Have a language expert improve your writing
Run a free plagiarism check in 10 minutes, generate accurate citations for free.
- Knowledge Base
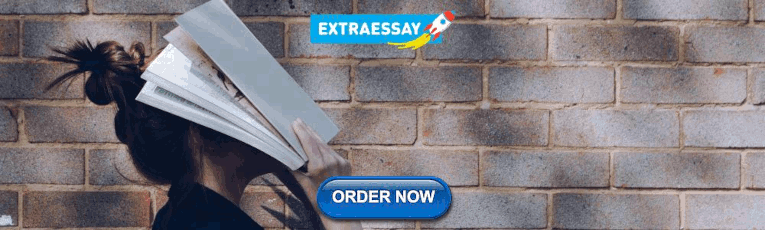
Hypothesis Testing | A Step-by-Step Guide with Easy Examples
Published on November 8, 2019 by Rebecca Bevans . Revised on June 22, 2023.
Hypothesis testing is a formal procedure for investigating our ideas about the world using statistics . It is most often used by scientists to test specific predictions, called hypotheses, that arise from theories.
There are 5 main steps in hypothesis testing:
- State your research hypothesis as a null hypothesis and alternate hypothesis (H o ) and (H a or H 1 ).
- Collect data in a way designed to test the hypothesis.
- Perform an appropriate statistical test .
- Decide whether to reject or fail to reject your null hypothesis.
- Present the findings in your results and discussion section.
Though the specific details might vary, the procedure you will use when testing a hypothesis will always follow some version of these steps.
Table of contents
Step 1: state your null and alternate hypothesis, step 2: collect data, step 3: perform a statistical test, step 4: decide whether to reject or fail to reject your null hypothesis, step 5: present your findings, other interesting articles, frequently asked questions about hypothesis testing.
After developing your initial research hypothesis (the prediction that you want to investigate), it is important to restate it as a null (H o ) and alternate (H a ) hypothesis so that you can test it mathematically.
The alternate hypothesis is usually your initial hypothesis that predicts a relationship between variables. The null hypothesis is a prediction of no relationship between the variables you are interested in.
- H 0 : Men are, on average, not taller than women. H a : Men are, on average, taller than women.
Receive feedback on language, structure, and formatting
Professional editors proofread and edit your paper by focusing on:
- Academic style
- Vague sentences
- Style consistency
See an example
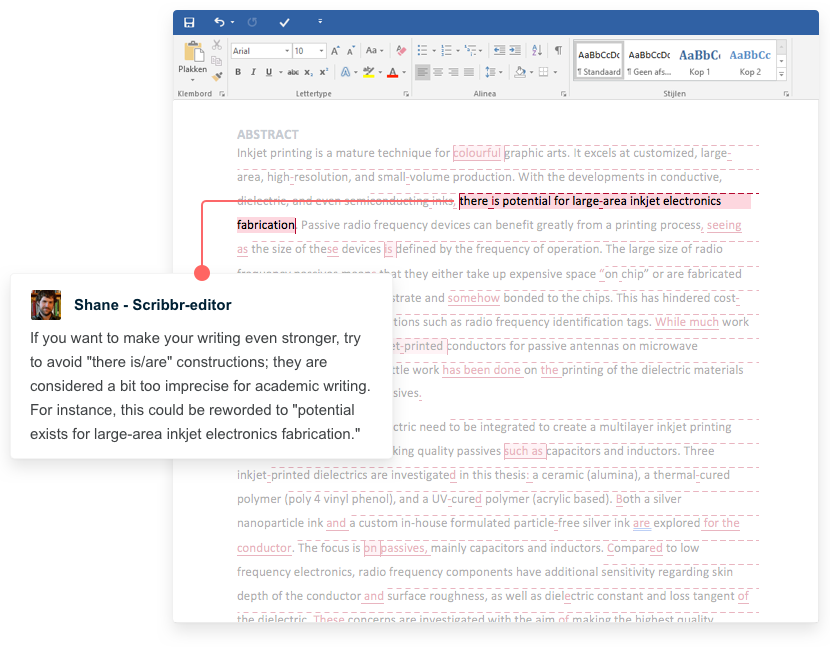
For a statistical test to be valid , it is important to perform sampling and collect data in a way that is designed to test your hypothesis. If your data are not representative, then you cannot make statistical inferences about the population you are interested in.
