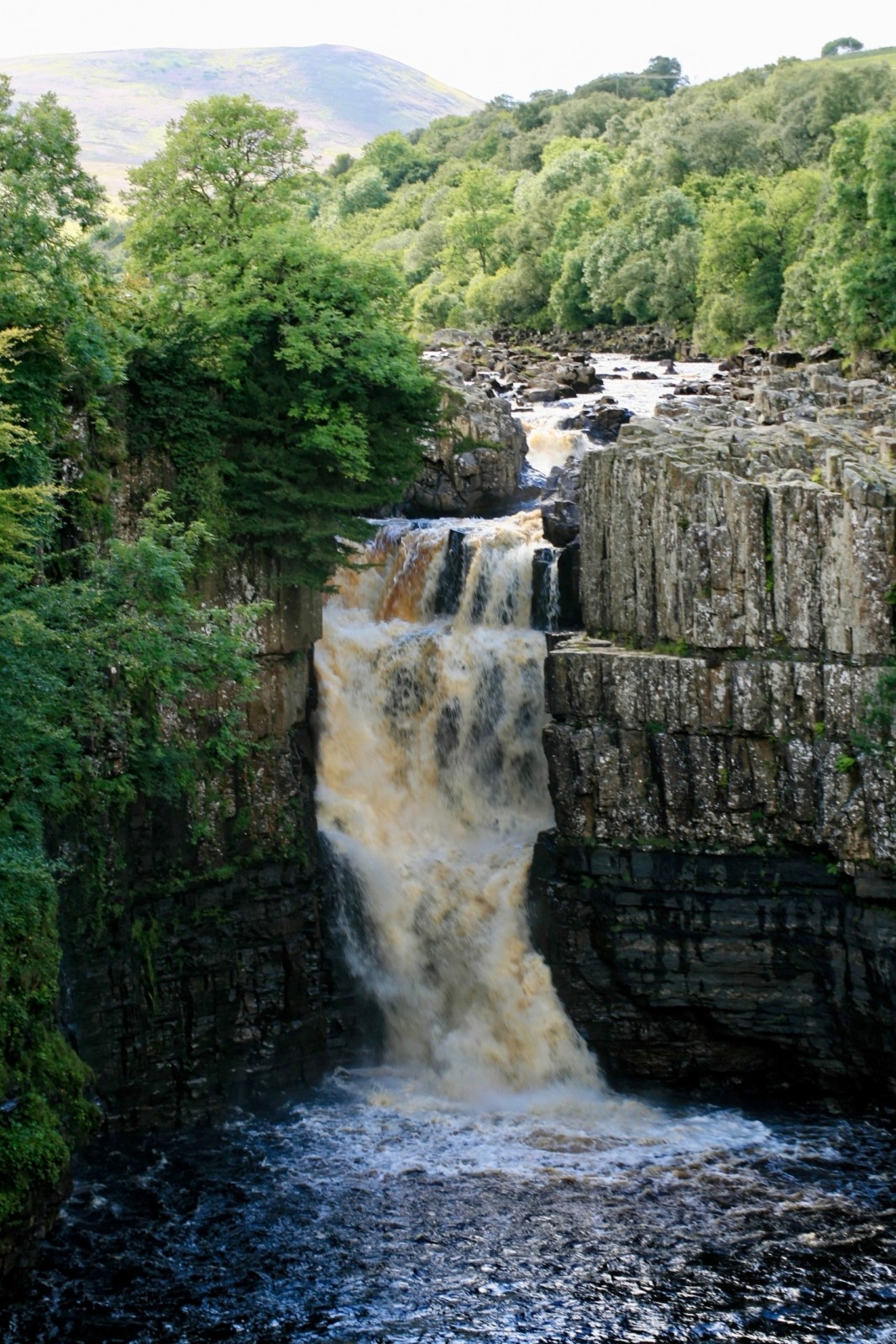
Which of the following explains the formation of High Force waterfall?
True or false a gorge has formed due to the upstream migration of high force waterfall., true or false the river tees has extensive meanders along its middle and lower course., true or false natural levées are found along the river tees in its middle and low course., which of the following are found towards the mouth of the river tees.