There are a variety of statistical tests available, but they are all based on the comparison of within-group variance (how spread out the data is within a category) versus between-group variance (how different the categories are from one another).
If the between-group variance is large enough that there is little or no overlap between groups, then your statistical test will reflect that by showing a low p -value . This means it is unlikely that the differences between these groups came about by chance.
Alternatively, if there is high within-group variance and low between-group variance, then your statistical test will reflect that with a high p -value. This means it is likely that any difference you measure between groups is due to chance.
Your choice of statistical test will be based on the type of variables and the level of measurement of your collected data .
- an estimate of the difference in average height between the two groups.
- a p -value showing how likely you are to see this difference if the null hypothesis of no difference is true.
Based on the outcome of your statistical test, you will have to decide whether to reject or fail to reject your null hypothesis.
In most cases you will use the p -value generated by your statistical test to guide your decision. And in most cases, your predetermined level of significance for rejecting the null hypothesis will be 0.05 – that is, when there is a less than 5% chance that you would see these results if the null hypothesis were true.
In some cases, researchers choose a more conservative level of significance, such as 0.01 (1%). This minimizes the risk of incorrectly rejecting the null hypothesis ( Type I error ).
The results of hypothesis testing will be presented in the results and discussion sections of your research paper , dissertation or thesis .
In the results section you should give a brief summary of the data and a summary of the results of your statistical test (for example, the estimated difference between group means and associated p -value). In the discussion , you can discuss whether your initial hypothesis was supported by your results or not.
In the formal language of hypothesis testing, we talk about rejecting or failing to reject the null hypothesis. You will probably be asked to do this in your statistics assignments.
However, when presenting research results in academic papers we rarely talk this way. Instead, we go back to our alternate hypothesis (in this case, the hypothesis that men are on average taller than women) and state whether the result of our test did or did not support the alternate hypothesis.
If your null hypothesis was rejected, this result is interpreted as “supported the alternate hypothesis.”
These are superficial differences; you can see that they mean the same thing.
You might notice that we don’t say that we reject or fail to reject the alternate hypothesis . This is because hypothesis testing is not designed to prove or disprove anything. It is only designed to test whether a pattern we measure could have arisen spuriously, or by chance.
If we reject the null hypothesis based on our research (i.e., we find that it is unlikely that the pattern arose by chance), then we can say our test lends support to our hypothesis . But if the pattern does not pass our decision rule, meaning that it could have arisen by chance, then we say the test is inconsistent with our hypothesis .
If you want to know more about statistics , methodology , or research bias , make sure to check out some of our other articles with explanations and examples.
- Normal distribution
- Descriptive statistics
- Measures of central tendency
- Correlation coefficient
Methodology
- Cluster sampling
- Stratified sampling
- Types of interviews
- Cohort study
- Thematic analysis
Research bias
- Implicit bias
- Cognitive bias
- Survivorship bias
- Availability heuristic
- Nonresponse bias
- Regression to the mean
Hypothesis testing is a formal procedure for investigating our ideas about the world using statistics. It is used by scientists to test specific predictions, called hypotheses , by calculating how likely it is that a pattern or relationship between variables could have arisen by chance.
A hypothesis states your predictions about what your research will find. It is a tentative answer to your research question that has not yet been tested. For some research projects, you might have to write several hypotheses that address different aspects of your research question.
A hypothesis is not just a guess — it should be based on existing theories and knowledge. It also has to be testable, which means you can support or refute it through scientific research methods (such as experiments, observations and statistical analysis of data).
Null and alternative hypotheses are used in statistical hypothesis testing . The null hypothesis of a test always predicts no effect or no relationship between variables, while the alternative hypothesis states your research prediction of an effect or relationship.
Cite this Scribbr article
If you want to cite this source, you can copy and paste the citation or click the “Cite this Scribbr article” button to automatically add the citation to our free Citation Generator.
Bevans, R. (2023, June 22). Hypothesis Testing | A Step-by-Step Guide with Easy Examples. Scribbr. Retrieved April 12, 2024, from https://www.scribbr.com/statistics/hypothesis-testing/
Is this article helpful?
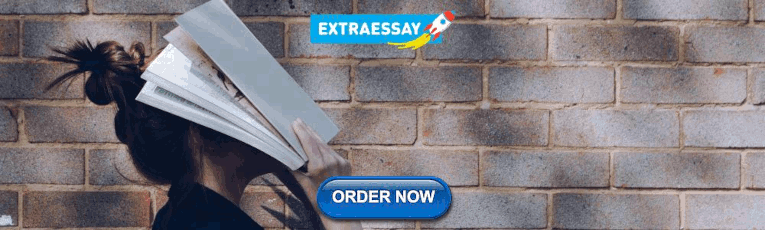
Rebecca Bevans
Other students also liked, choosing the right statistical test | types & examples, understanding p values | definition and examples, what is your plagiarism score.
Academia.edu no longer supports Internet Explorer.
To browse Academia.edu and the wider internet faster and more securely, please take a few seconds to upgrade your browser .
Enter the email address you signed up with and we'll email you a reset link.
- We're Hiring!
- Help Center
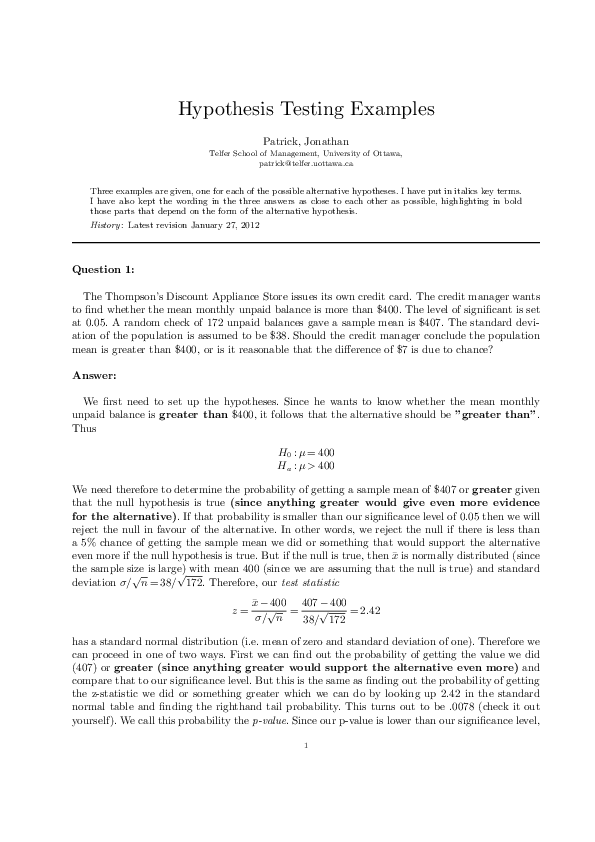
Hypothesis Testing Examples

Related Papers
Hedyan Poetra
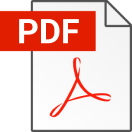
Siddharth Agrawal
8-1 We must deal with uncertainty in our decisions because we rarely know the values for population characteristics. In addition, there are other circumstances unknown to us in any given situation. Statistical analysis can reduce uncertainty, but not eliminate it entirely. 8-2 Theoretically, one could toss a coin a large number of times and see if the proportion of heads was very different from 5. Similarly by recording the outcomes of many dice rolls, one could see if the proportion of each side was very different from 1/6. You would need a large number of trials for each of these samples. 8-3 Yes, it is possible that a false hypothesis will be accepted. If the observed value does not differ enough from the hypothesized one, the hypothesis will be accepted. Acceptance of a hypothesis is based on probability and therefore we can never be absolutely certain that our decision is correct. 8-4 a) Assume hypothesis about population b) Collect sample data c) Calculate a sample statistic d) Use sample statistic (c) to evaluate hypothesis (a) e) Take decision. 8-5 There is always a statistical possibility that a sample does not accurately represent the population from which it has been drawn. 8-6 We mean that we would not have reasonably expected to find that particular sample if in fact the hypothesis had been true. 8-7 The level of probability, or certainty, depends upon how accurate our answer needs to be. 8-8 P(z 1.75) = 2(.5-.4599) =.0802 8-9 The z value which leaves (100-98)% = 2% in the tails is ± 2.33, so the interval should be the hypothesized value ± 2.33 standard errors.