Please select 2 correct answers
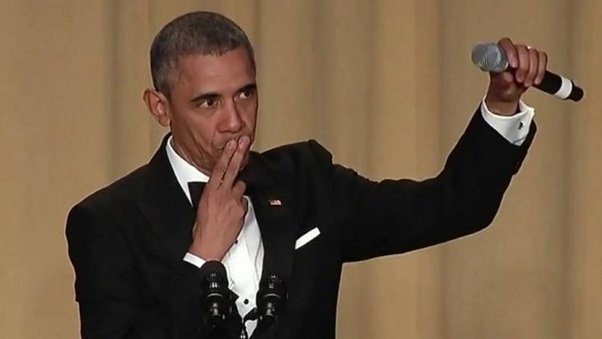
Share this:
- Click to share on Twitter (Opens in new window)
- Click to share on Facebook (Opens in new window)
- Click to share on Pinterest (Opens in new window)
- Click to email a link to a friend (Opens in new window)
- Click to share on WhatsApp (Opens in new window)
- Click to print (Opens in new window)
Please Support Internet Geography
If you've found the resources on this site useful please consider making a secure donation via PayPal to support the development of the site. The site is self-funded and your support is really appreciated.
Search Internet Geography
Top posts and pages.

Latest Blog Entries

Pin It on Pinterest
- Click to share
- Print Friendly
What caused Dubai floods? Experts cite climate change, not cloud seeding
- Medium Text
DID CLOUD SEEDING CAUSE THE STORM?
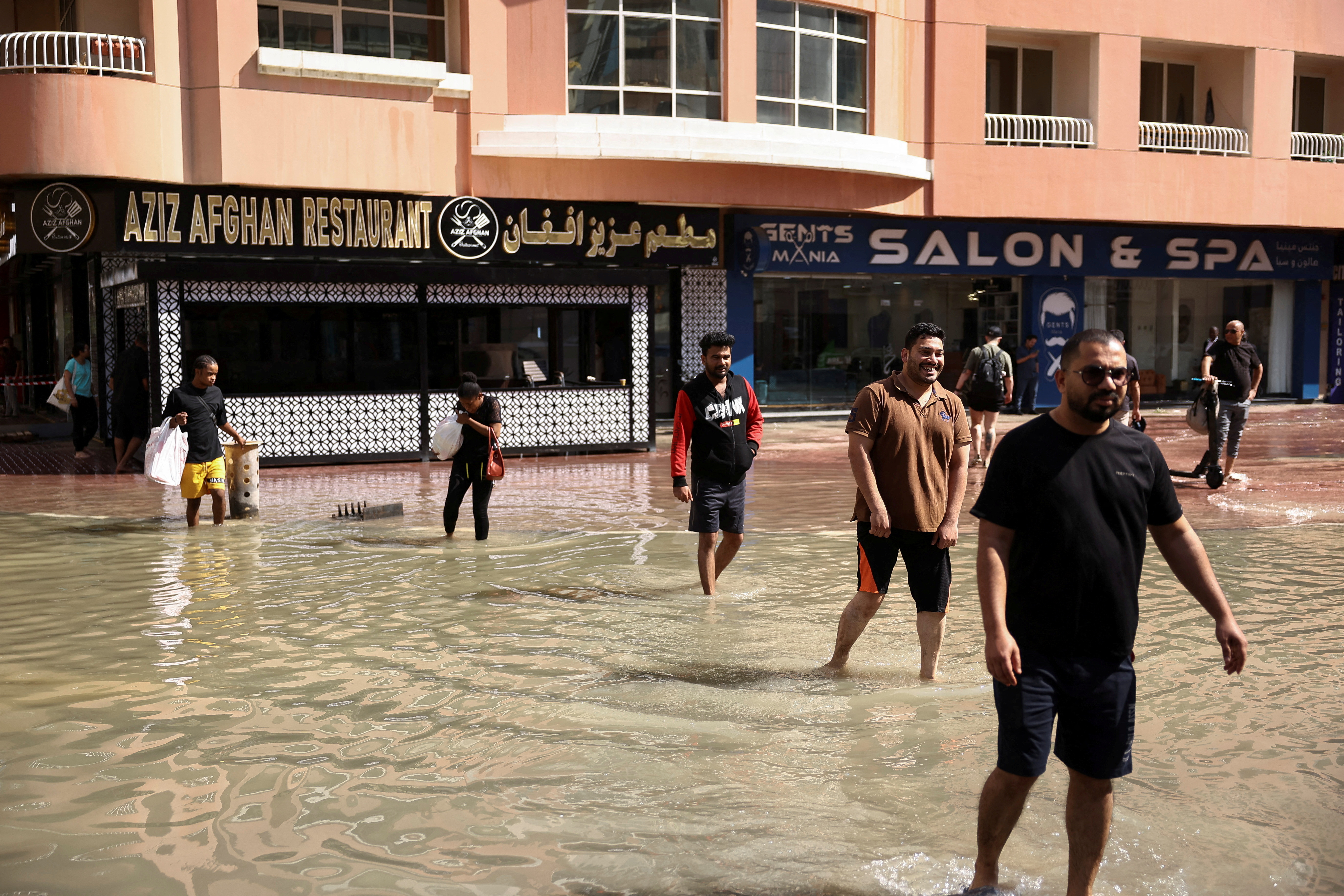
CAN'T CREATE CLOUDS FROM NOTHING
Sign up here.