jorge ponce
The National medical journal of India
Abhaya Indrayan
HEMANT SINGH
We present the various methods of hypothesis testing that one typically encounters in a mathematical statistics course. The focus will be on conditions for using each test, the hypothesis tested by each test, and the appropriate (and inappropriate) ways of using each test. We conclude by summarizing the different tests (what conditions must be met to use them, what the test statistic is, and what the critical region is).
BOHR Publishers
BOHR International Journal of Operations Management Research and Practices (BIJOMRP)
Many researchers and beginners in social research have several dilemmas and confusion in their mind about hypothesis statement and statistical testing of hypotheses. A distinction between the research hypothesis and statistical hypotheses, and understanding the limitations of the historically used null hypothesis statistical testing, is useful in clarifying these doubts. This article presents some data from the published research articles to support the view that the is format as well as the will format is appropriate to stating hypotheses. The article presents a social research framework to present the research hypothesis and statistical hypotheses is proper perspective.
Health Services Research
Harold Luft
Journal of Surgical Education
Todd Neideen
Florentin Smarandache
In this paper problem of testing of hypothesis is discussed when the samples have been drawn from normal distribution. The study of hypothesis testing is also extended to Baye’s set up.
ForsChem Research Reports
Hugo Hernandez
There are two very important steps in hypothesis testing that are commonly undervalued: Selecting the sample size and choosing the significance level. A minimum sample size can be obtained based on power analysis, but this method requires previously choosing the significance level. The significance level has been classically considered to be 5% (following the initial suggestion provided by Ronald Fisher) and while other typical values are sometimes employed (e.g. 10%, 1%, 0.1%) many practitioners of hypothesis testing do not have a clear, objective criterion for choosing this value. Considering that the significance level has a direct influence on the conclusion of the test, it should not be chosen using subjective methods. In this direction, a new approach for determining the optimal sample size and optimal significance level during hypothesis testing is presented. The focus in the first of a series of reports about this topic is discussing the tests of means: The Z-test and Student’s T-test. The optimization of the tests is done considering a desired Cohen resolution, which can be obtained from the particular problem conditions or by using default values based on the minimum viability of each test. The optimal sample size can be found by solving an economical optimization problem, involving the ratio between the cost of an erroneous decision and the cost of an individual observation. When this cost ratio is not available, a maximum tolerable test error can be used for optimizing the sample size. Once the sample size has been defined, the optimal significance level is found by minimizing the total test error, including both type I and type II errors. The hypothesis tests are then performed as usual. In order to simplify the interpretation of the tests, a decision value (D-value) is proposed. Positive D-values represent a positive decision (rejecting the null hypothesis), and negative D-values represent a negative decision (not rejecting the null hypothesis). D-values within a critical threshold around zero are considered inconclusive. Decisions based on D-values are shown to be less fluctuating than those based on P-values, and therefore, the former can lead to more reliable conclusions.