Reporting by Alexander Cornwell; editing by Maha El Dahan and Alexandra Hudson
Our Standards: The Thomson Reuters Trust Principles. New Tab , opens new tab
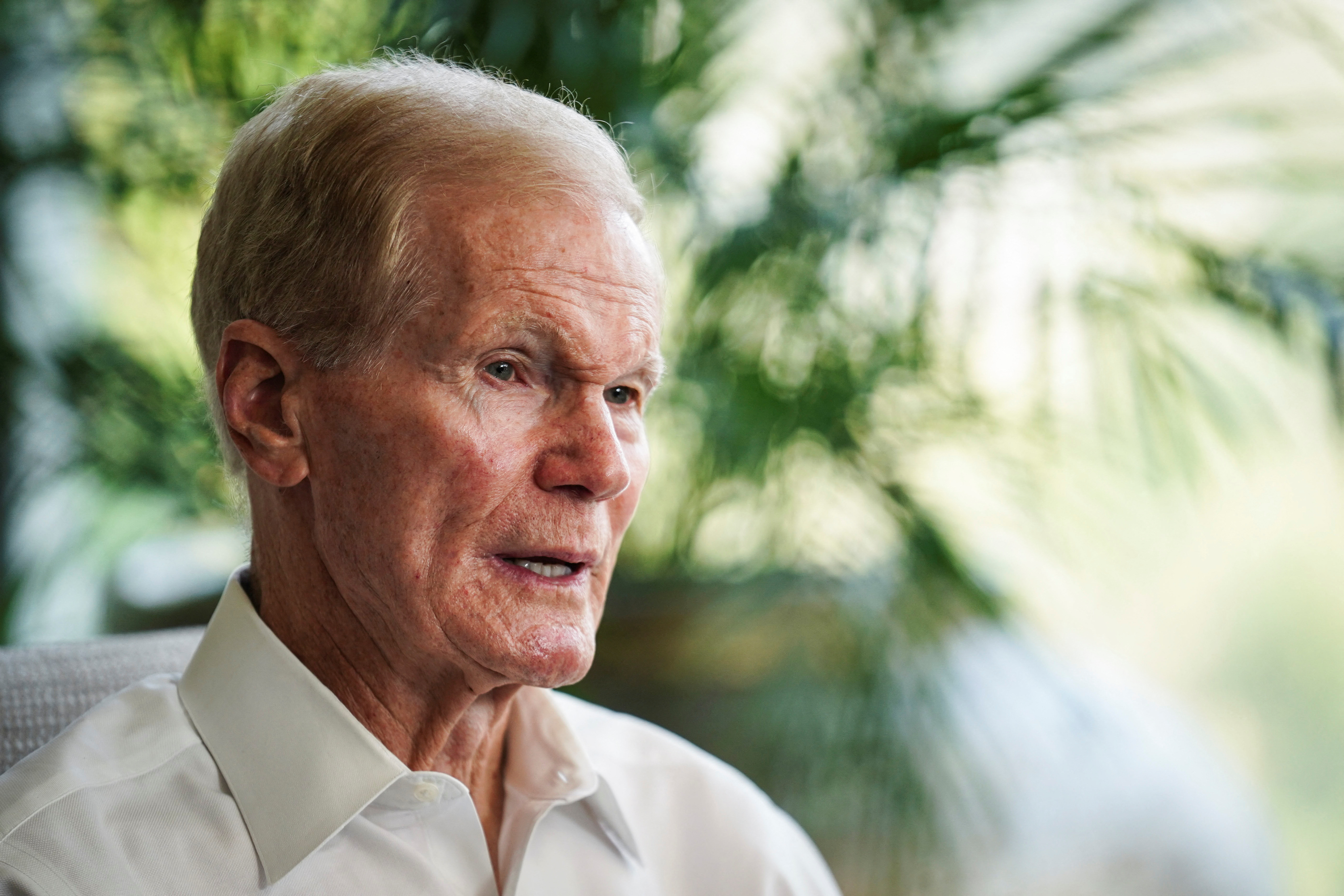
World Chevron
Nasa chief asks nations to work together on climate change.
NASA is hoping that nations will work together more closely in the future on topics such as climate change, including greenhouse gas emissions, the space agency's head, Bill Nelson, said on Tuesday.
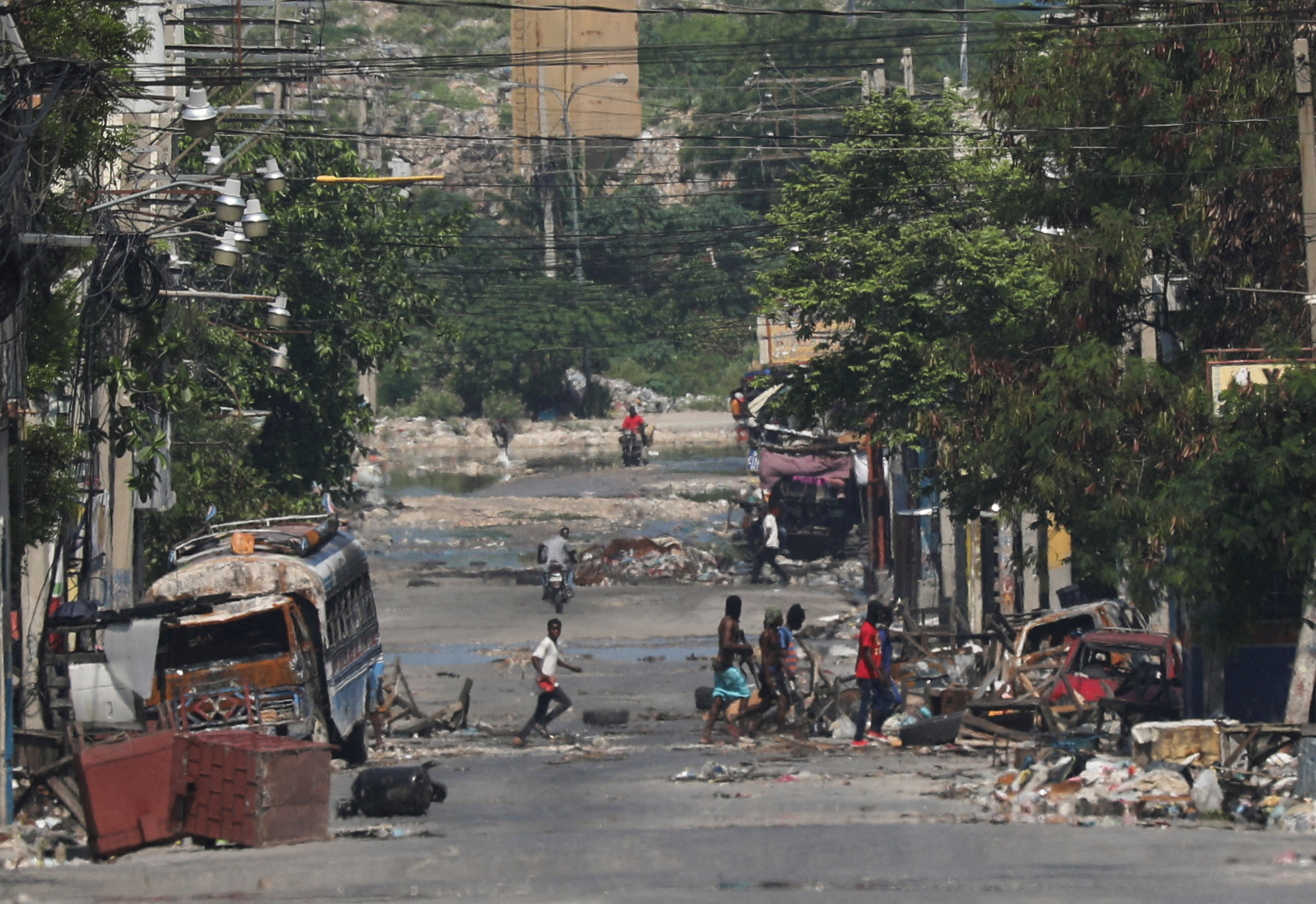
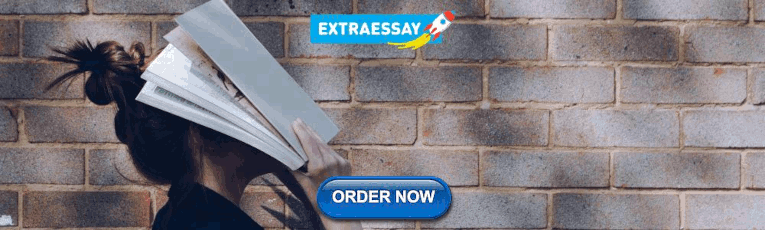
COMMENTS
The river Tees is located in the North-East of the UK and its source is 893 metres above sea level, in the Pennine Hills. Here there is an average of 2000mm of rainfall each year and the run-off rate is very high due to an impermeable bedrock; therefore this area is prone to flash floods. In the middle course of the river there are many large ...
The River Tees Case Study - landforms of erosion and deposition. The River Tees is located in the north of England. It flows 137 km (85 miles) east from its source in the Pennines to its mouth on the North Sea coast. The upper, the middle and lower course of the River Tees. The source of the River Tees is Cross Fell in the Pennines.
Rivers Case Study- The River Tees. The River Tees runs from Cross Fell (its source) in the Northern Pennines to its Mouth at Middlesbrough. Mr Knights. ... Whilst it will protect from flooding and provides leisure activities, it can be a hinderance to migrating fish. Reservoirs in the upper course.
The River Tees flows for 110km from Cross Fell in Cumbria eastwards towards the North Sea. This case study will take you on a journey from from the source to the mouth and will focus in on the landforms and changing features. The course and key features of the River Tees. Use the link below to discover the location of the River Tees within ...