RELATED PAPERS
ISRN Pediatrics
Basu Banerjee
Salsa Febrina
Emerson Fernandes Marçal
Gholam Kazemi
Journal of Minerals and Materials Characterization and Engineering
Ayodele Daniyan
Phytochemical Analysis
Fernando Ferreira
Jurnal Keperawatan Jiwa
Nia Agustiningsih
Pediatric Review: International Journal of Pediatric Research
Chetan Chovatiya
Gabi Mueller
Miftahul Janah
Tạp chí Khí tượng Thủy văn
Martine Furno
The European respiratory journal
Michael Campos
Journal of fish diseases
sabyasachi pattanayak
Revista Brasileira de Educação em Geografia
Thiago Luiz Calandro
iConference 2023
Maayan Nakash
Harga Tas Kanvas
chafidl tuwapatlan
National journal of maxillofacial surgery
Vipin Dehane
Abolfazl Soheilyfar
Avicenna Journal of Medicine
mohsin salih
yousif madani
Medical Devices: Evidence and Research
Sergio Golombek
International Journal of Manpower
Ciaran McFadden
Eliana González
RELATED TOPICS
- We're Hiring!
- Help Center
- Find new research papers in:
- Health Sciences
- Earth Sciences
- Cognitive Science
- Mathematics
- Computer Science
- Academia ©2024
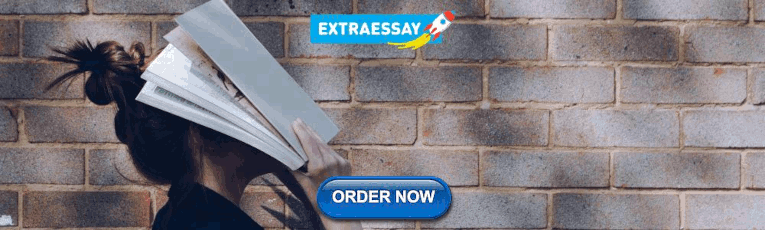
IMAGES
VIDEO
COMMENTS
23.1 How Hypothesis Tests Are Reported in the News 1. Determine the null hypothesis and the alternative hypothesis. 2. Collect and summarize the data into a test statistic. 3. Use the test statistic to determine the p-value. 4. The result is statistically significant if the p-value is less than or equal to the level of significance.
Case1: Population is normally or approximately normally distributed with known or unknown variance (sample size n may be small or large), Case 2: Population is not normal with known or unknown variance (n is large i.e. n≥30). 3.Hypothesis: we have three cases. Case I : H0: μ=μ0 HA: μ μ0. e.g. we want to test that the population mean is ...
8.2 FOUR STEPS TO HYPOTHESIS TESTING The goal of hypothesis testing is to determine the likelihood that a population parameter, such as the mean, is likely to be true. In this section, we describe the four steps of hypothesis testing that were briefly introduced in Section 8.1: Step 1: State the hypotheses. Step 2: Set the criteria for a decision.
z statistic. For the illustrative example, μ0 = 170. We know σ = 40. Take a random sample of n = 64. Therefore. SEx n 40 64 5. If we found a sample mean of 173, then. zstat . x 0 173 170 0.60 SE x 5.
Present the findings in your results and discussion section. Though the specific details might vary, the procedure you will use when testing a hypothesis will always follow some version of these steps. Table of contents. Step 1: State your null and alternate hypothesis. Step 2: Collect data. Step 3: Perform a statistical test.
Exam 1 (Previous Semester): Null Hypothesis (H0): treatment will have mean exam score (σ = 8) Alternative Hypothesis (H1): group mean exam score will from M = 85. STEP 2: SET CRITERIA FOR DECISION. Alpha Level/Level of Significance. probability value used to define the (unlikely) sample outcomes if the null hypothesis is true; e.g., α = .05 ...
The major purpose of hypothesis testing is to choose between two competing hypotheses about the value of a population parameter. For example, one hypothesis might claim that the wages of men and women are equal, while the alternative might claim that men make ... we work with the proportion p, the test statistic is N p q 0 p q 0 0 0 0 0 p .5/N ...
March 24, 2013. In this lecture note, we discuss the fundamentals of statistical hypothesis tests. Any statistical hypothesis test, no matter how complex it is, is based on the following logic of stochastic proof by contradiction. In mathematics, proof by contradiction is a proof technique where we begin by assuming the validity of a hypothesis ...