Case study - River Tees. The River Tees is located in the north of England. The. source. of the River Tees is located in the Pennines and it flows east to its. mouth. where the river joins the ...
Case Study: River Tees. The River Tees, located in Northern England, flows from its source at Cross Fell in the Pennines travelling 130 km until it reaches its mouth at Teesside, where it meets the North Sea. With a drainage basin spanning 1,800 km², the River Tees undergoes various processes that shape its course, influenced by both natural ...
Above - Properties at risk of flooding on River Tees. Flood Management along the Tees. The Flood defence scheme in Yarm Since the 1995 flood event a new flood defence scheme costing £2.1 million has been built. They have also used improved flood warning systems which liaise with the Meteorological Office, police and other emergency services.
The River TeesCase Study. The River Tees. Case Study. The source of the Tees rises on the eastward slope of Cross Fell in the Pennines at a height of about 750 m, and flows eastwards for about 85 miles (137 km) before emptying into the North Sea. The Tees drains an area of 708 miles 2 (1834 km 2 ).
GCSE; OCR; River landforms - OCR Case study - river basin - River Tees. Erosional landforms include V-shaped valleys, interlocking spurs, waterfalls and gorges. Meanders and oxbow lakes use ...
GCSE; Edexcel; River landforms - Edexcel Case study - river landforms: River Tees. Erosional landforms include interlocking spurs, waterfalls and gorges. Meanders and oxbow lakes use erosional and ...