2 Statistical*Hypotheses Statistical*hypothesis:* a*claim about*the*value*of*a* parameter*or*population*characteristic.* Examples:* • H:#µ=*75*cents,*where*µ ...
and wants to con rm this by using a hypothesis test. 2 In each question, you are given null and alternative hypothesis (where p stands for the population proportion), the signi cance level and the observed data. Decide whether or not there is suf cient evidence to reject the null hypothesis. a i H 0 : p 0.3, H 1 : p 0.3, signi cance level 5% ...
Carry out an appropriate statistical test and interpret your findings. ANSWER . Yes, a paired t-test suggests that the average difference in hours slept (Dalmane - Halcion) = 0.32 is statistically significant (one sided p-value = .018). SOLUTION . This question is asking for a hypothesis test of the equality of two means in the setting of ...
The logic of hypothesis testing, as compared to jury trials page 3 This simple layout shows an excellent correspondence between hypothesis testing and jury decision-making. t test examples page 4 Here are some examples of the very widely used t test. The t test through Minitab page 8 This shows an example of a two-sample problem, as performed ...
Instead, hypothesis testing concerns on how to use a random sample to judge if it is evidence that supports or not the hypothesis. Hypothesis testing is formulated in terms of two hypotheses: H0: the null hypothesis; H1: the alternate hypothesis. The hypothesis we want to test is if H1 is \likely" true. So, there are two possible outcomes:
Hypothesis testing will rely extensively on the idea that, having a pdf, one can compute the probability of all the corresponding events. Make sure you understand this point before going ahead. We have seen that the pdf of a random variable synthesizes all the probabilities of realization of the underlying events.
In hypothesis testing, we quantify our uncertainty by asking whether it is likely that data came from a particular distribution. We will focus on the following common type of hypothesis testing scenario: IThe data Y come from some distribution f(Yj ), with parameter . IThere are two possibilities for : either = . 0, or 6= .
no reason to doubt that the null hypothesis is true. Similarly, if the observed data is "inconsistent" with the null hypothesis (in our example, this means that the sam-ple mean falls outside the interval (90.2, 109.8)), then either a rare event has occurred (rareness is judged by thresholds 0.05 or 0.01) and the null hypothesis is true,
Hypothesis testing (or test of significance) is a procedure, based on a sample evidence and probability, used to test claims regarding a characteristic of one or ... Example 6: The p-value for a hypothesis test is P-value = 0.0237. What is your decision if the level of significance is a) = 0.05 and b) = 0.01?
The intent of hypothesis testing is formally examine two opposing conjectures (hypotheses), H0 and HA. These two hypotheses are mutually exclusive and exhaustive so that one is true to the exclusion of the other. We accumulate evidence - collect and analyze sample information - for the purpose of determining which of the two hypotheses is true ...
Similarly in hypothesis testing, we do not accept the null hypothesis, but instead fail to reject the null hypothesis. Just because there is not enough evidence to reject the null hypothesis does not mean that the null hypothesis is true! Table 1 summarizes the possible outcomes from a hypothesis test. Table 1: Outcomes of a hypothesis test ...
10 HYPOTHESIS TESTING. Objectives. After studying this chapter you should • be able to define a null and alternative hypothesis; • be able to calculate probabilities using an appropriate model to test a null hypothesis; • be able to test for the mean based on a sample; • understand when to use a one or two tailed test. 10.0 Introduction.
For the above example, X N ; 1=25 : If the null hypothesis is true, then. X. N 98:6; 1=25 : Hypothesis testing for the population mean. In reality, we have one value, x, for the sample mean. We can use this value to quantify the evidence of departure from the null hypothesis. Suppose that from our sample of 25 people we sample mean is x = 98:4.
View PDF. Hypothesis Testing Examples Patrick, Jonathan Telfer School of Management, University of Ottawa, [email protected] Three examples are given, one for each of the possible alternative hypotheses. I have put in italics key terms. I have also kept the wording in the three answers as close to each other as possible, highlighting in ...