Case study of river landforms on the River TeesThis is the forty-sixth video for the AQA GCSE 9-1 Geography course, and the thirteenth video of the Physical...
Affordable 1:1 tutoring from the comfort of your home. Tutors are matched to your specific learning needs. 30+ school subjects covered. Book a free trial lesson. The River Valley. Erosion. The River Tees is located in northeast England. The River Tees flows from the Pennine Hills to the North Sea.
tudorgeog. Technology Business. 1 of 17. Flooding 7 River Tees Case Study. Flooding 7 River Tees Case Study - Download as a PDF or view online for free.
The River Tees is located in northeast England. The River Tees flows from the Pennine Hills to the North Sea.
at 1995 flood, flood management at Yarm. £2.1 million defence system Wide, flat floodplains, a flashy river (limited infiltration) & large meanders in the Lower Course of the River Tees mean a number of the towns are highly prone to flooding - particularly Yarm, Neasham, Stockton, Darlington & Middlesbrough. This includes a number of industries and residential buildings.
Age range: 14-16. Resource type: Lesson (complete) File previews. pptx, 25.68 MB. This resource is designed for a GCSE Geography class. The learning objectives of the lesson are: To learn about the river basin management of the River Tees. To learn about the human activity around the River Tees. The lesson has a number of activities including:
A bend in a river is called a meander. Meanders have the fastest flowing, most erosive water on the outside of the bend. This causes erosion and river bank collapse creating river cliffs. On the inside of the bend the water is shallower so there is more friction and the flow is much slower.
The Somerset Levels Flood Case Study. The Somerset Levels are a coastal plain and wetland area in Somerset, England. Thousands of years ago, the area was covered by the sea, but today it's a landscape of rivers and wetlands - artificially drained, irrigated and modified to allow productive farming.. It is claimed that the Somerset Levels are one of the lowest areas in the UK.
AQA Geog. GCSE - Flood Management - River Tees Case Study. Flashcards; Learn; Test; Match; Q-Chat; Get a hint. Where is the River Tees located? ... How long is the River Tees? 128km long. Give two physical features in the upper course: - The 20m high High Force waterfall - The Windstone Rock.
The River Tees - landforms of erosion and deposition; The Water Cycle; What is a river basin? River Discharge; Flood Hydrographs; River Flooding and Management; Rivers - Hard Engineering; Rivers - Soft Engineering; Case Study - Ganges/Brahmaputra River Basin; The Great Floods of 2000; Kerala flood case study; Rocks, Resources and ...
The Tees Barrage consists of four gates which each weigh 50 tonnes. These plates are very durable, can regulate the flow of water, which reduces the discharge of the river downstream. This prevents tidal flooding, when at high tide, lots of water from the sea enters the river channel, causing the river to burst its banks.
GCSE Geography - River Tees case study. Subject: Geography. Age range: 14-16. Resource type: Lesson (complete) File previews. pptx, 3.79 MB. Complete lesson - Linked to OCR 2016 Geography syllabus. Based of case study sheet (included) and YouTube link. PowerPoint self explanatory.
Flood mitigation efforts (flood fighting), including raising awareness among local residents, have been crucial in Japan to reduce damage during flood events. In this study, interviews were conducted with three flood fighting management bodies on the Yodo and Yamato Rivers, which still have strong flood fighting corps, and field investigations were performed focusing on the actual operation of ...
UK Floods Case Study November 2019. The UK experienced an extreme weather event in November 2019 when exceptionally heavy rainfall caused flooding in parts of the UK. Heavy downpours across large parts of northern England led to surface water and river flooding in parts of Yorkshire, Nottinghamshire, Greater Manchester, Derbyshire and Lincolnshire.
The Great Floods of 2000; Kerala flood case study; Rocks, Resources and Scenery. Geological time is on a different time to human time; Rock Types and the Rock Cycle; ... Give the quiz another go, and don't forget to read up on this area by heading over to our River Tees case study resources using the case study link at the top of the page.
April 17, 20249:07 AM PDTUpdated 28 min ago. [1/5]People walk through flood water caused by heavy rains, in Dubai, United Arab Emirates, April 17, 2024. REUTERS/Amr Alfiky Purchase Licensing ...